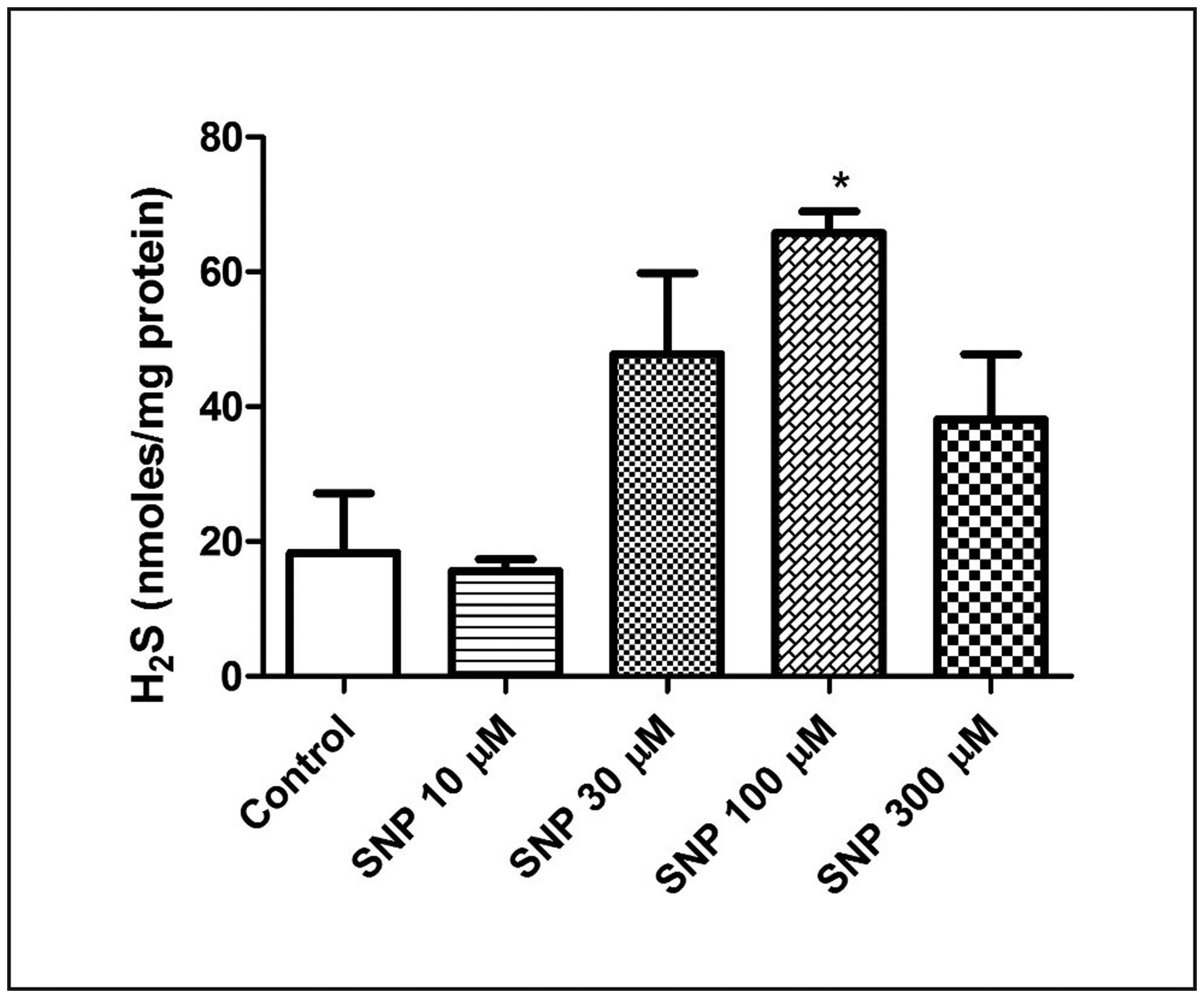
The coronavirus disease 2019 (COVID-19) outbreak has resulted in countless infections and deaths worldwide, posing increasing challenges for the health care system. The use of artificial intelligence to assist in diagnosis not only had a high accuracy rate but also saved time and effort in the sudden outbreak phase with the lack of doctors and medical equipment. This study aimed to propose a weakly supervised COVID-19 classification network (W-COVNet). This network was divided into three main modules: weakly supervised feature selection module (W-FS), deep learning bilinear feature fusion module (DBFF) and Grad-CAM++ based network visualization module (Grad-Ⅴ). The first module, W-FS, mainly removed redundant background features from computed tomography (CT) images, performed feature selection and retained core feature regions. The second module, DBFF, mainly used two symmetric networks to extract different features and thus obtain rich complementary features. The third module, Grad-Ⅴ, allowed the visualization of lesions in unlabeled images. A fivefold cross-validation experiment showed an average classification accuracy of 85.3%, and a comparison with seven advanced classification models showed that our proposed network had a better performance.
