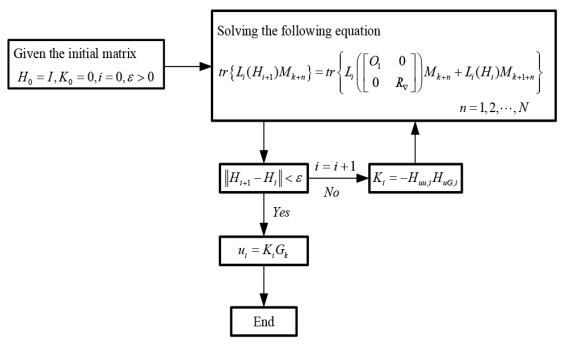
Despite the government's and development partners' unmatched efforts to ensure that every eligible person receives vaccinations, there have been concerns about vaccine fear, government mistrust, vaccine hesitancy and rejection expressed by the public, as well as various conspiracy theories involving the COVID-19 vaccines. This study assessed the public beliefs and willingness to accept COVID-19 vaccines and related factors among residents of Ondo State, Nigeria.
Using a convenience sample technique, a cross-sectional survey of the adult population was carried out in the months of February and March of 2022. Factors influencing beliefs and willingness to accept COVID-19 vaccines were found by using univariate and multivariate statistical analysis.
306 out of 323 respondents completed the survey and were included in the final analysis. The respondents mean age was 28.16 ± 16.2 years. Although n = 223, 72.9% of respondents reported to have received at least one dose of COVID-19 vaccines, (n = 205) 67.0% believed COVID-19 vaccines to be effective. Among the individuals who had not yet had any COVID-19 vaccinations, 2.6% (n = 8) of respondents were willing to accept the vaccines, whereas 14.1% (n = 43) were unwilling. Respondents' beliefs about the efficacy of COVID-19 vaccines were influenced by their gender, occupation, religion and educational attainment (p < 0.005).
The study revealed a good level of positive beliefs about the vaccine, which was mirrored in vaccination history. However, those who had not yet received the vaccine were unwilling to do so, opening the door for more aggressive risk communication to be able to alter the course of events. In addition to addressing additional COVID-19 vaccination myths, we advise policy-makers to develop communication strategies that emphasise the safety of the COVID-19 vaccine. It is advised that all relevant stakeholders be included in government COVID-19 vaccination programmes by sharing timely, transparent information that fosters accountability.
Citation: Itse Olaoye, Aniebet Ekong, Abiona Samuel, Eirini Kelaiditi, Kyriaki Myrissa, Tsemaye Jacdonmi, Famokun Gboyega. Public beliefs and willingness to accept COVID-19 vaccines among adults in South-Western Nigeria: A cross-sectional study[J]. AIMS Public Health, 2023, 10(1): 1-15. doi: 10.3934/publichealth.2023001
[1] | Abdon Atangana, Seda İğret Araz . Piecewise differential equations: theory, methods and applications. AIMS Mathematics, 2023, 8(7): 15352-15382. doi: 10.3934/math.2023785 |
[2] | Woocheol Choi, Young-Pil Choi . A sharp error analysis for the DG method of optimal control problems. AIMS Mathematics, 2022, 7(5): 9117-9155. doi: 10.3934/math.2022506 |
[3] | Xumei Zhang, Junying Cao . A high order numerical method for solving Caputo nonlinear fractional ordinary differential equations. AIMS Mathematics, 2021, 6(12): 13187-13209. doi: 10.3934/math.2021762 |
[4] | Xin Liu, Yan Wang . Averaging principle on infinite intervals for stochastic ordinary differential equations with Lévy noise. AIMS Mathematics, 2021, 6(5): 5316-5350. doi: 10.3934/math.2021314 |
[5] | Shifan Luo, Dongshu Wang, Wenxiu Li . Dynamic analysis of a SIV Filippov system with media coverage and protective measures. AIMS Mathematics, 2022, 7(7): 13469-13492. doi: 10.3934/math.2022745 |
[6] | Essam R. El-Zahar, Ghaliah F. Al-Boqami, Haifa S. Al-Juaydi . Piecewise approximate analytical solutions of high-order reaction-diffusion singular perturbation problems with boundary and interior layers. AIMS Mathematics, 2024, 9(6): 15671-15698. doi: 10.3934/math.2024756 |
[7] | Saima Rashid, Fahd Jarad, Sobhy A. A. El-Marouf, Sayed K. Elagan . Global dynamics of deterministic-stochastic dengue infection model including multi specific receptors via crossover effects. AIMS Mathematics, 2023, 8(3): 6466-6503. doi: 10.3934/math.2023327 |
[8] | Wedad Albalawi, Muhammad Imran Liaqat, Kottakkaran Sooppy Nisar, Abdel-Haleem Abdel-Aty . Qualitative study of Caputo Erdélyi-Kober stochastic fractional delay differential equations. AIMS Mathematics, 2025, 10(4): 8277-8305. doi: 10.3934/math.2025381 |
[9] | Yanshou Dong, Junfang Zhao, Xu Miao, Ming Kang . Piecewise pseudo almost periodic solutions of interval general BAM neural networks with mixed time-varying delays and impulsive perturbations. AIMS Mathematics, 2023, 8(9): 21828-21855. doi: 10.3934/math.20231113 |
[10] | Dumitru Baleanu, Babak Shiri . Nonlinear higher order fractional terminal value problems. AIMS Mathematics, 2022, 7(5): 7489-7506. doi: 10.3934/math.2022420 |
Despite the government's and development partners' unmatched efforts to ensure that every eligible person receives vaccinations, there have been concerns about vaccine fear, government mistrust, vaccine hesitancy and rejection expressed by the public, as well as various conspiracy theories involving the COVID-19 vaccines. This study assessed the public beliefs and willingness to accept COVID-19 vaccines and related factors among residents of Ondo State, Nigeria.
