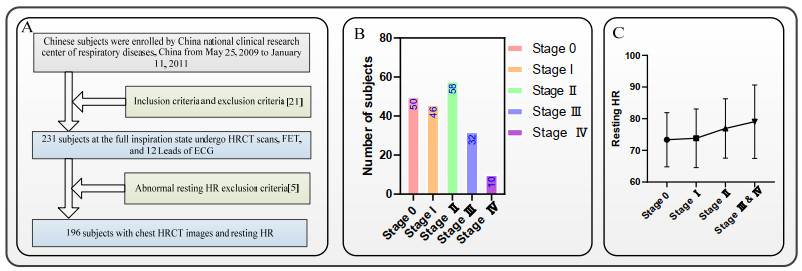
The resting HR is an upward trend with the development of chronic obstructive pulmonary disease (COPD) severity. Chest computed tomography (CT) has been regarded as the most effective modality for characterizing and quantifying COPD. Therefore, CT images should provide more information to analyze the lung and heart relationship. The relationship between HR variability and PFT or/and COPD has been fully revealed, but the relationship between resting HR variability and COPD radiomics features remains unclear. 231 sets of chest high-resolution CT (HRCT) images from "COPD patients" (at risk of COPD and stage I to IV) are segmented by the trained lung region segmentation model (ResU-Net). Based on the chest HRCT images and lung segmentation images, 231 sets of the original lung parenchyma images are obtained. 1316 COPD radiomics features of each subject are calculated by the original lung parenchyma images and its derived lung parenchyma images. The 13 selected COPD radiomics features related to the resting HR are generated from the Lasso model. A COPD radiomics features combination strategy is proposed to satisfy the significant change of the lung radiomics feature among the different COPD stages. Results show no significance between COPD stage Ⅰ and COPD stage Ⅱ of the 13 selected COPD radiomics features, and the lung radiomics feature Y1-Y4 (P > 0.05). The lung radiomics feature F2 with the dominant selected COPD radiomics features based on the proposed COPD radiomics features combination significantly increases with the development of COPD stages (P < 0.05). It is concluded that the lung radiomics feature F2 with the dominant selected COPD radiomics features not only can characterize the resting HR but also can characterize the COPD stage evolution.
Citation: Yingjian Yang, Wei Li, Yan Kang, Yingwei Guo, Kai Yang, Qiang Li, Yang Liu, Chaoran Yang, Rongchang Chen, Huai Chen, Xian Li, Lei Cheng. A novel lung radiomics feature for characterizing resting heart rate and COPD stage evolution based on radiomics feature combination strategy[J]. Mathematical Biosciences and Engineering, 2022, 19(4): 4145-4165. doi: 10.3934/mbe.2022191
[1] | Yingjian Yang, Wei Li, Yingwei Guo, Nanrong Zeng, Shicong Wang, Ziran Chen, Yang Liu, Huai Chen, Wenxin Duan, Xian Li, Wei Zhao, Rongchang Chen, Yan Kang . Lung radiomics features for characterizing and classifying COPD stage based on feature combination strategy and multi-layer perceptron classifier. Mathematical Biosciences and Engineering, 2022, 19(8): 7826-7855. doi: 10.3934/mbe.2022366 |
[2] | Yutao Wang, Qian Shao, Shuying Luo, Randi Fu . Development of a nomograph integrating radiomics and deep features based on MRI to predict the prognosis of high grade Gliomas. Mathematical Biosciences and Engineering, 2021, 18(6): 8084-8095. doi: 10.3934/mbe.2021401 |
[3] | Peng An, Xiumei Li, Ping Qin, YingJian Ye, Junyan Zhang, Hongyan Guo, Peng Duan, Zhibing He, Ping Song, Mingqun Li, Jinsong Wang, Yan Hu, Guoyan Feng, Yong Lin . Predicting model of mild and severe types of COVID-19 patients using Thymus CT radiomics model: A preliminary study. Mathematical Biosciences and Engineering, 2023, 20(4): 6612-6629. doi: 10.3934/mbe.2023284 |
[4] | Jingren Niu, Qing Tan, Xiufen Zou, Suoqin Jin . Accurate prediction of glioma grades from radiomics using a multi-filter and multi-objective-based method. Mathematical Biosciences and Engineering, 2023, 20(2): 2890-2907. doi: 10.3934/mbe.2023136 |
[5] | Jiaxi Lu, Yingwei Guo, Mingming Wang, Yu Luo, Xueqiang Zeng, Xiaoqiang Miao, Asim Zaman, Huihui Yang, Anbo Cao, Yan Kang . Determining acute ischemic stroke onset time using machine learning and radiomics features of infarct lesions and whole brain. Mathematical Biosciences and Engineering, 2024, 21(1): 34-48. doi: 10.3934/mbe.2024002 |
[6] | Qian Shao, Rongrong Xuan, Yutao Wang, Jian Xu, Menglin Ouyang, Caoqian Yin, Wei Jin . Deep learning and radiomics analysis for prediction of placenta invasion based on T2WI. Mathematical Biosciences and Engineering, 2021, 18(5): 6198-6215. doi: 10.3934/mbe.2021310 |
[7] | Jun Gao, Qian Jiang, Bo Zhou, Daozheng Chen . Convolutional neural networks for computer-aided detection or diagnosis in medical image analysis: An overview. Mathematical Biosciences and Engineering, 2019, 16(6): 6536-6561. doi: 10.3934/mbe.2019326 |
[8] | Chen Ma, Zhihao Yao, Qinran Zhang, Xiufen Zou . Quantitative integration of radiomic and genomic data improves survival prediction of low-grade glioma patients. Mathematical Biosciences and Engineering, 2021, 18(1): 727-744. doi: 10.3934/mbe.2021039 |
[9] | Chengkang Li, Ran Wei, Yishen Mao, Yi Guo, Ji Li, Yuanyuan Wang . Computer-aided differentiates benign from malignant IPMN and MCN with a novel feature selection algorithm. Mathematical Biosciences and Engineering, 2021, 18(4): 4743-4760. doi: 10.3934/mbe.2021241 |
[10] | Binju Saju, Neethu Tressa, Rajesh Kumar Dhanaraj, Sumegh Tharewal, Jincy Chundamannil Mathew, Danilo Pelusi . Effective multi-class lungdisease classification using the hybridfeature engineering mechanism. Mathematical Biosciences and Engineering, 2023, 20(11): 20245-20273. doi: 10.3934/mbe.2023896 |
The resting HR is an upward trend with the development of chronic obstructive pulmonary disease (COPD) severity. Chest computed tomography (CT) has been regarded as the most effective modality for characterizing and quantifying COPD. Therefore, CT images should provide more information to analyze the lung and heart relationship. The relationship between HR variability and PFT or/and COPD has been fully revealed, but the relationship between resting HR variability and COPD radiomics features remains unclear. 231 sets of chest high-resolution CT (HRCT) images from "COPD patients" (at risk of COPD and stage I to IV) are segmented by the trained lung region segmentation model (ResU-Net). Based on the chest HRCT images and lung segmentation images, 231 sets of the original lung parenchyma images are obtained. 1316 COPD radiomics features of each subject are calculated by the original lung parenchyma images and its derived lung parenchyma images. The 13 selected COPD radiomics features related to the resting HR are generated from the Lasso model. A COPD radiomics features combination strategy is proposed to satisfy the significant change of the lung radiomics feature among the different COPD stages. Results show no significance between COPD stage Ⅰ and COPD stage Ⅱ of the 13 selected COPD radiomics features, and the lung radiomics feature Y1-Y4 (P > 0.05). The lung radiomics feature F2 with the dominant selected COPD radiomics features based on the proposed COPD radiomics features combination significantly increases with the development of COPD stages (P < 0.05). It is concluded that the lung radiomics feature F2 with the dominant selected COPD radiomics features not only can characterize the resting HR but also can characterize the COPD stage evolution.
