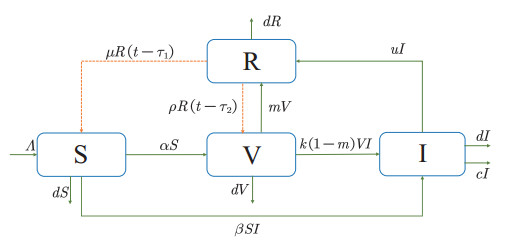
Since the outbreak of COVID-19, there has been widespread concern in the community, especially on the recent heated debate about when to get the booster vaccination. In order to explore the optimal time for receiving booster shots, here we construct an SVIR model with two time delays based on temporary immunity. Second, we theoretically analyze the existence and stability of equilibrium and further study the dynamic properties of Hopf bifurcation. Then, the statistical analysis is conducted to obtain two groups of parameters based on the official data, and numerical simulations are carried out to verify the theoretical analysis. As a result, we find that the equilibrium is locally asymptotically stable when the booster vaccination time is within the critical value. Moreover, the results of the simulations also exhibit globally stable properties, which might be more beneficial for controlling the outbreak. Finally, we propose the optimal time of booster vaccination and predict when the outbreak can be effectively controlled.
Citation: Zimeng Lv, Xinyu Liu, Yuting Ding. Dynamic behavior analysis of an SVIR epidemic model with two time delays associated with the COVID-19 booster vaccination time[J]. Mathematical Biosciences and Engineering, 2023, 20(4): 6030-6061. doi: 10.3934/mbe.2023261
[1] | Chuanqing Xu, Xiaotong Huang, Zonghao Zhang, Jing'an Cui . A kinetic model considering the decline of antibody level and simulation about vaccination effect of COVID-19. Mathematical Biosciences and Engineering, 2022, 19(12): 12558-12580. doi: 10.3934/mbe.2022586 |
[2] | Bruce Pell, Matthew D. Johnston, Patrick Nelson . A data-validated temporary immunity model of COVID-19 spread in Michigan. Mathematical Biosciences and Engineering, 2022, 19(10): 10122-10142. doi: 10.3934/mbe.2022474 |
[3] | Shishi Wang, Yuting Ding, Hongfan Lu, Silin Gong . Stability and bifurcation analysis of SIQR for the COVID-19 epidemic model with time delay. Mathematical Biosciences and Engineering, 2021, 18(5): 5505-5524. doi: 10.3934/mbe.2021278 |
[4] | Hongfan Lu, Yuting Ding, Silin Gong, Shishi Wang . Mathematical modeling and dynamic analysis of SIQR model with delay for pandemic COVID-19. Mathematical Biosciences and Engineering, 2021, 18(4): 3197-3214. doi: 10.3934/mbe.2021159 |
[5] | Xinyu Liu, Zimeng Lv, Yuting Ding . Mathematical modeling and stability analysis of the time-delayed SAIM model for COVID-19 vaccination and media coverage. Mathematical Biosciences and Engineering, 2022, 19(6): 6296-6316. doi: 10.3934/mbe.2022294 |
[6] | Sarita Bugalia, Jai Prakash Tripathi, Hao Wang . Mathematical modeling of intervention and low medical resource availability with delays: Applications to COVID-19 outbreaks in Spain and Italy. Mathematical Biosciences and Engineering, 2021, 18(5): 5865-5920. doi: 10.3934/mbe.2021295 |
[7] | Ugo Avila-Ponce de León, Angel G. C. Pérez, Eric Avila-Vales . Modeling the SARS-CoV-2 Omicron variant dynamics in the United States with booster dose vaccination and waning immunity. Mathematical Biosciences and Engineering, 2023, 20(6): 10909-10953. doi: 10.3934/mbe.2023484 |
[8] | Sarafa A. Iyaniwura, Rabiu Musa, Jude D. Kong . A generalized distributed delay model of COVID-19: An endemic model with immunity waning. Mathematical Biosciences and Engineering, 2023, 20(3): 5379-5412. doi: 10.3934/mbe.2023249 |
[9] | A. Q. Khan, M. Tasneem, M. B. Almatrafi . Discrete-time COVID-19 epidemic model with bifurcation and control. Mathematical Biosciences and Engineering, 2022, 19(2): 1944-1969. doi: 10.3934/mbe.2022092 |
[10] | Glenn Ledder . Incorporating mass vaccination into compartment models for infectious diseases. Mathematical Biosciences and Engineering, 2022, 19(9): 9457-9480. doi: 10.3934/mbe.2022440 |
Since the outbreak of COVID-19, there has been widespread concern in the community, especially on the recent heated debate about when to get the booster vaccination. In order to explore the optimal time for receiving booster shots, here we construct an SVIR model with two time delays based on temporary immunity. Second, we theoretically analyze the existence and stability of equilibrium and further study the dynamic properties of Hopf bifurcation. Then, the statistical analysis is conducted to obtain two groups of parameters based on the official data, and numerical simulations are carried out to verify the theoretical analysis. As a result, we find that the equilibrium is locally asymptotically stable when the booster vaccination time is within the critical value. Moreover, the results of the simulations also exhibit globally stable properties, which might be more beneficial for controlling the outbreak. Finally, we propose the optimal time of booster vaccination and predict when the outbreak can be effectively controlled.
The COVID-19 has been the most serious outbreak worldwide since 2000, and it is currently in the prevention and control phase. The novel coronavirus is in lineage B of the genus β-coronavirus of the coronavirus family, in which severe acute respiratory syndrome-related coronavirus (SARS-CoV) and Middle East respiratory syndrome-related coronavirus (MERS-CoV) are also included. These pathogens are enveloped positive-sense RNA viruses that are widespread in mammals, including humans, and they can destroy the respiratory systems of humans and cause severe acute respiratory syndrome [1,2,3]. Despite global vaccination efforts, the epidemic situation is still critical for two main reasons. One reason is that the COVID-19 vaccination proportion remains low in some countries, such as low-income countries in Africa [4], leading to a lack of global immune barriers. Another issue that cannot be ignored is that the antibodies produced in the body do not fully defeat variable strains, and the effectiveness of antibodies decreases as the virus mutates [5]. According to data published by the Israeli Ministry of Health, the protective effect of the Pfizer COVID-19 vaccine decreases after 6 months of vaccination. Based on available statistics, it has been confirmed that some people who have been vaccinated against COVID-19 have been diagnosed, and this number is increasing. Therefore, there has been a global consensus to implement the booster vaccinations. By receiving the booster vaccine, a strong immune response can be rapidly produced in the body, and the level of neutralizing antibodies can significantly increase, thereby improving the overall immunity level and achieving outbreak control.
In 1927, Kermack and McKendrick first used dynamic research methods to study the spread of infectious diseases [6]. Since then, an increasing number of researchers have engaged in mathematical modeling of infectious diseases considering their transmission mechanisms and control measures [7,8,9,10]. Vaccination and even booster shots were added to the model to study the dynamic behaviors of the corresponding infectious diseases [11,12,13]. In particular, since the outbreak of COVID-19, researchers worldwide have been very concerned, and corresponding infectious disease models have been developed to simulate epidemic trends to provide policy recommendations [14,15,16,17,18,19]. The models are not limited to ordinary differential equations (ODEs), and the use of partial differential equations (PDEs) is also a good way to study such problems [20,21,22].
During the early stage of the outbreak, the treatment process was difficult because people had never been exposed to this disease. Although we have better research on treatments and have continued to learn about the disease, studies have shown that COVID-19 drugs have side effects on our bodies [23,24]. Therefore, vaccination is considered an effective strategy to prevent COVID-19 and control the outbreak. Thus, as vaccines gradually enter clinical trials and continue to spread worldwide, an increasing number of scholars have added vaccination to their models[25,26]. Given the current situation, most people have been vaccinated, but the mutations may lead to the failure of original antibodies. To maintain sustained immunity, it is feasible and meaningful to vaccinate with booster shots [27].
Adding the necessary "time delay" to the model tends to make it more realistic. Sometimes, the model is sufficient to describe facts and phenomena accurately even with only one time delay [28,29,30,31]. However, sometimes, one time delay is not enough to describe complex phenomena fully, and double time delays or even multiple time delays are necessary [32,33,34,35,36,37,38]. When we add the effect of booster vaccination to the epidemic model, there are two periods of time that we cannot ignore: the time of antibody failure and the time of booster vaccination. Therefore, we introduce two time delays into the model to describe the problem better.
For differential equation models, stability and Hopf bifurcation analysis have always been popular research topics. Mahata et al. [39] studied a fractional-order dynamical system of Susceptible-Exposed-Infected-Recovered-Vaccinated (SEIRV) in the infectious population. The stability of all equilibria was analyzed with respect to the delay parameter, and threshold values of delay were found, beyond which the system exhibited Hopf bifurcation and the solutions were no longer periodic. Zhang et al. [40] studied an epidemic model involving nonlinear birth in population and vertical transmission. They discussed the existence of Hopf bifurcation and obtained the stability and direction of Hopf bifurcation by using normal form theory and center manifold theorem. References [41,42,43,45,46,47] discussed different dynamic properties of Hopf bifurcation in different systems. We hope that we can also analyze the dynamic properties after establishing the model and use the results to make reasonable recommendations for vaccination.
The motivations of our study are as follows. First, although COVID-19 vaccines have shown good protective efficacy and great safety in both clinical trials and real-life applications, there are some cases of infection in people who have been vaccinated, suggesting that vaccine-induced immunity is not permanent. Therefore, booster vaccination is necessary and we need to take this into account in our model. Second, there is a time delay between booster vaccination and initial vaccination, which has a profound impact on the development of the epidemic. Different booster vaccination time will cause different phenomena in the model, which is essential to understand the development of epidemic and to formulate the effective policies. Third, when is the booster vaccination most beneficial for the development of the epidemic when taking into account the economy, national power and medical level? What measures should we take to minimize the impact of the epidemic on human life? Finally, COVID-19 exhibits a constantly and dynamically evolving mutational landscape, with relatively abundant genetic diversity and a high evolutionary capability over time[48,49,50]. As the virus continues to mutate, what effect will this have on the time of booster vaccination?
Through the study of this paper, we have a more accurate grasp of when to carry out booster vaccination. By simulating the current epidemic state, a reasonable time range for booster vaccination is proposed, which can ensure that the epidemic can reach a stable state instead of getting out of control. This is meaningful to the formulation of epidemic prevention and control policies.
This paper is organized as follows. In Section 2, we establish a delayed differential system with two time delays to study the impact of vaccination on the epidemic and determine the optimal time for the booster vaccination. In Section 3, we analyze the conditions for the existence and stability of equilibria and the existence of Hopf bifurcation. Next, the normal form of Hopf bifurcation is deduced by using the multiple time scales method in Section 4. In Section 5, numerical simulations are carried out to verify the theoretical analysis based on the parameters through statistical analysis. Finally, we draw conclusions about the optimal time range of booster vaccination by combining all aspects and provide some reasonable suggestions in Section 6.
In the traditional model of infectious disease, susceptible people (S) can be infected through contact with a source of infection, which converts them into infected people (I). With treatment, those infected people can be cured and then become recovered people (R) with antibodies. Considering the characteristics of COVID-19, vaccination has become the global trend, and vaccinated people have become a large group that cannot be ignored. Therefore, we need to represent them as a separate compartment denoted as vaccinated people (V), which is a more accurate representation of the current outbreak. However, it is important to note that vaccinated people (V) are those who have completed the vaccination but have not fully developed antibodies. This group of people is still at risk of being infected. For those who have fully developed antibodies, we classify them as recovered people (R) since the process of producing antibodies is similar to the mechanism of cure after infection. This can be understood as recovering from the risk of infection. Combined with the above considerations, we choose the Susceptible-Vaccinated-Infective-Recovered (SVIR) model for our study of COVID-19 with booster vaccination.
Similar to the traditional SIR model, susceptible people (S) will be transformed into infected people (I) when they effectively contact I. Infected people (I) will be transformed into recovered people (R) after healing through treatment. As schematically shown in Figure 1, after vaccination, susceptible people (S) can be transformed into vaccinated people (V). However, not all vaccinated people are able to complete the immune response and further produce antibodies. For those who are able to produce the immune response (mV), we also classify them as recovered people (R) since they share the same characteristics as those who are cured after infection. By contrast, those who cannot produce antibodies due to individual variability ((1−m)V) are clearly at risk of infection with an infection rate of k. Therefore, the term to transfer from V to I is k(1−m)VI. In the case of COVID-19, even though antibodies have been produced in the body, this immunity is temporary. After a period of time, recovered people (R) are faced with losing immunity, thus returning to susceptible people. We call this "antibody failure". However, if the booster vaccination is given before antibody failure, there is a return to vaccinated people (V) and thus a chance to reproduce the immune response.