Citation: Jingyao Liu, Qinghe Feng, Yu Miao, Wei He, Weili Shi, Zhengang Jiang. COVID-19 disease identification network based on weakly supervised feature selection[J]. Mathematical Biosciences and Engineering, 2023, 20(5): 9327-9348. doi: 10.3934/mbe.2023409
[1] | I. Banegas, I. Prieto, A.B. Segarra, M. Martínez-Cañamero, M. de Gasparo, M. Ramírez-Sánchez . Angiotensin II, dopamine and nitric oxide. An asymmetrical neurovisceral interaction between brain and plasma to regulate blood pressure. AIMS Neuroscience, 2019, 6(3): 116-127. doi: 10.3934/Neuroscience.2019.3.116 |
[2] | Nicholas J. D. Wright . A review of the actions of Nitric Oxide in development and neuronal function in major invertebrate model systems. AIMS Neuroscience, 2019, 6(3): 146-174. doi: 10.3934/Neuroscience.2019.3.146 |
[3] | Valentina Bashkatova, Athineos Philippu . Role of nitric oxide in psychostimulant-induced neurotoxicity. AIMS Neuroscience, 2019, 6(3): 191-203. doi: 10.3934/Neuroscience.2019.3.191 |
[4] | Md. Mamun Al-Amin, Robert K. P. Sullivan, Suzy Alexander, David A. Carter, DanaKai Bradford, Thomas H. J. Burne . Impaired spatial memory in adult vitamin D deficient BALB/c mice is associated with reductions in spine density, nitric oxide, and neural nitric oxide synthase in the hippocampus. AIMS Neuroscience, 2022, 9(1): 31-56. doi: 10.3934/Neuroscience.2022004 |
[5] | Amber Woodard, Brandon Barbery, Reid Wilkinson, Jonathan Strozyk, Mathew Milner, Patrick Doucette, Jarred Doran, Kendra Appleby, Henry Atwill, Wade E. Bell, James E. Turner . The role of neuronal nitric oxide and its pathways in the protection and recovery from neurotoxin-induced de novo hypokinetic motor behaviors in the embryonic zebrafish (Danio rerio). AIMS Neuroscience, 2019, 6(1): 25-42. doi: 10.3934/Neuroscience.2019.1.25 |
[6] | Gigi Tevzadze, Tamar Barbakadze, Elisabed Kvergelidze, Elene Zhuravliova, Lali Shanshiashvili, David Mikeladze . Gut neurotoxin p-cresol induces brain-derived neurotrophic factor secretion and increases the expression of neurofilament subunits in PC-12 cells. AIMS Neuroscience, 2022, 9(1): 12-23. doi: 10.3934/Neuroscience.2022002 |
[7] | Ernest Greene . New encoding concepts for shape recognition are needed. AIMS Neuroscience, 2018, 5(3): 162-178. doi: 10.3934/Neuroscience.2018.3.162 |
[8] | Adeshina O. Adekeye, Adedamola A. Fafure, Ayoola E. Ogunsemowo, Linus A. Enye, Olusola S. Saka, Oluwatosin O. Ogedengbe . Naringin ameliorates motor dysfunction and exerts neuroprotective role against vanadium-induced neurotoxicity. AIMS Neuroscience, 2022, 9(4): 536-550. doi: 10.3934/Neuroscience.2022031 |
[9] | Nouhaila Chaoui, Hammou Anarghou, Meriem Laaroussi, Oumaima Essaidi, Mohamed Najimi, Fatiha Chigr . Long lasting effect of acute restraint stress on behavior and brain anti-oxidative status. AIMS Neuroscience, 2022, 9(1): 57-75. doi: 10.3934/Neuroscience.2022005 |
[10] | Ernest Greene, Michael J. Hautus . Demonstrating Invariant Encoding of Shapes Using A Matching Judgment Protocol. AIMS Neuroscience, 2017, 4(3): 120-147. doi: 10.3934/Neuroscience.2017.3.120 |
The coronavirus disease 2019 (COVID-19) outbreak has resulted in countless infections and deaths worldwide, posing increasing challenges for the health care system. The use of artificial intelligence to assist in diagnosis not only had a high accuracy rate but also saved time and effort in the sudden outbreak phase with the lack of doctors and medical equipment. This study aimed to propose a weakly supervised COVID-19 classification network (W-COVNet). This network was divided into three main modules: weakly supervised feature selection module (W-FS), deep learning bilinear feature fusion module (DBFF) and Grad-CAM++ based network visualization module (Grad-Ⅴ). The first module, W-FS, mainly removed redundant background features from computed tomography (CT) images, performed feature selection and retained core feature regions. The second module, DBFF, mainly used two symmetric networks to extract different features and thus obtain rich complementary features. The third module, Grad-Ⅴ, allowed the visualization of lesions in unlabeled images. A fivefold cross-validation experiment showed an average classification accuracy of 85.3%, and a comparison with seven advanced classification models showed that our proposed network had a better performance.