The resting heart rate (HR, beats per minute) variability is an important marker of the heart's neurophysiologic condition [1]. The resting HR can be measured to reflect the level of cardiopulmonary. Lung disease is a complex and diverse disease. As a common and non-infectious lung disease, chronic obstructive pulmonary disease (COPD) presents a preventable, treatable and progressive chronic disease with debilitating lung conditions characterized by persistent airflow limitation [2,3]. Due to the persistent airflow limitation of COPD, autonomic regulation of resting HR can be influenced [4]. Compared with people without COPD, patients with COPD cannot get enough air, which may increase the resting HR to get enough oxygen supply. As a result, the resting HR is an upward trend with the development of COPD severity [5]. After using a bronchodilator, the pulmonary function test (PFT) can assess COPD severity. The assessment parameters in PFT are the forced expiratory volume in 1 second/forced vital capacity (FEV1/FVC), and FEV1 % predicted [6]. The HR variability in COPD patients has been deeply studied [1,5,7,8]. There is no correlation between resting HR variability and FEV1 in COPD patients [1]. However, the COPD stage is determined by FEV1/FVC, and FEV1% predicted, and the relationship between the resting HR variability and the COPD stage is not revealed. Because the resting HR is an upward trend with the development of COPD severity, the resting HR can improve the risk prediction in COPD patients beyond that of PFT alone [5]. The HR is analyzed in the process of the Chester step tests to determine reliability and correlation with PFT results in COPD patients, according to advanced stages of the Chester step test and the number of steps [7], respectively. The effect of pulmonary rehabilitation on HR variability at peak exercise is revealed in COPD patients [8]. Symbolic analysis and complexity index of the HR variability are analyzed to assess cardiac autonomic modulation in COPD patients [9]. Association between the predictors of functional capacity and HR off-kinetics is also studied in COPD patients [10]. The relationship of the HR variability and the severity of COPD in PiZ alpha1-antitrypsin deficiency has also been revealed [11]. As the gold standard, PFT can only diagnose and evaluate COPD [6], but it cannot get the anatomical structure of the lung region. PFT may cause missed diagnosis of early-stage COPD or overdiagnosis in primary care [12]. Compared with PFT, chest computed tomography (CT) images can provide more information, such as the specific anatomical structure of the lung, the location, and the morphology of diseases. Chest CT images can provide the lung anatomical structure, including the trachea, the blood vessels, pulmonary lobes, and lung texture information. The lung anatomical structure and texture information can be quantitatively calculated from chest CT images to further analyze COPD [13,14,15]. Therefore, CT has been regarded as the most effective modality for characterizing and quantifying COPD [16].
Lung radiomics features calculated from chest CT images have been used in the spirometric assessment of emphysema presence and COPD severity [17]. The emerging role of radiomics in COPD has also been proposed [18]. The COPD radiomics features can provide feature classes about COPD extracted from original and derived images. Although the HR variability in COPD [1,5,7,8,9,10] has been fully studied, the relationship between the resting HR variability and COPD radiomics features remains unclear. Our contributions in this paper are briefly described as follows:
• The relationship between the COPD radiomics features and the resting HR is revealed.
• A new COPD radiomics feature combination algorithm is proposed to improve the significance among different COPD stages.
• A novel lung radiomics features with the dominant selected COPD radiomics features characterize both the resting heart rate and the COPD stage evolution.
This Section describes the Materials in Section 2.1 and methods in Section 2.2 (Figures 1−4, Eqs (1)−(3) and Table 1).
Detailed implementation process | |
Input | Coefficient array [β1, β2, …., βi] Initialization: preset significant condition (P = 0.005) |
Output | Lung radiomics feature Flung radiomics; N |
Computation | Absolute value operation for the coefficient array [|β1|, |β2|, …., |βi|] Sorting operation for the coefficient array [max {|β1|, |β2|, …., |βi|}, …., min {|β1|, |β2|, …., |βi|}] for N = 2; N ≤ 13; N ← N + 1 do { Form a new coefficient array →βN=[max{|β1|, |β2|, …., |βi|},….,min{|β1|, |β2|, …., |βi|}]⏟N Construct the candidate lung radiomics feature ZN=N∑i=1→βN·xi (4) Calculate the significances of ZN among different COPD stages if all significances < (P = 0.05) Flung radiomics=ZN break from for end if } end for return {Flung radiomics; N } |
Figure 1 shows the Chinese subjects selection flow diagram, the number of subjects at different COPD stages, and the changing trend of resting HR with COPD stage evolution. 231 Chinese subjects aged 40−79 are included in this study. The 231 subjects who rigorously followed the inclusion and exclusion criteria were enrolled in the national clinical research center of respiratory diseases [21]. The 231 subjects with full inspiration underwent chest high-resolution CT (HRCT) scans (manufacturer: TOSHIBA, kVp:120 kV, X-ray tube current:40 mA, slice thinkness:1.0 mm, window center: −600, window width: 1250) from May 25, 2009, to January 11, 2011, are included in this study. In addition, the 231 subjects, after 15 minutes of rest, underwent 12 Leads of ECG on the same day for the resting HR measurement.
