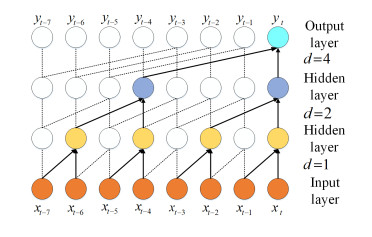
For sparse signal reconstruction (SSR) problem in compressive sensing (CS), by the splitting technique, we first transform it into a continuously differentiable convex optimization problem, and then a new self-adaptive gradient projection algorithm is proposed to solve the SSR problem, which has fast solving speed and pinpoint accuracy when the dimension increases. Global convergence of the proposed algorithm is established in detail. Without any assumptions, we establish global R−linear convergence rate of the proposed algorithm, which is a new result for constrained convex (rather than strictly convex) quadratic programming problem. Furthermore, we can also obtain an approximate optimal solution in a finite number of iterations. Some numerical experiments are made on the sparse signal recovery and image restoration to exhibit the efficiency of the proposed algorithm. Compared with the state-of-the-art algorithms in SSR problem, the proposed algorithm is more accurate and efficient.
Citation: Hengdi Wang, Jiakang Du, Honglei Su, Hongchun Sun. A linearly convergent self-adaptive gradient projection algorithm for sparse signal reconstruction in compressive sensing[J]. AIMS Mathematics, 2023, 8(6): 14726-14746. doi: 10.3934/math.2023753
[1] | Lihe Liang, Jinying Cui, Juanjuan Zhao, Yan Qiang, Qianqian Yang . Ultra-short-term forecasting model of power load based on fusion of power spectral density and Morlet wavelet. Mathematical Biosciences and Engineering, 2024, 21(2): 3391-3421. doi: 10.3934/mbe.2024150 |
[2] | Xiaoqiang Dai, Kuicheng Sheng, Fangzhou Shu . Ship power load forecasting based on PSO-SVM. Mathematical Biosciences and Engineering, 2022, 19(5): 4547-4567. doi: 10.3934/mbe.2022210 |
[3] | Faisal Mehmood Butt, Lal Hussain, Anzar Mahmood, Kashif Javed Lone . Artificial Intelligence based accurately load forecasting system to forecast short and medium-term load demands. Mathematical Biosciences and Engineering, 2021, 18(1): 400-425. doi: 10.3934/mbe.2021022 |
[4] | Mingju Chen, Fuhong Qiu, Xingzhong Xiong, Zhengwei Chang, Yang Wei, Jie Wu . BILSTM-SimAM: An improved algorithm for short-term electric load forecasting based on multi-feature. Mathematical Biosciences and Engineering, 2024, 21(2): 2323-2343. doi: 10.3934/mbe.2024102 |
[5] | Yongquan Zhou, Yanbiao Niu, Qifang Luo, Ming Jiang . Teaching learning-based whale optimization algorithm for multi-layer perceptron neural network training. Mathematical Biosciences and Engineering, 2020, 17(5): 5987-6025. doi: 10.3934/mbe.2020319 |
[6] | Yanmei Jiang, Mingsheng Liu, Jianhua Li, Jingyi Zhang . Reinforced MCTS for non-intrusive online load identification based on cognitive green computing in smart grid. Mathematical Biosciences and Engineering, 2022, 19(11): 11595-11627. doi: 10.3934/mbe.2022540 |
[7] | Fengyong Li, Meng Sun . EMLP: short-term gas load forecasting based on ensemble multilayer perceptron with adaptive weight correction. Mathematical Biosciences and Engineering, 2021, 18(2): 1590-1608. doi: 10.3934/mbe.2021082 |
[8] | Chun Li, Ying Chen, Zhijin Zhao . Frequency hopping signal detection based on optimized generalized S transform and ResNet. Mathematical Biosciences and Engineering, 2023, 20(7): 12843-12863. doi: 10.3934/mbe.2023573 |
[9] | Hao Yuan, Qiang Chen, Hongbing Li, Die Zeng, Tianwen Wu, Yuning Wang, Wei Zhang . Improved beluga whale optimization algorithm based cluster routing in wireless sensor networks. Mathematical Biosciences and Engineering, 2024, 21(3): 4587-4625. doi: 10.3934/mbe.2024202 |
[10] | Chongyi Tian, Longlong Lin, Yi Yan, Ruiqi Wang, Fan Wang, Qingqing Chi . Photovoltaic power prediction based on dilated causal convolutional network and stacked LSTM. Mathematical Biosciences and Engineering, 2024, 21(1): 1167-1185. doi: 10.3934/mbe.2024049 |
For sparse signal reconstruction (SSR) problem in compressive sensing (CS), by the splitting technique, we first transform it into a continuously differentiable convex optimization problem, and then a new self-adaptive gradient projection algorithm is proposed to solve the SSR problem, which has fast solving speed and pinpoint accuracy when the dimension increases. Global convergence of the proposed algorithm is established in detail. Without any assumptions, we establish global R−linear convergence rate of the proposed algorithm, which is a new result for constrained convex (rather than strictly convex) quadratic programming problem. Furthermore, we can also obtain an approximate optimal solution in a finite number of iterations. Some numerical experiments are made on the sparse signal recovery and image restoration to exhibit the efficiency of the proposed algorithm. Compared with the state-of-the-art algorithms in SSR problem, the proposed algorithm is more accurate and efficient.
Power load forecasting can be divided into long-term forecasting, medium-term forecasting, short-term forecasting and ultra-short-term forecasting according to the forecasting time-scale. The forecasting period of short-term power load is typical, as it is a critical basis for maintaining the stable operation of the power system and improving economic benefits. The accuracy of the short-term power forecast can play an important role in addressing the issue of the power decision department controlling power dispatch in the next step. Accurate short-term load forecasting can effectively reduce resource waste and improve economic benefits [1,2,3].
At present, load prediction methods primarily include a statistical prediction method composed of multiple linear regression [4], a Kalman filter [5,6] an autoregressive moving average and a machine learning method composed of a support vector machine [7,8,9], an expert system and artificial neural networks [10]. Research has consistently shown that the calculation model of the statistical method is too ordinary, as it can only deal with linear data but cannot grasp the inherent characteristics of nonlinear data reasonably. Although the machine learning method can deal with nonlinear data well, it cannot extract time-series data features effectively. With the development of deep learning, it becomes the focus of load forecasting. A large number of deep neural networks are widely employed in load prediction, such as convolutional neural networks (CNNs), recurrent neural networks (RNNs) [11] and short and long duration memory networks (LSTM) [12]. CNNs can effectively extract multidimensional data features, but it cannot deal with time-series features efficiently. RNNs can model long time-series data through a cyclic structure, but with the increase of load data, there are problems such as gradient disappearance or gradient explosion. As a special RNN, the LSTM network can better solve the deficiency of the RNN through the use of a gate structure. Nonetheless, with the increase in training data, it is difficult to select parameters for the LSTM network [13]. In order to effectively process multidimensional power load data, the CNN-LSTM hybrid neural network prediction method was proposed in the literature [14]. Feature extraction was carried out through a two-dimensional convolutional layer to reduce the training difficulty of the LSTM network model. Surveys such as that conducted by the authors of [15] showed that using the CNN to extract data features, using the gated recurrent neural (GRU) network to avoid the problem of multiple training parameters in the LSTM network and introducing an attention mechanism, can effectively improve the accuracy of power load forecasting. Reference [16] found that their CNN-BiGRU network improves data utilization in order to make data flow bidirectional in the network layer. According to the research, since CNN networks cannot predict time series data well, time series convolutional networks (TCNs) can be employed for sequence data prediction. And TCN can extract time series data features better than CNN and RNN [17]. Tian et al. [18] proposed a short-term wind speed prediction model employing empirical modal decomposition and an improved sparrow algorithm to optimize the LSTM neural network. The model decomposes the ultra-short-term wind speed by utilizing empirical modal decomposition, predicts it by employing the LSTM network and optimizes the LSTM network hyperparameters by improving the sparrow optimization algorithm. In [19] for short-term wind speed prediction, a prediction model based on local mean decomposition (LMD) with a combined kernel function least squares support vector machine (LSSVM) is proposed. Wind speed data are decomposed by the LMD algorithm and predicted by the LSSVM, and the firefly algorithm is employed to optimize the parameter selection. The authors of [20] proposed a time-series convolutional network with the multi-attentional mechanism. By introducing an initial structure into the TCN network, multidimensional information was extracted from convolutional kernels of disagreement scales, improving the accuracy of ultra-short-term load prediction effectively. The authors of [21] proposed a combined prediction model based on empirical modal decomposition to forecast traffic flow state information. The empirical modal decomposition is decomposed into components, the optimal prediction method is selected based on the results of adaptive analysis and the combined model weights are optimized by employing the fruit fly algorithm. The authors of [22] proposed a combined prediction model based on ensemble empirical modal decomposition and a regularized limit learning machine for wind speed prediction. The wind speed series of the ensemble empirical modal decomposition is predicted by employing the regularized limit learning machine, and the reliability of the prediction model is improved by cross-validation. Recent evidence suggests that complete ensemble empirical mode decomposition with adaptive noise (CEEMDAN) and variational model decomposition (VMD) can be employed to decompose power load data. Second, non-stationary components and stationary components are predicted by deep bidirectional long short-term memory (DBILSTM) and mixed logistic regression (MLR) networks respectively. Finally, the prediction accuracy is improved by combining the prediction structure with data reconstruction [23].
