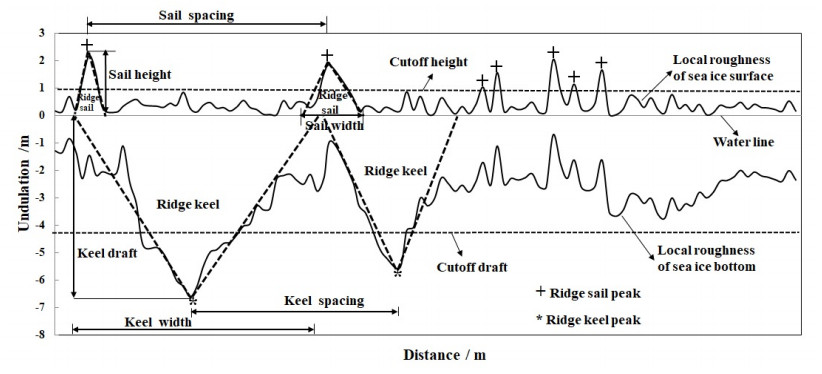
Optimal identification and numerical models are powerful tools that have been widely used in geoscience research for many years. In this study, we proposed a novel optimal method to simulate a key parameter (cutoff draft) of the ridge keels due to dynamic deformation of sea ice at bottom. The sea ice ridges were measured in the Northwestern Weddell Sea of Antarctic, by a helicopter-borne electromagnetic-induction (EM) system. An optimal model with nonlinear-statistical constraints was developed, by taking deviations between the theoretical and measured keel draft (spacing) distributions as the performance criterion, and cutoff draft as the identified parameter. The properties of the optimal model and the existence of the optimal parameter were demonstrated. We identified that the optimal cutoff draft was 3.78 m via an optimal numerical algorithm, this value was then employed to separate the ridge keels from the ice bottom. Finally, the relationship between the mean keel draft and frequency (number of keels per km) was analyzed, and the result showed that this relationship was modeled well by a logarithmic function with a correlation coefficient of 0.7. The present optimal modeling method will provide a new theoretical reference for separating accurately the ridge keels from undeformed sea ice bottom, and analyzing the relationship between the morphologies of sea ice surface and bottom and the inversions of sea ice bottom draft and ice thickness by the surface height.
Citation: Xingang Zhang, Bing Tan, Peng Lu, Bin Cheng, Ting Wang, Chunchun Gao, Zhijun Li. Numerical simulation for cutoff draft of sea ice ridge keels based on a novel optimal modeling with nonlinear-statistical constraints[J]. Electronic Research Archive, 2022, 30(5): 1708-1722. doi: 10.3934/era.2022086
[1] | Michael Barg, Amanda Mangum . Statistical analysis of numerical solutions to constrained phase separation problems. Electronic Research Archive, 2023, 31(1): 229-250. doi: 10.3934/era.2023012 |
[2] | Shasha Bian, Yitong Pei, Boling Guo . Numerical simulation of a generalized nonlinear derivative Schrödinger equation. Electronic Research Archive, 2022, 30(8): 3130-3152. doi: 10.3934/era.2022159 |
[3] | Sida Lin, Lixia Meng, Jinlong Yuan, Changzhi Wu, An Li, Chongyang Liu, Jun Xie . Sequential adaptive switching time optimization technique for maximum hands-off control problems. Electronic Research Archive, 2024, 32(4): 2229-2250. doi: 10.3934/era.2024101 |
[4] | Yun Ni, Jinqing Zhan, Min Liu . Topological design of continuum structures with global stress constraints considering self-weight loads. Electronic Research Archive, 2023, 31(8): 4708-4728. doi: 10.3934/era.2023241 |
[5] | Chao Ma, Hong Fu, Pengcheng Lu, Hongpeng Lu . Multi-objective crashworthiness design optimization of a rollover protective structure by an improved constraint-handling technique. Electronic Research Archive, 2023, 31(7): 4278-4302. doi: 10.3934/era.2023218 |
[6] | Xiaopeng Yi, Huey Tyng Cheong, Zhaohua Gong, Chongyang Liu, Kok Lay Teo . Dynamic optimization of a two-stage fractional system in microbial batch process. Electronic Research Archive, 2024, 32(12): 6680-6697. doi: 10.3934/era.2024312 |
[7] | N. U. Ahmed, Saroj Biswas . Optimal strategy for removal of greenhouse gas in the atmosphere to avert global climate crisis. Electronic Research Archive, 2023, 31(12): 7452-7472. doi: 10.3934/era.2023376 |
[8] | Chengyong Chen, Fagang Chang, Li Li, Wenqiang Dou, Changjing Xu . Optimization of intelligent compaction based on finite element simulation and nonlinear multiple regression. Electronic Research Archive, 2023, 31(5): 2775-2792. doi: 10.3934/era.2023140 |
[9] | Furong Xie, Yunkai Gao, Ting Pan, De Gao, Lei Wang, Yanan Xu, Chi Wu . Novel lightweight connecting bracket design with multiple performance constraints based on optimization and verification process. Electronic Research Archive, 2023, 31(4): 2019-2047. doi: 10.3934/era.2023104 |
