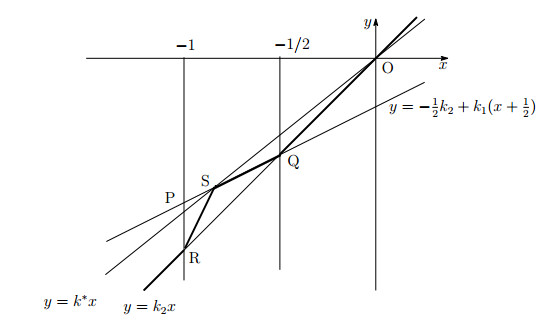
Induction motors have been widely used in industry, agriculture, transportation, national defense engineering, etc. Defects of the motors will not only cause the abnormal operation of production equipment but also cause the motor to run in a state of low energy efficiency before evolving into a fault shutdown. The former may lead to the suspension of the production process, while the latter may lead to additional energy loss. This paper studies a fuzzy rule-based expert system for this purpose and focuses on the analysis of many knowledge representation methods and reasoning techniques. The rotator fault of induction motors is analyzed and diagnosed by using this knowledge, and the diagnosis result is displayed. The simulation model can effectively simulate the broken rotator fault by changing the resistance value of the equivalent rotor winding. And the influence of the broken rotor bar fault on the motors is described, which provides a basis for the fault characteristics analysis. The simulation results show that the proposed method can realize fast fault diagnosis for rotators of induction motors.
Citation: Tangsheng Zhang, Hongying Zhi. A fuzzy set theory-based fast fault diagnosis approach for rotators of induction motors[J]. Mathematical Biosciences and Engineering, 2023, 20(5): 9268-9287. doi: 10.3934/mbe.2023406
[1] | Zhiyuan Wang, Chu Zhang, Shaopei Xue, Yinjie Luo, Jun Chen, Wei Wang, Xingchen Yan . Dynamic coordinated strategy for parking guidance in a mixed driving parking lot involving human-driven and autonomous vehicles. Electronic Research Archive, 2024, 32(1): 523-550. doi: 10.3934/era.2024026 |
[2] | Xiaoying Zheng, Jing Wu, Xiaofeng Li, Junjie Huang . UAV search coverage under priority of important targets based on multi-location domain decomposition. Electronic Research Archive, 2024, 32(4): 2491-2513. doi: 10.3934/era.2024115 |
[3] | Yu Shen, Hecheng Li . A multi-strategy genetic algorithm for solving multi-point dynamic aggregation problems with priority relationships of tasks. Electronic Research Archive, 2024, 32(1): 445-472. doi: 10.3934/era.2024022 |
[4] | Sida Lin, Lixia Meng, Jinlong Yuan, Changzhi Wu, An Li, Chongyang Liu, Jun Xie . Sequential adaptive switching time optimization technique for maximum hands-off control problems. Electronic Research Archive, 2024, 32(4): 2229-2250. doi: 10.3934/era.2024101 |
[5] | Ismail Ben Abdallah, Yassine Bouteraa, Saleh Mobayen, Omar Kahouli, Ali Aloui, Mouldi Ben Amara, Maher JEBALI . Fuzzy logic-based vehicle safety estimation using V2V communications and on-board embedded ROS-based architecture for safe traffic management system in hail city. Electronic Research Archive, 2023, 31(8): 5083-5103. doi: 10.3934/era.2023260 |
[6] | Jian Gong, Yuan Zhao, Jinde Cao, Wei Huang . Platoon-based collision-free control for connected and automated vehicles at non-signalized intersections. Electronic Research Archive, 2023, 31(4): 2149-2174. doi: 10.3934/era.2023111 |
[7] | Hao Li, Zhengwu Wang, Shuiwang Chen, Weiyao Xu, Lu Hu, Shuai Huang . Integrated optimization of planning and operation of a shared automated electric vehicle system considering the trip selection and opportunity cost. Electronic Research Archive, 2024, 32(1): 41-71. doi: 10.3934/era.2024003 |
[8] | Wenjie Wang, Suzhen Wen, Shen Gao, Pengyi Lin . A multi-objective dynamic vehicle routing optimization for fresh product distribution: A case study of Shenzhen. Electronic Research Archive, 2024, 32(4): 2897-2920. doi: 10.3934/era.2024132 |
[9] | Yineng Ouyang, Zhaotao Liang, Zhihui Ma, Lei Wang, Zhaohua Gong, Jun Xie, Kuikui Gao . A class of constrained optimal control problems arising in an immunotherapy cancer remission process. Electronic Research Archive, 2024, 32(10): 5868-5888. doi: 10.3934/era.2024271 |
[10] | Michael Barg, Amanda Mangum . Statistical analysis of numerical solutions to constrained phase separation problems. Electronic Research Archive, 2023, 31(1): 229-250. doi: 10.3934/era.2023012 |
Induction motors have been widely used in industry, agriculture, transportation, national defense engineering, etc. Defects of the motors will not only cause the abnormal operation of production equipment but also cause the motor to run in a state of low energy efficiency before evolving into a fault shutdown. The former may lead to the suspension of the production process, while the latter may lead to additional energy loss. This paper studies a fuzzy rule-based expert system for this purpose and focuses on the analysis of many knowledge representation methods and reasoning techniques. The rotator fault of induction motors is analyzed and diagnosed by using this knowledge, and the diagnosis result is displayed. The simulation model can effectively simulate the broken rotator fault by changing the resistance value of the equivalent rotor winding. And the influence of the broken rotor bar fault on the motors is described, which provides a basis for the fault characteristics analysis. The simulation results show that the proposed method can realize fast fault diagnosis for rotators of induction motors.
