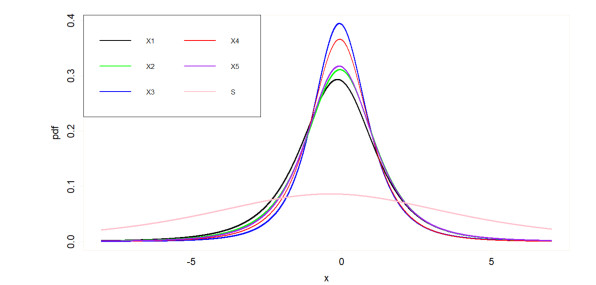
Ready-mixed-concrete (RMC) is an important green and clean building material which is widely used in modern civil engineering. For the large-scale planar foundations of urban public buildings, huge amounts of RMC need to be continuously delivered to the construction site according to strict time windows, which brings the problem of multi-plants collaborative supply. In this paper, considering transportation capacity, initial setting time, and interrupt pumping time, a collaborative scheduling model for production and transportation of RMC with the objective of minimizing the penalty cost of interruption pumping and vehicle waiting time and fuel consumption cost was established. According to the characteristics of the problem, a double chromosome synchronous evolution genetic algorithm was designed. Finally, the model and algorithm proposed in the paper were verified by data experiments. The computing results showed that in two cases of different scenarios, such as ordinary constructions and emergency constructions, the proposed scheduling model can save 18.6 and 24.8% cost respectively. The scheduling model and algorithm proposed in the paper can be applied directly to improve the operational efficiency of RMC supply chain.
Citation: Jing Yin, Ran Huang, Hao Sun, Taosheng Lin. A collaborative scheduling model for production and transportation of ready-mixed concrete[J]. Mathematical Biosciences and Engineering, 2023, 20(4): 7387-7406. doi: 10.3934/mbe.2023320
[1] | Baishuai Zuo, Chuancun Yin . Stein’s lemma for truncated generalized skew-elliptical random vectors. AIMS Mathematics, 2020, 5(4): 3423-3433. doi: 10.3934/math.2020221 |
[2] | Guangshuai Zhou, Chuancun Yin . Family of extended mean mixtures of multivariate normal distributions: Properties, inference and applications. AIMS Mathematics, 2022, 7(7): 12390-12414. doi: 10.3934/math.2022688 |
[3] | Remigijus Leipus, Jonas Šiaulys, Dimitrios Konstantinides . Minimum of heavy-tailed random variables is not heavy tailed. AIMS Mathematics, 2023, 8(6): 13066-13072. doi: 10.3934/math.2023658 |
[4] | Naif Alotaibi, A. S. Al-Moisheer, Ibrahim Elbatal, Salem A. Alyami, Ahmed M. Gemeay, Ehab M. Almetwally . Bivariate step-stress accelerated life test for a new three-parameter model under progressive censored schemes with application in medical. AIMS Mathematics, 2024, 9(2): 3521-3558. doi: 10.3934/math.2024173 |
[5] | Huifang Yuan, Tao Jiang, Min Xiao . The ruin probability of a discrete risk model with unilateral linear dependent claims. AIMS Mathematics, 2024, 9(4): 9785-9807. doi: 10.3934/math.2024479 |
[6] | Weiwei Ni, Chenghao Xu, Kaiyong Wang . Estimations for aggregate amount of claims in a risk model with arbitrary dependence between claim sizes and inter-arrival times. AIMS Mathematics, 2022, 7(10): 17737-17746. doi: 10.3934/math.2022976 |
[7] | Khaled M. Alqahtani, Mahmoud El-Morshedy, Hend S. Shahen, Mohamed S. Eliwa . A discrete extension of the Burr-Hatke distribution: Generalized hypergeometric functions, different inference techniques, simulation ranking with modeling and analysis of sustainable count data. AIMS Mathematics, 2024, 9(4): 9394-9418. doi: 10.3934/math.2024458 |
[8] | Gunduz Caginalp . Fat tails arise endogenously from supply/demand, with or without jump processes. AIMS Mathematics, 2021, 6(5): 4811-4846. doi: 10.3934/math.2021283 |
[9] | Hamid Reza Safaeyan, Karim Zare, Mohamadreza Mahmoudi, Mohsen Maleki, Amir Mosavi . A Bayesian approach on asymmetric heavy tailed mixture of factor analyzer. AIMS Mathematics, 2024, 9(6): 15837-15856. doi: 10.3934/math.2024765 |
[10] | Yanfang Zhang, Fuchang Wang, Yibin Zhao . Statistical characteristics of earthquake magnitude based on the composite model. AIMS Mathematics, 2024, 9(1): 607-624. doi: 10.3934/math.2024032 |
Ready-mixed-concrete (RMC) is an important green and clean building material which is widely used in modern civil engineering. For the large-scale planar foundations of urban public buildings, huge amounts of RMC need to be continuously delivered to the construction site according to strict time windows, which brings the problem of multi-plants collaborative supply. In this paper, considering transportation capacity, initial setting time, and interrupt pumping time, a collaborative scheduling model for production and transportation of RMC with the objective of minimizing the penalty cost of interruption pumping and vehicle waiting time and fuel consumption cost was established. According to the characteristics of the problem, a double chromosome synchronous evolution genetic algorithm was designed. Finally, the model and algorithm proposed in the paper were verified by data experiments. The computing results showed that in two cases of different scenarios, such as ordinary constructions and emergency constructions, the proposed scheduling model can save 18.6 and 24.8% cost respectively. The scheduling model and algorithm proposed in the paper can be applied directly to improve the operational efficiency of RMC supply chain.
One of the main challenges faced by financial companies is to evaluate market risks in a set of changes of the basic variables such as stock prices, interest rates or exchange rates. In this regard the Value-at-Risk (VaR) introduced by J. P. Morgan in the mid 1990s has become a standard risk measure of financial market risk. Despite its extensive use, the VaR is not a coherent risk measure because it fails to satisfy subadditivity property (see [1]). The VaR can not determine the expected loss of portfolio in q worst case, but it defines the minimum loss. Furthermore, the computation of the VaR is based on the assumption that financial data returns follow the normal distribution. However, as shown in the literatures, the underlying distributions of many financial data exhibit skewness, non-symmetric, heavy tails and excess kurtosis (see [10]). They suggest in particular that large losses occur with much higher probability than the normal distribution would suggest.
The tail conditional expectation (TCE) risk measure shares properties that are considered desirable in all cases. For instance, due to the additivity of expectations, TCE allows venture capital to decompose naturally among its various components.
Consider X to be a loss random variable whose cumulative distribution function (cdf) is denoted by FX(x). The TCE is defined as
TCEp(X)=E(X|X>xp),p∈(0,1), |
where xp=inf{x∈R:FX(x)≥p}=VaRp(X). The TCE has been discussed in many literatures (see e.g., [11,12,15,17]).
