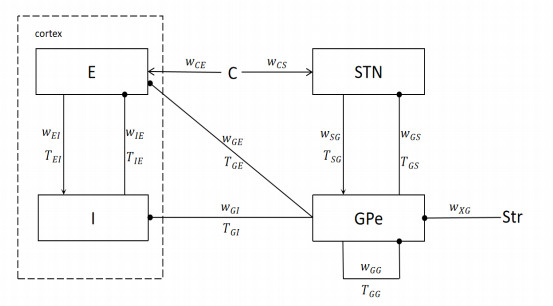
The origin, location and cause of Parkinson's oscillation are not clear at present. In this paper, we establish a new cortex-basal ganglia model to study the origin mechanism of Parkinson beta oscillation. Unlike many previous models, this model includes two direct inhibitory projections from the globus pallidus external (GPe) segment to the cortex. We first obtain the critical calculation formula of Parkinson's oscillation by using the method of Quasilinear analysis. Different from previous studies, the formula obtained in this paper can include the self-feedback connection of GPe. Then, we use the bifurcation analysis method to systematically explain the influence of some key parameters on the oscillation. We find that the bifurcation principle of different cortical nuclei is different. In general, the increase of the discharge capacity of the nuclei will cause oscillation. In some special cases, the sharp reduction of the discharge rate of the nuclei will also cause oscillation. The direction of bifurcation simulation is consistent with the critical condition curve. Finally, we discuss the characteristics of oscillation amplitude. At the beginning of the oscillation, the amplitude is relatively small; with the evolution of oscillation, the amplitude will gradually strengthen. This is consistent with the experimental phenomenon. In most cases, the amplitude of cortical inhibitory nuclei (CIN) is greater than that of cortical excitatory nuclei (CEX), and the two direct inhibitory projections feedback from GPe can significantly reduce the amplitude gap between them. We calculate the main frequency of the oscillation generated in this model, which basically falls between 13 and 30 Hz, belonging to the typical beta frequency band oscillation. Some new results obtained in this paper can help to better understand the origin mechanism of Parkinson's disease and have guiding significance for the development of experiments.
Citation: Minbo Xu, Bing Hu, Weiting Zhou, Zhizhi Wang, Luyao Zhu, Jiahui Lin, Dingjiang Wang. The mechanism of Parkinson oscillation in the cortex: Possible evidence in a feedback model projecting from the globus pallidus to the cortex[J]. Mathematical Biosciences and Engineering, 2023, 20(4): 6517-6550. doi: 10.3934/mbe.2023281
[1] | P. E. Greenwood, L. M. Ward . Rapidly forming, slowly evolving, spatial patterns from quasi-cycle Mexican Hat coupling. Mathematical Biosciences and Engineering, 2019, 16(6): 6769-6793. doi: 10.3934/mbe.2019338 |
[2] | Alan Dyson . Traveling wave solutions to a neural field model with oscillatory synaptic coupling types. Mathematical Biosciences and Engineering, 2019, 16(2): 727-758. doi: 10.3934/mbe.2019035 |
[3] | Peng Feng, Menaka Navaratna . Modelling periodic oscillations during somitogenesis. Mathematical Biosciences and Engineering, 2007, 4(4): 661-673. doi: 10.3934/mbe.2007.4.661 |
[4] | Xiebing Chen, Yuliang Ma, Xiaoyun Liu, Wanzeng Kong, Xugang Xi . Analysis of corticomuscular connectivity during walking using vine copula. Mathematical Biosciences and Engineering, 2021, 18(4): 4341-4357. doi: 10.3934/mbe.2021218 |
[5] | Van Dong Nguyen, Dinh Quoc Vo, Van Tu Duong, Huy Hung Nguyen, Tan Tien Nguyen . Reinforcement learning-based optimization of locomotion controller using multiple coupled CPG oscillators for elongated undulating fin propulsion. Mathematical Biosciences and Engineering, 2022, 19(1): 738-758. doi: 10.3934/mbe.2022033 |
[6] | Changgui Gu, Ping Wang, Tongfeng Weng, Huijie Yang, Jos Rohling . Heterogeneity of neuronal properties determines the collective behavior of the neurons in the suprachiasmatic nucleus. Mathematical Biosciences and Engineering, 2019, 16(4): 1893-1913. doi: 10.3934/mbe.2019092 |
[7] | Banghua Yang, Xuelin Gu, Shouwei Gao, Ding Xu . Classification accuracy and functional difference prediction in different brain regions of drug abuser prefrontal lobe basing on machine-learning. Mathematical Biosciences and Engineering, 2021, 18(5): 5692-5706. doi: 10.3934/mbe.2021288 |
[8] | Sujuan Zhou, Huajian Lin, Jiang Meng . Discrimination and chemical composition quantitative model of Raw Moutan Cortex and Moutan Cortex Carbon based on electronic nose and machine learning. Mathematical Biosciences and Engineering, 2022, 19(9): 9079-9097. doi: 10.3934/mbe.2022422 |
[9] | LanJiang Luo, Haihong Liu, Fang Yan . Dynamic behavior of P53-Mdm2-Wip1 gene regulatory network under the influence of time delay and noise. Mathematical Biosciences and Engineering, 2023, 20(2): 2321-2347. doi: 10.3934/mbe.2023109 |
[10] | Maria Conceição A. Leite, Yunjiao Wang . Multistability, oscillations and bifurcations in feedback loops. Mathematical Biosciences and Engineering, 2010, 7(1): 83-97. doi: 10.3934/mbe.2010.7.83 |
The origin, location and cause of Parkinson's oscillation are not clear at present. In this paper, we establish a new cortex-basal ganglia model to study the origin mechanism of Parkinson beta oscillation. Unlike many previous models, this model includes two direct inhibitory projections from the globus pallidus external (GPe) segment to the cortex. We first obtain the critical calculation formula of Parkinson's oscillation by using the method of Quasilinear analysis. Different from previous studies, the formula obtained in this paper can include the self-feedback connection of GPe. Then, we use the bifurcation analysis method to systematically explain the influence of some key parameters on the oscillation. We find that the bifurcation principle of different cortical nuclei is different. In general, the increase of the discharge capacity of the nuclei will cause oscillation. In some special cases, the sharp reduction of the discharge rate of the nuclei will also cause oscillation. The direction of bifurcation simulation is consistent with the critical condition curve. Finally, we discuss the characteristics of oscillation amplitude. At the beginning of the oscillation, the amplitude is relatively small; with the evolution of oscillation, the amplitude will gradually strengthen. This is consistent with the experimental phenomenon. In most cases, the amplitude of cortical inhibitory nuclei (CIN) is greater than that of cortical excitatory nuclei (CEX), and the two direct inhibitory projections feedback from GPe can significantly reduce the amplitude gap between them. We calculate the main frequency of the oscillation generated in this model, which basically falls between 13 and 30 Hz, belonging to the typical beta frequency band oscillation. Some new results obtained in this paper can help to better understand the origin mechanism of Parkinson's disease and have guiding significance for the development of experiments.
Parkinson's disease is the second most common neurodegenerative brain disease and has a higher incidence rate among the elderly [1,2]. Parkinson's disease places a great burden on society and the economy, and its research has attracted more and more attention from society. Postural imbalance, rigidity, tremors, etc. are typical symptoms of Parkinson's patients [3,4]. The loss of neurons in the substantia nigra pars compacta, which causes a large loss of dopamine in the striatum, is considered to be the main cause of these symptoms [5,6]. The precise pathological mechanism of dopamine loss is still unclear, and many different views have been formed. Moreover, the excessive beta frequency synchronous oscillation observed in electroencephalogram is generally considered to be closely related to the emergence and disappearance of Parkinson's symptoms [7,8,9].
In the past, many brain network computing models have been developed to explore the origin mechanism of beta oscillation [10,11,12,13,14,15,16,17,18]. Especially, many studies have shown that beta oscillations in the basal ganglia may be induced by anomalous interaction of circuits that consist of the subthalamic nucleus (STN) and the globus pallidus pars externa (GPe) [19,20,21,22,23,24]. The STN contains a large number of glutamatergic neurons that exert excitatory inputs to the GPe; in turn, the GPe is mainly composed of GABAergic neurons, which give inhibitory feedback to the STN. Together, they form an inhibitory-excitatory coupling loop. Nevado Holgado et al. pointed out that the STN-GPe network can produce beta oscillation independently, and coupling weights and delays in the loop have great influence on oscillation [19]. Recently, Hu et al. explored the bidirectional Hopf bifurcation mechanism in an STN-GPe model, which can uniformly explain the origin of the observed oscillation [24].
The cortex has a close input-output relationship with the basal ganglia. The cortex exerts strong excitatory projections to the basal ganglia, which is necessary for the existence of Parkinson's oscillation [25,26]. In fact, the beta oscillation may also originate in the cortex and spread to other brain regions [27,28]. The loss of dopamine in the striatum can disrupt cortical function, which may be the cause of Parkinson's symptoms [29]. The function of the primary motor cortex is damaged in Parkinson's disease, which may be an effective goal for neurostimulation in alleviating motor dysfunction [30]. In anatomical structure, the cortex is mainly composed of long-range excitatory pyramidal neurons (CEX) and short-range inhibitory interneurons (CIN) [27,28]; they also form excitatory-inhibitory circuits, the structure of which allows easy generation of oscillations independently [31]. Pavlides et al. proposed a STN-GPe-CEX-CIN mean-field network [32]. In this model, the CEX-CIN loop exerts excitatory inputs to the STN. They found that beta oscillations can occur in the cortex, and the STN-GPe loop resonated with inputs from the cortex. Recently, Chen et al. obtained theoretical critical conditions for Hopf bifurcation of beta oscillation in a similar model [33]. We note that the mechanism of beta oscillation in the cortex is rarely discussed in previous models.
