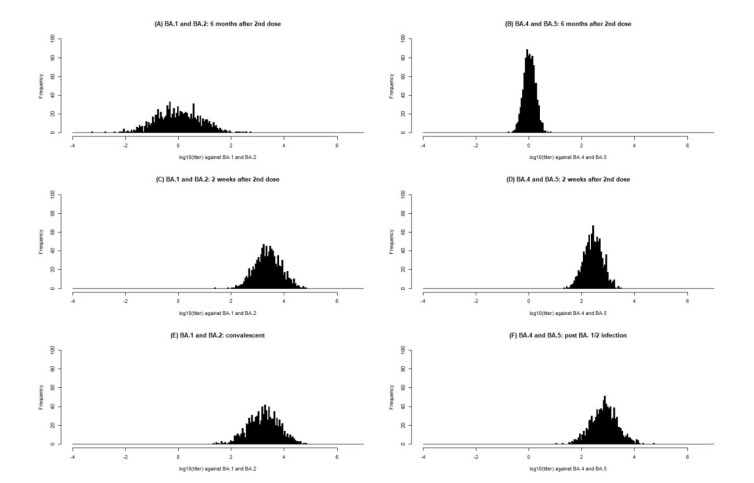
With continuing emergence of new SARS-CoV-2 variants, understanding the proportion of the population protected against infection is crucial for public health risk assessment and decision-making and so that the general public can take preventive measures. We aimed to estimate the protection against symptomatic illness caused by SARS-CoV-2 Omicron variants BA.4 and BA.5 elicited by vaccination against and natural infection with other SARS-CoV-2 Omicron subvariants. We used a logistic model to define the protection rate against symptomatic infection caused by BA.1 and BA.2 as a function of neutralizing antibody titer values. Applying the quantified relationships to BA.4 and BA.5 using two different methods, the estimated protection rate against BA.4 and BA.5 was 11.3% (95% confidence interval [CI]: 0.01–25.4) (method 1) and 12.9% (95% CI: 8.8–18.0) (method 2) at 6 months after a second dose of BNT162b2 vaccine, 44.3% (95% CI: 20.0–59.3) (method 1) and 47.3% (95% CI: 34.1–60.6) (method 2) at 2 weeks after a third BNT162b2 dose, and 52.3% (95% CI: 25.1–69.2) (method 1) and 54.9% (95% CI: 37.6–71.4) (method 2) during the convalescent phase after infection with BA.1 and BA.2, respectively. Our study indicates that the protection rate against BA.4 and BA.5 are significantly lower compared with those against previous variants and may lead to substantial morbidity, and overall estimates were consistent with empirical reports. Our simple yet practical models enable prompt assessment of public health impacts posed by new SARS-CoV-2 variants using small sample-size neutralization titer data to support public health decisions in urgent situations.
Citation: Yuta Okada, Taishi Kayano, Asami Anzai, Tong Zhang, Hiroshi Nishiura. Protection against SARS-CoV-2 BA.4 and BA.5 subvariants via vaccination and natural infection: A modeling study[J]. Mathematical Biosciences and Engineering, 2023, 20(2): 2530-2543. doi: 10.3934/mbe.2023118
[1] | Salman Safdar, Calistus N. Ngonghala, Abba B. Gumel . Mathematical assessment of the role of waning and boosting immunity against the BA.1 Omicron variant in the United States. Mathematical Biosciences and Engineering, 2023, 20(1): 179-212. doi: 10.3934/mbe.2023009 |
[2] | Sarafa A. Iyaniwura, Rabiu Musa, Jude D. Kong . A generalized distributed delay model of COVID-19: An endemic model with immunity waning. Mathematical Biosciences and Engineering, 2023, 20(3): 5379-5412. doi: 10.3934/mbe.2023249 |
[3] | Kimihito Ito, Chayada Piantham, Hiroshi Nishiura . Estimating relative generation times and reproduction numbers of Omicron BA.1 and BA.2 with respect to Delta variant in Denmark. Mathematical Biosciences and Engineering, 2022, 19(9): 9005-9017. doi: 10.3934/mbe.2022418 |
[4] | Yangyang Yu, Yuan Liu, Shi Zhao, Daihai He . A simple model to estimate the transmissibility of the Beta, Delta, and Omicron variants of SARS-COV-2 in South Africa. Mathematical Biosciences and Engineering, 2022, 19(10): 10361-10373. doi: 10.3934/mbe.2022485 |
[5] | Fang Wang, Lianying Cao, Xiaoji Song . Mathematical modeling of mutated COVID-19 transmission with quarantine, isolation and vaccination. Mathematical Biosciences and Engineering, 2022, 19(8): 8035-8056. doi: 10.3934/mbe.2022376 |
[6] | Ugo Avila-Ponce de León, Angel G. C. Pérez, Eric Avila-Vales . Modeling the SARS-CoV-2 Omicron variant dynamics in the United States with booster dose vaccination and waning immunity. Mathematical Biosciences and Engineering, 2023, 20(6): 10909-10953. doi: 10.3934/mbe.2023484 |
[7] | A. M. Elaiw, Raghad S. Alsulami, A. D. Hobiny . Global dynamics of IAV/SARS-CoV-2 coinfection model with eclipse phase and antibody immunity. Mathematical Biosciences and Engineering, 2023, 20(2): 3873-3917. doi: 10.3934/mbe.2023182 |
[8] | Tahir Khan, Roman Ullah, Gul Zaman, Jehad Alzabut . A mathematical model for the dynamics of SARS-CoV-2 virus using the Caputo-Fabrizio operator. Mathematical Biosciences and Engineering, 2021, 18(5): 6095-6116. doi: 10.3934/mbe.2021305 |
[9] | A. D. Al Agha, A. M. Elaiw . Global dynamics of SARS-CoV-2/malaria model with antibody immune response. Mathematical Biosciences and Engineering, 2022, 19(8): 8380-8410. doi: 10.3934/mbe.2022390 |
[10] | Wenhan Guo, Yixin Xie, Alan E Lopez-Hernandez, Shengjie Sun, Lin Li . Electrostatic features for nucleocapsid proteins of SARS-CoV and SARS-CoV-2. Mathematical Biosciences and Engineering, 2021, 18(3): 2372-2383. doi: 10.3934/mbe.2021120 |
With continuing emergence of new SARS-CoV-2 variants, understanding the proportion of the population protected against infection is crucial for public health risk assessment and decision-making and so that the general public can take preventive measures. We aimed to estimate the protection against symptomatic illness caused by SARS-CoV-2 Omicron variants BA.4 and BA.5 elicited by vaccination against and natural infection with other SARS-CoV-2 Omicron subvariants. We used a logistic model to define the protection rate against symptomatic infection caused by BA.1 and BA.2 as a function of neutralizing antibody titer values. Applying the quantified relationships to BA.4 and BA.5 using two different methods, the estimated protection rate against BA.4 and BA.5 was 11.3% (95% confidence interval [CI]: 0.01–25.4) (method 1) and 12.9% (95% CI: 8.8–18.0) (method 2) at 6 months after a second dose of BNT162b2 vaccine, 44.3% (95% CI: 20.0–59.3) (method 1) and 47.3% (95% CI: 34.1–60.6) (method 2) at 2 weeks after a third BNT162b2 dose, and 52.3% (95% CI: 25.1–69.2) (method 1) and 54.9% (95% CI: 37.6–71.4) (method 2) during the convalescent phase after infection with BA.1 and BA.2, respectively. Our study indicates that the protection rate against BA.4 and BA.5 are significantly lower compared with those against previous variants and may lead to substantial morbidity, and overall estimates were consistent with empirical reports. Our simple yet practical models enable prompt assessment of public health impacts posed by new SARS-CoV-2 variants using small sample-size neutralization titer data to support public health decisions in urgent situations.