The tail conditional expectation risk measure shares properties that are considered desirable in a variety of situations. For instance, due to the additivity of expectations, TCE allows for a natural decomposition of risk capital among its various constituents. The conception of capital allocation principle has long been introduced, in which the capital allocated to each risk unit can be expressed as its contribution to the tail conditional expectation of total risk. Risk allocation can not only help to evaluate and compare the performance of individual risk units, but also help to understand the risk contribution of each unit towards the total risk of the portfolio. Landsman and Valdez [17] derived the portfolio risk decomposition with TCE for the multivariate elliptical distribution. In [18], authors derived the portfolio risk decomposition with TCE for the exponential dispersion model, and Kim [14] for the exponential family class. The allocation for the class of exponential marginal was developed in []. The portfolio risk decomposition with TCE was further considered in [19] for the skew-normal distribution. Furman and Landsman [16] for the multivariate Gamma distribution. Cai and Li [2] for the phase-type distribution. Goovaerts et al. [9] and Chiragiev and Landsman [4] have provided the TCE-capital allocation for the multivariate Pareto distribution while Cossette et al. [5] have considered multivariate compound distribution. Ignatieva and Landsman [12] for generalized hyperbolic distribution. Recently, Kim and Kim [15] and Ignatieva and Landsman [12] investigated the TCE allocation for the family of multivariate normal mean-variance mixture distributions and skewed generalized hyperbolic, respectively. The univariate TCE and risk allocation formula for the generalized hyper-elliptical class were available in [13].
Furman and Landsman [8] observed that in many cases the TCE does not provide adequate information about the risks on the right tail. This point can be confirmed by the fact that the TCE does not include the information that the risk deviates from the upper tail expectation. Furman and Landsman [8] introduced the tail variance measure. The tail variance is defined as
TVp(X)=Var(X|X>xp)=E((X−TCEp(X))2|X>xp), |
and it has been discussed in many literatures (see e.g., [8,15]).
In this paper we consider a class of multivariate location-scale mixtures of elliptical (LSME) distributions which is known to be extremely flexible and contains many special cases as its members. Examples include the generalized hyper-elliptical distribution and generalized hyperbolic distribution.
The rest of the paper is organized as follows. Section 2 reviews the definition and properties of the multivariate LSME class, and introduces the generalized hyper-elliptical distribution as a representative subclass. Section 3 presents a theorem and proves the proposed TCE formula for the LSME and in Section 4, the development is extended to the portfolio risk decomposition with TCE for the multivariate LSME. In Section 5, we develop TV formula for univariate LSME. Section 6 deals with the special case of generalized hyperbolic distribution. Numercial illustration is presented in Section 7. Finally, concluding remarks are presented in Section 8.
In this section, we introduce the class of location-scale mixtures of elliptical distributions and some of its properties.
Let Ψn be a class of functions ψ(t):[0,∞)→R such that function ψ(∑ni=1t2i) is an n-dimensional characteristic function.
A random vector Y is said to have a multivariate elliptical distribution, denoted by Y∼En(μ,Σ,ψ), if its characteristic function can be expressed as
φY(t)=exp(itTμ)ψ(12tTΣt), | (2.1) |
for column-vector vector μ, n×n positive definite scale matrix Σ, and for function ψ(t)∈Ψn, which is called the characteristic generator.
In general, a multivariate elliptical distribution may not have a probability density function (pdf), but if its pdf exists then the form will be
fY(y)=cn√|Σ|gn[12(y−μ)TΣ−1(y−μ)], | (2.2) |
for function gn(⋅), which is called the density generator. The condition
∫∞0un2−1gn(u)du<∞ | (2.3) |
guarantees gn(u) to be the density generator ([7]). In addition, the normalizing constant cn is
cn=Γ(n2)(2π)n2(∫∞0un2−1gn(u)du)−1. |
Similarly, the elliptical distribution can also be introduced by the density generator and then written Y∼En(μ,Σ,gn).
From (2.1), it follows that, if Y∼En(μ,Σ,gn) and A is m×n matrix of rank m≤n and b is m-dimensional column-vector, then
AY+b∼Em(Aμ+b,AΣAT,gm). |
The following condition:
∫∞0g1(u)du<∞ |
guarantees the existence of the mean. If, in addition, |ψ′(0)|<∞, the covariance matrix exists and is equal to
Cov(Y)=−ψ′(0)Σ, |
(see [3]).
From (2.2) and (2.3), g1(x) can be a density generator of univariate elliptical distribution of the random variable Y∼E1(μ,σ2,g1) whose pdf can be expressed as
fY(y)=cσg1(12(y−μσ)2), |
where c is the normalizing constant. In this paper, we assume
Var(Z)=σ2Z<∞, | (2.4) |
where Z=Y−μσ is the spherical random variable. The cdf of the random variable Z can be written as the following integration form:
FZ(z)=c∫z−∞g1(12u2)du. |
We can obtain the mean and variance of Z:
μZ=0 |
and
σ2Z=2c∫∞0u2g1(12u2)du=−ψ′(0). |
Landsman and Valdez [17] showed that
fZ∗(z)=1σ2Z¯G(12z2) | (2.5) |
is the density of another spherical random variable Z∗ associated with Z, where
¯G(z)=∫∞zg1(u)du. |
The random vector X∼ LSME n(μ,Σ,γ,gn;Π) has an n-dimensional LSME distribution with location parameter μ, positive definite scale matrix Σ, if
X=m(Θ)+Θ12Σ12Y, | (2.6) |
in distribution, where
(1) Y∼En(0,In,gn), the n-dimensional multivariate elliptical variable;
(2) Non-negative scalar random variable Θ is independent of Y, whose pdf and cdf are π(θ) and Π(θ) respectively;
(3) m(Θ)=μ+Θγ, where μ=(μ1,…,μn)Tandγ=(γ1,…,γn)T are constant vectors in Rn.
The pdf of the LSME can be written as the following integration form:
fX(x)=cn√|Σ|∫∞01√θgn(12θ(x−μ−θγ)TΣ−1(x−μ−θγ))π(θ)dθ,x∈Rn. |
We find that the conditional distribution of X|θ is elliptical, that is
X|Θ=θ∼En(m(θ),θΣ,gn). | (2.7) |
We can obtain the mean and covariance of X:
E(X)=E[E(X|Θ)]=E(m(Θ))=μ+E(Θ)γ |
and
Cov(X)=E[Var(X|Θ)]+Var[E(X|Θ)]=E(ΘΣ)+Var(m(Θ))=E(Θ)Σ+Var(Θ)γγT. |
The characteristic function of X|Θ=θ exists and equals to
φX(t|Θ=θ)=exp(itTμ)exp(iθtTγ)ψ(12θtTΣt). |
Then the characteristic function of the LSME-distributed random vector X can be written as
φX(t)=exp(itTμ)E[exp(iθtTγ)ψ(12θtTΣt)]=exp(itTμ)∫∞0exp(iθtTγ)ψ(12θtTΣt)π(θ)dθ. | (2.8) |
Under the condition (2.2) and from (2.5), we can conclude ¯G(z) is the density generator of the associated elliptical variable Z∗, then
X∗=m(Θ)+√ΘσZ∗ | (2.9) |
is said to have a univariate LSME distributions, denoted by X∗∼LSME1(μ,σ2,γ,Θ,¯G;Π).
