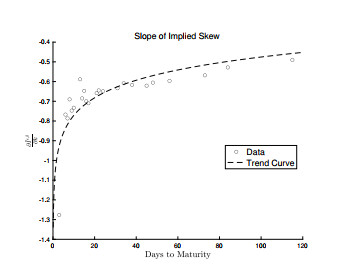
Qualitative and DNA analysis can be performed by taking a multidisciplinary approach to evaluate apple juices, the relevant values of which are a function of the origin, processing method and cultivar used. In detail, the aims of this study were to characterize apple juices through physiochemical analysis, sensory analysis and DNA analysis to evaluate the efficiency of simple sequence repeat (SSR) markers for cultivar identification. Six apple juices made with cv Golden Delicious, cv Granny Smith and a mix of these cultivars from an e-commerce platform (Samples A and B), DISAFA (Samples C and D) and a local farm (Piedmont, Italy) (Samples E and F) were considered. Apple juices A, B, E and F (clarified and pasteurized) can be considered as being of high quality, while Samples C and D were unclarified, unpasteurized and made with apples purchased from a local store. Considering the qualitative analysis, it was observed that the cultivar of apple affected the parameters assessed. In the case of total phenolic compounds, the highest values were observed for juices made only with cv Granny Smith, suggesting how this cultivar contributes to maintaining these nutraceutical compounds more than cv Golden Delicious. Regarding DNA analysis, a limited number of markers, i.e., 4 and 3, respectively, for the apple juices originating from e-commerce and a local farm could successfully produce reproducible amplified fragments. These results can be related to the different procedures used in processing apple juices of different origins.
Citation: Daniela Torello Marinoni, Paola Ruffa, Vera Pavese, Nicole Roberta Giuggioli. Apple juice evaluation: Qualitative analysis and microsatellite traceability[J]. AIMS Agriculture and Food, 2022, 7(4): 819-830. doi: 10.3934/agrfood.2022050
[1] | Yan Xie, Rui Zhu, Xiaolong Tan, Yuan Chai . Inhibition of absence seizures in a reduced corticothalamic circuit via closed-loop control. Electronic Research Archive, 2023, 31(5): 2651-2666. doi: 10.3934/era.2023134 |
[2] | Ariel Leslie, Jianzhong Su . Modeling and simulation of a network of neurons regarding Glucose Transporter Deficiency induced epileptic seizures. Electronic Research Archive, 2022, 30(5): 1813-1835. doi: 10.3934/era.2022092 |
[3] | Zhihui Wang, Yanying Yang, Lixia Duan . Dynamic mechanism of epileptic seizures induced by excitatory pyramidal neuronal population. Electronic Research Archive, 2023, 31(8): 4427-4442. doi: 10.3934/era.2023226 |
[4] | Xiaolong Tan, Hudong Zhang, Yan Xie, Yuan Chai . Electromagnetic radiation and electrical stimulation controls of absence seizures in a coupled reduced corticothalamic model. Electronic Research Archive, 2023, 31(1): 58-74. doi: 10.3934/era.2023004 |
[5] | Saeedreza Tofighi, Farshad Merrikh-Bayat, Farhad Bayat . Designing and tuning MIMO feedforward controllers using iterated LMI restriction. Electronic Research Archive, 2022, 30(7): 2465-2486. doi: 10.3934/era.2022126 |
[6] | Yang Song, Beiyan Yang, Jimin Wang . Stability analysis and security control of nonlinear singular semi-Markov jump systems. Electronic Research Archive, 2025, 33(1): 1-25. doi: 10.3934/era.2025001 |
[7] | Jichen Hu, Ming Zhu, Tian Chen . The nonlinear observer-based fault diagnosis method for the high altitude airship. Electronic Research Archive, 2025, 33(2): 907-930. doi: 10.3934/era.2025041 |
[8] | Ramalingam Sakthivel, Palanisamy Selvaraj, Oh-Min Kwon, Seong-Gon Choi, Rathinasamy Sakthivel . Robust memory control design for semi-Markovian jump systems with cyber attacks. Electronic Research Archive, 2023, 31(12): 7496-7510. doi: 10.3934/era.2023378 |
[9] | Yu-Jing Shi, Yan Ma . Finite/fixed-time synchronization for complex networks via quantized adaptive control. Electronic Research Archive, 2021, 29(2): 2047-2061. doi: 10.3934/era.2020104 |
[10] | Huan Luo . Heterogeneous anti-synchronization of stochastic complex dynamical networks involving uncertain dynamics: an approach of the space-time discretizations. Electronic Research Archive, 2025, 33(2): 613-641. doi: 10.3934/era.2025029 |
Qualitative and DNA analysis can be performed by taking a multidisciplinary approach to evaluate apple juices, the relevant values of which are a function of the origin, processing method and cultivar used. In detail, the aims of this study were to characterize apple juices through physiochemical analysis, sensory analysis and DNA analysis to evaluate the efficiency of simple sequence repeat (SSR) markers for cultivar identification. Six apple juices made with cv Golden Delicious, cv Granny Smith and a mix of these cultivars from an e-commerce platform (Samples A and B), DISAFA (Samples C and D) and a local farm (Piedmont, Italy) (Samples E and F) were considered. Apple juices A, B, E and F (clarified and pasteurized) can be considered as being of high quality, while Samples C and D were unclarified, unpasteurized and made with apples purchased from a local store. Considering the qualitative analysis, it was observed that the cultivar of apple affected the parameters assessed. In the case of total phenolic compounds, the highest values were observed for juices made only with cv Granny Smith, suggesting how this cultivar contributes to maintaining these nutraceutical compounds more than cv Golden Delicious. Regarding DNA analysis, a limited number of markers, i.e., 4 and 3, respectively, for the apple juices originating from e-commerce and a local farm could successfully produce reproducible amplified fragments. These results can be related to the different procedures used in processing apple juices of different origins.
Stochastic volatility models driven by fractional Brownian motion have been gaining attention in the area of mathematical finance, since many empirical studies find that the decay in the autocorrelation function of the volatility is better modeled by a power function than an exponential function and fractional stochastic volatility models generate better fits to the observed market implied volatility surface. Refer to, for example, Comte and Renault [9], Alˊos et al. [2], Bayer et al. [4], Garnier and Solna [17], Forde and Zhang [12], Gatheral et al. [19], Guennoun et al. [20], Kim et al. [21], Bennedsen et al. [5], Fukasawa and Gatheral [16], Shi and Yu [30], and Cont and Das [10], among others. While Comte and Renault [9] stressed that fractional Brownian motion was relevant to capture long memory in stochastic volatility, Gatheral et al. [19] showed that log-volatility behaves essentially as a fractional Brownian motion with a Hurst exponent of order 0.1, at any reasonable time scale. A recent paper by Wang et al. [33] also found that the logarithmic daily realized volatility series of various financial assets have rough sample paths. However, fractional Brownian motion is not a semimartingale unless the Hurst exponent is 1/2, as shown in Rogers [28], leading to a possible arbitrage opportunity (a free lunch with vanishing risk, as a general term). "Arbitrage" means profiting from a price gap between a derivative and a portfolio of assets that replicates the derivative's cash flows. So, to get around this problem while taking into account the long or short memory property, there have been studies using the mixed fractional Brownian motion of Cheridito [8], the approximate fractional Brownian motion of Thao [32], or the generalized fractional Brownian motion introduced by Pang and Taqqu [24].
We are interested in the approximate fractional Brownian motion introduced in Thao [32] in the context of modeling stochastic volatility for pricing derivatives. This process is a semimartingale even if the Hurst exponent is not 1/2 (contrary to the original fractional Brownian motion) and so the no-arbitrage theory can be applied to obtain a partial differential equation (PDE) for the option price. There are several works which have used this process for underlying asset price models or stochastic volatility models. Refer to, for instance, Dung [11] for the Black-Scholes model, Sattayatham and Intarasit [29] for a jump-diffusion model, Pospisil and Sobotka [26] for the Heston stochastic volatility model, and Chang et al. [7] for the double Heston stochastic volatility model. In the present paper, we apply approximate fractional Brownian motions to the multiscale stochastic volatility model of Fouque et al. [14]. The merit of this stochastic volatility model is that the resulting option price approximation is independent of the particular details of the volatility model and leads to more flexibility in the parametrization of the implied volatility surface. We take two approximate fractional Brownian motions with two Hurst exponents (instead of two standard Brownian motions) corresponding to two characteristic (fast and slow) time scales of the multiscale volatility model, respectively. As far as we know, this approach does not exist in the literature. However, this type of volatility formulation is consistent with some of the previous works related to fractional Brownian motion. According to Xiao and Yu [35,36], the asymptotic distribution for the estimator of the persistence parameter is different when the Hurst exponent is less than 1/2 from that when it is larger than 1/2 in the fractional Vasicek model. Alˊos and Leon [3] found, based on the Clark-Ocone-Haussman formula for the integrated variance, that the volatility can be composed of terms with a Hurst index less than 1/2 being more relevant at short scales and terms with Hurst index greater than 1/2 being more relevant at long scales. Also, Bennedsen et al. [5] discovered evidence consistent with the hypothesis that time series of realized volatility are both rough and very persistent. On the other hand, Cont and Das [10] observed interestingly that even when the instantaneous volatility has the same roughness as Brownian motion, the realized volatility exhibits behavior corresponding to a Hurst exponent significantly smaller than 1/2. This observation supports our use of approximate fractional Brownian motion instead of fractional Brownian motion. The approximate fractional Brownian motion is thought of as between the standard Brownian motion and the fractional Brownian motion. It is a stochastic process equipped with a Hurst parameter, i.e., a measure of long-term memory of the time series. It is, however, a semimartingale in the form of a Brownian motion plus a time (Riemann) integral of an adapted process. So, the arbitrage opportunity can be excluded from the fundamental theorem of asset pricing and we are allowed to use the replicating portfolio method to obtain the corresponding PDEs for European vanilla and exotic options. The contribution of this work is as follows. We obtain approximate closed-form formulas for the prices of European vanilla and two exotic options. Our results cover both long- and short-memory properties of volatilities and control the skew slope by selecting appropriate Hurst exponents of the fast and slow scale volatility movements. It unifies two previously known results regarding the Hurst parameter dependence of the blow-up and slow-flattening behavior of skews and smiles of implied volatility surfaces. Consequently, the implied volatility surfaces can be calibrated over a wide range of time-to-maturities. Also, we provide a calibration method by representing the observed SPX option prices in terms of the term structure of the implied volatility formula. Based on the calibration result, we find that the implied volatility becomes higher when the fast-scale motion of the (spot) volatility becomes "rougher" and the slow-scale motion of the volatility becomes "smoother", which in turn supports the necessity of a multiscale modeling framework for stochastic volatility.
The paper is organized as follows. In Section 2, we use approximate fractional Brownian motions to establish a stochastic volatility model. In Section 3, we apply the replicating portfolio method to obtain the corresponding PDE formula for the price of a European vanilla option and derive explicitly a closed-form formula for the approximate option price using the combination of singular and regular perturbations and the Mellin transform method. Subsequently, a closed-form formula for the implied volatility corresponding to a European call option is obtained in Section 4. We check the accuracy of the pricing formula, show how to calibrate the pricing parameters, and investigate the sensitivity of the implied volatilities to the Hurst exponents in Section 5. We extend the vanilla option price formula to two exotic-option cases in Section 6. Finally, Section 7 provides some concluding remarks.
A fractional Brownian motion BHt with a Hurst exponent H, 0<H<1, is defined by a centered Gaussian process satisfying the covariance function
E[BHtBHs]=12(|s|2H+|t|2H−|s−t|2H). |
The process BHt is a self-similar process but is neither a semimartingale nor a Markov process except in the case where H=1/2. Mandelbrot and van Ness [22] gave an integral representation of the general fractional Brownian motion as follows:
BHt=1Γ(H+12)[∫0−∞[(t−s)H−12−(−s)H−12]dWs+∫t0(t−s)H−12dWs],t>0, | (2.1) |
where Wt is a standard Brownian motion. The last integral part of (2.1) is a self-similar Gaussian process, which becomes a Brownian motion for H=1/2 and has non-stationary increments for H≠1/2. It is a truncated version of the general fractional Brownian motion and is usually called Riemann-Liouville fractional Brownian motion with a Hurst index H. This type of fractional Brownian motion has been widely used in the modeling of volatilities. See, for example, Comte and Renault [9], Alˊos et al. [2], Bayer et al. [4], and Gatheral et al. [19], among others. It has a simple representation but it is not a semimartingale for H≠1/2. Thus Thao [32] used a perturbation parameter, say γ, to introduce an approximate fractional Brownian motion defined as
Bγ,Ht:=∫t0(t−s+γ)H−12dWs,γ>0, |
where Wt is a standard Brownian motion, and proved that Bγ,Ht is a semimartingale and converges to the last integral of (2.1) in L2(Ω) sense and uniformly with respect to t∈[0,T] for any fixed positive number T when γ goes to 0.
In this paper, we use the process Bγ,Ht as a random source of the volatility of the underlying risky asset return to introduce a new model given by
dXt=f(Yt,Zt)XtdWxt,dYt=1ϵα(Yt)dt+1√ϵβ(Yt)dBγ,H1t,dZt=δg(Zt)dt+√δh(Zt)dBγ,H2t | (2.2) |
under a risk-neutral probability measure Q. The model (2.2) is the same as the multiscale stochastic volatility model in Fouque et al. [14] except that the standard Brownian motions driving the two stochastic volatility factors Yt and Zt are now replaced by the approximate fractional Brownian motions. Since, by the Itˆo formula (see Oksendal [23], for example), the differential of the approximate fractional Brownian motion is
dBγ,Ht=(H−12)(∫t0(t−s+γ)H−32dWs)dt+γH−12dWt, |
the initial model (2.2) becomes
dXt=f(Yt,Zt)XtdWxt,dYt=(1ϵα(Yt)+1√ϵ(H1−12)ϕ1,tβ(Yt))dt+1√ϵγH1−12β(Yt)dWyt,dZt=(δg(Zt)+√δ(H2−12)ϕ2,th(Zt))dt+√δγH2−12h(Zt)dWzt, | (2.3) |
where ϕ1,t and ϕ2,t are defined by
ϕ1,t:=∫t0(t−s+γ)H1−32dWys,ϕ2,t:=∫t0(t−s+γ)H2−32dWzs, | (2.4) |
where (t−s+γ)H−32 does not blow up at any s∈[0,t] and H∈(0,1). In the model (2.3), we assume that 0<δ≪ϵ≪√δ<1, Wxt, Wyt, and Wzt are standard Brownian motions defined on a filtered probability space (Ω,F,Ft,Q), they have a correlation structure given by d⟨Wx,Wy⟩t=ρxydt, d⟨Wx,Wz⟩t=ρxzdt, and d⟨Wy,Wz⟩t=ρyzdt, and H1∈(0,12) and H2∈(12,1). If Yt is a mean-reverting process, its mean itself is also mean-reverting slowly, and it is driven by Zt, then this situation is called double-mean-reverting (cf. Gatheral [18]). Wy and Wz are assumed to be correlated here so that the model can somewhat capture the double-mean-reverting property of stochastic volatility even if the dependence of Yt on Zt is not explicitly specified. The functions f, α, β, g, and h are assumed to satisfy necessary smooth and boundedness conditions for the stochastic differential equation for Xt to have a unique solution. The volatility factor Yt in (2.3) reflects rapid variation (for example, rapid mean reversion) while the volatility factor Zt represents slow variation because those processes correspond to the solutions of stochastic differential equations in which time t is replaced by t/ϵ (sped up) and δt (slowed down), respectively. Particularly, the process Yt is assumed to be ergodic and have an invariant distribution, denoted by Φ, which allows us to use averaging principles in Fouque et al. [15] to approximate the option price. Of course, the model (2.3) is reduced to the model of Fouque et al. [14] if both H1 and H2 are equal to 1/2.