Using a convenience sample technique, a cross-sectional survey of the adult population was carried out in the months of February and March of 2022. Factors influencing beliefs and willingness to accept COVID-19 vaccines were found by using univariate and multivariate statistical analysis.
306 out of 323 respondents completed the survey and were included in the final analysis. The respondents mean age was 28.16 ± 16.2 years. Although n = 223, 72.9% of respondents reported to have received at least one dose of COVID-19 vaccines, (n = 205) 67.0% believed COVID-19 vaccines to be effective. Among the individuals who had not yet had any COVID-19 vaccinations, 2.6% (n = 8) of respondents were willing to accept the vaccines, whereas 14.1% (n = 43) were unwilling. Respondents' beliefs about the efficacy of COVID-19 vaccines were influenced by their gender, occupation, religion and educational attainment (p < 0.005).
The study revealed a good level of positive beliefs about the vaccine, which was mirrored in vaccination history. However, those who had not yet received the vaccine were unwilling to do so, opening the door for more aggressive risk communication to be able to alter the course of events. In addition to addressing additional COVID-19 vaccination myths, we advise policy-makers to develop communication strategies that emphasise the safety of the COVID-19 vaccine. It is advised that all relevant stakeholders be included in government COVID-19 vaccination programmes by sharing timely, transparent information that fosters accountability.
It is well known that the optimal tracking control (OTC) problem plays an important role in the field of optimal control and develops fast in applications[1,2,3,4]. The goal of OTC problem is to design a controller, which can make the output of the system track the reference trajectory by minimizing the cost function. Traditional OTC problem is realized by feedback linearization [5] and object inversion [6], but this usually requires complex mathematical analysis. As for the linear quadratic tracking (LQT) problem, the traditional method of LQT problem is to solve the algebraic Riccati equation (ARE) and the noncausal difference equation. However, these methods require accurate system model[7]. In practical situations, the system parameters are partially unknown or completely unknown, so it is impossible to be realized by traditional methods.
The key to the OTC problem is to solve Hamilton-Jacobi-Bellman (HJB) equation. However, HJB equation involves solving difference or differential equations, so it is difficult to solve it. Although dynamic programming has always been an effective method to solve the HJB equation, it is not feasible in the calculation of large dimensions because of "the curse of dimensionality". To solve the solution of the HJB equation, adaptive dynamic programming (ADP) algorithms have been widely used and developed. In [8], a policy iteration (PI) scheme was adopted to approximate the optimal control for the partly unknown continuous-time systems. In [9], B. Kiumarsi solves the LQT problem online only by measuring the input, output, and reference trajectory data of the system. In [10], a Q-learning method was proposed to calculate the optimal control, only relying on system parameters and command generators.
In recent years, stochastic system control theory has become the focus of optimal control theory because of its academic difficulty and wide application, especially the model-free SLQ optimal tracking problem has attracted more and more attention[11,12,13,14,15]. In [14], ADP algorithm based on neural networks is proposed to solve the model-free SLQ optimal tracking control problem. In addition, the Q-learning algorithm is used to solve the model-free SLQ optimal tracking control problem in [15]. For all we know, there seem to be many research results on the model-free SLQ optimal tracking problem based on ADP algorithm, but the SLQ optimal tracking problem with delays has received little attention. Time delay [16] is an important factor that cannot be ignored. It exists in many practical systems, such as industrial processes, power grids, chemical reactions, and so on [17,18,19,20]. However, in these methods[11,12,13,14,15], the influence of time delay on the system is neglected. If the time delay is ignored, it will affect the control effect and even make the system divergence. The method proposed in [16] takes into account the time delay but ignores the influence of stochastic disturbance disturbances on the system. As far as we know, there is no research on the optimal tracking problem of stochastic linear systems with delays. Therefore, how to use ADP algorithm to deal with the model-free SLQ optimal tracking control problem has important practical significance. This is the motivation we study in this paper.
The main contributions of this paper include:
(1) For stochastic linear system, this paper proposes Q-learning to model-free solve SLQ optimal tracking control problem with delays for the first time, which enhances the practicability of ADP algorithm in tracking problems.
(2) By introducing the delay factor, the influence of delays on the subsequent algorithm can be effectively eliminated.
(3) In this paper, the Q-learning algorithm is used to solve the model-free SLQ optimal tracking control problem with delays. Compared with other methods which need accurate system model to obtain the optimal control, this method makes full use of the online system state information to obtain the optimal control and avoids solving augmented stochastic algebraic equation (SAE).
The structure of this paper is organized as follows. In section 2, we give the problem formulation and conversion. In section 3, we derive the Q-learning algorithm and prove its convergence. In section 4, we give the implementation steps of Q-learning algorithm. In section 5, a simulation example is given to verify the effectiveness of the algorithm. In section 6, the conclusion is given.