The resting HR of all subjects affected by COPD and the abnormal resting HR caused by other diseases were excluded in our study. Diagnosis of COPD classification was from stage I to IV according to Global Initiative for Chronic Obstructive Lung Disease (GOLD) 2008 criteria accepted by the American Thoracic Society and the European Respiratory Society. According to our definition, at risk of COPD (stage 0) was diagnosed. The definition for stage 0 is that chronic cough and sputum production are for at least three months in each of two consecutive years without any other condition explaining the cough and a post-bronchodilator FEV1/FVC ≥ 0.7 and FEV1 ≥ 80% predicted [21]. The resting HR of the 231 subjects need further screening to exclude the abnormal resting HR outside the interval ([60,100], beats per minute) [5]. After excluding the abnormal resting HR, 196 subjects are used to determine a lung radiomics feature for characterizing resting heart rate and COPD stage evolution in this study. COPD stage 0−IV has 50, 46, 58, 32 and 10 subjects, respectively.
The ethics committee had approved this study of the national clinical research center of respiratory diseases in Guangzhou medical university, China. Each subject had been provided written informed consent by the first affiliated hospital of Guangzhou medical university before chest HRCT scans and 12 Leads of ECG.
This Section describes the methods including the lung region segmentation (Section 2.2.1), COPD radiomics features calculation (Section 2.2.2), COPD radiomics features selection (Section 2.2.3), and COPD radiomics features combination strategy (Section 2.2.4) in detail.
Figure 2 shows the overall block diagram of this study. Figure 2(A) shows that the trained segmentation model segments the lung parenchyma mask images (512 × 512 × N) from the original chest HRCT images (512 × 512 × N). Figure 2(B) shows the calculation of the radiomics features based on the lung parenchyma images. The original lung parenchyma images are obtained based on the original chest HRCT images and lung parenchyma mask images. Then, the original lung parenchyma images are filtered to get the derived lung parenchyma images. The original chest HRCT images and the derived lung parenchyma images are used to calculate the COPD radiomics features according to a predetermined class of radiomics features. Figure 2(C) shows the selection of lung radiomics features related to HR and the selected COPD radiomics features combination to obtain lung radiomics features that characterize HR and COPD stage evolution. The COPD radiomics features and the corresponding resting HR data of 196 subjects are normalized together before importing the Lasso model. 13 selected COPD radiomics features are generated by the Lasso model. Finally, a COPD radiomics features feature is constructed by the proposed radiomics combination strategy for fully characterizing the resting HR and COPD stage evolution.
The lung region needs to be accurately segmented from the chest HRCT images to calculate the COPD radiomics features. U-Net has been widely used to segment biomedical images [22,23,24]. Some new convolution networks also are proposed, such as a PedNet for image segmentation [25]. Similarly, some networks have made innovations in the application, such as residual networks for the image quality assessment [26]. Based on U-Net and residual networks, a fully automatic segmentation model [27] named U-net (R231) is used to segment the lung region from the chest HRCT images in Figure 2(A). The U-net (R231), which had been trained by human chest CT images, is a U network model with residual building block (ResU-Net) [28]. The architecture of the ResU-Net model is described in detail in our previous study [28]. The trained ResU-Net is available on the website https://github.com/JoHof/lungmask.
Figure 3 shows the typical lung region segmentation results from the original chest HRCT images in the coronal,transverse,and sagittal planes. The images without red and green color are the original chest HRCT images,and the images with red and green colors are the corresponding lung region segmentation results. All slices of lung region segmentation results have been carefully checked and modified by three experienced radiologists using a tool named ITK-SNAP. The ITK-SNAP can be available on the website,http://www.itksnap.org/pmwiki/pmwiki.php?n=Downloads.SNAP3. The three experienced radiologists consider that all lung region segmentation results are acceptable for calculating COPD radiomics features.
COPD radiomics features are calculated based on the original and derived lung parenchyma images. Therefore, the lung parenchyma images should be extracted from the chest HRCT images. The method of extracting original lung parenchyma images is based on the chest HRCT images and can refer to our previous study [28,29].
Wavelet provides the different scales of the chest images for the image analysis by using its multi-resolution decomposition [30,31]. Laplacian of Gaussian filter (LoG) [32,33] as an edge enhancement filter can emphasize areas of gray level change in images which is crucial for COPD chest images. Because of their advantages, the wavelet filter and LoG filter are considered for generating the derived lung parenchyma images in this study.
Figure 4 shows the detailed process of the COPD radiomics features calculation features. First,the wavelet and LoG filters are applied to the original lung parenchyma images. Specifically,the wavelet filter yields 8 (23) decompositions per level with all possible combinations of applying either a high pass filter (H) or low pass filter (L) in each of the three dimensions. Therefore,the wavelet filter can derive eight types of derived lung parenchyma images. The LoG filter with sigma 1.0 to 5.0. can derive five types of derived lung parenchyma images. Finally,the COPD radiomics features are calculated based on the original and the 13 derived lung parenchyma images shown in Figure 3. Specifically,PyRadiomics (version 3.0.,a radiomics calculation model) [34] is applied to calculate the COPD radiomics features. The PyRadiomics is available on the website https://pyradiomics.readthedocs.io/en/latest/index.html, and the website also has given detailed explanations of radiomics. Finally, 1316 COPD radiomics features of each subject are obtained.
This paper uses the Lasso model [35,36] to select the COPD radiomics features related to HR. The mathematical form of the Lasso model is shown in expression (1):
arg min{n∑i=1(y∗i−β0−p∑j=1βjx∗ij)2+λp∑j=0|βj|} | (1) |
where x∗ij is the value of the independent variable after normalization, y∗i is the value of the dependent variable, λ is a penalty parameter (λ ≥ 0), and βj is the regression coefficient vector, i∈[1, n], and j∈[0, p].
A standard R package "lars 1.2" (parameter: type = "lasso", and use.Gram = FALSE) is performed by an operating environment RStudio to select the independent variable. A tenfold cross-validation (a standard R package "cv. Lars" with parameter: type = "lar", K = 10, and use.Gram = FALSE) is used to select the fraction (the minimus cross-validated MSE). The dependent variable is the resting HR∈[60,100], and the independent variable is the COPD radiomics features. The size of COPD radiomics features is 196 × 1316 (196 subjects and 1316 COPD radiomics features of each subject). However, the resting HR and the COPD radiomics features should be normalized before importing the Lasso model. The mathematical form of the normalization is shown in Eq (2):
{x∗ij=(xij−¯xj)/(xjmax−xjmin)y∗i=(yi−¯yi)/(yimax−yimin) | (2) |
where xijis the independent variable (COPD radiomics features) before normalization, yi is the dependent variable (the resting HR) before normalization, ¯xjis the mean of the independent variable xij, xjmax is the maximum of the independent variable xij, xjmin is the minimum of the independent variable xij, ¯yiis the mean of the dependent variable yi, yimax is the maximum of the dependent variable yi, and yimin is the minimum of the dependent variable yi.