[10] | Chunhua Chu, Yijun Chen, Qun Zhang, Ying Luo . Joint linear array structure and waveform design for MIMO radar under practical constraints. Electronic Research Archive, 2022, 30(9): 3249-3265. doi: 10.3934/era.2022165 |
Optimal identification and numerical models are powerful tools that have been widely used in geoscience research for many years. In this study, we proposed a novel optimal method to simulate a key parameter (cutoff draft) of the ridge keels due to dynamic deformation of sea ice at bottom. The sea ice ridges were measured in the Northwestern Weddell Sea of Antarctic, by a helicopter-borne electromagnetic-induction (EM) system. An optimal model with nonlinear-statistical constraints was developed, by taking deviations between the theoretical and measured keel draft (spacing) distributions as the performance criterion, and cutoff draft as the identified parameter. The properties of the optimal model and the existence of the optimal parameter were demonstrated. We identified that the optimal cutoff draft was 3.78 m via an optimal numerical algorithm, this value was then employed to separate the ridge keels from the ice bottom. Finally, the relationship between the mean keel draft and frequency (number of keels per km) was analyzed, and the result showed that this relationship was modeled well by a logarithmic function with a correlation coefficient of 0.7. The present optimal modeling method will provide a new theoretical reference for separating accurately the ridge keels from undeformed sea ice bottom, and analyzing the relationship between the morphologies of sea ice surface and bottom and the inversions of sea ice bottom draft and ice thickness by the surface height.
As a major component of the polar environment, sea ice cover affects the exchange of heat, energy, mass, and momentum between the atmosphere and the ocean in the polar regions [1,2,3,4]. Morphology of polar sea ice changes continuously under the influences of external driving forces, e.g., wind, ocean current and waves. The surface and bottom topographies of ridged sea ice differ greatly from that of other sea-ice types, and the most important morphological features of ridged sea ice are the ridge sails on surface and keels on bottom formed by the dynamic deformation, except for other roughness features [5,6]. The ridge sails/keels have major effects on the optimization of the ice thickness remote sensing algorithm based on sea ice roughness parameters and sea ice thermodynamics numerical simulation parameterization scheme, and the analysis of the relationship between polar sea ice and climate variations [7,8].
In general, the ridge sails/keels can be separated from sea ice surface/bottom undulations by a key parameter, namely, a cutoff height/draft: where any peaks lower than the cutoff height/draft are classified as non-ridged ice surface/bottom fluctuations, whereas other peaks exceeding the cutoff height/draft are defined as ridge sails/keels [9]. The sea ice morphology is shown in Figure 1 by approximating the ridge sail/keel cross-sections as the isoceles triangle, and a field photograph of sea ice ridge sails in Antarctic is shown in Figure 2. This height/draft affects estimations of the sea ice mass and thickness, and plays an important role in the establishment of large-scale sea ice dynamic models [10,11,12,13,14]. Therefore, the determination of the cutoff height/draft is an essential and significant stage for the analysis of the ridge morphologies and the improvement of sea ice thermodynamics numerical models. The Rayleigh criterion and in-situ measurement experience were always combined as a staple method to determine this cutoff height/draft [15,16]. However, the cutoff height/draft used in many previous studies were always arbitrary and empirical, resulting in confusions when comparing results from different observations, owing to no-upper limitation defined by the Rayleigh criterion. The cutoff height and morphology of the ice ridge sails were analyzed in our previous study [17]. So, a novel optimal modeling and method for the determination of the ridge keel cutoff draft are the main contribution of this research.