While fractional Brownian motion was stressed to capture long memory in the early age of fractional stochastic volatility model development such as the study of Comte and Renault [9], it has been discovered empirically since then that those models are valid only for long-term behavior of volatility, while some rough volatility models are more appropriate in the short run (see, in particular, Gatheral et al. [19]). This has led several authors to introduce volatility models incorporating both roughness, meaning exponentially decaying autocorrelation, and long memory, meaning non-integrable autocorrelation, corresponding to two different Hurst exponents. Refer to Alˊos and Leon [3] and Bennedsen et al. [5], for instance. This paper seeks to relate two characteristic (fast and slow) time scales of the multiscale stochastic volatility model of Fouque et al. [15] to the roughness and the long memory, respectively. This approach allows us to obtain an option pricing formula that can be calculated easily starting from the Black-Scholes price.
In this paper, the following lemmas are useful for asymptotic analysis. They are the solvability condition of a Poisson equation and the growth condition related to the infinitesimal generator of the ergodic process Yt, 1ϵA0, where A0 is a differential operator defined as
A0:=α(y)∂y+12β2(y)γ2H1−1∂yy. |
Lemma 3.1. The Poisson equation
A0p(t,x,y,z)+q(t,x,y,z)=0 |
has a solution p(t,x,y,z) if and only if the function q is centered with respect to the invariant distribution Φ of the process Y, i.e.,
⟨q(t,x,⋅,z)⟩:=∫q(t,x,y,z)Φ(y)dy=0. |
Proof. This is a version of the Fredholm alternative. Refer to Section 3.2 in Fouque et al. [15].
Lemma 3.2. Assume that equation A0p(t,x,y,z)=0 admits only solutions that do not grow as fast as
∂yp(t,x,y,z)∼e∫(−2α)/β2γ2H1−1dy,y→∞. |
Then the solution p does not depend on y.
Proof. Solving the equation A0p=0 directly leads to this result.
Since the approximate fractional Brownian motion Bγ,Ht is a semimartingale, the no-arbitrage theory is allowed for the model (2.3) by the fundamental theorem of asset pricing (see Pascucchi [25], for example). So, one can use the replicating portfolio approach to value the options. If Pϵ,δ(t,x,y,z;ϕ1,ϕ2) denotes the option price with a payoff function H(x) under the model (2.3) when Xt=x, Yt=y, Zt=z, ϕ1,t=ϕ1, and ϕ2,t=ϕ2, then the no-arbitrage argument with the self-financing assumption and the Itˆo formula leads to a final value problem expressed by
Aϵ,δPϵ,δ(t,x,y,z;ϕ1,ϕ2)=0,0≤t<T,Pϵ,δ(T,x,y,z;ϕ1,ϕ2)=H(x), | (3.1) |
where the multiscale operator Aϵ,δ is
Aϵ,δ:=1ϵA0+1√ϵA1+A2+√δϵA3+√δA4+δA5,A0:=α(y)∂y+12β2(y)γ2H1−1∂yy,A1:=β(y)(H1−12)ϕ1∂y+ρxyf(x,y)β(y)γH1−12D1∂y,A2:=∂t+12f2(y,z)D2,A3:=ρyzβ(y)h(z)γH1+H2−1∂yz,A4:=ρxzf(y,z)h(z)γH2−12D1∂z+h(z)(H2−12)ϕ2∂z,A5:=g(z)∂z+12h2(z)γ2H2−1∂zz, | (3.2) |
where the operator symbol Dn is defined by
Dn:=xn∂xn,n=1,2. |
From now on, the dependence of Pϵ,δ on ϕ1 and ϕ2 is omitted for notational simplicity. Since the PDE problem (3.1) is a singular perturbation problem, we are interested in an asymptotic solution of the form
Pϵ,δ(t,x,y,z)=∞∑i,j=0(√δ)i(√ϵ)jPij(t,x,y,z). | (3.3) |
Following the multiscale asymptotic analysis of Fouque et al. [15] and using the operator A BS defined by
A BS:=∂t+12ˉσ2f(z)D2,ˉσf(z):=√⟨f2(⋅,z)⟩, |
one can find that P00, P01, and P10 are independent of the variable y and they satisfy the PDE problems
A BSP00(t,x,z)=⟨A2⟩P00=0,P00(T,x,z)=H(x),A BSP01(t,x,z)=⟨A1A−10(A2−⟨A2⟩)⟩P00=AH11(z)D2P00(t,x,z)+AH12(z)D1D2P00(t,x,z),P01(T,x,z)=0,A BSP10(t,x,z)=−⟨A4⟩P00=−BH21(z)D1∂zP00(t,x,z)−BH22(z)∂zP00(t,x,z),P10(T,x,z)=0, | (3.4) |
respectively, where the functions AH11, AH12, BH21, and BH22 are
AH11(z):=12(H1−12)ϕ1⟨β∂yψ⟩,AH12(z):=12ρxyγH1−12⟨fβ∂yψ⟩,BH21(z):=ρxzγH2−12⟨f⟩h(z),BH22(z):=(H2−12)ϕ2h(z), | (3.5) |
respectively. Here, ψ(y,z) is a function defined by the solution to
A0ψ(y,z)=f2(y,z)−⟨f2(⋅,z)⟩. | (3.6) |
Note that AH11(z) and AH12(z) are related to the fast variation of volatility and a Hurst exponent less than 12 while BH21 and BH22 are connected with the slow-scale variation of volatility and a Hurst exponent larger than 12.
Since A BS is the differential operator ∂t plus the infinitesimal generator of a geometric Brownian motion solving the stochastic differential equation
dXt=ˉσf(z)XtdWxt |
as its notation suggests, the PDE problem A BSP00(t,x,z)=0 with the final condition P00(T,x,z)=H(x) gives us that P00 is the Black-Scholes option price (cf. Black and Scholes [6]) with constant volatility replaced by z-dependent volatility, and subsequently we use notation P BS(t,x,z) instead of P00(t,x,z) from now on.
By solving the PDE problems in (3.4) for P BS, P01, and P10, we obtain the following European option price formula.
Proposition 3.1. Under the dynamics of (2.3) of the underlying asset price, the option price Pϵ,δ is approximated by ¨Pϵ,δ:=PBS+√ϵP01+√δP10, that is
¨Pϵ,δ(t,x,z)=PBS(t,x,z)−(T−t)[Aϵ,H11(z)(D21−D1)−Aϵ,H12(z)(−D31+D21)]PBS(t,x,z),−(T−t)2[Bδ,H21(z)(−D31+D21)−Bδ,H22(z)(D21−D1)]PBS(t,x,z), | (3.7) |
where Dn1:=(D1)n=(x∂∂x)n and Aϵ,H11, Aϵ,H12, Bδ,H21, and Bδ,H22 are given by
Aϵ,H11(z):=√ϵAH11(z),Aϵ,H12(z):=√ϵAH12(z)Bδ,H21(z):=12√δˉσf(z)ˉσ′f(z)BH21(z),Bδ,H22(z):=12√δˉσf(z)ˉσ′f(z)BH22(z), |
respectively.
Proof. To solve the PDE problems in (3.4) for P BS, P01, and P10, we use the Mellin transform and its inverse transform defined by
(Mg)(ω):=ˆg(ω)=∫g(s)sω−1ds,(M−1ˆg)(s):=g(s)=12πi∫a+i∞a−i∞ˆg(ω)s−ωdω, |
respectively, where a is a real number, and obtain the ODE problems for ˆP01 and ˆP10 as follows.
∂tˆP BS(t,ω,z)+λ(ω,z)ˆP BS(t,ω,z)=0,ˆP BS(T,ω,z)=ˆh(ω),∂tˆP01(t,ω,z)+λ(ω,z)ˆP01(t,ω,z)=η1(ω,z)ˆP BS(t,ω,z),ˆP01(T,ω,z)=0,∂tˆP10(t,ω,z)+λ(ω,z)ˆP10(t,ω,z)=η2(ω,z)∂zˆP BS(t,ω,z),ˆP10(T,ω,z)=0, | (3.8) |
where ˆh(ω) is the Mellin transform of h(x) and the functions λ(ω,z), η1(ω,z), and η2(ω,z) are
λ(ω,z):=12ˉσ2f(z)ω(ω+1),η1(ω,z):=AH11(z)ω(ω+1)−AH12(z)ω2(ω+1),η2(ω,z):=BH21(z)ω−BH22(z), | (3.9) |
respectively. The solutions of (3.8) are given by
ˆP BS(t,ω,z)=eλ(ω,z)(T−t)ˆh(ω),ˆP01(t,ω,z)=−(T−t)η1(ω,z)eλ(ω,z)(T−t)ˆh(ω),ˆP10(t,ω,z)=−12(T−t)2η2(ω,z)ˉσf(z)ˉσ′f(z)ω(ω+1)eλ(ω,z)(T−t)ˆh(ω). | (3.10) |
Substituting (3.9) into (3.10), we obtain the following Mellin transform of ¨Pϵ,δ explicitly:
ˆ¨Pϵ,δ(t,ω,z)=ˆP BS(t,ω,z)−√ϵ(T−t)(AH11(z)(ω2+ω)−AH12(z)(ω3+ω2))ˆP BS(t,ω,z)−√δ(T−t)22ˉσf(z)ˉσ′f(z)(BH21(z)(ω3+ω2)−BH22(z)(ω2+ω))ˆP BS(t,ω,z). | (3.11) |
The pricing formula (3.11) is given by a linear combination of terms that are in the form of a product of ˆP BS and a polynomial function of ω. So, we can calculate the formula explicitly through the following property of the Mellin transform: M((D1)nf)(ω)=(−ω)nˆf(ω). Using an inverse Mellin transform on (3.11), we obtain a closed-form formula given in the proposition.
Therefore, once the Black-Scholes option price P BS is given, we can calculate the approximation ¨Pϵ,δ by computing the derivatives of P BS in the formula (3.7) and plugging the estimated group parameters Aϵ,H11, Aϵ,H12, Bδ,H21, and Bδ,H22 into (3.7). Note that the original model parameters and functions such as γ, ϵ, δ, α, β, g, and h are not required to be directly chosen for the purpose of the option price approximation. The group parameters are required to be chosen for calculating ¨Pϵ,δ. We use the implied volatility term structure of SPX call options to estimate those four group parameters. See Section 5.2 for details.
Remark: One can obtain the second-order terms P02, P11, and P20 further and a subsequent formula for the approximation ⃛Pϵ,δ:=P BS+√δP10+√ϵP01+δP02+√δϵP11+ϵP20 as shown in Appendix A, where the approximation error is also given. We note that the same approach (approximation to the Black-Scholes price) used in this section and the following section are also used in the more simple framework of the Heston model driven by the standard Brownian motion in Alˊos et al. [1].
All of the original model parameters given in the model (2.3) are not required to price derivatives. In fact, from Proposition 3.1, we notice that ˉσf, Aϵ,H11, Aϵ,H12, Bδ,H21, and Bδ,H22 are the ones required to be estimated. In order to estimate those pricing parameters, we can utilize calibration from near-the-money European call option implied volatilities. The volatility Iϵ,δ implied by the pricing formula (3.7) is defined by the solution to the equation P BS(t,x;Iϵ,δ)=¨Pϵ,δ(t,x,z), where P BS(t,x;σ) stands for the classical Black-Scholes call option price formula with volatility σ. Then the two correction terms I01 and I10 of the asymptotic expansion
Iϵ,δ(t,x,y,z)=∞∑i,j=0(√δ)i(√ϵ)jIij(t,x,y,z) |
are given by I01=(∂σPBS)−1P01 and I10=(∂σPBS)−1P10, respectively, and the leading term I00 is defined as
I00(t,x,y,z):=ˉσf(z). |
Using the vega-gamma and speed-gamma relationships, i.e.,
∂σPBS=ˉσf(T−t)x2∂xxPBS,∂xxxPBS=(d1ˉσf√T−t+1)(−1x)∂xxPBS,d1:=log(x/K)+12ˉσ2f(T−t)ˉσf√T−t, |
we can obtain an approximate implied volatility surface ¨Iϵ,δ:=I00+√ϵI01+√δI10 given by
¨Iϵ,δ(T−t,K)=ˉσf−1ˉσf[Aϵ,H11+Aϵ,H12(1−d1ˉσf√T−t)]+T−tˉσf[Bδ,H21(1−d1ˉσf√T−t)−Bδ,H22]. | (4.1) |
We are interested in the slope behavior of the implied volatility skew with respect to time-to-maturity and the Hurst exponent. The slope of the implied volatility skew is given by
∂¨Iϵ,δ∂k(T−t,K)=1ˉσ3f(−Aϵ,H12T−t+Bδ,H21), | (4.2) |
where k:=logK.
Based on the calibration result shown in Section 5.2 for SPX options, we do a numerical experiment to show how the skew slope behaves against time-to-maturity. Figure 1 presents the experimental result for the skew slope term structure. It shows that the slope tends to blow up as time-to-maturity becomes shorter.
Comte and Renault [9] considered a Hurst exponent with H>12 to explain the slow flattening of skews and smiles of the implied volatility surface when time-to-maturity increases, while Alòs et al. [2] gave a better description of the short time-to-maturity blow-up of the implied volatility surface with a Hurst exponent H<12. So, our result is consistent with these two results in that the skew slope becomes small when time-to-maturity increases while it becomes large when time-to-maturity decreases as seen in Figure 1. Our result unifies these two separate previous results. This is desirable in practice as the market volatility has both long- and short-memory properties depending on the situation. As described by (4.2), the skew slope ∂¨Iϵ,δ∂k can behave flexibly depending on the appropriate Hurt parameters H1 and H2 in the range of (0,12) and (12,1), respectively. As a consequence, the implied volatility surface can be calibrated over a wide range of time-to-maturities.