Since the original SARS-CoV-2 strain emerged in Wuhan, China during 2019 [1], the world has experienced a heavy burden owing to morbidity and mortality caused by variants of SARS-CoV-2. The route of SARS-CoV-2 transmission has been of interest since the emergence of this virus. It is now thought that transmission mainly occurs via direct human-to-human contact, although a role of indirect transmission via virus-contaminated surfaces is also reported in published studies [2,3]. In addition to the mode of contact that causes transmission, it is known that host factors, socioeconomic factors, and environmental factors also play key roles, contributing to transmission, and some of these factors may serve as targets of countermeasures against SARS-CoV-2 [4,5,6,7].
Mathematical and statistical models in epidemiology are recognized as powerful tools that can provide the basis for decision-making in public health settings, including optimization of vaccination strategies at both national and global levels [8,9,10,11,12,13,14,15,16,17,18]. However, the validity of models is always a concern, and determining the likely range of parameters used in the models are of crucial importance to improve model validity [19]. A key parameter is the rate of protection conferred by past infection or vaccination, as this strongly affects the host susceptibility to infection with SARS-CoV-2. In the era of COVID-19, updating the immune profile estimates whenever a new variant emerges is especially important because substantial mutations of SARS-CoV-2 that substantially change the characteristics of the virus are frequently introduced. Even before emergence of the Omicron variants, reports showed that neutralization titers and protection provided by vaccination or past natural infection (and their rate of decay) differ among variants. [20,21,22,23,24,25,26] Since emergence of the Omicron strains, immune escape is gaining attention, with reports of significant impairment of neutralization titers and vaccine effectiveness against Omicron subvariants BA.1 and BA.2 in comparison with previous strains, such as the Delta variant. [27,28] Although published studies suggest that booster mRNA vaccines offer extra protection against BA.1 and BA.2 [27,29], no studies to date have explicitly shown the relationship between neutralizing titers and the protection rate against Omicron subvariants BA.1 and BA.2.
At the time of this writing, many countries worldwide, including Japan are facing a rapid increase in the number of COVID-19 cases owing to SARS-CoV-2 Omicron subvariants BA.4 and BA.5. Recent in vitro and in silico studies suggest that BA.4 and BA.5 are even more evasive than BA.1 or BA.2 against humoral immunity induced by vaccines or previous infection with the BA.1 and BA.2 strains [30,31,32,33,34,35]. However, real-world links between neutralization titers and the protection rate against BA.4 and BA.5 variants have not yet been established. One approach to obtaining reasonable estimates of the protection rate against BA.4 and BA.5 with no information on the titer-protection relationship is to assume that this relationship in BA.4 and BA.5 is comparable to that in BA.1 and BA.2. Under this assumption, we estimated the rate of protection against BA.4 and BA.5 using neutralizing titer data from a recent study[30].
An established method to model the relationship between neutralizing titers and vaccine effectiveness is to use a logistic formula, which has been used not only for COVID-19 but also for vaccine effectiveness studies of influenza and pertussis. [24,36,37] Following previous studies, we used the following logistic formula to model the vaccine-induced protection against COVID-19:
Protection rate f(n|n50,k)=11+exp(−k(n−n50)) | (1) |
where n is the neutralizing titer value in decimal logarithm, n50 is the decimal logarithm of the neutralizing titer value providing a 50% protection rate, and k is a parameter determining the slope of the logistic curve. Determining the titer-protection relationship by determining the parameters in Eq (1) using neutralization titer and vaccine effectiveness data for BA.1 and BA.2, we propose a practical framework to estimate the unknown titer-protection relationship for BA.4 and BA.5. In this study, we also aimed to estimate the protection rate against BA.4 and BA.5 over time after immunizing events such as a third BNT162b2 dose or natural infection with BA.1 or BA.2, assuming the decay rate is equivalent to that estimated from a previous vaccine effectiveness study of Omicron subvariants BA.1 and BA.2 [27].