We consider the system of Hamilton-Jacobi equations
{λu1(x)+H1(Du1(x))+B1(u1(x),u2(x))=0 in Tn,λu2(x)+H2(Du2(x))+B2(u1(x),u2(x))=0 in Tn, | (1.1) |
where λ>0 is a given constant, the functions Hi:Rn→R and Bi:R2→R, with i=1,2, are given continuous functions, and Tn denotes the n-dimensional flat torus Rn/Zn.
In a recent paper [6], the authors have investigated the vanishing discount problem for a nonlinear monotone system of Hamilton-Jacobi equations
{λu1(x)+G1(x,Du1(x),u1(x),u2(x),…,um(x))=0 in Tn,λu1(x)+G1(x,Du1(x),u1(x),u2(x)⋮λum(x)+Gm(x,Dum(x),u1(x),u2(x),…,um(x))=0 in Tn, | (1.2) |
and established under some hypotheses on the Gi∈C(Tn×Rn×Rm) that, when uλ=(uλ,1,…,uλ,m)∈C(Tn)m denoting the (viscosity) solution of (1.2), the whole family {uλ}λ>0 converges in C(Tn)m to some u0∈C(Tn)m as λ→0+. The constant λ>0 in the above system is the so-called discount factor.
The hypotheses on the system are the convexity, coercivity, and monotonicity of the Gi as well as the solvability of (1.2), with λ=0. Here the convexity of Gi is meant that the functions Rn×Rm∋(p,u)↦Gi(x,p,u) are convex. We refer to [6] for the precise statement of the hypotheses.
Prior to work [6], there have been many contributions to the question about the whole family convergence (in other words, the full convergence) under the vanishing discount, which we refer to [1,3,4,6,8,9,10] and the references therein.
In the case of the scalar equation, B. Ziliotto [11] has recently shown an example of the Hamilton-Jacobi equation having non-convex Hamiltonian in the gradient variable for which the full convergence does not hold. In Ziliotto's approach, the first step is to find a system of two algebraic equations
{λu+f(u−v)=0,λv+g(v−u)=0, | (1.3) |
with two unknowns u,v∈R and with a parameter λ>0 as the discount factor, for which the solutions (uλ,vλ) stay bounded and fail to fully converge as λ→0+. Here, an "algebraic" equation is meant not to be a functional equation. The second step is to interpolate the two values uλ and vλ to get a function of x∈T1 which satisfies a scalar non-convex Hamilton-Jacobi equation in T1.
In the first step above, Ziliotto constructs f,g based on a game-theoretical and computational argument, and the formula for f,g is of the minimax type and not quite explicit. In [5], the author has reexamined the system given by Ziliotto, with a slight generality, as a counterexample for the full convergence in the vanishing discount.
Our purpose in this paper is to present a system (1.3), with an explicit formula for f,g, for which the solution (uλ,vλ) does not fully converge to a single point in R2. A straightforward consequence is that (1.1), with B1(u1,u2)=f(u1−u2) and B2(u1,u2)=g(u2−u1), has a solution given by
(uλ,1(x),uλ.2(x))=(uλ,vλ) for x∈Tn, |
under the assumption that Hi(x,0)=0 for all x∈Tn, and therefore, gives an example of a discounted system of Hamilton-Jacobi equations, the solution of which fails to satisfy the full convergence as the discount factor goes to zero.
The paper consists of two sections. This introduction is followed by Section 2, the final section, which is divided into three subsections. The main results are stated in the first subsection of Section 2, the functions f,g, the key elements of (1.3), are contstructed in the second subsection, and the final subsection provides the proof of the main results.
Our main focus is now the system
{λu+f(u−v)=0λv+g(v−u)=0, | (2.1) |
where f,g∈C(R,R) are nondecreasing functions, to be constructed, and λ>0 is a constant, to be sent to zero. Notice that (2.1) above is referred as (1.3) in the previous section.
We remark that, due to the monotonicity assumption on f,g, the mapping (u,v)↦(f(u−v),g(v−u)),R2→R2 is monotone. Recall that, by definition, a mapping (u,v)↦(B1(u,v),B2(u,v)),R2→R2 is monotone if, whenever (u1,v1),(u2,v2)∈R2 satisfy u1−u2≥v1−v2 (resp., v1−v2≥u1−u2), we have B1(u1,v1)≥B1(u2,v2) (resp., B2(u1,v1)≥B2(u2,v2)).
Our main results are stated as follows.
Theorem 1. There exist two increasing functions f,g∈C(R,R) having the properties (a)–(c):
(a) For any λ>0 there exists a unique solution (uλ,vλ)∈R2 to (2.1),
(b) the family of the solutions (uλ,vλ) to (2.1), with λ>0, is bounded in R2,
(c) the family {(uλ,vλ)}λ>0 does not converge as λ→0+.