In most previous models, the cortex had strong excitability projection to the subthalamic nucleus, and the effect of cortical outputs on beta oscillations has been widely studied [20,27,28,34,35,36]. In fact, there is a significant inhibitory feedback effect from basal ganglia to cortex [32], which also has a great influence on the origin of Parkinson's oscillation, but there are few relevant theoretical models. Recently, experiments have shown that GPe has a direct inhibitory output on both excitatory and inhibitory neurons in the cortex [37,38]. In addition, deep brain stimulation technology has a significant effect on GPe to alleviate the symptoms of Parkinson's disease [39,40,41], which may be regulated through these direct outputs to the cortex. Because many regions of the cortex have been reported to be closely related to the generation of Parkinson oscillations [42,43,44], we are interested to know whether the direct inhibitory projection from the basal ganglia to the cortex can explain the origin and disappearance of beta oscillation. Computational models can provide some possible mechanisms for these pathways in inducing and controlling oscillations, which can be confirmed in experiments.
In this paper, we propose a new model that consists of the STN, the GPe, the CEX and the CIN nucleus. In this model, there are direct inhibitory inputs from GPe to CEX and CIN, respectively. We mainly study the critical conditions of oscillation, Hopf bifurcation mechanism, amplitude and frequency characteristics of oscillation in the CEX-CIN circuit.
The new model is described in Section 2, and the stability analysis is given in Sections 3 and 4. The theoretical mechanism and main results are obtained in Sections 5 and 6. Finally, the conclusions are summarized in Section 7.
Figure 1 is our new model, which consists of cortex and basal ganglia. The cortex includes excitatory nuclei (CEX) and inhibitory nuclei (CIN), and the basal ganglia is composed of subthalamic nucleus (STN) and globus pallidus external (GPe) segment. C represents the constant excitatory input to CEX and STN, and Str represents the inhibitory input from the striatum. The arrow represents the excitatory projection regulated by glutamate, and the dot represents the inhibitory projection regulated by GABAergic. There is a self-feedback connection inside GPe.Tijandwij represent the time delay of signal transmission and coupling strength on the pathway, respectively, and subscripts i and j indicate the origin and termination positions of the signal, respectively. CEX-CIN and STN-GPe loops are both "excitatory-inhibitory" coupling structures. In this model, there are two inhibitory feedback projections from the GPe to cortex, which is different from many previous studies. For the convenience of the following description, CEX, CIN, STN and GPe are represented by marks E, I, S and G respectively.
We use the following average discharge rate equation to describe the coupling relationship and dynamic behavior between neuron groups in Figure 1 [19,20,32,45,46]:
τSS′(t)=FS(−wGSG(t−TGS)+wCSC)−S(t) | (1) |
τGG′(t)=FG(wSGS(t−TSG)−wGGG(t−TGG)−wXGStr)−G(t) | (2) |
τEE′(t)=FE(−wGEG(t−TGE)−wIEI(t−TIE)+wCEC)−E(t) | (3) |
τII′(t)=FI(wEIE(t−TEI)−wGIG(t−TGI))−I(t) | (4) |
where S(t), G (t), E(t) and I(t) represent the average discharge rates of STN, GPe, CEX and CIN, respectively; S(t-T), G(t-T), E(t-T) and I(t-T) represent the corresponding delay discharge rates. τX is the time constant corresponding to neuron group X. FY(Y = S, G, E, I) is the activation function of population Y, showing the effect of synaptic input on the average discharge rate [19,20,32].
FS(syt)=MS1+(MS−BSBS)exp(−4sytMS) | (5) |
FG(syt)=MG1+(MG−BGBG)exp(−4sytMG) | (6) |
FE(syt)=ME1+(ME−BEBE)exp(−4sytME) | (7) |
FI(syt)=MI1+(MI−BIBI)exp(−4sytMI) | (8) |
syt in Eqs (5)–(8) represents all possible inputs of the corresponding neuron group, MX is the maximum discharge rate of neuron group X, and BX is the basic discharge rate of neuron group X without external input. Similar to previous research [19,20,32], we show the curves of the activation function and its derivatives in Figure 2A, B, respectively. The activation functions of different neurons are heterogeneous, and all of them are S-shaped curves. The maximum derivative of the activation functions is 1.
All parameters and values used in this paper are listed in Tables 1 and 2, and they come from previous experiments and model studies [19,20,32,33,47,48,49,50,51,52,53,54,55,56,57,58,59,60,61,62]. Figure 3A, B show the simulated discharge rates in Parkinson's state and healthy state, respectively. In Parkinson's state, different neural nuclei show synchronous oscillation (OS). In the healthy state, the system is stable (SS), and the discharge rate of each neuron tends to be constant. Stability and oscillation are two basic states of the model.
Parameter | Value | Parameter | Value |
τS | 13 ms | τG | 20.3 ms |
τE | 12.1 ms | τI | 14.7 ms |
MS | 300 spk/s | MG | 400 spk/s |
ME | 75 spk/s | MI | 310 spk/s |
BS | 8.1 spk/s | BG | 19 spk/s |
BE | 5.5 spk/s | BI | 16.58 spk/s |
Str | 2.12 spk/s | C | 17.1 spk/s |
T | 6.12 ms |
Parameter | Value |
wGS | 10.63 |
wCS | 9.15 |
wSG | 20.12 |
wGG | 11.96 |
wXG | 135.1 |
wIE | 3.22 |
wEI | 2.97 |
wGE | 14.96 |
wCE | 27.18 |
wGI | 5.35 |
First, let Fj(syt)=syt(j=S,G,E,I) to obtain the linearized system (9)–(12) of Eqs (1)–(4). In this part, we use the Laplace transform and the theory of solutions of ordinary differential equations to obtain the oscillation critical conditions of system (9)–(12). For the feasibility of mathematical derivation, we assume that the time delay and time constant are the same for all projections and neurons, and they are recorded as T and τ respectively. Then, the following linear system is obtained:
τS′(t)=−wGSG(t−T)−S(t) | (9) |
τG′(t)=wSGS(t−T)−wGGG(t−T)−G(t) | (10) |
τE′(t)=−wGEG(t−T)−wIEI(t−T)−E(t) | (11) |
τI′(t)=wEIE(t−T)−wGIG(t−T)−I(t) | (12) |
By writing Eqs (9)–(12) in matrix form and shifting the terms, we can get
[S′(t)G′(t)E′(t)I′(t)]+A[S(t−T)G(t−T)E(t−T)I(t−T)]+B[S(t)G(t)E(t)I(t)]=0 | (13) |
where
A=[0wGSτ00−wSGτwGGτ0000wGEτwGIτ0wIEτ−wEIτ0] | (14) |
B=[1τ00001τ0000001τ001τ] | (15) |
The following operations and properties of the Laplace transform will be used [63]:
L[f(t−T)]=e−sTF(s), | (16) |
L[f(t)]=F(s), | (17) |
L[f′(t)]=sF(s)−F(0). | (18) |
By performing the corresponding Laplace transform on the matrices other than A and B in Eq (13), we can get
−(S(0)G(0)E(0)I(0))+s(S(s)G(s)E(s)I(s))+Ae−sT(S(s)G(s)E(s)I(s))+B(S(s)G(s)E(s)I(s))=0 | (19) |
Without losing generality, we set the initial conditions as
(S(0)G(0)E(0)I(0))=0. | (20) |
Then,
sI+Ae−sT+B=0, | (21) |
where s is the eigenvalue of the characteristic equation, and I is the identity matrix. Take the determinant on both sides of Eq (21):
det(sI+Ae−sT+B)=0, | (22) |
i.e.,
[s+1τwGSτe−sT00−wSGτe−sTs+1τ+wGGτe−sT0000wGEτe−sTwGIτe−sTs+1τwIEτe−sT−wEIτe−sTs+1τ]=0. | (23) |
Then,
((s+1τ)(s+1τ+wGGτe−sT)+wSGwGSτ2e−2sT)((s+1τ)2+wEIwIEτ2e−2sT)=0. | (24) |
Let
Δ1=((s+1τ)2+wEIwIEτ2e−2sT), | (25) |
Δ2=((s+1τ)(s+1τ+wGGτe−sT)+wSGwGSτ2e−2sT). | (26) |
From the knowledge of ordinary differential equations, we know that the stability of system (9)–(12) is determined by the sign of the real part of eigenvalue s in Eq (24). When the real part of s is greater than 0, the system is unstable; when the real part of s is less than 0, the system is stable. Therefore, if we find the case that s is a pure imaginary number, we can get the stable and unstable transition boundary of the system, that is, the stability boundary. When Δ1 or Δ2 is equal to 0, Eq (24) holds. Next, we will discuss these two situations.