In our model, we consider antibody failure in two main ways, partly because antibody levels decrease significantly over time and partly because the efficiency of existing antibodies against mutated strains is greatly reduced due to virus mutations. Both of these conditions increase the risk of infection. At the time of booster vaccination, the antibody level decreases compared to before and mutated strains are produced. Therefore, we believe that the current antibodies are much less efficient at the time of booster vaccination. For different mutated strains, booster shots are developed for their specific characteristics. Due to individual differences, it may occur that some people produce antibodies completely with the first vaccination but do not complete the immune response after booster vaccination, increasing the risk of infection. The specific definitions of variables and parameters are given in Table 1, and the rates are all expressed as the average over a year.
Symbol | Descriptions | Unit |
S | Number of suspected people | 105 persons |
V | Number of vaccinated people | 105 persons |
I | Number of infected people | 105 persons |
R | Number of recovered people | 105 persons |
Λ | Population nature growth | 105 persons |
d | Population natural mortality rate | – |
c | COVID-19 mortality rate of I | – |
β | Infection rate from S to I | – |
k | Proportional coefficient of transmission | – |
α | Vaccination rate | – |
m | Transmission rate from V to R | – |
ρ | Booster vaccination rate | – |
μ | Vaccine failure rate | – |
u | Cure rate | – |
Since we want to know when the booster vaccination is necessary and when it is most effective, we let τ2 represent the time delay between R and V for booster vaccination and simulate different vaccination time by changing the size of τ2. However, in the case of COVID-19 vaccines, the vaccines are nonpermanent, that is, antibodies produced by the vaccines have a problem of failure. The failure time is not negligible relative to τ2, so we use τ1 to express the time for antibody failure. In order to improve herd immunity and control the epidemic better, booster vaccination usually occurs before antibody failure, so we assume τ2<τ1.
Therefore, the SVIR epidemic model is constructed to explore the optimal booster vaccination time. The equation is presented as follows:
{dSdt=Λ+μR(t−τ1)−αS−βSI−dS,dVdt=αS+ρR(t−τ2)−mV−dV−k(1−m)VI,dIdt=βSI−dI−uI−cI+k(1−m)VI,dRdt=mV+uI−μR(t−τ1)−ρR(t−τ2)−dR, | (2.1) |
where τ1 is the time delay of antibody failure, τ2 is the time delay of booster vaccination and the specific definitions of variables and parameters are given in Table 1.
For convenience of calculation, we let γ≜k(1−m) in the following text.
The initial condition of system (2.1) is given by S(θ)=ϕ1(θ),V(θ)=ϕ2(θ),I(θ)=ϕ3(θ),R(θ)=ϕ4(θ), θ∈[−τ,0], τ=max{τ1,τ2}, with ϕ=[ϕ1,ϕ2,ϕ3,ϕ4]∈C such that ϕi≥0,i=1,2,3,4 for θ∈[−τ,0] where C denotes the Banach space of continuous functions mapping the interval [−τ,0] into R4+0={(S,V,I,R)|S>0,V>0,I>0,R>0}.
When t∈[0,τ], the solution of system (2.1) is as follows:
S(t)=e−∫t0(α+d+βI)dξ[S(0)+∫t0(Λ+μR(η−τ1))e∫η0(α+d+βI)dξdη],V(t)=e−∫t0(m+d+γV)dξ[A(0)+∫t0(αS(η)+ρR(η−τ2))e∫η0(m+d+γV)dξdη],I(t)=e−∫t0(−βS−γV+d+u+c)dξI(0),R(t)=e−(d)t[R(0)+∫t0(mV(η)+uI(η)−μR(η−τ1)−ρR(η−τ2))e−dηdη]. | (2.2) |
Obviously, I(t)>0. In the current state, the open policy leads to an increase in the number of infected people. The mutation of the virus makes many people choose booster vaccination when they face body failure. Therefore, the numbers of V and I might be higher. Although R(t) is large, R(t−τ1) and R(t−τ2) will not be large compared to the current values since the time delays are in years as a unit. Therefore, it is reasonable to assume that mV+uI−μR(t−τ1)−ρR(t−τ2)>0. Thus, R(t)>0 based on the expression of R(t) in Eq (2.2). Furthermore, we can get R(t−τ1)>0,R(t−τ2)>0 when t∈[0,τ]. Thus, S(t)>0,V(t)>0.
Overall, when t∈[0,τ], all the solutions of system (2.1) are positive after we consider the realistic situation and make some assumptions. Through the similar approach, it can be shown that all solutions of system (2.1) are also positive on [τ,2τ] under some restrictions. This conclusion can be extended to the interval [nτ,(n+1)τ](n∈N). Therefore, for any t>0, all solutions of system (2.1) are positive with the restrictions.
Although the positivity of our model is conditional, our analysis of the epidemic shows that the present state satisfies the conditions for the existence of positivity, therefore our model is reasonable and meaningful.
Let N(t)=S(t)+V(t)+I(t)+R(t), and N(t) represents the total number of people at time t. Adding four equations of system (2.1), we obtain N′(t)=Λ−dN(t)−cI(t). According to the discussion on the positivity of solutions, we can find that I(t)>0. Thus, N′(t)=Λ−dN(t)−cI(t)≤Λ−dN(t). Based on the comparison theorem, we can obtain:
0<N(t)≤e−dt[N(0)+Λ∫t0edηdη]=e−dt[N(0)−Λd]+Λd. |
Therefore, limt→∞supN(t)≤Λd and the solutions S(t), A(t), I(t), R(t) of system (2.1) are bounded when t>0.
The boundedness of the solutions of system (2.1) must hold. Its practical significance is that the number of people in each cabin can be stabilized within a specific range regardless of the current epidemic situation, which is optimistic for controlling the epidemic.
Under the positivity and boundedness of solutions discussed above, we mark the feasible region of the system (2.1) as:
Ω={S(t),A(t),I(t),R(t)|N(t)=S(t)+I(t)+Q(t)+R(t)≤Λd,S(t)≥0,A(t)≥0,I(t)≥0,R(t)≥0,mV+uI−μR(t−τ1)−ρR(t−τ2)>0.} | (2.3) |
Next, we will analyze system (2.1). It has three equilibria,
E1=(S∗1,V∗1,I∗1,R∗1),E2=(S∗2,V∗2,I∗2,R∗2),E3=(S∗3,V∗3,I∗3,R∗3), | (3.1) |
with
S∗1=Λ[m(μ+d)+d(u+d+ρ)]Π>0,V∗1=(μ+ρ+d)αΛΠ>0,I∗1=0,R∗1=αmΛΠ>0,S∗2=d+u+cβ−γβV∗2,V∗2=−A+√A2−4γpB2γp,I∗2=pV∗2+q,R∗2=mV∗2+uI∗2μ+ρ+d,S∗3=d+u+cβ−γβV∗3,V∗3=−A−√A2−4γpB2γp,I∗3=pV∗3+q,R∗3=mV∗3+uI∗3μ+ρ+d,Π=αmμ+d(α+d)(m+u+d+ρ)+mdμ,q=[Λβ−d(d+u+c)](μ+ρ+d)−βu(μ+ρ)+β(d+u+c)(μ+ρ+d),p=β(μ+ρ)m−β(m+d)(μ+ρ+d)+dγ(μ+ρ+d)−βu(μ+ρ)+β(d+u+c)(μ+ρ+d),A=αγβ−ρmμ+ρ+d−ρupμ+ρ+d+m+d+γq,B=−α(d+u+c)β−ρuqμ+ρ+d. |
Then, we show the following assumptions:
(H1)V∗2=−A+√A2−4γpB2rp>0,S∗2=d+u+cβ−γβV∗2>0,I∗2=pV∗2+q>0,(H2)V∗3=−A−√A2−4γpB2rp>0,S∗3=d+u+cβ−γβV∗3>0,I∗3=pV∗3+q>0, |
where A,B,p are given in Eq (3.1). We can easily find that there is always an equilibrium E1. If equilibrium E1 is stable, there are no infected people in the final stable state, that is, the virus can finally be eliminated, which is called the disease-free equilibrium. Then, equilibrium E2 makes sense under (H1) and equilibrium E3 is meaningful under (H2). If equilibrium E2 or E3 is stable, the final state is always accompanied by some infected people, which means that the virus will always coexist with humans and we have no way to eliminate it. We call it the endemic equilibrium.
Without loss of generality, we assume that the system (2.1) has three equilibria Ek(k=1,2,3). Then, we transfer the equilibrium Ek into the original point: ˜S=S−S∗k, ˜V=V−V∗k, ˜I=I−I∗k, ˜R=R−R∗k and re-denote ˜S,˜V,˜I,˜R as S,V,I,R. We obtain the following model:
{dSdt=Λ+μR(t−τ1)−αS−βSI−βS∗kI−βSI∗k−dS,dVdt=αS+ρR(t−τ2)−mV−dV−γVI−γV∗kI−γVI∗k,dIdt=βSI+βS∗kI+βSI∗k−dI−uI−cI+γVI+γV∗kI+γVI∗k,dRdt=mV+uI−μR(t−τ1)−ρR(t−τ2)−dR. | (3.2) |
For the equilibrium E1=(S∗1,V∗1,I∗1,R∗1), we can obtain the characteristic equation of system (3.2) as follows:
(λ−βS∗1−γV∗1+d+u+c)(λ+d)[(λ+m+d)(λ+α+d)+(λ+m+α+d)μe−λτ1+(λ+α+d)ρe−λτ2]=0. | (3.3) |
Similar to the equilibrium E1, we can also obtain the characteristic equation at the equilibrium Ek(k=2,3):
λ4+ϱ1,kλ3+ς2,kλ2+ϱ3,kλ+ϱ4,k+μe−λτ1(λ3+σ1,kλ2+σ2,kλ+σ3,k)+ρe−λτ2(λ3+ς1,kλ2+ς2,kλ+ς3,k)=0, | (3.4) |
where
ϱ1,k=d+Φ2,k,ϱ2,k=dΦ2,k+Φ1,k,ϱ3,k=dΦ1,k+Φ0,k,ϱ4,k=dΦ0,k,σ1,k=Φ2,k,σ2,k=Φ1,k+Ψ1,k,σ3,k=Φ0,k+Ψ0,k,ς1,k=Φ2,k−m,ς2,k=Φ1,k−Ω1,k,ς3=Φ0,k−Ω0,k,Φ2,k=m+d+γI∗k−βS∗k−γV∗k+d+u+c+α+d+βI∗k,Φ1,k=(m+d+γI∗k−βS∗k−γV∗k+d+u+c)(α+d+βI∗k)+(m+d+γI∗k)(−βS∗k−γV∗k+d+u+c)+γ2I∗kV∗k+β2S∗kI∗k,Φ0,k=(α+d+βI∗k)[(m+d+γ∗I∗k)(−βS∗k−γV∗k+d+u+c)+γ2I∗kV∗k]+βS∗k[αγI∗k−βI∗k(m+d+γI∗k)],Ψ1,k=−αm−uβI∗k,Ψ0,k=m[−α(−βS∗k−γV∗k+d+u+c)+βγV∗kI∗k]−u[αγI∗k+βI∗k(m+d+γI∗k)],Ω1,k=m(α+d+βI∗k−βS∗k−γV∗k+d+u+c)+uγI∗k,Ω0,k=m[(α+d+βI∗k)(−βS∗k−γV∗k+d+u+c)+β2S∗kI∗k]+uγI∗k(α+d+βI∗k),k=2,3. |
When τ1=τ2=0, (3.3) becomes:
(λ−βS∗1−γV∗1+d+u+c)(λ+d)[λ2+(m+α+2d+μ+ρ)λ+(m+d)(α+d)+μ(m+α+d)+ρ(α+d)]=0. | (3.5) |
Clearly, Equation (3.5) has the roots λ1=βS∗1+γV∗1−(d+u+c), λ2=−d, and the remaining roots λ3,λ4 are given by the following equation:
λ2+(m+α+2d+μ+ρ)λ+(m+d)(α+d)+μ(m+α+d)+ρ(α+d)=0. | (3.6) |
According to Vieta's theorem, we can obtain λ3+λ4=−(m+α+2d+μ+ρ)<0 and λ3λ4=(m+d)(α+d)+μ(m+α+d)+ρ(α+d)>0, thus, Re(λ3)<0 and Re(λ4)<0. Then, we show the following assumption:
(H3)βS∗1+γV∗1−(d+u+c)<0. |
Under (H3), we can find that all the characteristic roots of Eq (3.5) are negative, so the equilibrium E1 is locally asymptotically stable when τ1=τ2=0. If (H3) does not hold, λ1=βS∗1+γV∗1−(d+u+c)>0, the equilibrium E1 is unstable when τ1=τ2=0.