It should be noted that, as mentioned in the introduction, the above theorem has been somewhat implicitly established by Ziliotto [11]. In this note, we are interested in a simple and easy approach to finding functions f,g having the properties (a)–(c) in Theorem 1.
The following is an immediate consequence of the above theorem.
Corollary 2. Let Hi∈C(Rn,R), i=1,2, satisfy H1(0)=H2(0)=0. Let f,g∈C(R,R) be the functions given by Theorem 1, and set B1(u1,u2)=f(u1−u2) and B2(u1,u2)=g(u2−u1) for all (u1,u2)∈R2. For any λ>0, let (uλ,1,uλ,2) be the (viscosity) solution of (1.1). Then, the functions uλ,i are constants, the family of the points (uλ,1,uλ,2) in R2 is bounded, and it does not converge as λ→0+.
Notice that the convexity of Hi in the above corollary is irrelevant, and, for example, one may take Hi(p)=|p|2 for i∈I, which are convex functions.
We remark that a claim similar to Corollary 2 is valid when one replaces Hi(p) by degenerate elliptic operators Fi(x,p,M) as far as Fi(x,0,0)=0, where M is the variable corresponding to the Hessian matrices of unknown functions. (See [2] for an overview on the viscosity solution approach to fully nonlinear degenerate elliptic equations.)
If f,g are given and (u,v)∈R2 is a solution of (2.1), then w:=u−v satisfies
λw+f(w)−g(−w)=0. | (2.2) |
Set
h(r)=f(r)−g(−r) for r∈R, | (2.3) |
which defines a continuous and nondecreasing function on R.
To build a triple of functions f,g,h, we need to find two of them in view of the relation (2.3). We begin by defining function h.
For this, we discuss a simple geometry on xy-plane as depicted in Figure 1 below. Fix 0<k1<k2. The line y=−12k2+k1(x+12) has slope k1 and crosses the lines x=−1 and y=k2x at P:=(−1,−12(k1+k2)) and Q:=(−12,−12k2), respectively, while the line y=k2x meets the lines x=−1 and x=−12 at R:=(−1,−k2) and Q=(−12,−12k2), respectively.
Choose k∗>0 so that 12(k1+k2)<k∗<k2. The line y=k∗x crosses the line y=−12k2+k1(x+12) at a point S:=(x∗,y∗) in the open line segment between the points P=(−12,−12(k1+k2)) and Q=(−12,−12k2). The line connecting R=(−1,−k2) and S=(x∗,y∗) can be represented by y=−k2+k+(x+1), with k+:=y∗+k2x∗+1>k2.
We set
ψ(x)={k2x for x∈(−∞,−1]∪[−1/2,∞),min{−k2+k+(x+1),−12k2+k1(x+12)} for x∈(−1,−12). |
It is clear that ψ∈C(R) and increasing on R. The building blocks of the graph y=ψ(x) are three lines whose slopes are k1<k2<k+. Hence, if x1>x2, then ψ(x1)−ψ(x2)≥k1(x1−x2), that is, the function x↦ψ(x)−k1x is nondecreasing on R.
Next, we set for j∈N,
ψj(x)=2−jψ(2jx) for x∈R. |
It is clear that for all j∈N, ψj∈C(R), the function x↦ψj(x)−k1x is nondecreasing on R, and
ψj(x){>k2x for all x∈(−2−j,−2−j−1),=k2x otherwise. |
We set
η(x)=maxj∈Nψj(x) for x∈R. |
It is clear that η∈C(R) and x↦η(x)−k1x is nondecreasing on R. Moreover, we see that
η(x)=k2x for all x∈(−∞,−12]∪[0,∞), |
and that if −2−j<x<−2−j−1 and j∈N,
η(x)=ψj(x)>k2x. |
Note that the point S=(x∗,y∗) is on the graph y=ψ(x) and, hence, that for any j∈N, the point (2−jx∗,2−jy∗) is on the graph y=η(x). Similarly, since the point S=(x∗,y∗) is on the graph y=k∗x and for any j∈N, the point (2−jx∗,2−jy∗) is on the graph y=k∗x. Also, for any j∈N, the point (−2−j,−k22−j) lies on the graphs y=η(x) and y=k2x.
Fix any d≥1 and define h∈C(R) by
h(x)=η(x−d). |
For the function h defined above, we consider the problem
λz+h(z)=0. | (2.4) |
Lemma 3. For any λ≥0, there exists a unique solution zλ∈R of (2.4).
Proof. Fix λ≥0. The function x↦h(x)+λx is increasing on R and satisfies
limx→∞(h(x)+λx)=∞ and limx→−∞(h(x)+λx)=−∞. |
Hence, there is a unique solution of (2.4).
For any λ≥0, we denote by zλ the unique solution of (2.4). Since h(d)=0, it is clear that z0=d.