Consider the following linear stochastic systems with delays
xk+1=Axk+Adxk−d+Buk+Bduk−d+(Cxk+Cdxk−d+Duk+Dduk−d)ωk,yk=Exk+Edxk−d | (2.1) |
where xk∈Rn is the system state vector, uk∈Rm is the control input vector, yk∈Rq is the system output, while xk−d,uk−d and yk−d are the delay variables with delay index d∈N. A∈Rn×n, B∈Rn×m, C∈Rn×n, D∈Rn×m, E∈Rq×n are given constant, Ad∈Rn×n, Bd∈Rn×m, Cd∈Rn×n, Dd∈Rn×m, Ed∈Rq×n are their corresponding delay dynamics matrices. One-dimensional stochastic disturbance sequence ωk is defined on the given probability space (Ω,F,P,Fk), and meets the following condition E(ωk∣Fk)=0, E(ω2k∣Fk)=1. The initial state x0 is irrelevant with ωk.
Assume the reference trajectory of SLQ optimal tracking control is generated by a command generator
rk+1=Frk | (2.2) |
where rk∈Rq represents the reference system trajectory, and F is the constant matrix.
The tracking error can be expressed as
ek=yk−rk | (2.3) |
where rk is the reference trajectory.
The goal of the SLQ optimal tracking problem with delays is to design an optimal controller, which can not only ensure that the output of the target system track the reference trajectory stably, but also minimize the cost function. The cost function is denoted as
J(xk,rk,uk)=E∞∑i=kUi(xi,xi−d,ui) | (2.4) |
where Ui(xi,xi−d,ui)=(yi−ri)TO(yi−ri)+uTiRui+uTi−dRdui−d is the utility function. O=OT∈Rq×q≥0, R=RT∈Rm×m≥0, Rd=RTd∈Rm×m≥0 are the constant matrices.
Only when F is Hurwitz can the cost function (2.4) be used, that is, the reference trajectory system is required to be asymptotically stable. If the reference trajectory does not tend to zero with time delay, then the cost function (2.4) will be unbounded. In practice, this condition is difficult to achieve. Therefore, a discount factor γ is introduced into the cost function (2.4) to relax this restriction. Based on (2.4), the cost function with discount factor is redefined as
J(xk,rk,uk)=E∞∑i=kγi−kUi(xi,xi−d,ui)=E∞∑i=kγi−k(yi−ri)TO(yi−ri)+uTiRui+uTi−dRdui−d | (2.5) |
where 0<γ≤1 is the discount factor.
Definition 1 ([21]). uk is called mean-square stabilizing at e0 if there exists a linear feedback form of uk for every initial state e0 satisfies limk→∞E(eTkek)=0. The system (2.3) with a mean-square stabilizing control uk is called mean-square stabilizable.
Definition 2 ([21]). uk is said to be admissible if uk satisfies the following: (1) uk is a Fk adapted and measurable stochastic process; (2) uk is mean-square stabilizing; (3) It enables the cost function to reach the minimum value.
The goal of this paper is to seek an admissible control, which not only minimizes the cost function (2.5) but also stabilizes the system (2.3) for each initial state e0. We denote the optimal cost function as follows
V(e0)=minuJ(e0,u). | (2.6) |
In order to achieve the above goal, this paper establishes an augmented system composed of system (2.1) and the reference trajectory system (2.2), and then transforms the optimal tracking problem into an optimal regulation problem.
The system (2.1) can be rewritten as the following equivalent form:
xk+1=[AAd][xkxk−d]+[BBd][ukuk−d]+([CCd][xkxk−d]+[DDd][ukuk−d])ωk,yk=[EEd][xkxk−d]. | (2.7) |
According to [16,22,23], we define the delay operator ∇d satisfies ∇dxk=xk−d and (∇dxk)T=xTk−d. Then, the system (2.7) can be expressed as
xk+1=A∇xk+B∇uk+(C∇xk+D∇uk)ωk,yk=E∇xk | (2.8) |
where A∇=A+Ad∇d, B∇=B+Bd∇d, C∇=C+Cd∇d, D∇=D+Dd∇d, E∇=E+Ed∇d.
Based on the system (2.1) and the reference trajectory system (2.2), the augmented system can be defined as
Gk+1=[xk+1rk+1]=[A∇+C∇ωk00F][xkrk]+[B∇+D∇ωk0]uk=TGk+B0uk | (2.9) |
where Gk=[xkrk]∈Rn+q, T∈R(n+q)×(n+q), B0∈R(n+q)×m.
Based on the augmented system (2.9), the cost function (2.5) can be expressed as
J(Gk,uk)=E∞∑i=kγi−k[GTiO1Gi+uTiR∇ui] | (2.10) |
where O1=[E−I]TO[E−I]∈R(n+q)×(n+q), R∇=R+Rd∇d.
The state feedback linear controller is defined as
uk=KGk,K∈Rm×(n+q) | (2.11) |
where K represents the control gain matrix of the system.
Substituting (2.11) into (2.10), the cost function (2.10) can be transformed into
J(Gk,K)=E∞∑i=kγi−kGTi[O1+KTR∇K]Gi. | (2.12) |
Therefore, the target of SQL optimal tracking problem with delays can be further expressed as
V(G0,K)=minKJ(G0,K). | (2.13) |
Definition 3. The SLQ optimal control problem is well posed if
−∞<V(G0,K)<+∞. |
Before solving the SLQ control problem, we need to know whether it is well-posed. Therefore, we give the following lemma first.