The COPD stages Ⅲ and Ⅳ are taken as one stage (stage Ⅲ & Ⅳ) in this paper to balance the data at different COPD stages and meet the statistical need. The lung radiomics feature Yk is constructed by the following combination Eq (3) to observe the significant change among the different types of lung parenchyma images.
Yk=N∑i=1βixi=β1x1+β2x2+⋅⋅⋅+βNxN | (3) |
where k is the type of lung parenchyma images, N is the number of the selected COPD radiomics features belonging to one type of lung parenchyma images, and βi is the coefficient of the selected radiomics xi generated by the Lasso model. K = 1 denotes all types of lung parenchyma images. K = 2 denotes the original lung parenchyma images. K = 3 and 4 denote the derived lung parenchyma images generated from the LoG and wavelet filters.
A COPD radiomics features combination strategy is proposed in this paper to improve the significance among the COPD stages. Table 1 shows the specific algorithm of the COPD radiomics features combination strategy. The idea of the algorithm is to use the least COPD radiomics features to reflect the significances among different COPD stages and retain COPD radiomics features with the maximum coefficient array, which can reflect the resting HR.
Specifically, the coefficient array is the coefficients generated from the Lasso model. After initializing the preset significant condition, each coefficient in the coefficient array is changed to an absolute value. The coefficient array [β1, β2, …., βi] turns to [|β1|, |β2|, …., |βi|]. The absolute values are sorted from largest to smallest, getting the pending coefficient array [max {|β1|, |β2|, …., |βi|}, …., min {|β1|, |β2|, …., |βi|}]. Next, the selected number N is initialized to 2, which means that the first two coefficients are chosen in the pending coefficient array. The N-selected coefficients from the pending coefficient array form a new coefficient array →βN. Then a candidate lung radiomics feature ZN is constructed by the new coefficient array →βN and its COPD radiomics features using the linear combination. The linear combination Eq (4) is shown in Table 1. In the linear combination Eq (4) xi is the selected COPD radiomics features. Finally, the candidate lung radiomics feature's significances among different COPD stages are calculated. Dunn's multiple comparisons test in the statistical software GraphPadPrism (8.0.1) calculates all significances among different COPD stages. If all significances among different COPD stages < the preset significant condition (P = 0.05), the candidate lung radiomics feature ZN is considered as the lung radiomics feature, which characters the resting HR and COPD stage evolution.
All the significances of the 13 selected COPD radiomics features, the constructed lung radiomics features Y1−Y4, and the constructed lung radiomics features F1 and F2 among different COPD stages are analyzed in this Section.
Figure 5 shows the cross-validated mean square error (MES) with the fraction of final L1 norm by standard R package "cv. Lars" (K = 10, tenfold cross-validation). The optimal fraction (0.0909) is determined when the cross-validated MSE takes the minimum value. After the tenfold cross-validation, 13 COPD radiomics features of each subject are selected from the 1316 COPD radiomics based on Lasso.
Table 2 shows the name, class, definition and coefficient of the 13 selected COPD radiomics features. We rename the 13 selected COPD radiomics features as Radiomics 1−13 for convenient description in this paper. The coefficients of the 13 selected COPD radiomics features are generated from the Lasso model. Radiomics 1−3 are the radiomics of the original lung parenchyma images, and radiomics 4−8 are the radiomics of the derived lung parenchyma images generated by the LoG filter with the sigma 1.0−5.0. Radiomics 9−13 are the radiomics of the derived lung parenchyma images generated by the wavelet filter.
Name of the selected COPD radiomics features | Class | Coefficient | Definition |
original_shape_Elongation | Shape Features | 0.0502 | Radiomics1 |
original_shape_MajorAxisLength | Shape Features | −0.0128 | Radiomics2 |
original_shape_Maximum2DDiameterColumn | Shape Features | −0.0715 | Radiomics3 |
log.sigma.2.0.mm.3D_firstorder_Kurtosis | First Order Features | −0.0024 | Radiomics4 |
log.sigma.2.0.mm.3D_firstorder_Maximum | First Order Features | −0.0038 | Radiomics5 |
log.sigma.2.0.mm.3D_glszm_GrayLevelVariance | GLSZM 1 Features | −0.0231 | Radiomics6 |
log.sigma.4.0.mm.3D_glszm_GrayLevelVariance | GLSZM 1 Features | −0.0132 | Radiomics7 |
log.sigma.5.0.mm.3D_glszm_SizeZoneNonUniformity | GLSZM 1 Features | −0.0149 | Radiomics8 |
wavelet.HLH_firstorder_Skewness | First Order Features | 0.0069 | Radiomics9 |
wavelet.HLH_glcm_MCC | GLCM 2 Features | 0.0094 | Radiomics10 |
wavelet.HLH_glrlm_LongRunLowGrayLevelEmphasis | GLRLM 3 Features | −0.0156 | Radiomics11 |
wavelet.HLH_glszm_LargeAreaLowGrayLevelEmphasis | GLSZM 1 Features | −0.0590 | Radiomics12 |
wavelet.LLL_firstorder_10Percentile | First Order Features | −0.2494 | Radiomics13 |
*Note: 1 Gray Level Size Zone Matrix. 2 Gray Level Cooccurrence Matrix. 3 Gray Level Run Length Matrix. |
Table 2 gives the relationship (coefficient) between the 13 selected COPD radiomics features and resting HR. The symbol "-" indicates a positive correlation of the selected COPD radiomics feature and resting HR, and the omitted symbol "+" indicates a positive correlation. Since all COPD radiomics features have been normalized before the selection, the coefficient can represent the importance of its corresponding COPD radiomics features. Figure 6 shows the coefficient of the 13 selected COPD radiomics features and the number of the selected COPD radiomics features in each class. Figure 6(A) shows the order of importance is Radiomics13, 3, 12, 1, 6, 11, 8, 7, 2, 10, 9, 5, 4. Therefore, Radiomics13 is the dominant COPD radiomics feature to affect the resting HR. Figure 6(A) shows the number of the selected COPD radiomics features in each class. Although the numbers of the first order and GLSZM features are the same, the dominant COPD radiomics feature Radiomics13 belongs to the first order features. Therefore, the first order features have a greater impact on the resting HR than GLSZM features.
Figure 7(A)−(M) shows the changing trend with a boxplot of the 13 selected COPD radiomics features among different COPD stages. Table 3 shows significant differences in the selected COPD radiomics features among some different COPD stages, including Radiomics1, 3, 4, 6, 8, 9, 11, 12 and 13. However, there are no significant differences among all COPD stages in Radiomics2, 5, 7 and 10. Therefore, the results of Radiomics1, 3, 4, 6, 8, 9, 11, 12 and 13 are further analyzed.