This paper presents a multi-model fusion method for short-term power load forecasting based on VMD, improved whale optimization (WOA), Wavelet temporal convolutional network (WTCN)-BiGRU and CatBoost methods. First, VMD was employed to decompose power load data into contrast intrinsic mode functions(IMFs), and weather characteristic factors were added for each intrinsic mode. Then, the TCN was utilized to extract multidimensional data features, and the extracted features were sent to the BiGRU network for model training. The influence degree of necessary information can be effectively retained by adding an attention mechanism. The model adopts the improved WOA (IWOA) algorithm to optimize the hyperparameter selection of the TCN-BiGRU-attention network, which designs the parameter selection of the network layer of the model more effectively and predicts the stationary component of the sequence in parallel with the CatBoost network. The parallel prediction results of two-layer networks were combined with the mean absolute percentage error-reciprocal weight (MAPE-RW) algorithm. The model accuracy was verified by utilizing commenced loading data from an Australian region, and the model performance was evaluated using the root mean square error (RMSE) and mean absolute percentage error (MAPE). The contributions of this paper can be summarized as follows:
1) A multi-model fusion load forecasting method is proposed. First, the IWOA-WTCN-BiGRU-attention prediction model of VMD was constructed as Model one. Second, the CatBoost prediction model based on a random search algorithm was adopted as Model two. Finally, the MAPE-RW algorithm has been utilized to fuse the load prediction results of the two models to achieve an accurate prediction of the power load value.
2) The Morlet wavelet function is used to improve the TCN. The Morlet wavelet basis function is introduced as residual block activation function of the TCN.
3) An improved WOA is proposed. The traditional WOA is improved by introducing a nonlinear convergence factor and adaptive weight, which improves the convergence speed and the convergence accuracy of the algorithm.
The paper has been organized in the following way. In Section 2, the network principles employed in the multi-model structure are introduced. In Section 3, the load prediction model structure of the multi-model fusion network is proposed. In Section 4, the experimental validation of the multi-model fusion network is performed and the experimental results are analyzed. Finally, the whole paper is summarized and future work to be carried out is presented.
As mentioned in the literature [24], in 2014, Konstantin et al. proposed a VMD method for modal decomposition, which is an adaptive and completely non-recursive modal decomposition processing method. VMD has a more solid theoretical basis and can better suppress mode aliasing by controlling bandwidth. The decomposition method is suitable for non-stationary sequence data and can decompose the data set into multiple stationary sub-sequences with different frequency scales. The VMD solution procedure is as follows.
Structural constraint variational optimal problem is
{min{uk},{wk}{k∑k=1‖∂t[(d(t)+jpt)guk(t)]e−jwkt‖22}s.t.K∑k=1uk(t)=S | (2.1) |
where {uk},{ωk} denote the corresponding modal set and center frequency after VMD decomposition respectively, and K is the number of IMFs.
The penalty factor α and Lagrange operator λ are introduced into the constrained variational problem to transform it into the following unconstrained variational problem:
L(uk,ωk,λ)=αK∑k=1‖∂t[(δ(t)+jπt)⋅uk(t)]e−jωkt‖22+‖f(t)−K∑k=1uk(t)‖22+⟨λ(t),f(t)−K∑k=1uk(t)⟩ | (2.2) |
The above unconstrained variational problems are solved by the alternating direction multiplier method, and the solving process is as follows:
K∑k=1‖ˆun+1k−ˆunk‖22/K∑k=1‖ˆun+1k−ˆunk‖22‖ˆunk‖22‖ˆunk‖22<γ | (2.3) |
where γ is the allowable error, n is the number of iterations and the Fourier transform of ˆun+1k,f(t),λ(t) is ˆun+1k(ω),ˆf(ω),ˆλn(ω).
The TCN was first proposed by Bai et al. [25]. in 2018 and is mainly employed for timing prediction, probability prediction, time prediction and traffic prediction. The TCN evolved from CNN results and can extract load data features effectively. In this paper, a multi-model fusion network is introduced, and a TCN is employed to extract data feature information from time series to remove invalid features to improve the accuracy of power load prediction. The TCN is composed of causal convolution, expansion convolution and a residual block [26].
Causal convolution adopts the one-dimensional full convolutional network framework. The zero-fill module is introduced into the network so that the input layer, hidden layer and output layer can keep the same length, to avoid the loss of effective information. The input yt is related only to the input (xt−1,xt−2,⋯xt−n) before the current input xt and t. The convolutional calculation is shown in Figure 1.
Expansion convolution can increase the receptive field size of the output unit without increasing the number of parameters. The convolutional calculation method is as follows:
F(s)=(x⊗fd)(s)=k−1∑i=0f(i)⋅xt−d⋅i | (2.4) |
where fd is the expansion rate, d corresponds to the filter and xt−d⋅i is the input at the current time and the historical time.
The core idea of residual block is to introduce one or more layers of "hop connection" operation, and the network structure is shown in Figure 2. The left channel introduces weighted normalized accelerated gradient descent and a nonlinear activation function. The right channel is the convolution directly connected to the edge, which ensures that the input and output data dimensions are consistent. The residual block output is
h(x)=Activation(x+F(x)) | (2.5) |
where x, h(x) is the input and output of the residual block. The network output h(x) is the result of linear transformation and activation function mapping.The WTCN is based on TCN topology, and the Morlet wavelet basis function is introduced into the residual block as its activation function. The Morlet wavelet basis function is expressed as
y=cos(1.75x)e−x2/−x222 | (2.6) |
The GRU network is simpler than the LSTM network with two gating units. The network inherits the advantages of the LSTM network and improves the training speed on the premise of ensuring training accuracy [27]. The GRU network structure is shown in Figure 3. By changing the GRU network into a bidirectional GRU network, information can be transmitted bidirectionally in the network layer, and the prediction accuracy of the network model is effectively improved [28]. The network structure is shown in Figure 4.
The BiGRU network calculation formula is
{zt=σ(Wz⋅[ht−1,xt])rt=σ(Wr⋅[ht−1,xt])˜ht=tanh(W˜h⋅[rt∗ht−1,xt])ht=(1−zt)∗ht−1+zt∗˜ht | (2.7) |
{ht=GRU(xt,ht−1)hi=GRU(xt,ht−1)ht=wtht+vthi+bt | (2.8) |
where zt is the update gate and rt is the reset gate, both of which are jointly determined by the input xt, hidden layer output xt at the previous moment and activation function σ. ht is the hidden layer output. Wz,Wr and W˜h are all trainable parameter matrices. ht is the state of the forward hidden layer, hi is the state of the backward hidden layer and bt is the bias optimization parameter of the hidden layer at the current time.