For later use, observe that if λ>0, k>0, and (z,w)∈R2 is the point of the intersection of two lines y=−λx and y=k(x−d), then w=−λz=k(z−d) and
z=kdk+λ. | (2.5) |
Lemma 4. There are sequences {μj} and {νj} of positive numbers converging to zero such that
zμj=k2dk2+μj and zνj=k∗dk∗+νj. |
Proof. Let j∈N. Since (−2−j,−k22−j) is on the intersection of the graphs y=k2x and y=η(x), it follows that (−2−j+d,−k22−j) is on the intersection of the graphs y=k2(x−d) and y=h(x). Set
μj=k22−jd−2−j, | (2.6) |
and note that μj>0 and that
−μj(d−2−j)=−k22−j, |
which says that the point (d−2−j,−k22−j) is on the line y=−μjx. Combining the above with
−k22−j=h(d−2−j) |
shows that d−2−j is the unique solution of (2.4). Also, since (d−2−j,−μj(d−2−j))=(d−2−j,−k22−j) is on the line y=k2(x−d), we find by (2.5) that
zμj=k2dk2+μj. |
Similarly, since (2−jx∗,2−jy∗) is on the intersection of the graphs y=k∗x and y=η(x), we deduce that if we set
νj:=−2−jy∗d+2−jx∗=2−j|y∗|d−2−j|x∗|, | (2.7) |
then
zνj=k∗dk∗+νj. |
It is obvious by (2.6) and (2.7) that the sequences {μj}j∈N and {νj}j∈N are decreasing and converge to zero.
We fix k0∈(0,k1) and define f,g∈C(R) by f(x)=k0(x−d) and
g(x)=f(−x)−h(−x). |
It is easily checked that g(x)−(k1−k0)x is nondecreasing on R, which implies that g is increasing on R, and that h(x)=f(x)−g(−x) for all x∈R. We note that
f(d)=h(d)=g(−d)=0. | (2.8) |
We fix f,g,h as above, and consider the system (2.1).
Lemma 5. Let λ>0. There exists a unique solution of (2.1).
The validity of the above lemma is well-known, but for the reader's convenience, we provide a proof of the lemma above.
Proof. By choice of f,g, the functions f,g are nondecreasing on R. We show first the comparison claim: if (u1,v1),(u2,v2)∈R2 satisfy
λu1+f(u1−v1)≤0,λv1+g(v1−u1)≤0, | (2.9) |
λu2+f(u2−v2)≥0,λv2+g(v2−u2)≥0, | (2.10) |
then u1≤u2 and v1≤v2. Indeed, contrary to this, we suppose that max{u1−u2,v1−v2}>0. For instance, if max{u1−u2,v1−v2}=u1−u2, then we have u1−v1≥u2−v2 and u1>u2, and moreover
0≥λu1+f(u1−v1)≥λu1+f(u2−v2)>λu2+f(u2−v2), |
yielding a contradiction. The other case when max{u1−u2,v1−v2}=v1−v2, we find a contradiction, 0>λv2+g(v2−u2), proving the comparison.
From the comparison claim, the uniqueness of the solutions of (2.1) follows readily.
Next, we may choose a constant C>0 so large that (u1,v1)=(−C,−C) and (u2,v2)=(C,C) satisfy (2.9) and (2.10), respectively. We write S for the set of all (u1,u2)∈R2 such that (2.9) hold. Note that (−C,−C)∈S and that for any (u,v)∈S, u≤C and v≤C. We set
u∗=sup{u:(u,v)∈S for some v},v∗=sup{v:(u,v)∈S for some u}. |
It follows that −C≤u∗,v∗≤C. We can choose sequences
{(u1n,v1n)}n∈N,{(u2n,v2n)}n∈N⊂S |
such that {u1n},{v2n} are nondecreasing,
limn→∞u1n=u∗ and limn→∞v2n=v∗. |
Observe that for all n∈N, u2n≤u∗, v1n≤v∗, and
0≥λu1n+f(u1n−v1n)≥λu1n+f(u1n−v∗), |
which yields, in the limit as n→∞,
0≥λu∗+f(u∗−v∗). |
Similarly, we obtain 0≥λv∗+g(v∗−u∗). Hence, we find that (u∗,v∗)∈S.
We claim that (u∗,v∗) is a solution of (2.1). Otherwise, we have
0>λu∗+f(u∗−v∗) or 0>λv∗+g(v∗−u∗). |
For instance, if the former of the above inequalities holds, we can choose ε>0, by the continuity of f, so that
0>λ(u∗+ε)+f(u∗+ε−v∗). |
Since (u∗,v∗)∈S, we have
0≥λv∗+g(v∗−u∗)≥λv∗+g(v∗−u∗−ε). |
Accordingly, we find that (u∗+ε,v∗)∈S, which contradicts the definition of u∗. Similarly, if 0>λv∗+g(v∗−u∗), then we can choose δ>0 so that (u∗,v∗+δ)∈S, which is a contradiction. Thus, we conclude that (u∗,v∗) is a solution of (2.1).
Theorem 6. For any λ>0, let (uλ,vλ) denote the unique solution of (2.1). Let {μj},{νj} be the sequences of positive numbers from Lemma 2.4. Then
limj→∞uμj=k0dk2 and limj→∞uνj=k0dk∗. |
In particular,
lim infλ→0uλ≤k0dk2<k0dk∗≤lim supλ→0uλ. |
With our choice of f,g, the family of solutions (uλ,vλ) of (2.1), with λ>0, does not converge as λ→0.