The data used in our study were the neutralization antibody titer data from a study by Hachmann et al. [30] and vaccine effectiveness data in Andrews et al. [27]. In Hachmann et al. [30], specimens obtained from individuals who received a SARS-CoV-2 vaccine or had a history of SARS-CoV-2 infection underwent pseudovirus neutralizing antibody assay; the titer data for this study were provided by Hachmann and colleagues. Andrews et al. [27] used a test-negative case-control design to estimate vaccine effectiveness against symptomatic disease caused by the Omicron and Delta (B.1.617.2) variants in the United Kingdom.
Assuming that the decimal logarithm of neutralization antibody titer log10(titer) provided by Hachmann and colleagues [30] is normally distributed [24] with truncation owing to technical measurement limits, we used a Gaussian distribution to describe the empirical data (see the appendix for a histogram showing the distribution of the original titer data [30]) For simplicity and based on the fact that the immunogenic profiles of BA.1 and BA.2 are quite similar [38], titer values against BA.1 and BA.2 were dealt with as a single group. We grouped titers of BA.4 and BA.5 in the same manner. The distribution of titers at 6 months after a second dose of BNT162b2 vaccine, 2 weeks after a third BNT162b2 dose (nearly all individuals received two or three doses of mRNA vaccine, mainly BNT162b2), and during the convalescent period after BA.1 and 2 infection, are described as follows:
nBA.1,2(t)=log10(titerBA.1,2(t)) N(μBA.1,2(t),σBA.1,2(t)) | (2) |
nBA.4,5(t)=log10(titerBA.4,5(t)) N(μBA.4,5(t),σBA.4,5(t)) | (3) |
where t = 6 months after a second vaccine dose, 2 weeks after a third vaccine dose, and post infection; N(a,b) stands for a normal distribution with mean a and standard deviation b. From the distribution above, 1000 titer values were randomly drawn for the following analysis.
The neutralization titer data from Hachmann et al. [30] modeled as mentioned above were then matched to the vaccine effectiveness data from Andrews et al. [27] using the bootstrap method. Andrews et al. [27] reported the mean and 95% confidence interval (CI) of vaccine effectiveness against symptomatic infection at 2-4 weeks, 5-9 weeks, 10-14 weeks, 15-19 weeks, 20-24 weeks, and > 24 weeks after a second BNT162b2 dose. The vaccine effectiveness following a third (booster) dose of BNT162b2 was provided in the same manner. Assuming that the vaccine effectiveness values are normally distributed in each of these periods [27], we randomly sampled 1000 values of vaccine effectiveness at 20-24 weeks after the second BNT162b2 dose, and vaccine effectiveness at 2-4 weeks after the third BNT162b2 dose from the following distributions:
VE20−24weeks after 2nd dose N(0.115,0.0071) | (4) |
VE2weeks after 3rd doses N(0.672,0.0033) | (5) |
where VE is vaccine effectiveness (the mean and stand deviation in Eqs (4) and (5) were derived from the CI of VE in Andrews et al. [27]). To link the vaccine effectiveness data and titer values, we assumed the abovementioned relationship described in Eq (1).
Estimation of the parameters (n50,k) that determine the logistic formula was performed using two different methods. In the first method, we fitted the logistic formula to 1000 pairs of points,
(n2(i),ve2(i)),(n3(i),ve3(i)),i=1,2,3,…,1000 | (6) |
sampled from distributions described in Eqs (2)-(5) above. For i = 1, 2, …, 1000, Eq (1) was fitted to each pair of Eq (6), minimizing the sum of squared errors S(n50,k) :
S(n50,k)=∑1000i=1(ve2(i)−f(n2(i)|n50,k))2+∑1000i=1(ve3(i)−f(n3(i)|n50,k))2 | (7) |
thereby obtaining a pair of parameters (^n50,ˆk). We repeated this procedure 1000 times to obtain 1000 pairs of (^n50,ˆk).
In the second method, 1000 pairs of points were randomly obtained as shown in Eq (6), but for each ve2(i) and ve3(i), i = 1, 2, 3, …, 1000, we assumed that a random "success" (protected) or "failure" (infected) binomial outcome y exists:
yj(i) Bernoulli(prob=vej(i)) |
j=2 or 3,i=1,2,…,1000 |
Then, by finding (n50,k) that maximizes the likelihood of this simulated observation
L(n50,k)=∏3j=2∏1000i=1f(nj(i)|n50,k)yj(i)×(1−f(nj(i)|n50,k))1−yj(i), |
we obtained (^n50,ˆk). By repeating this procedure 1000 times, 1000 pairs of (^n50,ˆk) were obtained.
Hereafter, we assumed that the relationships described by the logistic curves obtained as above were also valid for quantifying the protection rate against BA.4 and BA.5. To obtain the distribution of protection rates at 6 months after the second BNT162b2 dose, 2 weeks after the third BNT162b2 dose, and soon after natural infection, we randomly sampled 10, 000 titer values from Eq (3). Then, for each titer value, a logistic formula defined by a randomly selected pair of parameters (n50,k) was assigned and the protection rate was obtained using this formula. Thus, we obtained 10, 000 values for the protection rate against BA.4 and BA.5 at 6 months after the second BNT162b2 dose, 2 weeks after the third BNT162b2 dose, and during the convalescent period after BA.1 and BA.2 infection.
