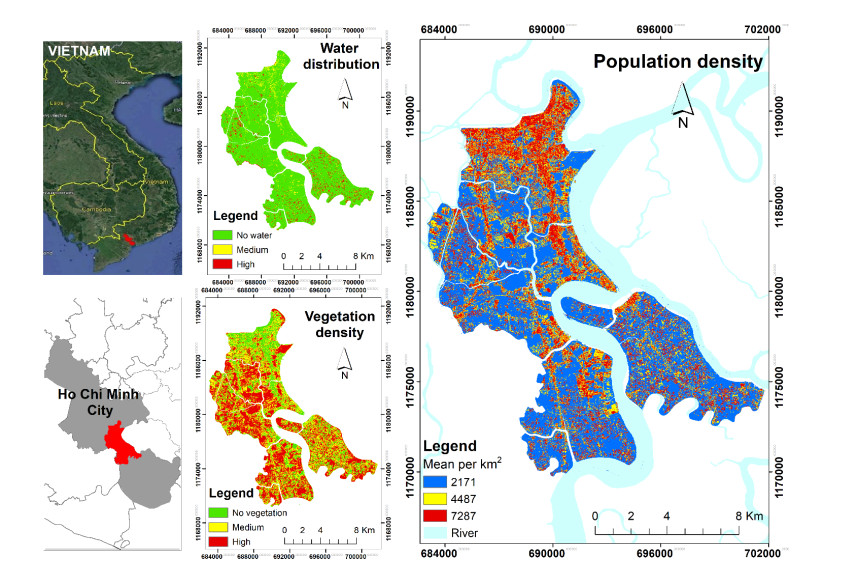
This paper shows how biological population dynamic models in the form of coupled reaction-diffusion equations with nonlinear reaction terms can be applied to heterogeneous landscapes. The presented systems of coupled partial differential equations (PDEs) combine the dispersal of disease-vector mosquitoes and the spread of the disease in a human population. Realistic biological dispersal behavior is taken into account by applying chemotaxis terms for the attraction to the human host and the attraction of suitable breeding sites. These terms are capable of generating the complex active movement patterns of mosquitoes along the gradients of the attractants. The nonlinear initial boundary value problems are solved numerically for geometries of heterogeneous landscapes, which have been imported from geographic information system data to construct a general-purpose finite-element solver for systems of coupled PDEs. The method is applied to the dispersal of the dengue disease vector for Aedes aegypti in a small-scale rural setting consisting of small houses and different breeding sites, and to a large-scale section of the suburban zone of a metropolitan area in Vietnam. Numerical simulations illustrate how the setup of model equations and geographic information can be used for the assessment of control measures, including the spraying patterns of pesticides and biological control by inducing male sterility.
Citation: Otto Richter, Anh Nguyen, Truc Nguyen. Application of reaction-diffusion equations for modeling human and breeding site attraction movement behavior of Aedes aegypti mosquito[J]. Mathematical Biosciences and Engineering, 2022, 19(12): 12915-12935. doi: 10.3934/mbe.2022603
[1] | Bo Zheng, Wenliang Guo, Linchao Hu, Mugen Huang, Jianshe Yu . Complex wolbachia infection dynamics in mosquitoes with imperfect maternal transmission. Mathematical Biosciences and Engineering, 2018, 15(2): 523-541. doi: 10.3934/mbe.2018024 |
[2] | Chen Liang, Hai-Feng Huo, Hong Xiang . Modelling mosquito population suppression based on competition system with strong and weak Allee effect. Mathematical Biosciences and Engineering, 2024, 21(4): 5227-5249. doi: 10.3934/mbe.2024231 |
[3] | Yanfeng Liang, David Greenhalgh . Estimation of the expected number of cases of microcephaly in Brazil as a result of Zika. Mathematical Biosciences and Engineering, 2019, 16(6): 8217-8242. doi: 10.3934/mbe.2019416 |
[4] | Martin Strugarek, Nicolas Vauchelet, Jorge P. Zubelli . Quantifying the survival uncertainty of Wolbachia-infected mosquitoes in a spatial model. Mathematical Biosciences and Engineering, 2018, 15(4): 961-991. doi: 10.3934/mbe.2018043 |
[5] | Biao Tang, Weike Zhou, Yanni Xiao, Jianhong Wu . Implication of sexual transmission of Zika on dengue and Zika outbreaks. Mathematical Biosciences and Engineering, 2019, 16(5): 5092-5113. doi: 10.3934/mbe.2019256 |
[6] | Bo Zheng, Lihong Chen, Qiwen Sun . Analyzing the control of dengue by releasing Wolbachia-infected male mosquitoes through a delay differential equation model. Mathematical Biosciences and Engineering, 2019, 16(5): 5531-5550. doi: 10.3934/mbe.2019275 |
[7] | Yunfeng Liu, Guowei Sun, Lin Wang, Zhiming Guo . Establishing Wolbachia in the wild mosquito population: The effects of wind and critical patch size. Mathematical Biosciences and Engineering, 2019, 16(5): 4399-4414. doi: 10.3934/mbe.2019219 |
[8] | Luis Almeida, Michel Duprez, Yannick Privat, Nicolas Vauchelet . Mosquito population control strategies for fighting against arboviruses. Mathematical Biosciences and Engineering, 2019, 16(6): 6274-6297. doi: 10.3934/mbe.2019313 |
[9] | Minna Shao, Hongyong Zhao . Dynamics and optimal control of a stochastic Zika virus model with spatial diffusion. Mathematical Biosciences and Engineering, 2023, 20(9): 17520-17553. doi: 10.3934/mbe.2023778 |
[10] | Daiver Cardona-Salgado, Doris Elena Campo-Duarte, Lilian Sofia Sepulveda-Salcedo, Olga Vasilieva, Mikhail Svinin . Optimal release programs for dengue prevention using Aedes aegypti mosquitoes transinfected with wMel or wMelPop Wolbachia strains. Mathematical Biosciences and Engineering, 2021, 18(3): 2952-2990. doi: 10.3934/mbe.2021149 |
This paper shows how biological population dynamic models in the form of coupled reaction-diffusion equations with nonlinear reaction terms can be applied to heterogeneous landscapes. The presented systems of coupled partial differential equations (PDEs) combine the dispersal of disease-vector mosquitoes and the spread of the disease in a human population. Realistic biological dispersal behavior is taken into account by applying chemotaxis terms for the attraction to the human host and the attraction of suitable breeding sites. These terms are capable of generating the complex active movement patterns of mosquitoes along the gradients of the attractants. The nonlinear initial boundary value problems are solved numerically for geometries of heterogeneous landscapes, which have been imported from geographic information system data to construct a general-purpose finite-element solver for systems of coupled PDEs. The method is applied to the dispersal of the dengue disease vector for Aedes aegypti in a small-scale rural setting consisting of small houses and different breeding sites, and to a large-scale section of the suburban zone of a metropolitan area in Vietnam. Numerical simulations illustrate how the setup of model equations and geographic information can be used for the assessment of control measures, including the spraying patterns of pesticides and biological control by inducing male sterility.