(ⅰ) Assume the characteristic value s=iλ (λ>0). Substitute the eigenvalue into Eq (25) and expand it by the Euler formula:
(−λ2+1τ2+2λiτ)+wEIwIEτ2(cos2λT−isin2λT)=0. | (27) |
Let τ=1,T1=Tτ, and then Eq (27) becomes
(−λ2+1+2λi)+wEIwIE(cos2λT1−isin2λT1)=0. | (28) |
Separating the real part and imaginary part of Eq (28), we can obtain
−λ2+1+wEIwIEcos2λT1=0, | (29) |
2λ−wEIwIEsin2λT1=0. | (30) |
Add the squares of Eqs (29) and (30), and we can get that
λ=√wEIwIE−1. | (31) |
The stability boundary condition can be obtained by substituting λ into Eq (29):
T1=12√wEIwIE−1arccos(1−2wEIwIE). | (32) |
Then, the conditions for oscillation of the linear system are as follows:
Tτ>12√wEIwIE−1arccos(1−2wEIwIE). | (33) |
(ⅱ) Assume characteristic value s=iλ (λ>0). We substitute the eigenvalue into Eq (26) and expand it by the Euler formula:
(iλ+1τ)(iλ+1τ+wGGτ(cosλT−isinλT))+wGSwSGτ2(cos2λT−isin2λT)=0. | (34) |
Set τ=1,T2=Tτ, and then Eq (34) can be written as
(iλ+1)(iλ+1+wGG(cosλT2−isinλT2))+wGSwSG(cos2λT2−isin2λT2)=0. | (35) |
Next, for the convenience and feasibility of derivation, we assume that the self-feedback connection of GPe is 0. That is, when wGG=0, Eq (35) is reduced to
(−λ2+1+2λi)+wSGwGS(cos2λT−isin2λT)=0. | (36) |
By separating the real part and imaginary part of Eq (36), Eqs (37) and (38) can be obtained:
−λ2+1+wSGwGScos2λT2=0, | (37) |
2λ−wSGwGSsin2λT2=0. | (38) |
Add the squares of Eqs (37) and (38) and find the expression of λ,
λ=√wSGwGS−1. | (39) |
Replace λ in Eq (37) with (39), and the stability boundary condition can be obtained:
T2=12√wSGwGS−1arccos(1−2wSGwGS). | (40) |
Then, the conditions for oscillation are as follows:
Tτ>12√wSGwGS−1arccos(1−2wSGwGS). | (41) |
In this part, we use the results of oscillation boundary conditions of linear systems to analyze the stability boundary conditions of nonlinear systems. First, the equilibrium point of the nonlinear system can be obtained by minimizing Eq (42), which is recorded as (S∗,G∗,E∗,I∗).
R=(FS(−wGSG(t−TGS)+wCSC)−S(t))2+(FG(−wSGS(t−TSG)−wGGG(t−TGG)−wXGStr)−G(t))2+(FE(−wGEG(t−TGG)−wIEI(t−TIE)+wCEC)−E(t))2+(FI(−wEIE(t−TEI)−wGIG(t−TGI))−I(t))2. | (42) |
The characteristic equation of the new system can be obtained by linearizing the activation function at the equilibrium point (S∗,G∗,E∗,I∗):
sI+A1e−sT1+B=0, | (43) |
where
A1=(0F′S∗wGSτ00−F′G∗wSGτF′G∗wGGτ0000F′E∗wGEτF′I∗wGIτ0F′E∗wIEτ−F′I∗wEIτ0) | (44) |
B=[1τ00001τ0000001τ001τ] | (45) |
The expressions of F′S∗,F′G∗,F′E∗,F′I∗ are as follows:
F′S∗=F′S(−wGSG∗+wCSC), | (46) |
F′G∗=F′G(wSGS∗−wGGG∗−wXGStr), | (47) |
F′E∗=F′E(−wGEG∗−wIEI∗+wCEC), | (48) |
F′I∗=F′I(wEIE∗−wGIG∗). | (49) |
Then, using the same steps as dealing with linear systems (Section 3), we can obtain two stability boundary conditions corresponding to nonlinear systems:
Tτ=12√F′E∗F′I∗wEIwIE−1arccos(1−2F′E∗F′I∗wEIwIE), | (50) |
Tτ=12√F′S∗F′G∗wSGwGS−1arccos(1−2F′S∗F′G∗wSGwGS). | (51) |
The corresponding two oscillation conditions are
Tτ>12√F′E∗F′I∗wEIwIE−1arccos(1−2F′E∗F′I∗wEIwIE), | (52) |
Tτ>12√F′S∗F′G∗wSGwGS−1arccos(1−2F′S∗F′G∗wSGwGS). | (53) |
In this part, we mainly analyze and study some possible generation mechanisms of the stability critical condition (50).
The E and I form an excitatory-inhibitory coupling circuit in this model, and this structure is prone to oscillation. In this section, we study the mechanism of coupling weights in this loop on oscillations.
Figure 4 describes the influence of coupling weight wEI on oscillation. Figure 4A shows the theoretical oscillation critical conditions obtained by simulating Eq (50). The system is oscillating above the curve and stable below the curve. Fix parameter Tτ at an appropriate value (such as 4), and with the increase of wEI, the state of the system can transition from stable to oscillation (as shown by the arrow in Figure 4A). The influence mechanism of wEI on nuclei E and I is different. From the connection structure in Figure 1, we know that the excitability coupling weight wEI acts directly on I, and its increase directly promotes the improvement of discharge ability of I. Therefore, with the increase of wEI, the ADR of I gradually increases, as shown in Figure 4B. When wEI increases to a certain value, Hopf bifurcation (HP1) occurs in the state of I, and oscillation occurs in I, as shown in Figure 4C. For the convenience of the following description, we define HP1 to represent the Hopf bifurcation transition between the ordinary stable state (SS) and the oscillating state (OS). wEI mainly affects the discharge state of E through pathway E→I→E, and we can infer that this effect is generally inhibitory from Figure 1. Therefore, with the increase of wEI, the ADR of E gradually decreases, as shown in Figure 4D. When wEI is relatively small, the ADR of E is relatively high, and the E is in a stable state of high discharge rate (HSS). Interestingly, when wEI increases to a certain value, Hopf bifurcation phenomenon (HP2) will also appear in E, which represents the transition between the stable state of high discharge rate and oscillation state. Figure 4F is the stable state obtained by taking wEI = 8; Figure 4G is the time series diagram of oscillation state obtained by taking wEI = 42.
Figure 5 shows the influence of coupling weight wIE on oscillation in the system. Figure 5A shows the theoretical oscillation critical condition obtained from Eq (50). The system is oscillating above the curve and stable below the curve. The oscillation critical curve presents a U-shape, and when Tτ is fixed at a value (such as 2), with the increase of wIE, the state of the system will be transferred from HSS to OS and finally to SS, as shown by the arrow in Figure 5A. wIE is the inhibitory coupling weight directly acting on E, and the increase of wIE will have a strong inhibitory effect on the activity of E. wIE mainly affects the discharge activity of I through indirect pathway "I→E→I", and we can infer from Figure 1 that the increase of wIE also exerts an inhibitory effect on I. Therefore, with the increase of wIE, the ADRs of nuclei E and I are decreasing, as shown in Figures 5B and 5C. In Figure 5D, E, we simulate the state bifurcation diagrams of E and I, respectively. When wIE is relatively small, the E-I circuit is in HSS; with the increase of wIE, its inhibitory effect on E and I gradually increased. When wIE increases to a certain value, HP2 bifurcation occurs in E-I loop, and HSS transitions to OS. If wIE is too large, the oscillation state will disappear, and HP1 bifurcation will appear in E and I. The two-way bifurcation phenomenon shown in Figure 5D, E is consistent with the U-shaped curve in Figure 5A. Figure 5F–H describe three different states of loop E-I. Figure 5F shows the HSS state, with a relatively high average discharge rate. Figure 5G shows the OS state, and Figure 5H shows the SS state.
In addition to the coupling weight, time delay is another key parameter in the model. In Figures 4A and 5A, we can observe that the time delay must reach a certain value before oscillation can occur in the system. Figure 6 describes the effect of time delay on loop E-I oscillation. Figure 6A is a bifurcation diagram of the state of E with an increase in delay. Figure 6B is a state transition diagram of I with the change of delay. They show that when the time delay reaches a certain value, Hopf bifurcation will occur in E and I, and the oscillation amplitude will increase with the increase of time delay. Figure 6C is the stable time series obtained by taking T = 0.003 s. Figure 6D is the oscillation time series obtained by taking T = 0.007 s.
In this part, we study the influence of direct and indirect feedback inputs from STN and GPe on the oscillation in E and I.
Figure 7 describes the effect of the direct inhibitory pathway "GPe→E" on oscillation. wGE is the coupling weight on the pathway "GPe→E". Figure 7A is the oscillation critical curve simulated by Eq (50). The system is oscillating above the curve and stable below the curve. As shown by the arrow in Figure 7A, when we fix abscissa Tτ at an appropriate value (such as 7), with the increase of wGE, the system can realize bidirectional Hopf bifurcation transition between different stable states and oscillation states. wGE is the inhibitory coupling weight directly acting on E; therefore, the increase of wGE will lead to the decrease of ADR of E, as shown in Figure 7B. wGE mainly affects the activities of I through the pathway "GPe→E →I". From Figure 1, we can infer that the increase of wGE generally exerts an inhibitory effect on I; therefore, the ADR of I also decreases gradually, as shown in Figure 7C. Figure 7D, E show the bifurcation of different oscillation states of E and I, respectively. With the increase of wGE, loop E-I realizes the synchronous transfer of HSS, OS and SS. When wGE is small, its inhibitory effect is relatively small, and E and I are in the HSS state. When wGE increases to a certain value, HP2 bifurcation appears in loop E-I, and oscillation begins to appear. When wGE is too large, its inhibitory effect on loop E-I is too strong, and the oscillation of E and I disappears with the appearance of HP1. Figure 7F–H show time series diagrams obtained by taking wGE as 5, 70 and 100, respectively, and they visually show the different discharge states of loop E-I.