Next, to obtain estimates of the protection rate against BA.4 and BA.5 over time, we first fitted the randomly sampled data in the same manner as in Eqs (4) and (5) for all time points post vaccination in Andrews et al. [27] in the exponential decay model of vaccine effectiveness [39,40,41]:
VE=m×e−γt, |
where t is the time after vaccination. We assumed that the post-vaccination time points in Andrews et al. [27] were 24 days (median 2-4 weeks), 52 days (5-9 weeks), 87 days (10-14 weeks), 122 days (15-19 weeks), 157 days (20-24 weeks) and 175 days (>24 weeks) for the second BNT162b2 dose; in the same manner, we assumed the time points post-third BNT162b2 vaccination to be 4 days, 18 days, 46 days, and 81 days. By fitting this model to 1000 randomly generated data sets, we obtained 1000 pairs of parameters (m,γ).
Next, we assumed that the parameter γ, which determines the rate of decay for vaccine effectiveness or the protection rate, was comparable between BA.1 or BA.2 and BA.4 or BA.5. Under this assumption, we obtained the trajectory of the protection rate against BA.4 and BA.5 following two doses of BNT162b2, three doses of BNT162b2, and natural infection caused by BA.1 and BA.2.
All statistical and numerical analyses were performed using R version 4.1.2 (The R Project for Statistical Computing, Vienna, Austria).
Figure 1 shows the original data of neutralizing titer values and the distributions of randomly sampled titer values from the truncated normal distribution fitted to the original data.
Fitting of the logistic formula in Eq (1) was done using the two methods described in the Methods; the logistic curve with 95% CI given by the 1000 sets of parameters (n50,k) is shown in Figure 2.
By matching the 1000 logistic formulas obtained as above from each method to the 10, 000 titer values sampled randomly from Eq (3), we obtained the distribution of protection rates against BA.4 and BA.5 corresponding to titers at 6 months after the second BNT162b2 dose, 2 weeks after the third BNT162b2 dose, and after natural infection with BA.1 and BA.2, as shown in Figure 3.
The protection rate was estimated to be 11.3% (95% CI: 0.01-25.4) (method 1) and 12.9% (95% CI: 8.8-18.0) (method 2) at 6 months after the second BNT162b2 dose, 44.3% (95% CI: 20.0-59.3) (method 1) and 47.3% (95% CI: 34.1-60.6) (method 2) at 2 weeks after the third BNT162b2 dose, and 52.3% (95% CI: 25.1-69.2) (method 1) and 54.9% (95% CI: 37.6-71.4) (method 2) soon after natural infection with BA.1 and BA.2. The estimated exponential decay model of vaccine effectiveness against BA.4 and BA.5 is shown in Figure 4 as a function of time since the latest immunizing event. As expected from the neutralizing titer, the overall strength as well as the duration of protection against BA.4 and BA.5 were lower than those against BA.1 and BA.2. The half-life of protection conferred by the second and third BNT162b2 doses against BA.4 and BA.5 is likely less than 3 months and that conferred by natural infection is comparable with that of a third vaccine dose.
To the best of our knowledge, our study is the first to link neutralization titer and protection rate of BA.1 and BA.2 in a general manner using a logistic formula. We used a logistic formula because it is known to validly capture the relationship between neutralizing antibody titers and vaccine effectiveness against symptomatic infection [24,36,37].
Our study is also the first to provide preliminary estimates of protection rates against BA.4 and BA.5. Assuming that the protection rate-titer relationship and decay speed of the protection rate of BA.4 and BA.5 are comparable to those of BA.1 and BA.2, we showed that the existing effectiveness in preventing symptomatic illness conferred by second and third doses of the BNT162b2 vaccine is fairly low, and the protection conferred by natural infection with BA.1 and BA.2 is comparable to (or slightly better than) that conferred by a third dose of vaccine. Our findings support the existing notion that the BA.4 and BA.5 subvariants have acquired the ability to escape existing immunity and therefore have an advantage that can help these strains to transmit more easily among the present-day population. [30,31,32,38,42,43]
Another important aspect of our study is that our framework enables preliminary estimation of protection rates against novel variants in the future, even during the early phase of an epidemic. In particular, estimates can be attained even using only small sample-size data of neutralization antibody titers. Together with insights on preparedness against future pandemics[9,17,18], even a rough estimate of protection against novel variants may lead to better decision-making regarding public health by policymakers.
The key limitations of our study were the small sample size of neutralizing titer data and lack of epidemiological data on the protection rate against BA.4 and BA.5. The neutralizing titer data used in this study shows consistency with recent in vivo reports on immunity against BA.1 and BA.2 induced by breakthrough infection among vaccinated individuals [44]; however, some uncertainty may exist considering that no other neutralizing titer data against BA.4 and BA.5 were available at the time of this writing. To overcome the lack of data on the protection rate against BA 4 and BA.5, we used two different models to connect the neutralizing titer data with the protection rate for further simulation. The consistency between the results using each method is encouraging, but careful validation in epidemiological studies with sufficient data and an adequate design is warranted. Several preprint studies providing epidemiological data on protection rates against BA.4 and BA.5 have become available during the writing of this article. Among these, regarding the protection rate provided by previous BA.1 or BA.2 infection in patients who received three doses of vaccine, our results seem to be quite consistent with estimates reported in a recent preprint study from Qatar with a test-negative design. [45] The findings of other recent studies from Portugal [46] and Denmark [47] were not comparable to our results; the former study did not have test-negative controls, and the latter study pooled those with prior BA.2 and BA.5 infection in the same exposed group in the analysis of protection rate provided by previous infections. Because the BA.4 and BA.5 variants are somewhat new, further studies on these variants are needed to confirm our results. The simulation of protection decay over time also needs validation; owing to waning protection against Omicron BA.4 and BA.5 subvariants, we assumed an exponential decay model with a decay rate that is comparable to that after a third BNT162b2 dose.