The presence of enzymes responsible for the biosynthesis of hydrogen sulfide (H2S) from the amino acid, L-cysteine in mammalian tissues has stimulated a surge of interest in its potential physiological significance in biological processes [1],[2]. There is evidence that H2S can share similar physiological and pharmacological actions with other gaseous transmitters such as nitric oxide (NO) and carbon monoxide (CO) [3]–[5]. In some pathophysiological conditions, the three gaseous molecules are present in specific concentrations in disease states such as Alzheimer's when their endogenous production is known to be compromised [6]–[8]. Although these gases possess similar features and modes of action, H2S has been reported to exhibit a number of distinct characteristics from both NO and CO [7]. For instance, H2S has greater water solubility and the lowest potential to penetrate lipid bilayers, and has a different pathway that leads to its biosynthesis when compared with its two gaseous counterparts [9]. The basal production of H2S is dependent upon the activity of two pyridoxal-5′-phosphate dependent-enzymes, cystathionine β-synthase (CBS) and cystathionine γ-lyase (CSE), whilst NO is synthesized from L-arginine by NO synthase and CO is endogenously produced from heme by heme oxygenase [10]–[15]. In the presence of aspartate aminotransferase, L-cysteine can also be converted to H2S by the enzyme, 3-mercaptopyruvate sulfur-transferase (3MST) [16]–[20]. Since the physiological and pharmacological targets for H2S are analogous to NO and CO, evidence available from literature supports an interaction between these gaseous transmitters in mammalian organs and tissues [7],[21],[22]. Indeed, studies in neuronal tissues and the vasculature show that the interaction between these gases can affect their biosynthetic pathways, as well as, induce changes in their biological responses [23]. While H2S has been shown to alter the activity and expression of NO producing enzyme (eNOS), there is evidence that NO substrate or donors can also modulate expression and activity of H2S producing enzymes [3],[7],[24]–[26]. Furthermore, studies by Whiteman et al. [27] suggest that H2S may interact with NO to form an unidentified nitrosothiol moiety, which may then regulate physiological functions of both NO and H2S [24]. Likewise, there is compelling evidence of an interaction between H2S and CO. H2S has been reported to play a regulatory role in CO/heme oxygenase pathways in cardiac and pulmonary diseases [28]. Indeed, under physiological conditions, CO/heme oxygenase and H2S/CSE pathways have been demonstrated to inhibit each other in aortic smooth muscle cells [29]. However, it remains to be determined whether NO and CO can interact with the pathway leading to H2S biosynthesis in the retina. The aim of the present study was, therefore, to investigate whether inhibitors and/or activators of both NO and CO pathways can alter H2S concentrations in the bovine isolated neural retina. Parts of the work described in this paper have been communicated in an abstract form [30].
Hemin, zinc protoporphyrin (ZnPP-IX), L-nitroarginine methyl ester (L-NAME), sodium nitroprusside (SNP), aminooxyacetic acid (AOAA), ethylenediamine tetra-acetic acid solution (EDTA), zinc acetate, ferric chloride, borate buffer (pH 11.0) and N, N-dimethyl-p-phenylenediamine sulfate were all purchased from Sigma–Aldrich, St. Louis, MO. All reagents were freshly prepared in distilled water, except N, N-dimethyl-p-phenylenediamine which was prepared in 7.2 M hydrochloric acid, immediately before use in the series of experiments.
Bovine eyes were obtained from Vision Tech, Dallas, TX within 24 hours following enucleation and transported to the laboratory on ice. An incision was made along the equator of each eye and the vitreous humor and lens were delicately removed. The neural retinae were isolated by gentle removal from the posterior segment of the eye and immediately immersed in ice-cold 50 mM potassium phosphate buffer (pH 7.4). Time elapsed between animal sacrifice and tissue preparation was less than 24 hours.
H2S production was measured using the Methylene Blue method, a widely used assay that has been shown to produce quantifiable measurements of H2S production in mammalian tissues comparable to data obtained using H2S probes [31]–[35]. The methodology employed for H2S measurement in retinal tissues was essentially the same as previously reported by Xia et al. [36] and Kulkarni et al. [37], with some minor modifications. In brief, isolated bovine neural retina were homogenized in freshly prepared 50 mM ice-cold potassium phosphate buffer (pH 7.4) using a hand held tissue grinder consisting of a pestle and mortar. The tissue homogenates (0.25 ml) were incubated in the presence or absence of NO synthase inhibitor, L-nitroarginine methyl ester (L-NAME; 300 nM–500 µM), NO donor, sodium nitroprusside (SNP; 10–300 µM), hemoxygenase-1 inhibitor, zinc protoporphyrin (ZnPP-IX; 300 nM–10 µM) and hemoxygenase-1 inducer, hemin (300 nM–20 µM). The drug concentrations chosen were based on previously published reports on the pharmacological effects of NO and CO on mammalian tissues and cells [29],[37]. Incubation was carried at 37 °C for 90 min in tightly sealed eppendorf tubes for the enzymatic reaction to take place for optimum production of H2S as reported by [36],[37]. EDTA (0.125 ml; 5 mM) was injected into the reaction tubes to stop the reaction, followed by 0.5 ml of zinc acetate (1%) and 0.5 ml of borate buffer (pH 11.0). The tubes were sealed and then incubated at 37°C for another 30 minutes. The reaction solution was mixed with 0.5 ml of N, N-dimethyl-p-phenylenediamine sulfate (20 mM, in 7.2 M HCl) and 0.5 ml of FeCl3 (30 mM) at 37 °C for an additional 10 minutes and then centrifuged at 5000g for 3 min. The absorbance of resulting solution was measured at 670 nm with a spectrophotometer. The H2S concentration was calculated against the calibration curve of standard H2S solutions (10–750 µM). The protein content of the residual pellet after centrifugation was measured using the Bradford method.
Results were expressed as nanomolar of H2S produced per milligram soluble protein. Values given are arithmetic means ± SEM. The means were determined from three separate experiments performed in triplicates. Significance of differences between control and treatment groups were evaluated using one-way analysis of variance (ANOVA) followed by Newman-Keul comparison test. (Graph Pad Prism Software, San Diego, CA). The level of significance was chosen as p < 0.05.
In a previous study, we showed evidence that endogenous concentrations of H2S in isolated bovine retinae were enhanced by the substrate, L-cysteine and attenuated by inhibitors of enzymes responsible for the biosynthesis of this gas [37]. In the present study, the NO donor, SNP (10–100 µM) caused a concentration-dependent increase in H2S levels reaching a maximum at 100 µM (65.7 nm/mg of protein) (Figure 1). On other hand, the NO inhibitor L-NAME (300 nM–500 µM) elicited a concentration-dependent decrease in the basal endogenous levels of H2S from 19.2 to 2.6 nm/mg of protein (Figure 2).