The modeling of dispersal through the use of reaction-diffusion equations has a long history. As early as 1937, it was shown that a diffusion model with logistic growth terms can generate traveling waves [1]. From this seminal work sprang numerous mathematical publications on the existence of traveling wave solutions, see, for example, Hadeler and Rothe [2] and the review articles of Volpert and Petrovskii [3] and Wang [4]. Coupled nonlinear reaction-diffusion equations also have a long tradition of application to model the spread of diseases. The classical SIR epidemiological model has been applied in combination with diffusion to generate epizootic waves, as has been modeled for rabies dispersal in England by Murray and Seward [5] and Murray et al. [6]. There exist many publications on the dispersal of insect-related disease vectors within the frame of reaction-diffusion equations. Maidana and Yang [7] analyzed the spread of dengue disease via the disease vector Aedes aegypti and developed criteria for the onset of traveling waves in one dimension. Almeida et al. [8] evaluated the use of insecticides and male sterility induction as a means of blocking traveling waves from the mathematical point of view in a one-dimensional setting, providing also a theoretical proof of the efficiency of the sterile insect technique (SIT). Also, in a one-dimensional setting, Anguelov et al. [9] proved that bistable traveling wave solutions exist if sterile males are introduced; they illustrated their theorem via numerical investigations. In other papers, the focus is on dispersal in realistic two-dimensional spatial settings. Lutambi et al. [10] discretized the space by introducing heterogeneous hexagonal patches. For each patch, a system of ordinary differential equations for the mosquito dynamics was setup, and it allowed for movement between neighboring patches. Yamashita et al. [11] carried out two-dimensional numerical simulations of a reaction-diffusion model for Aedes aegypti in a residential environment consisting of streets, blocks and a beach by using a finite-volume method. Knight [12] developed a model of the mosquito-mangrove basin ecosystem, detailing the habitat of the saltwater mosquito Aedes vigilax of a mangrove forest in Australia. Richter et al. [13] modeled the invasion of a new species by coupling geographic information system data on temperature using a finite-element tool.
Recent experimental studies have shown that the heat emanating from humans [14] and olfactory agents from breeding sites [15] control mosquito movement patterns. It was found that host seeking by mosquitoes and other pathogen-spreading insects relies on the detection of host-associated cues, including body heat [14,16,17,18,19]. Heat strongly stimulates mosquito blood feeding, as heat seeking is a part of a multimodal host-seeking program followed by mosquitoes in which body heat serves as an important cue of the host [14,17,20,21]. After a blood meal from a host, female mosquitoes look for suitable oviposition sites to guarantee success of the offspring. In order to remember its breeding site, mosquitoes need to adapt by learning to respond to odor cues from larval, pupal or early adult vectors [22]. Aedes aegypti mosquitoes are capable of information retention, which is acquired either during larval development, hatching or foraging, and that new information could influence their behavior [15]. Learning from its own experience could influence the vector behavior, such as the potential preferences in vector-host interactions [23,24].
Reaction-diffusion equations with simple diffusion terms do not reflect realistic dispersal patterns of mosquitoes, which are driven by an attraction to prey (humans) and the attraction of breeding sites. The application of reaction-diffusion equations for the dispersal of mosquitoes (and other species) therefore faces two challenges:
1) Coupling the governing equations with geographic information on landscape heterogeneity e.g., types of human settlements and possible breeding sites.
2) Accounting for animal behavior in the equations.
It was the purpose of this study to model and analyze mosquito movement at small scales, including attractant behavior, and to model the dispersal of mosquitoes and spread of diseases in a human population at the landscape scale. To this end, the governing equations were implemented in the finite-element tool COMSOL Multiphysics. For the landscape scale studies, landscape data extracted from Sentinel satellite images were imported using a geographic information system.
The chosen area for this model application is a suburban zone of a metropolitan area in the south of Vietnam. In Vietnam, mosquitoes and mosquito-borne diseases pose severe problems. They occur in most provinces and cities across the country, but are more common in the southern region, especially in accelerating urbanization zones such as the southern area of Ho Chi Minh (HCM) city (Figure 1). Therefore, for our study area, we chose a section of the urbanization zone in the south of HCM city; it has a mixture of vegetation, water bodies, rural settlements and urban residential areas. The study area includes District 7, Nha Be District and the Binh Khanh Ward (Figure 1). The area belongs to the downstream area of the Dong Nai and Saigon river system. This study area was previously covered by a mangrove forest and has slowly been urbanized since 1972. The urbanization has been accelerating since 1990.