Another limitation of our study is that we only considered data that show the extent of humoral immunity. Because both humoral and cellular immunity provide protection against SARS-CoV-2 [48,49], only considering the humoral effect as in our study may result in underestimation of the actual protection rate, to some extent.
Despite the abovementioned limitations and the need for further validation, the predicted protection rate distributions against BA.4 and BA.5 are useful as indicators to inform public health policy. The prediction framework proposed in this study is important because it is a simple method of calculating protection rate estimates, and the prediction can be easily improved using data from future studies. In the future, how to improve protection against severe illness and death owing to COVID-19 must be explored. We believe these cannot be sufficiently inferred using neutralization titer data alone.
Our findings indicated that accelerating the availability of a fourth dose of the BNT162b2 vaccine is essential to avoid preventable clusters among vulnerable populations (e.g., workers in health care and welfare facilities).
It is vital to remind the working-age population that a previous "fully" vaccinated status is not sufficient to provide protection against symptomatic illness owing to infection with BA.4 or BA.5.
In the present study, we estimated the neutralizing titer-protection rate relationship of the BA.1 and BA.2 subvariants by applying a logistic formula to the limited data using two methods. We then extended this framework to predict the protection rate against BA.4 and BA.5 in several situations as well as over time. The estimates in our study showed that a greater impact owing to morbidity and mortality from BA.4 and BA.5 should be expected, in comparison with BA.1 and BA.2,
In reality, the decay rate of BA.4 and BA.5 may be greater than the rate assumed in the present study. Thus, to avert health risks as much as possible, further promotion of vaccination is deemed essential. Our models also show that, even with a small sample of neutralization titer data, we can infer the protection rate against future variants with simple yet robust methods. This means that the application of models like ours during the early phase of an outbreak caused by a novel SARS-CoV-2 variant is crucial for prompt decision-making in terms of policies regarding vaccination strategies and nonpharmaceutical interventions at both the community and national levels.
We thank Professor Dan Barouch and his colleagues for kindly providing the neutralizing antibody titer data published in N Engl J Med 2022; 387:86-88. T. K. received funding from the JSPS KAKENHI (21K10495). A. A. received funding from JSPS KAKENHI (22J14304). H. N. received funding from Health and Labour Sciences Research Grants (20CA2024, 20HA2007, 21HB1002, and 21HA2016), the Japan Agency for Medical Research and Development (JP20fk0108140, JP20fk0108535, and JP21fk0108612), the JSPS KAKENHI (21H03198 and 22K19670), Environment Research and Technology Development Fund (JPMEERF20S11804) of the Environmental Restoration and Conservation Agency of Japan, and the Japan Science and Technology Agency SICORP program (JPMJSC20U3 and JPMJSC2105) and RISTEX program for Science of Science, Technology and Innovation Policy (JPMJRS22B4). The funders had no role in the study design, data collection and analysis, decision to publish, or preparation of the manuscript. We also thank Edanz (https://jp.edanz.com/ac) for editing a draft of this manuscript.
All authors declare no conflicts of interest related to this paper.
Original neutralizing titer data from Hachmann et al. [1].
1. N.P. Hachmann, J. Miller, A. Y. Collier, J. D. Ventura, J. Yu, M. Rowe, et al., Neutralization Escape by SARS-CoV-2 Omicron Subvariants BA.2.12.1, BA.4, and BA.5, New Engl. J. Med., (2022).
[1] |
N. Zhu, D. Zhang, W. Wang, X. Li, B. Yang, J. Song, et al., A novel coronavirus from patients with pneumonia in China, 2019, New Engl. J. Med., 382 (2020), 727–733. https://doi.org/10.1056/NEJMoa2001017 doi: 10.1056/NEJMoa2001017
![]() |
[2] |
E. A. Meyerowitz, A. Richterman, R. T. Gandhi, P. E. Sax, Transmission of SARS-CoV-2: A review of viral, host, and environmental factors, 174 (2021), 69–79. https://doi.org/10.7326/M20-5008 doi: 10.7326/M20-5008
![]() |
[3] |
A. Núñez-Delgado, E. Bontempi, M. Coccia, M. Kumar, K. Farkas, J. L. Domingo, SARS-CoV-2 and other pathogenic microorganisms in the environment, Environ. Res., 201 (2021), 111606. https://doi.org/10.1016/j.envres.2021.111606 doi: 10.1016/j.envres.2021.111606