Proposition 1. If X∼LSMEn(μ,Σ,γ,gn;Π) and Y=BX+b where B is m×n (m≤n) matrix and b is m-dimensional column-vector, then it holds that Y∼LSMEm(Bμ+b,BΣBT,Bγ,gm;Π).
Proof. Using the characteristic function (2.8), we write
φY(t)=E(eitT(BX+b))=exp(itTb)φX(BTt)=exp(itTb)exp(i(BTt)Tμ)∫∞0exp(iθ(BTt)Tγ)ψ(12θ(BTt)TΣ(BTt))π(θ)dθ=exp(itT(Bμ+b))∫∞0exp(iθtTBγ)ψ(12θtTBΣBTt)π(θ)dθ, | (2.10) |
i.e., Y∼LSMEm(Bμ+b,BΣBT,Bγ,gm;Π).
Example2.1 (Generalized hyper-elliptical distribution). The GHE distribution is constructed by mixing a generalized inverse Gaussian distribution with elliptical distribution. A positive random variable Θ is said to have a generalized inverse Gaussian distribution, denoted by Θ∼GIG(λ,a,b), if its pdf is given by
π(θ;λ,a,b)=a−λ(√ab)λ2Kλ(√ab)θλ−1exp(−12(aθ−1+bθ)),θ>0, | (2.11) |
where parameters follow
{a≥0andb>0,ifλ>0,a>0andb≥0,ifλ<0,a>0andb>0,ifλ=0 |
and Kλ(⋅) denotes the modified Bessel function of the second kind with index λ∈R. A random vector X∼GHEn(μ,Σ,γ,gn,λ,a,b) has an n-dimensional GHE distribution, if there exists a random vector Y follows (2.6) such that
X=m(Θ)+Θ12Σ12Y, | (2.12) |
where Θ∼GIG(λ,a,b).
The univariate LSME variable is given by n=1 in the multivariate definition. That is, the univariate LSME variable X∼LSME1(μ,σ2,γ,g1;Π) satisfies
X=m(Θ)+√ΘσZ, |
where Z∼E1(0,1,g1) is the standard elliptical variable, and non-negative scalar random variable Θ with pdf π(θ) is independent of Z. From (2.7), we have
X|θ∼E1(m(θ),θσ2,g1). | (3.1) |
Assuming that both the conditional distribution and the mixed distribution are continuous, the pdf of X produced by the mixed distribution can be written as
fX(x)=∫Ωθf(x|θ)π(θ)dθ, | (3.2) |
where f(x|θ) is the pdf of X|θ and Ωθ is the support of π(θ). Now let xp be the quantile of the LSME variable X. Then the TCE of X can be expressed as
E(X|X>xp)=11−p∫∞xpxfX(x)dx=11−p∫Ωθ∫∞xpxf(x|θ)dxπ(θ)dθ=11−p∫ΩθEX|θ(X|X>xp)¯FX|θ(xp)π(θ)dθ. | (3.3) |
The TCE formula for a univariate elliptical distribution is introduced by [17], and equals to
TCEp(X|θ)=EX|θ(X|X>xp)=m(θ)+1√θσfZ∗(κ(xp;θ))¯FZ(κ(xp;θ))σ2Zθσ2=m(θ)+1√θσfZ∗(κ(xp;θ))¯FZ(κ(xp;θ))Var(X|θ), | (3.4) |
where
κ(x;θ)=x−m(θ)√θσ. |
We now give a general TCE formula for the univariate LSME distributions.
Theorem 1. Let X∼LSME1(μ,σ2,γ,g1;Π) and π′(θ)=(c′)−1θπ(θ) be a mixing pdf with c′=E(Θ)<∞. Then the TCE of X can be computed by:
E(X|X>xp)=μ+γc′1−p¯FLSME,1(xp;μ,σ2,γ,g1;Π′)+c′σ2σ2Z1−pfLSME,1(xp;μ,σ2,γ,¯G;Π′), | (3.5) |
where Π′ is the cdf corresponding to the pdf π′.
Proof. From (3.3) and (3.4), we have
E(X|X>xp)=11−p∫∞0[m(θ)+1√θσfZ∗(κ(xp;θ))¯FZ(κ(xp;θ))Var(X|θ)]¯FX|θ(xp)π(θ)dθ=11−p∫∞xp∫∞0m(θ)√θσfZ(κ(x;θ))π(θ)dθdx+σ2σ2Z1−p∫∞0fZ∗(κ(xp;θ))√θσθπ(θ)dθ=11−p∫∞xpμf(x)dx+γ1−p∫∞xp∫∞0f(x|θ)θπ(θ)dθdx+σ2σ2Z1−p∫∞0fZ∗(κ(xp;θ))√θσθπ(θ)dθ. | (3.6) |
From the definition of LSME distributions and (3.2), we have
∫∞0f(x|θ)θπ(θ)dθ=c′∫∞0f(x|θ)π′(θ)dθ=c′fLSME,1(x;μ,σ2,γ,g1;Π′). |
As a result, (3.6) can be further simplified
E(X|X>xp)=μ+γc′1−p∫∞xp∫∞0f(x|θ)π′(θ)dθdx+c′σ2σ2Z1−p∫∞0fZ∗(κ(xp;θ))√θσπ′(θ)dθ=μ+γc′1−p∫∞xpfLSME,1(x;μ,σ2,γ,g1;Π′)dx+c′σ2σ2Z1−p∫∞0fZ∗(κ(xp;θ))√θσπ′(θ)dθ=μ+γc′1−p¯FLSME,1(xp;μ,σ2,γ,g1;Π′)+c′σ2σ2Z1−pfLSME,1(xp;μ,σ2,γ,¯G;Π′). |
Corollary 1. Let X∼GHE1(μ,σ2,γ,g1,λ,a,b). Assume the conditions in Theorem 1 are satisfied, then the TCE of GHE can be computed by:
TCEp(X)=μ+γ1−p√abKλ+1(√ab)Kλ(√ab)¯FGHE,1(xp;μ,σ2,γ,g1,λ+1,a,b)+σ2σ2Z1−p√abKλ+1(√ab)Kλ(√ab)fGHE,1(xp;μ,σ2,γ,¯G,λ+1,a,b). | (3.7) |
Proof. From the GIG density in (2.11), we conclude
θπ(θ;λ,a,b)=√abKλ+1(√ab)Kλ(√ab)π(θ;λ+1,a,b), |
by setting
c′=√abKλ+1(√ab)Kλ(√ab), |
then
π′(θ)=(c′)−1θπ(θ)=π(θ;λ+1,a,b), |
which also is the GIG density. Using (3.5) we can directly obtain (3.7).
Consider a risk vector Y=(Y1,…,Yn)T and S=Y1+…+Yn. We denote sp as the p-quantile of S, then
E(S|S>sp)=n∑i=1E(Yi|S>sp), |
where E(Yi|S>sp) is the contribution of the i-th risk to the aggregated risks.
Let Y=(Y1,…,Yn)∼En(μ,Σ,gn) and S=Y1+…+Yn, then ([6])
E(Yi|S=s)=∫∞−∞yif(yi|s)dyi=E(Yi)+Cov(Yi,S)Var(S)(s−E(S)). |
The contribution of risk Yi,1≤i≤n, to the total TCE can be expressed as
E(Yi|S>sp)=∫∞spE(Yi|S=s)dFS(s|S>sp)=∫∞spE(Yi|S=s)fS(s)1−FS(sp)ds=11−p∫∞spE(Yi|S=s)fS(s)ds. |
We now exploit this formulation to the multivariate LSME to obtain its portfolio risk decomposition with TCE.