The target mosquito species of this study is the Aedes aegypti species, which is involved in the transmission of numerous viruses, including dengue, chikungunya and Zika. There are many studies on the mosquito habitat and life cycle in Vietnam, and the earliest work could be the work of Herbert et al. [25], who conducted mosquito surveys and analyzed their distribution. Later, Huber et al. [26] studied the ecology of Aedes aegypti, genetic differentiation, variability in competence as a vector for dengue virus and resistance to insecticides; this was to assess the role of the vector in the changing pattern of the disease in Vietnam. Jeffery et al. [27] collected and classified water containers from large tanks to small jars in the center of Vietnam to determine if Aedes aegypti populations were spatially and temporally homogeneous.
The objectives of the study were to perform a numerical investigation that analyzed models of the dynamics of mosquito populations that are driven by an attraction to prey (humans) and the attraction of breeding sites, as well as of the spread of vector-borne diseases in urban-wetland areas.
Notations
State variables
A: mosquitoes (aquatic phase)
M: mosquitoes winged phase (general)
Ms: mosquitoes (not vector of virus)
Mi: mosquitoes (vector of virus)
M1: mosquitoes (searching for blood meal)
M2: mosquitoes (searching for breeding site)
Mij: mosquitoes in search mode i and infectious mode j
Hs: non-infected humans
Hi: infected humans
Hr: removed (immune) humans
Parameters
c(x, y): environmental capacity (aquatic phase)
D: diffusion coefficient
ϕ: oviposition rate
γ: hatching rate
μA: mortality rate aquatic phase
μM: mortality rate winged phase
μs: mortality rate due to insecticide spraying
d: width of spraying corridor
y0: position of spraying corridor
r: survival of hatched larvae
ß: contact rate for mosquito-susceptible humans (small-scale model)
ß1: contact rate for not virus bearing mosquitoes with infectious humans
ß2: contact rate for infectious mosquito-susceptible humans
τ−1: infectious period
ω12: rate of change between mosquito states
v: conservative flux convection coefficient
The general form of a system of reaction-diffusion equations for biological populations with a density yi is given by
∂yi∂t=Ly[yi]+fi(y1,y2 KyP;u1,u2…uE)i=1,Λ,p | (1) |
where the spatial operator has the form
Ly[y]=∇⋅(D∇y−vy) | (2) |
with the conservative flux coefficient
v=a+χ(c)∇c | (3) |
which allows for movement in the direction of a gradient of an attractant c. The reaction term fi accounts for the population dynamic and genetic processes [28]. Note that the system may also include components that are stationary, as is the case for a stage-structured population.
For a mosquito population, the basic model consists of the aquatic phase (A) and the winged phase (M) [7]. The capacity term varies in space.
∂A∂t=ϕM(1−AC(x,y))−γA−μAA | (4) |
The winged phase is coupled to the aquatic phase via the hatching term rγA, as follows:
∂M∂t=∇·(D∇M−vM)+rγA−μMM | (5) |
In Eq (5), the first through fourth terms respectively represent the random dispersal, vectored dispersal, hatching and mortality rate.
This model takes into account the blood-feeding status of female mosquitoes, differing with respect to dispersal patterns. Unsaturated mosquitoes hover around their prey, attracted by the body heat. It was recently found that a receptor (IR21a) is activated when mosquitoes move toward cooler temperatures [14]. After the blood meal, mosquitoes search for breeding sites for oviposition, guided by olfactory preferences [15]. The different movement patterns are expressed by chemotaxis terms; for humans (in terms of temperature), the term is
vT=−χT∇T | (6) |
for breeding sites (in terms of olfactory agents), the term is
vB=−χB∇B | (7) |
The chemotaxis fields are generated by a linear diffusion process with humans and breeding sites as sources and boundary absorption conditions.
Mosquitoes become vectors of disease if they bite infectious humans. Therefore, in the model, the population is differentiated into susceptible (Index s) and infected (Index i) subpopulations. The system is solved over a region G, which includes breeding sites denoted as B, human settlements and vegetation.
∂M1s∂t=∇·(D∇M1s−vTM1s)+rγA−μMM1s−β(Hs+Hi)M1s+ωM2s | (8) |
Mosquitoes in blood-seeking mode that have hatched at a breeding site (rγA) disperse and seek humans (term: vTM1s). After biting either non-infected or infected humans, they switch into the breeding-site seeking mode (β(Hs+Hi)M1s). The last term represents the switching following oviposition.
∂M1i∂t=∇·(D∇M1i−vTM1i)−μMM1i−β(Hs+Hi)M1i+ωM2i | (9) |
Infected mosquitoes originate from encounters with infected humans and do not develop from eggs. Otherwise, their behavior is the same as that of the non-infected type.
∂M2s∂t=∇·(D∇M2s−vBM2s)−μMM2s+βHsM1s−ωM2s | (10) |
Non-infected mosquitoes that have become saturated as a result of biting humans at a rate βHsM1s are attracted to breeding sites (term: vBM2s) and switch back to the blood-seeking mode after oviposition (last term).
∂M2i∂t=∇·(D∇M2i−vBM2i)−μMM2i+βHiM1s+β(Hs+Hi)M1i−ωM2i | (11) |
Saturated mosquitoes that have become infected as a result of biting infectious humans at a rate βHiM1s are attracted to breeding sites (term: vBM2i) and switch back to the blood-seeking mode after oviposition (last term), where
ω={ω12 if(x,y)∈B0 if(x,y)∉B} | (12) |
where B⊂G.