We next investigated the pharmacological action of the heme oxygenase-1 inducer, hemin (300 nM–20 µM) on basal concentrations of H2S in the bovine isolated retina. As shown in Figure 3, hemin produced a concentration-dependent attenuation of basal levels of H2S from 18.9 to 3.6 nm/mg of protein. Conversely, the heme oxygenase inhibitor, ZnPP-IX (300 nM–10 µM) significantly increased the concentration of H2S from 19.2 to 26.5 nm/mg of protein in a concentration-dependent manner, reaching a maximal effect at 10 µM (Figure 4).
It is well-established that H2S can serve as a gaseous neurotransmitter/neuromodulator in several mammalian tissues and organs. Indeed, there is abundant evidence that H2S is involved in several physiological and pathophysiological processes as diverse as learning and memory, inflammation, and the regulation of blood pressure [6],[38],[39]. In the cardiovascular system, H2S has been shown to play an important role in maintenance of vascular smooth muscle tone whereas in the central nervous system, this gas has been found to act as a neurotransmitter/neuromodulator at synapses [40]–[43]. Furthermore, H2S has also been reported to exert a neuroprotective action on neurons [11]. The observed biological actions of H2S in the vasculature and brain have been reported to resemble those of other established gaseous transmitters like CO and NO [44],[45]. Although, studies reported in literature have focused on understanding the physiological and pharmacological roles of H2S in cardiovascular, central nervous and immune systems, there is limited evidence of an interaction between NO, CO and H2S in regulating some of the observed responses in the above-named systems [8]. In the eye, changes in cyclic GMP levels have been reported due to an interaction between these gaseous transmitters in the salamander retina and the rabbit ophthalmic artery [46],[47]. In the present study, we investigated the possibility that activation or inhibition of pathways involved in NO and CO biosynthesis can alter basal endogenous levels of H2S in the bovine isolated retina.
There is evidence that in the cardiovascular and central nervous systems, nitric oxide synthase (NOS) substrates or NO donors can up-regulate the expression or activity of H2S producing enzymes [48]–[51]. Other investigators also report that L-arginine can attenuate pulmonary artery pressure by augmenting the expression and activity of CSE mRNA in lung tissue [52]. Furthermore, there is evidence suggesting that a synergistic interaction exists between NO and H2S in regulating vascular function [53]–[56]. In the present study, we measured endogenous levels of H2S in the presence of NO donor, SNP. The incubation time period for optimal production of endogenous basal levels of H2S was previously established in our laboratory [37]. We found that SNP increased the basal endogenous levels of H2S suggesting that increased NO availability can regulate the production of this gas in the retina. We also observed that at a high concentration of SNP (300 µM) the basal endogenous levels of H2S was decreased, suggesting that NO may also have the ability to inhibit H2S production. The precise mechanism for this effect is not clear. However, it may be due to the fact that at high concentrations of SNP, the NO generated binds to CBS and thus has the potential to inhibit its activity and therefore the generation of H2S. Despite this, our observations correlate well with studies in the vasculature depicting the ability of SNP to increase the biosynthesis of H2S [48]–[50]. Indeed, there is evidence that in the presence of NO, the pharmacological actions of H2S are significantly enhanced [8],[56]. The ability of NO to stimulate H2S production may be due to an augmented activity of enzymes responsible for the biosynthesis of H2S by the NO-induced S-nitrosylation of their cysteine residues or indirect stimulation of CSE by NO through an increase in the activity of cyclic GMP-dependent kinases [8],[35],[45]. Taken together, these results indicate that NO may interact directly with CBS and CSE to augment H2S production. To confirm the nature of this interaction, we investigated the effect of an inhibitor of NO synthase, L-NAME on endogenous H2S production. Our results demonstrate that L-NAME reduced basal H2S levels suggesting that endogenously generated NO can modulate the basal levels of H2S presumably through an interaction with pathways involved in H2S production. Other studies in the vasculature have also reported a similar inhibitory effect of L-NAME on H2S production and activity [8],[53]. The exact mechanisms +that underlie H2S and NO interactions in the neural retinae are unknown and merits further investigation.
In addition to studying the possible interaction between NO and H2S, we designed experiments to determine whether changes in CO production can regulate the endogenous levels of H2S in neural retinae. In these studies, hemin and ZnPP-IX were employed as an inducer and an inhibitor of heme oxygenase, respectively [57],[58]. We found that hemin caused a concentration-related decrease in basal levels of H2S. In contrast, ZnPP-IX elicited a concentration-dependent increase in endogenous H2S levels. Our results reveal that increasing CO production led to a decrease in basal concentrations of H2S whereas, inhibiting CO biosynthesis can cause an elevation of H2S levels. These results correlate well with studies reported by Jin et al. [29] who showed that endogenous CO down-regulates the H2S production in aortic smooth muscle cells. A similar interaction between these two gaseous transmitters has also been observed in the carotid body, the liver, and the microvasculature of the brain [59]–[61]. It appears that in the bovine retina, reduction of CO biosynthesis removes a tonic inhibitory action of this gas on H2S production. It is, however, unclear how CO acts to regulate the biosynthesis of H2S in the retina.