To seek an effective method for determining the keel cutoff draft and analyzing the pressure ridge morphology, data measured by a helicopter-borne electromagnetic-induction (EM) bird in northwestern Weddell Sea of Antarctic during the ANT-XXIII/7 cruise carried out by Alfred Wegener Institute for Polar and Marine Research were collected [10]. The length, diameter, and weight of the EM instrument were 3.5 m, 0.35 m, and 105 kg, and the sampling frequency was 10 Hz. During the cruise, total accumulated distance of measurement was nearly 3000 km, and EM survey flights were segmented into 94 profiles for the avoidance of open water in the survey areas. Different ice types and ice regimes in northwestern Weddell Sea were perfectly covered.
EM induction determines sea ice thickness remotely and has high precision and reliability based on the difference between the electrical properties of sea ice and sea water [11]. The observations provide ground validations to satellite remote sensing and numerical simulations [20], thus have been extensively employed in sea ice investigations [11,18,19,20,21,22,23,24]. Generally, sea ice thickness derived by EM induction are consistently reliable over undeformed ice, and usually within 10% of that by drilling [21]. While over rough and deformed ice, the estimations are possibly affected by the water within the porous keels and 3D structure of keels, which may lead to underestimation of the maximum keel draft [20]. However, the EM fields can penetrate through the water and are also sensitive to ice bottom further below. Thus, EM measurements can distinguish between thinner and deeper keels, and the rationality and validity of the EM data had been discussed by numerous researchers [11,18,20,22]. The detailed description of EM ice thickness measurement and data processing can refer to Haas et al. [10,11].
The accuracy of EM measurements was better than 0.1m over level ice, becoming lower for rough and deformed ice. For a helicopter flight speed varying between 80 and 90 knots, the spatial sampling distances of EM ranged from 3 to 4 m, respectively. The bottom draft is relative to the local level ice surface.
A novel optimal model with nonlinear-statistical constraints is proposed to find out a reasonable and effective value for the cutoff draft. The performance function consists of the relative deviation between the numerical results and the measured data of the keel draft, and that between the numerical results and the measured data of the keel spacing, and the cutoff draft is taken as the identified parameter. Then, the properties of the model and the existence of the optimal parameter are discussed. The optimal value of the cutoff draft is identified by a numerical algorithm and employed to separate the ridge keels from undeformed sea ice bottom. Finally, the correlation between the keel draft and frequency (number of ridge keels per km) is analyzed and discussed.
This paper is organized as follows. In Section 2, a novel optimal model with nonlinear-statistical constraints for the determination of the cutoff draft is established, based on the traditional models of the keel draft and spacing, the properties of the proposed model and the existence of the optimal parameter are proved. Moreover, the optimal cutoff draft is identified using a numerical algorithm and applied to separate the ridge keels from undeformed sea ice bottom in Section 3. Section 4 discusses the correlation between the keel draft and frequency in detail. Conclusions and some discussions are given in the final section.
To find out the optimal value of the cutoff draft and separate effectively ridge keels from other sea ice bottom morphology, a novel optimal model with nonlinear-statistical constrains is formulated, the properties and the existence of the optimal parameter are proved in this section.
The probability functions of the traditional keel spatial distributions are introduced firstly in the following.
Let the function fkd = fkd(d;dk - c,Φk) denote the theoretical density for the ridge keel draft, which is Lipchitz continuous, where d∈Dk is the keel draft with the set Dk:=[dk−c,dk−max], dk−c is the cutoff draft, dk−max∈R+ is the maximum keel draft and it is a limited constant (dk−c<dk−max), Φk is a parameter set related to the cutoff draft dk−c.
There are two traditional theoretical functions of the ice ridge keel draft distribution. One was presented by Hibler et al. [26] as follows.
fkd(d;dk - c,λ1)=2√λ1π⋅exp(−λ1d2)⋅1g(dk - c√λ1),d⩾dk - c,d∈Dk, | (1) |
where
g(x)=2π∫+∞xe−t2dt, | (2) |
and λ1 is the distribution shape parameter in set Φk, and related to the mean keel draft _d by the following equation.