"GPe→I" is a direct inhibitory pathway applied from globus pallidus to I. wGI is the coupling weight on the pathway "GPe → I". We simulate the effect of wGI on oscillation in Figure 8A. As shown by the arrow in the figure, when the delay is fixed, the increase of wGI can make the state of loop E-I transition from oscillation to stability, and the mechanism of oscillation in E and I is different. The GPe mainly acts on E through the pathway "GPe →I→E" and the two coupling weights on this pathway are inhibitory. Therefore, the increase of wGI exerts an excitatory effect on E, and the ADR of E increases with the increase of wGI (as shown in Figure 8B). When wGI increases to a certain value, HP2 bifurcation will occur in E, the oscillation of E disappears, and E enters into HSS state, as shown in Figure 8C. On the contrary, wGI exerts a direct inhibitory effect on I; therefore, the ADR of I decreases with the increase of wGI (as shown in Figure 8D). When wGI increases to a certain value, HP1 bifurcation will occur in I, the oscillation of I disappears, and I enters into SS state, as shown in Figure 8E. Figure 8F is a stable time series diagram of loop E-I, and Figure 8G is an oscillation time series diagram of loop E-I. They are obtained by taking wGI = 38.1 and wGI = 10 respectively.
In this model, we assume that there is a constant excitatory input from cortex to E, and wCE represents the coupling weight in the input. Figure 9 describes the influence of coupling weight wCE on oscillation. Figure 9A shows the theoretical boundary conditions obtained from Eq (50). The system is oscillating above the curve and stable below the curve. The left side of the curve is SS state, and the right side of the curve is HSS state. When Tτ is fixed at a suitable value (such as 3), the increase of wCE can realize the bidirectional Hopf bifurcation transition of loop E-I. wCE acts directly on E, and its increase will certainly promote the improvement of discharge capacity of E. wCE acts on I indirectly through the pathway "E→I" and"E→I" is an exciting pathway. Therefore, the increase of wCE also promotes the improvement of the discharge capacity of I. Therefore, as shown in Figure 9B, C, the ADRs of E and I both increase with the increase of wCE. When wCE is small, the discharge capacity of E-I is low, and the circuit is in a stable state. When wCE increases to a certain value, HP1 bifurcation occurs in E and I, and the loop begins to oscillate. When wCE is too large, the oscillation will disappear, and the loop will enter into HSS state. Figure 9D, E intuitively show the bidirectional Hopf bifurcation phenomenon of E and I.
The influence of subthalamic nucleus and globus pallidus circuit on E and I is indirect. In this part, we mainly study the influence mechanism of coupling weights related to STN-GPe loop on E-I loop oscillation.
wGS is an inhibitory coupling weight inside the loop STN-GPe. Figure 10 shows the effect of wGS on oscillation. Figure 10A is the oscillation critical curve simulated by Eq (50). The system is oscillating above the curve and stable below the curve. As shown by the arrow in Figure 10A, when we fix Tτ at a suitable value (such as 10), the increase of wGS can make the state of the system transition from stability to oscillation. From the connection structure in Figure 1, we can analyze that wGS affects nuclei E and I through the pathways "GPe→STN→GPe→E" and "GPe→STN→GPe→I", respectively. From the competition of inhibition and excitation coupling weights in these two pathways, we can infer that the increase of wGS can promote the discharge of E and I in general. Therefore, the ADR of E and I will increase with the increase of wGS, as shown in Figure 10B, C. When wGS increases to a certain value, the discharge level of loop E-I exceeds a certain threshold, and HP1 bifurcation occurs in E and I, thus starting oscillation. Figure 10D, E are bifurcation diagrams of E and I with the increase of wGS. In order to observe the stable and oscillating states more intuitively, we take wGS = 6 and wGS = 85, respectively, to simulate the time series diagram, as shown in Figure 10F, G.
In this model, we assume that the striatum has an inhibitory constant input Str to GPe. Figure 11 shows the effect of Str on oscillation. Figure 11A is the oscillation critical curve simulated by Eq (50). The system is oscillating above the curve and stable below the curve. As shown by the arrow in Figure 11A, when we fix Tτ at a suitable value (such as 1), the increase of Str can make the state of the system transition from stability to oscillation. From the connection structure in Figure 1, we can analyze that Str affects nuclei E and I through the pathways "Str→GPe→E" and "Str→GPe→I", respectively. The coupling weights in these two pathways are all inhibitory, and we can speculate that the increase of Str can promote the discharge of E and I in general. Therefore, the ADR of E and I will increase with the increase of Str, as shown in Figure 11B, C. When Str increases to a certain value, the discharge level of loop E-I exceeds a certain threshold, and HP1 bifurcation occurs in E and I, thus starting oscillation, as shown in Figure 11D, E. In order to observe the stable and oscillating states more intuitively, we take Str = 3 and Str = 20 to simulate the time series diagrams, as shown in Figure 11F, G.
WSG is the excitability coupling weight inside the loop STN-Gpe. In Figure 12, we simulate the influence of wSG on the oscillation in system E-I. The curve in Figure 12A is the critical condition of Hopf bifurcation, which is simulated by mathematical Eq (50). If the parameters fall above the curve, the system is oscillatory; if the parameters fall below the curve, the system is stable. As shown by the arrow in Figure 12A, when Tτ is fixed at a certain value (such as 0.8), the increase of wSG can make the state of the system transition from oscillation to stability, and the mechanism of oscillation in E and I is different. It can be seen from Figure 1 that wSG mainly affects the active state of I through the inhibitory pathway "Gpe→I" and the increase of wSG will strengthen the inhibitory effect of "GPe→I". Therefore, the ADR of I decreases with the increase of wSG, as shown in Figure 12B. When wSG increases to a certain value, the oscillation of I is suppressed, and HP1 bifurcation occurs in I, as shown in Figure 12C. On the other hand, wSG affects the active state of E through two pathways: "GPe→E" and "GPe→I→E". Obviously, the increase of wSG will strengthen the inhibitory effect of "GPe→E". However, the two coupling weights on the pathway "GPe→I→E" are both inhibitory, and the increase of wSG generally strengthens the excitatory effect of "GPe→I→E". Because "I→E" is the internal pathway of loop E-I, we speculate that its impact on E is stronger than the external pathway. Therefore, through the competition between the pathways "GPe→E" and "GPe→I→E", the increase of wSG generally exerts an excitatory effect on E, and the ADR of E also increases, as shown in Figure 12D. When wSG reaches a certain value, the oscillation state of E shifts to HSS, and HP2 occurs, as shown in Figure 12E. Figure 12F, G show time series diagrams obtained by taking wSG = 5 and wSG = 20, respectively.
In this model, we assume that there is an inhibitory self-feedback projection in the GPe, and wGG is the coupling weight in this projection. Obviously, wGG affects the discharge activities of nuclei E and I through inhibitory pathways "Gpe→E" and "Gpe→I", respectively. Because wGG itself is inhibitory, the increase of wGG exerts an excitatory effect on E and I. Figure 13A shows the oscillation critical conditions simulated on plane (wGG, Tτ). As shown in the figure, with the increase of wGG, the state of the system will transition from oscillation to HSS. The ADR of E and I gradually increases with the increase of wGG, as shown in Figure 13B, C. When wGG increases to a certain value, HP2 bifurcation occurs in both E and I, as shown in Figure 13D, E. Figure 13F is the oscillation time series obtained by taking wGG = 5. Figure 13G is the stable time series diagram of high discharge rate obtained by taking wGG = 50.
In this model, we assume that the cortex has a constant input C to both the STN and E. Next, we consider the influence of the change of C on the oscillations of E and I. From the connection structure in Figure 1, we know that the influence of C acting on E on circuit E-I is direct, and the influence of C acting on STN on circuit E-I is indirect. Therefore, we speculate that the direct effect on E is the main one, which is also consistent with the simulation results in Figure 14. C mainly affects I through the excitatory pathway "E→I". Therefore, the increase of C exerts excitatory effects on both E and I. It can be seen from the critical curve in Figure 14A that the increase of C can make the system have bidirectional Hopf bifurcation. When C is small, the system is in a stable state; when C is large, the system is in HSS state. The ADRs of E and I both increase with the increase of C, as shown in Figure 14B, C. Figure 14D, E show two specific state bifurcation diagrams, and the direction of bidirectional Hopf bifurcation is consistent with Figure 14A. Figure 14F–H show state time series diagrams obtained by taking C = 2, C = 9 and C = 14, respectively, which visually show the discharge state of loop E-I under different parameters.
wCS is the excitatory coupling weight directly acting on STN by the cortex. Obviously, wCS mainly acts on nuclei E and I through the pathways "STN→GPe→E" and "STN→GPe→I", respectively, and these two pathways are generally inhibitory. When wCS is relatively small, the discharge rate of loop E-I is relatively high, and E and I are in HSS state. When wCS increases to a certain value, HSS will be suppressed, and the system will enter the oscillation state, as shown by the arrow in Figure 15A. To sum up, the ADR of E and I will decrease with the increase of wCS, as shown in Figure 15B, C. Figure 15D, E show two specific state bifurcation diagrams. As shown in the figures, when wCS increases to a certain value, HP2 bifurcation occurs in E and I.
Interestingly, when we simulate some parameters, we observe that HP bifurcation may occur at both ends of the steady state, which is a relatively rare phenomenon in previous model studies. As shown in Figure 16A, when wSG increases, the oscillation state of E first enters stability after HP2 bifurcation, and then the stable state enters oscillation state with the emergence of HP1. In Figure 16B, we simulate the ADR of E; we observe that the slope of ADR changes greatly at the bifurcation point, as shown by SLP1 and SLP2 in the figure. With the increase of wSG, HP1 and HP2 appear in the state of I, as shown in Figure 16C. The slope of ADR of I also changes greatly at the bifurcation point, as shown in Figure 16D. Therefore, we speculate that the sudden change of the slope of ADR is also an important reason for bifurcation transition. A similar phenomenon can also be observed in Figure 17.
Oscillation amplitude is an important feature of periodic motion. The change of oscillation amplitude is closely related to the evolution of the disease and Parkinson's symptoms. In this part, we mainly discuss the influence of coupling weight on oscillation amplitude.