Previous evidence from our laboratory and others suggests that crosstalk between the three gasotransmitters exists in ocular tissues [46],[47],[62]–[64]. In the salamander retina, all three gases were found to play interactive roles in modulating retinal cGMP signaling, as NO and CO significantly increased cGMP-like immunoreactivity (cGMP-LI) both alone and synergistically [46]. NaHS was found to inhibit the NO-induced increase in cGMP-LI from the inner nuclear layer to the ganglion cell layer, indicating an interaction in these three gases in the retina [46]. H2S was found to enhance phenylephrine induced tone in the rabbit ophthalmic artery, while CO and NO both caused concentration dependent relaxations [47]. The mechanisms behind these pharmacological actions may be attributed to the gaseous transmitters' ability to modulate cGMP production in the rabbit model, as H2S was found to reduce cGMP levels and prevent a NO induced increase in cGMP [47]. The relaxing effect of hydrogen sulfide donors on smooth muscles in both bovine and porcine ocular tissues have also been found to be dependent, at least in part, on the presence and activity of nitric oxide [62]–[64]. Taken together, these studies implicate an interactive regulatory role of these gaseous modulators in ocular tissues.
In conclusion, changes in the biosynthesis and availability of both NO and CO can interfere with the pathway/s involved in the production of H2S in the bovine isolated retina. The present observation that basal levels of H2S can be altered by manipulation of pathways involved in NO/CO production is of great interest and merits further investigation.
Despite these novel findings, the results should be interpreted with prudence due to limitation of our study protocol. For instance the colorimetric methodology used for determination of endogenous production of H2S is based on the assumption that tissue concentration of sulfide are high, and hence it does not distinguish between acid labile sulfur stores or bound sulfane stores. In addition the enzyme inhibitors being used in the studies are pyridoxal-5′ phosphate (PLP) enzyme inhibitors that are not specific for H2S biosynthesizing enzymes, CBS and CSE as these enzymes are known to be involved in other cellular processes in mammals. Thus, it would be worthwhile to investigate whether H2S synthesis alters the expression of these biosynthesizing enzymatic proteins. Despite the limitations of the study protocol, the results provide a basis for future studies on the role of H2S in the posterior segment of the eye.
[1] |
O. A. Ataguba, J. E. Ataguba, Social determinants of health: the role of effective communication in the COVID-19 pandemic in developing countries, Global Health Action, 1 (2020), 1788263. https://doi.org/10.1080/16549716.2020.1788263 doi: 10.1080/16549716.2020.1788263
![]() |
[2] | World Health Organization, WHO coronavirus disease (COVID-19) Dashboard, 2022. Available from: https://covid19.who.int/?gclid = Cj0KCQjwtZH7BRDzARIsAGjbK2ZXWRpJROEl97HGmSOx0_ydkVbc02Ka1FlcysGjEI7hnaIeR6xWhr4aAu57EALw_wcB. |
[3] |
Q. Huang, X. Huang, Z. Kong, X. Li, D. Tao, Bi-phase evolutionary searching for biclusters in gene expression data, IEEE Trans. Evol. Comput., 5 (2018), 803–814. https://doi.org/10.1109/TEVC.2018.2884521 doi: 10.1109/TEVC.2018.2884521
![]() |
[4] |
Q. Huang, J. Yao, J. Li, M. Li, M. R. Pickering, X. Li, Measurement of quasi-static 3-D knee joint movement based on the registration from CT to US, IEEE Trans. Ultrason. Free, 6 (2020), 1141–50. https://doi.org/10.1109/TUFFC.2020.2965149 doi: 10.1109/TUFFC.2020.2965149
![]() |
[5] |
J. Xi, Z. Miao, L. Liu, X. Yang, W. Zhang, Q. Huang, et al., Knowledge tensor embedding framework with association enhancement for breast ultrasound diagnosis of limited labeled samples, Neurocomputing, 468 (2022), 60–70. https://doi.org/10.1016/j.neucom.2021.10.013 doi: 10.1016/j.neucom.2021.10.013
![]() |
[6] |
Q. Huang, F. Pan, W. Li, F. Yuan, H. Hu, J. Huang, et al., Differential diagnosis of atypical hepatocellular carcinoma in contrast-enhanced ultrasound using spatio-temporal diagnostic semantics, IEEE J. Biomed. Health, 10 (2020), 2860–2869. https://doi.org/10.1109/JBHI.2020.2977937 doi: 10.1109/JBHI.2020.2977937
![]() |
[7] |
J. Xi, D. Wang, X. Yang, W. Zhang, Q. Huang, Cancer omic data based explainable AI drug recommendation inference: A traceability perspective for explainability, Biomed. Signal Process., 79 (2023), 104144. https://doi.org/10.1016/j.bspc.2022.104144 doi: 10.1016/j.bspc.2022.104144