For the equilibrium Ek(k=2,3), Equation (3.4) is transformed to the following form when τ1=τ2=0:
λ4+a1,kλ3+a2,kλ2+a3,kλ+a4,k=0, | (3.7) |
where a1,k=ϱ1,k+μ+ρ,a2,k=ϱ2,k+μσ1,k+ρς1,k,a3,k=ϱ3,k+μσ2,k+ρς2,k,a4,k=ϱ4,k+μσ3,k+ρς3,k with ϱ1,k, ϱ2,k, ϱ3,k, ϱ4,k, σ1,k, σ2,k, σ3,k, ς1,k, ς2,k, ς3,k given in Eq (3.4). According to Routh-Hurwitz stability criterion, we propose the following hypothesis:
(H4)a1,ka2,k−a3,k>0,a3,k(a1,ka2,k−a3,k)>a21,ka4,k,a4,k>0. |
If (H4) is satisfied, all eigenvalues of Eq (3.7) have negative real parts, the equilibrium Ek(k=2,3) of model (2.1) is locally asymptotically stable when τ1=τ2=0.
Theorem 3.1. For system (2.1) with τ1=0,τ2=0, the stability results for equilibrium Ek(k=1,2,3) are given as follows.
1) Equilibrium E1 always exists. If (H3) holds, equilibrium E1 is locally asymptotically stable. On the contrary, if (H3) does not hold, equilibrium E1 is unstable;
2) When the assumption (H1) or (H2) holds, the equilibrium E2 or E3 is meaningful respectively. Further, if (H4) holds, equilibrium E2 or E3 is locally asymptotically stable. If (H4) does not hold, equilibrium E2 and E3 are unstable.
For the equilibrium E1, when τ2=0,τ1>0, the characteristic equation of system (3.3) becomes:
(λ−βS∗1−γV∗1+d+u+c)(λ+d)[(λ+m+d+ρ)(λ+α+d)+(λ+m+α+d)μe−λτ1]=0. | (3.8) |
If (H3) holds, λ1=βS∗1+γV∗1−(d+u+c)<0, λ2=−d<0. We only need to consider the remaining part of Eq (3.8):
(λ+m+d+ρ)(λ+α+d)+(λ+m+α+d)μe−λτ1=0. | (3.9) |
Substituting λ=iω(ω>0) into Eq (3.9) and separating the real and imaginary parts, we obtain:
{μωsin(ωτ1)+μ(m+α+d)cos(ωτ1)=ω2−(m+d+ρ)(α+d),μ(m+α+d)sin(ωτ1)−μωcos(ωτ1)=ω(α+m+2d+ρ). | (3.10) |
Thus,
{sin(ωτ1)=ω3−(m+α+d)(α+d)ω+(α+m+2d+ρ)(m+α+d)ωμ(m+α+d)2+μω2,cos(ωτ1)=−ω2(d+ρ)−(m+α+d)(m+d+ρ)(α+d)μ(m+α+d)2+μω2. | (3.11) |
Adding the square of the two equations in Eq (3.11) and letting z=ω2, we obtain:
h(z)=z2+κ1z+κ0, | (3.12) |
where κ1=−2(m+d+ρ)(α+d)+(α+m+2d+ρ)2−μ2,κ0=(m+d+ρ)2(α+d)2−mu2(m+α+d)2. Therefore, we show the following assumptions:
(H5)κ0<0,(H6)κ21−4κ0>0,κ1<0,κ0>0. |
If (H5) and (H6) do not hold, the equilibrium E1 is always locally asymptotically stable for any τ1>0. If (H5) holds, Equation (3.12) has the unique positive root z1, h′(z1)>0. Under (H6), Equation (3.12) has two positive roots: z2 and z3. Assuming z2>z3, we get h′(z2)>0,h′(z3)<0. By substituting ω11,l=√zl(l=1,2,3) into Eq (3.11), we can obtain:
τ(j)11,l={1ω11,l[arccos(P11,l)+2jπ], Q11,l≥0,1ω11,l[2π−arccos(P11,l)+2jπ], Q11,l<0, | (3.13) |
where Q11,l=sin(ωτ1)|ω=ω11,l,τ=τ(j)11,l,P11,l=cos(ωτ1)|ω=ω11,l,τ=τ(j)11,l, sin(ωτ1) and cos(ωτ1) are given in Eq (3.11).
Then the following transversality condition holds:
Re(dλdτ1)−1|τ1=τ(j)11,l=Re(dτ1dλ)|τ1=τ(j)11,l=h′(ω211,l)μ2[ω211,l+(m+α+d)2]≠0(j=0,1,2,⋯). |
For the equilibrium Ek(k=2,3), Equation (3.4) becomes the following form when τ1>0 and τ2=0.
λ4+υ1,kλ3+υ2,kλ2+υ3,kλ+υ4,k+μe−λτ2(λ3+σ1,kλ2+σ2,kλ+σ3,k)=0, | (3.14) |
where υ1,k=ϱ1,k+ρ,υ2,k=ϱ2,k+ρς1,k,υ3,k=ϱ3,k+ρς2,k,υ4,k=ϱ4,k+ρς3,k with ϱ1,k, ϱ2,k, ϱ3,k, ϱ4,k, σ1,k, σ2,k, σ3,k, ς1,k, ς2,k, ς3,k given in Eq (3.4).
Assuming that λ=iω(ω>0) is a pure imaginary root of Eq (3.14), we substitute it into Eq (3.14) and separate the real and imaginary parts, and we have:
{ω4−υ2,kω2+υ4,k=−μ(−σ1,kω2+σ3,k)cos(ωτ1)−μ(−ω3+σ2,kω)sin(ωτ1),−υ1,kω3+υ3,kω=μ(−σ1,kω2+σ3,k)sin(ωτ1)−μ(−ω3+σ2,kω)cos(ωτ1). | (3.15) |
Thus,
{sin(ωτ1)=(−υ1,kω3+υ3,kω)(−σ1,kω2+σ3,k)−(ω4−υ2,kω2+υ4,k)(−ω3+σ2,kω)μ(−σ1,kω2+σ3,k)2+μ(−ω3+σ2,kω)2,cos(ωτ1)=−(ω4−υ2,kω2+υ4,k)(−σ1,kω2+σ3,k)+(−υ1,kω3+υ3,kω)(−ω3+σ2,kω)μ(−σ1,kω2+σ3,k)2+μ(−ω3+σ2,kω)2. | (3.16) |
Adding the square of the two equations in Eq (3.16) and letting z=ω2, we get:
h(z)=z4+˜κ1,kz3+˜κ2,kz2+˜κ3,kz+˜κ4,k, | (3.17) |
where ˜κ1,k=−2υ2,k+υ21,k−μ2,˜κ2,k=υ22,k+2υ4,k−2υ1,kυ3,k−μ2(σ21,k−2σ2,k),˜κ3,k=−2υ2,kυ4,k+υ23,k−μ2(−2σ1,kσ3,k+σ22,k),˜κ4,k=υ24,k−μ2σ23,k with σ1,k,σ2,k,σ3,k given in Eq (3.4) and υ1,k,υ2,k,υ3,k,υ4,k given in Eq (3.14). We hypothesize that Eq (3.17) has l(l=1,2,3,4) positive roots marked as z1>z2>⋯>zl, (l=1,2,3,4), so h′(zl)≠0. Substituting ω1k,l=√zl into Eq (3.16), we can get the expression of τ(j)1k,l:
τ(j)1k,l={1ω1k,l[arccos(P1k,l)+2jπ], Q1k,l≥0,1ω1k,l[2π−arccos(P1k,l)+2jπ], Q1k,l<0, | (3.18) |
where Q1k,l=sin(ωτ1)|ω=ω1k,l,τ=τ(j)1k,l,P1k,l=cos(ωτ1)|ω=ω1k,l,τ=τ(j)1k,l, sin(ωτ1) and cos(ωτ1) are given in Eq (3.16).
Thus, we obtain the transversality condition:
Re(dλdτ1)−1|τ1=τ(j)1k,l=Re(dτ1dλ)|τ1=τ(j)1k,l=h′(ω21k,l)μ2[(−σ1,kω21k,l+σ3,k)2+(−ω31k,l+σ2,kω1k,l)2]≠0(j=0,1,2,⋯). |
Theorem 3.2. For system (2.1) with τ2=0,τ1>0, the following conclusions hold when the stability conditions of Theorem 3.1 hold.
1) Equilibrium E1
(a) If (H5) and (H6) do not hold, the equilibrium E1 is locally asymptotically stable when τ1>0.
(b) If (H5) holds, h(z) has only one positive root z1, the system (2.1) undergoes Hopf bifurcation at E1 when τ1=τ(0)11,1, where τ(0)11,1 is given by Eq (3.13). If τ1∈[0,τ(0)11,1), the equilibrium E1 is locally asymptotically stable. When τ1∈[τ(0)11,1,+∞), the equilibrium E1 is unstable.
(c) If (H6) holds, h(z) has two positive roots z2 and z3, and system (2.1) undergoes Hopf bifurcation at E1 when τ1=τ(0)11,2,τ(0)11,3, where τ(0)11,2 and τ(0)11,3 are given by Eq (3.18). Supposing z2>z3, we get h′(z2)>0,h′(z3)<0. Then, ∃m∈N, which can make 0<τ(0)11,2<τ(0)11,3<τ(1)11,2<τ(1)11,3<⋯<τ(m)11,2<τ(m+1)11,2. When τ1∈(0,τ(0)11,2)∪⋃mi=1(τ(i−1)11,3,τ(i)11,2), the equilibrium E1 of the model (2.1) is locally asymptotically stable. When τ1∈⋃m−1i=0(τ(i)11,2,τ(i)11,3)∪(τ(m)11,2,+∞), the equilibrium E1 is unstable.
2) Equilibrium Ek(k=2,3)
(a) If h(z) has no positive root, the equilibrium Ek is locally asymptotically stable when τ1>0.
(b) If h(z) only has one positive root z1, the system (2.1) undergoes Hopf bifurcation at Ek when τ1=τ(j)1k,1 and h′(z1)>0. We get ∀0<τ1<τ(0)1k,1, the equilibrium Ek is asymptotically stable, and when τ1>τ(0)1k,1, the equilibrium Ek is unstable.
(c) If h(z) has two positive roots z1, z2, the system (2.1) undergoes Hopf bifurcation at Ek when τ1=τ(j)1k,1 and τ1=τ(j)1k,2. Assuming z2<z1, we can get h′(z1)>0,h′(z2)<0. Thus, assuming τ(0)1k,1<τ(0)1k,2, there exists m, which makes 0<τ(0)1k,1<τ(0)1k,2<τ(1)1k,1<τ(1)1k,2<⋯<τ(m)1k,1<τ(m+1)1k,1. When τ1∈[0,τ(0)1k,1)∪⋃mi=1(τ(i−1)1k,2,τ(i)1k,1), the equilibrium Ek is locally asymptotically stable. When τ1∈⋃m−1i=0(τ(i)1k,1,τ(i)1k,2)∪(τ(m)1k,1,+∞), the equilibrium Ek is unstable.
(d) If h(z) has three positive roots z1, z2, z3, the system (2.1) undergoes Hopf bifurcation at Ek when τ1=τ(j)1k,l(l=1,2,3). We assume z3<z2<z1, so we have h′(z1)>0,h′(z2)<0,h′(z3)>0. Similar to the analysis of (c), the equilibrium Ek switches between stability and instability with increasing τ1. Finally, the equilibrium Ek is unstable.
(e) If h(z) has four positive roots z1, z2, z3 and z4, the system (2.1) undergoes Hopf bifurcation at Ek when τ=τ(j)1k,l(l=1,2,3,4). Assuming that z4<z3<z2<z1, we can obtain h′(z1)>0,h′(z2)<0,h′(z3)>0,h′(z4)<0. Similar to the analysis of (c), the equilibrium Ek switches between stability and instability with increasing τ1. Ultimately, the equilibrium Ek is unstable.
In this subsection, we suppose τ1=τ∗1∈(0,τ1k,0),τ1k,0=minl,jτ(j)1k,l(k=1,2,3;l=1,2,3,4;j=1,2,⋯) which means that the antibody failure time is τ∗1. Then, we choose τ2 as the bifurcation parameter, which is the booster vaccination time. Similar to the situation of τ1>0,τ2=0, we have the following conclusions. The calculation details are presented in Appendix A.