Proof. If we set zλ=uλ−vλ, then zλ satisfies (2.4). By Lemma 4, we find that
zμj=k2dk2+μj and zνj=k∗dk∗+νj. |
Since uλ satisfies
0=λuλ+f(zλ)=λuλ+k0(zλ−d), |
we find that
uμj=−k0(zμj−d)μj=−k0dμj(k2k2+μj−1)=−k0dμj−μjk2+μj=k0dk2+μj, |
which shows that
limj→∞uμj=k0dk2. |
A parallel computation shows that
limj→∞uνj=k0dk∗. |
Recalling that 0<k∗<k2, we conclude that
lim infλ→0uλ≤k0dk2<k0dk∗≤lim supλ→0uλ. |
We remark that, since
limλ→0zλ=d and vλ=uλ−zλ, |
limj→∞vμj=k0dk2−d and limj→∞vνj=k0dk∗−d. |
We give the proof of Theorem 1.
Proof of Theorem 1. Assertions (a) and (c) are consequences of Lemma 5 and Theorem 6, respectively.
Recall (2.8). That is, we have f(d)=h(d)=g(−d)=0. Setting (u2,v2)=(d,0), we compute that for any λ>0,
λu2+f(u2−v2)>f(d)=0 and λv2+g(v2−u2)=g(−d)=0. |
By the comparison claim, proved in the proof of Lemma 5, we find that uλ≤d and vλ≤0 for any λ>0. Similarly, setting (u1,v1)=(0,−d), we find that for any λ>0,
λu1+f(u1−v1)=f(d)=0 and λv1+g(v1−u1)≤g(v1−u1)=g(−d)=0, |
which shows by the comparison claim that uλ≥0 and vλ≥−d for any λ>0. Thus, the sequence {(uλ,vλ)}λ>0 is bounded in R2, which proves assertion (b).
Proof of Corollary 2. For any λ>0, let (uλ,vλ)∈R2 be the unique solution of (2.1). Since H1(0)=H2(0)=0, it is clear that the constant function (uλ,1(x),uλ,2(x)):=(uλ,vλ) is a classical solution of (1.1). By a classical uniqueness result (see, for instance, [7,Theorem 4.7]), (uλ,1,uλ,2) is a unique viscosity solution of (1.1). The rest of the claims in Corollary 2 is an immediate consequence of Theorem 1.
Some remarks are in order. (ⅰ) Following [11], we may use Theorem 6 as the primary cornerstone for building a scalar Hamilton-Jacobi equation, for which the vanishing discount problem fails to have the full convergence as the discount factor goes to zero.
(ⅱ) In the construction of the functions f,g∈C(R,R) in Theorem 6, the author has chosen d to satisfy d≥1, but, in fact, one may choose any d>0. In the proof, the core step is to find the function h(x)=f(x)−g(−x), with the properties: (a) the function x↦h(x)−εx is nondecreasing on R for some ε>0 and (b) the curve y=h(x), with x<d, meets the lines y=p(x−d) and y=q(x−d), respectively, at Pj and Qj for all j∈N, where p,q,d are positive constants such that ε<p<q, and the sequences {Pj}j∈N,{Qj}j∈N converge to the point (d,0). Obviously, such a function h is never left-differentiable at x=d nor convex in any neighborhood of x=d. Because of this, it seems difficult to select f,g∈C(R,R) in Theorem 1, both smooth everywhere. In the proof of Theorem 6, we have chosen ε=k0, p=k∗, q=k2, Pj=(uνj,k∗(uνj−d)), and Qj=(uμj,k2(uμj−d))
Another possible choice of h among many other ways is the following. Define first η:R→R by η(x)=x(sin(log|x|)+2) if x≠0, and η(0)=0 (see Figure 2). Fix d>0 and set h(x)=η(x−d) for x∈R. we remark that η∈C∞(R∖{0}) and h∈C∞(R∖{d}). Note that if x≠0,
η′(x)=sin(log|x|)+cos(log|x|)+2∈[2−√2,2+√2], |
and that if we set xj=−exp(−2πj) and ξj=−exp(−2πj+π2), j∈N, then
η(xj)=2xj and η(ξj)=3ξj. |
The points Pj:=(xj+d,2xj) are on the intersection of two curves y=h(x) and y=2(x−d), while the points Qj:=(d+ξj,3ξj) are on the intersection of y=h(x) and y=3(x−d). Moreover, limPj=limQj=(d,0).
The author would like to thank the anonymous referees for their careful reading and useful suggestions. He was supported in part by the JSPS Grants KAKENHI No. 16H03948, No. 20K03688, No. 20H01817, and No. 21H00717.
The author declares no conflict of interest.