In this section, we check the accuracy and performance of the price formula given by (3.7) in Proposition 3.1 for European call options via Monte Carlo simulation. We give an example of calibrating the pricing parameters for three different time-to-maturities. We also investigate the sensitivity of the implied volatility to the Hurst exponents H1 and H2.
Using the well-known Greeks in the Black-Scholes model, one can verify easily that the derivative price ¨P(t,x,z) given by (3.7) satisfies the following identity for a European call option with strike K:
¨P(t,x,z)=xN(d1(x,z;K))−KN(d2(x,z;K))−K(T−t)φ(d2(x,z;K),0,1)ˉσf(z)√T−t[Aϵ,H11−d2(x,z;K)ˉσf(z)√T−tAϵ,H12]−K(T−t)2φ(d2(x,z;K),0,1)ˉσf(z)√T−t[d2(x,z)ˉσf(z)√T−tBδ,H21−Bδ,H22], | (5.1) |
where N, d1, d2, and φ are given by
N(ω):=∫ω−∞φ(ϖ,0,1)dϖ,d1(x,z;ϖ)):=ln(x/ϖ))ˉσf(z)√T−t+12ˉσf(z)√T−t,d2(x,z;ϖ)):=ln(x/ϖ))ˉσf(z)√T−t−12ˉσf(z)√T−t,φ(ϖ,μ,σ):=1√2σ2πe−(ϖ−μ)22σ2, | (5.2) |
respectively.
In this section, we calculate option prices under three different models and a Monte Carlo simulation result (with 1 million simulations) and compare them with real market data, that is, the SPX option data observed on December 7, 2022. Notations Pmarket, PMC, PfMSV, PMSV, and PBS stand for the market option price, the Monte Carlo (MC) simulation result, the option price computed by the formula (5.1) corresponding to the fractional multiscale stochastic volatility (fMSV) model (2.3), the option price computed under a multiscale stochastic volatility (MSV) model corresponding to the case of H1=H2=12 in the formula (5.1), and the Black-Scholes option price, respectively.
For Monte Carlo simulation, we need random numbers generated by the stochastic processes ϕ1,t and ϕ2,t in (2.4). The time interval [0,T] is discretized into t0(=0), t1, t2, ⋯, tn(=T) satisfying t0<t1<⋯<tn with Δt=ti−ti−1, i=1,2,⋯,n. Omitting the subscript number and superscript letter of ϕ1,t, ϕ2,t, H1, H2, Wys, and Wzs (and so using ϕt, H, and Ws), the random source ϕt satisfies the following recursive equation by the Itˆo calculus:
ϕtk=k∑i=1(tk−ti−1+γ)H−32(Wti−Wi−1)d=k∑i=1(Δt(k−i+1)+γ)H−32√ΔtZid=k∑i=1(Δt(k−i+1)+γ)H−32√ΔtZk−i−1d=k∑i=1(iΔt+γ)H−32√ΔtZid=(kΔt+γ)H−32√ΔtZk+ϕtk−1, |
where the notation d= denotes distributional equality and the Zi's are independent and identically (standard normal) distributed. Thus, we generate the random sources ϕti, i=1,2,⋯,n, recursively, by the following algorithm:
ϕti←(iΔt+γ)H−32√ΔtZi+ϕti−1. |
Applying this algorithm to (2.3), we can obtain a Monte Carlo simulation result PMC for the European options. Table 1 represents the setting of the related parameters and functions for finding the price PMC.
Parameter | Value |
H1 | 0.0998 |
H2 | 0.7984 |
γ | 0.6986 |
dt | 3.9683×10−5 |
ϵ | 0.01 |
δ | 0.001 |
S0 | 3941.26 |
Y0 | 0.001 |
Z0 | 0.001 |
ϕ1, ϕ2 | 1 |
Function | Choice |
f(y,z) | ν1+e−x+e−y,ν=0.5988 |
α(y) | −y |
β(y) | √2 |
g(z) | z |
h(z) | z |
We calculate Pmarket, PMC, PfMSV, PMSV, and PBS for a European call option with two time-to-maturities, where PMC is obtained based on the setting in Table 1. Using these results, we compute the square norms ∥Pmarket−PMC∥, ∥Pmarket−PfMSV∥, ∥Pmarket−PMSV∥, and ∥Pmarket−PBS∥ for the purpose of comparing the Monte Carlo simulation and the three different volatility models. Table 2 presents the result. It shows that the fMSV model outperforms the other models including the Monte Carlo simulation. This tends to be the case more conspicuously when time-to-maturity becomes shorter. Note that shorter time-to-maturity options tend to have higher trading volume in general. Therefore, using the approximate fractional Brownian motion instead of the standard Brownian motion for volatility seems to provide a great advantage in option pricing. On the other hand, Table 3 provides the elapsed time of option pricing based on the Monte Carlo simulation (reputation number = 1,000,000 and dt = 1/25200), and the three different volatility models. The computer used for the computation is specified as Intel(R) Core(TM)-i9-10900 CPU, Windows 10 Pro O/S and 64GB RAM. Moreover, the program used for the computation is MATLAB R2022b. The Monte Carlo simulation method takes much more time than the analytic methods based on the three different volatility models while the three different models are relatively similar to each other in terms of the elapsed pricing time.
Days to Maturity: 31 | |||
∥Pmarket−PMC∥ | ∥Pmarket−PfMSV∥ | ∥Pmarket−PMSV∥ | ∥Pmarket−PBS∥ |
28.7959 | 18.3497 | 31.6503 | 36.7458 |
Days to Maturity: 56 | |||
∥Pmarket−PMC∥ | ∥Pmarket−PfMSV∥ | ∥Pmarket−PMSV∥ | ∥Pmarket−PBS∥ |
97.7220 | 69.4679 | 90.5983 | 90.8465 |
PMC | PfMSV | PMSV | PBS |
307.7409 | 3.9800×10−4 | 2.8880×10−4 | 2.3640×10−4 |
Among those parameters required to be estimated, i.e., ˉσf, Aϵ,H11, Aϵ,H12, Bδ,H21, and Bδ,H22, the parameter ˉσf is first estimated from historical SPX data over a period of time in the near past, where the slow-scale variable (z) dependence of ˉσf accounts for updating the long-run average from time to time. To estimate the group parameters Aϵ,H11, Aϵ,H12, Bδ,H21, and Bδ,H22, we rewrite the implied volatility surface (4.1) as
¨Iϵ,δ(T−t,K)=ˉσf+[aϵ+cδ(T−t)]+[bϵ+dδ(T−t)]ln(K/x)T−t, |
where the parameters aϵ, bϵ, cδ, and dδ are related to the pricing parameters Aϵ,H11, Aϵ,H12, Bδ,H21, and Bδ,H22 through the relationship
Aϵ,H11=−ˉσf(aϵ+12ˉσ2fbϵ),Aϵ,H12=−ˉσ3fbϵ,Bδ,H21=ˉσ3fdδ,Bδ,H22=ˉσf(cδ+12ˉσ2fdδ). | (5.3) |
So, once aϵ, bϵ, cδ, and dδ are estimated from calibration to the implied volatility term structure of SPX call options, one can use the relationship (5.3) to estimate the pricing group parameters Aϵ,H11, Aϵ,H12, Bδ,H21, and Bδ,H22 and calculate the derivative price ¨Pϵ,δ(t,x,z) obtained in Proposition 3.1.
More concretely, the averaged volatility ˉσf is first estimated using the 10-day historical volatilities calculated from the SPX data obtained from the site http://www.investing.com and then the parameters aϵ, bϵ, cδ, and dδ are estimated using the SPX call option data obtained from the site http://www.barchart.com. Figure 2 shows the implied volatilities of the SPX option in the real market and the curve ¨Iϵ,δ fitted to the market data. From this fit, aϵ, bϵ, cδ, and dδ are estimated and then the pricing group parameters Aϵ,H11, Aϵ,H12, Bδ,H21, and Bδ,H22 are determined. Figure 3 demonstrates the corresponding result observed at one day.
The Hurst exponents H1 and H2 related to the fast- and slow-scale variations of the volatility, respectively, are important parameters in our underlying asset price model (2.3). To investigate the dependence of the implied volatility (4.1) on the Hurst exponents, we rewrite (4.1) as follows:
¨Iϵ,δ(T−t,K)=ˉσf−1ˉσf[aϵ1(H1)(H1−12)+aϵ2(H1)γH1−12(1−d1ˉσf√T−t)]+(T−t)ˉσf[bδ1(H2)γH2−12(1−d1ˉσf√T−t)−bδ2(H2)(H2−12)], |
where aϵ1(H1), aϵ2(H1), bδ1(H2), and bδ2(H2) are
aϵ1(H1)=Aϵ,H11(H1−12)−1,aϵ2(H1)=Aϵ,H12γ−H1+12,bδ1(H2)=Bδ,H21γ−H2+12,bδ2(H2)=Bδ,H22(H2−12)−1, |
respectively.
In Figure 4, we demonstrate the behavior of the implied volatility ¨I with respect to the Hurst parameters H1 and H2. Figure 4(a) shows ¨I against H1 for a variety of H2 while Figure 4(b) shows ¨I against H2 for a variety of H1. The figures indicate that ¨I increases as H1 decreases or H2 increases for any fixed H2 or H1, respectively. So, the implied volatility becomes higher when the fast-scale motion of the volatility becomes "rougher" and the slow-scale motion of the volatility becomes "smoother". This is an interesting result in view of modeling stochastic volatility. This provides us with one of the reasons why we need a multiscale framework for stochastic volatility.
In this section, we check the accuracy of the approximation PfMSV with respect to the parameters γ, ϵ, and δ. We choose a very small value, close to zero, of each of the parameters and show that the approximations converge to the Monte Carlo simulation result, denoted by PMC*, with the small parameter(s).
Table 4 shows how PfMSV converges to PMC*=41.2643 as γ goes to 0.0001. On the other hand, Table 5 shows how the approximations PfMSV move to the Monte Carlo simulation result PMC*=41.2576 when ϵ and δ go to 1.0000×10−6 and 1.0000×10−9, respectively. We note that the correlation term ρyz does not appear in the first-order approximation of our interest in this article but it would appear in higher order approximation. We assume ρyz=0 in the numerical experiment in order to match the situation given by our first-order approximation.
Convergence of PfMSV to PMC* | ||
γ | PfMSV | ‖PMC*−PfMSV‖ |
0.99000 | 40.8679 | 0.3964 |
0.89101 | 40.8901 | 0.3742 |
0.79202 | 40.9096 | 0.3548 |
0.69303 | 40.9347 | 0.3300 |
0.59404 | 40.9613 | 0.3030 |
0.49505 | 40.9914 | 0.2730 |
0.39606 | 41.0247 | 0.2396 |
0.29707 | 41.0629 | 0.2014 |
0.19808 | 41.1082 | 0.1562 |
0.09909 | 41.1661 | 0.0983 |
0.00010 | 41.2595 | 0.0048 |
Convergence of PfMSV to PMC* | |||
δ | ϵ | PfMSV | ‖PMC*−PfMSV‖ |
1.0000×10−5 | 1.0000×10−4 | 39.7545 | 1.5031 |
9.0001×10−6 | 9.0100×10−5 | 39.8316 | 1.4260 |
8.0002×10−6 | 8.0200×10−5 | 39.9131 | 1.3445 |
7.0003×10−6 | 7.0300×10−5 | 39.9997 | 1.2578 |
6.0004×10−6 | 6.0400×10−5 | 40.0928 | 1.1648 |
5.0005×10−6 | 5.0500×10−5 | 40.1938 | 1.0638 |
4.0006×10−6 | 4.0600×10−5 | 40.3053 | 0.9523 |
3.0007×10−6 | 3.0700×10−5 | 40.4315 | 0.8261 |
2.0008×10−6 | 2.0800×10−5 | 40.5803 | 0.6773 |
1.0009×10−6 | 1.0900×10−5 | 40.7715 | 0.4861 |
1.0000×10−9 | 1.0000×10−6 | 41.1209 | 0.1367 |
In general, the prices of exotic options can be determined after option pricing models are calibrated to market data of plain vanilla options. In this section, we extend the pricing result for European vanilla options under the fractional multiscale stochastic volatility model (2.3) to two types of path-dependent exotic options, i.e., barrier and lookback options.
Barrier options are similar to vanilla options but they only become activated or extinguished when the underlying asset hits a specific price level (the so-called "barrier"). So, the value of barrier options can jump up or down greatly. This type of option is commonly traded in the foreign exchange and equity markets.
Given the model (2.3), let Uϵ,δ(t,x,y,z) be the price of a down-and-out (D/O) barrier option, where a payoff function is given by
H(XT)=(XT−K)+1{inf | (6.1) |
with a strike price K , a barrier level B , and an expiration time T . From the no-arbitrage theory with the self-financing condition and the It \hat{\mbox{o}} formula, U^{\epsilon, \delta}(t, x, y, z) satisfies the PDE problem
\begin{equation} \begin{split} \mathcal{A}^{\epsilon, \delta} U^{\epsilon, \delta}(t, x, y, z) & = \, 0, \quad 0\leq t < T, \\ U^{\epsilon, \delta}(T, x, y, z) & = \, (x-K)^{+}, \quad U^{\epsilon, \delta}(t, B, y, z) = \, 0, \end{split} \end{equation} | (6.2) |
where the multiscale operator \mathcal{A}^{\epsilon, \delta} is defined by (3.2).
We are going to derive a solution of the form
\begin{equation} U^{\epsilon, \delta}(t, x, y, z) = \sum\limits_{i, j = 0}^{\infty}(\sqrt{\delta})^{i}(\sqrt{\epsilon})^{j}\, U_{ij}(t, x, y, z) \end{equation} | (6.3) |
as an approximate solution of the PDE problem (6.2). Substituting the series expansion (6.3) into the PDE problem (6.2) and using the same methodology as used for Proposition 3.1, one can have the following PDE problems for the terms U_{ij}(t, x, y, z) , (i, j) \in \left\{(0, 0), (0, 1), (1, 0) \right\} :
\begin{equation} \begin{split} \mathcal{A}_{ \text{ BS}} U_{00}(t, x, z) & = \, \langle\mathcal{A}_2\rangle U_{00} = 0, \\ U_{00}(T, x, z)& = (x-K)^{+}, \\ U_{00}(T, B, z)& = 0, \\ & \\ \mathcal{A}_{ \text{ BS}} U_{01}(t, x, z) & = \, A_{1}^{H_1}(z)\mathcal{D}_{2}U_{00}(t, x, z) + A_{2}^{H_1}(z)\mathcal{D}_{1}\mathcal{D}_{2}U_{00}(t, x, z), \\ U_{01}(T, x, z)& = 0, \\ U_{01}(t, B, z)& = 0, \\ & \\ \mathcal{A}_{ \text{ BS}} U_{10}(t, x, z) & = \, -B_{1}^{H_2}(z)\mathcal{D}_{1}\partial_z U_{00}(t, x, z)-B_{2}^{H_2}(z) \partial_z U_{00}(t, x, z), \\ U_{10}(T, x, z)& = 0, \\ U_{10}(t, B, z)& = 0, \end{split} \end{equation} | (6.4) |
where A_{1}^{H_1}(z) , A_{2}^{H_1}(z) , B_{1}^{H_2}(z) , and B_{2}^{H_2}(z) are given by (3.5).