Let us assume X=(X1,…,Xn)T∼LSMEn(μ,Σ,γ,gn;Π). Denote the (i,j) element of Σ by σij, define
S=X1+…+Xn. |
Then, E(Xi|S=s) can be further expanded by conditioning on θ as follows:
E(Xi|S=s)=∫∞−∞xif(xi|s)dxi=∫∞−∞xif(xis)dxifS(s)=1fS(s)∫∞−∞xi∫∞0f(xi,s|θ)dπ(θ)dxi=1fS(s)∫∞−∞xi∫∞0f(xi|s,θ)f(s|θ)π(θ)dθdxi=1fS(s)∫∞0[∫∞−∞xif(xi|s,θ)dxi]f(s|θ)π(θ)dθ. | (4.1) |
To deal with the inner integral, we define a matrix Bi of size 2×n:
Bi=[00…010…011……………1]. | (4.2) |
The first row vector has 1 in the ith position. If we keep the general form
m(θ)=(m1(θ),…,mn(θ))T, |
we have
BiX|θ=(Xi,S|θ)T=Bim(θ)+θ12BiΣ12Y, |
here (Xi,S|θ)T stands for a random column vector of size 2×1, with each element being Xi|θ and S|θ, respectively. Thus, the joint distribution of (Xi,S) under the condition of Θ=θ is a bivariate elliptical distribution
(Xi,S|θ)T∼E2(Bim(θ),θBiΣBTi,g2), |
where the mean vector and convariance matrix of (Xi,S|θ) are given by
E(BiX|θ)=Bim(θ)=(E(Xi|θ),E(S|θ))T=[mi(θ)∑nj=1mj(θ)], |
Cov(BiX|θ)=−ψ′(0)θBiΣBTi=−ψ′(0)[θσiiθ∑nj=1σijθ∑nj=1σijθσ2S], |
where
σ2S=1TΣ1=n∑i=1n∑j=1σij. |
Therefore, if we impose another condition on S, we see that f(xi|s,θ) is an elliptical density. In particular
∫∞−∞xif(xi|s,θ)dxi=E(Xi|S=s,Θ=θ)=E(Xi|θ)+Cov(Xi,S|θ)Var(S|θ)(s−E(S|θ))=mi(θ)+−ψ′(0)∑nj=1σij−ψ′(0)σ2S(s−n∑j=1mj(θ))=mi(θ)+∑dj=1σijσ2S(s−n∑j=1mj(θ)). |
Consequently
E(Xi|S=s)=1fS(s)∫∞0[∫∞−∞xif(xi|s,θ)dxi]f(s|θ)π(θ)dθ=1fS(s)∫∞0[mi(θ)+∑nj=1σijσ2S(s−n∑j=1mj(θ))]×1√θσSfZ(s−∑nj=1mj(θ)√θσS)π(θ)dθ. | (4.3) |
Eventually
E(Xi|S>sp)=11−p∫∞spE(Xi|S=s)fS(s)ds=11−p∫∞sp∫∞0[mi(θ)+∑nj=1σijσ2S(s−n∑j=1mj(θ))]×1√θσSfZ(s−∑nj=1mj(θ)√θσS)π(θ)dθds. | (4.4) |
This expression, though complex, can produce a closed-form quantity to properly select π(θ) and mj(θ).
The portfolio risk decomposition with TCE is additive, that is, the sum of all portfolio risk decomposition must amount to the TCE for S. We can verify this
n∑i=1E(Xi|S>sp)=11−pn∑i=1∫∞sp∫∞0[mi(θ)+∑nj=1σijσ2S(s−n∑j=1mj(θ))]1√θσSfZ(s−∑nj=1mj(θ)√θσS)π(θ)dθds=11−p∫∞sp∫∞0[n∑i=1mi(θ)+∑ni=1∑nj=1σijσ2S(s−n∑j=1mj(θ))]1√θσSfZ(s−∑nj=1mj(θ)√θσS)π(θ)dθds=11−p∫∞sp∫∞0[n∑i=1mi(θ)+(s−n∑j=1mj(θ))]1√θσSfZ(s−∑nj=1mj(θ)√θσS)π(θ)dθds=11−p∫∞sp∫∞0s1√θσSfZ(s−∑nj=1mj(θ)√θσS)π(θ)dθds=E(S|S>sp), |
as required. Now the general portfolio risk decomposition with TCE formula for the multivariate LSME distributions class in presented is a more concrete and compact manner when m(θ) is linear in θ.
Theorem 2. Let X=(X1,X2,⋯,Xn)T∼LSMEn(μ,Σ,γ,gn;Π) and denote the pdf of S=1TX by fS(s). Let π′(θ)=(c′)−1θπ(θ) be a mixing pdf with c′=E(Θ)<∞.
Then the portfolio risk decomposition with TCE for the i-th marginal variable is given by
E(Xi|S>sp)=b0,i+b1,iE(S|S>sp)+b2,i1−pc′¯FLSME,1(sp;1Tμ,1TΣ1,1Tγ,g1;Π′), | (4.5) |
where Π′ is the cdf corresponding to the pdf π′, the coefficients b0,i,b1,i, and b2,i are defined as
b0,i=μi−b1,in∑j=1μj;b1,i=n∑j=1σijσ2S;b2,i=γi−b1,in∑j=1γj, |
and sp is the p-quantile of S.
Proof. Let mi(θ)=μi+θγi, and from (4.3) we have
E(Xi|S=s)=1fS(s)∫∞0[μi+∑nj=1σijσ2S(s−n∑j=1μj)+(γi−n∑j=1γj∑nj=1σijσ2S)θ]×1√θσSfZ(s−∑nj=1μj−θ∑nj=1γj√θσS)π(θ)dθ=1fS(s)∫∞0[b0,i+b1,is+b2,iθ]1√θσSfZ(s−∑nj=1μj−θ∑nj=1γj√θσS)π(θ)dθ=1fS(s)[b0,ifS(s)+b1,isfS(s)+b2,ic′fLSME,1(sp;1Tμ,1TΣ1,1Tγ,g1;Π′)]=b0,i+b1,is+b2,ic′fLSME,1(sp;1Tμ,1TΣ1,1Tγ,g1;Π′)fS(s). |
By inserting this into the portfolio risk decomposition with TCE formulation (4.4), we complete the proof as
E(Xi|S>sp)=∫∞spE(Xi|S=s)f(s|S>sp)ds=∫∞spE(Xi|S=s)fS(s)1−pds=11−p∫∞sp(b0,i+b1,is)fS(s)ds+11−pb2,ic′∫∞spfLSME,1(s;1Tμ,1TΣ1,1Tγ,g1;Π′)ds=b0,i+b1,iE(S|S>sp)+b2,i1−pc′¯FLSME,1(sp;1Tμ,1TΣ1,1Tγ,g1;Π′). | (4.6) |
Notice that ∑ni=1b0,i=0,∑ni=1b1,i=1, and ∑ni=1b2,i=0, which can be used to verify that the sum of these portfolio risk decomposition amounts to E(S|S>sp).