_d=exp(−λ1dk - c2)⋅1√λ1π⋅g(dk - c√λ1). | (3) |
The other was advanced by Wadhams [27], who showed that the keel draft distribution can be fitted well by the following exponential function.
fkd(d;dk - c,λ2)=λ2⋅exp[−λ2(d−dk - c)],d⩾dk - c,d∈Dk, | (4) |
where λ2 is the distribution shape parameter in set Φk determined by
λ2−1=_d−dk - c. | (5) |
Set the function fks = fks(s;dk - c,Ψk) as the theoretical density for the ridge keel spacing, which is also Lipchitz continuous, where s∈Sk is the keel spacing, and the set Sk is defined as Sk:=[sk−min,sk−max], sk−min,sk−max∈R+ are the minimum and maximum keel spacings (sk−min<sk−max), respectively, and they are both limited constants, Ψk is a parameter set related to the cutoff draft dk−c.
One traditional function of the keel spacing distribution was presented by Hibler et al. [26] as the following exponential form.
fks(s;dk - c,λ3)=λ3⋅exp(−λ3s),d⩾dk - c,d∈Dk,s∈Sk, | (6) |
where λ3∈Φk related to the mean keel spacing _s by the following equation.
λ3=_s−1. | (7) |
Following, a lognormal distribution density function was proposed and showed fitted better the keel spacing by the by Wadhams and Davy [28].
fks(s;dk - c,θk,μk,σk)=exp[−(ln(s - θk)−μk)22σk2]⋅1√2π⋅σk⋅(s−θk) , s>θk,d⩾dk - c,d∈Dk,s∈Sk, | (8) |
where θk is the shift parameter, μk and σk are the mean and standard error of ln(s - θk), respectively, and determined by the following equation.
_s = θk+exp(μk+σk22). | (9) |
To find out the optimal value of the cutoff draft and separate accurately the ridge keels from the local roughness of sea ice bottom, a novel optimal model with nonlinear-statistical constrains is formulated, in which, the deviation between the numerical results and the measured data of the keel draft and that between the numerical results and the measured data of the spacing are combined as the performance criterion, and the cutoff draft dk−c is the identified parameter. Then, the properties of the proposed model and the existence of the optimal parameter are discussed in this section.
Let Ekd(dk−c) and Eks(dk−c) be the relative deviation between the theoretical function and the measured keel draft distribution and that between the theoretical function and the measured keel spacing distribution, respectively, and described by the following equations.
Ekd(dk−c):=n∑i=1‖ | (10) |
(11) |
where and are the measured keel draft and spacing corresponding to the cutoff draft , respectively, and are the probability densities of the measured ridge keel draft and spacing , and and .
Let be the identified parameter, where is the admissible parameter set limited by the Rayleigh criterion and cover a sufficient range of the measured ridge keel drafts.
(12) |
Obviously, the admissible parameter set is a nonempty, bounded, closed, and convex.
For simplicity, Eq (1) is referred to as Hibler'72 function and Eq (4) as Wadhams'80 function in the following study. The initial cutoff draft of m and step increment of m are employed to search for the best fits to the measured ridge keel draft and spacing distributions. The results in Figure 3 indicate that for any cutoff draft in the parameter set , the Wadhams'80 and lognormal functions yield the best fits to the measured keel draft and spacing distributions, respectively.
Effects of the ridge sail and spacing on the sail intensity (the ratio of the sail height to spacing) were very different, and the contribution of sail spacing is much larger than that of sail height in general [17]. To comprehensively evaluate the effects of the cutoff draft on both the measured keel draft and spacing distributions, the following performance criterion is defined by considering the different effects of the keel draft and spacing on the keel intensity (the ratio of the keel draft to spacing).
(13) |
with
(14) |
(15) |
where is the performance function, is the relative deviation between the Wadhams'80 function and the measured keel draft distribution, is the relative deviation between the lognormal function and the measured keel spacing distribution, is the probability density function of the Wadhams'80 distribution for the keel draft [refer to Eq (4)], and is the probability density function of the lognormal distribution for the keel spacing [refer to Eq (8)], and . A novel optimal model with nonlinear-statistical constraints (NOPM-NS) is then established as follows.
(16) |
For the strictness of the above model (NOPM-NS), namely, the existence of the optimal cutoff draft, we set
(17) |
and
(18) |
Then,
(19) |
Set
(20) |
and
(21) |
Then,
(22) |
Define the feasible region set of the model (NOPM-NS) as
(23) |
Theorem 1. The feasible region set defined by Eq (23) of the model (NOPM-NS) is compact.
Proof: Let be the set of positive real numbers. Obviously, the admissible parameter set , and is nonempty, bounded and closed, namely, it is compact. The probability density function is continuous on the parameter , so, the set is compact.