At the landscape scale (areas of several square kilometers), the movement from local breeding sites to humans is neglected. The objective of models at this scale is to simulate the spread of the disease in consideration of mosquito densities and the densities of human populations. To this end, capacity terms are formulated to reflect the availability of typical breeding sites. Two types of breeding sites are distinguished according to different carrying capacities: water bodies outside human settlements, such as ditches, or small ponds with low carrying capacities and small water bodies in plastic containers, mud dishes, mud pots, ditches and discarded tires with high capacities [29]. The latter breeding sites are connected to the types of human settlements. Here, we distinguish rural settlements with lots of such breeding sites and cities with a highly developed infrastructure and not much littering. The governing equations are based on the work of Maidana and Yang [7], modifications to the chemotaxis terms and nonlinear dispersal.
∂A∂t=ϕ(Ms+Mi)(1−AC(x,y))−γA−μAA | (13) |
∂Ms∂t=∇·(D∇Ms−vTMs)+rγA−μMMs−β1HiMs | (14) |
∂Mi∂t=∇·(D∇Mi−vTMs)−μMMi+β1HiMs | (15) |
∂Hs∂t=−β2HsMi | (16) |
∂Hi∂t=β2HsMs−τHi | (17) |
∂Hr∂t=τHi | (18) |
Note that parameters such as the environmental capacity or contact rates are dependent on the infrastructure of the study area. The parameter values are shown in Table 1.
Parameter | Notation | Value |
Carrying capacity | c | Maximum 0.8/m2 |
Diffusion coefficient | D | 1.25 103 m2/day |
Hatching rate | γ | 0.38/day Range (0.2, 0.5) |
Survival of hatched larvae | r | 0.5 |
Oviposition rate | ϕ | 25/day Range (10, 300) |
Mortality rate (mosquitoes, winged phase) | μM | 0.029/day |
Mortality rate (mosquitoes, aquatic phase) | μA | 0.055/day |
Attraction coefficient (humans) | χT | 1.7 × 104 |
Attraction coefficient (breeding sites) | χB | 2.4 × 104 |
Diffusion coefficient for attractants | DT DB |
1.0 × 103 m2/day |
contact rate for not virus bearing mosquitoes with infectious humans | ß1 | 1.65 m2/day |
Contact rate for infectious mosquito-susceptible human | ß2 | 3.75 m2/day |
Infectious period | τ−1 | 7 days |
Rate of change between mosquito states (only in the small-scale model) | ω12 | 90/day |
Release of sterile males.
The effect of this method is the decrease of fertile mating by the factor f = Ms+MiMs+Mi+S, where S denotes the density of sterile males under the condition of a sex ratio of 1:1.
The number of eggs is therefore reduced by this factor and Eq (13) is modified as follows.
∂A∂t=ϕ(Ms+Mi)(1−AC(x,y))f−γA−μAA | (19) |
The spread of sterile mosquitoes is modeled by a diffusion equation with a mortality term and a source term, which summarize the release rate Ri at each location (xi,yi).
∂S∂t=∇·(D∇S)−μMS+∑ni=1Ri | (20) |
Spraying in a corridor.
A spraying corridor parallel to the x axis is defined by the y position y0, the width d of the corridor and the form parameter n, which determines the reduction in pesticide action. The spraying introduces an additional mortality rate.
m(x,y)=μsexp[−(y−y0)d44] | (21) |
where μs denotes the maximum mortality, which decreases with distance from the centre of the corridor y0.
For the small-scale model, Neumann conditions were applied to the walls of the houses and the zero Dirichlet condition was applied to the outer boundaries. For the large-scale model, the zero Dirichlet condition was applied to the boundaries of the region. The results are only slightly influenced if the outer boundary conditions are Neumann.
The governing equations were implemented using the finite-element tool COMSOL Multiphysics version 6.0 (www.comsol.de). This tool is well adapted for the combination with geographical data. For time-dependent problems, the variable order backward differentiation formula solver in COMSOL was used. For the simulation of dispersal on the landscape scale, COMSOL was linked to a geographic information system that provided population density data and the landscape geometry. Geo-referenced data for the study area were imported from the resultant raster maps for vegetation density level, water body coverage and mean population density distribution.
This information was extracted from Sentinel-2 imagery. Sentinel-2 is a high-spatial-resolution (10, 20, 60 m), high-temporal-resolution (5 days), multispectral (13 bands) imaging satellite carrying a multispectral imager (MSI) [30]. The level 1C data of an image acquired on January 17, 2021 were downloaded from the Copernicus Open Access Hub (https://scihub.copernicus.eu/). These data were pre-processed using a SNAP Desktop platform (Version 8.0.0; SNAP 2020) with the Sentinel-2 Toolbox. This Level 1C image with top-of-atmosphere (TOA) reflectance was processed to a Level-2A bottom-of-atmosphere (BOA) product using the European Space Agency Sentinel Application Platform (ESA-SNAP) Sentinel-2 Toolbox with the additional Sen2Cor plug-in for atmospheric correction. Sentinel-2 bands 5, 6, 7, 8a, 11 and 12 (all are short-wave infrared) were resampled to 10 m × 10 m from their native resolution of 20 m × 20 m to match the resolution of the other bands. These processed bands were used to extract the levels of vegetation cover, the water body and the urban-nonurban areas. The population density was obtained from the Worldpop (www.worldpop.org) global high-resolution population denominators project, which provides gridded population counts at 100-m spatial resolution (3 arc-seconds).