Theorem 3.3. For system (2.1) with τ2>0,τ1>0, when the stability conditions of Theorems 3.1 and 2 hold, the following conclusions hold.
1) For equilibrium E1, under (H7) and (H8), the equilibrium E1 of system (2.1) is locally asymptotically stable when τ2∈[0,τ21,0) for the chosen τ∗1.
2) For equilibrium Ek(k=2,3), under (H9) and (H10), the equilibrium E2 or E3 of system (2.1) is locally asymptotically stable when τ2∈[0,τ2k,0) for the chosen τ∗1.
In this section, we derive the normal form of Hopf bifurcation about system (2.1) by using multiple time scales method. When τ1=τ∗1,τ2=τ2k,0(k=1,2,3), we assume that the characteristic equation (3.3) or (3.4) has a pair of pure imaginary roots λ=±iω∗ at which system (2.1) undergoes Hopf bifurcation at equilibrium Ek=(S∗k,V∗k,I∗k,R∗k)(k=1,2,3). For convenience, we let S∗≜S∗k, V∗≜V∗k, I∗≜I∗k, R∗≜R∗k. Since we are more concerned about the effect of booster vaccination time on the epidemic, we select τ2 as the bifurcation parameter. We can obtain the normal form as follows. The calculation details can be referred to Appendix B.
˙G=MτεG+HG2ˉG, |
where G is the coordinate projected on the center manifold and M,H are given in Eqs (B9) and (B14).
Let G=reiθ and substitute it into Eq (B15), we can obtain the normal form of Hopf bifurcation in polar coordinates:
{˙r=Re(M)τεr+Re(H)r3,˙θ=Im(M)τε+Im(H)r2. | (4.1) |
Then, we have the theorem as follows.
Theorem 4.1. If Re(M)τεRe(H)τc<0 holds, the system (2.1) exists periodic solution around the equilibrium.
1) If Re(M)τε<0, the periodic solution of system (2.1) is unstable.
2) If Re(M)τε>0, the periodic solution of system (2.1) is stable.
Proof. Although system (4.1) is two-dimensional, we find that the first equation is independent of θ, which represents the argument component in polar coordinates, so we only need to consider the first equation of system (4.1). If Re(M)τεRe(H)τc<0, ˙r=Re(M)τεr+Re(H)r3 has a nontrivial fixed point r∗=√−Re(M)τεRe(H)τc. At this equilibrium r∗, the eigenvalue is λ=−2Re(M)τϵ. According to Lyapunov indirect method, if Re(M)τϵ<0, the equilibrium r∗ is unstable. On the contrary, if Re(M)τϵ>0, the equilibrium r∗ is stable. Therefore, the periodic solution of system (2.1) is unstable if Re(M)τε<0 and is stable if Re(M)τε>0.
In this section, we first analyze the official data for rationalization and select the parameter values that are realistic. Second, the accuracy of the theoretical analysis is verified by simulating under these parameters. According to the results, we select the most suitable booster vaccination time and provide some reasonable suggestions to control the epidemic.
In this section, we use statistical methods to analyze the values of parameters, and on this basis, we select two groups of parameters most consistent with reality.
1) Cure rate: u
We obtain the average annual cure rates in different countries from the World Health Organization website (https://www.who.int/). By eliminating the missing values and outliers, we get the cure rates for 65 countries and plot the histogram in Figure 2.
We can clearly find that the cure rates of these countries are primarily concentrated in two categories: one is countries with cure rates greater than 0.8, which are mainly concentrated at approximately 0.95. We call it the first echelon. The other group is countries with cure rates between 0.6 and 0.8. We call it the second echelon, typically represented by the United States, with a cure rate of 0.66. Clearly, the higher the cure rate is in a country, the easier it is to control the outbreak. However, since we want to control the epidemic as effectively as possible, we focus on countries in the second echelon, choosing the cure rate u=0.7∈(0.6,0.8).
2) Vaccination rates: α
By visiting the official website of Our World in Data (https://ourworldindata.org/covid-vaccinations), we get different vaccination amounts from different countries around the world. Following data collation, the global intercontinental distribution of vaccination was obtained, as shown in Figure 3. According to the law of large numbers, the more experiments there are, the closer the frequency is to the probability, that is, the more accurately and reliably we can approximate the probability with frequency. Based on this, after obtaining the vaccination rates of each continent, we use them as the weights of the vaccination rates of each continent so that the coverage rate is more reliable.
Based on the intercontinental distribution of global vaccination, we can easily see that although 4.4 billion doses have been vaccinated worldwide, the distribution is very uneven. At the intercontinental level, Asia accounts for 65% of global vaccinations with more than 2.8 billion doses, followed by Europe with 15%, North America with 12%, and South America with 6%, while Africa, with nearly 20% of global population, accounts for less than 2% of global vaccinations. Therefore, we need to find a vaccination rate that reflects the global vaccination situation. Our approach is a weighted average of cure rates across continents. Europe and North America are the continents with the highest vaccination rates per 100 people, and Europe has recently surpassed North America with nearly 90 doses per 100 people. South America is at the same level as Asia, with an average of 55 doses per 100 people. Oceania ranks fifth with 32 doses per 100 people, and Africa has only five doses per 100 people, which is much lower than others. Based on the above information, we weight the vaccination rates of each continent and finally obtain the average annual vaccination rate α=0.63.
3) Natural mortality rate: d
Since 2019, the outbreak of the COVID-19 epidemic has caused thousands of deaths and a new increase in mortality. However, for the natural mortality rate of the population, we no longer have access to data from official statistics due to the interference of deaths from infection. Here, our approach is to count the official population mortality rates (https://databank.worldbank.org/) before the outbreak and obtain an estimate of the natural mortality rate after the COVID-19 outbreak through fitting. With the 95% confidence interval, we select the following fit results based on the merits of the best fit, which are shown in Table 2. We find that the error sum of squares SSE is small, indicating that the error is small and the fit is great.
Country | Function | SSE |
Australia | y1=0.006549+4.366e−5cos(0.3491t)+8.852e−5sin(0.3491t) | 1.724e−8 |
Canada | y2=0.007141+7.21e−5cos(0.6981t)+2.463e−6sin(0.6981t) | 1.09e−7 |
Switzerland | y3=0.008143−0.0002755cos(0.2715t)+5.128e−5sin(0.2715t) | 4.291e−7 |
China | y4=0.006841−0.0002921cos(0.2853t)+0.0002776sin(0.2853t) | 1.352e−7 |
Based on the fitting results, we predict the natural mortality rates of the population after the outbreak, and the results for the next five years are shown in Figure 4.
We find that the natural population mortality rate is distributed between 0.0065 and 0.0085 for the four representative countries in 2019–2025, so we choose d=0.00714 as the natural population mortality rate.
4) COVID-19 mortality rate: c
We also obtain the average annual COVID-19 mortality data of different countries from the official website of the World Health Organization (https://www.who.int/). After data processing, we select the rates from 160 countries and draw the corresponding histogram with the beta distribution density curve, as shown in Figure 5. We find that the frequency of the distribution of COVID-19 mortality rates peaks at approximately c=0.01 and c=0.02. To simulate the real situation because of the different medical capacities in different regions and the different characteristics of mutated virus at different time, we finally choose c=0.01 and c=0.02 as the COVID-19 mortality rates.
5) Booster vaccination rates: ρ
For the booster vaccination every year on average, we use the analytical estimation method. Since the booster vaccination is for people who already have antibodies, that is, the booster vaccination rate is relative to R and a large portion of R comes from vaccination, the initial vaccination has already screened out those who could not be vaccinated due to individual reasons. Therefore, R has a stronger desire to be vaccinated than S, so we believe that the rate of booster vaccination is higher than that of initial vaccination. With the above considerations, we choose the average annual booster vaccination rate ρ=0.7.
6) Infection rate: β, γ
Since the global COVID-19 outbreak, countries have paid great attention to the infection and have adopted policies to stop the spread of the epidemic. One of the most important parts is wearing masks in public, which has led to a significant reduction in the infection rate. However, since the epidemic differs in its ability to spread in different countries, the infection rate also varies. Here, we take β to be 0.0009 and 0.00015 respectively. In addition, the immune barrier of V has not been fully formed although these individuals are vaccinated. Therefore, there is also a specific transmission rate for them, but this rate is significantly smaller than that of susceptible people. Therefore, we take γ=0.0002 and γ=0.00008.
Based on the above discussion and estimating the reality of the situation, we take the following two groups of parameters:
(Ⅰ): Λ=10, μ=0.7, α=0.63, β=0.0009, γ=0.0002, d=0.00714, ρ=0.7, u=0.7, c=0.02, m=0.9;
(Ⅱ): Λ=80, μ=0.8, α=0.63, β=0.00015, γ=0.00008, d=0.00714, ρ=0.7, u=0.7, c=0.01, m=0.9.
For the group of parameters (Ⅰ): Λ=10,μ=0.7,α=0.63,β=0.0009,γ=0.0002,d=0.00714, ρ=0.7,u=0.7,c=0.02,m=0.9, we calculate the disease-free equilibrium E1=[431,591,0,378]. We find that the assumptions (H1) and (H2) are not valid, so equilibria E2 and E3 do not exist. Under the group of parameters, (H3) holds, so E1=[431,591,0,378] is locally asymptotically stable when τ1=τ2=0. When τ1>0,τ2=0, we can obtain (H4) holds and h(z) only has one positive root, ω11,1=0.2073, sin(ω11,1τ(0)11,1)=0.3039, cos(ω11,1τ(0)11,1)=−0.9527, τ11,0=τ(0)11,1=13.66. We choose τ1=τ∗1=4.5 and substitute it into Eqs (9.1)−(9.4), we have ω21,1=0.61, sin(ω21,1τ(0)21,1)=0.5821, cos(ω11,1τ(0)11,1)=−0.8131, τ21,0=τ(0)21,1=1.57. If (H7) and (H8) hold, the equilibrium E1 is locally asymptotically stable when τ2∈[0,τ21,0).
When τ1=τ2=0, the equilibrium E1 is locally asymptotically stable according to Theorem 3.1. This means that the antibody expires instantaneously, and people maintain the effectiveness of the antibodies through uninterrupted booster shots. First, we choose [S(t),V(t),I(t),R(t)]T=[500,600,100,500]T for t∈[−τ2,0] as the initial function, which represents the initial state of the epidemic and then draw a time history graph in Figure 6(b). We can see that in this case, the epidemic basically reaches stability in 5 years and is eliminated after 10 years. This result is very optimistic. Next, we select three sets of initial functions 1) [S(t),V(t),I(t),R(t)]T=[500,600,100,500]T, 2) [S(t),V(t),I(t),R(t)]T=[5000,6000,1000,5000]T, 3) [S(t),V(t),I(t),R(t)]T=[10,000,20,000,10,000,10,000]T for t∈[−τ2,0], which represent different initial states of the epidemic. We find that when the initial functions are far from equilibrium, the fluctuations are large during the initial years, and the time taken to reach stability is long, as shown in Figure 6(a). However, the epidemic eventually reaches stability regardless of the initial functions, so we conjecture that the equilibrium is not only locally asymptotically stable, but may also be globally stable. This finding suggests that under this set of parameters, even if the epidemic is severe in a given region, the epidemic will eventually reach a manageable level and will be eliminated through uninterrupted booster vaccination. However, as far as reality is concerned, the vaccines we have developed cannot be immediate and uninterrupted, so this situation does not exist.
When τ1=4.5∈(0,τ11,0)=(0,13.66), τ2=1∈(0,τ21,0)=(0,1.57), the equilibrium E1 is locally asymptotically stable according to Theorem 3.3. τ1=4.5 means the vaccine will fail 4.5 years after individuals acquires antibodies, and τ2=1 means that people begin the booster vaccination after 1 year to cope with the decline in antibodies.
First, we still choose the initial function [S(t),V(t),I(t),R(t)]T=[500,600,100,500]T for t∈[−τ2,0] and show the variation in the number of people in different compartments over time in Figure 7(b). This figure suggests that receiving the booster vaccinations after one year will lead to a stable epidemic in 10 years and the society will persist in this state consistently. In addition, the number of infected people would eventually approach 0, meaning that the epidemic can eventually be eliminated. That is the most desirable outcome. After that, we choose 1) [S(t),V(t),I(t),R(t)]T=[500,600,100,500]T, 2) [S(t),V(t),I(t),R(t)]T=[5000,6000,1000,5000]T, 3) [S(t),V(t),I(t),R(t)]T=[10,000,20,000,10,000,10,000]T for t∈[−τ2,0] as three sets of initial functions that are same as τ1=τ2=0, and the results are shown in Figure 7(a). Although the amplitude of fluctuation and the time taken to reach stability increase gradually as the initial function moves away from equilibrium, it will eventually stabilize. Thus, if we carry out booster vaccination after one year, the outbreak will be controlled. However, the time needed to contain the outbreak depends on the current situation. The more severe the epidemic is, the more difficult it will be to control. According to the above results, we guess that the equilibrium E1 is not only locally asymptotically stable but also globally stable under this group of parameters.