[1] |
Z. Guo, K. Yu, N. Kumar, W. Wei, S. Mumtaz, M. Guizani, Deep distributed learning-based POI recommendation under mobile edge networks, IEEE Internet Things J., 10 (2022), 303–317. https://doi.org/10.1109/JIOT.2022.3202628 doi: 10.1109/JIOT.2022.3202628
![]() |
[2] |
Y. Li, H. Ma, L. Wang, S. Mao, G. Wang, Optimized content caching and user association for edge computing in densely deployed heterogeneous networks, IEEE Trans. Mob. Comput., 21 (2020), 2130–2142. https://doi.org/10.1109/TMC.2020.3033563 doi: 10.1109/TMC.2020.3033563
![]() |
[3] |
Z. Guo, K. Yu, A. K. Bashir, D. Zhang, Y. D. Al-Otaibi, M. Guizani, Deep information fusion-driven POI scheduling for mobile social networks, IEEE Network, 36 (2022), 210–216. https://doi.org/10.1109/MNET.102.2100394 doi: 10.1109/MNET.102.2100394
![]() |
[4] |
L. Yang, Y. Li, S. X. Yang, Y. Lu, T. Guo, K. Yu, Generative adversarial learning for intelligent trust management in 6G wireless networks, IEEE Network, 36 (2022), 134–140. https://doi.org/10.1109/MNET.003.2100672 doi: 10.1109/MNET.003.2100672
![]() |
[5] |
Q. Zhang, K. Yu, Z. Guo, S. Garg, J. J. P. C. Rodrigues, M. M. Hassan, et al., Graph neural networks-driven traffic forecasting for connected internet of vehicles, IEEE Trans. Network Sci. Eng., 9 (2022), 3015–3027. https://doi.org/10.1109/TNSE.2021.3126830 doi: 10.1109/TNSE.2021.3126830
![]() |
[6] |
L. Zhao, Z. Bi, A. Hawbani, K. Yu, Y. Zhang, M. Guizani, ELITE: An intelligent digital twin-based hierarchical routing scheme for softwarized vehicular networks, IEEE Trans. Mob. Comput., 2022 (2022). https://doi.org/10.1109/TMC.2022.3179254 doi: 10.1109/TMC.2022.3179254
![]() |
[7] |
Y. Zhu, W. Zheng, Observer-based control for cyber-physical systems with DoS attacks via a cyclic switching strategy, IEEE Trans. Autom. Control, 65 (2019), 3714–3721. https://doi.org/10.1109/TAC.2019.2953210 doi: 10.1109/TAC.2019.2953210
![]() |
[8] |
L. Zhao, Z. Yin, K. Yu, X. Tang, L. Xu, Z. Guo, et al., A fuzzy logic based intelligent multi-attribute routing scheme for two-layered SDVNs, IEEE Trans. Netw. Serv. Manage., 2022 (2022). https://doi.org/10.1109/TNSM.2022.3202741 doi: 10.1109/TNSM.2022.3202741
![]() |
[9] |
L. Chen, Y. Zhu, C. K. Ahn, Adaptive neural network-based observer design for switched systems with quantized measurements, IEEE Trans. Neural Networks Learn. Syst., 2021 (2021), 1–14. https://doi.org/10.1109/TNNLS.2021.3131412 doi: 10.1109/TNNLS.2021.3131412
![]() |
[10] |
J. Zhang, Q. Yan, X. Zhu, K. Yu, Smart industrial IoT empowered crowd sensing for safety monitoring in coal mine, Digital Commun. Networks, 2022 (2022). https://doi.org/10.1016/j.dcan.2022.08.002 doi: 10.1016/j.dcan.2022.08.002
![]() |
[11] |
Z. Cai, X. Zheng, A private and efficient mechanism for data uploading in smart cyber-physical systems, IEEE Trans. Network Sci. Eng., 7 (2020), 766–775. https://doi.org/10.1109/TNSE.2018.2830307 doi: 10.1109/TNSE.2018.2830307
![]() |
[12] |
Z. Guo, K. Yu, A. Jolfaei, F. Ding, N. Zhang, Fuz-Spam: Label smoothing-based fuzzy detection of spammers in internet of things, IEEE Trans. Fuzzy Syst., 30 (2022), 4543–4554. https://doi.org/10.1109/TFUZZ.2021.3130311 doi: 10.1109/TFUZZ.2021.3130311
![]() |
[13] |
Z. Cai, X. Zheng, J. Yu, A differential-private framework for urban traffic flows estimation via taxi companies, IEEE Trans. Ind. Inf., 15 (2019), 6492–6499. https://doi.org/10.1109/TⅡ.2019.2911697 doi: 10.1109/TⅡ.2019.2911697
![]() |
[14] |
Z. Zhou, Y. Li, J. Li, K. Yu, G. Kou, M. Wang, et al., Gan-siamese network for cross-domain vehicle re-identification in intelligent transport systems, IEEE Trans. Network Sci. Eng., 2022 (2022), 1–12. https://doi.org/10.1109/TNSE.2022.3199919 doi: 10.1109/TNSE.2022.3199919
![]() |
[15] |
Z. Zhou, Y. Su, J. Li, K. Yu, Q. J. Wu, Z. Fu, et al., Secret-to-image reversible transformation for generative steganography, IEEE Trans. Dependable Secure Comput., 2022 (2022), 1–17. https://doi.org/10.1109/TDSC.2022.3217661 doi: 10.1109/TDSC.2022.3217661
![]() |
[16] |