Lemma 1. If there exists an admissible control uk=KGk, then the SLQ optimal tracking control is well-posed, and the cost function can be expressed as
J(Gk,K)=E(GTkPGk) | (2.14) |
where the matrix P∈R(n+q)×(n+q) satisfies the following augmented SAE
P=γ(A1+B1K)TP(A1+B1K)+γ(C1+D1K)TP(C1+D1K)+O1+KTR∇K | (2.15) |
where A1=[A∇00F]∈R(n+q)×(n+q), B1=[B∇0]∈R(n+q)×m, C1=[C∇000]∈R(n+q)×(n+q), D1=[D∇0]∈R(n+q)×m.
Proof. Assuming that the control uk is admissible and the matrix P satisfies (2.15), then
E∞∑i=k[γGi+1TPGi+1−GiTPGi]=E∞∑i=k{γ[(A1+B1K)Gi+(C1ωi+D1Kωi)Gi]TP[(A1+B1K)Gi+(C1ωi+D1Kωi)Gi]−GiTPGi}=E∞∑i=k{GiT[γ(A1+B1K)TP(A1+B1K)+γ(C1+D1K)TP(C1+D1K)−P]Gi}. |
Based on (2.12) and (2.15), we have
J(Gk,K)=E∞∑i=kγi−kGTi[O1+KTR∇K]Gi]=E∞∑i=kγi−kGTi[P−γ(A1+B1K)TP(A1+B1K)−γ(C1+D1K)TP(C1+D1K)]Gi=−E∞∑i=kγi−k[γGTi+1PGi+1−GTiPGi]=E(GTkPGk)−limi→∞γi−k+1E(GTiPGi)=E(GTkPGk). |
Since the feedback control uk is admissible, we can obtain J(Gk,K)=E(GkTPGk), which satisfies the well-posedness of SLQ optimal tracking control problem.
To make sure the mean-square stable control, we make the following assumption.
Assumption 1. The system (2.9) is mean-square stabilizable.
At present, ADP algorithm has achieved great success in the optimal tracking control of deterministic systems [24,25,26], which inspires us to transform stochastic problems into deterministic problems through system transformation.
Let Mk=E(GkGTk), then the system (2.9) can be converted to
Mk+1=E(Gk+1GTk+1)=E((TGk+B0uk)(TGk+B0uk)T)=(A1+B1K)Mk(A1+B1K)T+(C1+D1K)Mk(C1+D1K)T | (2.16) |
where Mk∈R(n+q)×(n+q) is the state of a deterministic system and M0 is the initial state.
Therefore, the cost function (2.10) can be rewritten as
J(Mk,K)=tr{∞∑i=kγi−k[(O1+KTR∇K)Mk]}. | (2.17) |
Remark 1. After system transformation, the stochastic system is transformed into deterministic system. The system (2.17) completely gets rid of stochastic disturbance ωk and will only be dependent on the initial state M0 and control gain matrix K, which makes preparation for the derivation and application of Q-learning algorithm.
In this paper, Q-learning method is used to solve the SLQ optimal tracking problem, which avoids the need for accurate system model. Thus we first give the formula of the optimal control and the corresponding augmented SAE.
Lemma 2. Given the admissible control uk, we can get the following optimal control
u∗k=K∗Gk=−(R∇+γBT1PB1)−1γ(BT1PA1+DT1PD1)Gk | (3.1) |
and the optimal cost function
V(Gk)=E(GTkPGk)=tr(PMk) | (3.2) |
where the matrix P satisfies the following augmented SAE
{P=O1+γ(AT1PA1+CT1PC1)−γ(AT1PB1+CT1PD1)×(R∇+γBT1PB1+γDT1PD1)−1γ(BT1PA1+DT1PC1)R∇+γBT1PB1+DT1PD1>0. | (3.3) |
Proof. Suppose uk is an admissible control. According to Lemma 1 and (2.17), the cost function can be written as
J(Mk,K)=tr{∞∑i=kγi−k[(O1+KTR∇K)Mi]}=tr{(O1+KTR∇K)Mi}+tr{∞∑i=k+1γi−k[(O1+KTR∇K)Mi]}=tr{(O1+KTR∇K)Mi}+J(Mk+1,K). | (3.4) |
According to Bellman optimality principle, the optimal cost function satisfies
V(Mk)=minK{tr{(O1+KTR∇K)Mk}+V(Mk+1)}. | (3.5) |
The optimal control gain matrix can be obtained as follow
K∗(Mk)=argminK{tr{(O1+KTR∇K)Mk}+V(Mk+1)}. | (3.6) |
Considering the first-order necessary condition
∂[tr{(O1+KTR∇K)Mk}+V(Mk+1)]∂K=0, | (3.7) |
we can obtain
(R∇+γBT1PB1+γDT1PDT)KGk+γ(BT1PA1+DT1PC1)Gk=0 | (3.8) |
where the matrix P satisfies augmented SAE (2.15).
Supposing R∇+γBT1PB1+γDT1PDT>0, we have
K∗=−(R∇+γBT1PB1)−1γ(BT1PA1+DT1PD1). | (3.9) |
When taking (3.9) into the (2.15), we can obtain
P=O1+γ(AT1PA1+CT1PC1)−γ(AT1PB1+CT1PD1)×(R∇+γBT1PB1+γDT1PDT)−1γ(BT1PA1+DT1PC1). | (3.10) |
From Lemma 2, the SQL optimal tracking problem can be dealt with by the solution of augmented SAE (3.3). However, solving augmented SAE (3.3) requires accurate system model, so this method is not feasible when the dynamics are unknown.