Figure 18 describes the influence of excitability-inhibition coupling weights (wEI, wIE) inside loop E-I on oscillation amplitude. Figure 18A, B show the effect of wEI and wIE on the amplitude of E in three-dimensional and two-dimensional cases, respectively. As shown in Figure 18B, when wEI is fixed at a suitable value, the increase or decrease of wIE will make the oscillation amplitude disappear, as shown by the two-way arrow in the figure. Figure 18C shows the variation trend of the amplitude of E with the increase of wIE, which is obtained by taking wEI = 18. When wIE is relatively small or too large, the oscillation amplitude will be 0. Within the range of oscillation parameters, the amplitude of E first increases and then decreases, which is consistent with the bidirectional bifurcation in Figure 5D. In the oscillation region, the influence of wEI on the amplitude of E is relatively small. Figure 18D shows the change trend of the amplitude of E with the increase of wEI, which is obtained by taking wIE = 5. When wEI increases to a certain value, the oscillation amplitude begins to appear and E begins to oscillate, which is consistent with the situation described in bifurcation diagram Figure 4E. Figure 18E, F describe the change trend of the oscillation amplitude of I in the plane (wEI, wIE). The amplitude of I is much higher than that of E. Although the bifurcation mechanism of oscillation in E and I is different, their amplitude change trend under the influence of parameters wEI and wIE is similar, as shown in Figure 18F. Figure 18G, H show the amplitude changes of I obtained by taking wEI = 18 and wIE = 5, respectively, and they are consistent with the bifurcation trends shown in Figures 4C and 5E.
In this model, we assume that there are two external constant inputs: striatal inhibitory input Str and cortical excitatory input C. Figure 19 depicts the effect of these two inputs on the oscillation amplitudes of nuclei E and I. Figure 19A is a three-dimensional stereogram of the amplitude of E, and Figure 19B is a corresponding plane graph. As shown by the two-way arrow in Figure 19B, the increase or decrease of C can make the oscillation amplitude disappear. Figure 19C is a specific amplitude change graph, where we fix Str to 25. With the increase of C, the E experiences SS, OS and HSS in turn, which is consistent with the bifurcation analysis in Figure 14D. As shown by the white arrow in Figure 19B, the increase or decrease of Str can also make the oscillation disappear, and this direction depends on the size of C. For example, we take C = 7 and get a change trend of amplitude with Str in Figure 19D. When Str increases to a certain value, the oscillation begins to appear, and the oscillation amplitude basically remains unchanged, which is consistent with the bifurcation result in Figure 11D. Due to the synchronous resonance of E and I, the characteristics of oscillation amplitude in I are similar to those in E, as shown in Figure 19E–H. The amplitude of I is also greater than that of E, but their gap is smaller than that in Figure 18.
wGI and wGE are two direct inhibitory coupling weights feedback from GPe to cortex. Figure 20 simulates the variation trend of the oscillation amplitude of loop E-I with the increase of wGI and wGE. Figure 20A is a three-dimensional rendering. Figure 20B is a graph of the amplitude change of E obtained in the parameter plane (wGE, wGI). When both wGI and wGE are small, the oscillation amplitude of E is relatively large. When wGI is fixed (such as 12), the increase of wGE will increase the amplitude of E from 0. When wGE increases to a certain value, the oscillation amplitude will drop to 0, as shown in Figure 20C; this is consistent with the bidirectional bifurcation case described in Figure 7D. When wGE = 10 is fixed, the oscillation amplitude will disappear with the increase of wGI, as shown in Figure 20D; this is consistent with the bifurcation trend in Figure 8C. Figure 20E, F describe the changing trend of the amplitude of I. Under the influence of parameters wGI and wGE, the amplitude difference between E and I is not very obvious, and even in some parameter ranges, the amplitude of I is lower than that of E. This is mainly because the increase of wGI and wGE both exert inhibitory effects on I.
As mentioned in the introduction, excessive beta frequency oscillation activity is a typical feature of Parkinson's patients. Figure 21 describes the oscillation frequency of the system under the influence of four different parameters. In the steady state, the oscillation frequency is 0; in the oscillation state, the frequency of the system falls in the range of 13–30 Hz, which is a typical beta frequency band oscillation. Figure 21 shows that the model we establish is effective for studying Parkinson's beta oscillation.
In this paper, we propose a new improved cortex-basal ganglia model to study the origin and control mechanism of Parkinson beta oscillation. In this model, the cortex contains excitatory and inhibitory nuclei. The basal ganglia mainly contains the subthalamic nucleus and globus pallidus external segment, and the striatum is simplified as a constant input. Different from many previous models, in this new model, we assume that there are two direct inhibitory projections from GPe to the two nuclei of the cortex. This model includes two excitatory-inhibitory coupling loops: E-I and STN-GPe. Theoretically, the circuit with this structure is prone to oscillation under certain conditions.
First, we obtain two nonlinear oscillation critical conditions (50) and (51) of systems (1)–(4) by means of quasi linearization, Laplace transform and eigenvalue analysis. These two formulas show the relationship between the parameters in the model and the conditions to be met when the oscillation occurs. Equation (50) mainly describes the relationship between parameters and time delay in loop E-I, which rarely appears in previous model research, and is a new calculation formula. Moreover, we do not need to assume that the self-feedback connection of GPe is 0 to get Eq (50), which is also different from previous studies. Equation (51) is a calculation formula obtained by assuming that the self-feedback connection of GPe is 0, and it mainly describes the relationship between the coupling weight, time delay and time constant of the loop STN-GPe. We mainly use Eq (50) to simulate the critical conditions of oscillation, combined with bifurcation analysis and calculation of average discharge rate to discuss the generation of oscillation and transition dynamic mechanism. Since the oscillation characteristics of E and I nuclei have received little attention in previous studies, our simulation and analysis mainly focus on these two nuclei.
We first discuss the influence of the two coupling weights wEI and wIE in the loop E-I and the time delay on the oscillation. The critical condition of oscillation is a monotonic or U-shaped curve. The system above the curve is oscillatory, and the system below the curve is stable. The same parameter has different effects on E and I, and the origin mechanism of oscillation in different nuclei may also be different. For example, the increase of wEI promotes the discharge of I but suppresses the discharge of E. Average discharge rate (ADR) describes the discharge capacity of nuclei. Generally, when ADR exceeds a certain value, the nucleus will oscillate, and the state of the nucleus will change from the stability of lower ADR to oscillation. We denote this type of Hopf bifurcation as HP1, as shown in Figure 4C. In some cases, if the ADR of the nucleus is too large, the nucleus will be in the discharge saturation state or high discharge rate state; and in order to produce oscillation, it is necessary to reduce the ADR of the nucleus, as shown in Figure 4E. We call the bifurcation in this case HP2. HP1 and HP2 can explain all bifurcation behaviors in this model. The increase of wIE exerts both inhibitory effects on E and I, and at this time, the origin mechanism of oscillation in E and I is similar, as shown by the U-shaped critical curve and bidirectional bifurcation diagram in Figure 5. Time delay is an indispensable parameter in this model, as we know from Eqs (52) and (53) that the system will oscillate only when the time delay exceeds a certain value. In Figure 6, we simulate the state transition diagram of E and I with respect to the increase of delay. As shown in the figure, when the time delay is large enough, HP1 bifurcation will occur in E and I, and the oscillation amplitude also increases with the increase of T.
Then, we study the influence of several projections that directly affect the loop E-I on the oscillation. wGE and wGI are the two inhibitory feedback coupling weights from GPe to E-I loop. wGE exerts an inhibitory effect on E and I, and the increase of wGE can make the loop state realize the transfer of HSS-OS-SS, as shown in Figure 7. The effect of wGI on E and I is just the opposite. In general, the increase of wGI has an exciting effect on E and an inhibitory effect on I. Therefore, the oscillation in E can disappear through HP2 bifurcation, and the oscillation in I can disappear through HP1 bifurcation, as shown in Figure 8C, E. In addition, wCE is the excitatory projection from cortical tissue to E, which is also the only excitatory projection in this model that has a direct effect on circuit E-I. Obviously, the increase of wCE exerts both excitatory effects on E and I, so the loop realizes the transition from SS to OS and then to HSS, as shown in Figure 9D, E.
In addition to the E-I loop, this model also includes the STN-GPe loop, which is also an inhibitory-excitatory coupling structure. The oscillation in E-I may be spontaneous or induced by the oscillation in the loop STN-GPe, at least affected by the loop STN-GPe. We systematically discuss the influence of the coupling weight and input related to the loop STN-GPe on the oscillation. wGS and wSG are a pair of coupling weights within the loop STN-GPe. After analysis, wGS exerts excitatory influence on E and I, and the oscillation of loop E-I is transferred from HP1 bifurcation, as shown in Figure 10. wSG has different effects on E and I, as it exerts inhibitory effects on I and excitatory effects on E. Therefore, when wSG increases to a certain value, the oscillation in I disappears due to HP1, and the oscillation in E disappears due to HP2, as shown in Figure 12C, E. Str is an inhibitory striatal constant input acting on GPe, and its mechanism is similar to that of wGS. The increase of Str exerts an excitatory effect on E and I. Therefore, the oscillation mechanism shown in Figure 11 is similar to that of wGS.
wGG is the only self-feedback projection in this model. In many previous theoretical studies [20], in order to make the derivation feasible, it is always assumed that wGG = 0, and the influence of wGG on oscillation is ignored. In this model, the Eq (50) we derived can include parameter wGG, which is an improvement on the previous research. In Figure 13, we simulate the effect of wGG on oscillation. In general, the increase of wGG exerts an excitatory effect on E and I. When the E-I circuit is in oscillation, the increase of wGG will enhance the discharge capacity of the system and enter the HSS state, as shown in Figure 13D, E.