COPD stage comparison | Radiomics1 | Radiomics2 | Radiomics3 | Radiomics4 | Radiomics5 |
Stage 0 vs. Stage Ⅰ | 0.0067 | 0.0675(ns) | < 0.0001 | 0.0018 | > 0.9999 (ns) |
Stage 0 vs. Stage Ⅱ | 0.0034 | 0.0951(ns) | 0.0001 | 0.0062 | > 0.9999 (ns) |
Stage 0 vs. Stage Ⅲ & Ⅳ | < 0.0001 | > 0.9999 (ns) | 0.0003 | > 0.9999 (ns) | > 0.9999 (ns) |
Stage Ⅰ vs. Stage Ⅱ | > 0.9999 (ns) | > 0.9999 (ns) | > 0.9999 (ns) | > 0.9999 (ns) | > 0.9999 (ns) |
Stage Ⅰ vs. Stage Ⅲ & Ⅳ | 0.0495 | 0.0700(ns) | > 0.9999 (ns) | 0.0425 | > 0.9999 (ns) |
Stage Ⅱ vs. Stage Ⅲ & Ⅳ | 0.03318 | 0.0987(ns) | > 0.9999 (ns) | 0.1213(ns) | > 0.9999 (ns) |
COPD stage comparison | Radiomics6 | Radiomics7 | Radiomics8 | Radiomics9 | Radiomics10 |
Stage 0 vs. Stage Ⅰ | > 0.9999 (ns) | > 0.9999 (ns) | 0.0295 | > 0.9999 (ns) | > 0.9999 (ns) |
Stage 0 vs. Stage Ⅱ | 0.06115 (ns) | > 0.9999 (ns) | 0.3687 (ns) | > 0.9999 (ns) | > 0.9999 (ns) |
Stage 0 vs. Stage Ⅲ & Ⅳ | > 0.9999 (ns) | > 0.9999 (ns) | > 0.9999 (ns) | 0.0014 | 0.5143 (ns) |
Stage Ⅰ vs. Stage Ⅱ | 0.0508 (ns) | > 0.9999(ns) | > 0.9999 (ns) | > 0.9999 (ns) | > 0.9999 (ns) |
Stage Ⅰ vs. Stage Ⅲ & Ⅳ | > 0.9999 (ns) | > 0.9999 (ns) | 0.3202 (ns) | 0.0070 | 0.8122 (ns) |
Stage Ⅱ vs. Stage Ⅲ & Ⅳ | 0.0480 | 0.8283 (ns) | > 0.9999 (ns) | 0.0125 | > 0.9999 (ns) |
COPD stage comparison | Radiomics11 | Radiomics12 | Radiomics13 | ||
Stage 0 vs. Stage Ⅰ | > 0.9999 (ns) | 0.0002 | < 0.0001 | ||
Stage 0 vs. Stage Ⅱ | > 0.9999 (ns) | 0.0081 (ns) | < 0.0001 | ||
Stage 0 vs. Stage Ⅲ & Ⅳ | 0.0330 | < 0.0001 | < 0.0001 | ||
Stage Ⅰ vs. Stage Ⅱ | > 0.9999(ns) | > 0.9999 (ns) | 0.4088 (ns) | ||
Stage Ⅰ vs. Stage Ⅲ & Ⅳ | 0.4837(ns) | 0.3096 (ns) | < 0.0001 | ||
Stage Ⅱ vs. Stage Ⅲ & Ⅳ | 0.1819(ns) | 0.0089 | 0.0030 | ||
*Note: ns: no significance. |
Figure 7(A) shows that Radiomics 1 increases with the development of COPD stages. The mean ± the standard error of mean (SEM) of Radiomics 1 from the COPD stage 0 to Ⅲ & IV is 0.8068 ± 0.0105, 0.8575 ± 0.0088, 0.8585 ± 0.0081, and 0.8950 ± 0.0084, respectively. However, there is no significant change only between COPD stage I and COPD stage Ⅱ (P > 0.9999). Figure 7(C) shows that Radiomics 3 increases with the COPD stages evolution. Compared to COPD stage 0, Radiomics 3 in COPD stage I, Ⅱ, Ⅲ & IV rises. The mean ± SEM of Radiomics 3 from the COPD stage 0 to Ⅲ & IV is 314.5 ± 3.289,336.1 ± 2.425,332.7 ± 2.580 and 333.5 ± 2.607, respectively. The significant change exists only between COPD stage 0 and COPD stage I, Ⅱ, Ⅲ & IV (P < 0.0001, P = 0.0001, P = 0.0003). Figure 7(D) and Table 3 show that Radiomics 4 significantly increases form COPD stage 0 to COPD stage I, Ⅱ (P = 0.0018 and 0.0062). The mean ± SEM of Radiomics 4 from the COPD stage 0 to Ⅲ & IV is 6.552 ± 0.0781, 6.919 ± 0.0691, 6.884 ± 0.0644 and 6.662 ± 0.0576, respectively. Figure 7(F) shows that the mean of Radiomics 6 reaches the maximum at COPD stage Ⅱ (32.67 ± 0.3414) among all the COPD stages. The mean ± SEM of Radiomics 6 at the COPD stage 0, COPD stage I, and COPD stage Ⅲ & IV is 31.41 ± 0.3988, 31.55 ± 0.4403, 31.42 ± 0.4855, respectively. There are significant changes of Radiomics 6 between COPD stage Ⅱ and Ⅲ & IV (P = 0.0480) shown in Table 3. Figure 7(H) and Table 3 show that Radiomics 8 significantly arises only from COPD stage 0 to COPD stage I (P = 0.0295). The mean ± SEM of Radiomics 8 from the COPD stage 0 to Ⅲ & IV is 6559 ± 235.6, 7466 ± 234.5, 7139 ± 202.7 and 6851 ± 191.4, respectively. Figure 5(J) and Table 3 show that Radiomics 9 significantly arises from COPD stage 0, I, Ⅱ to COPD stage Ⅲ & IV (P = 0.0014, P = 0.0070, P = 0.0125). The mean ± SEM of Radiomics 9 from the COPD stage 0 to Ⅲ & IV is 0.1388 ± 0.0085, 0.1458 ± 0.0074, 0.1512 ± 0.0072 and 0.1875 ± 0.0096, respectively. Figure 7(K) and Table 3 show that Radiomics 11 significantly arises from the COPD stage 0 to Ⅲ & IV (P = 0.0330). The mean ± SEM of Radiomics11 at the COPD stage 0 and Ⅲ & IV is 0.1868 ± 0.0064 and 0.2140 ± 0.0102, respectively. Figure 7(L) and Table 3 show that Radiomics 12 significantly arises from the COPD stage 0 to COPD stage I (P = 0.0002) and from COPD stage 0, Ⅱ to COPD stage Ⅲ & IV (P < 0.0001, P = 0.0089). The mean ± SEM of Radiomics 12 from the COPD stage 0 to Ⅲ & IV is 2.956×107 ± 0.3952 × 107, 6.869 × 107 ± 1.006×107, 4.515 × 107 ± 0.3997×107 and 10.83 × 107 ± 1.797×107, respectively. Figure 5(N) shows that Radiomics 13 decreases with the development of COPD stages. The mean ± SEM of Radiomics 13 from the COPD stage 0 to Ⅲ & IV is 2575 ± 9.006, 2624 ± 6.475, 2662 ± 6.043 and 2718 ± 8.700, respectively. However, there is no significant change between COPD stage I and COPD stage Ⅱ (P = 0.4088).