The attention mechanism is an intuitive interpretation method that imitates human visual mechanisms. It is often exploited in deep learning tasks such as natural language processing, image analysis and load prediction. The human visual mechanism will pay attention to the critical information of the object deliberately and ignore the irrelevant information. Consequently, it has been found that the relevant time-series information can be effectively preserved by adding an attention mechanism and weight allocation principle in the network model [29]. The structure of attention is shown in Figure 5.
The WOA is a bionic meta-heuristic optimization algorithm proposed by Australian scholars Mirjalili and Lewis in recent years based on the predation behavior of model humpback whales. The algorithm highlights the local search behavior of the network model by imitating the whale hunting behavior and realizes the global search of the network through the random search strategy. The WOA has the advantages of faster speed and higher precision in solving model parameter optimization, so it has wide application prospects. Nevertheless, the increase of power load data and influencing factors may cause the traditional WOA to have some limitations in the coordination of global search and local mining. Among them, the convergence factor a of the WOA cannot reflect the optimization process well with the linear decrease. Therefore, the nonlinear convergence factor a is proposed:
a=2−2sin(utmax_iterπ+φ) | (2.9) |
where u and φ are the set parameters and u=2 and φ=0 represent. max_iter is the maximum number of iterations. When the value is large at the initial stage of training, the searching range of optimal parameters can be effectively increased by the slowly decreasing convergence factor. With the increase in the number of iterations, the reduction speed of the convergence factor gradually increases and the convergence speed accelerates.
The introduction of the nonlinear factor a can improve the performance of the algorithm. However, in the traditional WOA, the whale motion position vector is not effectively utilized, so the population flexibility will be reduced and the optimization result will be affected. In this paper, the adaptive weight ω is introduced to enhance the global search capability of the algorithm and increase the total group diversity of the WOA. The formula for calculating ω is as follows:
ω=0.2cos(π2(1−tmax_iter)) | (2.10) |
In this paper, the performance of the IWOA is verified by introducing the benchmark test function f(x)=∑ni=1[x2i−10cos(2πxi)+10]. The number of iterations of the algorithm was set to 500 and the dimension of the base test function is 30. To ensure the reliability of the optimization results, the average of 10 experimental results is employed to indicate its average level. The WOA, improved nonlinear convergence factor (NWOA) and IWOA with adaptive weights and a nonlinear convergence factor are compared for algorithm performance. The experimental results are shown in Figure 6. It is known that the WOA with improved adaptive weights and a nonlinear convergence factor (IWOA) not only improves the convergence speed of the algorithm, but also improves the convergence accuracy. The flow chart of the improved WOA is shown in Figure 7.
CatBoost is a machine learning library that the Russian search giant Yandex opened source in 2017, and it is an improvement on the gradient boosting decision tree (GBDT) algorithm [30]. The CatBoost algorithm has fewer parameters than the GBDT algorithm. The algorithm effectively solves the problems of gradient deviation and prediction deviation, reduces the risk of model overfitting and improves the generalization ability of the algorithm. CatBoost algorithms are often used in data mining and load forecasting.
Noise and low-frequency data interference can be effectively reduced by adding prior distribution terms to the gradient decision tree. Its algorithm is as follows:
ˆxik=p−1∑j[xσj,k=xσi,k]⋅Yσj+a⋅pp−1∑j[xσj,k=xσi,k]+a | (2.11) |
where σ represents the weight coefficient of the prior term, and p represents the prior term. The CatBoost usually captures the mean of the data set as the first item when solving regression problems.
The MAPE-RW algorithm can fuse disagreement models according to the degree of prediction error and output the optimal prediction results. The proportion of the predicted value of each model was determined by finding the optimal weight. The final predicted value calculated by the algorithm is as follows:
{ωi=MjMi+Mjffinal=ωVTWBAfCatboost+ωCatboostfVTWBA | (2.12) |
where ωi is the corresponding model weight, and ffinal is the final prediction output of multi-model fusion. fCatboost and fVTWBA are the predicted outputs of the CatBoost model and VMD-decomposed WTCN-IWOA-BiGRU-attention model, respectively.
There is a lot of power load influencing factors in time-series data, so the traditional prediction model cannot extract the data feature law effectively. In this paper, a multi-model fusion short-term power load forecasting model is proposed by combining a deep learning algorithm and a machine learning algorithm. Combined with the advantages of different algorithms, the characteristic information between data can be effectively mined to improve the accuracy of load prediction. The prediction model design of the multi-model fusion network is shown in Figure 8.
1) Data processing. The model validation analysis is carried out by employing a public power load data set from 2006 to 2010 in an Australian region. This data set contains six-dimensional feature vectors, and the feature parameters are shown in Table 1 below. The data set captures 30 min as the sampling point, and load prediction is carried out by using a sliding window, with a sliding window size of 10 and sliding step size of 1. Therefore, 10 sets of historical data are employed to predict the electric load value at the next moment. The data set is divided into the training set, verification set and test set according to 3:1:1. The multi-fusion network model employs the verification set to conduct parameter tuning in the training process. In order to make the network model evaluation more accurate, the test set will not participate in the network model training.
Characteristic parameters | Parameter types | Character descriptions |
Data | Time | Samples were taken every 30 minutes |
Weather factors | Dew-point humidity | Equilibrium temperature |
Dry-bulb humidity | Aerothermodynamic temperature | |
Wet-bulb temperature | Thermodynamic saturation temperature | |
Humidity | Degree of atmospheric dryness | |
Economic factors | Degree | Price per kWh |
2) Input layer. Characteristic data and power load data are exploited as input for the prediction model. The input data with length n is filled with missing values and normalized into the prediction model.
3) VMD layer. The power load data are employed as the input of the prediction model. The long time-series data were input into the prediction Model one after missing-value filling and normalization. In the VMD, the values of k and alpha are determined by the central frequencies in the decomposition. The value of the central frequency is calculated by changing the values of k and alpha. By choosing a reasonable value of k, the phenomenon of model mixing can be avoided, and fewer network parameters for the WOA-based model one can also be generated. As can be seen from Table 2, at k = 5 and alpha = 1850, the central frequency has been relatively stable with the least number of decomposition layers, which makes further training produce fewer parameters and improves the model training speed. The penalty factor of decomposition of the variational model alpha=1850, the tolerance difference of collection tol=1e−7 and the number of decomposition modes k=5 are set. The decomposition of each mode is shown in Figure 9.
k | Simulated signal center frequency | |||||
2 | 0.0001 | 0.02243 | ||||
3 | 0.0001 | 0.02080 | 0.04274 | |||
4 | 0.0001 | 0.02076 | 0.04167 | 0.06471 | ||
5 | 0.0001 | 0.02076 | 0.04162 | 0.06469 | 0.34702 | |
6 | 0.0001 | 0.02076 | 0.04162 | 0.06238 | 0.08467 | 0.35872 |
4) IWOA-based hyperparameter optimization. The optimal hyperparameters are obtained by employing WOA, and in order to perform the optimization search within the range of valid parameter selections, the range of network parameter values selected has been defined as shown in Table 3. The components and weather characteristics generated from the decomposition of load information by VMD are the input of the WTCN-BiGRU-attention network, respectively. And, the IWOA is employed to optimize the network hyperparameters. The optimal network hyperparameter search structure of each component is shown in Table 4.