To solve model-free SQL optimal tracking problem with delays, we give the definition of the Q function and the corresponding matrix H.
Based on (2.10) and Bellman optimality principle, we know that the optimal cost function satisfies Hamilton Jacobi Bellman (HJB) equation
V(Gk)=minuk{E[GTkO1Gk+uTkR∇uk]+γV(Gk+1)}. | (3.11) |
The Q-function is defined as
Q(Gk,uk)=E[GkTO1Gk+uTkR∇uk]+γV(Gk+1). | (3.12) |
According to Lemma 1, V(Gk+1) can be written as
V(Gk+1)=E(GTk+1PGk+1)=E{(TGk+B0uk)TP(TGk+B0uk)}=E{[(A1Gk+C1ωkGk)+(B1uk+D1ωkuk)]TP[(A1Gk+C1ωkGk)+(B1uk+D1ωkuk)]}. | (3.13) |
Substitute (3.13) into (3.12), we can get
Q(Gk,uk)=E{[Gkuk]T[HGGHGuHuGHuu][Gkuk]}=E{[Gkuk]TH[Gkuk]} | (3.14) |
where H=HT∈R(n+q+m)×(n+q+m),
H=[HGGHGuHuGHuu]=[O1+γAT1PA1+γCT1PC1γAT1PB1+γCT1PD1γBT1PA1+γDT1PC1γBT1PB1+γDT1PD1+R∇]. | (3.15) |
Let ∂Q(Gk,uk)∂uk=0, then the optimal control can be obtained as follow
u∗k=−H−1uuHuGGk. | (3.16) |
From Lemma 1 and (3.15), we can know the relationship between matrix P and matrix H.
P=[IKT]H[IKT]T. | (3.17) |
As can be seen from (3.16), the optimal control only depends on the matrix H, which is completely get rid of the constraints of the system parameters. Next, we will present the Q-learning iterative algorithm for estimating the matrix H.
In this section, we propose Q-learning iterative algorithm based on the Ⅵ. This method starts with the initial value Q0(Gk,uk)=0 and the initial admissible control u0(Gk), Q1(Gk,uk) will be updated by the initial value and the initial control as follows
Q1(Gk,uk)=E[GkTO1Gk+uT0(Gk)R∇u0(Gk)]+γQ0(Gk+1,u0(Gk+1)). | (3.18) |
The control is updated as follows
u1(Gk)=argminu(Gk)Q1(Gk,uk) | (3.19) |
for i≥1, Q-learning algorithm iterates between
Qi+1(Gk,uk)=E[GkTO1Gk+uTi(Gk)R∇ui(Gk)]+γQi(Gk+1,ui(Gk+1)) | (3.20) |
and
ui+1(Gk)=argminuk{E[GTkO1Gk+uTkR∇uk]+minuk+1Qi(Gk+1,uk+1)} | (3.21) |
where i is the iteration index and k is time index.
According to (3.14), the Q function can be rewritten as
Qi+1(Gk,uk)=[GkTuTi(Gk)]Hi+1[GkTuTi(Gk)]T=E{[GkTuTi(Gk)][O100R∇][GkTuTi(Gk)]T+γ[Gk+1TuTi(Gk+1)]Hi[Gk+1TuTi(Gk+1)]T} | (3.22) |
and we can obtain the optimal controller
ui(Gk)=−H−1uu,iHuG,iGk. | (3.23) |
According to (3.17), we can get
Pi=[IKTi]Hi[IKTi]T. | (3.24) |
Before proving the convergence of Q-learning algorithm, we first give the following two lemmas.
Lemma 3. Q-learning algorithm (3.22) and (3.23) is equivalent to
Pi+1=O1+γ(AT1PiA1+CT1PiC1)−γ(AT1PiB1+CT1PiD1)×(R+γBT1PiB1+γDT1PiD1)−1γ(BT1PiA1+DT1PiC1). | (3.25) |
Proof. According to (2.11), the last term of (3.22) can be written as
E{[Gk+1TuTi(Gk+1)]Hi[Gk+1TuTi(Gk+1)]T}=E{Gk+1T[IKTi]Hi[IKTi]TGk+1}=E{[(A1Gk+C1ωkGk)+(B1ui(Gk)+D1ωkui(Gk))]T[IKTi]Hi[IKTi]T(A1Gk+C1ωkGk)+(B1ui(Gk)+D1ωkui(Gk))]}=E{[GkTuiT(Gk)][A1B1]T[IKiT]Hi[IKiT]T[A1B1][GkTuiT(Gk)]T+[GkTuiT(Gk)][C1D1]T[IKiT]Hi[IKiT]T[C1D1][GkTuiT(Gk)]T}. | (3.26) |
Substitute (3.26) into (3.22), according to (3.24), we can get
Hi+1=[O100R∇]+[γAT1PiA1γAT1PiB1γBT1PiA1γBT1PiB1]+[γCT1PiC1γCT1PiD1γDT1PiC1γDT1PiD1]. | (3.27) |
Based on (3.24), we have
Pi+1=[IKTi+1]Hi+1[IKTi+1]T. | (3.28) |
Substitute (3.27) into (3.28), we can get
Pi+1=O1+γ(AT1PiA1+CT1PiC1)−γ(AT1PiB1+CT1PiD1)×(R+γBT1PiB1+γDT1PiD1)−1γ(BT1PiA1+DT1PiC1) | (3.29) |
where R∇+γBT1PB1+DT1PD1>0.