Four lung radiomics features Y1−Y4 are constructed using the Eq (3). Specifically, the lung radiomics feature Y1 is constructed with all Radiomics 1−13 and their coefficients. Next, the lung radiomics feature Y2 is constructed with Radiomics 1−3 and their coefficients belonging to the original lung parenchyma images. Then, the lung radiomics feature Y3 is constructed with Radiomics 4−8 and their coefficients belonging to the derived lung parenchyma images generated from the Log filter. Finally, the lung radiomics feature Y4 is constructed with Radiomics 9−13 and their coefficients belonging to the derived lung parenchyma images generated from the wavelet filter. Figure 8 shows the significance of the lung radiomics features Y1−Y4 among different COPD stages. Table 4 shows the adjusted P-value of the lung radiomics features Y1−Y4 among different COPD stages.
COPD stage comparison | Y1 | Y2 | Y3 | Y4 |
Stage 0 vs. Stage Ⅰ | 0.0542 (ns) | 0.0641 (ns) | > 0.9999 (ns) | < 0.0013 |
Stage 0 vs. Stage Ⅱ | < 0.0001 | 0.1686 (ns) | 0.0686 (ns) | < 0.0001 |
Stage 0 vs. Stage Ⅲ & Ⅳ | < 0.0001 | > 0.9999 (ns) | > 0.9999 (ns) | < 0.0001 |
Stage Ⅰ vs. Stage Ⅱ | 0.0718 (ns) | > 0.9999 (ns) | 0.3835 (ns) | 0.1287 (ns) |
Stage Ⅰ vs. Stage Ⅲ & Ⅳ | < 0.0001 | 0.0729 (ns) | > 0.9999 (ns) | < 0.0001 |
Stage Ⅱ vs. Stage Ⅲ & Ⅳ | < 0.0039 | 0.1851(ns) | 0.1143 (ns) | 0.0619 (ns) |
*Note: ns: no significance. |
Table 4 shows no significant change in lung radiomics feature Y2 and Y3 among the COPD stages. Therefore, the lung radiomics feature Y1 and Y4 are further analyzed. Figure 8(A) shows that lung radiomics feature Y1 increases with the development of COPD stages. The mean ± SEM of lung radiomics feature Y1 from the COPD stage 0 to Ⅲ & IV is −0.1960 ± 0.0303, −0.0507 ± 0.0268, 0.04254 ± 0.0201 and 0.2302 ± 0.0311, respectively. However, there is no significant change between COPD stage 0 and COPD stage I (P = 0.0542) and between COPD stage I and COPD stage Ⅱ (P = 0.0718) shown in Table 4. Like lung radiomics feature Y1, Figure 8(D) shows that the lung radiomics feature Y4 also increases with the development of COPD stages. The mean ± SEM of lung radiomics feature Y4 from the COPD stage 0 to Ⅲ & IV is −0.2234 ± 0.0320, −0.0272 ± 0.0235, 0.0651 ± 0.0220 and 0.2058 ± 0.0327, respectively. There is no significant change between COPD stage I and COPD stage Ⅱ (P = 0.1287) and between COPD stage Ⅱ and COPD stage Ⅲ & IV (P = 0.0619) shown in Table 4.
Two lung radiomics features, F1 and F2, are constructed using the other COPD radiomics combination strategy seen in Table 5. Figure 9 shows the changing trend of the lung radiomics features F1 and F2 constructed by the COPD radiomics features combination strategy (Section 2.2.4) among different COPD stages. Figure 9(A) shows the changing trend of the lung radiomics feature F1 constructed by Radiomics 13 with the coefficient −0.2494 and Radiomics 3 with the coefficient −0.0715. Figure 9(B) shows the changing trend of the lung radiomics feature F1 constructed by Radiomics 13, Radiomics 3, and Radiomics 12 with the coefficient −0.2494, −0.0715, −0.0590, respectively. Figure 9(A) and Table 5 show that except for the significance between COPD stage Ⅰ and Ⅱ (P = 0.0646), the lung radiomics feature F1 significantly increases with the development of COPD stages. The mean ± SEM of the lung radiomics feature F1 from the COPD stage 0 to Ⅲ & IV is −0.1990 ± 0.0259, −0.0433 ± 0.0218, 0.0398 ± 0.0177, and 0.2294 ± 0.0268, respectively. Figure 9(A) and Table 5 also show that the lung radiomics feature F2 significantly increases with the development of COPD stages. The mean ± SEM of the lung radiomics feature F2 from the COPD stage 0 to Ⅲ & IV is −0.1739 ± 0.0255, −0.0502 ± 0.0215, 0.0521 ± 0.0183, and 0.1901 ± 0.0280, respectively.
COPD stage comparison | F1 | F2 |
Stage 0 vs. Stage Ⅰ | 0.0031 | 0.0479 |
Stage 0 vs. Stage Ⅱ | < 0.0001 | < 0.0001 |
Stage 0 vs. Stage Ⅲ & Ⅳ | < 0.0001 | < 0.0001 |
Stage Ⅰ vs. Stage Ⅱ | 0.0646 (ns) | 0.0085 |
Stage Ⅰ vs. Stage Ⅲ & Ⅳ | < 0.0001 | < 0.0001 |
Stage Ⅱ vs. Stage Ⅲ & Ⅳ | < 0.0014 | 0.0342 |
*Note: ns: no significance. |
The selected COPD radiomics features, the lung radiomics features Y1−Y4, and the lung radiomics features F1−F2 related to the resting HR are discussed in Figures 6−9 and Tables 3−5.