Additionally, is continuous on the parameter based on the definition of Eq (5), it is clear that the set is also compact. Therefore, the set is obviously compact based on Eq (19).
Similarly, we can also prove that the set defined by Eq (22) is compact. Therefore, the feasible region set in Eq (23) is compact, namely, the feasible region set of the novel optimal model (NOPM-NS) is compact.
Theorem 2. The performance function of the model (NOPM-NS) is continuous in the feasible region set .
Proof: The admissible parameter set is nonempty, bounded, closed and convex, and the function is continuous on the parameter , so, the relative deviation function is continuous on the parameter according to its definition [refer to Eq (14)].
Similarly, the relative deviation function is continuous on the parameter according to its definition [refer to Eq (15)].
Therefore, combining with the definition [refer to Eq (13)], the performance function of the novel model (NOPM-NS) is continuous in the feasible region set .
From the above two theorems, we can obtain the following theorem easily.
Theorem 3. , such that , holds. Namely, the optimal solution of the model (NOPM-NS) exists.
It is known that the optimal solution of the optimal model (NOPM-NS) exists from Theorem 3. A numerical algorithm is developed for the determination of the optimal cutoff draft and result is analyzed in this section.
The linear search method is applied to make the performance function of the novel optimal model (NPM-NS) attain its minimum, and the numerical algorithm is described as follows.
Step 1. Initialize: (cutoff draft), (step increment), and (maximum of the cutoff draft). Set .
Step 2. Import: measured keel draft () and spacing (), mean keel draft and spacing .
Step 3. Identify: the parameters , , and by Eqs (5) and (9), respectively.
Step 4. Calculate:, and by Eqs (4), (8) and (16) (model NOPM-NS), respectively.
Step 5. Set , if , go to Step 2. Else, Set
Step 6. If , set , stop. Else, set , go to Step 5.
Set the initial cutoff draft , step increment , and maximum of the cutoff draft . The result of the above numerical algorithm shows that the optimal cutoff draft of the ridge keels is m. The variations in the relative deviations and , and the performance function of the novel optimal model (NOPM-NS) as the cutoff draft increase are all shown in Figure 4. It is obvious that and , and all reach their minima at the cutoff draft of 3.78 m. According to novel optimal model (NOPM-NS), m should be taken as the optimal cutoff draft.
Granberg and Leppäranta [29] proposed that the cutoff height should be much larger than the standard deviation of the ice surface elevation or larger than twice this value. In the present study, the same method is applied to assess the keel cutoff draft. The standard deviation of the ice bottom draft is m and it is obvious that the optimal cutoff draft m is much larger than twice of the standard deviation . So, this optimal cutoff draft is taken on as the lower limit of the keel peak and the deepest point of a ridge keel is defined as its peak (Figure 5). Davis and Wadhams [30] set the cutoff draft as 5 m to analyze the Arctic ridge keel morphology. Ekeberg et al. [15] also obtained a cutoff draft of 5 m by the Rayleigh criterion based the upward looking sonar data in the Fram Strait. Obert and Brown [31] analyzed the morphologies of 3199 ridge keels in Northumberland Strait of Arctic with a cutoff draft of 2 m. The different cutoff draft results of the present and previous studies are likely caused by a combination of the following reasons: 1) the physical properties of the ice/ridge are directly related to the seasonal and interannual variations. 2) Different dynamical processes might lead to differences in the dominant formation mechanisms of the ridges in different regions. 3) Differences in the methods of determining the cutoff draft.
The draft and frequency (numbers of keels per km) denote the vertical and level features of ridge keels, respectively. To accurately describe the keel morphology and spatial distribution, it is necessary to analyze the relationship between the mean keel draft and frequency, as shown in Figure 6. There was scatter but the obvious logarithmic relationship yielded a correlation coefficient of 0.7 between the mean keel draft and frequency with reasonable confidence. This logarithmic function showed that the mean keel draft increased logarithmically, while the ratio of the increments in the keel draft and frequency decreased as the corresponding frequency increased. Moreover, according to the measured data shown in Figure 6, for a given increase in ridge frequency, the increases in the keel spacing and draft were both greater in the lower ridging intensity regime than the larger regime. In addition, the logarithmic function showed that the mean ridge keel varied much less than the mean spacing, reflect well the real observation. Therefore, the proposed logarithmic function is a suitable relationship for describing the ridge keel morphology.