The following lemma is useful to solve the PDE problems for the terms U_{01} and U_{10} .
Lemma 6.1. Consider the PDE problems
\begin{equation*} \begin{split} \mathcal{A}_{ \mathit{\text{BS}}} u(t, x, z) & = \, \left(T-t\right)^{n} \xi(t, x, z), \quad t < T, \, \, \, x > B, \quad (n = 0, 1, 2, \cdots), \\ u(T, x, z) & = \, 0, \quad u(t, B, z) = \, 0. \end{split} \end{equation*} |
If \xi satisfies the equation \mathcal{A}_{ \mathit{\text{BS}}} \xi(t, x, z) = 0 , then the solution u(t, x, z) can be decomposed into
\begin{equation*} \begin{split} u(t, x, z) = u_{1}(t, x, z)+u_{2}(t, x, z), \end{split} \end{equation*} |
where u_{1} and u_{2} are solutions to the PDE problems given by
\begin{equation*} \begin{split} \mathcal{A}_{ \mathit{\text{BS}}} u_{1}(t, x, z)& = \left(T-t\right)^{n}\xi(t, x, z), \\ u_{1}(T, x, z)& = 0, \\ u_{1}(t, B, z)& = -\frac{1}{n+1}\left(T-t\right)^{n+1}\xi(t, B, z), \\ & \\ \mathcal{A}_{ \mathit{\text{BS}}} u_{2}(t, x, z)& = 0, \\ u_{2}(T, x, z)& = 0, \\ u_{2}(t, B, z)& = \frac{1}{n+1}\left(T-t\right)^{n+1}\xi(t, B, z), \end{split} \end{equation*} |
respectively. Furthermore, the solutions u_{1} and u_{2} are given by
\begin{equation*} \begin{split} u_{1}(t, x, z) & = \, -\frac{1}{n+1}\left(T-t\right)^{n+1}\xi(t, x, z), \\ u_{2}(t, x, z) & = \, \frac{\left(x/B\right)^{1/2}}{n+1}\left(T-t\right)^{n+1}\frac{\ln{\left(x/B\right)}}{\bar{\sigma}_{f}\sqrt{2\pi}}\\ &\quad \times \int_{t}^{T}\frac{1}{\left(\tau-t\right)^{3/2}}\, \exp{\left[-\left(\frac{1}{8}\bar{\sigma}_f ^2\, \left(\tau-t\right)+\frac{\ln^2{\left(x/B\right)}}{2\bar{\sigma}^{2}_f \, (\tau-t)}\right)\right]}\, \xi(t, B, z)\, d\tau, \end{split} \end{equation*} |
respectively.
Proof. This lemma is related to the Black-Scholes framework with volatility \bar{\sigma}_{f} . Refer to Section 6.2 in Fouque et al. [15] for a proof.
Based on Lemma 6.1, we obtain the following semi-closed form formula for an approximate value of U^{\epsilon, \delta}(t, x, y, z) .
Proposition 6.1. Under the dynamics of (2.3), the option price U^{\epsilon, \delta}(t, x, y, z) is approximated by \ddot{U}^{\epsilon, \delta}\!: = \! U_{ \mathit{\text{BS}}}+\sqrt{\epsilon}U_{01}+\sqrt{\delta}U_{10} , that is
\begin{equation*} \begin{split} \label{b_pricingformula} \ddot{U}^{\epsilon, \delta}(t, x, z) = & \, U_{ \mathit{\text{BS}}}(t, x, z)-\left[(T-t) \mathcal{H}_{ \mathit{\text{01}}}^{\epsilon, H_1} +(T-t)^2 \mathcal{H}_{ \mathit{\text{10}}}^{\delta, H_2} \right] U_{ \mathit{\text{BS}}}(t, x, z)\\ &+\left(x/B\right)^{1/2}\frac{\ln{\left(x/B\right)}}{\bar{\sigma}_{f}\sqrt{2\pi}}\int_{t}^{T}\frac{1}{\left(\tau-t\right)^{3/2}} \, \exp{\left(-\frac{1}{8}\bar{\sigma}_{f}^2\, \left(\tau-t\right)-\frac{\ln^2{\left(x/B\right)}}{2\bar{\sigma}^{2}_f\, \left(\tau-t\right)}\right)}\\ &\qquad\qquad\qquad\qquad\qquad \times \left((T-t) \mathcal{H}_{ \mathit{\text{01}}}^{\epsilon, H_1}+(T-t)^2 \mathcal{H}_{ \mathit{\text{01}}}^{\delta, H_2}\right) U_{ \mathit{\text{BS}}}(\tau, B, z)\, d\tau, \end{split} \end{equation*} |
where U_{ \mathit{\text{BS}}}(t, x, z) is defined by
\begin{equation*} \begin{split} U_{ \mathit{\text{BS}}}(t, x, z) : = \, \left\{ \begin{array}{ll} P_{ \mathit{\text{BS}}}\left(t, x, z; K\right)-\frac{x}{B}P_{ \mathit{\text{BS}}}\left(t, \frac{B^2}{x}, z; K\right), &{\rm if}\, \, K > B \\ P_{ \mathit{\text{BS}}}\left(t, x, z; B\right))-\frac{x}{B}P_{ \mathit{\text{BS}}}\left(t, \frac{B^2}{x}, z; B\right), &{\rm if}\, \, K < B\\ \end{array} \right. \end{split} \end{equation*} |
and \mathcal{H}^{\epsilon, H_1}_{ \mathit{\text{01}}} and \mathcal{H}^{\delta, H_2}_{ \mathit{\text{10}}} are differential operators defined by
\begin{equation} \begin{split} & \mathcal{H}_{ \mathit{\text{01}}}^{\epsilon, H_1}: = \, A^{\epsilon, H_1}_1(z) \left(\mathcal{D}_{1}^2 - \mathcal{D}_{1} \right) - A^{\epsilon, H_1}_2(z) \left(-\mathcal{D}_{1}^3 + \mathcal{D}_{1}^2\right), \\ &\mathcal{H}_{ \mathit{\text{10}}}^{\delta, H_2}: = \, B^{\delta, H_2}_1(z) \left(-\mathcal{D}_{1}^3 + \mathcal{D}_{1}^2\right) - B^{\delta, H_2}_2(z) \left(\mathcal{D}_{1}^2 - \mathcal{D}_{1}\right), \end{split} \end{equation} | (6.5) |
respectively. Here, P_{ \mathit{\text{BS}}}\left(t, x, z; \varpi\right) is the Black-Scholes call option price given by
\begin{equation} \begin{split} P_{ \mathit{\text{BS}}}\left(t, x, z; \varpi\right) = x\mathcal{N}\bigl(d_1(x, z; \varpi)\bigr)+\varpi\mathcal{N}\bigl(d_2(x, z; \varpi)\bigr), \end{split} \end{equation} | (6.6) |
where d_{i}(s, z; \varpi) (i = 1, 2) are defined in (5.1). The group parameters A^{\epsilon, H_1}_1(z) , A^{\epsilon, H_1}_2(z) , B^{\delta, H_2}_1(z) , and B^{\delta, H_2}_2(z) are defined in Proposition 3.1.
Proof. First of all, since U_{00} satisfies a PDE problem for a D/O barrier option under the Black-Scholes model with volatility \bar{\sigma}_{f} as seen in (6.4), it becomes U_{ \text{ BS}} defined in the proposition.
On the other hand, from (6.4), we have the following PDE problems for \ddot{U}_{01}: = \sqrt{\epsilon}U_{01} and \ddot{U}_{10}: = \sqrt{\delta}U_{10} :
\begin{equation} \begin{split} \mathcal{A}_{ \text{ BS}} \ddot{U}_{01}(t, x, z) & = \, \mathcal{H}_{ \text{ 01}}^{\epsilon, H_1} U_{ \text { BS}}(t, x, z), \\ \ddot{U}_{01}(T, x, z)& = 0, \\ \ddot{U}_{01}(t, B, z)& = 0, \\ & \\ \mathcal{A}_{ \text{ BS}} \ddot{U}_{10}(t, x, z) & = \, 2(T-t)\mathcal{H}_{ \text{ 10}}^{\delta, H_2} U_{ \text { BS}}(t, x, z), \\ \ddot{U}_{10}(T, x, z)& = 0, \\ \ddot{U}_{10}(t, B, z)& = 0, \end{split} \end{equation} | (6.7) |
respectively. Applying Lemma 6.1 to (6.7) directly, one can obtain solutions for \ddot{U}_{01} and \ddot{U}_{10} which lead to \ddot{U}^{\epsilon, \delta}(t, x, z) given in the proposition when they are added to U_{00} .
A lookback option is an exotic option that allows the holder to exercise an option at the most favorable (minimum or maximum) price of the underlying asset over the life of the option. The floating strike lookback option eliminates the risk associated with the market entry time. In this section, we obtain a pricing formula for the floating strike lookback call option under the model (2.3). In terms of a stochastic process defined by
\begin{equation*} \begin{split} m_t = \inf\limits_{ \{ 0 \leq \tau \leq t \} }X_{\tau} \end{split} \end{equation*} |
(the minimum value from the contract time 0 until the current time t ), we let V^{\epsilon, \delta}(t, m, x, y, z) denote the price of the lookback call option, where the payoff function H(x, m) is given by
\begin{equation} \begin{split} H(x, m) = (x-m)^{+}. \end{split} \end{equation} | (6.8) |
From the no-arbitrage theory with the self-financing condition and the It \hat{\mbox{o}} formula, V^{\epsilon, \delta}(t, m, x, y, z) satisfies the PDE problem
\begin{equation} \begin{split} \mathcal{A}^{\epsilon, \delta} V^{\epsilon, \delta}(t, x, m, y, z) & = \, 0, \quad 0\leq t < T, \quad x > m, \\ V^{\epsilon, \delta}(T, x, m, y, z) & = \, (x-m)^{+}, \quad \frac{\partial}{\partial x}V^{\epsilon, \delta}(t, m, m, y, z) = \, 0. \end{split} \end{equation} | (6.9) |
We solve the PDE problem (6.9) for V^{\epsilon, \delta}(t, x, m, y, z) using the asymptotic series expansion
\begin{equation} V^{\epsilon, \delta}(t, x, m, y, z) = \sum\limits_{i, j = 0}^{\infty}(\sqrt{\delta})^{i}(\sqrt{\epsilon})^{j}\, V_{ij}(t, x, m, y, z). \end{equation} | (6.10) |
Plugging (6.10) into the PDE in (6.9) and applying the same methodology as used for Proposition 3.1 yield that V_{ij} (t, x, m, y, z) , (i, j)\in \left\{(0, 0), (0, 1), (1, 0) \right\} , satisfy the PDE problems
\begin{equation} \begin{split} \mathcal{A}_{ \text{ BS}} V_{00}(t, x, m, z) & = \, \langle\mathcal{A}_2\rangle V_{00} = 0, \\ V_{00}(T, x, m, z)& = (x-m)^{+}, \\ \partial_x V_{00}(t, m, m, z)& = 0, \\ & \\ \mathcal{A}_{ \text{ BS}} V_{01}(t, x, m, z) & = \, A_{1}^{H_1}(z)\mathcal{D}_{2}V_{00}(t, x, m, z) + A_{2}^{H_1}(z)\mathcal{D}_{1}\mathcal{D}_{2}V_{00}(t, x, z), \\ V_{01}(T, x, m, z)& = 0, \\ \partial_x V_{01}(t, m, m, z)& = 0, \\ & \\ \mathcal{A}_{ \text{ BS}} V_{10}(t, x, m, z) & = \, -B_{1}^{H_2}(z)\mathcal{D}_{1}\partial_z U_{00}(t, x, z)-B_{2}^{H_2}(z) \partial_z V_{00}(t, x, m, z), \\ V_{10}(T, x, m, z)& = 0, \\ \partial_xV_{10}(t, m, m, z)& = 0, \end{split} \end{equation} | (6.11) |
respectively.
The following lemma is useful to solve the PDE problems for the terms V_{01} and V_{10} .
Lemma 6.2. Consider a PDE problem given by
\begin{equation} \begin{split} \left(\frac{\partial }{\partial t} +\frac{1}{2}\bar{\sigma}^2_{f}(z)x^2\frac{\partial ^2}{\partial x^2}\right)v(t, x, z) & = \, \zeta(t, x, z), \, \, t < T, \, \, \, x > 0, \\ v(T, x, z) & = \, 0, \quad v_{x}(t, 0, z) = \, v(t, 0, z). \end{split} \end{equation} | (6.12) |
Then the solution v(t, x, z) is given by
\begin{equation*} \begin{split} v(t, x, z) = -\int_{t}^{T}\int_{0}^{\infty}\exp{\left(\frac{1}{2}\left(\ln{x}-w \right)\right)}\, \zeta\left(T+t-\tau, e^{w}, z\right)G(\tau, w, x, z)dwd\tau, \end{split} \end{equation*} |
where G(\tau, w, x, z) is given by
\begin{equation*} \begin{split} G(\tau, w, x, z) = &\, \left[ \varphi\left(\ln{x}, w, \bar{\sigma}_f(z)\sqrt{T-\tau} \right) +\varphi\left(\ln{x}, -w, \bar{\sigma}_f(z)\sqrt{T-\tau} \right) \right. \\ & \quad -\left. \int_{0}^{\infty}\varphi \left(\ln{x}, -(w+y), \bar{\sigma}_f(z)\sqrt{T-\tau}\right)\exp{\left(-\frac{1}{2}y\right)}dy \right]. \end{split} \end{equation*} |
Here, the function \varphi is defined in (5.2).
Proof. As the PDE in (6.12) is a non-homogeneous linear PDE with a Neumann boundary condition, by the change of variables, it can become a non-homogeneous heat equation whose solution is well-known. Refer to Polyanin and Nazaiknskii [27] for details.
Based on Lemma 6.2, we obtain the following formula for an approximate value of V^{\epsilon, \delta}(t, x, m, z) .