![]() |
[8] |
W. Shi, W. N. Chen, S. Kwong, J. Zhang, H. Wang, T. Gu, et al., A coevolutionary estimation of distribution algorithm for group insurance portfolio, IEEE Trans. Syst. Man CY-S, 11 (2021), 6714–28. https://doi.org/10.1109/TSMC.2021.3096013 doi: 10.1109/TSMC.2021.3096013
![]() |
[9] |
Y. Yuan, M. Chao, Y.C. Lo, Automatic skin lesion segmentation using deep fully convolutional networks with jaccard distance, IEEE Trans. Med. Imaging, 9 (2017), 1876–1886. https://doi.org/10.1109/TMI.2017.2695227 doi: 10.1109/TMI.2017.2695227
![]() |
[10] |
P. Liskowski, K. Krawiec, Segmenting retinal blood vessels with deep neural networks, IEEE Trans. Med. Imaging, 11 (2016), 2369–2380. https://doi.org/10.1109/TMI.2016.2546227 doi: 10.1109/TMI.2016.2546227
![]() |
[11] |
H. Fu, J. Cheng, Y. Xu, D. W. K. Wong, J. Liu, X. Cao, Joint optic disc and cup segmentation based on multi-label deep network and polar transformation, IEEE Trans. Med. Imaging, 7 (2018), 1597–1605. https://doi.org/10.1109/TMI.2018.2791488 doi: 10.1109/TMI.2018.2791488
![]() |
[12] |
H. Fu, Y. Xu, S. Lin, X. Zhang, D. W. K. Wong, J. Liu, et al., Segmentation and quantification for angle-closure glaucoma assessment in anterior segment OCT, IEEE Trans. Med. Imaging, 9 (2017), 1930–1938. https://doi.org/10.1109/TMI.2017.2703147 doi: 10.1109/TMI.2017.2703147
![]() |
[13] |
M. Anthimopoulos, S. Christodoulidis, L. Ebner, A. Christe, S. Mougiakakou, Lung pattern classification for interstitial lung diseases using a deep convolutional neural network, IEEE Trans. Med. Imaging, 5 (2016), 1207–1216. https://doi.org/10.1109/TMI.2016.2535865 doi: 10.1109/TMI.2016.2535865
![]() |
[14] |
J. M. Wolterink, T. Leiner, M. A. Viergever, I. Išgum, Generative adversarial networks for noise reduction in low-dose CT, IEEE Trans. Med. Imaging, 12 (2017), 2536–2345. https://doi.org/10.1109/TMI.2017.2708987 doi: 10.1109/TMI.2017.2708987
![]() |
[15] |
S. Albarqouni, C. Baur, F. Achilles, V. Belagiannis, S. Demirci, N. Navab, Aggnet: Deep learning from crowds for mitosis detection in breast cancer histology images, IEEE Trans. Med. Imaging, 5 (2016), 1313–1321. https://doi.org/10.1109/TMI.2016.2528120 doi: 10.1109/TMI.2016.2528120
![]() |
[16] |
X. Zhang, G. Wang, S. G. Zhao, CapsNet-COVID19: Lung CT image classification method based on CapsNet model, Math. Biosci. Eng., 19 (2022), 5055–5074. https://doi.org/10.3934/mbe.2022236 doi: 10.3934/mbe.2022236
![]() |
[17] |
M. J. Horry, S. Chakraborty, B. Pradhan, M. Fallahpoor, H. Chegeni, M. Paul, Factors determining generalization in deep learning models for scoring COVID-CT images, Math. Biosci. Eng., 18 (2021), 9264–9293. https://doi.org/10.3934/mbe.2021456 doi: 10.3934/mbe.2021456
![]() |
[18] |
A. Singh, K. K. Singh, M. Greguš, I. Izonin, CNGOD-An improved convolution neural network with grasshopper optimization for detection of COVID-19, Math. Biosci. Eng., 19 (2022), 12518–12531. https://doi.org/10.3934/mbe.2022584 doi: 10.3934/mbe.2022584
![]() |
[19] |
S. H. Wang, S. C. Satapathy, M. X. Xie, Y. D. Zhang, ELUCNN for explainable COVID-19 diagnosis, Soft Comput., 2023 (2023), 1–17. https://doi.org/10.1007/s00500-023-07813-w doi: 10.1007/s00500-023-07813-w
![]() |
[20] |
Y. Zhang, M. A. Khan, Z. Zhu, S. Wang, SNELM: SqueezeNet-guided ELM for COVID-19 recognition, Comput. Syst. Sci. Eng., 1 (2023), 13–26. https://doi.org/10.32604/csse.2023.034172 doi: 10.32604/csse.2023.034172
![]() |
[21] |
Y. Zhang, X. Zhang, W. Zhu, ANC: Attention network for COVID-19 explainable diagnosis based on convolutional block attention module, CMES COMP Model. Eng., 3 (2021), 1037–1058. https://doi.org/10.32604/cmes.2021.015807 doi: 10.32604/cmes.2021.015807
![]() |
[22] |
C. C. Lai, T. P. Shih, W. C. Ko, H. J. Tang, P. R. Hsueh, Severe acute respiratory syndrome coronavirus 2 (SARS-CoV-2) and coronavirus disease-2019 (COVID-19): The epidemic and the challenges, Int. J. Antimicrob. Ag., 3 (2020), 105924. https://doi.org/10.1016/j.ijantimicag.2020.105924 doi: 10.1016/j.ijantimicag.2020.105924
![]() |
[23] |
E. Irmak, Implementation of convolutional neural network approach for COVID-19 disease detection, Physiol. Genomics, 12 (2020), 590–601. https://doi.org/10.1152/physiolgenomics.00084.2020 doi: 10.1152/physiolgenomics.00084.2020
![]() |
[24] |