The data were interpolated within COMSOL by using the two-dimensional linear interpolation option in the function menu. The implementation scheme is shown in Figure 2. The environmental capacity of mosquitoes is related to the residential environment, which has been characterized by scores ranging from 0 to 3. In a rural setting (Score 2), the preferred breeding sites of Aedes aegypti, like small water containers, pots or tires, are more common than in dense urban areas (Score 3). Scores 0 and 1 are given to water bodies and vegetation. For numerical reasons a smooth curve was created to avoid abrupt changes in the parameters following transitions of infrastructural features (Figure 3).
We applied this model to investigate the movements of mosquito populations and the transmission of dengue disease to humans by infected vectors. Two scales were setup for the geometry settings. At the fine scale, this model can predict how mosquito populations move between the houses and breeding sites and how the humans are infected by the disease in the area. At the landscape scale, the model can simulate the dispersal of infectious mosquitos and humans and the effects of control measures.
Here, we have constructed a typical rural setting consisting of small houses and different breeding sites. Between the houses (rectangular shapes), there is a dich, near the houses there are small breeding sites representing containers, pots, and other mosquito-preferred sites. The small circles represent humans and their range of movement. In the model, breeding sites and humans constitute the source of the respective attractants.
The circles in the houses represent humans and their range of movement. In the model, breeding sites and humans constitute the source of the respective attractants. Figure 4 shows the velocity fields of mosquitoes in the non-saturated and saturated states. Due to the chemotaxis effects, one can clearly distinguish between different movement patterns.
Along with mosquito movement, an infection is spread if infectious humans are present. This is shown in the simulation results in Figure 5. The small circles in the houses represent humans and their radius of interaction. At the beginning, one human outside of a house is infected (red circle, Figure 5(a)). Note that humans are fixed in space, i.e., there are no diffusion terms in the governing equations. After 3 days, a portion of the humans are infected (circles with colors, Figure 5(b)); after 5 days, all humans are infected (Figure 5(c)). The movement pattern is determined by the exchange between breeding sites and humans (Figure 5(d)).
The following simulations show the attractive effects in a real landscape. The dispersal of mosquito populations has been followed from a focal point in the area. Different types of dispersals were observed, including the free dispersal of mosquitoes under the conditions of no treatment regime and the spread of mosquitoes and disease transmission with the aforementioned control management.
The governing equations, presented as Eqs (13)–(18), are solved for the study area. Here, the detailed movement between breeding and feeding sites is not considered; however, the directed movement to humans is maintained. Figure 6 shows the movement pattern of mosquitoes for a section of the study area.
As was to be expected from the many theoretical studies mentioned in the introduction, traveling waves occur if certain parameter constellations are given. Figure 7 shows a 3D representation of a traveling wave of infected humans, as obtained for a homogeneous domain. In a nonhomogeneous environment, traveling wave fronts are distorted as shown in Figure 8.
Figure 8 shows the wave of the spread of infected humans. Distorted wave fronts reflect the heterogeneity of the landscape. Note that the underlying heterogeneity of the resources and human population density are given in Figure 1.
Accordingly, the density of the susceptible population is decreasing in a complementary manner. Figure 9(a)–(d) show the spatiotemporal evolution of the susceptible population from Day 0 to Day 250. One can clearly see that the disease progresses like a wave with an irregular wavefront, reflecting the landscape heterogeneity.
It is also interesting to look at the time evolution of the epidemic at single points (Figure 10). One can recognize the typical time courses of the classical SIR model, irrespective of the time delay due to the spatial separation. At any given time, individual humans will be in one of three states, namely, S (susceptible), I (infectious) or R (recovered/immune). The infected mosquitoes in the model do not have a recovered/immune state, as it has been assumed that mosquitoes remain infected until they die. One can see that dengue transmission peaks travel along with the traveling wave fronts.
An understanding of the movement behavior of mosquitoes and their preferable breeding sites enables the assessment of management strategies (method, frequency, area). Here, we show some simulations of control measures for illustrative purposes. Management methods were incorporated into the model, and they include conventional means of vector control, i.e., insecticide spraying and the use of sterile males to decrease the frequency of fertile mating. One question to answer was as follows: how can a traveling wave be stopped by spraying? In the simulation, a corridor of intensive spraying was implemented. This was achieved by introducing a high mortality rate to a selected strip. The simulations show that the success of this measure depends on the breadth of the spraying zone, as shown in Figure 11.
If the breadth of the corridor is too small, the traveling waves are delayed but are able to persist. So, there exists a threshold value for this parameter, which is related to the minimal viable population size, as discussed by Richter et al. [13]. The sterile-male-release technique has recently seen a comeback [31]. The effect of this method is a decrease in fertile mating. This control measure has been incorporated into the model in a straightforward way by simply adding a further equation for sterile males and the locations of release (cf. Eqs (19) and (20)). The success of this measure depends both on the release rate and the population density at the location of the release sites. As was proved in the paper written by Anguelov et al. [9], the release of sterile males leads to a bistable reaction-diffusion equation. They found that "the dynamics of our system is driven by a sterile insect technique (SIT)-threshold number above which the SIT control becomes effective and drives the system to elimination". For the parameters of our model, this threshold was found to be higher than the environmental capacity. However, due to the property of bistability, elimination can be reached at lower release rates if the insect population is low. This behavior was also immanent in our model equations. Here, we show only one result of many simulations runs.
Figure 12 shows the density of sterile males released at four locations and their effects on the mosquito population.
For heterogeneous landscapes, there are quite a few approaches wherein the region is subdivided into patches, each of which with different environmental variables [10,32]; the ordinary differential equations are solved for each patch, allowing for migration between patches. Such approaches are similar to reaction-diffusion equations.