From the above results, a single selected COPD radiomics features related to the resting HR cannot characterize the significant changes of COPD stage evolution among different COPD stages, especially from COPD stage I to COPD stage Ⅱ. Although Radiomics 1 and Radiomics 13 can reflect significant changes in most COPD stages, they only fail to characterize the significant changes from COPD stage I to COPD stage Ⅱ. Compared to Radiomics 1, the significance from COPD stage I to COPD stage Ⅱ in Radiomics 13 (P = 0.4088) is better than that of Radiomics 1(P > 0.9999). Radiomics 3 with significance (P < 0.0001) is the sensitive COPD radiomics feature related to resting HR from the risk of COPD (COPD stage 0) to suffering from COPD (COPD stage I−Ⅲ & IV). At the same time, the significance of Radiomics 13 among other COPD stages is also better than that of Radiomics 1. Most importantly, Radiomics 13 is the dominant COPD radiomics feature affecting the resting HR. Therefore, the dominant COPD radiomics feature Radiomics 13 not only characterizes the resting HR but also characterizes COPD stages evolution (except for the significance between COPD stage I and COPD stage Ⅱ).
The selected COPD radiomics features obtained by different types of lung parenchyma images are also further discussed in this paper. Unfortunately, the lung radiomics feature Y2, calculated by the original lung parenchyma images, fails to characterize the evolution of COPD stages. Likewise, the lung radiomics feature Y3, calculated by the derived lung parenchyma images (LoG filters), only characterizes the COPD stage 0 to COPD stage Ⅱ. However, the lung radiomics feature Y4, calculated by the derived lung parenchyma images (wavelet filter), only fails to characterize the COPD stage I to COPD stage Ⅱ. Therefore, the selected COPD radiomics features calculated based on wavelet filter can better characterize COPD stage evolution than the LoG filter. At the same time, it can be seen from the lung radiomics feature Y1, which fully characterizes the resting HR, that it improves the overall significance among the different COPD stages. In particular, Radiomics 13 is also calculated from the derived lung parenchyma images based on the wavelet filter. The lung radiomics feature F1 and F2 are also discussed. The lung radiomics feature F2 constructed by Radiomics13, Radiomics 3, and Radiomics12 improves the significance from COPD stage I to COPD stage Ⅱ (P = 0.0085 < 0.05). Although Radiomics13 is the dominant COPD radiomics feature, other selected COPD radiomics features Radiomics 3 and Radiomics 12 are also needed to characterize the COPD evolution. No matter what, finding a lung radiomics feature is a competitive process between different COPD stages.
The COPD radiomics features are calculated based on chest HRCT images with different COPD stages. The selected COPD radiomics features related to the resting HR are further determined. The selected COPD radiomics features can reflect the resting HR variability. Therefore, the relationship between the COPD radiomics features and the resting HR is revealed. The selected COPD radiomics features and/or the lung radiomics feature F2 may be a predictor of the resting HR variability of the subjects with COPD. The resting HR at different COPD stages may be predicted by the selected COPD radiomics features and/or the lung radiomics feature F2. Clinically, the resting HR variability has many causes. Although the abnormal resting HR caused by other diseases and outside the interval [5] was excluded in our study, it will also be more meaningful to analyze the patients with cardiopulmonary disease in future research.
The COPD radiomics features are calculated from the lung parenchyma images, reflecting the state of "COPD patients" at stages 0−IV. Compared with chest HRCT images, the COPD radiomics features can express the hidden information at different COPD stages. This hidden information is more helpful to characterize the differences of varying COPD stages and releases the relationship between resting HR and COPD evolution. There are also some limitations of this study. First, when chest HRCT images are collected, the patient's inspiratory state can be controlled, but the heart's movement cannot be controlled. Therefore, the blood state in pulmonary vessels must impact the calculation of the COPD radiomics features. Second, the number of subjects with COPD stage IV is only 10. Therefore, we take COPD stage Ⅲ and IV as one COPD stage, affecting the analysis of the results.
Massive COPD radiomics features are calculated based on the lung region segmented by the trained ResU-Net. The 13 selected COPD radiomics features related to the resting HR are selected from the massive COPD radiomics features using the Lasso model. A COPD radiomics features combination strategy is proposed to provide a lung radiomics feature for characterizing the resting HR and the COPD stage evolution. Because the lung radiomics feature Y1 is constructed by all the selected COPD radiomics features, it is considered that it can fully characterize the resting HR. However, the lung radiomics feature Y1 fails to characterize the COPD stage evolution from COPD stage 0 to I, and from COPD stage I to Ⅱ. Compared with the P-value of Y1, that of the lung radiomics feature F2 has been improved 0.63% between COPD stage 0 and stage Ⅰ, and 6.33% between COPD stage Ⅰ and stage Ⅱ, resulting in the P-value of F2 less than 0.05. Based on the COPD radiomics features combination strategy, the lung radiomics feature F2 with the dominant selected COPD radiomics features not only can characterize the resting HR but also can characterize the COPD stage evolution.
Thanks to the Department of Radiology, the First Affiliated Hospital of Guangzhou Medical University for providing the dataset and the funding support from the National Natural Science Foundation of China (62071311), Natural Science Foundation of Guangdong Province, China (2019A1515011382), Stable Support Plan for Colleges and Universities in Shenzhen, China (SZWD2021010), and Scientific Research Fund of Liaoning Province, China (JL201919).
The authors declare no conflict of interest.