For the group of parameters (Ⅱ): Λ=90,μ=0.8,α=0.63,β=0.00015,γ=0.00008,d=0.00714, ρ=0.7,u=0.7,c=0.02,m=0.9, we find that (H1) holds, so equilibrium E2 makes sense and is [2817, 3683, 875, 2605]. (H2) and (H3) do not hold, equilibrium E1 is unstable and equilibrium E3 is meaningless. Substituting this group of parameters into Eq (3.7), (H4) is satisfied, so equilibrium E2 is locally asymptotically stable when τ1=τ2=0. When τ1>0,τ2=0, we can get that h(z) has three roots and z3=0.0004<z2=0.0206<z1=0.1458, ω12,1=0.38 sin(ω12,1τ(0)12,1)=0.4150, cos(ω12,1τ(0)12,1)=−0.9098, τ12,0=τ(0)12,1=7.11. Choosing τ1=4, we obtain that (H9) and (H10) hold and ω22,1=0.66, sin(ω22,1τ(0)22,1)=0.5738, cos(ω22,1τ(0)22,1)=−0.8190, τ22,0=τ(0)22,1=0.92. Substitute the parameters (Ⅱ) into Eqs. (B9) and (B14), we have Re(M)>0,Re(H)<0. We can deduce τε>0, Re(Mk)τε>0, the periodic solution is stable based on Theorem 4.1.
When τ1=τ2=0, we choose 1) [S(t),V(t),I(t),R(t)]T = [3000,4000,1000,3000]T, 2) [S(t),V(t),I(t),R(t)]T = [5000,8000,2000,5000]T, 3) [S(t),V(t),I(t),R(t)]T = [10,000,20,000,5000,10,000]T for t∈[−τ2,0] as the initial functions separately. We draw a plot of the epidemic over time for the three sets of initial functions and a separate time history graph with initial function 1) in Figure 8.
It is easy to observe from Figure 8(b) that the epidemic reaches stability rapidly within 8 years. However, it cannot be eradicated and coexists with humans for a long time. In Figure 8(a), the initial function 3) takes longer to reach the steady state, and the stabilization effect is not as good as that of 1) and 2), but it eventually reaches stability. Therefore, we conjecture that the equilibrium E2 is globally stable. Thus, we can get the following results: it is effective to control the epidemic through uninterrupted booster vaccination, but the time needed to control the epidemic mainly depends on the current state. The more severe the epidemic, the more difficult it will be. This finding shows that booster vaccination is of great significance, but considering that the vaccines developed at present are not instantaneous failures and vaccines cannot be administered in a short period of time, τ1=τ2=0 is basically impossible.
When τ1=4∈(0,τ12,0)=(0,7.11),τ2=0.8∈(0,τ22,0)=(0,0.92), the antibody expires after 4 years and we re-inject booster shots after 0.8 years. We also choose the three sets of initial functions: 1) [S(t),V(t),I(t),R(t)]T=[500,600,100,500]T, 2) [S(t),V(t),I(t),R(t)]T=[5000,6000,1000,5000]T, 3) [S(t),V(t),I(t),R(t)]T=[10,000,20,000,10,000,10,000]T for t∈[−τ2,0] and make figures respectively similar to the above situation. The results are shown in Figure 9.
With antibody failure after 4 years and booster vaccination after 0.8 years, the system quickly becomes stable when the initial function is chosen near equilibrium according to (b) in Figure 9. From the figure, we also find that although the epidemic cannot be eliminated, it can always stay in a stable state. In Figure 9(a), as the initial function moves away from equilibrium, the epidemic worsens. It fluctuates dramatically in a short period of time and will take decades to stabilize. But no matter what initial function is chosen, the epidemic will eventually be controlled. Therefore, we hypothesize that this stability is global. Even if the current epidemic is not optimistic in some countries, the trend will be better with the booster vaccination in time.
When τ1=4,τ2=1.11>τ22,0=0.92, which means that the antibody will expire after 3.9 years and booster vaccination will be carried out after 1.11 years, the equilibrium E2 of system (2.1) is unstable. According to Theorem 4.1, system (2.1) has a forward Hopf bifurcation near τ(0)22,1=0.92 and the periodic solution is stable. We choose [S(t),V(t),I(t),R(t)]T=[3000,4000,1000,3000]T for t∈[−τ2,0] as the initial function, and the epidemic situation is shown in Figure 10.
In this case, the epidemic will continue to fluctuate, which is not promising for controlling the epidemic.
Based on the above simulations, we know that the only way to avoid out-of-control epidemic is to carry out booster vaccinations within the critical time. However, when is the best time to vaccinate? Next, we will analyze the optimal time of booster vaccination. First, we take the first set of parameters as the study object given τ2=0.1,0.4,0.7,1.0,1.3 respectively, and then we draw the images of the infected people (I) with time as shown in Figure 11.
In Figure 11(a), we find that the time to eventual stabilization is essentially the same regardless of the booster vaccination time we select, but when we focus on the case near the peak in Figure 11(b), we find that the smaller the booster vaccination time is, the smaller the number of infections will be. This finding reveals that the earlier the booster vaccination is performed, the fewer people will be infected with COVID-19. However, considering human and material resources and financial resources, it is not an easy task to complete community-wide vaccination, so we need to implement booster vaccinationss as soon as possible according to the local reality and within the scope of ensuring the normal operation of the community.
From a medical point of view, it is generally believed that the immune effect weakens after six months of vaccination, so 6 months after the completion of the initial vaccine is the critical period for booster vaccination [51,52]. For the optimal strategy of vaccine distribution, researchers solve the optimal scheduling of the mixed vaccination strategy by using different optimization methods [53,54,55]. From the point of view of completing one round of vaccination, we need to allow sufficient time for booster vaccination [56]. Taking these considerations into account, we can obtain the optimal booster vaccination time.
Based on the above simulations and analysis, we have the following results.
1) When the time of antibody expiration is determined, the epidemic will become increasingly not controllable as the time of booster vaccination increases, and when the time of booster vaccination exceeds the critical value, the system (2.1) will be unstable, and the epidemic will be out of control. However, the time of booster vaccination is not as small as possible. In reality, we need to consider the comprehensive capacity of the country to ensure that booster vaccination time is minimized within the capacity.
2) After simulating the changes in epidemic development under three different sets of initial functions, we find that the epidemic will eventually be controlled, so we assume that the stability of equilibrium Ek(k=1,2,3) is not only locally asymptotically stable, but may also be globally stable. That is, even if the current epidemic is not optimistic in a certain region, the possible global stability ensures that the epidemic can eventually reach effective control after timely booster vaccination.
3) When the equilibrium E1 satisfies the condition of locally asymptotical stability, the epidemic can eventually be eliminated. If the selected parameters meet the existence and stability of equilibrium E2 or E3, it can reach a stable state. Although the epidemic cannot be eliminated, to some extent, it can be considered to be effectively controlled.
4) Our model is flexible. The current state of the epidemic varies from country to country, and we can simulate the epidemic of different countries by changing the parameters. For example, when a mutant strain is found in a country and has been confirmed to spread widely around the country, we can simulate this situation by increasing the infection rate α and determine the population size by natural population growth Λ. If the current vaccine is found to be less resistant to the mutant strain, we can simultaneously increase γ, μ and determine population size by natural population growth Λ. After determining the parameters, we can calculate the critical booster vaccination time. When the booster vaccination time is less than the critical value, the system can reach stability, that is, the current outbreak can be controlled. Based on the above analysis, we can develop a policy applicable to this region that allows to control the epidemic as much as possible within the capacity of the region.
Based on the above conclusions, we give the following recommendations.
1) The time of the booster vaccination should be kept within the threshold so that the epidemic can be controlled eventually. However, the overall strength of the area, such as economic development, medical level, etc., also needs to be assessed. For example, in the second group of parameters, τ22,0 = 0.92. If this is a well-developed country, we would suggest that the booster shot is needed after 0.5–0.92 years. As the epidemic continues to evolve, we are concerned about more than just one booster shot. Our model can apply to more than one booster shot, gradually developing a presence similar to that of the annual influenza vaccination. So we need booster vaccinations every 0.5–0.92 years to ensure the continued effectiveness of the antibodies.
2) Even if the development of the epidemic in a given country is not promising, it should not be taken lightly. Although providing booster vaccination at an appropriate time cannot guarantee that the epidemic will reach control within a short period of time, it has a positive effect on the development of the epidemic. Because the possible global stability ensures that the epidemic will eventually be effectively controlled.
3) It is necessary to detect changes in the epidemic continuously, such as the emergence of new mutant strains and changes in the time of antibody expiration. We can regain a critical booster vaccination time through calculation and then change the booster vaccination strategy in time to cope with the variable COVID-19.
In this paper, we have constructed an SVIR model with two time delays to study the suitable time for booster vaccination. We have considered the existence and stability of equilibria in our model. Then, we have also analyzed the existence and dynamic properties of Hopf bifurcation. In Section 5, we chose two groups of parameters by analyzing the data from official websites. Then, we have carried out numerical simulations to verify the analytical results and provide some reasonable suggestions to control the development of the epidemic.
According to the results of numerical simulations, we found that the epidemic would become increasingly difficult to control as the booster vaccination time τ2 increased. Considering the factors of all aspects, we suggest that it is suitable to vaccine with the booster shots approximately 6–11 months later for the group of parameters (Ⅱ). We also found that the equilibrium of system (2.1) might be globally stable when τ2 is within the threshold value. That means, the booster vaccination is effective for outbreak control, so it is crucial to provide booster vaccination in time even if the current epidemic is serious. In addition, considering that the selection of parameters might change due to virus mutation or other factors, we need to continuously monitor the status of the epidemic in real time and adjust the strategy to ensure that the epidemic can be effectively controlled.
This study was funded by Fundamental Research Funds for the Central Universities of China (Grant No.2572022DJ06).
All the authors declare no conflicts of interest in this paper.
For equilibrium E1, we let λ=iω(ω>0) be a pure imaginary root of Eq (3.3) and substitute it into Eq (3.3). Then separating the real part and imaginary part, we have:
{−ω2+b2+μωsin(ωτ∗1)+μc1cos(ωτ∗1)=−ρc2cos(ωτ2)−ρωsin(ωτ2),b1ω+μωcos(ωτ∗1)−μc1sin(ωτ∗1)=ρc2sin(ωτ2)−ρωcos(ωτ2), | (A1) |
where b1=m+α+2d,b2=(m+d)(α+d),c1=m+α+d,c2=α+d. Eq (A1) leads to:
{sin(ωτ2)=−ω[−ω2+b2+μωsin(ωτ∗1)+μc1cos(ωτ∗1)]ρ(c22+ω2)+c2[b1ω+μωcos(ωτ∗1)−μc1sin(ωτ∗1)]ρ(c22+ω2),cos(ωτ2)=−c2[−ω2+b2+μωsin(ωτ∗1)+μc1cos(ωτ∗1)]ρ(c22+ω2)+−ω[b1ω+μωcos(ωτ∗1)−μc1sin(ωτ∗1)]ρ(c22+ω2). | (A2) |
Adding the square of the two equations in Eq (A2), we get:
F1(ω)=ω4+(−2b2+b21+μ2−ρ2)ω2+b22μ2c21−ρ2c22+2sin(ωτ∗1)[−μω3+b2μω−b1μωc1]+2cos(ωτ∗1)[−μc1ω2+μb2c1+b1μω2], | (A3) |
where b1,b2,c1,c2 are given in Eq (A1). We suppose:
(H7)μ2c21−ρ2c22+2μb2c1<0. |
Under (H7), we have F1(0)=μ2c21−ρ2c22+2μb2c1<0, F1(∞)>0. Therefore, there is at least one positive root of F1(ω)=0. We assume there are l positive roots of F1(ω)=0, hence, there is the expression of τ(j)21,l,j=0,1,2,⋯.
τ(j)21,l={1ω21,l[arccos(P21,l)+2jπ], Q21,l≥0,1ω21,l[2π−arccos(P21,l)+2jπ], Q21,l<0, | (A4) |
where Q21,l=sin(ωτ2)|ω=ω21,l,τ=τ(j)21,l,P21,l=cos(ωτ2)|ω=ω21,l,τ=τ(j)21,l, sin(ωτ2) and cos(ωτ2) are given in Eq (A2).