Lemma 4 ([27]). The value iteration algorithm iterates between
Vi+1(Gk)=E(GTk(O1+KiTR∇Ki)Gk)+γVi(Gk+1) | (3.30) |
and
Ki+1=argminK{E(GTk(O1+KiTR∇Ki)Gk)+γVi(Gk+1)} | (3.31) |
is the convergence, then
limi→∞Vi(Gk)=V(Gk)=E(GkTPGk)=tr{PMk}, |
limi→∞Ki=K∗=−(R∇+γBT1PB1+γDT1PD1)−1γ(BT1PA1+DT1PC1) |
where the matrix P satisfies the augmented SAE (3.3).
Theorem 3.1. Assuming that system (2.9) is mean-square stabilizable, the matrix sequence {Hi} calculated by Q-learning algorithm (3.22) converges to matrix H and the matrix sequence {Pi} calculated by (3.24) converges to the solution P of augmented SAE (3.3).
Proof. According to Lemma 4, (3.30) can be rewritten as
Vi+1(Gk)=E(GkTPi+1Gk)=E[GkT(O1+KiR∇Ki)Gk]+E(GTk+1PiGk+1)=E{GkT(O1+KiR∇Ki)Gk+[(A1+B1K)Gi+(C1ωi+D1Kωi)Gi]TP[(A1+B1K)Gi+(C1ωi+D1Kωi)Gi]}=E(GiT[(A1+B1K)TP(A1+B1K)+(C1+D1K)TP(C1+D1K)+O1+KiTR∇Ki]Gi). | (3.32) |
We can update the control gain matrix by (3.31) as follows
Ki=−(R∇+γBT1PiB1+γDT1PiD1)−1γ(BT1PiA1+DT1PiC1). | (3.33) |
Substituting (3.33) into (3.32), we can get
Pi+1=O1+γ(AT1PiA1+CT1PiC1)−γ(AT1PiB1+CT1PiD1)×(R+γBT1PiB1+γDT1PiD1)−1γ(BT1PiA1+DT1PiC1). | (3.34) |
According to Lemmas 3 and 4, we can conclude limi→∞Pi=P. when i→∞, the matrix P satisfies
P=O1+γ(AT1PA1+CT1PC1)−γ(AT1PB1+CT1PD1)×(R+γBT1PB1+γDT1PD1)−1γ(BT1PA1+DT1PC1). | (3.35) |
Based on (3.27), we can know H satisfies limi→∞Hi=H, where
H=[γAT1PA1+γCT1PC1+Q1γAT1PB1+γCT1PD1BT1PA1+γDT1PC1γBT1PB1+γDT1PD1+R∇]. | (3.36) |
So the Q-learning algorithm converges.
Due to the existence of stochastic disturbance, the output trajectory of the system is uncertain, and the cost function has expectations, the online algorithm cannot achieve the function. Therefore, it is necessary to transform the stochastic Q-learning algorithm into a deterministic Q-learning algorithm. In this section, we will give the implementation steps of deterministic Q-learning algorithm. The flow chart of Q learning algorithm is shown in Figure 1.
According to Eq (2.11), the left side of (3.22) can be simplified to
E{[GkTuTi(Gk)]Hi+1[GkTuTi(Gk)]}=E{GkT[IKTi]Hi+1[IKTi]TGk}=tr{[IKTi]Hi+1[IKTi]TMk}. | (4.1) |
The right side of (3.22) can be simplified as
E{GkT[IKTi][O100R∇][IKTi]TGk+Gk+1T[IKTi]Hi[IKTi]TGk+1}=tr{[IKTi][O100R∇][IKTi]TMk+[IKTi]Hi[IKTi]TMk+1}. | (4.2) |
For simplicity, let
Li(Hi)=[IKTi]Hi[IKTi]T,i=1,2,3,⋯. | (4.3) |
Then (3.22) can be simplified as
tr{Li(Hi+1)Mk}=tr{Li([O100R∇])Mk+Li(Hi)Mk+1}. | (4.4) |
The Q-learning iterative algorithm consisting of (4.4) and (3.23) only relies on determining the state Mk of the system (2.16) and iteratively controlling the gain matrix Ki, avoiding the constraints of system parameters and stochastic disturbance.
Remark 2. The Q-learning algorithm based on Ⅵ is performed online and solves (4.4) using least squares (LS) without knowing augmented system. In fact, (4.4) is a scalar equation and H is a symmetric (n+q+m)×(n+q+m) matrix with (n+q+m)×(n+q+m+1)/2 independent elements. Therefore, at least (n+q+m+1)×(n+q+m+1)/2 data tuples are required before (4.4) can be solved using LS.
Remark 3. Q-learning algorithm based on Ⅵ requires a persistent excitation (PE) condition [28] to ensure the sufficient exploration of the state space.
In this section, a simulation example is given to illustrate the effectiveness of Q-learning algorithm. Consider the following stochastic linear system with delays
xk+1=Axk+Adxk−d+Buk+Bduk−d+(Cxk+Cdxk−d+Duk+Dduk−d)ωk,yk=Exk+Edxk−d |
in which A=(0.2−0.80.5−0.7), Ad=(0.2−0.20.10.15), B=(0.03−0.5), Bd=(0.3−0.2), C=(−0.040.4−0.30.13), Cd=(0.2−0.10.20.11), D=(0.05−0.3), Dd=(0.10.1), E=(33), Ed=(0.10.12).
Suppose the reference trajectory is as follows
rk+1=−rk |
where r0=1.