![]() |
[4] |
M. Cevik, J. L. Marcus, C. Buckee, T. C.Smith, Severe acute respiratory syndrome coronavirus 2 (SARS-CoV-2) transmission dynamics should inform policy, Clin. Infect. Dis., 73 (2021), S170–S176. https://doi.org/10.1093/cid/ciaa1442 doi: 10.1093/cid/ciaa1442
![]() |
[5] |
D. Nash, M. S. Rane, M. M. Robertson, M. Chang, S. G. Kulkarni, R. Zimba, et al., SARS-CoV-2 incidence and risk factors in a national, community-based prospective cohort of U.S. adults, Clin. Infect. Dis., (2022). https://doi.org/10.1101/2021.02.12.21251659 doi: 10.1101/2021.02.12.21251659
![]() |
[6] |
M. Coccia, Factors determining the diffusion of COVID-19 and suggested strategy to prevent future accelerated viral infectivity similar to COVID, Sci. Total. Environ., 729 (2020), 138474. https://doi.org/10.1016/j.scitotenv.2020.138474 doi: 10.1016/j.scitotenv.2020.138474
![]() |
[7] |
M. Coccia, COVID-19 pandemic over 2020 (with lockdowns) and 2021 (with vaccinations): similar effects for seasonality and environmental factors, Environ. Res., 208 (2022), 112711. https://doi.org/10.1016/j.envres.2022.112711 doi: 10.1016/j.envres.2022.112711
![]() |
[8] |
M. Coccia, Optimal levels of vaccination to reduce COVID-19 infected individuals and deaths: A global analysis, Environ. Res., 204 (2022). https://doi.org/10.1016/j.envres.2021.112314 doi: 10.1016/j.envres.2021.112314
![]() |
[9] |
M. Coccia, Preparedness of countries to face COVID-19 pandemic crisis: Strategic positioning and factors supporting effective strategies of prevention of pandemic threats, Environ. Res., 203 (2022), 111678. https://doi.org/10.1016/j.envres.2021.111678 doi: 10.1016/j.envres.2021.111678
![]() |
[10] |
I. Benati, M. Coccia, Global analysis of timely COVID-19 vaccinations: Improving governance to reinforce response policies for pandemic crises, Int. J. Health Gov., 27 (2022), 240–253. https://doi.org/10.1108/IJHG-07-2021-0072 doi: 10.1108/IJHG-07-2021-0072
![]() |
[11] |
L. Matrajt, J. Eaton, T. Leung, E. R. Brown, Vaccine optimization for COVID-19: Who to vaccinate first?, Sci. Adv., 7 (2021). https://doi.org/10.1126/sciadv.abf1374 doi: 10.1126/sciadv.abf1374
![]() |
[12] |
L. Thul, W. Powell, Stochastic optimization for vaccine and testing kit allocation for the COVID-19 pandemic, Eur. J. Oper. Res., 304 (2023), 325–338. https://doi.org/10.1016/j.ejor.2021.11.007 doi: 10.1016/j.ejor.2021.11.007
![]() |
[13] |
W. Cao, J. Zhu, X. Wang, X. Tong, Y. Tian, H. Dai, et al., Optimizing spatio-temporal allocation of the COVID-19 vaccine under different epidemiological landscapes, Front. Public Health, 10 (2022), 1828. https://doi.org/10.3389/fpubh.2022.921855 doi: 10.3389/fpubh.2022.921855
![]() |
[14] |
M. Bicher, C. Rippinger, M. Zechmeister, B. Jahn, G. Sroczynski, N. Mühlberger, et al., An iterative algorithm for optimizing COVID-19 vaccination strategies considering unknown supply, PLoS One, 17 (2022), e0265957. https://doi.org/10.1371/journal.pone.0265957 doi: 10.1371/journal.pone.0265957
![]() |
[15] |
L. Matrajt, J. Eaton, T. Leung, D. Dimitrov, J. T. Schiffer, D. A. Swan, et al., Optimizing vaccine allocation for COVID-19 vaccines shows the potential role of single-dose vaccination, Nat. Commun., 12 (2021), 1–18. https://doi.org/10.1038/s41467-021-23761-1 doi: 10.1038/s41467-021-23761-1
![]() |
[16] | M. Coccia, Comparative critical decisions in management, in Global Encyclopedia of Public Administration, Public Policy, and Governance, (2020), 1–10. https://doi.org/10.1007/978-3-319-31816-5_3969-1 |
[17] |
M. Coccia, Improving preparedness for next pandemics: Max level of COVID-19 vaccinations without social impositions to design effective health policy and avoid flawed democracies, Environ. Res., 213 (2022), 113566. https://doi.org/10.1016/j.envres.2022.113566 doi: 10.1016/j.envres.2022.113566
![]() |
[18] |
M. Coccia, Pandemic prevention: Lessons from COVID-19, Encyclopedia, 1 (2021), 433–444. https://doi.org/10.3390/encyclopedia1020036 doi: 10.3390/encyclopedia1020036
![]() |
[19] |
R. P. Kumar, P. K. Santra, G. S. Mahapatra, Global stability and analysing the sensitivity of parameters of a multiple-susceptible population model of SARS-CoV-2 emphasising vaccination drive, Math. Comput. Simul., 203 (2023), 741–766. https://doi.org/10.1016/j.matcom.2022.07.012 doi: 10.1016/j.matcom.2022.07.012
![]() |
[20] |
Z. Wang, F. Schmidt, Y. Weisblum, F. Muecksch, C. O. Barnes, S. Finkin, et al., mRNA vaccine-elicited antibodies to SARS-CoV-2 and circulating variants, Nature, 592 (2021), 616–622. https://doi.org/10.1038/s41586-021-03324-6 doi: 10.1038/s41586-021-03324-6
![]() |