Proposition 6.2. Under the dynamics of (2.3), the option price V^{\epsilon, \delta}(t, x, m, z) is approximated by \ddot{V}^{\epsilon, \delta}\!: = \! V_{ \mathit{\text{BS}}}+\sqrt{\epsilon}V_{01}+\sqrt{\delta}V_{10} , that is
\begin{equation} \begin{split} \ddot{V}^{\epsilon, \delta}(t, x, m, z) = & \, V_{ \mathit{\text{BS}}}(t, x, m, z)\\ &-m\int_{t}^{T}\int_{0}^{\infty}\exp{\left(\frac{1}{2}\left(\ln{\left(\frac{x}{m}\right)}-w\right)\right)} \left(\mathcal{H}_{ \mathit{\text{01}}}^{\epsilon, H_1} +2\left(\tau -t \right)\mathcal{H}_{ \mathit{\text{10}}}^{\delta, H_2}\right)\\ &\qquad\qquad\qquad \times V_{ \mathit{\text{BS}}}\left(T+t-\tau, e^{w}, 1, z\right)G\left(\tau, w, \frac{x}{m}, z \right)dw d \tau, \end{split} \end{equation} | (6.13) |
where V_{ \mathit{\text{BS}}}(t, x, m, z) is defined as
\begin{equation} \begin{split} V_{ \mathit{\text{BS}}}(t, x, m, z) = &\, P_{ \mathit{\text{BS}}}(t, x, z; m)\\ &+x\, \bar{\sigma}_{f}\sqrt{T-t}\, \, \biggl( \varphi \, \Bigl(d_1(x, z; m), 0, 1\Bigr)-d_1(x, z; m) \, \mathcal{N}\, \Bigl(-d_1(x, z; m)\Bigr) \, \biggr), \end{split} \end{equation} | (6.14) |
\mathcal{H}^{\epsilon, H_1}_{ \mathit{\text{01}}} and \mathcal{H}^{\delta, H_2}_{ \mathit{\text{10}}} are the differential operators defined by (6.5), and G is the function defined in Lemma 6.2. Here, P_{ \mathit{\text{BS}}}(t, x, z; \varpi) , \varphi\left(\varpi, \mu, \sigma\right) , and d_1(x, z; \varpi) are defined in (6.6) and (5.2), respectively.
Proof. Above all, as the solution V_{00} of the first PDE problem in (6.11) is exactly the price of the floating strike lookback call option under the Black-Scholes model whose volatility is \bar{\sigma}_f(z) , it is the same as V_{ \text{ BS}} given by (6.14) which can be found in, for instance, Wilmott [34].
To derive approximate closed-form formulas for V^{\epsilon, \delta} , we let \ddot{V}_{01}: = \sqrt{\epsilon}V_{01} and \ddot{V}_{10}: = \sqrt{\delta}V_{10} , and apply the reduction method of the dimension used in Shreve [31] to the PDE problems in (6.11). Letting w: = \frac{x}{m} and \ddot{V}_{ij}(t, x, m, z) = m\ddot{V}_{ij}\left(t, \frac{x}{m}, 1, z\right) = :mW_{ij}(t, w, z) , (i, j) = \{(0, 1), (1, 0)\} , (6.11) becomes
\begin{equation} \begin{split} \mathcal{A}_{ \text{ BS}}[w] W_{ \text { BS}}(t, w, z) & = 0, \\ W_{ \text{ BS}}(T, w, z)& = w-1, \\ W_{ \text { BS}}(t, 1, z)& = \partial_w W_{ \text { BS}}(t, 1 , z), \\ & \\ \mathcal{A}_{ \text{ BS}}[w] W_{01}(t, w, z) & = \, \mathcal{H}_{ \text{ 01}}^{\epsilon, H_1}[w] W_{ \text { BS}}(t, w, z), \\ W_{01}(T, w, z)& = 0, \\ W_{01}(t, 1, z)& = \partial_w W_{01}(t, 1 , z), \\ & \\ \mathcal{A}_{ \text{ BS}}[w] W_{10}(t, w, z) & = \, 2(T-t)\mathcal{H}_{ \text{ 10}}^{\delta, H_2}[w] W_{ \text { BS}}(t, w, z), \\ W_{10}(T, w, z)& = 0, \\ W_{10}(t, 1, z)& = \partial_w W_{10}(t, 1 , z), \end{split} \end{equation} | (6.15) |
where
\begin{equation*} \begin{split} \label{rewrtten_operator} \mathcal{D}_{n}[w] & = w^{n}\partial_{w^n}, \quad n = 1, 2, \\ \mathcal{A}_{ \text{ BS}}[w]& = \, \partial_t + \frac{1}{2}\, \bar{\sigma}_f^2(z)\, \mathcal{D}_{2}[w], \\ \mathcal{H}_{ \text{ 01}}^{\epsilon, H_1}[w]& = \, A^{\epsilon, H_1}_1(z) \left(\mathcal{D}^2_{1}[w] - \mathcal{D}_{1}[w] \right) - A^{\epsilon, H_1}_2(z) \left(-\mathcal{D}_{1}^3[w] + \mathcal{D}_{1}^2[w]\right), \\ \mathcal{H}_{ \text{ 10}}^{\delta, H_2}[w]& = \, B^{\delta, H_2}_1(z) \left(-\mathcal{D}_{1}^3[w] + \mathcal{D}_{1}^2[w]\right) - B^{\delta, H_2}_2(z) \left(\mathcal{D}_{1}^2[w] - \mathcal{D}_{1}[w]\right). \end{split} \end{equation*} |
Applying Lemma 6.2 to the PDE problems (6.15) for W_{10} and W_{01} directly, one can obtain the solutions corresponding to the formula (6.13).
In this paper, we have introduced a semimartingale approximation of fractional stochastic volatility in terms of two approximate fractional Brownian motions corresponding to two characteristic time scales. Based on the semimartingale property, we make use of the replicating portfolio method to obtain the parabolic PDE problems for European vanilla, barrier, and lookback options, and then solve those problems explicitly and derive approximate closed-form formulas for the option prices. The mixture of the Hurst parameters and the multiple time scales of volatility can unify effectively the previously known separate results about the time-to-maturity dependence of the blow-up or flattening behavior of the skews of the implied volatility. So, knowing that stochastic volatility models driven by fractional Brownian motions can generate better fits to implied volatility surfaces, our uniform approximation result can contribute to the situation that the volatility parameters including the Hurst exponent should be calibrated over a wide range of time-to-maturities.
Min-Ku Lee: Resources, software, data curation, methodology, validation, visualization, and writing-original draft; Jeong-Hoon Kim: Conceptualization, formal analysis, writing-original draft, writing-review & editing, supervision, project administration, and funding acquisition. All authors have read and agreed to the published version of the manuscript.
The authors declare they have not used Artificial Intelligence (AI) tools in the creation of this article.
Datasets analyzed during the current study are available through the sites http://www.investing.com and http://www.barchart.com. Code for parameter calibration is provided as part of the replication package and is available at https://sites.google.com/view/two-scale-sv-fbms.
The authors deeply thank the four anonymous reviewers for their valuable comments and suggestions to improve this paper.
The author J.-H. Kim gratefully acknowledges the financial support from the National Research Foundation of Korea grant NRF2021R1A2C1004080.
The authors certify that there is no actual or potential conflict of interest in relation to this article.
In this section, we obtain the second-order terms P_{02} , P_{11} , and P_{20} in the asymptotic series expansion (3.3). The corresponding PDE problems have the terminal conditions \langle P_{02}(T, x, \cdot, z) \rangle = 0 , P_{11}(T, x, y, z) = 0 , and P_{20}(T, x, y, z) = 0 , respectively. Here, the averaged terminal condition \langle P_{02}(T, x, \cdot, z) \rangle = 0 and the condition
\begin{equation} \begin{split} \langle \psi(\cdot, z) \rangle = 0 \end{split} \end{equation} | (A.1) |
are imposed based on the terminal layer analysis given by Fouque et al. [13].
We first obtain the following lemma for the terms P_{02} , P_{12} , and P_{03} in (3.3).
Lemma A.1. The second-order terms P_{02} , P_{12} , and P_{03} in (3.3) can be expressed as
\begin{equation} \begin{split} P_{02}(t, x, y, z)& = -\frac{1}{2}\psi(y, z)\mathcal{D}_2 P_{ \mathit{\text{BS}}}+F_{02}(t, x, z), \\ P_{12}(t, x, y, z)& = -\frac{1}{2}\psi(y, z)\mathcal{D}_2 P_{10} - \rho_{xz} d(z) \gamma^{H_2 -\frac{1}{2}}\eta(x, z)\mathcal{D}_{1}\partial_{z}P_{ \mathit{\text{BS}}}+F_{12}(t, x, z), \\ P_{03}(t, x, y, z)& = \frac{1}{2}(H_2-\frac{1}{2})\phi_1 \xi \mathcal{D}_2P_{ \mathit{\text{BS}}}+\frac{1}{2}\rho_{xy}\gamma^{H_1-\frac{1}{2}}\zeta \mathcal{D}_1\mathcal{D}_2P_{ \mathit{\text{BS}}}-\frac{1}{2}\psi \mathcal{D}_2 P_{01}+F_{03}(t, x, z), \end{split} \end{equation} | (A.2) |
for some functions F_{02} , F_{12} , and F_{03} independent of the variable y , where \eta , \xi , and \zeta are the solutions of
\begin{equation} \begin{split} \mathcal{A}_0 \eta(y, z) & = f(y, z)-\langle f(\cdot, z) \rangle, \\ \mathcal{A}_0 \xi(y, z) & = \beta(y, z) \partial_{y} \psi(y, z)-\langle \beta(\cdot, z) \partial_{y} \psi(\cdot, z) \rangle, \\ \mathcal{A}_0 \zeta(y, z) & = f(y, z) \beta(y, z) \partial_{y} \psi(y, z)-\langle f(\cdot, z) \beta(\cdot, z) \partial_{y} \psi(\cdot, z) \rangle, \end{split} \end{equation} | (A.3) |
respectively.
Proof. Putting (3.3) into (3.1), Lemmas 3.1 and 3.2 draw forth Poisson equations given by
\begin{equation} \begin{split} \mathcal{A}_0P_{02}& = -\left(\mathcal{A}_{2}-\langle \mathcal{A}_{2}\rangle \right)P_{ \text{ BS}}, \\ \mathcal{A}_0P_{12}& = -\left( \left( \mathcal{A}_{2}- \langle \mathcal{A}_2 \rangle\right)P_{10}+\left( \mathcal{A}_{4}-\langle \mathcal{A}_{4}\rangle \right)P_{ \text{ BS}} \right), \\ \mathcal{A}_0P_{03}& = -\left( \left( \mathcal{A}_{1}P_{02}-\langle \mathcal{A}_{1}P_{02}\rangle \right) +\left( \mathcal{A}_{2}-\langle \mathcal{A}_{2}\rangle\right)P_{01}\right). \end{split} \end{equation} | (A.4) |
Using the solutions \eta , \xi , and \zeta of (A.3), we can derive the solutions P_{02} , P_{12} , and P_{30} in the form of (A.2) for some y -independent functions F_{02} , F_{12} , and F_{03} .
Next, we obtain PDEs for F_{02} (and so P_{02} ), P_{11} , and P_{20} .
Proposition A.1. The second-order solutions F_{02} in (A.2), P_{11} , and P_{20} are independent on the variable y and satisfy the following PDEs
\begin{equation} \begin{split} \mathcal{A}_{ \mathit{\text{BS}}} F_{02}& = - \langle \mathcal{A}_{1}P_{03}\rangle +\frac{1}{4} \langle \psi f^2\rangle \mathcal{D}^2_2P_{ \mathit{\text{BS}}}, \\ \mathcal{A}_{ \mathit{\text{BS}}} P_{11}& = -\left( \langle \mathcal{A}_{1}P_{12}\rangle +\langle \mathcal{A}_{3}P_{02}\rangle+\langle\mathcal{A}_{4}\rangle P_{01}\right), \\ \mathcal{A}_{ \mathit{\text{BS}}} P_{20}& = -\left(\langle \mathcal{A}_{4}\rangle P_{10}+\mathcal{A}_5 P_{ \mathit{\text{BS}}}\right) \end{split} \end{equation} | (A.5) |
with the boundary conditions F_{02}(T, x, z) = P_{11}(T, x, z) = P_{20}(T, x, z) = 0 .
Proof. First of all, similarly with the proof of Lemma A.1, we can obtain the PDEs given by
\begin{equation*} \begin{split} \label{Poi_eqn} \mathcal{A}_0P_{11} = \mathcal{A}_0P_{20} = \mathcal{A}_0P_{21} = 0 \end{split} \end{equation*} |
which yields that P_{11} , P_{20} , and P_{21} are independent on the variable y . Additionally, we can get the PDE given by
\begin{equation} \begin{split} & \mathcal{A}_{0}P_{04}+\mathcal{A}_{1}P_{03}+\mathcal{A}_{2}P_{02} = 0, \\ & \mathcal{A}_{0}P_{13}+\mathcal{A}_{1}P_{12}+\mathcal{A}_{2}P_{11}+\mathcal{A}_{3}P_{02}+\mathcal{A}_{4}P_{01} = 0, \\ & \mathcal{A}_{0}P_{22}+\mathcal{A}_{1}P_{21}+\mathcal{A}_{2}P_{20}+\mathcal{A}_{3}P_{11}+\mathcal{A}_{4}P_{10}+\mathcal{A}_{5}P_{ \text{ BS}} = 0. \end{split} \end{equation} | (A.6) |
Applying Lemma 3.1 and the y -independence of P_{11} , P_{20} , and P_{21} into (A.6), we can have the PDEs
\begin{equation} \begin{split} \langle \mathcal{A}_{2}P_{02}\rangle = -\langle \mathcal{A}_{1}P_{03} \rangle, \end{split} \end{equation} | (A.7) |
and
\begin{equation} \begin{split} & \langle\mathcal{A}_{1}P_{12}\rangle+ \mathcal{A}_{ \text{ BS}} P_{11}+\langle\mathcal{A}_{3}P_{02}\rangle+\langle\mathcal{A}_{4}\rangle P_{01} = 0, \\ & \mathcal{A}_{ \text{ BS}} P_{20}+\langle\mathcal{A}_{4}\rangle P_{10}+\mathcal{A}_{5}P_{ \text{ BS}} = 0. \end{split} \end{equation} | (A.8) |
Thus, we can obtain the PDEs for P_{11} and P_{02} in (A.5).