Based on helicopter-borne EM data collected in northwestern Weddell Sea, we determine the best fit to measured ridge keel draft distribution which is represented by the Wadhams'80 function (refer to Eq (4)), and the spacing is achieved by a lognormal function(refer to Eq (8)).
We propose a novel optimal model with nonlinear-statistical constraints (NOPM-NS) to determine the optimal cutoff draft for ridge keel, by minimizing the deviations of the theoretical and measured ridge keel draft and spacing distributions, and the cutoff draft is taken as the identified variable. The properties of the novel model (NOPM-NS) and the existence of the optimal parameter are proved, and the optimal value of cutoff draft is identified by a developed numerical algorithm as m, which is employed to separate the ridge keels from undeformed sea ice bottom.
We obtain a logarithmic relationship yielded a correlation coefficient of 0.70 between the mean keel draft and frequency in the present study, with a 95% confidence degree. This relationship indicates that there is a general increase in the mean keel draft as the mean keel frequency increases, whereas the ratios of the increments in the keel draft and frequency decrease as the ridge frequency increases.
Due to climate change, sea ice in the polar region subject to significant deformation because of thinner ice thickness and smaller ice concentration. Ice ridge parameterization is getting more attention and become more important in sea ice dynamic modeling. Therefore, the present novel optimal model (NOPM-NS) for determining the cutoff draft has the potential to be applied for the separation of ridge keels from other ice bottom undulations in any sea-ice region, and we believe that it is a promising approach worthy of further studies under different environmental conditions in the polar regions. We expect this study can provide more accurate standard as analyzing data of sea ice thickness, benefiting to a comprehensive understand on ice mass balance.
It must be noted that the present optimal model (NOPM-NS) and method of analyses are proposed for helicopter-borne EM data. We focus on the mathematical theoretic method in data analyses, and the possible inaccuracies of EM for deformed ice is somewhat beyond the scope of this study. However, more validations to the universality and effectivity of EM data especially on deformed ice, are still necessary by using other instruments, e.g., upword looking sonar or radar, and will be conducted in our future work.
We would like to thank Dr. Christian Haas and Dr. Marcel Nicolaus from the Alfred Wegener Institute for Polar and Marine Research (AWI) for providing the valuable EM data, Dr. Qingkai Wang for providing the field photograph of sea ice ridge sails during the 36th Antarctic scientific expedition of china. The Arctic and Antarctic Administration is also thanked for supporting the participation of Zhijun Li in the expedition organized by AWI. This work was supported by the National Nature Science Foundation of China (Nos. 41876219, 41922045, 42074094), the Key Science Foundation for Universities of Henan Province (No. 22A110017), and the project of Nanyang Normal University (STP2017023, 2022STP014).
The authors declare there is no conflict of interest.
[1] |
Q. Yang, S. N. Losa, M. Losch, X. Tian-Kunze, L. Nerger, J. Liu, et al., Assimilating SMOS sea ice thickness into a coupled ice-ocean model using a local SEIK filter, JGR: Oceans, 119 (2014), 6680–6692. https://doi.org/10.1002/2014JC009963 doi: 10.1002/2014JC009963
![]() |
[2] |
Q. Bai, R. Li, Z. Li, M. Leppäranta, L. Arvola, M. Li, Time-series analyses of water temperature and dissolved oxygen concentration in Lake Valkea-Kotinen (Finland) during ice season, Eco. Inf.s, 36 (2016), 181–189. https://doi.org/10.1016/j.ecoinf.2015.06.009 doi: 10.1016/j.ecoinf.2015.06.009