Although the theoretical basis of animal dispersal and the dispersal of epidemics in the form of reaction-diffusion equations was established decades ago, such approaches still constitute a powerful tool for realistic simulations, especially when finite-element methods are coupled with geographic information. Furthermore, the underlying mathematical theory has been well elaborated and allows one to decide whether the problems of the solution of the initial value boundary problems are well posed; it also guides us in the understanding and evaluation of the numerically obtained results. Our simulation experiments show that complex movement patterns of mosquitoes can be well incorporated into reaction-diffusion equations. The model takes into account the implication that female mosquitoes retain information from the larval and pupal environment from which they emerge as adult. This was discussed in [33,34]. The dissociation between the oviposition site and remote food source (human blood) requires the ability for long- or mid-range orientation, and it would necessitate "some form of learnt behavior" [35,36]. Breeding site preferences determine the movement patterns. Gaburro et al. [15] found that semiochemicals derived from Aedes aegypti larval rearing affect the future female choice for oviposition. If infected by the dengue virus, preferences for special breeding sites might be lost. Olfactory learning processes were altered during dengue infection. They hypothesized that dengue virus infection alters gene expression in the mosquito's head and is associated with a loss of olfactory preferences, possibly modifying the oviposition site choice of female mosquitoes.
Reaction-diffusion models of the kind presented here, when being coupled with breeding site preferences and driving variables such as the diurnal rhythms of mosquito activity and seasonal changes in moisture and temperature, show that predator–prey interactions can become a powerful tool in predicting infected mosquito behavior and the transmission of mosquito-borne disease at several scales. For long-term predictions, it is also important to consider the evolution of resistance against pesticides.
For future development and application of the model, experiments and observational studies need to be carried out to contribute to model parameters, such as the transition rate between mosquito states, and parameters related to the dispersal and strength of attractants. A promising method for parameter identification is the combination of models and Bayesian statistics, as has been recently applied to the spread of Aedes aegypti in urban areas [37]. Once the model is fully parameterized, it can be used for the realistic planning of various control measures, such as the reduction of breeding sites and the reduction of contact rates via application of repellents and biological and genetic control measures.
Although our mathematical model for the movement patterns of mosquitoes, which is based on the mode of selection between blood-meal sites and breeding sites, is just at the theoretical stage, it has potential for real-world applications. When being coupled with other processes and observational data, the model can help to (ⅰ) assess the effects of fast land-use changes and sanitary conditions of an area from the perspective of the dynamics and life cycle of mosquito population, (ⅱ) assess the effects of the heterogeneous characteristics of the landscape and of the mosquito population on the transmission of the vector-borne disease to humans and (ⅲ) assess different landscape designs and the treatment methods to prevent the transmission of mosquito-borne disease. Future work should focus on updating the methods to retrieve plausible parameter sets for the model.
The authors wish to thank the anonymous reviewer for his thoroughly reading the manuscript and for many helpful suggestions.
The authors declare that there is no conflict of interest.
[1] |
R. A. Fisher, The wave of advance of advantageous genes, Ann. Eugen., 7 (1937), 355–369. https://doi.org/10.1111/j.1469-1809.1937.tb02153.x doi: 10.1111/j.1469-1809.1937.tb02153.x
![]() |
[2] |
K. P. Hadeler, F. Rothe, Travelling fronts in nonlinear diffusion equations, J. Math. Biol., 2 (1975), 251–263. https://doi.org/10.1007/BF00277154 doi: 10.1007/BF00277154
![]() |
[3] |
V. Volpert, S. Petrovskii, Reaction-diffusion waves in biology, Phys. Life Rev., 6 (2009), 267–310. https://doi.org/10.1016/j.plrev.2009.10.002 doi: 10.1016/j.plrev.2009.10.002
![]() |
[4] |
Z. A. Wang, Mathematics of traveling waves in chemotaxis—review paper, Discrete Contin. Dyn. Syst. B, 18 (2013), 601. https://doi.org/10.3934/dcdsb.2013.18.601 doi: 10.3934/dcdsb.2013.18.601
![]() |
[5] |
J. Murray, W. Seward, On the spatial spread of rabies among foxes with immunity, J. Theor. Biol., 156 (1992), 327–348. https://doi.org/10.1016/S0022-5193(05)80679-4 doi: 10.1016/S0022-5193(05)80679-4
![]() |
[6] |