Corollary 2. Let X=(X1,X2,⋯,Xn)T∼GHEn(μ,Σ,γ,gn,λ,a,b). The portfolio risk decomposition with TCE for the i-th marginal variable is given by
E(Xi|S>sp)=μi+σ2Zn∑j=1σij1−p√abKλ+1(√ab)Kλ(√ab)fGHE,1(sp;1Tμ,1TΣ1,1Tγ,¯G;λ+1,a,b)+γi1−p√abKλ+1(√ab)Kλ(√ab)¯FGHE,1(sp;1Tμ,1TΣ1,1Tγ,g1,λ+1,a,b). | (4.7) |
Proof. We can know S∼GHE1(sp;1Tμ,1TΣ1,1Tγ,g1,λ+1,a,b) by using Proposition 1. Using (4.5), we see that TCE of S is given by
E(S|S>sp)=μi+σ2Zn∑j=1σij1−p√abKλ+1(√ab)Kλ(√ab)fGHE,1(sp;1Tμ,1TΣ1,1Tγ,¯G,λ+1,a,b)+γi1−p√abKλ+1(√ab)Kλ(√ab)¯FGHE,1(sp;1Tμ,1TΣ1,1Tγ,g1,λ+1,a,b). |
Therefore
E(Xi|S>sp)=μi+b1,iσ2Sσ2Z1−p√abKλ+1(√ab)Kλ(√ab)fGHE,1(sp;1Tμ,1TΣ1,1Tγ,¯G,λ+1,a,b)+γi1−p√abKλ+1(√ab)Kλ(√ab)¯FGHE,1(sp;1Tμ,1TΣ1,1Tγ,g1,λ+1,a,b)=μi+σ2Z∑nj=1σij1−p√abKλ+1(√ab)Kλ(√ab)fGHE,1(sp;1Tμ,1TΣ1,1Tγ,¯G,λ+1,a,b)+γi1−p√abKλ+1(√ab)Kλ(√ab)¯FGHE,1(sp;1Tμ,1TΣ1,1Tγ,g1,λ+1,a,b). |
The TV of the univariate elliptical distribution is introduced by [8]. From (3.1), We can write the TV for X|θ as
TVp(X|θ)=Var(X|θ)[r(κ(xp;θ))+hZ,Z∗(κ(xp;θ))(κ(xp;θ)−hZ,Z∗(κ(xp;θ))σ2Z)], |
where κ(x;θ) is the same as in (3.4),
r(z)=¯FZ∗(z)¯FZ(z) |
is the distorted ratio function, and
hZ,Z∗(z)=fZ∗(z)¯FZ(z) |
is the distorted hazard function.
TV can be rewritten as:
TVp(X)=Var(X|X>xp)=E[(X−TCEp(X))2|X>xp]=E(X2|X>xp)−[TCEp(X)]2. | (5.1) |
Consequently, we need to derive the second order conditional tail moment E(X2|X>xp). We now provide its analytic expression in the following result.
Proposition 2. Assume a random variable X∼LSME1(μ,σ2,γ,g1;Π). Let π′(θ)=(c′)−1θπ(θ) and π″(θ)=(c″)−1θ2π(θ) be two different mixing pdfs with c′=E(Θ)<∞ and c″=E(Θ2)<∞ respectively. Then
E(X2|X>xp)=σ2σ2Z1−p[(xp+μ)c′fLSME,1(xp;μ,σ2,γ,¯G;Π′)+γc″fLSME,1(xp;μ,σ2,γ,¯G;Π″)+c′¯FLSME,1(xp;μ,σ2,γ,¯G;Π′)]+γ1−p[2μc′¯FLSME,1(xp;μ,σ2,γ,g1;Π′)+γc″¯FLSME,1(xp;μ,σ2,γ,g1;Π″)]+μ2, | (5.2) |
where Π′ and Π″ are two cdfs corresponding to the two different pdfs π′ and π″, respectively.
Proof. From observing
E(X2|X>xp)=11−p∫∞xpx2fX(x)dx=11−p∫∞0∫∞xpx2fX|θ(x)π(θ)dxdθ=11−p∫∞0EX|θ(X2|X>xp)¯FX|θ(xp)π(θ)dθ. |
To deal with the second order conditional tail moment in the integration, we write it as
EX|θ(X2|X>xp)=TVp(X|θ)+[TCEp(X|θ)]2. | (5.3) |
From [17], we know
TCEp(X|θ)=m(θ)+hZ,Z∗(κ(xp;θ))Var(X|θ)√θσ, |
taking Var(X|θ)=θσ2σ2Z into consideration, then (5.3) becomes
EX|θ(X2|X>xp)=Var(X|θ)[r(κ(xp;θ))+hZ,Z∗(κ(xp;θ))(κ(xp;θ)−hZ,Z∗(κ(xp;θ))σ2Z)]+(m(θ)+hZ,Z∗(κ(xp;θ))Var(X|θ)√θσ)2=Var(X|θ)r(κ(xp;θ))+Var(X|θ)hZ,Z∗(κ(xp;θ))xp−m(θ)√θσ−Var(X|θ)(hZ,Z∗(κ(xp;θ)))2σ2Z+m2(θ)+2m(θ)hZ,Z∗(κ(xp;θ))Var(X|θ)√θσ+(hZ,Z∗(κ(xp;θ)))2θσ2σ2ZVar(X|θ)θσ2=m2(θ)+Var(X|θ)(r(κ(xp;θ))+hZ,Z∗(κ(xp;θ))xp+m(θ)√θσ). |
As a result
E(X2|X>xp)=11−p∫∞0EX|θ(X2|X>xp)¯FX|θ(xp)π(θ)dθ=11−p∫∞0Var(X|θ)[xp+m(θ)√θσhZ,Z∗(κ(xp;θ))+r(κ(xp;θ))]ׯFX|θ(xp)π(θ)dθ+11−p∫∞0m2(θ)¯FX|θ(xp)π(θ)dθ=11−p∫∞0Var(X|θ)[xp+m(θ)√θσfZ∗(κ(xp;θ))+¯FZ∗(κ(xp;θ))]×π(θ)dθ+11−p∫∞0¯FX|θ(xp)π(θ)(μ2+2μθγ+θ2γ2)dθ=σ2σ2Z1−p[(xp+μ)c′fLSME,1(xp;μ,σ2,γ,¯G;Π′)+γc″fLSME,1(xp;μ,σ2,γ,¯G;Π″)+c′¯FLSME,1(xp;μ,σ2,γ,¯G;Π′)]+γ1−p[2μc′¯FLSME,1(xp;μ,σ2,γ,g1;Π′)+γc″¯FLSME,1(xp;μ,σ2,γ,g1;Π″)]+μ2. |
Theorem 3. Under assumptions of Proposition 2, the TV of X is given by
TVp(X)=σ2σ2Z1−p[(xp−μ)c′fLSME,1(xp;μ,σ2,γ,¯G;Π′)+γc″fLSME,1(xp;μ,σ2,γ,¯G;Π″)+c′¯FLSME,1(xp;μ,σ2,γ,¯G;Π′)]+γ21−pc″¯FLSME,1(xp;μ,σ2,γ,g1;Π″)−(c′1−p)2[γ¯FLSME,1(xp;μ,σ2,γ,g1;Π′)+σ2σ2ZfLSME,1(xp;μ,σ2,γ,¯G;Π′)]2, | (5.4) |
where Π′ and Π″ are two cdfs corresponding to the two different pdfs π′ and π″, respectively.