F. Xie, J. Xi, Q. Duan, Driver attribute filling for genes in interaction network via modularity subspace-based concept learning from small samples, Complexity, 2020 (2020), 1–12. https://doi.org/10.1155/2020/6643551 doi: 10.1155/2020/6643551
![]() |
[25] |
Q. Huang, F. Zhang, X. Li, A new breast tumor ultrasonography CAD system based on decision tree and BI-RADS features, World Wide Web, 21 (2018), 1491–1504. https://doi.org/10.1007/s11280-017-0522-5 doi: 10.1007/s11280-017-0522-5
![]() |
[26] |
G. Dong, Z. C. Zhang, J. Feng, X. M. Zhao, MorbidGCN: Prediction of multimorbidity with a graph convolutional network based on integration of population phenotypes and disease network, Brief Bioinform., 4 (2022). https://doi.org/10.1093/bib/bbac255 doi: 10.1093/bib/bbac255
![]() |
[27] |
S. Wang, P. Li, P. Chen, P. Phillips, G. Liu, S. Du, et al., Pathological brain detection via wavelet packet tsallis entropy and real-coded biogeography-based optimization, Fund. Inform., 4 (2017), 275–291. https://doi.org/10.3233/FI-2017-1492 doi: 10.3233/FI-2017-1492
![]() |
[28] |
L. V. Fulton, D. Dolezel, J. Harrop, Y. Yan, C. P. Fulton, Classification of Alzheimer's disease with and without imagery using gradient boosted machines and ResNet-50, Brain Sci., 9 (2019), 212. https://doi.org/10.3390/brainsci9090212 doi: 10.3390/brainsci9090212
![]() |
[29] | Q. Guan, Y. Huang, Z. Zhong, Z. Zheng, L. Zheng, Y. Yang, Diagnose like a radiologist: Attention guided convolutional neural network for thorax disease classification, preprint, arXiv: 1801.09927. |
[30] | P. P. Ypsilantis, G. Montana, Learning what to look in chest X-rays with a recurrent visual attention model, preprint, arXiv: 1701.06452. |
[31] |
E. Pesce, S. J. Withey, P. P. Ypsilantis, R. Bakewell, V. Goh, G. Montana, Learning to detect chest radiographs containing pulmonary lesions using visual attention networks, Med. Image Anal., 53 (2019), 26–38. https://doi.org/10.1016/j.media.2018.12.007 doi: 10.1016/j.media.2018.12.007
![]() |
[32] |
M. Toğaçar, B. Ergen, Z. Cömert, COVID-19 detection using deep learning models to exploit Social Mimic Optimization and structured chest X-ray images using fuzzy color and stacking approaches, Comput. Biol. Med., 121 (2020), 103805.https://doi.org/10.1016/j.compbiomed.2020.103805 doi: 10.1016/j.compbiomed.2020.103805
![]() |
[33] |
J. P. Cohen, L. Dao, K. Roth, P. Morrison, Y. Bengio, A. F. Abbasi, et al., Predicting covid-19 pneumonia severity on chest x-ray with deep learning, Cureus J. Med. Sci., 12 (2020), e9448. https://doi.org/10.7759/cureus.9448 doi: 10.7759/cureus.9448
![]() |
[34] |
Q. Ni, Z. Y. Sun, L. Qi, W. Chen, Y. Yang, L. Wang, et al., A deep learning approach to characterize 2019 coronavirus disease (COVID-19) pneumonia in chest CT images, Eur. Radiol., 30 (2020), 6517–6527. https://doi.org/10.1007/s00330-020-07044-9 doi: 10.1007/s00330-020-07044-9
![]() |
[35] |
H. Ko, H. Chung, W. S. Kang, K. W. Kim, Y. Shin, S. J. Kang, et al., COVID-19 pneumonia diagnosis using a simple 2D deep learning framework with a single chest CT image: model development and validation, J. Med. Int. Res., 6 (2020), e19569. https://doi.org/10.2196/19569 doi: 10.2196/19569
![]() |
[36] |
X. Wang, X. Deng, Q. Fu, Q. Zhou, J. Feng, H. Ma, et al., A weakly-supervised framework for COVID-19 classification and lesion localization from chest CT, IEEE Trans. Med. Imaging, 8 (2020), 2615–2625. https://doi.org/10.1109/TMI.2020.2995965 doi: 10.1109/TMI.2020.2995965
![]() |
[37] |
A. I. Khan, J. L. Shah, M. M. Bhat, CoroNet: A deep neural network for detection and diagnosis of COVID-19 from chest x-ray images, Comput. Method Prog. Bio., 196 (2020), 105581. https://doi.org/10.1016/j.cmpb.2020.105581 doi: 10.1016/j.cmpb.2020.105581
![]() |
[38] |
L. Hussain, T. Nguyen, H. Li, A. A. Abbasi, K. J. Lone, Z. Zhao, et al., Machine-learning classification of texture features of portable chest X-ray accurately classifies COVID-19 lung infection, Biomed. Eng. Online, 19 (2020), 1–18. https://doi.org/10.1186/s12938-020-00831-x doi: 10.1186/s12938-020-00831-x
![]() |
[39] | J. Zhao, Y. Zhang, X. He, P. Xie, Covid-ct-dataset: a ct scan dataset about covid-19, 2020. |
[40] | COVID-19 CT segmentation dataset. Available from: https://medicalsegmentation.com/covid19/. |
[41] |
D. P. Fan, T. Zhou, G. P. Ji, Y. Zhou, G. Chen, H. Fu, et al., Inf-net: Automatic covid-19 lung infection segmentation from ct images, IEEE Trans. Med. Imaging, 8 (2020), 2626–2637. https://doi.org/10.1109/TMI.2020.2996645 doi: 10.1109/TMI.2020.2996645
![]() |