J. D. Murray, E. A. Stanley, D. L. Brown, On the spatial spread of rabies among foxes, Proc. R. Soc. London, Ser. B, 229 (1986), 111–150. https://doi.org/10.1098/rspb.1986.0078 doi: 10.1098/rspb.1986.0078
![]() |
[7] |
N. A. Maidana, H. M. Yang, Describing the geographic spread of dengue disease by traveling waves, Math. Biosci., 215 (2008), 64–77. https://doi.org/10.1016/j.mbs.2008.05.008 doi: 10.1016/j.mbs.2008.05.008
![]() |
[8] | L. Almeida, A. Leculier, N. Vauchelet, Analysis of the "rolling carpet" strategy to eradicate an invasive species, preprint, arXiv: 210611252. |
[9] | R. Anguelov, Y. Dumont, I. V. Y. Djeumen, On the use of traveling waves for pest/vector elimination using the sterile Insect technique, preprint, arXiv: 201000861. |
[10] |
A. M. Lutambi, M. A. Penny, T. Smith, N. Chitnis, Mathematical modelling of mosquito dispersal in a heterogeneous environment, Math. Biosci., 241 (2013), 198–216. https://doi.org/10.1016/j.mbs.2012.11.013 doi: 10.1016/j.mbs.2012.11.013
![]() |
[11] |
W. M. Yamashita, S. S. Das, G. Chapiro, Numerical modeling of mosquito population dynamics of Aedes aegypti, Parasites Vectors, 11 (2018), 1–14. https://doi.org/10.1186/s13071-018-2829-1 doi: 10.1186/s13071-018-2829-1
![]() |
[12] |
J. M. Knight, A model of mosquito-mangrove basin ecosystems with implications for management, Ecosystems, 14 (2011), 1382–1395. https://doi.org/10.1007/s10021-011-9487-x doi: 10.1007/s10021-011-9487-x
![]() |
[13] |
O. Richter, S. Moenickes, F. Suhling, Modelling the effect of temperature on the range expansion of species by reaction-diffusion equations, Math. Biosci., 235 (2012), 171–181. https://doi.org/10.1016/j.mbs.2011.12.001 doi: 10.1016/j.mbs.2011.12.001
![]() |
[14] |
C. Greppi, W. J. Laursen, G. Budelli, E. C. Chang, A. M. Daniels, L. Van Giesen, et al., Mosquito heat seeking is driven by an ancestral cooling receptor, Science, 367 (2020), 681–684. https://doi.org/10.1126/science.aay9847 doi: 10.1126/science.aay9847
![]() |
[15] |
J. Gaburro, P. N. Paradkar, M. Klein, A. Bhatti, S. Nahavandi, J. B. Duchemin, Dengue virus infection changes Aedes aegypti oviposition olfactory preferences, Sci. Rep., 8 (2018), 1–11. https://doi.org/10.1038/s41598-018-31608-x doi: 10.1038/s41598-018-31608-x
![]() |
[16] |
A. W. Brown, The attraction of mosquitoes to hosts, JAMA, 196 (1966), 249–252. https://doi.org/10.1001/jama.1966.03100160099028 doi: 10.1001/jama.1966.03100160099028
![]() |
[17] |
R. T. Cardé, Multi-cue integration: how female mosquitoes locate a human host, Curr. Biol., 25 (2015), R793–R795. https://doi.org/10.1016/j.cub.2015.07.057 doi: 10.1016/j.cub.2015.07.057
![]() |
[18] |
C. R. Lazzari, The thermal sense of blood-sucking insects: Why physics matters, Curr. Opin. Insect Sci., 34 (2019), 112–116. https://doi.org/10.1016/j.cois.2019.05.006 doi: 10.1016/j.cois.2019.05.006
![]() |
[19] |
F. Howlett, The influence of temperature upon the biting of mosquitoes, Parasitology, 3 (1910), 479–484. https://doi.org/10.1017/S0031182000002304 doi: 10.1017/S0031182000002304
![]() |
[20] |
C. J. McMeniman, R. A. Corfas, B. J. Matthews, S. A. Ritchie, L. B.Vosshall, Multimodal integration of carbon dioxide and other sensory cues drives mosquito attraction to humans, Cell, 156 (2014), 1060–1071. https://doi.org/10.1016/j.cell.2013.12.044 doi: 10.1016/j.cell.2013.12.044
![]() |
[21] |
R. A. Corfas, L. B. Vosshall, The cation channel TRPA1 tunes mosquito thermotaxis to host temperatures, Elife, 4 (2015), e11750. https://doi.org/10.7554/eLife.11750 doi: 10.7554/eLife.11750
![]() |
[22] |
G. Menda, J. H. Uhr, R. A. Wyttenbach, F. M. Vermeylen, D. M. Smith, L. C. Harrington, et al., Associative learning in the dengue vector mosquito, Aedes aegypti: avoidance of a previously attractive odor or surface color that is paired with an aversive stimulus, J. Exp. Biol., 216 (2013), 218–223. https://doi.org/10.1242/jeb.074898 doi: 10.1242/jeb.074898
![]() |
[23] |
C. Mwandawiro, M. Boots, N. Tuno, W. Suwonkerd, Y. Tsuda, M. Takagi, Heterogeneity in the host preference of Japanese encephalitis vectors in Chiang Mai, northern Thailand, Trans. R. Soc. Trop. Med. Hyg., 94 (2000), 238–242. https://doi.org/10.1016/S0035-9203(00)90303-1 doi: 10.1016/S0035-9203(00)90303-1
![]() |
[24] |
A. Vantaux, T. Lefèvre, K. R. Dabiré, A. Cohuet, Individual experience affects host choice in malaria vector mosquitoes, Parasites Vectors, 7 (2014), 1–7. https://doi.org/10.1186/1756-3305-7-249 doi: 10.1186/1756-3305-7-249
![]() |
[25] | E. Herbert, R. Meyer, P. Turbes, A comparison of mosquito catches with CDC light traps and CO2-baited traps in the Republic of Vietnam, Mosq. News, 32 (1972), 212–214. |
[26] | K. Huber, L. Le Loan, T. H. Hoang, T. K. Tien, F. Rodhain, A. B. Failloux, Aedes aegypti in South Vietnam: Ecology, genetic structure, vectorial competence and resistance to insecticides, Southeast Asian J. Trop. Med. Public Health, 34 (2003), 81–86. |
[27] |