On the other hand, putting P_{02} in (A.2) into (A.7), we can obtain
\begin{equation*} \begin{split} \label{PDE_F02} \langle \mathcal{A}_{2}P_{02}\rangle& = -\frac{1}{2}\langle \psi(\cdot, z) \mathcal{L}_{2} \rangle \mathcal{D}_2P_{00}(t, x, z)+ \mathcal{A}_{ \text{ BS}}F_{02}(t, x, z)\\ & = -\frac{1}{2} \langle \psi(\cdot, z) \left( \mathcal{A}_{2} -\langle \mathcal{A}_{2} \rangle \right) \rangle \mathcal{D}_2P_{00}(t, x, z)+ \mathcal{A}_{ \text{ BS}}F_{02}(t, x, z)\\ & = -\frac{1}{2} \langle \psi(\cdot, z) \left( f^2(y, z) -\langle f^2(\cdot, z) \rangle \right)\mathcal{D}_{2} \rangle \mathcal{D}_2P_{00}(t, x, z)+ \mathcal{A}_{ \text{ BS}}F_{02}(t, x, z)\\ & = -\frac{1}{2} \left( \langle \psi(\cdot, z) f^2(\cdot, z)\rangle -\langle \psi(\cdot, z)\rangle \langle f^2(\cdot, z) \rangle \right)\mathcal{D}^2_{2} P_{00}(t, x, z)+ \mathcal{A}_{ \text{ BS}}F_{02}(t, x, z)\\ & = -\frac{1}{2} \langle \psi(\cdot, z) f^2(\cdot, z)\rangle\mathcal{D}^2_{2} P_{00}(t, x, z)+ \mathcal{A}_{ \text{ BS}}F_{02}(t, x, z)\\ & = -\langle\mathcal{A}_1P_{03}\rangle, \end{split} \end{equation*} |
where the assumption (A.1) has been used. Thus the first equation for F_{02} in (A.5) has been derived.
Finally, the second-order approximation \dddot{P}(t, x, y, z): = P_{ \text{ BS}}+\sqrt{\delta}P_{10}+\sqrt{\epsilon}P_{01}+\delta P_{02}+\sqrt{\delta\epsilon}P_{11}+\epsilon P_{20} and its accuracy are obtained as follows by solving the PDEs (A.5) in Proposition A.1.
Proposition A.2. Under the dynamics of (2.3) of the underlying asset price, the option price P^{\epsilon, \delta} is approximated by \dddot{P}(t, x, y, z): = P_{ \mathit{\text{BS}}}+\sqrt{\delta}P_{10}+\sqrt{\epsilon}P_{01}+\delta P_{02}+\sqrt{\delta\epsilon}P_{11}+\epsilon P_{20} , where the first approximation part \ddot{P}(t, x, y, z): = P_{ \mathit{\text{BS}}}+\sqrt{\delta}P_{10}+\sqrt{\epsilon}P_{01} is given by Proposition 3.1 and P_{02} , P_{11} , and P_{20} are given by
\begin{equation} \begin{split} P_{02}(t, x, y, z)& = -\frac{1}{2}\psi(y, z)\mathcal{D}_2 P_{ \mathit{\text{BS}}}+ (T-t)\sum\limits_{k = 1}^{4}A_{02}^{k}\mathcal{D}^{k}_{1}P_{ \mathit{\text{BS}}}+(T-t)^2\sum\limits_{k = 2}^{6}B_{02}^{k}\mathcal{D}^{k}_{1} P_{ \mathit{\text{BS}}}, \\ P_{11}(t, x, z)& = (T-t)^2\sum\limits_{k = 1}^{k}B_{11}^{k}\mathcal{D}^{k}_{1}P_{ \mathit{\text{BS}}}+(T-t)^3\sum\limits_{k = 2}^{6}C^{k}_{11}\mathcal{D}^{k}_{1} P_{ \mathit{\text{BS}}}, \\ P_{20}(t, x, z)& = (T-t)^2\sum\limits_{k = 1}^{2}B_{20}^{k}\mathcal{D}^{k}_{1}P_{ \mathit{\text{BS}}}+(T-t)^3\sum\limits_{k = 1}^{4}C^{k}_{20}\mathcal{D}^{k}_{1}+(T-t)^4\sum\limits_{k = 1}^{4}D^{k}_{20}\mathcal{D}^{k}_{1} P_{ \mathit{\text{BS}}}, \end{split} \end{equation} | (A.9) |
where A^{k}_{ij} , B^{k}_{ij} , C^{k}_{ij} , and D^{k}_{ij} are set aside in Appendix B for comfortable readability. Moreover, the approximation has the accuracy
\begin{equation*} \begin{split} \Vert P(t, x, y, z)-\dddot{P}(t, x, y, z) \Vert = \mathcal{O}\left( \epsilon^{1+l/2}+\epsilon\sqrt{\delta}+\delta\sqrt{\epsilon}+\delta^{3/2} \right) \end{split} \end{equation*} |
for any l < 1 .
Proof. First of all, we can rewrite the solutions P_{01} and P_{10} in Proposition 3.1 as
\begin{equation} \begin{split} P_{01}&: = -(T-t)\mathcal{B}[z]P_{ \text{ BS}}, \\ P_{10}&: = -\frac{1}{2}(T-t)^2 \bar{\sigma}_f'(z) \bar{\sigma}_f(z)\mathcal{C}[z]P_{ \text{ BS}}, \end{split} \end{equation} | (A.10) |
where \mathcal{B} and \mathcal{C} are the operators defined by
\begin{equation*} \begin{split} \mathcal{B}[z]& = A^{H_1}_1(z) \mathcal{D}_{1}^3 + \left(A^{H_1}_1(z)- A^{H_1}_2(z) \right)\mathcal{D}_{1}^2- A^{H_1}_1(z) \mathcal{D}_1, \\ \mathcal{C}[z]& = \left(-B^{H_2}_1(z)\right)\mathcal{D}_{1}^3 +\left(B^{H_2}_1(z)-B^{H_2}_2(z) \right) \mathcal{D}_{1}^2 +B^{H_2}_2(z) \mathcal{D}_{1}, \end{split} \end{equation*} |
respectively. Putting the solutions (A.10) for P_{01} and P_{10} and (A.2) for P_{02} , P_{12} , and P_{03} into (A.5), we have the following PDEs
\begin{equation} \begin{split} \mathcal{A}_{ \text{ BS}}F_{02}& = \left(\mathcal{E}[z] -(T-t)\mathcal{F}[z]\mathcal{B}[z]\right)P_{ \text{ BS}}, \\ \mathcal{A}_{ \text{ BS}}P_{11}& = \left(\bar{\sigma}_f'\mathcal{G}[z]\frac{\partial}{\partial \sigma}-(T-t)\mathcal{H}[z]\left( \mathcal{B}'[z] +\bar{\sigma}_f'\mathcal{B}[z]\frac{\partial}{\partial \sigma}\right) -\frac{1}{2}(T-t)^2 \bar{\sigma}_f' \bar{\sigma}_f\mathcal{M}[z]\mathcal{C}[z]\right)P_{ \text{ BS}}, \\ \mathcal{A}_{ \text{ BS}}P_{20}& = \left(E^{H_2}_1\left(\bar{\sigma}_f"\frac{\partial}{\partial \sigma}+\left(\bar{\sigma}_f'\right)^2 \frac{\partial^2}{\partial \sigma^2} \right)+E^{H_2}_2\bar{\sigma}_f'\frac{\partial}{\partial \sigma}-(T-t)^2\mathcal{N}[z]\left(\mathcal{C}'[z]+\bar{\sigma}_f\mathcal{C}[z]\frac{\partial}{\partial \sigma}\right)\right)P_{ \text{ BS}}, \end{split} \end{equation} | (A.11) |
where \mathcal{G} , \mathcal{H} , \mathcal{M} , and \mathcal{N} are the differential operators defined by
\begin{equation*} \begin{split} \mathcal{G}[z]& = \left(D^{H_1, H_2}_{1}(z)+D^{H_1, H_2}_2(z)\right)\mathcal{D}_{1}^2+\left( D^{H_1, H_2}_1(z)+D^{H_1, H_2}_3(z)\right) \mathcal{D}_1, \\ \mathcal{H}[z]& = D^{H_2}_1(z)\mathcal{D}_{1} +D^{H_2}_2(z), \\ \mathcal{M}[z]& = D^{H_1}_1(z)\mathcal{D}_{1}^3 +\left(-D^{H_1}_1(z)+D^{H_1}_2(z) \right) \mathcal{D}_{1}^2 +\left(-D^{H_1}_2(z) \right) \mathcal{D}_{1}, \\ \mathcal{N}[z]& = E^{H_2}_3\mathcal{D}_{1}(z)+E^{H_2}_4(z). \end{split} \end{equation*} |
Here, D^{H_1, H_2}_{i} , D^{H_1}_{i} , D^{H_2}_{i} , and E^{H_2}_{i} are defined in Appendix B. By applying the following results (motivational equations) in Fouque et al. [13] to the PDEs (A.11), we solve the PDEs (A.11) to obtain the solutions (A.9).
\begin{equation} \begin{split} & \mathcal{A}_{ \text{ BS}}\left(\frac{(T-t)^{n+1}}{n+2}\frac{\partial}{\partial \sigma}P_{ \text{ BS}}\right) = -(T-t)^n\frac{\partial}{\partial \sigma}P_{ \text{ BS}}, \\ &\mathcal{A}_{ \text{ BS}}\left(\frac{(T-t)^{n+1}}{n+3}\left(\frac{\partial^2}{\partial \sigma^2}+\frac{1}{\bar{\sigma}_f(n+2)}\frac{\partial}{\partial \sigma}\right)P_{ \text{ BS}}\right) = -(T-t)^n\frac{\partial^2}{\partial \sigma^2}P_{ \text{ BS}}. \end{split} \end{equation} | (A.12) |
The proof of the error estimate should be similar to the proof in Fouque et al. [13] and so we omit the proof.
\begin{equation*} \begin{split} \label{A02} A^4_{02}&: = -\left(C_1^{H_1}+C_4^{H_1} \right), \qquad\qquad\qquad\qquad\qquad\qquad\qquad\qquad\qquad\qquad\qquad\qquad\qquad\qquad\\ A^3_{02}&: = C_1^{H_1}-C_2^{H_1}+2C_4^{H_1}, \\ A^2_{02}&: = C_2^{H_1}-C_3^{H_1}-C_4^{H_1}, \\ A^1_{02}&: = C_3^{H_1} \end{split} \end{equation*} |
\begin{equation*} \begin{split} \label{B02} B^6_{02}&: = \frac{1}{2} C^{H_1}_5 A_2^{H_1}, \\ B^5_{02}&: = \frac{1}{2}\left(C^{H_1}_5\left(A^{H_1}_1-2A^{H_1}_2\right)+C^{H_1}_6A^{H_1}_2 \right), \\ B^4_{02}&: = -\frac{1}{2}\left(C^{H_1}_5\left(2A^{H_1}_1-A^{H_1}_2\right)+C^{H_1}_6(A^{H_1}_1-2A^{H_1}_2 \right), \qquad\qquad\qquad\qquad\qquad\qquad\qquad\qquad\\ B^3_{02}&: = \frac{1}{2}\left(C^{H_1}_5A^{H_1}_1-C^{H_1}_6\left(2A^{H_1}_1-A^{H_1}_2\right) \right), \\ B^2_{02}&: = \frac{1}{2}C^{H_1}_6A^{H_1}_1, \end{split} \end{equation*} |
\begin{equation*} \begin{split} \;\;\;\;\;\;\label{B11} B^4_{11}&: = -\frac{1}{2}\left( \bar{\sigma}_f' \bar{\sigma}_f \left(D^{H_1, H_2}_1+D^{H_1, H_2}_2\right)-D^{H_2}_1 \frac{\partial}{\partial z} A^{H_1}_1 \right), \\ \;\;\;\;\;\;B^3_{11}&: = \frac{1}{2}\left( \bar{\sigma}_f' \bar{\sigma}_f\left(2D^{H_1, H_2}_1+D^{H_1, H_2}_2-D^{H_1, H_2}_3\right)+\left[D^{H_2}_1 \frac{\partial}{\partial z}\left( A^{H_1}_1-A^{H_1}_2 \right) +D^{H_2}_2\frac{\partial}{\partial z} A^{H_1}_2 \right] \right), \\ \;\;\;\;\;\;B^2_{11}&: = -\frac{1}{2}\left(\bar{\sigma}_f' \bar{\sigma}_f \left(D^{H_1, H_2}_1-D^{H_1, H_2}_3\right)+\left[D^{H_2}_1\frac{\partial}{\partial z}A^{H_1}_1-D^{H_2}_2\frac{\partial}{\partial z}\left(A^{H_1}_1-A^{H_1}_2\right)\right] \right)\\ \;\;\;\;\;\;B^1_{11}&: = -\frac{1}{2}D^{H_2}_2 \frac{\partial}{\partial z}A^{H_1}_1, \\ \end{split} \end{equation*} |
\begin{equation*} \begin{split}\;\;\;\;\;\; \label{C11} C^6_{11}&: = \frac{1}{3} \bar{\sigma}_f' \bar{\sigma}_f \left(D^{H_2}_1A^{H_1}_2-\frac{1}{2}D^{H_1}_1B^{H_2}_2\right), \\ \;\;\;\;\;\; C^5_{11}&: = \frac{1}{3} \bar{\sigma}_f' \bar{\sigma}_f \left(D^{H_2}_1\left(A^{H_1}_1 -2A^{H_1}_2\right)+D^{H_2}_2A^{H_1}_2+\frac{1}{2}\left[ D^{H_1}_1 \left(2B^{H_2}_1-B^{H_2}_2\right)-D^{H_1}_2B^{H_2}_1\right]\right), \\ \;\;\;\;\;\;C^4_{11}&: = -\frac{1}{3} \bar{\sigma}_f' \bar{\sigma}_f \left(D^{H_2}_1\left(2A^{H_1}_1-A^{H_1}_2\right)-D^{H_2}_2 \left( A^{H_1}_1-2A^{H_1}_2\right) \right.\\ \;\;\;\;\;\;&\qquad \left.+\frac{1}{2}\left[ D^{H_1}_1\left(B^{H_2}_1-2B^{H_2}_2\right) -D^{H_1}_{2}\left( 2B^{H_2}_1 -B^{H_2}_2\right) \right]\right), \\ \;\;\;\;\;\;C^3_{11}&: = \frac{1}{3} \bar{\sigma}_f' \bar{\sigma}_f \left(D^{H_2}_1A^{H_1}_2 -D^{H_2}_2\left(2A^{H_1}_1-A^{H_1}_2\right)-\frac{1}{2}\left[ D^{H_1}_1B^{H_2}_2+D^{H_1}_2\left(B^{H_2}_1-2B^{H_2}_2\right)\right]\right), \\ \;\;\;\;\;\;C^2_{11}&: = \frac{1}{3} \bar{\sigma}_f' \bar{\sigma}_f \left(D^{H_2}_2A^{H_1}_1 -\frac{1}{2} D^{H_1}_2B^{H_2}_2\right), \end{split} \end{equation*} |
\begin{equation*} \begin{split} \label{B20} B^2_{20}&: = -\frac{1}{2}\left( E^{H_2}_1\left( \bar{\sigma}_f" \bar{\sigma}_f +\left( \bar{\sigma}_f'\right)^2 \right)+E^{H_2}_2\bar{\sigma}_f' \bar{\sigma}_f\right) , \qquad\qquad\qquad\qquad\qquad\qquad\qquad\qquad\qquad\qquad\qquad\qquad\\ B^1_{20}&: = \frac{1}{2}\left( E^{H_2}_1\left( \bar{\sigma}_f" \bar{\sigma}_f +\left( \bar{\sigma}_f'\right)^2 \right)+E^{H_2}_2\bar{\sigma}_f' \bar{\sigma}_f\right), \end{split} \end{equation*} |