Theoretically, we also observe the phenomenon of secondary bifurcation at both ends of the steady state, as shown in Figures 16 and 17. This is not involved in the previous theoretical model research. Through analysis, we believe that the sudden change of the slope of ADR is also an important reason for Hopf bifurcation.
Finally, we study the characteristics of beta oscillation amplitude. In general, the amplitude is the smallest near the bifurcation point; as the oscillation evolves, the amplitude will gradually increase. This is consistent with the observation in the experiment; the change of oscillation amplitude is closely related to the evolution of Parkinson's disease. Different parameters have different effects on oscillation amplitude. In the parameter pair (wEI, wIE), wIE is relatively sensitive to the influence of amplitude. When both wEI and wIE are relatively small, the amplitude of E is relatively large. When wEI is relatively large, the amplitude of I is also relatively large. In general, under the influence of parameters wEI and wIE, the amplitude of I is much larger than that of E. In the parameter pair (C, Str), Str has little effect on the amplitude within the range of oscillation parameters; the increase of C will increase the amplitude first and then decrease. Under the influence of parameters (C, Str), the amplitude of I is also relatively larger than that of E, but the amplitude difference between E and I is smaller than that in parameter pair (wEI, wIE). In the parameter pair (wGE, wGI), the amplitude difference between E and I is small; when both wGE and wGI are small, the amplitude is large, as shown in Figure 20. We simulate the influence of several key parameters on the oscillation frequency, and we observe that the frequency of the system basically falls between 13 and 30 Hz.
In the study of oscillation mechanism, we also notice that some control methods can make oscillation disappear. As shown by the two-way arrow in Figure 18, the increase or decrease of wIE can make the oscillation activity disappear. Obviously, wIE directly and indirectly regulates the discharge capacity of E and I, respectively. Similarly, as shown by the two-way black arrow in Figure 19, the role of C is also to directly or indirectly regulate the discharge capacity of circuit E-I. When we adjust C to a proper value, the oscillation will disappear. wGE and wGI in Figure 20 are two coupling weights that directly adjust the discharge capacity of E and I, and their increase or decrease can also make the oscillation disappear. One of the main principles of deep brain stimulation technology commonly used in clinical medicine is to alleviate or eliminate the oscillatory activity by regulating the electrophysiological activity of neurons at the stimulation site. Therefore, we speculate that the cortex may be an effective stimulation area to control Parkinson's disease [64,65,66], which can provide a basis for the study of Parkinson's oscillation regulation strategy.
In this paper, we mainly study the influence of various key physiological parameters on the oscillation activity in the E-I circuit. In this model, in addition to the factors of the E-I circuit itself, the STN-GPe circuit has obvious input to the E and I. Figure 22 depicts some possible relationships of the oscillation modes between four populations. When the loop STN-GPe is in a stable state, the E may be stable or oscillating, as shown in Figure 22A, B. When the loop STN-GPe is in an oscillating state, the E may oscillate or stabilize, as shown in Figure 22C, D. Therefore, we speculate that some oscillations in loop E-I are spontaneous, and some are caused by the oscillation activity in STN-GPe loop. It is meaningful to accurately distinguish these different oscillation origin mechanisms, which will rely on more complex network models and the introduction of heterogeneity parameters.
In conclusion, in the model proposed in this paper, we systematically study the effects of some main parameters on the oscillations of E and I by mathematical derivation, bifurcation analysis and numerical simulation. The advantage of a mathematical formula is that it can directly describe the relationship between various parameters, can quickly simulate the critical conditions of oscillation and is convenient for theoretical mechanism analysis. The theoretical boundary conditions obtained in this paper are consistent with the trend of bifurcation analysis, which can better explain the mechanism of oscillation origin. The symptoms of Parkinson's patients vary from person to person, and the time delay and coupling strength are two key parameters to describe the interaction between neurons. The results obtained in this paper can help further uncover the pathogenesis of Parkinson's disease and provide guidance for the development of experiments.
This research was supported by the National Science Foundation of China (Grant No. 11602092), the Natural Science Foundation of Hubei Province (Grant No. 2018CFB628) and the China Postdoctoral Science Foundation (No. 2018M632184).
The authors have no relevant financial or nonfinancial interests to disclose.
[1] |
E. Wressle, C. Engstrand, A. K. Granérus, Living with Parkinson's disease: elderly patients' and relatives' perspective on daily living, Aust. Occup. Ther. J., 54 (2007), 131–139. https://doi.org/10.1111/j.1440-1630.2006.00610.x doi: 10.1111/j.1440-1630.2006.00610.x
![]() |
[2] |
P. Mahlknecht, A. Gasperi, P. Willeit, S. Kiechl, H. Stockner, J. Willeit, et al., Prodromal Parkinson's disease as defined per MDS research criteria in the general elderly community, Mov. Disord., 31 (2016), 1405–1408. https://doi.org/10.1002/mds.26674 doi: 10.1002/mds.26674
![]() |
[3] |
M. Politis, K. Wu, S. Molloy, P. G. Bain, K. R. Chaudhuri, P. Piccini, Parkinson's disease symptoms: the patient's perspective, Mov. Disord., 25 (2010), 1646–1651. https://doi.org/10.1002/mds.23135 doi: 10.1002/mds.23135
![]() |
[4] |
C. Váradi, Clinical features of Parkinson's disease: the evolution of critical symptoms, Biology, 9 (2020), 103. https://doi.org/10.3390/biology9050103 doi: 10.3390/biology9050103
![]() |
[5] |
D. J. Surmeier, Determinants of dopaminergic neuron loss in Parkinson's disease, FEBS J., 285 (2018), 3657–3668. https://doi.org/10.1111/febs.14607 doi: 10.1111/febs.14607
![]() |
[6] |
C. Raza, R. Anjum, Parkinson's disease: Mechanisms, translational models and management strategies, Life Sci., 226 (2019), 77–90. https://doi.org/10.1016/j.lfs.2019.03.057 doi: 10.1016/j.lfs.2019.03.057
![]() |
[7] |
A. B. Holt, E. Kormann, A. Gulberti, M. Pötter-Nerger, C. G. McNamara, H. Cagnan, et al., Phase-dependent suppression of beta oscillations in Parkinson's disease patients, J. Neurosci., 39 (2019), 1119–1134. https://doi.org/10.1523/JNEUROSCI.1913-18.2018 doi: 10.1523/JNEUROSCI.1913-18.2018
![]() |
[8] |
A. Singh, R. C. Cole, A. I. Espinoza, D. Brown, J. F. Cavanagh, N. S. Narayanana, Frontal theta and beta oscillations during lower-limb movement in Parkinson's disease, Clin. Neurophysiol., 131 (2020), 694-702. https://doi.org/10.1016/j.clinph.2019.12.399 doi: 10.1016/j.clinph.2019.12.399
![]() |
[9] |
M. H. Trager, M. M. Koop, A. Velisar, Z. Blumenfeld, J. S. Nikolau, E. J. Quinn, et al., Subthalamic beta oscillations are attenuated after withdrawal of chronic high frequency neurostimulation in Parkinson's disease, Neurobiol. Dis., 96 (2016), 22–30. https://doi.org/10.1016/j.nbd.2016.08.003 doi: 10.1016/j.nbd.2016.08.003
![]() |
[10] |
C. Hammond, H. Bergman, P. Brown, Pathological synchronization in Parkinson's disease: networks, models and treatments, Trends Neurosci., 30 (2007), 357–364. https://doi.org/10.1016/j.tins.2007.05.004 doi: 10.1016/j.tins.2007.05.004
![]() |
[11] |
Z. Wang, B. Hu, W. Zhou, M. Xu, D. Wang, Hopf bifurcation mechanism analysis in an improved cortex-basal ganglia network with distributed delays: An application to Parkinson's disease, Chaos, Solitons Fractals, 166 (2023), 113022. https://doi.org/10.1016/j.chaos.2022.113022 doi: 10.1016/j.chaos.2022.113022