[21] |
Y. Liu, J. Liu, H. Xia, X. Zhang, C. R. Fontes-Garfias, K. A. Swanson, et al., Neutralizing activity of BNT162b2-Elicited serum, N. Engl. J. Med., 384 (2021), 1466–1468. https://doi.org/10.1056/NEJMc2102017 doi: 10.1056/NEJMc2102017
![]() |
[22] |
R. E. Chen, X. Zhang, J. B. Case, E. S. Winkler, Y. Liu, L. A. VanBlargan, et al., Resistance of SARS-CoV-2 variants to neutralization by monoclonal and serum-derived polyclonal antibodies, Nat. Med., 27 (2021), 717–726.https://doi.org/10.1038/s41591-021-01294-w doi: 10.1038/s41591-021-01294-w
![]() |
[23] |
P. Wang, M. S. Nair, L. Liu, S. Iketani, Y. Luo, Y. Guo, et al., Antibody resistance of SARS-CoV-2 variants B.1.351 and B.1.1.7, Nature, 593 (2021), 130–135. https://doi.org/10.1038/s41586-021-03398-2 doi: 10.1038/s41586-021-03398-2
![]() |
[24] |
D. S. Khoury, D. Cromer, A. Reynaldi, T. E. Schlub, A. K. Wheatley, J. A. Juno, et al., Neutralizing antibody levels are highly predictive of immune protection from symptomatic SARS-CoV-2 infection, Nat. Med., 27 (2021), 1205–1211. https://doi.org/10.1038/s41591-021-01377-8 doi: 10.1038/s41591-021-01377-8
![]() |
[25] |
L. Wang, M. H. Kainulainen, N. Jiang, H. Di, G. Bonenfant, L. Mills, et al., Differential neutralization and inhibition of SARS-CoV-2 variants by antibodies elicited by COVID-19 mRNA vaccines, Nat. Commun., 13 (2022), 1–10. https://doi.org/10.1038/s41467-022-31929-6 doi: 10.1038/s41467-022-31929-6
![]() |
[26] |
S. P. Andeweg, B. de Gier, D. Eggink, C. van den Ende, N. van Maarseveen, L. Ali, et al., Protection of COVID-19 vaccination and previous infection against Omicron BA.1, BA.2 and Delta SARS-CoV-2 infections, Nat. Commun., 13 (2022), 1–9. https://doi.org/10.1038/s41467-022-31838-8 doi: 10.1038/s41467-022-31838-8
![]() |
[27] |
N. Andrews, J. Stowe, F. Kirsebom, S. Toffa, T. Rickeard, E. Gallagher, et al., Covid-19 Vaccine effectiveness against the omicron (B.1.1.529) variant, N. Engl. J. Med., 386 (2022), 1532–1546. https://doi.org/10.1056/NEJMoa2119451 doi: 10.1056/NEJMoa2119451
![]() |
[28] |
G. Regev-Yochay, T. Gonen, M. Gilboa, M. Mandelboim, V. Indenbaum, S. Amit, et al., Efficacy of a fourth dose of Covid-19 mRNA vaccine against Omicron, N. Engl. J. Med., 386 (2022), 1377–1380. https://doi.org/10.1056/NEJMc2202542 doi: 10.1056/NEJMc2202542
![]() |
[29] |
E. Nanishi, M. E. McGrath, T. R. O'Meara, S. Barman, J. Yu, H. Wan, et al., mRNA booster vaccination protects aged mice against the SARS-CoV-2 Omicron variant, Commun. Biol., 5 (2022), 1–8. https://doi.org/10.1038/s42003-022-03765-3 doi: 10.1038/s42003-022-03765-3
![]() |
[30] |
N. P. Hachmann, J. Miller, A. Y. Collier, J. D. Ventura, J. Yu, M. Rowe, et al., Neutralization Escape by SARS-CoV-2 Omicron Subvariants BA.2.12.1, BA.4, and BA.5, N. Engl. J. Med., 387 (2022). https://doi.org/10.1101/2022.05.16.22275151 doi: 10.1101/2022.05.16.22275151
![]() |
[31] |
Y. Cao, A. Yisimayi, F. Jian, W. Song, T. Xiao, L. Wang, et al., BA.2.12.1, BA.4 and BA.5 escape antibodies elicited by Omicron infection, Nature, 608 (2022), 1–3. https://doi.org/10.1038/s41586-022-04980-y doi: 10.1038/s41586-022-04980-y
![]() |
[32] |
Q. Wang, Y. Guo, S. Iketani, M. S. Nair, Z. Li, H. Mohri, et al., Antibody evasion by SARS-CoV-2 Omicron subvariants BA.2.12.1, BA.4, & BA.5, Nature, 608 (2022), 1–3. https://doi.org/10.1038/s41586-022-05053-w doi: 10.1038/s41586-022-05053-w
![]() |
[33] |
M. Huang, L. Wu, A. Zheng, Y. Xie, Q. He, X. Rong, et al., Atlas of currently-available human neutralizing antibodies against SARS-CoV-2 and escape by Omicron sub-variants BA.1/BA.1.1/BA.2/BA.3, Immunity, 55 (2022). https://doi.org/10.1016/j.immuni.2022.06.005 doi: 10.1016/j.immuni.2022.06.005
![]() |
[34] |
K. Khan, F. Karim, Y. Ganga, M. Bernstein, Z. Jule, K. Reedoy, et al., Omicron BA.4/BA.5 escape neutralizing immunity elicited by BA.1 infection, Nat. Commun., 13 (2022), 1–7. https://doi.org/10.1038/s41467-022-32396-9 doi: 10.1038/s41467-022-32396-9
![]() |
[35] |
L. Bellusci, G. Grubbs, F. T. Zahra, D. Forgacs, H. Golding, T. M. Ross, et al., Antibody affinity and cross-variant neutralization of SARS-CoV-2 Omicron BA.1, BA.2 and BA.3 following third mRNA vaccination, Nat. Commun., 13 (2022), 1–9. https://doi.org/10.1038/s41467-022-32298-w doi: 10.1038/s41467-022-32298-w
![]() |
[36] |
L. Qin, P. B. Gilbert, L. Corey, M. J. McElrath, S. G. Self, A framework for assessing immunological correlates of protection in vaccine trials, J. Infect. Dis., 196 (2007), 1304–1312. https://doi.org/10.1086/522428 doi: 10.1086/522428
![]() |
[37] |