\begin{equation*} \begin{split} \;\;\;\;\;\;\label{C20} C^4_{20}&: = -\frac{1}{3}\left(\left( \bar{\sigma}_f ' \right)^2 \bar{\sigma}_f^2 E^{H_2}_1+ \frac{1}{2} \left( \bar{\sigma}_f" \bar{\sigma}_f +\left(\bar{\sigma}_f'\right)^2 \right)E^{H_2}_3B^{H_2}_1+\frac{1}{2}\bar{\sigma}_f' \bar{\sigma}_f E^{H_2}_{3}\frac{\partial}{\partial z}\left( \bar{\sigma}_f' \bar{\sigma}_f B^{H_2}_1\right)\right), \\ \;\;\;\;\;\;C^3_{20}&: = \frac{1}{3}\left(2\left( \bar{\sigma}_f'\right)^2 \bar{\sigma}_f^2 E^{H_2}_1 +\frac{1}{2}\left( \bar{\sigma}_f" \bar{\sigma}_f +\left(\bar{\sigma}_f'\right)^2 \right)\left( E^{H_2}_3\left(B^{H_2}_1-B^{H_2}_2\right)-E^{H_2}_4B^{H_2}_1\right) \right.\\ \;\;\;\;\;\;&\left.\qquad+\frac{1}{2}\bar{\sigma}_f'\bar{\sigma}_f \left[E^{H_2}_3 \frac{\partial}{\partial z}\left(B^{H_2}_1-B^{H_2}_2 \right) -E^{H_2}_4\frac{\partial}{\partial z}\left( B^{H_2}_1 \right)\right]\right), \qquad\\ \;\;\;\;\;\;C^2_{20}&: = -\frac{1}{3}\left(\left( \bar{\sigma}_f'\right)^2 \bar{\sigma}_f^2 E^{H_2}_1-\frac{1}{2} \left( \bar{\sigma}_f" \bar{\sigma}_f +\left(\bar{\sigma}_f'\right)^2 \right)\left( E^{H_2}_3 B^{H_2}_2+E^{H_2}_4\left( B^{H_2}_1-B^{H_2}_2 \right)\right)\right.\\ \;\;\;\;\;\;&\qquad\left.- \frac{1}{2} \bar{\sigma}_f' \bar{\sigma}_f \left[E^{H_2}_3 \frac{\partial}{\partial z}\left( B^{H_2}_2\right)+E^{H_2}_4 \frac{\partial}{\partial z}\left( B^{H_2}_1- B^{H_2}_2\right)\right]\right), \\ \;\;\;\;\;\;C^1_{20}&: = \frac{1}{6}\left(\left( \bar{\sigma}_f" \bar{\sigma}_f+\left(\bar{\sigma}_f )^2\right)\right) E^{H_2}_4B^{H_2}_2+\bar{\sigma}_f '\bar{\sigma}_f E^{H_2}_4 \frac{\partial}{\partial z}B^{H_2}_2\right), \end{split} \end{equation*} |
\begin{equation*} \begin{split} \label{D20} D^6_{20}&: = -\frac{1}{8}\left( \bar{\sigma}_f ' \right)^2 \bar{\sigma}_f^2 E^{H_2}_3 B^{H_2}_1, \\ D^5_{20}&: = \frac{1}{8} \left( \bar{\sigma}_f'\right)^2 \bar{\sigma}_f^2 \left(E^{H_2}_3\left(2B^{H_2}_1-B^{H_2}_2\right)-E^{H_2}_{4}B^{H_2}_1\right), \\ D^4_{20}&: = -\frac{1}{8} \left( \bar{\sigma}_f'\right)^2 \bar{\sigma}_f^2 \left(E^{H_2}_3\left( B^{H_2}_1-2B^{H_2}_2\right)-E^{H_2}_4\left(2B^{H_2}_1-B^{H_2}_2\right)\right), \qquad\qquad\qquad\qquad\qquad\qquad\qquad\qquad\\ D^3_{20}&: = -\frac{1}{8} \left( \bar{\sigma}_f'\right)^2 \bar{\sigma}_f^2 \left(E^{H_2}_3B^{H_2}_2+E^{H_2}_4\left( B^{H_2}_1-2B^{H_2}_2\right)\right), \\ D^2_{20}&: = -\frac{1}{8} \left( \bar{\sigma}_f'\right)^2 \bar{\sigma}_f^2 E^{H_2}_4 B^{H_2}_2, \end{split} \end{equation*} |
\begin{equation*} \begin{split} \label{P02_CST} &C^{H_1}_{1}(z): = -\frac{1}{2}\rho^{2}_{xy}\gamma^{2H_1-1} \langle f(\cdot, z) \beta(\cdot) \frac{\partial \zeta}{\partial y}(\cdot, z)\rangle , \\ &C^{H_1}_{2}(z): = -\frac{1}{2}\rho_{xy}\gamma^{H_1-\frac{1}{2}}\left(H_1-\frac{1}{2}\right) \phi_1\left( \langle \beta(\cdot) \frac{\partial \zeta}{\partial y}(\cdot, z)\rangle + \langle f(\cdot, z) \beta(\cdot) \frac{\partial \xi}{\partial y}(\cdot, z)\rangle \right), \qquad\qquad\qquad\qquad\qquad\qquad\\ &C^{H_1}_{3}(z): = -\frac{1}{2}\left( H_1-\frac{1}{2}\right)^2 \phi_1^2 \langle \beta(\cdot) \frac{\partial \xi}{\partial y}(\cdot, z)\rangle , \\ &C^{H_1}_{4}(z): = \frac{1}{4} \langle \psi(\cdot, z) f^2(\cdot, z)\rangle , \\ &C^{H_1}_{5}(z): = \frac{1}{2}\rho_{xy}\gamma^{H_1-\frac{1}{2}} \langle f(\cdot, z) \beta(\cdot) \frac{\partial \psi}{\partial y}(\cdot, z)\rangle, \\ &C^{H_1}_{6}(z): = \frac{1}{2}\left(H_1-\frac{1}{2}\right)\phi_1 \langle \beta(\cdot) \frac{\partial \psi}{\partial y}(\cdot, z)\rangle, \end{split} \end{equation*} |
\begin{equation*} \begin{split} \;\;\;\label{P11_CST} &D^{H_1, H_2}_{1}(z): = \frac{1}{2}\rho_{yz}h(z)\gamma^{H_1+H_2-1} \langle f(\cdot, z) \beta(\cdot) \frac{\partial \psi}{\partial y}(\cdot, z)\rangle , \\ \;\;\;&D^{H_1, H_2}_{2}(z): = \rho_{xy}\rho_{xz}h(z)\gamma^{H_1+H_2-1} \langle f(\cdot, z) \beta(\cdot) \frac{\partial \eta}{\partial y}(\cdot, z)\rangle, \\ \;\;\;&D^{H_1, H_2}_{3}(z): = \rho_{xz}h(z)\gamma^{H_2-\frac{1}{2}}\left(H_1-\frac{1}{2}\right) \phi_1 \langle \beta(\cdot) \frac{\partial \eta}{\partial y}(\cdot, z)\rangle, \qquad\qquad\qquad\qquad\qquad\qquad\qquad\qquad\qquad\\ \;\;\;&D^{H_2}_{1}(z): = -\rho_{xz}h(z)\gamma^{H_2-\frac{1}{2}} \langle f(\cdot, z)\rangle, \\ \;\;\;&D^{H_2}_{2}(z): = -h(z)\left(H_1-\frac{1}{2}\right)\phi_2, \\ \;\;\;&D^{H_1}_{1}(z): = \frac{1}{2}\rho_{xy}\gamma^{H_1-\frac{1}{2}} \langle f(\cdot, z) \beta(\cdot) \frac{\partial \psi}{\partial y}(\cdot, z)\rangle, \\ \;\;\;&D^{H_1}_{2}(z): = \frac{1}{2}\left( H_1-\frac{1}{2}\right)\phi_1 \langle \beta(\cdot) \frac{\partial \psi}{\partial y}(\cdot, z)\rangle, \end{split} \end{equation*} |
\begin{equation*} \begin{split} \;\;\;\label{P20_CST} E^{H_2}_{1}(z)&: = -\frac{1}{2}h^2(z)\gamma^{2H_2-1}, \\ \;\;\;E^{H_2}_{2}(z)&: = -g(z), \\ \;\;\;E^{H_2}_{3}(z)&: = -\rho_{xz}h(z)\gamma^{H_2-\frac{1}{2}}\langle f(\cdot, z) \rangle, \qquad\qquad\qquad\qquad\qquad\qquad\qquad\qquad\qquad\qquad\qquad\qquad\qquad\\ \;\;\;E^{H_2}_{4}(z)&: = -h(z)\left(H_2-\frac{1}{2}\right)\phi_2. \end{split} \end{equation*} |
[1] |
Gołębiewska E, Kalinowska M, Yildiz G (2022) Sustainable use of apple pomace (AP) in different industrial sectors. Materials 15: 1788. https://doi.org/10.3390/ma15051788 doi: 10.3390/ma15051788
![]() |
[2] | Priyadarshini A, Priyadarshini A (2018) Chapter 2—Market dimensions of the fruit juice Industry. In: Rajauria G, Tiwari BK (Eds.), Fruit Juices, Academic Press, San Diego, 15–32. https://doi.org/10.1016/B978-0-12-802230-6.00002-3 |
[3] |
Saadat S, Pandya H, Dey A, et al. (2022) Food forensics: Techniques for authenticity determination of food products. Forensic Sci Int 333: 11243. https://doi.org/10.1016/j.forsciint.2022.111243 doi: 10.1016/j.forsciint.2022.111243
![]() |
[4] |
Fanelli V, Mascio I, Miazzi MM, et al. (2021) Molecular approaches to agri-food traceability and authentication: An updated review. Foods 10: 1644. https://doi.org/10.3390/ foods10071644 doi: 10.3390/foods10071644
![]() |
[5] |
Catalano V, Moreno-Sanz P, Lorenzi S, et al. (2016) Experimental review of DNA-based methods for wine traceability and development of a single-nucleotide polymorphism (SNP) genotyping assay for quantitative varietal authentication J Agric Food Chem 64: 6969–6984. https://doi.org/10.1021/acs.jafc.6b02560 doi: 10.1021/acs.jafc.6b02560
![]() |
[6] |
Galimberti A, Labra M, Sandionigi A, et al. (2014) DNA barcoding for minor crops and food traceability. Adv Agric 2014: 831875. https://doi.org/10.1155/2014/831875 doi: 10.1155/2014/831875
![]() |
[7] |
Piarulli L, Savoia MA, Taranto F, et al. (2019) A robust DNA isolation protocol from filtered commercial olive oil for PCR-based fingerprinting. Foods 8: 462. https://doi.org/10.3390/foods8100462 doi: 10.3390/foods8100462
![]() |
[8] |
Zambianchi S, Soffritti G, Stagnati L, et al. (2021) Applicability of DNA traceability along the entire wine production chain in the real case of a large Italian cooperative winery. Food Control 124: 107929. https://doi.org/10.1016/j.foodcont.2021.107929 doi: 10.1016/j.foodcont.2021.107929
![]() |
[9] |
Korir NK, Han J, Shangguan L, et al. (2013) Plant variety and cultivar identification: advances and prospects. Crit Rev Biotechnol 33: 111–125. https://doi.org/10.3109/07388551.2012.675314 doi: 10.3109/07388551.2012.675314
![]() |
[10] |
Scarano D, Rao R (2014) DNA markers for food products authentication. Diversity 6: 579–596. https://doi.org/10.3390/d6030579 doi: 10.3390/d6030579
![]() |
[11] | Kumar P, Gupta V, Misra A, et al. (2009) Potential of molecular markers in plant biotechnology. Plant Omics J 4: 141–162. |
[12] |
Melchiade D, Foroni I, Corrado G, et al. (2007) Authentication of the 'Annurca' apple in agro-food chain by amplification of microsatellites loci. Food Biotechnol 21: 33–43. https://doi.org/10.1080/08905430701191114 doi: 10.1080/08905430701191114
![]() |
[13] | Sabetta W, Miazzi MM, di Rienzo V, et al. (2017) Development and application of protocols to certify the authenticity and traceability of Apulian typical products in olive sector. Riv Ital Delle Sostanze Grasse 94: 37–43. |
[14] |
Stagnati L, Soffritti G Martino M, et al. (2020) Cocoa beans and liquor fingerprinting: A real case involving SSR profiling of CCN51 and "Nacional" varieties. Food Control 118: 107392. https://doi.org/10.1016/j.foodcont.2020.107392 doi: 10.1016/j.foodcont.2020.107392
![]() |
[15] | Talucci G, Vallauri G, Pavese V, et al. (2022) Identification of the hazelnut cultivar in raw kernels and in semi‑processed and processed products. Eur Food Res Technol 22: 532–541. |
[16] |
Yamamoto T, Kimura T, Hayashi T, et al. (2006) DNA profiling of fresh and processed ruits in pear. Breed Sci 56:165–171. https://doi.org/10.1270/jsbbs.56.165 doi: 10.1270/jsbbs.56.165
![]() |
[17] |
Wu Y, Li M, Yang Y, et al. (2018) Authentication of small berry fruit in fruit products by DNA barcoding method. J Food Sci 83: 1494–1504. https://doi.org/10.1111/1750-3841.14177 doi: 10.1111/1750-3841.14177
![]() |
[18] |
Hu Y, Lu X (2020) Rapid pomegranate juice authentication using a simple sample-to-answer hybrid paper/polymer-based lab-on-a chip device. ACS Sens 5: 2168–2176. https://doi.org/10.1021/acssensors.0c00786 doi: 10.1021/acssensors.0c00786
![]() |
[19] |
Ibrahim GE, Hassan IM, Abd-Elrashid AM, et al. (2011) Effect of clouding agents on the quality of apple juice during storage. Food Hydrocolloids 25: 91–97. https://doi.org/10.1016/j.foodhyd.2010.05.009 doi: 10.1016/j.foodhyd.2010.05.009
![]() |
[20] | Slinkard K, Singleton VL (1977) Total phenol analysis: Automation and comparison with manual methods. Am J Enoly Vitic 28: 49–55. |
[21] |
Okayasu H, Naito S (2001) Sensory characteristics of apple juice evaluated by consumer and trained panels. J Food Sci 66: 1025–1029. https://doi.org/10.1111/j.1365-2621.2001.tb08229.x doi: 10.1111/j.1365-2621.2001.tb08229.x
![]() |
[22] |
Boccacci P, Akkak A, Torello Marinoni D, et al. (2012) Genetic traceability of Asti Spumante and Moscato d'Asti musts and wines using nuclear and chloroplast microsatellite markers. Eur Food Res Technol 255: 439–446. https://doi.org/10.1007/s00217-012-1770-3 doi: 10.1007/s00217-012-1770-3