Proof. From the Theorem 1, the TCE formula is
E(X|X>xp)=μ+γc′1−p¯FLSME,1(xp;μ,σ2,γ,g1;Π′)+c′σ2σ2Z1−pfLSME,1(xp;μ,σ2,γ,¯G;Π′). |
Hence, the result can be derived by using Proposition 2 and (5.1).
Corollary 3. Let X∼GHE1(μ,σ2,γ,g1,λ,a,b). Assume the conditions in Theorem 3 are satisfied, then the TV for GHE can be computed by:
TVp(X)=σ2σ2Z1−p[(xp−μ)√abKλ+1(√ab)Kλ(√ab)fGHE,1(xp;μ,σ2,γ,¯G;λ+1,a,b)+γabKλ+2(√ab)Kλ(√ab)fGHE,1(xp;μ,σ2,γ,¯G;λ+2,a,b)+√abKλ+1(√ab)Kλ(√ab)¯FGHE,1(xp;μ,σ2,γ,¯G;λ+1,a,b)]+γ21−pabKλ+2(√ab)Kλ(√ab)¯FGHE,1(xp;μ,σ2,γ,g1,λ+2,a,b)−(11−p√abKλ+1(√ab)Kλ(√ab))2[γ¯FGHE,1(xp;μ,σ2,γ,g1,λ+1,a,b)+σ2σ2ZfGHE,1(xp;μ,σ2,γ,¯G;λ+1,a,b)]2. | (5.5) |
Proof. From the GIG density in (2.11), we conclude
θπ(θ;λ,a,b)=√abKλ+1(√ab)Kλ(√ab)π(θ;λ+1,a,b) |
and
θ2π(θ;λ,a,b)=abKλ+2(√ab)Kλ(√ab)π(θ;λ+2,a,b). |
By setting
c′=√abKλ+1(√ab)Kλ(√ab),c″=abKλ+2(√ab)Kλ(√ab), |
the two pdfs can be presented as
π′(θ)=(c′)−1θπ(θ)=π(θ;λ+1,a,b) |
and
π″(θ)=(c″)−1θ2π(θ)=π(θ;λ+2,a,b), |
which also are two GIG pdfs. Using (5.4) we can directly obtain (5.5).
Example6.1 (Generalized hyperbolic distribution). If μ=0, Σ=In and density generator g(u)=e−u in (2.2), then the elliptical vector Y is said to have a multivariate normal distribution, denoted by Y∼Nn(0,In). Letting Y∼Nn(0,In) in (2.6), then the random vector X∼GHn(μ,Σ,γ,λ,a,b) is an n-dimensional generalized hyperbolic (GH) distribution. Therefore, the pdf of the GH distribution is (see [15])
fGHn(x,μ,Σ,γ,λ,a,b)=cKλ−(n/2)(√(a+(x−μ)TΣ−1(x−μ))(b+γTΣ−1γ))e(x−μ)TΣ−1γ(a+(x−μ)TΣ−1(x−μ))(b+γTΣ−1γ))n4−λ2, |
where the normalizing constant is
c=(ab)−λ2bλ(b+γTΣ−1γ)(n/2)−λ(2π)n/2|Σ|1/2Kλ√ab. |
From Corollary 1, TCE of GH distribution is given by
TCEp(X)=μ+γ1−p√abKλ+1(√ab)Kλ(√ab)¯FGH,1(xp;μ,σ2,γ,λ+1,a,b)+σ21−p√abKλ+1(√ab)Kλ(√ab)fGH,1(xp;μ,σ2,γ,λ+1,a,b). |
From Corollary 2, portfolio risk decomposition with TCE for the i-th marginal of GH distribution is given by
E(Xi|S>sp)=μi+n∑j=1σij1−p√abKλ+1(√ab)Kλ(√ab)fGH,1(sp;1Tμ,1TΣ1,1Tγ,λ+1,a,b)+γi1−p√abKλ+1(√ab)Kλ(√ab)¯FGH,1(sp;1Tμ,1TΣ1,1Tγ,λ+1,a,b). |
From Corollary 3, TV of GH distribution is given by
TVp(X)=σ21−p[(xp−μ)√abKλ+1(√ab)Kλ(√ab)fGH,1(xp;μ,σ2,γ,λ+1,a,b)+γabKλ+2(√ab)Kλ(√ab)fGH,1(xp;μ,σ2,γ,λ+2,a,b)+√abKλ+1(√ab)Kλ(√ab)¯FGH,1(xp;μ,σ2,γ,λ+1,a,b)]+γ21−pabKλ+2(√ab)Kλ(√ab)¯FGH,1(xp;μ,σ2,γ,λ+2,a,b)−(11−p√abKλ+1(√ab)Kλ(√ab))2[γ¯FGH,1(xp;μ,σ2,γ,λ+1,a,b)+σ2fGH,1(xp;μ,σ2,γ,λ+1,a,b)]2. |
In this section we discuss the TV of five stocks (Amazon, Goldman Sachs, IBM, Google, and Apple) and aggregate portfolio covering a time frame from the 1st of January 2015 to the 1st of January 2017. Ignatieva and Landsman [12] fitted a GH model to five stocks and aggregate portfolio, and obtained the following parameter set based on the maximum likelihood technique.
λ=−1.18336,a=1.272016,ψ=0.348483,μ=(−0.09977−0.04555−0.09355−0.03669−0.10367),γ=(−0.08626−0.008030.07928−0.052300.08534), |
Σ=(3.3871.4071.1031.8281.3541.4073.0141.2881.2091.4341.1031.2881.8701.0611.1551.8281.2091.0612.1711.2201.3541.4341.1551.2202.891). |
For the risk analysis, we denote five stocks as X1,⋯,X5. We also consider aggregate portfolio S where each stock has equal weight for simplicity, so that the aggregate portfolio is defined as S=X1+⋯+X5. Figure 1 shows the densities for five stocks Xi,i=1,⋯,5 and aggregate portfolio S. The pdf of S has the largest variance, and Amazon has the largest dispersion among five stocks. IBM has the smallest dispersion. Figure 2 presents the TV for five stocks Xi,i=1,⋯,5 and aggregate portfolio S. All the risk measures increase over the quantile with the TV. Also Figure 2 shows the differences in the TV measure along with five stocks and aggregate portfolio. For the same quantile, the TV of Apple is the largest one and the TV of IBM is the smallest one among the five stocks.