The cost function is considered as (2.5) with R=1, Rd=1, O=10 and delay index d=1. The initial state for augmented system (2.9) is chosen as G0=[10−101]T. The initial control gain matrix is selected as K=[000]. In each iteration of the algorithm, 21 samples are collected to update the control gain matrix K.
In order to verify the effectiveness of the iterative Q-learning algorithm, we compared K with optimal solution K∗ solved by SAE (3.1). Figure 2 shows the control gain matrix K converges to the optimal control gain matrix K∗ as the number of iterations increases. Figure 3 shows the convergence process of H to its optimal values H∗, which can be calculated by (3.15). The goal of the optimal tracking problem is to trace the reference signal trajectory. In Figure 4, the expectation of system output E(y) can track the reference trajectory rk. This further proves the effectiveness of the proposed Q-learning algorithm.
For the model-free SLQ optimal tracking problem with delays, Q-learning algorithm based on Ⅵ is proposed in this paper. This method makes full use of the system information to approximate the optimal control online, and never needs the system parameter information. In the iterative process of the algorithm, the H matrix sequence and the control gain matrix K sequence are guaranteed to approximate the optimal value. Finally, the simulation results show that the system output can track the reference trajectory effectively.
The authors declare that they have no conflicts of interest.
[1] | World Health OrganizationWHO Director-General's opening remarks at the media briefing on COVID-19-11 March 2020 (2020). Available from: https://www.who.int/dg/speeches/detail/who-director-general-s-opening-remarks-at-the-media-briefing-on-covid-19---11-march-2020. |
[2] | World Health OrganizationCoronavirus disease (COVID-19) weekly epidemiological update and weekly operational update. Available from: https://www.who.int/emergencies/diseases/novel-coronavirus-2019/situation-reports. |
[3] | World Health OrganizationCOVID-19 vaccines. Available from: https://www.who.int/emergencies/diseases/novel-coronavirus-2019/covid-19-vaccines. |
[4] | NCDC COVID-19 Nigeria Sitrep. Available from: https://covid19.ncdc.gov.ng/. |
[5] | Landmark moment as first NHS patient receives COVID-19 vaccination. Available from: https://www.england.nhs.uk/2020/12/landmark-moment-as-first-nhs-patient-receives-covid-19-vaccination/. |
[6] | Ritchie H, Mathieu E, Rodés-Guirao L, et al. (2020) Coronavirus pandemic (COVID-19). Our world in data . |
[7] | World Health OrganizationCOVAX updates participants on delivery delays for vaccines from Serum Institute of India (SII) and AstraZenec (2021). Available from: https://www.gavi.org/news/media-room/covax-updates-participants-delivery-delays-vaccines-serum-institute-india-sii-az. |
[8] | PremiumTimesFirst phase of COVID-19 vaccination only for health, frontline workers–Lagos Govt 2021 (2021). Available from: https://www.premiumtimesng.com/regional/ssouth-west/449895-first-phase-of-covid-19-vaccination-only-for-health-frontline-workers-lagos-govt.html. |
[9] | National Primary Health Care Development Agency COVID-19 Vaccination info. Available from: https://nphcda.gov.ng/. |
[10] | Nigeria Health WatchConspiracy theories and COVID-19 vaccine introduction in Nigeria 2021 (2021). Available from: http://nigeriahealthwatch.com/conspiracy-theories-and-covid-19-vaccine-introduction-in-Nigeria/. |
[11] |
Adejumo OA, Ogundele OA, Madubuko CR, et al. (2021) Perceptions of the COVID-19 vaccine and willingness to receive vaccination among health workers in Nigeria. Osong Public Health Res Perspec 12: 236. https://doi.org/10.24171/j.phrp.2021.0023 ![]() |
[12] |
Tobin EA, Okonofua M, Adeke A, et al. (2021) Willingness to accept a COVID-19 vaccine in Nigeria: a population-based cross-sectional study. Cent Afr J Public Health 7: 53. https://doi.org/10.11648/j.cajph.20210702.12 ![]() |
[13] |
Adedeji-Adenola H, Olugbake OA, Adeosun SA (2022) Factors influencing COVID-19 vaccine uptake among adults in Nigeria. PLoS One 17: e0264371. https://doi.org/10.1371/journal.pone.0264371 ![]() |
[14] | Okehie-Offoha MU, Sadiku MN (1996) Ethnic and Cultural Diversity in Nigeria.Africa World Press. |
[15] | National Health Management Information System, Nigeria. Available from: https://www.dhis2nigeria.org.ng |
[16] | National Bureau of StatisticsNigeria national immunization coverage survey (nics): national brief (2018). |
[17] | Macro ICF (2008) Nigeria demographic and health survey 2008. National Population Commission . Available from: https://dhsprogram.com/pubs/pdf/fr222/fr222.pdf. National Population Commission and MEASURE DHS ICF Macro, 2009 |
[18] | Dhand NK, Khatkar MS (2014) Statulator: An online statistical calculator. Sample Size Calculator for Estimating a Single Proportion . Available from: http://statulator.com/SampleSize/ss1P.html. |