![]() |
[12] |
B. Hu, X. Diao, H. Guo, et al., The beta oscillation conditions in a simplified basal ganglia network, Cogn Neurodynamics, 13(2019), 201-217. https://doi.org/10.1007/s11571-018-9514-0 doi: 10.1007/s11571-018-9514-0
![]() |
[13] |
A. B. Holt, T. I. Netoff, Origins and suppression of oscillations in a computational model of Parkinson's disease, J. Comput. Neurosci., 37 (2014), 505–521. https://doi.org/10.1007/s10827-014-0523-7 doi: 10.1007/s10827-014-0523-7
![]() |
[14] |
A. B. Holt, E. Kormann, A. Gulberti, M. Pötter-Nerger, C. G. McNamara, H. Cagnan, et al., Phase-dependent suppression of beta oscillations in Parkinson's disease patients, J. Neurosci., 39 (2019), 1119–1134. https://doi.org/10.1523/JNEUROSCI.1913-18.2018 doi: 10.1523/JNEUROSCI.1913-18.2018
![]() |
[15] |
L. L. Grado, M. D. Johnson, T. I. Netoff, Bayesian adaptive dual control of deep brain stimulation in a computational model of Parkinson's disease, PLoS Comput. Biol., 14 (2018), e1006606. https://doi.org/10.1371/journal.pcbi.1006606 doi: 10.1371/journal.pcbi.1006606
![]() |
[16] |
J. E. Fleming, E. Dunn, M. M. Lowery, Simulation of closed-loop deep brain stimulation control schemes for suppression of pathological beta oscillations in Parkinson's disease, Front. Neurosci., 14 (2020), 166. https://doi.org/10.3389/fnins.2020.00166 doi: 10.3389/fnins.2020.00166
![]() |
[17] |
A. B. Holt, D. Wilson, M. Shinn, J. Moehlis, T. I. Netoff, Phasic burst stimulation: a closed-loop approach to tuning deep brain stimulation parameters for Parkinson's disease, PLoS Comput. Biol., 12 (2016), e1005011. https://doi.org/10.1371/journal.pcbi.1005011 doi: 10.1371/journal.pcbi.1005011
![]() |
[18] |
K. Kumaravelu, D. T. Brocker, W. M. Grill, A biophysical model of the cortex-basal ganglia-thalamus network in the 6-OHDA lesioned rat model of Parkinson's disease, J. Comput. Neurosci., 40 (2016), 207–229. https://doi.org/10.1007/s10827-016-0593-9 doi: 10.1007/s10827-016-0593-9
![]() |
[19] |
A. J. N. Holgado, J. R. Terry, R. Bogacz, Conditions for the generation of beta oscillations in the subthalamic nucleus-globus pallidus network, J. Neurosci., 30 (2010), 12340–12352. https://doi.org/10.1523/JNEUROSCI.0817-10.2010 doi: 10.1523/JNEUROSCI.0817-10.2010
![]() |
[20] |
A. Pavlides, S. J. Hogan, R. Bogacz, Improved conditions for the generation of beta oscillations in the subthalamic nucleus-globus pallidus network, Eur. J. Neurosci., 36 (2012), 2229–2239. https://doi.org/10.1111/j.1460-9568.2012.08105.x doi: 10.1111/j.1460-9568.2012.08105.x
![]() |
[21] |
Gillies, D. Willshaw, Z. Li, Subthalamic-pallidal interactions are critical in determining normal and abnormal functioning of the basal ganglia, Proc. R. Soc. London, Ser. B, 269 (2002), 545–551. https://doi.org/10.1098/rspb.2001.1817 doi: 10.1098/rspb.2001.1817
![]() |
[22] |
J. E. Rubin, D. Terman, High frequency stimulation of the subthalamic nucleus eliminates pathological thalamic rhythmicity in a computational model, J. Comput. Neurosci., 16 (2004), 211–235. https://doi.org/10.1023/B:JCNS.0000025686.47117.67 doi: 10.1023/B:JCNS.0000025686.47117.67
![]() |
[23] |
D. Terman, J. E. Rubin, A. C. Yew, C. J. Wilson, Activity patterns in a model for the subthalamopallidal network of the basal ganglia, J. Neurosci., 22 (2002), 2963–2976. https://doi.org/10.1523/JNEUROSCI.22-07-02963.2002 doi: 10.1523/JNEUROSCI.22-07-02963.2002
![]() |
[24] |
B. Hu, M. Xu, L. Zhu, J. Lin, Z. Wang, D. Wang, et al., A bidirectional Hopf bifurcation analysis of Parkinson's oscillation in a simplified basal ganglia model, J. Theor. Biol., 536 (2022), 110979. https://doi.org/10.1016/j.jtbi.2021.110979 doi: 10.1016/j.jtbi.2021.110979
![]() |
[25] |
S. R. Cole, R. van der Meij, E. J. Peterson, C. de Hemptinne, P. A. Starr, B. Voytek, Nonsinusoidal beta oscillations reflect cortical pathophysiology in Parkinson's disease, J. Neurosci., 37 (2017), 4830–4840. https://doi.org/10.1523/JNEUROSCI.2208-16.2017 doi: 10.1523/JNEUROSCI.2208-16.2017
![]() |
[26] |
B. Pollok, V. Krause, W. Martsch, C. Wach, A. Schnitzler, M. Südmeyer, Motor‐cortical oscillations in early stages of Parkinson's disease, J.Physiol., 590 (2012), 3203–3212. https://doi.org/10.1113/jphysiol.2012.231316 doi: 10.1113/jphysiol.2012.231316
![]() |
[27] |
S. J. van Albada, P. A. Robinson, Mean-field modeling of the basal ganglia-thalamocortical system. I: Firing rates in healthy and parkinsonian states, J. Theor. Biol., 257 (2009), 642–663. https://doi.org/10.1016/j.jtbi.2008.12.018 doi: 10.1016/j.jtbi.2008.12.018
![]() |
[28] |
S. J. van Albada, R. T. Gray, P. M. Drysdale, P. A. Robinson, Mean-field modeling of the basal ganglia-thalamocortical system. Ⅱ: dynamics of parkinsonian oscillations, J. Theor. Biol., 257 (2009), 664–688. https://doi.org/10.1016/j.jtbi.2008.12.013 doi: 10.1016/j.jtbi.2008.12.013
![]() |
[29] |
G. W. Arbuthnott, M. Garcia-Munoz, Are the symptoms of parkinsonism cortical in origin?, Comput. Struct. Biotechnol. J., 15 (2017), 21–25. https://doi.org/10.1016/j.csbj.2016.10.006 doi: 10.1016/j.csbj.2016.10.006
![]() |
[30] |
C. F. Underwood, L. C. Parr-Brownlie, Primary motor cortex in Parkinson's disease: Functional changes and opportunities for neurostimulation, Neurobiol. Dis., 147 (2021), 105159. https://doi.org/10.1016/j.nbd.2020.105159 doi: 10.1016/j.nbd.2020.105159
![]() |
[31] |
M. D. Bevan, P. J. Magill, D. Terman, J. P. Bolam, C. J Wilson, Move to the rhythm: oscillations in the subthalamic nucleus-external globus pallidus network, Trends Neurosci., 25 (2002), 525–531. https://doi.org/10.1016/S0166-2236(02)02235-X doi: 10.1016/S0166-2236(02)02235-X
![]() |
[32] |
A. Pavlides, S. J. Hogan, R. Bogacz, Computational models describing possible mechanisms for generation of excessive beta oscillations in Parkinson's disease, PLoS Comput. Biol., 11 (2015), e1004609. https://doi.org/10.1371/journal.pcbi.1004609 doi: 10.1371/journal.pcbi.1004609
![]() |
[33] |
Y. Chen, J. Wang, Y. Kang, M. B. Ghori, Emergence of beta oscillations of a resonance model for Parkinson's disease, Neural Plast., 2020 (2020), 1–15. https://doi.org/10.1155/2020/8824760 doi: 10.1155/2020/8824760
![]() |
[34] |
M. M. McGregor, A. B. Nelson, Circuit mechanisms of Parkinson's disease, Neuron, 101 (2019), 1042–1056. https://doi.org/10.1016/j.neuron.2019.03.004 doi: 10.1016/j.neuron.2019.03.004
![]() |
[35] |
M. D. Humphries, J. A. Obeso, J. K. Dreyer, Insights into Parkinson's disease from computational models of the basal ganglia, J. Neurol., Neurosurg. Psychiatry, 89 (2018), 1181–1188. https://doi.org/10.1136/jnnp-2017-315922 doi: 10.1136/jnnp-2017-315922
![]() |
[36] |
B. C. M. van Wijk, H. Cagnan, V. Litvak, V. Litvak, A. A. Kühn, K. J. Friston, et al., Generic dynamic causal modelling: An illustrative application to Parkinson's disease, NeuroImage, 181 (2018), 818–830. https://doi.org/10.1016/j.neuroimage.2018.08.039 doi: 10.1016/j.neuroimage.2018.08.039
![]() |
[37] |
M. C. Chen, L. Ferrari, M. D. Sacchet, L. C. Foland-Ross, M. Qiu, I. H. Gotlib, et al., Identification of a direct GABA ergic pallidocortical pathway in rodents, Eur. J. Neurosci., 41 (2015), 748–759. https://doi.org/10.1111/ejn.12822 doi: 10.1111/ejn.12822
![]() |
[38] |
A. Saunders, I. A. Oldenburg, V. K. Berezovskii, C. A. Johnson, N. D. Kingery, H. L. Elliott, et al., A direct GABAergic output from the basal ganglia to frontal cortex, Nature, 521 (2015), 85–89. https://doi.org/10.1038/nature14179 doi: 10.1038/nature14179
![]() |
[39] | P. R. Castillo, E. H. Middlebrooks, S. S. Grewal, L. Okromelidze, J. F. Meschia, A. Quinones-Hinojosa, et al., Globus pallidus externus deep brain stimulation treats insomnia in a patient with Parkinson disease, in Mayo Clinic Proceedings, Elsevier, 95 (2020), 419–422. https://doi.org/10.1016/j.mayocp.2019.11.020 |
[40] |
J. Dong, S. Hawes, J. Wu, W. Le, H. Cai, Connectivity and functionality of the globus pallidus externa under normal conditions and Parkinson's disease, Front. Neural Circuits, 15 (2021), 8. https://doi.org/10.3389/fncir.2021.645287 doi: 10.3389/fncir.2021.645287
![]() |
[41] |