Let τ21,0=minτ(j)21,l=τ(0)21,l,j=0,1,2,⋯. When τ2=τ21,0, Equation (3.5) has a pair of purely imaginary roots ±iω21,0. Assume:
(H8)Re(dλdτ2)−1|τ=τ21,0≠0. |
Under (H8), the equilibrium E1 is locally asymptotically stable when τ2∈[0,τ21,0).
For equilibrium Ek(k=2,3), similar to the above analysis, we let λ=iω(ω>0) be a pure imaginary root of characteristic equation (3.4) and substitute it into Eq (3.4). Then, separating the real part and imaginary part, we have:
{ω4−ϱ2,kω2+ϱ4,k+μcos(ωτ∗1)(−σ1,kω2+σ3,k)+μsin(ωτ∗1)(−ω3+σ2,kω)=−ρ(−ς1,kω2+ς3,k)cos(ωτ2)−ρ(−ω3+ς2,kω)sin(ωτ2),−ϱ1,kω3+ϱ3,kω+μcos(ωτ∗1)(−ω3+σ2,kω)−μsin(ωτ∗1)(−σ1,kω2+σ3,k)=ρ(−ς1,kω2+ς3,k)sin(ωτ2)−ρ(−ω3+ς2,kω)cos(ωτ2). | (A5) |
Then, we can obtain:
{sin(ωτ2)=−(ω4−ϱ2,kω2+ϱ4,k)(−ω3+ς2,kω)+(−ϱ1,kω3+ϱ3,kω)(−ς1,kω2+ς3,k)ρ[(−ς1,kω2+ς3,k)2+(−ω3+ς2,kω)2]+μcos(ωτ∗1)[−(−σ1,kω2+σ3,k)(−ω3+ς2,kω)+(−ω3+σ2,kω)(−ς1,kω2+ς3,k)]ρ[(−ς1,kω2+ς3,k)2+(−ω3+ς2,kω)2]+μsin(ωτ∗1)[−(−ω3+σ2,kω)(−ω3+ς2,kω)−(−σ1,kω2+σ3,k)(−ς1,kω2+ς3,k)]ρ[(−ς1,kω2+ς3,k)2+(−ω3+ς2,kω)2].cos(ωτ2)=−(ω4−ϱ2,kω2+ϱ4,k)(−ς1,kω2+ς3,k)+(−ϱ1,kω3+ϱ3,kω)(−ω3+ς2,kω)ρ[(−ς1,kω2+ς3,k)2+(−ω3+ς2,kω)2]−μcos(ωτ∗1)[(−σ1,kω2+σ3,k)(−ς1,kω2+ς3,k)+(−ω3+σ2,kω)(−ω3+ς2,kω)]ρ[(−ς1,kω2+ς3,k)2+(−ω3+ς2,kω)2]+μsin(ωτ∗1)[−(−ω3+σ2,kω)(−ς1,kω2+ς3,k)+(−σ1,kω2+σ3,k)(−ω3+ς2,kω)]ρ[(−ς1,kω2+ς3,k)2+(−ω3+ς2,kω)2]. | (A6) |
Adding the square of the two equations in (A6), we have:
Fk(ω)=(ω4−ϱ2,kω2+ϱ4,k)2+(−ϱ1,kω3+ϱ3,kω)2+μ2(−σ1,kω2+σ3,k)2+μ2(−ω3+σ2,kω)2+2μcos(ωτ∗1)[(−σ1,kω2+σ3,k)(ω4−ϱ2,kω2+ϱ4,k)+(−ω3+σ2,kω)(−ϱ1,kω3+ϱ3,kω)]+2μsin(ωτ∗1)[(−ω3+σ2,kω)(ω4−ϱ2,kω2+ϱ4,k)−(−σ1,kω2+σ3,k)(−ϱ1,kω3+ϱ3,kω)]−ρ2(−ς1,kω2+ς3,k)2−ρ2(−ω3+ς2,kω)2=0,k=2,3. | (A7) |
Then we give the following assumption:
(H9)ϱ24,k+μ2σ23,k+2μσ3,kϱ4,k−ρ2ς23,k<0. |
Under (H9), we can deduce Fk(0)<0 and Fk(∞)>0. Thus, Fk(ω)=0 has at least one positive root. We assume that there are l positive roots of Fk(ω)=0 and denote them as ω2k,l.
τ(j)2k,l={1ω2k,l[arccos(P2k,l)+2jπ], Q2k,l≥0,1ω2k,l[2π−arccos(P2k,l)+2jπ], Q2k,l<0, | (A8) |
where Q2k,l=sin(ωτ2)|ω=ω2k,l,τ=τ(j)2k,l,P2k,l=cos(ωτ2)|ω=ω2k,l,τ=τ(j)2k,l, sin(ωτ2) and cos(ωτ2) are given in Eq (A6).
Let τ2k,0=minτ(j)2k,l,j=0,1,2,⋯,k=2,3. When τ2=τ2k,0, Equation (3.5) has a pair of purely imaginary roots ±iω2k,0. Assume
(H10) Re(dλdτ2)−1|τ=τ2k,0≠0. |
Under (H10), the equilibrium Ek(k=2,3) is locally asymptotically stable if τ2∈[0,τ2k,0).
The system (2.1) can be written as follows:
X′(t)=AX(t)+BX(t−τ∗1)+CX(t−τ2)+F[X(t),X(t−τ∗1),X(t−τ2)], | (B1) |
where
X(t)=(S,V,I,R)T,X(t−τ∗1)=(S(t−τ∗1),V(t−τ∗1),I(t−τ∗1),R(t−τ∗1))T,X(t−τ2)=(S(t−τ2),V(t−τ2),I(t−τ2),R(t−τ2))T,A=(−α−βI∗−d0−βS∗0α−m−γI∗−d−γV∗0βI∗γI∗βS∗+rV∗−d−u−c00mu−d),B=(000μ00000000000−μ),C=(0000000ρ0000000−ρ),F=(FSFVFIFR)=(−βSI−γVIβSI+γVI0). |
Let h=(h1,h2,h3,h4)T be the eigenvector of the linear operator corresponding to the eigenvalue iω∗, and let h∗=(h∗1,h∗2,h∗3,h∗4)T be the normalized eigenvector of the adjoint operator of the linear operator corresponding to the eigenvalues −iω∗ satisfying the inner product <h∗,h>=1. By a simple calculation, we can obtain:
h1=1,h2=−uh3+(iω∗+d+μe−iω∗τ∗1+ρe−iω∗τ2)h4m,h3=mμe−iω∗τ∗1βI∗−mγI∗(iω+d+μe−iω∗τ∗1+ρe−iω∗τ2)(iω∗+α+βI∗+d)[(iω∗−βS∗−γV∗+d+u+c)m+uγI∗]μe−iω∗τ∗1+γI∗βS∗(iω∗+d+μe−iω∗τ∗1+ρe−iω∗τ2),h4=(iω+α+βI∗+d)+βS∗h3μe−iω∗τ∗1,˜h∗2=(iω∗+α+βI∗+d)˜h∗1−βI∗α,h∗j=d˜h∗j,d=1h1¯˜h∗1+h2¯˜h∗2+h3¯˜h∗3+h4¯˜h∗4,˜h∗1=βI∗[−um(−iω∗+m+d+γI∗)+γV∗]−α(−iω∗−βS∗−γV∗+d+u+c+umγI∗)αβS∗+[−um(−iω∗+m+d+γI∗)+γV∗](−iω∗+α+βI∗+d),˜h∗3=1,˜h∗4=(−iω∗+m+d+γI∗)˜h∗2−γI∗˜h∗3m. | (B2) |
The solution of Eq (B1) X(t) can be written as:
X(t)=X(T0,T1,T2,⋯)=∞∑n=1εnXn(T0,T1,T2,⋯), | (B3) |
where X(T0,T1,T2,⋯)=[S(T0,T1,T2,⋯),V(T0,T1,T2,⋯),I(T0,T1,T2,⋯),R(T0,T1,T2,⋯)]T, Xn(T0,T1,T2,⋯)=[Sn(T0,T1,T2,⋯),Vn(T0,T1,T2,⋯),In(T0,T1,T2,⋯),Rn(T0,T1,T2,⋯)]T, Ti=ϵit, i=0,1,2,⋯ and Ti is the scaling transform in the time direction.
X′(t) can be written as:
X′(t)=dX(t)dt=εdX1dt+ε2dX2dt+ε3dX3dt+⋯=ε(∂X1∂T0+ε∂X1∂T1+ε2∂X1∂T2)+ε2(∂X2∂T0+ε∂X2∂T1)+ε3∂X3∂T0+⋯=εD0X1+ε2D1X1+ε3D2X1+ε2D0X2+ε3D1X2+ε3D0X3+⋯, | (B4) |
where Di=∂∂Ti(i=1,2,3,⋯) is differential operator.
Since we are more concerned about the influence of booster vaccination time, we take τ2 as the bifurcation parameter. We let τ2=τc+ετε, where τc is the critical time delay given in Eqs (A4) or (A8), τε is the disturbance parameter and ε is the dimensionless scale parameter. Using Taylor expansion of X(t−τ∗1) and X(t−τ2) respectively, we have:
X(t−τ∗1)=εX1,τ∗1+ε2(X2,τ∗1−D1X1,τ∗1)+ε3(X3,τ∗1−D1X2,τ∗1−D2X1,τ∗1)+⋯,X(t−τ2)=εX1,τc+ε2X2,τc+ε3X3,τc−ε2τεD0X1,τc−ε3τεD0X2,τc−ε2τcD1X1,τc−ε3τεD1X1,τc−ε3τcD2X1,τc−ε3τcD1X2,τc+⋯, | (B5) |
where Xj,τ∗1=Xj(T0−τ∗1,T1,T2,⋯), Xj,τc=Xj(T0−τc,T1,T2,⋯), j=1,2,3⋯. Then we substitute Eqs (B3)–(B5) into Eq (B1). For the ε-order terms, we have:
{D0S1+αS1+βS∗I1+βI∗S1+dS1−μR1,τ∗1=0,D0V1−αS1+mV1+γV∗I1+γI∗V1+dV1−ρR1,τc=0,D0I1−βS∗I1−βI∗S1+dI1+uI1+cI1−γV∗I1−γI∗V1=0,D0R1−mV1−uI1+dR1+μR1,τ∗1+ρR1,τc=0. | (B6) |
Since ±iω∗ are the eigenvalues of the linear part of Eq (B1), the solution of Eq (B6) can be expressed in the following form:
X1(T1,T2,T3,⋯)=G(T1,T2,T3,⋯)eiω∗T0h+ˉG(T1,T2,T3,⋯)e−iω∗T0ˉh, | (B7) |
where h is given in Eq (B2).
For the ε2-order terms, we obtain:
{D0S2+αS2+βS∗I2+βI∗S2+dS2−μR2,τ∗1=−D1S1−βS1I1−μD1R1,τ∗1,D0V2−αS2+mV2+γV∗I2+γI∗V2+dV2−ρR2,τc=−D1V1−γV1I1−ρτεD0R1,τc−ρτcD0R1,τc,D0I2−βS∗I2−βI∗S2+dI2+uI2+cI2−γV∗I2−γI∗V2=−D1I1+γV1I1+βS1I1,D0R2−mV2−uI2+dR2+μR2,τ∗1+ρR2,τc=−D1R1+μD1R1,τ∗1+ρτεD0R1,τc+ρτcD0R1,τc. | (B8) |
Then, we substitute Eq (B7) into the right side of Eq (B8) and mark the coefficient before eiω∗T0 as vector m1. In accordance with the solvability condition <h∗,m1>=0, we can obtain the expression of ∂G∂T1:
∂G∂T1=MτεG, | (B9) |
where M=ρiω∗(h4ˉh∗4−h4ˉh∗2)1−μe−iω∗τ∗1(h4ˉh∗4−h4ˉh∗1)−ρτce−iω∗τc(h4ˉh∗4−h4ˉh∗2).