![]() |
[23] | Doyle JJ, Doyle JL (1987) A rapid DNA isolation procedure from small quantities of fresh leaf tissue. Phytochemical Bulletin 19: 11–15. |
[24] |
Han J, Wu Y, Huang W, et al. (2012) PCR and DHPLC methods used to detect juice ingredient from 7 fruits. Food Control 25: 696–703. https://doi.org/10.1016/j.foodcont.2011.12.001 doi: 10.1016/j.foodcont.2011.12.001
![]() |
[25] |
Guyot S, Marnet N, Sanoner P, et al. (2003) Variability of the polyphenolic composition of cider apple (Malus domestica) fruits and juices. J Agric Food Chem 51: 6240–6240. https://doi.org/10.1021/jf0301798 doi: 10.1021/jf0301798
![]() |
[26] |
Rydzak L, Kobus Z, Nadulski R, et al. (2020) Analysis of selected physicochemical properties of commercial apple juices. Processes 8: 1457. https://doi.org/10.3390/pr8111457 doi: 10.3390/pr8111457
![]() |
[27] |
Karadeniz F, Ekşi A (2002) Sugar composition of apple juices. Eur Food Res Technol 215: 145–148. https://doi.org/10.1007/s00217-002-0505-2 doi: 10.1007/s00217-002-0505-2
![]() |
[28] |
Niu S, Xu Z, Fang Y, et al. (2010) Comparative study on cloudy apple juice qualities from apple slices treated by high pressure carbon dioxide and mild heat. Innovative Food Sci Emerging Technol 11: 91–97. https://doi.org/10.1016/j.ifset.2009.09.002 doi: 10.1016/j.ifset.2009.09.002
![]() |
[29] |
Alonso-Salces RM, Ndjoko K, Queiroz EF, et al. (2004) On-line characterisation of apple polyphenols by liquid chromatography coupled with mass spectrometry and ultraviolet absorbance detection. J Chromatogr A 1046: 89–100. https://doi.org/10.1016/j.chroma.2004.06.077 doi: 10.1016/j.chroma.2004.06.077
![]() |
[30] |
Van Der Sluis AA, Dekker M, Boekel MA (2005). Activity and concentration of polyphenolic antioxidant in apple juice. 3. Stability during storage. J Agric Food Chem 53: 1073–1080. https://doi.org/10.1021/jf040270r doi: 10.1021/jf040270r
![]() |
[31] |
Renard CMGC, Le Quer JM, Bauduin R, et al. (2011) Modulating apple juices phenolic composition by manipulating the pressing conditions. Food Chem 124: 117–125. https://doi.org/10.1016/j.foodchem.2010.05.113 doi: 10.1016/j.foodchem.2010.05.113
![]() |
[32] |
Dasenaki ME, Thomaidis NS (2019) Quality and authenticity control of fruit juices review. Molecules 24: 1014. https://doi.org/10.3390/molecules24061014 doi: 10.3390/molecules24061014
![]() |
[33] |
Liebhard R, Gianfranceschi L, Koller B, et al. (2002) Development and characterization of 140 new microsatellites in apple (Malus x domestica Borkh). Mol Breed 10: 217–241. https://doi.org/10.1023/A:1020525906332 doi: 10.1023/A:1020525906332
![]() |
[34] |
Schrader C, Schielke A, Ellerbroek L, et al. (2012) PCR inhibitors—occurrence, properties and removal. J Appl Microbiol 113: 1014–1026. https://doi.org/10.1111/j.1365-2672.2012.05384.x doi: 10.1111/j.1365-2672.2012.05384.x
![]() |
[35] |
Cavanna M, Torello Marinoni D, Bounous G, et al. (2008) Genetic diversity in ancient apple germplasm from northwest Italy. J Hortic Sci Biotechnol 83: 549–554. https://doi.org/10.1080/14620316.2008.11512421 doi: 10.1080/14620316.2008.11512421
![]() |
[36] |
Corrado G (2016) Advances in DNA typing in the agro-food supply chain. Trends Food Sci Technol 52: 80–89. https://doi.org/10.1016/j.tifs.2016.04.003 doi: 10.1016/j.tifs.2016.04.003
![]() |
[37] | Sato K, Mori H, Matsui O, et al. (1991) Encyclopedia of fruit horticulture. Yokendo, Tokio. |
[38] | Pompei C (2005) La trasformazione industriale di frutta e ortaggi. Edagricole—Edizione Agricole de Il Sole 24 ORE Edagricole S.r.l. Bologna, 41–204. |
[39] |
Tsuji S, Ushio M, Sakurai S, et al. (2017) Water temperature-dependent degradation of environmental DNA and its relation to bacterial abundance. PLoS ONE 12: e0176608. https://doi.org/10.1371/journal.pone.0176608.t001 doi: 10.1371/journal.pone.0176608.t001
![]() |
[40] |
De Paepe D, Coudijzer K, Noten B, et al. (2015) A comparative study between spiral-filter press and belt press implemented in a cloudy apple juice production process. Food Chem173: 986–996. https://doi.org/10.1016/j.foodchem.2014.10.019 doi: 10.1016/j.foodchem.2014.10.019
![]() |
1. | Ying Xu, Huixin Qin, Dynamical Response in an Electromechanical Arm Driven by Temperature-Dependent Neural Circuit, 2024, 05779073, 10.1016/j.cjph.2024.11.010 | |
2. | D. Vignesh, Shaobo He, Santo Banerjee, A review on the complexities of brain activity: insights from nonlinear dynamics in neuroscience, 2024, 0924-090X, 10.1007/s11071-024-10558-2 | |
3. | Yitong Guo, Chunni Wang, Jun Ma, Model approach of artificial muscle and leg movements, 2025, 529, 03759601, 130069, 10.1016/j.physleta.2024.130069 | |
4. | Denggui Fan, Jin Chen, Songan Hou, Zhengyong Song, Gerold Baier, Qingyun Wang, From experimental phenomena to computational models: Exploring the synchronization mechanisms of phase-locked stimulation in the hippocampal–thalamic–cortical circuit for memory consolidation, 2025, 191, 09600779, 115754, 10.1016/j.chaos.2024.115754 | |
5. | Qun Guo, Guodong Ren, Chunni Wang, Zhigang Zhu, Reliability and energy function of an oscillator and map neuron, 2025, 251, 03032647, 105443, 10.1016/j.biosystems.2025.105443 |
Parameter | Value |
H_1 | 0.0998 |
H_2 | 0.7984 |
\gamma | 0.6986 |
dt | 3.9683 \times 10^{-5} |
\epsilon | 0.01 |
\delta | 0.001 |
S_0 | 3941.26 |
Y_0 | 0.001 |
Z_0 | 0.001 |
\phi_1 , \phi_2 | 1 |
Function | Choice |
f(y, z) | \frac{\nu}{1+e^{-x}+e^{-y}}, \, \, \nu= 0.5988 |
\alpha(y) | -y |
\beta(y) | \sqrt{2} |
g(z) | z |
h(z) | z |
Days to Maturity: 31 | |||
\parallel P_{\text{market}}-P_{\text{MC}} \parallel | \parallel P_{\text{market}}-P_{\text{fMSV}} \parallel | \parallel P_{\text{market}}-P_{\text{MSV}} \parallel | \parallel P_{\text{market}}-P_{\text{BS}} \parallel |
28.7959 | 18.3497 | 31.6503 | 36.7458 |
Days to Maturity: 56 | |||
\parallel P_{\text{market}}-P_{\text{MC}} \parallel | \parallel P_{\text{market}}-P_{\text{fMSV}} \parallel | \parallel P_{\text{market}}-P_{\text{MSV}} \parallel | \parallel P_{\text{market}}-P_{\text{BS}} \parallel |
97.7220 | 69.4679 | 90.5983 | 90.8465 |
P_{\text{MC}} | P_{\text{fMSV}} | P_{\text{MSV}} | P_{\text{BS}} |
307.7409 | 3.9800 \times 10^{-4} | 2.8880 \times 10^{-4} | 2.3640 \times 10^{-4} |
Convergence of PfMSV to PMC* | ||
\gamma | P_{\text{fMSV}} | \lVert P_{\text{MC*}}-P_{\text{fMSV}}\rVert |
0.99000 | 40.8679 | 0.3964 |
0.89101 | 40.8901 | 0.3742 |
0.79202 | 40.9096 | 0.3548 |
0.69303 | 40.9347 | 0.3300 |
0.59404 | 40.9613 | 0.3030 |
0.49505 | 40.9914 | 0.2730 |
0.39606 | 41.0247 | 0.2396 |
0.29707 | 41.0629 | 0.2014 |
0.19808 | 41.1082 | 0.1562 |
0.09909 | 41.1661 | 0.0983 |
0.00010 | 41.2595 | 0.0048 |
Convergence of PfMSV to PMC* | |||
\delta | \epsilon | P_{\text{fMSV}} | \lVert P_{\text{MC*}}-P_{\text{fMSV}}\rVert |
1.0000 \times 10^{-5} | 1.0000 \times 10^{-4} | 39.7545 | 1.5031 |
9.0001 \times 10^{-6} | 9.0100 \times 10^{-5} | 39.8316 | 1.4260 |
8.0002 \times 10^{-6} | 8.0200 \times 10^{-5} | 39.9131 | 1.3445 |
7.0003 \times 10^{-6} | 7.0300 \times 10^{-5} | 39.9997 | 1.2578 |
6.0004 \times 10^{-6} | 6.0400 \times 10^{-5} | 40.0928 | 1.1648 |
5.0005 \times 10^{-6} | 5.0500 \times 10^{-5} | 40.1938 | 1.0638 |
4.0006 \times 10^{-6} | 4.0600 \times 10^{-5} | 40.3053 | 0.9523 |
3.0007 \times 10^{-6} | 3.0700 \times 10^{-5} | 40.4315 | 0.8261 |
2.0008 \times 10^{-6} | 2.0800 \times 10^{-5} | 40.5803 | 0.6773 |
1.0009 \times 10^{-6} | 1.0900 \times 10^{-5} | 40.7715 | 0.4861 |
1.0000 \times 10^{-9} | 1.0000 \times 10^{-6} | 41.1209 | 0.1367 |
Parameter | Value |
H_1 | 0.0998 |
H_2 | 0.7984 |
\gamma | 0.6986 |
dt | 3.9683 \times 10^{-5} |
\epsilon | 0.01 |
\delta | 0.001 |
S_0 | 3941.26 |
Y_0 | 0.001 |
Z_0 | 0.001 |
\phi_1 , \phi_2 | 1 |
Function | Choice |
f(y, z) | \frac{\nu}{1+e^{-x}+e^{-y}}, \, \, \nu= 0.5988 |
\alpha(y) | -y |
\beta(y) | \sqrt{2} |
g(z) | z |
h(z) | z |
Days to Maturity: 31 | |||
\parallel P_{\text{market}}-P_{\text{MC}} \parallel | \parallel P_{\text{market}}-P_{\text{fMSV}} \parallel | \parallel P_{\text{market}}-P_{\text{MSV}} \parallel | \parallel P_{\text{market}}-P_{\text{BS}} \parallel |
28.7959 | 18.3497 | 31.6503 | 36.7458 |
Days to Maturity: 56 | |||
\parallel P_{\text{market}}-P_{\text{MC}} \parallel | \parallel P_{\text{market}}-P_{\text{fMSV}} \parallel | \parallel P_{\text{market}}-P_{\text{MSV}} \parallel | \parallel P_{\text{market}}-P_{\text{BS}} \parallel |
97.7220 | 69.4679 | 90.5983 | 90.8465 |
P_{\text{MC}} | P_{\text{fMSV}} | P_{\text{MSV}} | P_{\text{BS}} |
307.7409 | 3.9800 \times 10^{-4} | 2.8880 \times 10^{-4} | 2.3640 \times 10^{-4} |
Convergence of PfMSV to PMC* | ||
\gamma | P_{\text{fMSV}} | \lVert P_{\text{MC*}}-P_{\text{fMSV}}\rVert |
0.99000 | 40.8679 | 0.3964 |
0.89101 | 40.8901 | 0.3742 |
0.79202 | 40.9096 | 0.3548 |
0.69303 | 40.9347 | 0.3300 |
0.59404 | 40.9613 | 0.3030 |
0.49505 | 40.9914 | 0.2730 |
0.39606 | 41.0247 | 0.2396 |
0.29707 | 41.0629 | 0.2014 |
0.19808 | 41.1082 | 0.1562 |
0.09909 | 41.1661 | 0.0983 |
0.00010 | 41.2595 | 0.0048 |
Convergence of PfMSV to PMC* | |||
\delta | \epsilon | P_{\text{fMSV}} | \lVert P_{\text{MC*}}-P_{\text{fMSV}}\rVert |
1.0000 \times 10^{-5} | 1.0000 \times 10^{-4} | 39.7545 | 1.5031 |
9.0001 \times 10^{-6} | 9.0100 \times 10^{-5} | 39.8316 | 1.4260 |
8.0002 \times 10^{-6} | 8.0200 \times 10^{-5} | 39.9131 | 1.3445 |
7.0003 \times 10^{-6} | 7.0300 \times 10^{-5} | 39.9997 | 1.2578 |
6.0004 \times 10^{-6} | 6.0400 \times 10^{-5} | 40.0928 | 1.1648 |
5.0005 \times 10^{-6} | 5.0500 \times 10^{-5} | 40.1938 | 1.0638 |
4.0006 \times 10^{-6} | 4.0600 \times 10^{-5} | 40.3053 | 0.9523 |
3.0007 \times 10^{-6} | 3.0700 \times 10^{-5} | 40.4315 | 0.8261 |
2.0008 \times 10^{-6} | 2.0800 \times 10^{-5} | 40.5803 | 0.6773 |
1.0009 \times 10^{-6} | 1.0900 \times 10^{-5} | 40.7715 | 0.4861 |
1.0000 \times 10^{-9} | 1.0000 \times 10^{-6} | 41.1209 | 0.1367 |