S. Xia, Z. Yao, Y. Li, S. Mao, Online distributed offloading and computing resource management with energy harvesting for heterogeneous MEC-enabled IoT, IEEE Trans. Wireless Commun., 20 (2021), 6743–6757. https://doi.org/10.1109/TWC.2021.3076201 doi: 10.1109/TWC.2021.3076201
![]() |
[17] |
Z. Guo, Y. Shen, S. Wan, W. Shang, K. Yu, Hybrid intelligence-driven medical image recognition for remote patient diagnosis in internet of medical things, IEEE J. Biomed. Health Inf., 26 (2022), 5817–5828. https://doi.org/10.1109/JBHI.2021.3139541 doi: 10.1109/JBHI.2021.3139541
![]() |
[18] |
Z. Cai, Q. Chen, Latency-and-coverage aware data aggregation scheduling for multihop battery-free wireless networks, IEEE Trans. Wireless Commun., 20 (2021), 1770–1784. https://doi.org/10.1109/TWC.2020.3036408 doi: 10.1109/TWC.2020.3036408
![]() |
[19] |
C. Chen, Z. Liao, Y. Ju, C. He, K. Yu, S. Wan, Hierarchical domain-based multi-controller deployment strategy in SDN-enabled space-air-ground integrated network, IEEE Trans. Aerosp. Electron. Syst., 58 (2022), 4864–4879. https://doi.org/10.1109/TAES.2022.3199191 doi: 10.1109/TAES.2022.3199191
![]() |
[20] |
H. Jafari, J. Poshtan, Fault detection and isolation based on fuzzy‐integral fusion approach, IET Sci. Meas. Technol., 13 (2019), 296–302. https://doi.org/10.1049/iet-smt.2018.5005 doi: 10.1049/iet-smt.2018.5005
![]() |
[21] |
Y. Lu, L. Yang, S. X. Yang, Q. Hua, A. K. Sangaiah, T. Guo, et al., An intelligent deterministic scheduling method for ultralow latency communication in edge enabled industrial internet of things, IEEE Trans. Ind. Inf., 19 (2022), 1756–1767. https://doi.org/10.1109/TⅡ.2022.3186891 doi: 10.1109/TⅡ.2022.3186891
![]() |
[22] |
S. K. Gundewar, P. V. Kane, Condition monitoring and fault diagnosis of induction motor, J. Vib. Eng. Technol., 9 (2021), 643–674. https://doi.org/10.1007/s42417-020-00253-y doi: 10.1007/s42417-020-00253-y
![]() |
[23] |
A. Choudhary, D. Goyal, S. L. Shimi, A. Akula, Condition monitoring and fault diagnosis of induction motors: A review, Arch. Comput. Methods Eng., 26 (2019), 1221–1238. https://doi.org/10.1007/s11831-018-9286-z doi: 10.1007/s11831-018-9286-z
![]() |
[24] |
D. Fan, G. P. Jiang, Y. R. Song, Y. W. Li, G. Chen, Novel epidemic models on PSO-based networks, J. Theor. Biol., 477 (2019), 36–43. https://doi.org/10.1016/j.jtbi.2019.06.006 doi: 10.1016/j.jtbi.2019.06.006
![]() |
[25] |
I. Dilmi, A. Bouguerra, A. Djrioui, L. Chrifi-Alaoui, Interval type-2 fuzzy logic-second order sliding mode based fault detection and active fault-tolerant control of brushless DC motor, J. Eur. Syst. Automatisés, 54 (2021), 475–485. https://doi.org/10.18280/jesa.540311 doi: 10.18280/jesa.540311
![]() |
[26] |
O. E. Hassan, M. Amer, A. K. Abdelsalam, B. W. Williams, Induction motor broken rotor bar fault detection techniques based on fault signature analysis–a review, IET Electr. Power Appl., 12 (2018), 895–907. https://doi.org/10.1049/iet-epa.2018.0054 doi: 10.1049/iet-epa.2018.0054
![]() |
[27] | H. MERABET, T. Bahi, K. BEDOUD, D. DRICI, A fuzzy logic based approach for the monitoringof open switch fault in a SVM voltage sourceinverter fed induction motor drive, J. Autom. Syst. Eng., 12 (2018), 48–66. |
[28] |
P. Kumar, A. S. Hati, Deep convolutional neural network based on adaptive gradient optimizer for fault detection in SCIM, ISA Trans., 111 (2021), 350–359. https://doi.org/10.1016/j.isatra.2020.10.052 doi: 10.1016/j.isatra.2020.10.052
![]() |
[29] |
D. K. Soother, J. Daudpoto, A brief review of condition monitoring techniques for the induction motor, Trans. Can. Soc. Mech. Eng., 43 (2019), 499–508. https://doi.org/10.1139/tcsme-2018-0234 doi: 10.1139/tcsme-2018-0234
![]() |
[30] |
A. Mehta, A. Choudhary, D. Goyal, B. S. Pabla, Infrared thermography based fault diagnosis and prognosis for rotating machines, J. Univ. Shanghai Sci. Technol., 23 (2021), 22–29. https://doi.org/10.1155/2021/9947300 doi: 10.1155/2021/9947300
![]() |
[31] |