J. A. L. Jeffery, N. Thi Yen, V. S. Nam, L. T. Nghia, A. A. Hoffmann, B. H. Kay, et al., Characterizing the Aedes aegypti population in a Vietnamese village in preparation for a Wolbachia-based mosquito control strategy to eliminate dengue, PLoS Neglected Trop. Dis., 3 (2009), e552. https://doi.org/10.1371/journal.pntd.0000552 doi: 10.1371/journal.pntd.0000552
![]() |
[28] |
A. Okubo, Dynamical aspects of animal grouping: swarms, schools, flocks, and herds, Adv. Biophys., 22 (1986), 1–94. https://doi.org/10.1016/0065-227X(86)90003-1 doi: 10.1016/0065-227X(86)90003-1
![]() |
[29] |
D. Getachew, H. Tekie, T. Gebre-Michael, M. Balkew, A. Mesfin, Breeding sites of Aedes aegypti: potential dengue vectors in Dire Dawa, East Ethiopia, Interdiscip. Perspect. Infect. Dis., 2015 (2015). https://doi.org/10.1155/2015/706276 doi: 10.1155/2015/706276
![]() |
[30] |
Q. Wang, P. M. Atkinson, Spatio-temporal fusion for daily Sentinel-2 images, Remote Sens. Environ., 204 (2018), 31–42. https://doi.org/10.1016/j.rse.2017.10.046 doi: 10.1016/j.rse.2017.10.046
![]() |
[31] |
R. S. Lees, J. R. Gilles, J. Hendrichs, M. J. B. Vreysen, K. Bourtzis, Back to the future: the sterile insect technique against mosquito disease vectors, Curr. Opin. Insect Sci., 10 (2015), 156–162. https://doi.org/10.1016/j.cois.2015.05.011 doi: 10.1016/j.cois.2015.05.011
![]() |
[32] |
D. Brown, A. Bruder, M. Kummel, Endogenous spatial heterogeneity in a multi-patch predator-prey system: insights from a field-parameterized model, Theor. Ecol., 14 (2021), 107–122. https://doi.org/10.1007/s12080-020-00483-6 doi: 10.1007/s12080-020-00483-6
![]() |
[33] |
M. R. Sanford, J. K. Tomberlin, Conditioning individual mosquitoes to an odor: sex, source, and time, PloS One, 6 (2011), e24218. https://doi.org/10.1371/journal.pone.0024218 doi: 10.1371/journal.pone.0024218
![]() |
[34] |
E. K. Lutz, C. Lahondere, C. Vinauger, A. Riffell, Olfactory learning and chemical ecology of olfaction in disease vector mosquitoes: A life history perspective, Curr. Opin. Insect Sci., 20 (2017), 75–83. https://doi.org/10.1016/j.cois.2017.03.002 doi: 10.1016/j.cois.2017.03.002
![]() |
[35] |
J. Charlwood, P. M. Graves, T. F. de Marshall, Evidence for a "memorized" home range in Anopheles farauti females from Papua New Guinea, Med. Vet. Entomol., 2 (1988), 101–108. https://doi.org/10.1111/j.1365-2915.1988.tb00059.x doi: 10.1111/j.1365-2915.1988.tb00059.x
![]() |
[36] |
P. McCall, F. Mosha, K. Njunwa, K. Sherlock, Evidence for memorized site-fidelity in Anopheles arabiensis, Trans. R. Soc. Trop. Med. Hyg., 95 (2001), 587–590. https://doi.org/10.1016/S0035-9203(01)90087-2 doi: 10.1016/S0035-9203(01)90087-2
![]() |
[37] |
O. A. Bruzzone, M. E. Utgés, Analysis of the invasion of a city by Aedes aegypti via mathematical models and Bayesian statistics, Theor. Ecol., 15 (2022), 1–16. https://doi.org/10.1007/s12080-022-00528-y doi: 10.1007/s12080-022-00528-y
![]() |
1. | San Migue, Tomás Valentín, Da Re Daniele, Andreo Verónica, A systematic review of Aedes aegypti population dynamics models based on differential equations, 2024, 0001706X, 107459, 10.1016/j.actatropica.2024.107459 | |
2. | Lutfiah Sania Sumardi, Irma Palupi, Hilda Fahlena, 2025, Simulating the Effectiveness of Wolbachia-Based Vector for Dengue in Bandung Using Disease Spread Model, 979-8-3315-1332-0, 1, 10.1109/ICADEIS65852.2025.10933111 |
Parameter | Notation | Value |
Carrying capacity | c | Maximum 0.8/m2 |
Diffusion coefficient | D | 1.25 103 m2/day |
Hatching rate | γ | 0.38/day Range (0.2, 0.5) |
Survival of hatched larvae | r | 0.5 |
Oviposition rate | ϕ | 25/day Range (10, 300) |
Mortality rate (mosquitoes, winged phase) | μM | 0.029/day |
Mortality rate (mosquitoes, aquatic phase) | μA | 0.055/day |
Attraction coefficient (humans) | χT | 1.7 × 104 |
Attraction coefficient (breeding sites) | χB | 2.4 × 104 |
Diffusion coefficient for attractants | DT DB |
1.0 × 103 m2/day |
contact rate for not virus bearing mosquitoes with infectious humans | ß1 | 1.65 m2/day |
Contact rate for infectious mosquito-susceptible human | ß2 | 3.75 m2/day |
Infectious period | τ−1 | 7 days |
Rate of change between mosquito states (only in the small-scale model) | ω12 | 90/day |
Parameter | Notation | Value |
Carrying capacity | c | Maximum 0.8/m2 |
Diffusion coefficient | D | 1.25 103 m2/day |
Hatching rate | γ | 0.38/day Range (0.2, 0.5) |
Survival of hatched larvae | r | 0.5 |
Oviposition rate | ϕ | 25/day Range (10, 300) |
Mortality rate (mosquitoes, winged phase) | μM | 0.029/day |
Mortality rate (mosquitoes, aquatic phase) | μA | 0.055/day |
Attraction coefficient (humans) | χT | 1.7 × 104 |
Attraction coefficient (breeding sites) | χB | 2.4 × 104 |
Diffusion coefficient for attractants | DT DB |
1.0 × 103 m2/day |
contact rate for not virus bearing mosquitoes with infectious humans | ß1 | 1.65 m2/day |
Contact rate for infectious mosquito-susceptible human | ß2 | 3.75 m2/day |
Infectious period | τ−1 | 7 days |
Rate of change between mosquito states (only in the small-scale model) | ω12 | 90/day |