[42] |
S. H. Wang, V. V. Govindaraj, J. M. Górriz, X. Zhang, Y. D. Zhang, Covid-19 classification by FGCNet with deep feature fusion from graph convolutional network and convolutional neural network, Inform. Fusion, 67 (2021), 208–229. https://doi.org/10.1016/j.inffus.2020.10.004 doi: 10.1016/j.inffus.2020.10.004
![]() |
[43] |
R. R. Selvaraju, M. Cogswell, A. Das, R. Vedantam, D. Parikh, D. Batra, et al., Grad-cam: Visual explanations from deep networks via gradient-based localization, Int. J. Comput. Vision, 2 (2020), 336–359. https://doi.org/10.1007/s11263-019-01228-7 doi: 10.1007/s11263-019-01228-7
![]() |
[44] | A. Chattopadhay, A. Sarkar, P. Howlader, V. N. Balasubramanian, Grad-cam++: Generalized gradient-based visual explanations for deep convolutional networks, in 2018 IEEE winter conference on applications of computer vision (WACV), (2018), 839–847. https://doi.org/10.1109/WACV.2018.00097 |
[45] |
D. Chicco, N. Tötsch, G. Jurman, The Matthews correlation coefficient (MCC) is more reliable than balanced accuracy, bookmaker informedness, and markedness in two-class confusion matrix evaluation, Biodata Min., 1 (2021), 1–22. https://doi.org/10.1186/s13040-021-00244-z doi: 10.1186/s13040-021-00244-z
![]() |
[46] |
Q. Huang, Y. Lei, W. Xing, C. He, G. Wei, Z. Miao, et al., Evaluation of pulmonary edema using ultrasound imaging in patients with COVID-19 pneumonia based on a non-local Channel attention ResNet, Ultrasound Med. Biol., 5 (2022), 945–953. https://doi.org/10.1016/j.ultrasmedbio.2022.01.023 doi: 10.1016/j.ultrasmedbio.2022.01.023
![]() |
[47] |
F. J. P. Montalbo, Diagnosing Covid-19 chest x-rays with a lightweight truncated DenseNet with partial layer freezing and feature fusion, Biomed. Signal Process., 68 (2021), 102583. https://doi.org/10.1016/j.bspc.2021.102583 doi: 10.1016/j.bspc.2021.102583
![]() |
[48] |
R. Mastouri, N. Khlifa, H. Neji, S. Hantous-Zannad, A bilinear convolutional neural network for lung nodules classification on CT images, Int. J. Comput. Ass Rad, 16 (2021), 91–101. https://doi.org/10.1007/s11548-020-02283-z doi: 10.1007/s11548-020-02283-z
![]() |
[49] |
A. Garg, S. Salehi, M. La Rocca, R. Garner, D. Duncan, Efficient and visualizable convolutional neural networks for COVID-19 classification using Chest CT, Expert Syst. Appl., 195 (2022), 116540. https://doi.org/10.1016/j.eswa.2022.116540 doi: 10.1016/j.eswa.2022.116540
![]() |
[50] | F. Chollet, Xception: Deep learning with depthwise separable convolutions, in Proceedings of the IEEE conference on computer vision and pattern recognition (CVPR), (2017), 1251–1258. https://doi.org/10.48550/arXiv.1610.02357 |
[51] |
H. Panwar, P. Gupta, M. K. Siddiqui, R. Morales-Menendez, P. Bhardwaj, V. Singh, A deep learning and grad-CAM based color visualization approach for fast detection of COVID-19 cases using chest X-ray and CT-Scan images, Chaos Soliton Fract., 140 (2020), 110190. https://doi.org/10.1016/j.chaos.2020.110190 doi: 10.1016/j.chaos.2020.110190
![]() |
1. | Davood Nourabadi, Tourandokht Baluchnejadmojarad, Seyed M. M. Zarch, Samira Ramazi, Morteza N. Serenjeh, Mehrdad Roghani, Fetal Hypothyroidism Impairs Aortic Vasorelaxation Responses in Adulthood: Involvement of Hydrogen Sulfide and Nitric Oxide Cross talk, 2021, 77, 0160-2446, 238, 10.1097/FJC.0000000000000948 | |
2. | Tamás Gáll, Dávid Pethő, Annamária Nagy, György Balla, József Balla, Therapeutic Potential of Carbon Monoxide (CO) and Hydrogen Sulfide (H2S) in Hemolytic and Hemorrhagic Vascular Disorders—Interaction between the Heme Oxygenase and H2S-Producing Systems, 2020, 22, 1422-0067, 47, 10.3390/ijms22010047 | |
3. | Zeinab Hamidizad, Mehri Kadkhodaee, Seyed Morteza Karimian, Mina Ranjbaran, Fatemeh Heidari, Enayatollah Bakhshi, Farzaneh Kianian, Elham Zahedi, Behjat Seifi, Therapeutic effects of CORM3 and NaHS in chronic kidney disease induced cognitive impairment via the interaction between carbon monoxide and hydrogen sulfide on Nrf2/HO-1 signaling pathway in rats, 2022, 368, 00092797, 110217, 10.1016/j.cbi.2022.110217 | |
4. | Luísa Gouveia Lana, Lara Matos de Araújo, Thamara Ferreira Silva, Luzia Valentina Modolo, Interplay between gasotransmitters and potassium is a K+ey factor during plant response to abiotic stress, 2021, 169, 09819428, 322, 10.1016/j.plaphy.2021.11.023 | |
5. | Yu-Bo Shi, Lin Cheng, Yue Lyu, Ze-Jing Shi, The new perspective of gasotransmitters in cancer metastasis, 2025, 10898603, 10.1016/j.niox.2025.02.002 |