In this paper we generalize the tail risk measure and portfolio risk decomposition with TCE formula derived by [15] for the class of multivariate normal mean-variance mixture distributions to the larger class of multivariate elliptical location-scale mixtures distributions. A prominent member in the normal mean-variance mixture class is the generalized hyperbolic (GH) distribution, which itself can construct a Leˊvy process. The \text{GH} is a special case of normal mean-variance mixture random variable with \boldsymbol{X}\sim \text{N}_n{(\boldsymbol{0}, \boldsymbol{I_n})} and the distribution of \Theta given by a generalized inverse Gaussian (GIG) distribution with three parameters (see [12,15] for details). Prominent member in the elliptical location-scale mixtures class is the generalized hyper-elliptical ( \text{GHE} ) distribution. The \text{GHE} distribution provides excellent fit to univariate and multivariate data, allowing to capture a long right tail in the distribution of losses even more effectively than the \text{GH} distribution considered in [12]. And \text{GHE} is a special case of elliptical location-scale mixtures random variable with \boldsymbol{X}\sim \text{N}_n{(\boldsymbol{0}, \boldsymbol{I_n})} and the distribution of \Theta given by a generalized inverse Gaussian (GIG) distribution with three parameters. Although the univariate \text{TCE} and portfolio risk decomposition with \text{TCE} formula for the \text{GHE} class was available in [13], it can be derived more efficiently and seen as a special case of \text{TCE} for the unified location-scale mixtures of elliptical distributions and risk allocation formula in Theorems 1 and 2, respectively. And the univariate \text{TV} formula for the \text{GHE} class can be derived efficiently and seen as a special case of \text{TV} for the unified location-scale mixtures of elliptical distributions in Theorem 3.
The research was supported by the National Natural Science Foundation of China (No. 12071251).
The authors declare no conflict of interest.
[1] |
M. Maghrebi, T. Waller, C. Sammut, Sequential meta-heuristic approach for solving large-scale ready-mixed concrete-dispatching problems, J. Comput. Civil Eng. , 30 (2016), 4014177. https://doi.org/10.1061/(ASCE)CP.1943-5487.0000453 doi: 10.1061/(ASCE)CP.1943-5487.0000453
![]() |
[2] |
S. Y. Yan, W. S. Lai, An optimal scheduling model for ready mixed concrete supply with overtime considerations, Autom. Constr. , 16 (2007), 734–744. https://doi.org/10.1016/j.autcon.2006.12.009 doi: 10.1016/j.autcon.2006.12.009
![]() |
[3] |
S. Y. Yan, W. S. Lai, M. N. Chen, Production scheduling and truck dispatching of ready mixed concrete, Transp. Res. Part E Logist. Transp. Rev. , 44 (2008), 164–179. https://doi.org/10.1016/j.tre.2006.05.001 doi: 10.1016/j.tre.2006.05.001
![]() |
[4] | K. M. Xu, Optimize the Schedule of Dispatching RMC Trucks Based on GA, Master thesis, Dalian Maritime University, 2012. |
[5] |
M. Hsiea, C. Y. Huang, W. T. Hsiao, M. Y. Wu, Y. C. Liu, Optimization on ready-mixed concrete dispatching problem via sliding time window searching, KSCE J. Civil Eng. , 26 (2022), 3173–3187. https://doi.org/10.1007/s12205-022-1273-0 doi: 10.1007/s12205-022-1273-0
![]() |
[6] |
J. Yang, B. Yue, F. F. Feng, J. F. Shi, H. Y. Zong, J. X. Ma, et al., Concrete vehicle scheduling based on immune genetic algorithm, Math. Probl. Eng. , 2022 (2022), 1–15. https://doi.org/10.1155/2022/4100049 doi: 10.1155/2022/4100049
![]() |
[7] |
D. J. Thomas, P. M. Griffin, Coordinated supply chain management, Eur. J. Oper. Res. , 94 (1996), 1–15. https://doi.org/10.1016/0377-2217(96)00098-7 doi: 10.1016/0377-2217(96)00098-7
![]() |
[8] |
Z. L. Chen, Integrated production and outbound distribution scheduling: review and extensions, Oper. Res. , 58 (2010), 130–148. https://doi.org/10.1287/opre.1080.0688 doi: 10.1287/opre.1080.0688
![]() |
[9] |
D. Y. Wang, O. Grunder, A. E. Moudni, Integrated scheduling of production and distribution operations: a review, Int. J. Ind. Syst. Eng. , 19 (2015), 94–122. https://doi.org/10.1504/IJISE.2015.065949 doi: 10.1504/IJISE.2015.065949
![]() |
[10] |
S. Moons, K. Ramaekers, A. Caris, Y. Arda, Integrating production scheduling and vehicle routing decisions at the operational decision level: a review and discussion, Comput. Ind. Eng. , 104 (2017), 224–245. https://doi.org/10.1016/j.cie.2016.12.010 doi: 10.1016/j.cie.2016.12.010
![]() |
[11] |
C. W. Feng, T. M. Cheng, H. T. Wu, Optimizing the schedule of dispatching rmc trucks through genetic algorithms, Autom. Constr. , 13 (2004), 327–340. https://doi.org/10.1016/j.autcon.2003.10.001 doi: 10.1016/j.autcon.2003.10.001
![]() |
[12] |
C. W. Feng, H. T. Wu, Integrating Fm GA and cyclone to optimize the schedule of dispatching RMC trucks, Autom. Constr. , 15 (2006), 186–199. https://doi.org/10.1016/j.autcon.2005.04.001 doi: 10.1016/j.autcon.2005.04.001
![]() |
[13] | M. H. Fazel Zarandi, A. A. Sadat Asl, S. Sotudian, O. Castillo, A state of the art review of intelligent scheduling, Int. Sci. Eng. J. , 53 (2020), 9–12. |
[14] |
M. Durbin, K. Hoffman, The dance of the thirty-ton trucks dispatching and scheduling in a dynamic environment, Oper. Res. , 56 (2008), 3–19. https://doi.org/10.1287/opre.1070.0459 doi: 10.1287/opre.1070.0459
![]() |
[15] |
P. C. Lin, J. H. Wang, S. H. Huang, Y. T. Wang, Dispatching ready mixed concrete trucks under demand postponement and weight limit regulation, Autom. Constr. , 19 (2010), 8–807. https://doi.org/10.1016/j.autcon.2010.05.002 doi: 10.1016/j.autcon.2010.05.002
![]() |
[16] |
A. K. Gaikwad, S. B. Thakare, Genetic algorithm based optimized un-interrupted dispatching schedule for ready mix concrete truck with user friendly interface on single window (single plant multi-sites), Int. J. Recent Technol. Eng. , 8 (2019), 3276–3281. https://doi.org/10.35940/ijrte.B2890.078219 doi: 10.35940/ijrte.B2890.078219
![]() |
[17] |
S. Y. Yan, W. C. Wang, G. W. Chang, H. C. Lin, Effective ready mixed concrete supply adjustments with inoperative mixers under stochastic travel times, Transp. Lett. , 8 (2016), 286–300. https://doi.org/10.1080/19427867.2016.1188522 doi: 10.1080/19427867.2016.1188522