Parameters | Parameter values | |
Parameter settings | Population size | 5 |
Max iterations | 5 | |
Constant b | 2 | |
Search for upper and lower bounds | Learn rate | [0.001, 0.01] |
Epoch | [10,100] | |
Batchsize | [16,128] | |
BiGRU node number | [1,20] | |
Number of nodes at the full | [1,100] |
Modal tags | IMF1 | IMF2 | IMF3 | IMF4 | IMF5 |
Learn rate | 0.0047 | 0.0027 | 0.0064 | 0.0054 | 0.0024 |
Epoch | 38 | 26 | 61 | 70 | 89 |
Batchsize | 72 | 19 | 72 | 37 | 22 |
BiGRU node number | 8 | 18 | 8 | 17 | 2 |
BiGRU node number | 16 | 11 | 7 | 17 | 19 |
Number of nodes at the full | 49 | 4 | 46 | 14 | 97 |
5) WTCN layer. The influential factors of the load characteristics were added to the modes decomposed by the VMD layer, respectively. The Morlet wavelet function is used as a residual block activation function. The network extracts load characteristics and influencing factors through the WTCN layer. It normalizes the weight of the convolutional kernel. The dropout coefficient can be set to 0.2 to prevent over-fitting of the model. We set the expansion coefficient as (1, 2, 4, 8, 16, 32). We set the number of filters to 128.
6) BiGRU layer. The model builds two BiGRU layers to learn the features extracted from the WTCN, design full utilization of the data features and capture its internal change rules.
7) Attention layer. The input of the attention mechanism is the output data activated by the two-layer BiGRU network. The corresponding proportions of disagreement feature vectors are calculated according to the weight allocation principle, and the optimal weight parameter matrix is searched by using continuous updates and iteration.
8) CatBoost prediction model. A random search algorithm is employed to select the CatBoost network hyperparameters. The optimal network hyperparameters are shown in Table 5. The input power load and weather characteristic factors are modeled.
Verbose | Learning rate | Iterations | Depth |
50 | 0.03 | 900 | 12 |
9) Output layer. The IWOA-WTCN-BiGRU-attention network is set as Model one, and the CatBoost network is set as Model two. The MAPE-RW algorithm was exploited to calculate the weight of the output results of Model one and Model two. Finally, the load prediction output of the multi-model fusion network is obtained by effective fusion of the model prediction results.
Adam's optimization algorithm was selected as the parameter optimization method of network Model one. Adam is a first-order optimization algorithm that can effectively replace the traditional gradient descent process. The algorithm can update and iterate the weight of the network according to the data so that the loss function can be optimized. The loss function of the model is calculated by employing the mean square error, and its formula is
MSE=1NN∑i=1(yi−ˆyi)2 | (3.1) |
where N is the number of samples; yi and ˆyi are the actual load value and predicted load value of model i, respectively.
The minimum-maximum normalization method is exploited to normalize the original data and increase the training speed of the model. The inverse normalization of the predicted data designs the comparison between the predicted value and the real value more intuitive. Its calculation formula is
xn=x−xminxmax−xmin | (4.1) |
where x is the original load data. xmax and xmin are, respectively, the maximum value and minimum value of the sample data. xn is the normalized data.
The RMSE, MAPE, mean absolute error (MAE) and R-square were utilized as evaluation indexes. The calculation formulas are as follows:
{RMSE=√1NN∑i=1(˜xi−xi)2MAPE=100NN∑i=1|˜xi−xiP0|MAE=1nn∑i=1|˜xi−xi|R−square=1−n∑i=1(˜xi−xi)2n∑i=1(˜xi−ˉxi)2 | (4.2) |
where N is the number of samples. ˜xi is the true value of the sample point i. xi is the predicted value of the ith sample point.
The prediction results of the proposed model were compared with those of the traditional single model or mixed deep learning models such as GRU, LSTM, TCN, WTCN, WTCN-GRU, WTCN-LSTM, TCN-BiGRU, WTCN-BiGRU and TCN-BiGRU-attention models. The results of the load forecast data for December 20, 2010, are plotted to show more visually the accuracy advantages of the power load forecast model proposed in this paper. The load forecasting curves are shown in Figure 10. Different curves represent the prediction results and trends of disagreement prediction models. As can be seen from the prediction trends of the disagreement models shown in Figure 10, the prediction results of the prediction model proposed in this paper are more accurate, stable and closer to the real load. Table 6 shows the test lumped prediction and evaluation indexes of each model.
Prediction model | RMSE | MAPE | MAE | R-square |
IWOA-WTCN-BiGRU-attention | 110.964 | 0.914 | 82.189 | 0.993 |
CatBoost | 89.089 | 0.727 | 63.407 | 0.996 |
Fusion model of this paper | 77.495 | 0.632 | 56.103 | 0.997 |
WTCN-BiGRU-attention | 103.452 | 0.86 | 76.179 | 0.994 |
WTCN-BiGRU | 97.573 | 0.803 | 69.903 | 0.995 |
WTCN-GRU | 96.584 | 0.814 | 70.868 | 0.995 |
WTCN-LSTM | 100.309 | 0.843 | 73.649 | 0.995 |
WTCN | 103.558 | 0.869 | 75.975 | 0.994 |
TCN | 103.969 | 0.89 | 78.024 | 0.994 |
LSTM | 147.665 | 1.261 | 108.342 | 0.988 |
GRU | 109.991 | 0.932 | 82.114 | 0.994 |
CNN | 337.913 | 3.241 | 275.52 | 0.939 |
Therefore, the forecasting effects of different models were evaluated by analyzing the effects of the forecasting data of different models every month. Table 7 shows the error values of the monthly forecasting results of the different models. The analysis of the model evaluation metrics shows that the smaller the error values of MAPE, MAE and RMSE, the better the forecasting performance of the models. The larger the R-square value, the closer the predicted value is to the real value. After analyzing the data in Table 6 and Figure 10, the following conclusions can be drawn:
RMSE | Model one | Model two | Fusion model | WT-BG-a | WT-BG | WT-G | WT-L | WT |
Jan. | 108.372 | 106.039 | 97.048 | 126.77 | 116.382 | 96.321 | 107.057 | 121.456 |
Feb. | 102.257 | 84.791 | 76.02 | 108.625 | 89.625 | 90.072 | 88.652 | 103.947 |
Mar. | 85.485 | 72.585 | 61.48 | 84.297 | 76.175 | 80.666 | 85.047 | 86.109 |
Apr. | 96.362 | 106.033 | 83.271 | 105.568 | 110.002 | 108.207 | 109.236 | 113.008 |
May | 121.924 | 95.097 | 80 | 101.731 | 101.456 | 101.066 | 100.091 | 108.432 |
Jun. | 141.496 | 86.97 | 82.599 | 100.258 | 91.622 | 93.661 | 98.118 | 104.279 |
Jul. | 131.409 | 86.787 | 77.983 | 101.809 | 87.982 | 92.821 | 95.962 | 100.363 |
Aug. | 170.315 | 84.826 | 102.867 | 105.915 | 103.802 | 105.615 | 112.917 | 107.68 |
Sept. | 127.524 | 83.933 | 81.581 | 104.565 | 99.724 | 99.005 | 101.172 | 100.071 |
Oct. | 85.224 | 89.977 | 65.933 | 95.965 | 93.855 | 99.785 | 101.756 | 97.116 |
Nov. | 79.742 | 84.105 | 62.097 | 99.21 | 95.347 | 101.174 | 106.564 | 100.065 |
Dec. | 79.927 | 82.657 | 63.727 | 103.142 | 101.035 | 92.024 | 101.432 | 101.644 |
1) Compared with VMD-IWOA-WTCN-BiGRU-attention and CatBoost alone, the prediction results are more accurate by combining the proposed multiple models. The RMSE decreased by 33.469 and 11.594, MAPE decreased by 0.282 and 0.095% and MAE decreased by 26.086 and 7.304, respectively. By analyzing the reasons, it can be seen that VMD-IWOA-WTCN-BiGRU-attention has a large prediction deviation in load fluctuation hours because VMD caused a loss of part of the data. The CatBoost model is more accurate in the prediction of stationary components, but the prediction deviation is larger when the data fluctuation is larger. Therefore, the MAPE-RW algorithm is used to integrate the advantages of the two models to create a prediction effect that is more accurate.
2) Compared with other independent prediction models, the prediction results of the model proposed in this paper are closer to the real value. Compared with the WTCN-BiGRU prediction model, the RMSE decreased by 20.078, MAPE decreased by 0.171% and MAE decreased by 13.4. It can be seen that the algorithm based on the bottom combination model also achieves good training results, but it has the disadvantage of low prediction accuracy.