We assume that the solution of Eq (B8) will take the following form:
S2=g1e2iω∗τcT0G2+ˉg1e−2iω∗τcT0ˉG2+l1GˉG,A2=g2e2iω∗τcT0G2+ˉg2e−2iω∗τcT0ˉG2+l2GˉG,I2=g3e2iω∗τcT0G2+ˉg3e−2iω∗τcT0ˉG2+l3GˉG,R2=g4e2iω∗τcT0G2+ˉg4e−2iω∗τcT0ˉG2+l4GˉG. | (B10) |
Substituting them into Eq (B8), we can solve the expression of g1, g2, g3, g4, l1, l2, l3, l4 from the following equations:
[(2iω∗+ϵ1)0βS∗−μe−2iωτ∗1−α(2iω∗+ϵ2)γV∗−ρe−2iωτc−βI∗−γI∗(2iω∗+ϵ3)00−m−u(2iω∗+ϵ4)][g1g2g3g4]=[−βh1h3−γh2h3γh2h3+βh1h30], | (B11) |
[ϵ10βS∗−μ−αϵ2γV∗−ρ−βI∗−γI∗ϵ300−m−u(d+μ+ρ)][l1l2l3l4]=[−βh1ˉh3−βˉh1h3−γˉh2h3−γh2ˉh3γˉh2h3+βˉh1h3+γh2ˉh3+βh1ˉh30], | (B12) |
where ϵ1=α+βI∗+d,ϵ2=m+d+γI∗,ϵ3=−βS∗−γV∗+d+u+c,ϵ4=d+μe−iω∗τ∗1+ρe−iω∗τ2. For ε3-term, we have:
{D0S3+αS3+βS∗I3+βI∗S3+dS3−μR3,τ∗1=−D2S1−βS1I2−μD1R2,τ∗1−D1S2−βS2I1−μD2R1,τ∗1,D0V3−αS3+mV3+γV∗I3+γI∗V3+dV3−ρR3,τc=−D2V1−γV2I1−ρτεD1R1,τc−ρτcD1R2,τc−D1V2−γV1I2−ρτεD0R2,τc−ρτcD2R1,τc,D0I3−βS∗I3−βI∗S3+dI3+uI3+cI3−γV∗I3−γI∗V3=−D2I1+γV1I2+βS2I1−D1I2+γV2I1+βS1I2,D0R3−mV3−uI3+dR3+μR3,τ∗1+ρR3,τc=−D2R1+μD1R2,τ∗1+ρτεD0R2,τc+ρτcD1R2,τc−D1R2+μD2R1,τ∗1+ρτεD1R1,τc+ρτcD2R1,τc. | (B13) |
We substitute Eqs (B7), (B9) and (B10) into the right expression of Eq (B13) and note the coefficient of eiω∗T0 as vector m2. According to solvability condition <h∗,m2>=0, we have the expression of ∂G∂T2. Since τ2ε has less impact on the normal form, we can ignore the τ2εG term. Thus, we can obtain:
∂G∂T2=HG2ˉG, | (B14) |
where H=β(h1l3+ˉh1g3+h3l1+ˉh3g1)(−ˉh∗1+ˉh∗3)1+μ(h4ˉh∗1−h4ˉh∗4)e−iω∗τ∗1+ρτce−iω∗τc(h4ˉh∗1−h4ˉh∗4)+γ(h2l3+ˉh2g3+h3l2+ˉh3g2)(−ˉh∗2+ˉh∗3)1+μ(h4ˉh∗1−h4ˉh∗4)e−iω∗τ∗1+ρτce−iω∗τc(h4ˉh∗1−h4ˉh∗4).
Then, we let G→G/ε. Therefore, we obtain the normal form of Hopf bifurcation for system (2.1):
˙G=MτεG+HG2ˉG, | (B15) |
where M,H are given in Eqs (B9) and (B14).
[1] |
N. Zhu, D. Zhang, W. Wang, X. Li, B. Yang, J. Song, et al., A novel coronavirus from patients with pneumonia in China, N. Engl. J. Med., 382 (2020), 727–733. https://doi.org/10.1056/NEJMoa2001017 doi: 10.1056/NEJMoa2001017
![]() |
[2] |
H. Zhang, F. Du, X. Cao, X. Feng, H. Zhang, Z. Wu, et al., Clinical characteristics of coronavirus disease 2019 (COVID-19) in patients out of Wuhan from China: a case control study, BMC Infect. Dis., 21 (2021), 207. https://doi.org/10.1186/s12879-021-05897-z doi: 10.1186/s12879-021-05897-z
![]() |
[3] |
C. Huang, Y. Wang, X. Li, L. Ren, J. Zhao, Y. Hu, et al., Clinical features of patients infected with 2019 novel coronavirus in Wuhan, China, Lancet, 395 (2020), 497–506. https://doi.org/10.1016/S0140-6736(20)30183-5 doi: 10.1016/S0140-6736(20)30183-5
![]() |
[4] |
M. A. Hassan, A. A. Bala, A. I. Jatau, Low rate of COVID-19 vaccination in Africa: a cause for concern, Ther. Adv. Vaccines Immun., 10 (2022), 1–3. https://doi.org/10.1177/25151355221088159 doi: 10.1177/25151355221088159
![]() |
[5] |
S. O. Minka, F. H. Minka, A tabulated summary of the evidence on humoral and cellular responses to the SARS-CoV-2 Omicron VOC, as well as vaccine efficacy against this variant, Immunol. Lett., 243 (2022), 38–43. https://doi.org/10.1016/j.imlet.2022.02.002 doi: 10.1016/j.imlet.2022.02.002
![]() |
[6] |
W. O. Kermack, A. G. Mckendrick, A contribution to the mathematical theory of epidemics, Proc. Roy. Soc., 115 (1927), 111–124. https://doi.org/10.1098/rspa.1927.0118 doi: 10.1098/rspa.1927.0118
![]() |
[7] |
A. Din, Y. Li, F. M. Khan, Z. U. Khan, P. Liu, On Analysis of fractional order mathematical model of Hepatitis B using Atangana Baleanu Caputo (ABC) derivative, Fractals, 30 (2022), 2240017. https://doi.org/10.1142/S0218348X22400175 doi: 10.1142/S0218348X22400175
![]() |
[8] |
B. Boukanjime, M. E. Fatini, A stochastic Hepatitis B epidemic model driven by Levy noise, Physica A, 521 (2019), 796–806. https://doi.org/10.1016/j.physa.2019.01.097 doi: 10.1016/j.physa.2019.01.097
![]() |
[9] |
Q. Liu, D. Jiang, T. Hayat, A. Alsaedi, Dynamical behavior of a stochastic epidemic model for cholera, J. Franklin Inst., 115 (1927), 111–124. https://doi.org/10.1016/j.jfranklin.2018.11.056 doi: 10.1016/j.jfranklin.2018.11.056
![]() |
[10] |
P. Liu, A. Din, Impact of information intervention on stochastic dengue epidemic model, Alex. Eng. J., 60 (2021), 5725–5739. https://doi.org/10.1016/j.aej.2021.03.068 doi: 10.1016/j.aej.2021.03.068
![]() |
[11] |
Z. Wang, G. Rost, S. M. Moghadas, Delay in booster schedule as a control parameter in vaccination dynamics, J. Math. Biol., 79 (2019), 2157–2182. https://doi.org/10.1007/s00285-019-01424-6 doi: 10.1007/s00285-019-01424-6
![]() |
[12] |
R. M. Anderson, B. T. Grenfell, Quantitative investigations of different vaccination policies for the control of congentila rubella syndrome (CRS) in the United Kingdom, Epidemiol. Infect., 96 (1986), 305–333. https://doi.org/10.1017/s0022172400066079 doi: 10.1017/s0022172400066079
![]() |
[13] |
M. E. Alexander, S. M. Moghadas, P. Rohani, A. R. Summers, Modelling the effect of a booster vaccination on disease epidemiology, J. Math. Biol., 52 (2006), 290–306. https://doi.org/10.1007/s00285-005-0356-0 doi: 10.1007/s00285-005-0356-0
![]() |
[14] |
T. Marinov, R. Marinova, Inverse problem for adaptive SIR model: Application to COVID-19 in Latin America, Infect. Dis. Modell., 7 (2022), 234–148. https://doi.org/10.1016/j.idm.2021.12.001 doi: 10.1016/j.idm.2021.12.001
![]() |
[15] |
H. Qiu, Q. Wang, Q. Wu, H. Zhou, Does flattening the curve make a difference? An investigation of the COVID-19 pandemic based on an SIR model, Int. Rev. Econ. Finance, 80 (2022), 159–165. https://doi.org/10.1016/j.iref.2022.02.063 doi: 10.1016/j.iref.2022.02.063
![]() |
[16] |
S. Annas, M. I. Pratama, M. Rifandi, W. Sanusi, S. Side, Stability analysis and numerical simulation of SEIR model for pandemic COVID-19 spread in Indonesia, Chaos Solitons Fractals, 139 (2020), 110072. https://doi.org/10.1016/j.chaos.2020.110072 doi: 10.1016/j.chaos.2020.110072
![]() |
[17] |
S. Paul, A. Mahata, U. Ghosh, B. Roy, Study of SEIR epidemic model and scenario analysis of COVID-19 pandemic, Ecol. Genet. Genom., 19 (2021), 100087. https://doi.org/10.1016/j.egg.2021.100087 doi: 10.1016/j.egg.2021.100087
![]() |
[18] |
D. Efimov, R. Ushirobira, On an interval prediction of COVID-19 development based on a SEIR epidemic model, Annu. Rev. Control, 51 (2021), 477–487. https://doi.org/10.1016/j.arcontrol.2021.01.006 doi: 10.1016/j.arcontrol.2021.01.006
![]() |
[19] |
Z. Chen, L. Feng, H. A. Lay Jr, K. Furati, A. Khaliq, SEIR model with unreported infected population and dynamic parameters for the spread of COVID-19, Math. Comput. Simul., 198 (2022), 31–46. https://doi.org/10.1016/j.matcom.2022.02.025 doi: 10.1016/j.matcom.2022.02.025
![]() |
[20] |
R. Li, Y. Song, H. Wang, G. Jiang, M. Xiao, Reactive diffusion epidemic model on human mobility networks: Analysis and applications to COVID-19 in China, Physica A, 609 (2023), 128337. https://doi.org/10.1016/j.physa.2022.128337 doi: 10.1016/j.physa.2022.128337
![]() |
[21] |
F. A. C. C. Chaluba, M. O. Souza, The SIR epidemic model from a PDE point of view, Math. Comput. Modell., 53 (2011), 1568–1574. https://doi.org/10.1016/j.mcm.2010.05.036 doi: 10.1016/j.mcm.2010.05.036
![]() |
[22] |
L. Bttcher, M. Xia, T. Chou, Why case fatality ratios can be misleading: individual and population based mortality estimates and factors influencing them, Phys. Biol., 17 (2020), 065003. https://doi.org/10.1088/1478-3975/ab9e59 doi: 10.1088/1478-3975/ab9e59
![]() |
[23] |
Q. Wu, X. Fan, H. Hong, Y. Gua, Z. Liu, S. Fang, Comprehensive assessment of side effects in COVID-19 drug pipeline from a network perspective, Food Chem. Toxicol., 145 (2020), e13476. https://doi.org/10.1016/j.fct.2020.111767 doi: 10.1016/j.fct.2020.111767
![]() |
[24] |
U. Tursen, B. Tursen, T. Lotti, Cutaneous side-effects of the potential COVID-19 drugs, Dermatol. Ther., 33 (2020), 31–46. https://doi.org/10.1111/dth.13476 doi: 10.1111/dth.13476
![]() |
[25] |
P. Wintachai, K. Prathom, Stability analysis of SEIR model related to efficiency of vaccines for COVID-19 situation, Heliyon, 7 (2021), e06812. https://doi.org/10.1016/j.heliyon.2021.e06812 doi: 10.1016/j.heliyon.2021.e06812
![]() |
[26] |
P. Jarumaneeroj, P. Dusadeerungsikul, T. Chotivanich, T. Nopsopon, K. Pongpirul, An epidemiology-based model for the operational allocation of COVID-19 vaccines: A case study of Thailand, Comput. Ind. Eng., 167 (2022), 108031. https://doi.org/10.1016/j.cie.2022.108031 doi: 10.1016/j.cie.2022.108031
![]() |
[27] |
L. Böher, J. Nagler, Decisive conditions for strategic vaccination against SARS-CoV-2, Chaos, 31 (2021), 101105. https://doi.org/10.1063/5.0066992 doi: 10.1063/5.0066992
![]() |
[28] |
A. Mahata, S. Paul, S. Mukherjee, B. Roy, Stability analysis and Hopf bifurcation in fractional order SEIRV epidemic model with a time delay in infected individuals, Partial Differ. Equations Appl. Math., 5 (2022), 100282. https://doi.org/10.1016/j.padiff.2022.100282 doi: 10.1016/j.padiff.2022.100282
![]() |
[29] |
H. Yang, Y. Wang, S. Kundu, Z. Song, Z. Zhang, Dynamics of an SIR epidemic model incorporating time delay and convex incidence rate, Results Phys., 32 (2022), 105025. https://doi.org/10.1016/j.rinp.2021.105025 doi: 10.1016/j.rinp.2021.105025
![]() |
[30] |
A. Khan, R. Ikram, A. Din, U. W. Humphries, A. Akgul, Stochastic COVID-19 SEIQ epidemic model with time-delay, Results Phys., 30 (2021), 104775. https://doi.org/10.1016/j.rinp.2021.104775 doi: 10.1016/j.rinp.2021.104775
![]() |
[31] |