J. Storsaeter, H. O. Hallander, L. Gustafsson, P. Olin, Levels of anti-pertussis antibodies related to protection after household exposure to Bordetella pertussis, Vaccine, 16 (1998), 1907–1916. https://doi.org/10.1016/S0264-410X(98)00227-8 doi: 10.1016/S0264-410X(98)00227-8
![]() |
[38] |
Y J. Yu, A. Y. Collier, M. Rowe, F. Mardas, J. D. Ventura, H. Wan, et al., Neutralization of the SARS-CoV-2 Omicron BA.1 and BA.2 Variants, N. Engl. J. Med., 386 (2022), 1579–1580. https://doi.org/10.1056/NEJMc2201849 doi: 10.1056/NEJMc2201849
![]() |
[39] |
S. de Montigny, B. J. S. Adamson, B. R. Mâsse, L. P. Garrison, J. G. Kublin, P. B. Gilbert, et al., Projected effectiveness and added value of HIV vaccination campaigns in South Africa: A modeling study, Sci. Rep., 8 (2018), 1–12. https://doi.org/10.1038/s41598-018-24268-4 doi: 10.1038/s41598-018-24268-4
![]() |
[40] |
K. M. Andersson, J. Stover, The potential impact of a moderately effective HIV vaccine with rapidly waning protection in South Africa and Thailand, Vaccine, 29 (2011), 6092–6099. https://doi.org/10.1016/j.vaccine.2011.06.060 doi: 10.1016/j.vaccine.2011.06.060
![]() |
[41] |
J. A. C. Hontelez, N. Nagelkerke, T. Bärnighausen, R. Bakker, F. Tanser, M. L. Newell, et al., The potential impact of RV144-like vaccines in rural South Africa: A study using the STDSIM microsimulation model, Vaccine, 29 (2011), 6100–6106. https://doi.org/10.1016/j.vaccine.2011.06.059 doi: 10.1016/j.vaccine.2011.06.059
![]() |
[42] |
L. B. Shrestha, C. Foster, W. Rawlinson, N. Tedla, R. A. Bull, C. A. R. Bull, Evolution of the SARS-CoV-2 omicron variants BA.1 to BA.5: Implications for immune escape and transmission, Rev. Med. Virol., 32 (2022), e2381. https://doi.org/10.1002/rmv.2381 doi: 10.1002/rmv.2381
![]() |
[43] |
A. Tuekprakhon, J. Huo, R. Nutalai, A. Dijokaite-Guraliuc, D. Zhou, H. M. Ginn, et al., Antibody escape of SARS-CoV-2 Omicron BA.4 and BA.5 from vaccine and BA.1 serum, Cell, 185 (2022), 2422–2433. https://doi.org/10.1016/j.cell.2022.06.005 doi: 10.1016/j.cell.2022.06.005
![]() |
[44] | H. Kared, A. S. Wolf, A. Alirezaylavasani, A. Ravussin, G. Solum, T. T. Tran, et al., Immune responses in Omicron SARS-CoV-2 breakthrough infection in vaccinated adults, Nat. Commun., 13 (2022), 1–12. |
[45] |
H. N. Altarawneh, H. Chemaitelly, H. H. Ayoub, M. R. Hasan, P. Coyle, H. M. Yassine, et al., Protection of SARS-CoV-2 natural infection against reinfection with the Omicron BA.4 or BA.5 subvariants, MedRxiv, (2022). https://doi.org/10.1101/2022.07.11.22277448 doi: 10.1101/2022.07.11.22277448
![]() |
[46] |
J. Malato, R. M. Ribeiro, P. P. Leite, P. Casaca, E. Fernandes, C. Antunes, et al., Risk of BA.5 infection in individuals exposed to prior SARS-CoV-2 variants, MedRxiv, (2022). https://doi.org/10.1101/2022.07.27.22277602 doi: 10.1101/2022.07.27.22277602
![]() |
[47] |
C. H. Hansen, N. U. Friis, P. Bager, M. Stegger, J. Fonager, A. Fomsgaard, et al., Risk of reinfection, vaccine protection, and severity of infection with the BA.5 Omicron subvariant: A danish nation-wide population-based study, SSRN Electron. J., (2022). https://doi.org/10.2139/ssrn.4165630 doi: 10.2139/ssrn.4165630
![]() |
[48] |
J. T. Castro, P. Azevedo, M. J. Fumagalli, N. S. Hojo-Souza, N. Salazar, G. G. Almeida, et al., Promotion of neutralizing antibody-independent immunity to wild-type and SARS-CoV-2 variants of concern using an RBD-Nucleocapsid fusion protein, Nat. Commun., 13 (2022), 1–16. https://doi.org/10.1038/s41467-022-32547-y doi: 10.1038/s41467-022-32547-y
![]() |
[49] |
M. Reinscheid, H. Luxenburger, V. Karl, A. Graeser, S. Giese, K. Ciminski, et al., COVID-19 mRNA booster vaccine induces transient CD8+ T effector cell responses while conserving the memory pool for subsequent reactivation, Nat. Commun., 13 (2022), 1–11. https://doi.org/10.1038/s41467-022-32324-x doi: 10.1038/s41467-022-32324-x
![]() |
1. | Cameron Zachreson, Ruarai Tobin, Joshua Szanyi, Camelia Walker, Deborah Cromer, Freya M Shearer, Eamon Conway, Gerard Ryan, Allen Cheng, James M McCaw, Nicholas Geard, Individual variation in vaccine immune response can produce bimodal distributions of protection, 2023, 41, 0264410X, 6630, 10.1016/j.vaccine.2023.09.025 | |
2. | Jiaying Qiao, Hiroshi Nishiura, Public holidays increased the transmission of COVID-19 in Japan, 2020–2021: a mathematical modelling study , 2024, 2092-7193, e2024025, 10.4178/epih.e2024025 | |
3. | Taishi Kayano, Misaki Sasanami, Hiroshi Nishiura, Science-based exit from stringent countermeasures against COVID-19: Mortality prediction using immune landscape between 2021 and 2022 in Japan, 2024, 20, 25901362, 100547, 10.1016/j.jvacx.2024.100547 |