To verify the feasibility and accuracy of the model in different forecasting areas. We employed the 2018 annual measured power generation of the domestic Ningxia Wuzhong Sun Mountain photovoltaic (PV) power plant for PV power generation prediction, as well as five environmental data types, i.e., total solar irradiation, PV panel module temperature, ambient temperature, atmospheric pressure and relative humidity, measured by the environmental detector corresponding to this PV array. The data sets were collected at 15-minute intervals. Since the PV array only emits energy during the daytime, the valid data of the daily 7:30–16:30 PV-emitted power were selected as the model validation data. The data are divided according to the ratio of 10:1:1, and the first 10 months are taken as the training data, November has been applied as the validation data during training and December data as the test set. The prediction model parameters are set in the same way as the power load prediction model parameters. The WTCN-BiGRU-attention network hyperparameters were selected by the WOA algorithm for the PV power generation prediction model, as shown in Table 8, and the best network hyperparameters were selected by the random search algorithm for the CatBoost network, as shown in Table 9.
Modal tags | IMF1 | IMF2 | IMF3 | IMF4 | IMF5 |
Learn rate | 0.0038 | 0.0012 | 0.0059 | 0.0067 | 0.0030 |
Epoch | 72 | 54 | 50 | 73 | 96 |
Batchsize | 32 | 57 | 118 | 117 | 70 |
BiGRU node number | 5 | 1 | 16 | 10 | 3 |
BiGRU node number | 6 | 7 | 15 | 13 | 12 |
Number of nodes at the full | 44 | 12 | 54 | 84 | 12 |
Verbose | Learning rate | Iterations | Depth |
50 | 0.05 | 500 | 9 |
The prediction results of the model are compared with the hybrid neural network models WTCN-BiGRU-attention, TCN-BiGRU-attention and CNN-BiGRU-attention. The daily power generation forecasting results of PV panels for two days are plotted to show more intuitively the advantages of the multi-model fusion forecasting network proposed in this paper. The accuracy of the load curve prediction results is shown in Figures 11 and 12, where different curves represent the prediction results and trends of different models. From the figures, it can be seen that the proposed multi-modal fusion forecasting network has higher accuracy. Table 10 shows the total prediction evaluation index of each model test set.
Prediction model | RMSE | MAPE | MAE | R-square |
Model one | 1.996 | 24.380 | 1.190 | 0.964 |
Model two | 1.434 | 13.119 | 1.023 | 0.982 |
Fusion model of this paper | 1.285 | 12.285 | 0.924 | 0.985 |
WTCN-BiGRU-attention | 3.737 | 26.8 | 2.315 | 0.875 |
TCN-BiGRU-attention | 3.739 | 23.808 | 2.466 | 0.875 |
CNN-BiGRU-attention | 3.738 | 26.243 | 2.365 | 0.875 |
To sum up, the multiple models proposed in this paper combined with the short-term power load prediction model have more outstanding prediction performance, and the prediction results are relatively more stable, meaning that the model can be better used to predict the power load data with multidimensional feature inputs.
The major objective of this study was to build a forecasting model by integrating multiple models to improve the accuracy of power load forecasting. In Model one, decompose the data into multiple components by VMD decomposition. Then, an IWOA is exploited to optimize the super parameters of the WTCN-BiGRU-attention network model. At the same time, Model two is designed for the parallel prediction of multi-dimensional load data by the CatBoost algorithm. Finally, the MAPE-RW algorithm is employed to fuse the prediction results of the two models to achieve accurate and personal measurements of short-term power load data. Taking multidimensional load data of an area in Australia as a model example, the feasibility verification analysis was carried out, and the main conclusions are as follows:
1) Based on the power load data of a certain region in Australia, we constructed a multi-dimensional power load feature set to better predict the non-stationary components with strong fluctuation of power load data, i.e., Model one.
2) The stationary components of multi-dimensional power load data are predicted by Model two, and the model prediction results are fused by the MAPE-RW algorithm, which improve the power load prediction accuracy of multi-model fusion.
To sum up, the hybrid neural network combined model for multidimensional characteristic power load data prediction has been proposed in this paper. This research sheds new light and not only provides reference and choices for short-term power load forecasting methods, but also has good reference significance for other power fields, such as wind power generation forecasting and energy storage unit service-life forecasting. However, the structure of the multi-model fusion network is overly complex, which increases the model prediction time and wastes computer resources while improving the accuracy of power load prediction. Therefore, in the future, the authors will work on designing a more concise interval prediction model for power loading with suitable accuracy. While overcoming the training time, the interval prediction makes the prediction results more meaningful for the power sector to conduct power dispatching and effectively avoid the waste of power resources.
This work was supported by the State Grid Corporation of China Headquarters Science and Technology Project (5400-202122573A-0-5-SF). The authors thank the editors and the anonymous reviewers for their helpful comments and suggestions that have improved the presentation of this manuscript.
The authors declare that there is no conflict of interest.
[1] |
E. J. Candès, J. Romberg, T. Tao, Robust uncertainty principles: Exact signal reconstruction from highly incomplete frequency information, IEEE T. Inform. Theory, 52 (2006), 489–509. https://doi.org/10.1109/TIT.2005.862083 doi: 10.1109/TIT.2005.862083
![]() |
[2] |
E. J. Candès, M. B. Wakin, An introduction to compressive sampling, IEEE Signal Proc. Mag., 25 (2008), 21–30. https://doi.org/10.1109/MSP.2007.914731 doi: 10.1109/MSP.2007.914731
![]() |
[3] |
D. L. Donoho, For most large underdetermined systems of equations, the minimal ℓ1-norm near-solution approximates the sparsest near-solution, Commun. Pur. Appl. Math., 59 (2006), 907–934. https://doi.org/10.1002/cpa.20131 doi: 10.1002/cpa.20131