T. Tsuboi, M. Charbel, D. T. Peterside, M. Rana, A. Elkouzi, W. Deeb, et al., Pallidal connectivity profiling of stimulation-induced dyskinesia in Parkinson's disease, Mov. Disord., 36 (2021), 380–388. https://doi.org/10.1002/mds.28324 doi: 10.1002/mds.28324
![]() |
[42] |
R. G. Burciu, D. E. Vaillancourt, Imaging of motor cortex physiology in Parkinson's disease, Mov. Disord., 33 (2018), 1688–1699. https://doi.org/10.1002/mds.102 doi: 10.1002/mds.102
![]() |
[43] |
G. Foffani, J. A. Obeso, A cortical pathogenic theory of Parkinson's disease, Neuron, 99 (2018), 1116–1128. https://doi.org/10.1016/j.neuron.2018.07.028 doi: 10.1016/j.neuron.2018.07.028
![]() |
[44] |
A. Guerra, D. Colella, M. Giangrosso, A. Cannavacciuolo, G. Paparella, G. Fabbrini, et al., Driving motor cortex oscillations modulates bradykinesia in Parkinson's disease, Brain, 145 (2022), 224–236. https://doi.org/10.1093/brain/awab257 doi: 10.1093/brain/awab257
![]() |
[45] |
Z. Wang, B. Hu, L. Zhu, J, Lin, M. Xu, D. Wang, Hopf bifurcation analysis for Parkinson oscillation with heterogeneous delays: A theoretical derivation and simulation analysis, Commun. Nonlinear. Sci., 114 (2022), 106614. https://doi.org/10.1016/j.cnsns.2022.106614 doi: 10.1016/j.cnsns.2022.106614
![]() |
[46] |
T. P. Vogels, K. Rajan, L. F. Abbott, Neural network dynamics, Annu. Rev. Neurosci., 28 (2005), 357–376. https://doi.org/10.1146/annurev.neuro.28.061604.135637 doi: 10.1146/annurev.neuro.28.061604.135637
![]() |
[47] |
H. Kita, Y. Tachibana, A. Nambu, S. Chiken, Balance of monosynaptic excitatory and disynaptic inhibitory responses of the globus pallidus induced after stimulation of the subthalamic nucleus in the monkey, J. Neurosci., 25 (2005), 8611–8619. https://doi.org/10.1523/JNEUROSCI.1719-05.2005 doi: 10.1523/JNEUROSCI.1719-05.2005
![]() |
[48] |
J. T. Paz, J. M. Deniau, S. Charpier, Rhythmic bursting in the cortico-subthalamo-pallidal network during spontaneous genetically determined spike and wave discharges, J. Neurosci., 25 (2005), 2092–2101. https://doi.org/10.1523/JNEUROSCI.4689-04.2005 doi: 10.1523/JNEUROSCI.4689-04.2005
![]() |
[49] |
H. Kita, S. T. Kitai, Intracellular study of rat globus pallidus neurons: membrane properties and responses to neostriatal, subthalamic and nigral stimulation, Brain Res., 564 (1991), 296–305. https://doi.org/10.1016/0006-8993(91)91466-E doi: 10.1016/0006-8993(91)91466-E
![]() |
[50] |
M. A. Lebedev, S. P. Wise, Oscillations in the premotor cortex: single-unit activity from awake, behaving monkeys, Exp. Brain Res., 130 (2000), 195–215. https://doi.org/10.1007/s002210050022 doi: 10.1007/s002210050022
![]() |
[51] |
W. Schultz, R. Romo, Neuronal activity in the monkey striatum during the initiation of movements, Exp. Brain Res., 71 (1988), 431–436. https://doi.org/10.1007/BF00247503 doi: 10.1007/BF00247503
![]() |
[52] |
N. E. Hallworth, C. J. Wilson, M. D. Bevan, Apamin-sensitive small conductance calcium-activated potassium channels, through their selective coupling to voltage-gated calcium channels, are critical determinants of the precision, pace, and pattern of action potential generation in rat subthalamic nucleus neurons in vitro, J. Neurosci., 23 (2003), 7525–7542. https://doi.org/10.1523/JNEUROSCI.23-20-07525.2003 doi: 10.1523/JNEUROSCI.23-20-07525.2003
![]() |
[53] |
H. Kita, Globus pallidus external segment, Prog. Brain Res., 160 (2007), 111–133. https://doi.org/10.1016/S0079-6123(06)60007-1 doi: 10.1016/S0079-6123(06)60007-1
![]() |
[54] |
H. Kita, A. Nambu, K. Kaneda, Y. Tachibana, M. Takada, Role of ionotropic glutamatergic and GABAergic inputs on the firing activity of neurons in the external pallidum in awake monkeys, J. Neurophysiol., 92 (2004), 3069–3084. https://doi.org/10.1152/jn.00346.2004 doi: 10.1152/jn.00346.2004
![]() |
[55] |
H. Nakanishi, H. Kita, S. T. Kitai, Intracellular study of rat substantia nigra pars reticulata neurons in an in vitro slice preparation: electrical membrane properties and response characteristics to subthalamic stimulation, Brain Res., 437 (1987), 45–55. https://doi.org/10.1016/0006-8993(87)91525-3 doi: 10.1016/0006-8993(87)91525-3
![]() |
[56] |
K. Fujimoto, H. Kita, Response characteristics of subthalamic neurons to the stimulation of the sensorimotor cortex in the rat, Brain Res., 609 (1993), 185–192. https://doi.org/10.1016/0006-8993(93)90872-K doi: 10.1016/0006-8993(93)90872-K
![]() |
[57] |
A. Gillies, D. Willshaw, Membrane channel interactions underlying rat subthalamic projection neuron rhythmic and bursting activity, J. Neurophysiol., 95 (2006), 2352–2365. https://doi.org/10.1152/jn.00525.2005 doi: 10.1152/jn.00525.2005
![]() |
[58] |
H. Kita, S. T. Kitai, Intracellular study of rat globus pallidus neurons: membrane properties and responses to neostriatal, subthalamic and nigral stimulation, Brain Res., 564 (1991), 296–305. https://doi.org/10.1016/0006-8993(91)91466-E doi: 10.1016/0006-8993(91)91466-E
![]() |
[59] |
Y. Hirai, M. Morishima, F. Karube, Y. Kawaguchi, Specialized cortical subnetworks differentially connect frontal cortex to parahippocampal areas, J. Neurosci., 32 (2012), 1898–1913. https://doi.org/10.1523/JNEUROSCI.2810-11.2012 doi: 10.1523/JNEUROSCI.2810-11.2012
![]() |
[60] |
Y. H. Tanaka, Y. Tanaka, F. Fujiyama, T. Furuta, Y. Yanagawa, T. Kaneko, Local connections of layer 5 GABAergic interneurons to corticospinal neurons, Front. Neural Circuits, 5 (2011), 12. https://doi.org/10.3389/fncir.2011.00012 doi: 10.3389/fncir.2011.00012
![]() |
[61] |
M. H. Higgs, S. J. Slee, W. J. Spain, Diversity of gain modulation by noise in neocortical neurons: regulation by the slow afterhyperpolarization conductance, J. Neurosci., 26 (2006), 8787–8799. https://doi.org/10.1523/JNEUROSCI.1792-06.2006 doi: 10.1523/JNEUROSCI.1792-06.2006
![]() |
[62] |
A. L. Barth, J. F. A. Poulet, Experimental evidence for sparse firing in the neocortex, Trends Neurosci., 35 (2012), 345–355. https://doi.org/10.1016/j.tins.2012.03.008 doi: 10.1016/j.tins.2012.03.008
![]() |
[63] |
A. P. Prudnikov, Y. A. Brychkov, O. I. Marichev, Integrals and series: direct laplace transforms, Routledge, 2018. https://doi.org/10.1201/9780203750643 doi: 10.1201/9780203750643
![]() |
[64] |
K. Udupa, N. Bahl, Z. Ni, C. Gunraj, F. Mazzella, E. Moro, et al., Cortical plasticity induction by pairing subthalamic nucleus deep-brain stimulation and primary motor cortical transcranial magnetic stimulation in Parkinson's disease, J. Neurosci., 36 (2016), 396–404. https://doi.org/10.1523/JNEUROSCI.2499-15.2016 doi: 10.1523/JNEUROSCI.2499-15.2016
![]() |
[65] |
M. Dagan, T. Herman, R. Harrison, J. Zhou, N. Giladi, G. Ruffini, et al., Multitarget transcranial direct current stimulation for freezing of gait in Parkinson's disease, Mov. Disord., 33 (2018), 642–646. https://doi.org/10.1002/mds.27300 doi: 10.1002/mds.27300
![]() |
[66] |
E. Lattari, S. S. Costa, C. Campos, A. J. Oliveira, S. Machado, G. A. M. Neto, Can transcranial direct current stimulation on the dorsolateral prefrontal cortex improves balance and functional mobility in Parkinson's disease?, Neurosci. Lett., 636 (2017), 165–169. https://doi.org/10.1016/j.neulet.2016.11.019 doi: 10.1016/j.neulet.2016.11.019
![]() |
Parameter | Value | Parameter | Value |
τS | 13 ms | τG | 20.3 ms |
τE | 12.1 ms | τI | 14.7 ms |
MS | 300 spk/s | MG | 400 spk/s |
ME | 75 spk/s | MI | 310 spk/s |
BS | 8.1 spk/s | BG | 19 spk/s |
BE | 5.5 spk/s | BI | 16.58 spk/s |
Str | 2.12 spk/s | C | 17.1 spk/s |
T | 6.12 ms |
Parameter | Value |
wGS | 10.63 |
wCS | 9.15 |
wSG | 20.12 |
wGG | 11.96 |
wXG | 135.1 |
wIE | 3.22 |
wEI | 2.97 |
wGE | 14.96 |
wCE | 27.18 |
wGI | 5.35 |
Parameter | Value | Parameter | Value |
τS | 13 ms | τG | 20.3 ms |
τE | 12.1 ms | τI | 14.7 ms |
MS | 300 spk/s | MG | 400 spk/s |
ME | 75 spk/s | MI | 310 spk/s |
BS | 8.1 spk/s | BG | 19 spk/s |
BE | 5.5 spk/s | BI | 16.58 spk/s |
Str | 2.12 spk/s | C | 17.1 spk/s |
T | 6.12 ms |
Parameter | Value |
wGS | 10.63 |
wCS | 9.15 |
wSG | 20.12 |
wGG | 11.96 |
wXG | 135.1 |
wIE | 3.22 |
wEI | 2.97 |
wGE | 14.96 |
wCE | 27.18 |
wGI | 5.35 |