[19] |
Sherman SM, Smith LE, Sim J, et al. (2021) COVID-19 vaccination intention in the UK: results from the COVID-19 vaccination acceptability study (CoVAccS), a nationally representative cross-sectional survey. Hum Vaccin Immunothe 17: 1612-1621. https://doi.org/10.1080/21645515.2020.1846397 ![]() |
[20] | Eze UA, Ndoh KI, Ibisola B, et al. (2021) Determinants for acceptance of COVID-19 vaccine in Nigeria. Cureus 13. https://doi.org/10.7759/cureus.19801 |
[21] | Olomofe CO, Soyemi VK, Udomah BF, et al. (2021) Predictors of uptake of a potential Covid-19 vaccine among Nigerian adults. medRxiv 2012–20. https://doi.org/10.1101/2020.12.28.20248965 |
[22] | Sulat JS, Prabandari YS, Sanusi R, et al. (2018) The validity of health belief model variables in predicting behavioral change: A scoping review. Health Educ . https://doi.org/10.1108/HE-05-2018-0027 |
[23] |
Wong MCS, Wong ELY, Huang J, et al. (2021) Acceptance of the COVID-19 vaccine based on the health belief model: A population-based survey in Hong Kong. Vaccine 39: 1148-1156. https://doi.org/10.1016/j.vaccine.2020.12.083 ![]() |
[24] |
Wong LP, Alias H, Wong PF, et al. (2020) The use of the health belief model to assess predictors of intent to receive the COVID-19 vaccine and willingness to pay. Hum Vaccin Immunother 16: 2204-2214. https://doi.org/10.1080/21645515.2020.1790279 ![]() |
[25] |
Erubami JA, Okpeki PI, Ohaja EU, et al. (2022) Beyond Health Belief: Modeling the Predictors of COVID-19 Vaccine Uptake among Social Media Users in Nigeria. Studies in Media and Communication 10: 39-52. https://doi.org/10.11114/smc.v10i2.5600 ![]() |
[26] |
Faries MD (2016) Why we don't “just do it” understanding the intention-behavior gap in lifestyle medicine. Am J Lifestyle Med 10: 322-329. https://doi.org/10.1177/1559827616638017 ![]() |
[27] |
Abubakar AA, Shehu AU, Umar AA, et al. (2022) Willingness to accept Covid 19 Vaccines in a Rural Community in Kaduna State, Northwestern Nigeria. Int J Infect Di 116: S62. https://doi.org/10.1016/j.ijid.2021.12.145 ![]() |
[28] | Apuke OD, Asude Tunca E (2022) Modelling the Factors That Predict the Intention to Take COVID-19 Vaccine in Nigeria. J Asian Afr Stud 00219096211069642. https://doi.org/10.1177/00219096211069642 |
[29] |
Ekowo OE, Manafa C, Isielu RC, et al. (2022) A cross-sectional regional study looking at the factors responsible for the low COVID-19 vaccination rate in Nigeria. Pan Afr Med J 41. https://doi.org/10.11604/pamj.2022.41.114.30767 ![]() |
[30] | Joshi A, Kaur M, Kaur R, et al. (2021) Predictors of COVID-19 vaccine acceptance, intention, and hesitancy: a scoping review. Front Public Health 9. https://doi.org/10.3389/fpubh.2021.698111 |
[31] |
Anakpo G, Mishi S (2022) Hesitancy of COVID-19 vaccines: Rapid systematic review of the measurement, predictors, and preventive strategies. Hum Vaccin Immunother 18: 2074716. https://doi.org/10.1080/21645515.2022.2074716 ![]() |
[32] |
Marzo RR, Ahmad A, Islam MS, et al. (2022) Perceived COVID-19 vaccine effectiveness, acceptance, and drivers of vaccination decision-making among the general adult population: A global survey of 20 countries. PLoS Negl Trop Dis 16: e0010103. https://doi.org/10.1371/journal.pntd.0010103 ![]() |
[33] |
Ackah BBB, Woo M, Stallwood L, et al. (2022) COVID-19 vaccine hesitancy in Africa: a scoping review. Glob Health Res Policy 7: 1-20. https://doi.org/10.1186/s41256-022-00255-1 ![]() |
[34] |
Jegede AS (2007) What led to the Nigerian boycott of the polio vaccination campaign?. PLoS Med 4: e73. https://doi.org/10.1371/journal.pmed.0040073 ![]() |
[35] | Al-Mustapha AI, Okechukwu O, Olayinka A, et al. (2022) A national survey of COVID-19 vaccine acceptance in Nigeria. Vaccine . https://doi.org/10.1016/j.vaccine.2022.06.050 |
[36] | Ayandele O, Okafor CT, Oyedele O The role of Nigeria's faith-based organisations in tackling health crises like COVID-19 (2021). |
[37] |
Angelo AT, Alemayehu DS, Dachew AM (2021) Health care workers intention to accept COVID-19 vaccine and associated factors in southwestern Ethiopia, 2021. PLoS One 16: e0257109. https://doi.org/10.1371/journal.pone.0257109 ![]() |
[38] |
Qattan AMN, Alshareef N, Alsharqi O, et al. (2021) Acceptability of a COVID-19 vaccine among healthcare workers in the Kingdom of Saudi Arabia. Front Med (Lausanne) 8: 644300. https://doi.org/10.3389/fmed.2021.644300 ![]() |
[39] |
Andrade C (2021) The inconvenient truth about convenience and purposive samples. Indian J Psychol Med 43: 86-88. https://doi.org/10.1177/0253717620977000 ![]() |
1. | Heng Zhang, Na Li, Data‐driven policy iteration algorithm for continuous‐time stochastic linear‐quadratic optimal control problems, 2024, 26, 1561-8625, 481, 10.1002/asjc.3223 |