![]() |
[4] |
B. K. Natarajan, Sparse approximate solutions to linear systems, SIAM J. Comput., 24 (1995), 227–234. https://doi.org/10.1137/S0097539792240406 doi: 10.1137/S0097539792240406
![]() |
[5] |
S. S. Chen, D. L. Donoho, M. A. Saunders, Automatic decomposition by basis pursuit, SIAM Rev., 43 (2001), 129–159. https://doi.org/10.1137/S003614450037906X doi: 10.1137/S003614450037906X
![]() |
[6] |
S. J. Kim, K. Koh, M. Lustig, S. Boyd, D. Gorinevsky, An interior-point method for large-scale ℓ1-regularized least squares, IEEE J-STSP, 1 (2007), 606–617. https://doi.org/10.1109/JSTSP.2007.910971 doi: 10.1109/JSTSP.2007.910971
![]() |
[7] |
M. A. T. Figueiredo, R. D. Nowak, S. J. Wright, Gradient projection for sparse reconstruction: Application to compressed sensing and other inverse problems, IEEE J-STSP, 1 (2007), 586–597. https://doi.org/10.1109/JSTSP.2007.910281 doi: 10.1109/JSTSP.2007.910281
![]() |
[8] |
Y. H. Dai, Y. K. Huang, X. W. Liu, A family of spectral gradient methods for optimization, Comput. Optim. Appl., 74 (2019), 43–65. https://doi.org/10.1007/s10589-019-00107-8 doi: 10.1007/s10589-019-00107-8
![]() |
[9] |
S. Huang, Z. Wan, A new nonmonotone spectral residual method for nonsmooth nonlinear equations, J. Comput. Appl. Math., 313 (2017), 82–101. https://doi.org/10.1016/j.cam.2016.09.014 doi: 10.1016/j.cam.2016.09.014
![]() |
[10] |
L. Zheng, L. Yang, Y. Liang, A conjugate gradient projection method for solving equations with convex constraints, J. Comput. Appl. Math., 375 (2020), 112781. https://doi.org/10.1016/j.cam.2020.112781 doi: 10.1016/j.cam.2020.112781
![]() |
[11] |
J. F. Yang, Y. Zhang, Alternating direction algorithms for ℓ1−problems in compressive sensing, SIAM J. Sci. Comput., 33 (2011), 250–278. https://doi.org/10.1137/090777761 doi: 10.1137/090777761
![]() |
[12] |
I. Daubechies, M. Defrise, C. D. Mol, An iterative thresholding algorithm for linear inverse problems with a sparsity constraint, Commun. Pur. Appl. Math., 57 (2004), 1413–1457. https://doi.org/10.1002/cpa.20042 doi: 10.1002/cpa.20042
![]() |
[13] |
M. A. T. Figueiredo, R. D. Nowak, An EM algorithm for wavelet-based image restoration, IEEE T. Image Process., 12 (2003), 906C916. https://doi.org/10.1109/TIP.2003.814255 doi: 10.1109/TIP.2003.814255
![]() |
[14] |
E. T. Hale, W. T. Yin, Y. Zhang, Fixed-point continuation for ℓ1-Minimization: Methodology and convergence, SIAM J. Optim., 19 (2008), 1107–1130. https://doi.org/10.1137/070698920 doi: 10.1137/070698920
![]() |
[15] |
A. Beck, M. Teboulle, A fast iterative shrinkage-thresholding algorithm for linear inverse problems, SIAM J. Imaging Sci., 2 (2009), 183–202. https://doi.org/10.1137/080716542 doi: 10.1137/080716542
![]() |
[16] |
J. M. Bioucas-Dias, M. A. T. Figueiredo, A new TwIst: Two-step iterative shrinkage/thresholding algorithm for image restoration, IEEE T. Image Process., 16 (2007), 2992–3004. https://doi.org/10.1109/TIP.2007.909319 doi: 10.1109/TIP.2007.909319
![]() |
[17] |
P. L. Combettes, J. C. Pesquet, Proximal thresholding algorithm for minimization over orthonormal bases, SIAM J. Optim., 18 (2007), 1351–1376. https://doi.org/10.1137/060669498 doi: 10.1137/060669498
![]() |
[18] |
E. van den Berg, M. P. Friedlander, Probing the Pareto frontier for basis pursuit solutions, SIAM J. Sci. Comput., 31 (2008), 890–912. https://doi.org/10.1137/080714488 doi: 10.1137/080714488
![]() |
[19] |
S. Becker, J. Bobin, E. J. Cands, NESTA: A fast and accurate first-order method for sparse recovery, SIAM J. Imaging Sci., 4 (2011), 1–39. https://doi.org/10.1137/090756855 doi: 10.1137/090756855
![]() |
[20] |
S. J. Wright, R. D. Nowak, M. A. T. Figueiredo, Sparse reconstruction by separable approximation, IEEE Trans. Signal Proces., 57 (2009), 2479–2493. https://doi.org/10.1109/TSP.2009.2016892 doi: 10.1109/TSP.2009.2016892
![]() |
[21] |
N. Keskar, J. Nocedal, F. Oztoprak, A. Waechter, A second-order method for convex ℓ1-regularized optimization with active-set prediction, Optim. Metod. Softw., 31 (2016), 605–621. https://doi.org/10.1080/10556788.2016.1138222 doi: 10.1080/10556788.2016.1138222
![]() |
[22] | X. T. Xiao, Y. F. Li, Z. W. Wen, L. W. Zhang, Semi-smooth second-order type methods for composite convex programs, arXiv: 1603.07870v2 [math.OC], 2016. https://doi.org/10.48550/arXiv.1603.07870 |
[23] |
A. Milzarek, M. Ulbrich, A semismooth Newton method with multidimensional filter globalization for l1-optimization, SIAM J. Optim., 24 (2014), 298–333. https://doi.org/10.1137/120892167 doi: 10.1137/120892167
![]() |
[24] |
R. H. Byrd, J. Nocedal, F. Oztoprak, An inexact successive quadratic approximation method for L1 regularized optimization, Math. Program., 157 (2016), 375–396. https://doi.org/10.1007/s10107-015-0941-y doi: 10.1007/s10107-015-0941-y
![]() |
[25] |
Y. H. Xiao, H. Zhu, A conjugate gradient method to solve convex constrained monotone equations with applications in compressive sensing, J. Math. Anal. Appl., 405 (2013), 310–319. https://doi.org/10.1016/j.jmaa.2013.04.017 doi: 10.1016/j.jmaa.2013.04.017
![]() |
[26] |
M. Sun, M. Y. Tian, A class of derivative-free CG projection methods for nonsmooth equations with an application to the LASSO problem, B. Iran. Math. Soc., 46 (2020), 183–205. https://doi.org/10.1007/s41980-019-00250-2 doi: 10.1007/s41980-019-00250-2
![]() |
[27] |
H. C. Sun, M. Sun, B. H. Zhang, An inverse matrix-free proximal point algorithm for compressive sensing, ScienceAsia, 44 (2018), 311–318. https://doi.org/10.2306/scienceasia1513-1874.2018.44.311 doi: 10.2306/scienceasia1513-1874.2018.44.311
![]() |
[28] |
D. X. Feng, X. Y. Wang, A linearly convergent algorithm for sparse signal reconstruction, J. Fix. Point Theory Appl., 20 (2018), 154. https://doi.org/10.1007/s11784-018-0635-1 doi: 10.1007/s11784-018-0635-1
![]() |
[29] |
Y. H. Xiao, Q. Y. Wang, Q. J. Hu, Non-smooth equations based method for ℓ1-norm problems with applications to compressed sensing, Nonlinear Anal., 74 (2011), 3570–3577. https://doi.org/10.1016/j.na.2011.02.040 doi: 10.1016/j.na.2011.02.040
![]() |
[30] |
J. K. Liu, S. J. Li, A projection method for convex constrained monotone nonlinear equations with applications, Comput. Math. Appl., 70 (2015), 2442–2453. https://doi.org/10.1016/j.camwa.2015.09.014 doi: 10.1016/j.camwa.2015.09.014