S. Kavitha, N. S. Bhuvaneswari, R. Senthilkumar, N. R. Shanker, Magnetoresistance sensor-based rotor fault detection in induction motor using non-decimated wavelet and streaming data, Automatika, 63 (2022), 525–541. https://doi.org/10.1080/00051144.2022.2052533 doi: 10.1080/00051144.2022.2052533
![]() |
[32] | A. Ebrahimi, H. Ahmad, R. Roshanfekr, Stator winding short circuit fault detection in three-phase Induction Motors using combination type-2 Fuzzy logic and Support Vector Machine classifier optimized by Fractional-order Chaotic Particle Swarm optimization algorithm, Comput. Intell. Electr. Eng., 12 (2021), 37–48. |
[33] |
A. Chouhan, P. Gangsar, R. Porwal, C. K. Mechefske, Artificial neural network–based fault diagnosis for induction motors under similar, interpolated and extrapolated operating conditions, Noise Vibr. Worldwide, 52 (2021), 323–333. https://doi.org/10.1177/09574565211030709 doi: 10.1177/09574565211030709
![]() |
[34] |
C. G. Dias, C. M. de Sousa, A neuro-fuzzy approach for locating broken rotor bars in induction motors at very low slip, J. Control Autom. Electr. Syst., 29 (2018), 489–499. https://doi.org/10.1007/s40313-018-0388-5 doi: 10.1007/s40313-018-0388-5
![]() |
[35] | D. Bouneb, T. Bahi, H. MERABET, Vibration for detection and diagnosis bearing faults using adaptive neurofuzzy inference system, J. Electr. Syst., 14 (2018), 95–104. |
[36] |
Z. Zhu, Y. Lei, G. Qi, Y. Chai, N. Mazur, Y. An, et al., A review of the application of deep learning in intelligent fault diagnosis of rotating machinery, Measurement, 206 (2022), 112346. https://doi.org/10.1016/j.measurement.2022.112346 doi: 10.1016/j.measurement.2022.112346
![]() |
[37] |
X. Huang, G. Qi, N. Mazur, Y. Chai, Deep residual networks-based intelligent fault diagnosis method of planetary gearboxes in cloud environments, Simul. Modell. Pract. Theory, 116 (2022), 102469. https://doi.org/10.1016/j.simpat.2021.102469 doi: 10.1016/j.simpat.2021.102469
![]() |
[38] |
G. Qi, Z. Zhu, K. Erqinhu, Y. Chen, Y. Chai, J. Sun, Fault-diagnosis for reciprocating compressors using big data and machine learning, Simul. Modell. Pract. Theory, 80 (2018), 104–127. https://doi.org/10.1016/j.simpat.2017.10.005 doi: 10.1016/j.simpat.2017.10.005
![]() |
[39] |
X. Shen, G. Shi, H. Ren, W. Zhang, Biomimetic vision for zoom object detection based on improved vertical grid number YOLO algorithm, Front. Bioeng. Biotechnol., 10 (2022), 905583. https://doi.org/10.3389/fbioe.2022.905583 doi: 10.3389/fbioe.2022.905583
![]() |
[40] |
X. Zhang, T. Feng, Q. Niu, X. Deng, A novel swarm optimization algorithm based on a mixed-distribution model, Appl. Sci., 8 (2018), 632. https://doi.org/10.3390/app8040632 doi: 10.3390/app8040632
![]() |
[41] |
A. Glowacz, Thermographic fault diagnosis of shaft of BLDC motor, Sensors, 22 (2022), 8537. https://doi.org/10.3390/s22218537 doi: 10.3390/s22218537
![]() |
[42] |
A. Głowacz, W. Głowacz, Z. Głowacz, Recognition of armature current of DC generator depending on rotor speed using FFT, MSAF-1 and LDA, Ekspl. Niezawodność, 17 (2015), 64–69. https://doi.org/10.17531/ein.2015.1.9 doi: 10.17531/ein.2015.1.9
![]() |
[43] |
A. Glowacz, Fault diagnostics of acoustic signals of loaded synchronous motor using SMOFS-25-EXPANDED and selected classifiers, Tehnički vjesnik, 23 (2016), 1365–1372. https://doi.org/10.17559/TV-20150328135652 doi: 10.17559/TV-20150328135652
![]() |
[44] |
O. AlShorman, F. Alkahatni, M. Masadeh, M. Irfan, A. Glowacz, F. Althobiani, et al., Sounds and acoustic emission-based early fault diagnosis of induction motor: A review study, Adv. Mech. Eng., 13 (2021). https://doi.org/10.1177/1687814021996915 doi: 10.1177/1687814021996915
![]() |
[45] |
A. Glowacz, R. Tadeusiewicz, S. Legutko, W. Caesarendra, M. Irfan, H. Liu, et al., Fault diagnosis of angle grinders and electric impact drills using acoustic signals, Appl. Acoust., 179 (2021), 108070. https://doi.org/10.1016/j.apacoust.2021.108070 doi: 10.1016/j.apacoust.2021.108070
![]() |
1. | Yuandong Chen, Jinhao Pang, Yuchen Gou, Zhiming Lin, Shaofeng Zheng, Dewang Chen, Research on the A* Algorithm for Automatic Guided Vehicles in Large-Scale Maps, 2024, 14, 2076-3417, 10097, 10.3390/app142210097 |