![]() |
[18] | N. Mayteekrieangkrai, W. Wongthatsanekorn, Optimized ready mixed concrete truck scheduling for uncertain factors using bee algorithm, Songklanakarin J. Sci. Technol. , 37 (2015), 221–230. |
[19] | H. Q. Cai, Optimize the Schedule of Dispatching RMC Trucks Base on Multi-objective GA and Simulation, Master thesis, Shanghai Jiaotong University, 2007. |
[20] | X. W. Wu, Concrete transportation vehicles scheduling scheme based on the ant colony algorithm, Concrete, 12 (2009), 123–125. |
[21] | X. W. Gao, Scheduling for Concrete Transportation Based on GPS, GIS Vehicle Localization Supervisory Dispatching System, Master thesis, Shanghai Jiaotong University, 2008. |
[22] | W. Liang, The Optimization Strategy of Distribution in Commercial Concrete, Master thesis, Central South University of Forestry Science and Technology, 2013. |
[23] |
N. F. Matsatsinis, Towards a decision support system for the ready concrete distribution system: a case of a Greek company, Eur. J. Oper. Res. , 152 (2004), 487–499. https://doi.org/10.1016/S0377-2217(03)00039-0 doi: 10.1016/S0377-2217(03)00039-0
![]() |
[24] | Y. F. Liu, X. T. Liu, X. K. Song, K. Chen, S. Q. Wang, Cooperative supply mode and distribution scheme designing of multiple concrete mixing station, Concrete, 7 (2016), 127–129. |
[25] |
D. Naso, M. Surico, B. Turchiano, U. Kaymak, Genetic algorithms for supply-chain scheduling: A case study in the distribution of ready-mixed concrete, Eur. J. Oper. Res. , 177 (2007), 2069–2099. https://doi.org/10.1016/j.ejor.2005.12.019 doi: 10.1016/j.ejor.2005.12.019
![]() |
[26] |
V. Schmid, K. F. Doerner, R. F. Hartl, J. J. Salazar-González, Hybridization of very large neighborhood search for ready-mixed concrete delivery problems, Comput. Oper. Res. , 37 (2010), 559–574. https://doi.org/10.1016/j.cor.2008.07.010 doi: 10.1016/j.cor.2008.07.010
![]() |
[27] |
Z. Y. Liu, Y. K. Zhang, M. H. Yu, X. L. Zhou, Heuristic algorithm for ready-mixed concrete plant scheduling with multiple mixers, Autom. Constr. , 84 (2017), 1–13. https://doi.org/10.1016/j.autcon.2017.08.013 doi: 10.1016/j.autcon.2017.08.013
![]() |
[28] | N. Wu, Research on RMC of Vehicle Dispatch Based on SA-PSO Optimization, Master thesis, Hebei University of Engineering, 2011. |
[29] |
S. Srichandum, S. Pothiya, Multiple plants multiple sites ready mixed concrete planning using improved ant colony optimization, Int. J. GEOMATE, 19 (2020), 88–95. https://doi.org/10.21660/2020.72.9355 doi: 10.21660/2020.72.9355
![]() |
[30] | J. Yang, H. Y. Zong, J. X. Ma, D. Guo, J. F. Shi, L. Q. Wang, Coordinated dispatching of distribution vehicles in concrete batching plant, China Sciencepaper, 16 (2021), 644–648. |
[31] |
N. N. Dawood, Scheduling in the precast concrete industry using the simulation modelling approach, Build. Environ. , 30 (1995), 197–207. https://doi.org/10.1016/0360-1323(94)00039-U doi: 10.1016/0360-1323(94)00039-U
![]() |
[32] |
M. Kalantari, M. Rabbani, M. Ebadian, A decision support system for order acceptance/rejection in hybrid MTS/MTO production systems, Appl. Math. Modell. , 35 (2010), 1363–1377. https://doi.org/10.1016/j.apm.2010.09.015 doi: 10.1016/j.apm.2010.09.015
![]() |
[33] |
M. Weiszer, G. Fedorko, V. Molnár, Z. Tučková, M. Poliak, Dispatching policy evaluation for transport of ready mixed concrete, Open Eng. , 10 (2020), 120–128. https://doi.org/10.1515/eng-2020-0030 doi: 10.1515/eng-2020-0030
![]() |
[34] |
K. Kianfar, S. M. T. F. Ghomi, B. Karimi, New dispatching rules to minimize rejection and tardiness costs in a dynamic flexible flow shop, Int. J. Adv. Manuf. Technol. , 45 (2009), 759–771. https://doi.org/10.1007/s00170-009-2015-x doi: 10.1007/s00170-009-2015-x
![]() |
[35] |
S. Abdollahpour, J. Rezaian, Two new meta-heuristics for no-wait flexible flow shop scheduling problem with capacitated machines, mixed make-to-order and make-to-stock policy, Soft Comput. , 21 (2017), 3147–3165. https://doi.org/10.1007/s00500-016-2185-z doi: 10.1007/s00500-016-2185-z
![]() |
[36] |
G. C. Zhang, J. C. Zeng, J. H. Zhang, Rescheduling strategy of ready-mixed concrete vehicles: A case study of dynamic requirements of customers, Proc. Inst. Mech. Eng., Part B: J. Eng. Manuf. , 231 (2017), 2223–2237. https://doi.org/10.1177/0954405415623481 doi: 10.1177/0954405415623481
![]() |
[37] |
Z. G. Guo, Y. F. Liu, C. J. Ao, A solution for the rational dispatching of concrete transport vehicles, Sci. Rep. , 12 (2022), 16770. https://doi.org/10.1038/s41598-022-21011-y doi: 10.1038/s41598-022-21011-y
![]() |
[38] |
Q. Chen, B. G. de Soto, B. T. Adey, Supplier-contractor coordination approach to managing demand fluctuations of ready-mix concrete, Autom. Constr. , 121 (2021), 103423. https://doi.org/10.1016/j.autcon.2020.103423 doi: 10.1016/j.autcon.2020.103423
![]() |
[39] |
B. L. Wang, T. K. Li, H. F. Wang, Order acceptance and scheduling on parallel machines with setup time and machine-eligibility constraints, Chin. J. Eng. , 41 (2019), 528–538. https://doi.org/10.13374/j.issn2095-9389 doi: 10.13374/j.issn2095-9389
![]() |
[40] | M. J. Li, T. S. Tong, A partheno genetic algorithm and analysis on its global convergence, Acta Autom. Sin. , 25 (1999), 68. |
[41] |
J. L. Wang, W. B. Huang, G. W. Ma, S. J. Chen, An improved partheno genetic algorithm for multi-objective economic dispatch in cascaded hydropower systems, Int. J. Electr. Power Energy Syst., 67 (2015), 591–597. https://doi.org/10.1016/j.ijepes.2014.12.037 doi: 10.1016/j.ijepes.2014.12.037
![]() |
[42] |
C. M. Joo, B. S. Kim, Hybrid genetic algorithms with dispatching rules for unrelated parallel machine scheduling with setup time and production availability, Comput. Ind. Eng., 85 (2015), 102–109. https://doi.org/10.1016/j.cie.2015.02.029 doi: 10.1016/j.cie.2015.02.029
![]() |
1. | Mengxin He, Zhong Li, Dynamic behaviors of a Leslie-Gower predator-prey model with Smith growth and constant-yield harvesting, 2024, 32, 2688-1594, 6424, 10.3934/era.2024299 |