![]() |
[31] |
J. K. Liu, Y. M. Feng, A derivative-free iterative method for nonlinear monotone equations with convex constraints, Numer. Algorithms, 82 (2019), 245–262. https://doi.org/10.1007/s11075-018-0603-2 doi: 10.1007/s11075-018-0603-2
![]() |
[32] |
Y. J. Wang, G. L. Zhou, L. Caccetta, W. Q. Liu, An alternative lagrange-dual based algorithm for sparse signal reconstruction, IEEE Trans. Signal Proces., 59 (2011), 1895–1901. https://doi.org/10.1109/TSP.2010.2103066 doi: 10.1109/TSP.2010.2103066
![]() |
[33] |
G. Landi, A modified Newton projection method for ℓ1-regularized least squares image deblurring, J. Math. Imaging Vis., 51 (2015), 195–208. https://doi.org/10.1007/s10851-014-0514-3 doi: 10.1007/s10851-014-0514-3
![]() |
[34] |
B. Xue, J. K. Du, H. C. Sun, Y. J. Wang, A linearly convergent proximal ADMM with new iterative format for BPDN in compressed sensing problem, AIMS Mathematics, 7 (2022), 10513–10533. https://doi.org/10.3934/math.2022586 doi: 10.3934/math.2022586
![]() |
[35] |
H. J. He, D. R. Han, A distributed Douglas-Rachford splitting method for multi-block convex minimization problems, Adv. Comput. Math., 42 (2016), 27–53. https://doi.org/10.1007/s10444-015-9408-1 doi: 10.1007/s10444-015-9408-1
![]() |
[36] |
M. Sun, J. Liu, A proximal Peaceman-Rachford splitting method for compressive sensing, J. Appl. Math. Comput., 50 (2016), 349–363. https://doi.org/10.1007/s12190-015-0874-x doi: 10.1007/s12190-015-0874-x
![]() |
[37] |
B. S. He, F. Ma, X. M. Yuan, Convergence study on the symmetric version of ADMM with larger step sizes, SIAM J. Imaging Sci., 9 (2016), 1467–1501. https://doi.org/10.1137/15M1044448 doi: 10.1137/15M1044448
![]() |
[38] |
H. J. He, C. Ling, H. K. Xu, An implementable splitting algorithm for the ℓ1-norm regularized split feasibility problem, J. Sci. Comput., 67 (2016), 281–298. https://doi.org/10.1007/s10915-015-0078-4 doi: 10.1007/s10915-015-0078-4
![]() |
[39] |
B. Qu, N. H. Xiu, A note on the CQ algorithm for the split feasibility problem, Inverse Probl., 21 (2005), 1655–1665. https://doi.org/10.1088/0266-5611/21/5/009 doi: 10.1088/0266-5611/21/5/009
![]() |
[40] | E. H. Zarantonello, Projections on convex sets in Hilbert space and spectral theory, In: Contributions to Nonlinear Functional Analysis, New York: Academic Press, 1971. https://doi.org/10.1016/B978-0-12-775850-3.50013-3 |
[41] |
M. A. Noor, General variational inequalities, Appl. Math. Lett., 1 (1988), 119–121. https://doi.org/10.1016/0893-9659(88)90054-7 doi: 10.1016/0893-9659(88)90054-7
![]() |
[42] |
J. M. Ortega, W. C. Rheinboldt, Iterative solution of nonlinear equations in several variables, Classics Appl. Math., 2000. https://doi.org/10.1137/1.9780898719468 doi: 10.1137/1.9780898719468
![]() |
[43] |
N. H. Xiu, J. Z. Zhang, Global projection-type error bound for general variational inequalities, J. Optim. Theory Appl., 112 (2002), 213–228. https://doi.org/10.1023/a:1013056931761 doi: 10.1023/a:1013056931761
![]() |
[44] |
M. K. Riahi, I. A. Qattan, On the convergence rate of Fletcher-Reeves nonlinear conjugate gradient methods satisfying strong Wolfe conditions: Application to parameter identification in problems governed by general dynamics, Math. Method Appl. Sci., 45 (2022), 3644–3664. https://doi.org/10.1002/mma.8009 doi: 10.1002/mma.8009
![]() |
[45] |
M. K. Riahi, A new approach to improve ill-conditioned parabolic optimal control problem via time domain decomposition, Numer. Algorithms, 3 (2016), 635–666. https://doi.org/10.1007/s11075-015-0060-0 doi: 10.1007/s11075-015-0060-0
![]() |
[46] |
E. J. Candˊes, Y. Plan, Tight oracle inequalities for low-rank matrix recovery from a minimal number of noisy random measurements, IEEE Trans. Inform. Theory, 57 (2011), 2342–2359. https://doi.org/10.1109/TIT.2011.2111771 doi: 10.1109/TIT.2011.2111771
![]() |
[47] |
W. D. Wang, F. Zhang, J. J. Wang, Low-rank matrix recovery via regularized nuclear norm minimization, Appl. Comput. Harmon. Anal., 54 (2021), 1–19. https://doi.org/10.1016/j.acha.2021.03.001 doi: 10.1016/j.acha.2021.03.001
![]() |
1. | Ruihan Diao, Yang Lv, Yangyang Ding, Short-term power load comparison based on time series and neural networks considering multiple features, 2023, 2625, 1742-6588, 012002, 10.1088/1742-6596/2625/1/012002 | |
2. | Lingcong Xu, Lanfeng Zhou, 2024, Short-Term Load Forecasting Based on TVF-EMD and CNN-GRU Optimized By DBO, 979-8-3503-0963-8, 1503, 10.1109/ACPEE60788.2024.10532328 | |
3. | Mingju Chen, Fuhong Qiu, Xingzhong Xiong, Zhengwei Chang, Yang Wei, Jie Wu, BILSTM-SimAM: An improved algorithm for short-term electric load forecasting based on multi-feature, 2024, 21, 1551-0018, 2323, 10.3934/mbe.2024102 | |
4. | Lei Dai, Haiying Wang, An Improved WOA (Whale Optimization Algorithm)-Based CNN-BIGRU-CBAM Model and Its Application to Short-Term Power Load Forecasting, 2024, 17, 1996-1073, 2559, 10.3390/en17112559 | |
5. | Qifan Chen, Yunfei Ding, Kun Tian, Qiancheng Sun, 2025, Chapter 4, 978-3-031-73406-9, 33, 10.1007/978-3-031-73407-6_4 |
Characteristic parameters | Parameter types | Character descriptions |
Data | Time | Samples were taken every 30 minutes |
Weather factors | Dew-point humidity | Equilibrium temperature |
Dry-bulb humidity | Aerothermodynamic temperature | |
Wet-bulb temperature | Thermodynamic saturation temperature | |
Humidity | Degree of atmospheric dryness | |
Economic factors | Degree | Price per kWh |
k | Simulated signal center frequency | |||||
2 | 0.0001 | 0.02243 | ||||
3 | 0.0001 | 0.02080 | 0.04274 | |||
4 | 0.0001 | 0.02076 | 0.04167 | 0.06471 | ||
5 | 0.0001 | 0.02076 | 0.04162 | 0.06469 | 0.34702 | |
6 | 0.0001 | 0.02076 | 0.04162 | 0.06238 | 0.08467 | 0.35872 |
Parameters | Parameter values | |
Parameter settings | Population size | 5 |
Max iterations | 5 | |
Constant b | 2 | |
Search for upper and lower bounds | Learn rate | [0.001, 0.01] |
Epoch | [10,100] | |
Batchsize | [16,128] | |
BiGRU node number | [1,20] | |
Number of nodes at the full | [1,100] |
Modal tags | IMF1 | IMF2 | IMF3 | IMF4 | IMF5 |
Learn rate | 0.0047 | 0.0027 | 0.0064 | 0.0054 | 0.0024 |
Epoch | 38 | 26 | 61 | 70 | 89 |
Batchsize | 72 | 19 | 72 | 37 | 22 |
BiGRU node number | 8 | 18 | 8 | 17 | 2 |
BiGRU node number | 16 | 11 | 7 | 17 | 19 |
Number of nodes at the full | 49 | 4 | 46 | 14 | 97 |