![]() |
[3] |
L. Zhou, J. Gao, D. Li, An engineering method for simulating dynamic interaction of moored ship with first-year ice ridge, Ocean Eng., 171 (2019), 417–428. https://doi.org/10.1016/j.oceaneng.2018.11.027 doi: 10.1016/j.oceaneng.2018.11.027
![]() |
[4] |
R. Lei, D. Gui, P. Heil, J. K. Hutchings, M. Ding, Comparisons of sea ice motion and deformation, and their responses to ice conditions and cyclonic activity in the western Arctic Ocean between two summers, Cold Reg. Sci. Technol., 170(2020), 102925. https://doi.org/10.1016/j.coldregions.2019.102925 doi: 10.1016/j.coldregions.2019.102925
![]() |
[5] |
T. Martin, Comparison of different ridge formation models of Arctic sea ice with observations from laser profiling, Ann. Glaciol., 44 (2006), 403–410. https://doi.org/10.3189/172756406781811132 doi: 10.3189/172756406781811132
![]() |
[6] |
K. V. Høyland, Consolidation of first-year sea ice ridges, JGR: Oceans, 107 (2002), 15-1–15-16. https://doi.org/10.1029/2000JC000526 doi: 10.1029/2000JC000526
![]() |
[7] |
R. Lei, M. Leppäranta, B. Cheng, P. Heil, Z. Li, Changes in ice-season characteristics of a European Arctic lake from 1964 to 2008, Clim. Change, 115 (2012), 725–739. https://doi.org/10.1007/s10584-012-0489-2 doi: 10.1007/s10584-012-0489-2
![]() |
[8] |
Y. Zu, P. Lu, M. Leppäranta, B. Cheng, Z. Li, On the form drag coefficient under ridged ice: Laboratory experiments and numerical simulations from ideal scaling to deep water, JGR: Ocean, 126 (2021), e2020JC016976. https://doi.org/10.1029/2020JC016976. doi: 10.1029/2020JC016976
![]() |
[9] | M. Leppäranta, The drift of sea ice, 2nd edition, Springer, Berlin, (2011), 347. https://doi.org/10.1007/978-3-642-04683-4_4 |
[10] | C. Haas, A. Friedrich, Z. Li, M. Nicolaus, A. Pfaffling, T. Toyota, Regional variability of sea ice properties and thickness in the northwestern Weddell Sea obtained by in-situ and satellite measurements, in Peter Lemke, The Expedition of the Research Vessel "Polarstern" to the Antarctic in 2006 (ANT-XXX Ⅲ/7), Reports on Polar and Marine Research, 586 (2009), 36–74. |
[11] |
C. Haas, J. Lobach, S. Hendricks, L. Rabenstein, A. Pfaffling, Helicopter-borne measurements of sea ice thickness, using a small and lightweight, digital EM system, J. Appl. Geophys., 67 (2009), 234–241. https://doi.org/10.1016/j.jappgeo.2008.05.005 doi: 10.1016/j.jappgeo.2008.05.005
![]() |
[12] |
R. Lei, X. Tian-Kunze, B. Li, P. Heil, Z. Tian, Characterization of summer Arctic sea ice morphology in the 135°-175°W sector using multi-scale methods, Cold Reg. Sci. Technol., 133 (2017), 108–120. https://doi.org/10.1016/j.coldregions.2016.10.009 doi: 10.1016/j.coldregions.2016.10.009
![]() |
[13] |
B. Tan, L. Wang, P. Lu, Z. Li, A novel strategy to analyze the form drag on pressure ridges and air-ice drag coefficient in northwestern Weddell Sea, Appl. Math. Model., 58 (2018), 158–165. https://doi.org/10.1016/j.apm.2017.09.046 doi: 10.1016/j.apm.2017.09.046
![]() |
[14] |
R. B. Guzenko, Y. U. Mironov, R. May, V. S. Porubaev, P. А. Tarasov. Morphometry and internal Structure of ice ridges in the Kara and Laptev Seas, Int. J. Offshore Polar, 30 (2020), 194–201. https://doi.org/10.17736/ijope.2020.jc784 doi: 10.17736/ijope.2020.jc784
![]() |
[15] |
O. Ekeberg, K. Høyland, E. Hansen, Ice ridge keel geometry and shape derived from one year of upward looking sonar data in the Fram Strai, Cold Reg. Sci. Technol., 109 (2015), 78–86. https://doi.org/10.1016/j.coldregions.2014.10.003 doi: 10.1016/j.coldregions.2014.10.003
![]() |
[16] |
T. M. O. Jeffries. Morphology of deformed first-year sea ice features in the southern ocean, Cold Reg. Sci. Technol., 36 (2003), 141–163. https://doi.org/10.1016/S0165-232X(03)00008-9 doi: 10.1016/S0165-232X(03)00008-9