C. Zhu, J. Zhu, Dynamic analysis of a delayed COVID-19 epidemic with home quarantine in temporal-spatial heterogeneous via global exponential attractor method, Chaos Solitons Fractals, 143 (2021), 110546. https://doi.org/10.1016/j.chaos.2020.110546 doi: 10.1016/j.chaos.2020.110546
![]() |
[32] |
E. M. Farah, S. Amine, K. Allali, Dynamics of a time-delayed two-strain epidemic model with general incidence rates, Chaos Solitons Fractals, 153 (2021), 111527. https://doi.org/10.1016/j.chaos.2021.111527 doi: 10.1016/j.chaos.2021.111527
![]() |
[33] |
H. Li, X. Liu, R. Yan, C. Liu, Hopf bifurcation analysis of a tumor virotherapy model with two time delays, Physica A, 553 (2020), 124266. https://doi.org/10.1016/j.physa.2020.124266 doi: 10.1016/j.physa.2020.124266
![]() |
[34] |
B. Sun, M. Li, F. Zhang, H. Wang, J. Liu, The characteristics and self-time-delay synchronization of two-time-delay complex Lorenz system, J. Franklin Inst., 356 (2019), 334–350. https://doi.org/10.1016/j.jfranklin.2018.09.031 doi: 10.1016/j.jfranklin.2018.09.031
![]() |
[35] |
M. Akio, S. Ferenc, Time delays and chaos in two competing species revisited, Appl. Math. Comput., 395 (2021), 125862. https://doi.org/10.1016/j.amc.2020.125862 doi: 10.1016/j.amc.2020.125862
![]() |
[36] |
H. Zhou, Z. Wang, D. Yuan, H. Song, Hopf bifurcation of a free boundary problem modeling tumor growth with angiogenesis and two time delays, Chaos Solitons Fractals, 153 (2021), 111578. https://doi.org/10.1016/j.chaos.2021.111578 doi: 10.1016/j.chaos.2021.111578
![]() |
[37] |
A. R. Hakimi, M. Azhdari, T. Binazadeh, Limit cycle oscillator in nonlinear systems with multiple time delays, Chaos Solitons Fractals, 153 (2021), 111454. https://doi.org/10.1016/j.chaos.2021.111454 doi: 10.1016/j.chaos.2021.111454
![]() |
[38] |
A. Adhikary, A. Pal, A six compartments with time-delay model SHIQRD for the COVID-19 pandemic in India: During lockdown and beyond, Alex. Eng. J., 61 (2022), 1403–1412. https://doi.org/10.1016/j.aej.2021.06.027 doi: 10.1016/j.aej.2021.06.027
![]() |
[39] |
A. Mahata, S. Paul, S. Mukherjee, B. Roy, Stability analysis and Hopf bifurcation in fractional order SEIRV epidemic model with a time delay in infected individuals, Partial Differ. Equations Appl. Math., 5 (2022), 100282. https://doi.org/10.1016/j.padiff.2022.100282 doi: 10.1016/j.padiff.2022.100282
![]() |
[40] |
Y. Zhang, J. Jia, Hopf bifurcation of an epidemic model with a nonlinear birth in population and vertical transmission, Appl. Math. Comput., 230 (2014), 164–173. https://doi.org/10.1016/j.amc.2013.12.084 doi: 10.1016/j.amc.2013.12.084
![]() |
[41] |
X. Duan, J. Yin, X. Li, Global Hopf bifurcation of an SIRS epidemic model with age-dependent recovery, Chaos Solitons Fractals, 104 (2017), 613–624. https://doi.org/10.1016/j.chaos.2017.09.029 doi: 10.1016/j.chaos.2017.09.029
![]() |
[42] |
Z. Zhang, S. Kundu, J. P. Tripathi, S. Bugalia, Stability and Hopf bifurcation analysis of an SVEIR epidemic model with vaccination and multiple time delays, Chaos Solitons Fractals, 131 (2020), 109483. https://doi.org/10.1016/j.chaos.2019.109483 doi: 10.1016/j.chaos.2019.109483
![]() |
[43] |
H. Yang, Y. Wang, S. Kundu, Z. Song, Z. Zhang, Dynamics of an SIR epidemic model incorporating time delay and convex incidence rate, Results Phys., 32 (2022), 105025. https://doi.org/10.1016/j.rinp.2021.105025 doi: 10.1016/j.rinp.2021.105025
![]() |
[44] |
Y. Ding, L. Zheng, Mathematical modeling and dynamics analysis of delayed nonlinear VOC emission system, Nonlinear Dyn., 109 (2022), 3157–3167. https://doi.org/10.1007/s11071-022-07532-1 doi: 10.1007/s11071-022-07532-1
![]() |
[45] |
Y. Ding, L. Zheng, J. Guo, Stability analysis of nonlinear glue flow system with delay, Math. Methods Appl. Sci., 45 (2022), 6861–6877. https://doi.org/10.1002/mma.8211 doi: 10.1002/mma.8211
![]() |
[46] |
Y. Song, Y. Peng, T. Zhang, The spatially inhomogeneous Hopf bifurcation induced by memory delay in a memory-based diffusion system, J. Differ. Equations, 300 (2021), 597–624. https://doi.org/10.1016/j.jde.2021.08.010 doi: 10.1016/j.jde.2021.08.010
![]() |
[47] |
Y. Song, H. Jiang, Y. Yuan, Turing-Hopf bifurcation in the reaction-diffusion system with delay and application to a diffusive predator-prey model, J. Appl. Anal. Comput., 9 (2019), 1132–1164. https://doi.org/10.1016/j.cnsns.2015.10.002 doi: 10.1016/j.cnsns.2015.10.002
![]() |
[48] |
I. Alam, A. Radovanovic, R. Incitti, A. A. Kamau, M. Alarawi, E. I. Azhar, et al., CovMT: an interactive SARS-CoV-2 mutation tracker, with a focus on critical variants, Lancet Infect. Dis., 21 (2021), 602. https://doi.org/10.1016/s1473-3099(21)00078-5 doi: 10.1016/s1473-3099(21)00078-5
![]() |
[49] |
T. Phan, Genetic diversity and evolution of SARS-CoV-2, Infect. Genet. Evol., 81 (2020), 104260. https://doi.org/10.1016/j.meegid.2020.104260 doi: 10.1016/j.meegid.2020.104260
![]() |
[50] |
M. Pachetti, B. Marini, F. Giudici, F. Benedetti, S. Angeletti, M. Ciccozzi, et al., Impact of lockdown on COVID-19 case fatality rate and viral mutations spread in 7 countries in europe and north america, J. Transl. Med., 18 (2020), 1–7. https://doi.org/10.1186/s12967-020-02501-x doi: 10.1186/s12967-020-02501-x
![]() |
[51] |
S. Y. Tartof, J. M. Slezak, H. Fischer, V. Hong, B. K. Ackerson, O. N. Ranasinghe, et al., Effectiveness of mRNA BNT162b2 COVID-19 vaccine up to 6 months in a large integrated health system in the USA: a retrospective cohort study, Lancet, 398 (2021), 1407–1416. https://doi.org/10.1016/S0140-6736(21)02183-8 doi: 10.1016/S0140-6736(21)02183-8
![]() |
[52] |
J. L. Bayart, J. Douxfils, C. Gillot, C. David, F. Mullier, M. Elsen, et al., Waning of IgG, total and neutralizing antibodies 6 months post-vaccination with BNT162b2 in healthcare workers, Vaccines, 9 (2021), 1092. https://doi.org/10.3390/vaccines9101092 doi: 10.3390/vaccines9101092
![]() |
[53] |
S. Liu, X. Yang, Y. Bia, Y. Li, Dynamic behavior and optimal scheduling for mixed vaccination strategy with temporary immunity, Discrete Contin. Dyn. Syst., 24 (2019), 1469–1483. https://doi.org/10.3934/dcdsb.2018216 doi: 10.3934/dcdsb.2018216
![]() |
[54] |
C. T. Ng, T. C. E. Heng, D. Tsadikovich, E. Levner, A. Elalouf, S. Hovav, A multi-criterion approach to optimal vaccination planning: Method and solution, Comput. Ind. Eng., 126 (2018), 637–649. https://doi.org/10.1016/j.cie.2018.10.018 doi: 10.1016/j.cie.2018.10.018
![]() |
[55] |
M. Xia, L. Böher, T. Chou, Controlling epidemics through optimal allocation of test kits and vaccine doses across networks, IEEE Trans. Network Sci. Eng., 9 (2022), 1422–1436. https://doi.org/10.1109/TNSE.2022.3144624 doi: 10.1109/TNSE.2022.3144624
![]() |
[56] |
Z. Lv, J. Zeng, Y. Ding, X. Liu, Stability analysis of time-delayed SAIR model for duration of vaccine in the context of temporary immunity for COVID-19 situation, Electron. Res. Arch., 31 (2023), 1004–1030. https://doi.org/10.3934/era.2023050 doi: 10.3934/era.2023050
![]() |
1. | Anuj Kumar, Yasuhiro Takeuchi, Prashant K Srivastava, Stability switches, periodic oscillations and global stability in an infectious disease model with multiple time delays, 2023, 20, 1551-0018, 11000, 10.3934/mbe.2023487 | |
2. | Deepika Solanki, Sumit Kaur Bhatia, Harendra Pal Singh, Praveen Kumar, Dynamic analysis of a communicable disease fractional order model incorporating vaccination and multiple time delays, 2025, 116, 11100168, 147, 10.1016/j.aej.2024.12.055 | |
3. | Mohammed Amine Menouer, Salih Djilali, Anwar Zeb, Ilyas Khan, Abdoalrahman S.A. Omer, The role of imperfect vaccination and treatment in containing an epidemic for a delayed SVITR epidemic model, 2025, 33, 2769-0911, 10.1080/27690911.2025.2461095 |
Symbol | Descriptions | Unit |
S | Number of suspected people | 105 persons |
V | Number of vaccinated people | 105 persons |
I | Number of infected people | 105 persons |
R | Number of recovered people | 105 persons |
Λ | Population nature growth | 105 persons |
d | Population natural mortality rate | – |
c | COVID-19 mortality rate of I | – |
β | Infection rate from S to I | – |
k | Proportional coefficient of transmission | – |
α | Vaccination rate | – |
m | Transmission rate from V to R | – |
ρ | Booster vaccination rate | – |
μ | Vaccine failure rate | – |
u | Cure rate | – |
Country | Function | SSE |
Australia | y1=0.006549+4.366e−5cos(0.3491t)+8.852e−5sin(0.3491t) | 1.724e−8 |
Canada | y2=0.007141+7.21e−5cos(0.6981t)+2.463e−6sin(0.6981t) | 1.09e−7 |
Switzerland | y3=0.008143−0.0002755cos(0.2715t)+5.128e−5sin(0.2715t) | 4.291e−7 |
China | y4=0.006841−0.0002921cos(0.2853t)+0.0002776sin(0.2853t) | 1.352e−7 |
Symbol | Descriptions | Unit |
S | Number of suspected people | 105 persons |
V | Number of vaccinated people | 105 persons |
I | Number of infected people | 105 persons |
R | Number of recovered people | 105 persons |
Λ | Population nature growth | 105 persons |
d | Population natural mortality rate | – |
c | COVID-19 mortality rate of I | – |
β | Infection rate from S to I | – |
k | Proportional coefficient of transmission | – |
α | Vaccination rate | – |
m | Transmission rate from V to R | – |
ρ | Booster vaccination rate | – |
μ | Vaccine failure rate | – |
u | Cure rate | – |
Country | Function | SSE |
Australia | y1=0.006549+4.366e−5cos(0.3491t)+8.852e−5sin(0.3491t) | 1.724e−8 |
Canada | y2=0.007141+7.21e−5cos(0.6981t)+2.463e−6sin(0.6981t) | 1.09e−7 |
Switzerland | y3=0.008143−0.0002755cos(0.2715t)+5.128e−5sin(0.2715t) | 4.291e−7 |
China | y4=0.006841−0.0002921cos(0.2853t)+0.0002776sin(0.2853t) | 1.352e−7 |