Verbose | Learning rate | Iterations | Depth |
50 | 0.03 | 900 | 12 |
Prediction model | RMSE | MAPE | MAE | R-square |
IWOA-WTCN-BiGRU-attention | 110.964 | 0.914 | 82.189 | 0.993 |
CatBoost | 89.089 | 0.727 | 63.407 | 0.996 |
Fusion model of this paper | 77.495 | 0.632 | 56.103 | 0.997 |
WTCN-BiGRU-attention | 103.452 | 0.86 | 76.179 | 0.994 |
WTCN-BiGRU | 97.573 | 0.803 | 69.903 | 0.995 |
WTCN-GRU | 96.584 | 0.814 | 70.868 | 0.995 |
WTCN-LSTM | 100.309 | 0.843 | 73.649 | 0.995 |
WTCN | 103.558 | 0.869 | 75.975 | 0.994 |
TCN | 103.969 | 0.89 | 78.024 | 0.994 |
LSTM | 147.665 | 1.261 | 108.342 | 0.988 |
GRU | 109.991 | 0.932 | 82.114 | 0.994 |
CNN | 337.913 | 3.241 | 275.52 | 0.939 |
RMSE | Model one | Model two | Fusion model | WT-BG-a | WT-BG | WT-G | WT-L | WT |
Jan. | 108.372 | 106.039 | 97.048 | 126.77 | 116.382 | 96.321 | 107.057 | 121.456 |
Feb. | 102.257 | 84.791 | 76.02 | 108.625 | 89.625 | 90.072 | 88.652 | 103.947 |
Mar. | 85.485 | 72.585 | 61.48 | 84.297 | 76.175 | 80.666 | 85.047 | 86.109 |
Apr. | 96.362 | 106.033 | 83.271 | 105.568 | 110.002 | 108.207 | 109.236 | 113.008 |
May | 121.924 | 95.097 | 80 | 101.731 | 101.456 | 101.066 | 100.091 | 108.432 |
Jun. | 141.496 | 86.97 | 82.599 | 100.258 | 91.622 | 93.661 | 98.118 | 104.279 |
Jul. | 131.409 | 86.787 | 77.983 | 101.809 | 87.982 | 92.821 | 95.962 | 100.363 |
Aug. | 170.315 | 84.826 | 102.867 | 105.915 | 103.802 | 105.615 | 112.917 | 107.68 |
Sept. | 127.524 | 83.933 | 81.581 | 104.565 | 99.724 | 99.005 | 101.172 | 100.071 |
Oct. | 85.224 | 89.977 | 65.933 | 95.965 | 93.855 | 99.785 | 101.756 | 97.116 |
Nov. | 79.742 | 84.105 | 62.097 | 99.21 | 95.347 | 101.174 | 106.564 | 100.065 |
Dec. | 79.927 | 82.657 | 63.727 | 103.142 | 101.035 | 92.024 | 101.432 | 101.644 |
Modal tags | IMF1 | IMF2 | IMF3 | IMF4 | IMF5 |
Learn rate | 0.0038 | 0.0012 | 0.0059 | 0.0067 | 0.0030 |
Epoch | 72 | 54 | 50 | 73 | 96 |
Batchsize | 32 | 57 | 118 | 117 | 70 |
BiGRU node number | 5 | 1 | 16 | 10 | 3 |
BiGRU node number | 6 | 7 | 15 | 13 | 12 |
Number of nodes at the full | 44 | 12 | 54 | 84 | 12 |
Verbose | Learning rate | Iterations | Depth |
50 | 0.05 | 500 | 9 |
Prediction model | RMSE | MAPE | MAE | R-square |
Model one | 1.996 | 24.380 | 1.190 | 0.964 |
Model two | 1.434 | 13.119 | 1.023 | 0.982 |
Fusion model of this paper | 1.285 | 12.285 | 0.924 | 0.985 |
WTCN-BiGRU-attention | 3.737 | 26.8 | 2.315 | 0.875 |
TCN-BiGRU-attention | 3.739 | 23.808 | 2.466 | 0.875 |
CNN-BiGRU-attention | 3.738 | 26.243 | 2.365 | 0.875 |
Characteristic parameters | Parameter types | Character descriptions |
Data | Time | Samples were taken every 30 minutes |
Weather factors | Dew-point humidity | Equilibrium temperature |
Dry-bulb humidity | Aerothermodynamic temperature | |
Wet-bulb temperature | Thermodynamic saturation temperature | |
Humidity | Degree of atmospheric dryness | |
Economic factors | Degree | Price per kWh |
k | Simulated signal center frequency | |||||
2 | 0.0001 | 0.02243 | ||||
3 | 0.0001 | 0.02080 | 0.04274 | |||
4 | 0.0001 | 0.02076 | 0.04167 | 0.06471 | ||
5 | 0.0001 | 0.02076 | 0.04162 | 0.06469 | 0.34702 | |
6 | 0.0001 | 0.02076 | 0.04162 | 0.06238 | 0.08467 | 0.35872 |
Parameters | Parameter values | |
Parameter settings | Population size | 5 |
Max iterations | 5 | |
Constant b | 2 | |
Search for upper and lower bounds | Learn rate | [0.001, 0.01] |
Epoch | [10,100] | |
Batchsize | [16,128] | |
BiGRU node number | [1,20] | |
Number of nodes at the full | [1,100] |
Modal tags | IMF1 | IMF2 | IMF3 | IMF4 | IMF5 |
Learn rate | 0.0047 | 0.0027 | 0.0064 | 0.0054 | 0.0024 |
Epoch | 38 | 26 | 61 | 70 | 89 |
Batchsize | 72 | 19 | 72 | 37 | 22 |
BiGRU node number | 8 | 18 | 8 | 17 | 2 |
BiGRU node number | 16 | 11 | 7 | 17 | 19 |
Number of nodes at the full | 49 | 4 | 46 | 14 | 97 |
Verbose | Learning rate | Iterations | Depth |
50 | 0.03 | 900 | 12 |
Prediction model | RMSE | MAPE | MAE | R-square |
IWOA-WTCN-BiGRU-attention | 110.964 | 0.914 | 82.189 | 0.993 |
CatBoost | 89.089 | 0.727 | 63.407 | 0.996 |
Fusion model of this paper | 77.495 | 0.632 | 56.103 | 0.997 |
WTCN-BiGRU-attention | 103.452 | 0.86 | 76.179 | 0.994 |
WTCN-BiGRU | 97.573 | 0.803 | 69.903 | 0.995 |
WTCN-GRU | 96.584 | 0.814 | 70.868 | 0.995 |
WTCN-LSTM | 100.309 | 0.843 | 73.649 | 0.995 |
WTCN | 103.558 | 0.869 | 75.975 | 0.994 |
TCN | 103.969 | 0.89 | 78.024 | 0.994 |
LSTM | 147.665 | 1.261 | 108.342 | 0.988 |
GRU | 109.991 | 0.932 | 82.114 | 0.994 |
CNN | 337.913 | 3.241 | 275.52 | 0.939 |
RMSE | Model one | Model two | Fusion model | WT-BG-a | WT-BG | WT-G | WT-L | WT |
Jan. | 108.372 | 106.039 | 97.048 | 126.77 | 116.382 | 96.321 | 107.057 | 121.456 |
Feb. | 102.257 | 84.791 | 76.02 | 108.625 | 89.625 | 90.072 | 88.652 | 103.947 |
Mar. | 85.485 | 72.585 | 61.48 | 84.297 | 76.175 | 80.666 | 85.047 | 86.109 |
Apr. | 96.362 | 106.033 | 83.271 | 105.568 | 110.002 | 108.207 | 109.236 | 113.008 |
May | 121.924 | 95.097 | 80 | 101.731 | 101.456 | 101.066 | 100.091 | 108.432 |
Jun. | 141.496 | 86.97 | 82.599 | 100.258 | 91.622 | 93.661 | 98.118 | 104.279 |
Jul. | 131.409 | 86.787 | 77.983 | 101.809 | 87.982 | 92.821 | 95.962 | 100.363 |
Aug. | 170.315 | 84.826 | 102.867 | 105.915 | 103.802 | 105.615 | 112.917 | 107.68 |
Sept. | 127.524 | 83.933 | 81.581 | 104.565 | 99.724 | 99.005 | 101.172 | 100.071 |
Oct. | 85.224 | 89.977 | 65.933 | 95.965 | 93.855 | 99.785 | 101.756 | 97.116 |
Nov. | 79.742 | 84.105 | 62.097 | 99.21 | 95.347 | 101.174 | 106.564 | 100.065 |
Dec. | 79.927 | 82.657 | 63.727 | 103.142 | 101.035 | 92.024 | 101.432 | 101.644 |
Modal tags | IMF1 | IMF2 | IMF3 | IMF4 | IMF5 |
Learn rate | 0.0038 | 0.0012 | 0.0059 | 0.0067 | 0.0030 |
Epoch | 72 | 54 | 50 | 73 | 96 |
Batchsize | 32 | 57 | 118 | 117 | 70 |
BiGRU node number | 5 | 1 | 16 | 10 | 3 |
BiGRU node number | 6 | 7 | 15 | 13 | 12 |
Number of nodes at the full | 44 | 12 | 54 | 84 | 12 |
Verbose | Learning rate | Iterations | Depth |
50 | 0.05 | 500 | 9 |
Prediction model | RMSE | MAPE | MAE | R-square |
Model one | 1.996 | 24.380 | 1.190 | 0.964 |
Model two | 1.434 | 13.119 | 1.023 | 0.982 |
Fusion model of this paper | 1.285 | 12.285 | 0.924 | 0.985 |
WTCN-BiGRU-attention | 3.737 | 26.8 | 2.315 | 0.875 |
TCN-BiGRU-attention | 3.739 | 23.808 | 2.466 | 0.875 |
CNN-BiGRU-attention | 3.738 | 26.243 | 2.365 | 0.875 |