![]() |
[17] |
B. Tan, Z. Li, P. Lu, C. Haas, M. Nicolaus, Morphology of sea ice pressure ridges in the Northwestern Weddell Sea in winter, JGR: Oceans, 117 (2012), C06024. https://doi.org/10.1029/2011JC007800 doi: 10.1029/2011JC007800
![]() |
[18] |
H. Eicken, W. Tucker, D. Perovich, Indirect measurements of the mass balance of summer Arctic sea ice with an electromagnetic induction technique, Ann. Glaciol., 33 (2001), 194–200. https://doi.org/10.3189/172756401781818356 doi: 10.3189/172756401781818356
![]() |
[19] |
A. Renner, S. Hendricks, S. Gerland, J. Beckers, C. Haas, T. Krumpen, Large-scale ice thickness distribution of first-year sea ice in spring and summer north of Svalbard, Ann. Glaciol., 54 (2013), 13–18. https://doi.org/10.3189/2013AoG62A146 doi: 10.3189/2013AoG62A146
![]() |
[20] |
B. A. Lange, J. F. Beckers, J. A. Casey, C. Haas, Airborne observations of summer thinning of multiyear sea ice originating from the lincoln sea, JGR: Oceans, 124 (2019). https://doi.org/10.1029/2018JC014383 doi: 10.1029/2018JC014383
![]() |
[21] |
A. P. Worby, P. W. Griffin, V. I. Lytle, R. A. Massom, On the use of electromagnetic induction sounding to determine winter and spring sea ice thickness in the Antarctic, Cold Reg. Sci. Technol., 29 (1999), 49–58. https://doi.org/10.1016/S0165-232X(99)00003-8 doi: 10.1016/S0165-232X(99)00003-8
![]() |
[22] |
C. Haas, Evalation of ship-based electromagnetic-inductive thickness measurements of summer sea-ice in the Bellingshausen and Amundsen seas, Antarctica, Cold Reg. Sci. Technol., 27 (1998), 1–16. https://doi.org/10.1016/S0165-232X(97)00019-0 doi: 10.1016/S0165-232X(97)00019-0
![]() |
[23] |
J. E. Reid, A. Pfaffling, A. P. Worby, J. R. Bishop, In situ measurements of the direct-current conductivity of antarctic sea ice: implications for airborne electromagnetic sounding of sea-ice thickness, Ann. Glaciol., 44 (2006), 217–223. https://doi.org/10.3189/172756406781811772 doi: 10.3189/172756406781811772
![]() |
[24] |
J. Guo, S. Bo, G. Tian, The application of electromagnetic-induction on the measurement of sea ice thickness in the Antarctic, Appl. Geophys., 4 (2007), 214–220. https://doi.org/10.1007/s11770-007-0024-9 doi: 10.1007/s11770-007-0024-9
![]() |
[25] |
C. Wang, J. Negrel, S. Gerland, D. V. Divine, P. Dodd, M. A. Granskog, Thermodynamics of fast ice off the northeast coast of greenland (79°n) over a full year (2012-2013), JGR: Oceans, 125 (2020). https://doi.org/10.1029/2019JC015823 doi: 10.1029/2019JC015823
![]() |
[26] |
W. D. Hibler, W. F. Weeks, S. J. Mock, Statistical aspects of sea ice ridge distributions, JGR, 77 (1972), 5954–5970. https://doi.org/10.1029/JC077i030p05954 doi: 10.1029/JC077i030p05954
![]() |
[27] | P. Wadhams, A comparison of sonar and laser profiles along corresponding tracks in the Arctic Ocean, in Sea Ice Processes and Models, University of Washington Press, (1980), 283–299. |
[28] |
P. Wadhams, T. Davy, On the spacing and draft distributionfor pressure ridge keels, JGR, 91 (1986), 10697–10708. https://doi.org/10.1029/JC091iC09p10697 doi: 10.1029/JC091iC09p10697
![]() |
[29] |
H. B. Granberg, M. Leppäranta, Observations of sea ice ridging in the Weddell Sea, JGR: Oceans, 104 (1999), 25735–25745. https://doi.org/10.1029/1999jc900160 doi: 10.1029/1999jc900160
![]() |
[30] |
N. R. Davis, P. Wadhams, A statistical analysis of arctic pressure ridge morphology, JGR: Oceans, 100.C6 (1995), 10915. https://doi.org/10.1029/95JC00007 doi: 10.1029/95JC00007
![]() |
[31] |
K. M. Obert, T. G. Brown, Ice ridge keel characteristics and distribution in the northumberland strait, Cold Reg. Sci. Technol., 66 (2011), 53–64. https://doi.org/10.1016/j.coldregions.2011.01.004 doi: 10.1016/j.coldregions.2011.01.004
![]() |