[1] |
C. A. Camillo, F. Pitta, H. V. Possani, M. V. R. A. Barbosa, D. S. O. Marques, V. Cavalheri, et al., Heart rate variability and disease characteristics in patients with COPD, Lung, 186 (2008), 393-401. https://doi.org/10.1007/s00408-008-9105-7 doi: 10.1007/s00408-008-9105-7
![]() |
[2] |
D. Singh, A. Agusti, A. Anzueto, P. J. Barnes, J. Bourbeau, B. R. Celli, et al., Global strategy for the diagnosis, management, and prevention of chronic obstructive lung disease: the GOLD science committee report 2019, Eur. Respir. J., (2019), 1900164. https://doi.org/10.1183/13993003.00164-2019 doi: 10.1183/13993003.00164-2019
![]() |
[3] |
M. C. Matheson, G. Bowatte, J. L. Perret, A. J. Lowe, C. V. Senaratna, G. L. Hall, et al., Prediction models for the development of COPD: a systematic review, Int. J. Chronic Obstr. Pulm. Dis., 13 (2018), 1927-1935. https://doi.org/10.2147/COPD.S155675 doi: 10.2147/COPD.S155675
![]() |
[4] |
M. J. Lewis, J. Annandale, K. E. Lewis, Influence of long-term oxygen therapy on heart rate and QT time-series in hypoxic patients with chronic obstructive pulmonary disease, Clin. Physiol. Funct. Imaging, 31 (2010), 431-439. https://doi.org/10.1111/j.1475-097X.2009.00891.x doi: 10.1111/j.1475-097X.2009.00891.x
![]() |
[5] |
M. T. Jensen, J. L. Marott, P. Lange, J. Vestbo, P. Schnohr, O. W. Nielsen, et al., Resting heart rate is a predictor of mortality in chronic obstructive pulmonary disease, Eur. Respir. J., 42 (2013), 341-349. https://doi.org/10.1183/09031936.00072212 doi: 10.1183/09031936.00072212
![]() |
[6] | GOLD 2021: Global initiative for chronic obstructive lung disease, 2021. |
[7] |
A. A. de Camargo, T. Justino, C. H. S. de Andrade, C. Malaguti, S. D. Corso, Chester step test in patients with copd: reliability and correlation with pulmonary function test results, Resp. Care, 56 (2011), 995-1001. https://doi.org/10.4187/respcare.01047 doi: 10.4187/respcare.01047
![]() |
[8] |
S. T. Cheng, Y. K. Wu, M. C. Yang, C. Y. Huang, H. C. Huang, W. H. Chu, et al., Pulmonary rehabilitation improves heart rate variability at peak exercise, exercise capacity and health-related quality of life in chronic obstructive pulmonary disease, Heart Lung, 43 (2014), 249-255. https://doi.org/10.1016/j.hrtlng.2014.03.002 doi: 10.1016/j.hrtlng.2014.03.002
![]() |
[9] |
N. F. Serrão, A. Porta, V. Minatel, A. A. M. Castro, A. Borghi-Silva, Complexity analysis of heart rate variability in chronic obstructive pulmonary disease: relationship with severity and symptoms, Clin. Auton. Res., 30 (2020), 1-8. https://doi.org/10.1007/s10286-019-00659-z doi: 10.1007/s10286-019-00656-2
![]() |
[10] |
P. F. Camargo, L. Ditomaso-Luporini, L. C. S. de Carvalho Jr, C. da Luz Goulart, R. Sebold, P. B. Dos Santos, et al., Association between the predictors of functional capacity and heart rate off-kinetics in patients with chronic obstructive pulmonary disease, Int. J. Chronic Obstr. Pulm. Dis., 15 (2020), 1977-1986. https://doi.org/10.2147/COPD.S260284 doi: 10.2147/COPD.S260284
![]() |
[11] |
P. K. Stein, P. Nelson, J. N. Rottman, D. Howard, S. M. Ward, R. E. Kleiger, et al., Heart rate variability reflects severity of COPD in PiZ α1-Antitrypsin deficiency, Chest, 113 (1998), 327-333. https://doi.org/10.1378/chest.113.2.327 doi: 10.1378/chest.113.2.327
![]() |
[12] |
L. Josephs, D. Culliford, M. Johnson, M. Thomas, COPD overdiagnosis in primary care: a UK observational study of consistency of airflow obstruction, NPJ Prim. Care Resp. Med., 29 (1998), 1-9. https://doi.org/10.1038/s41533-019-0145-7 doi: 10.1038/s41533-019-0145-7
![]() |
[13] |
R. Onoe, T. Yamashiro, H. Hiroshi, S. Azagami, S. Matsuoka, T. Inoue, et al., 3D-measurement of tracheobronchial angles on inspiratory and expiratory chest CT in COPD: respiratory changes and correlation with airflow limitation, Int. J. Chronic Obstr. Pulm. Dis, 13 (2018), 2399-2407. https://doi.org/10.2147/COPD.S165824 doi: 10.2147/COPD.S165824
![]() |
[14] |
Q. Li, L. Chen, X. Li, X. Lv, S. Xia, Y. Kang, PRF-RW: a progressive random forest-based random walk approach for interactive semi-automated pulmonary lobes segmentation, Int. J. Mach. Learn. Cyb., 11 (2020), 2221-2235. https://doi.org/10.1007/s13042-020-01111-9 doi: 10.1007/s13042-020-01111-9
![]() |
[15] |
Y. Ohno, K. Aoyagi, D. Takenaka, T. Yoshikawa, A. Ikezaki, Y. Fujisawa, et al., Machine learning for lung CT texture analysis: improvement of inter-observer agreement for radiological finding classification in patients with pulmonary diseases, Eur. J. Radiol., 134, (2021), 109410. https://doi.org/10.1016/j.ejrad.2020.109410 doi: 10.1016/j.ejrad.2020.109410
![]() |
[16] |
D. A. Lynch, Progress in imaging COPD, 2004-2014, Chronic Obstr. Pulm. Dis. J. COPD Found., 1 (2014), 73-82. https://doi.org/10.15326/jcopdf.1.1.2014.0125 doi: 10.15326/jcopdf.1.1.2014.0125
![]() |
[17] |
M. Occhipinti, M. Paoletti, B. J. Bartholmai, S. Rajagopalan, R. A. Karwoski, C. Nardi, et al., Spirometric assessment of emphysema presence and severity as measured by quantitative CT and CT-based radiomics in COPD, Respir. Res., 20 (2019), 1-11. https://doi.org/10.1186/s12931-019-1049-3 doi: 10.1186/s12931-018-0967-9
![]() |
[18] |
G, Wu, A. Ibrahim, I. Halilaj, R. T. Leijenaar, W. Rogers, H. A. Gietema, et al., The emerging role of radiomics in COPD and lung cancer, Respiration, 99 (2020), 99-107. https://doi.org/10.1159/000505429 doi: 10.1159/000505429
![]() |
[19] |
A. Wheeler, A. Zanobetti, D. R. Gold, J. Schwartz, P. Stone, H. H. Suh, The relationship between ambient air pollution and heart rate variability differs for individuals with heart and pulmonary disease, Environ. Health Persp., 114 (2006), 560-566. https://doi.org/10.1289/ehp.8337 doi: 10.1289/ehp.8337
![]() |
[20] |
M. S. Bianchim, E. F. Sperandio, G. S. Martinhao, A. C. Matheus, V. T. Lauria, R. P. Da Silva, et al., Correlation between heart rate variability and pulmonary function adjusted by confounding factors in healthy adults, Braz. J. Med. Biol. Res., 49 (2016), 1-7. https://doi.org/10.1590/1414-431X20154435 doi: 10.1590/1414-431X20154435
![]() |
[21] |
Y. Zhou, P. L. Bruijnzeel, C. Mccrae, J. Zheng, U. Nihlen, R. Zhou, et al., Study on risk factors and phenotypes of acute exacerbations of chronic obstructive pulmonary disease in Guangzhou, China-design and baseline characteristics, J. Thorac. Dis. 7 (2015), 720-733. https://doi.org/10.3978/j.issn.2072-1439.2015.04.14 doi: 10.3978/j.issn.2072-1439.2015.04.14
![]() |
[22] | O. Ronneberger, P. Fischer, T. Brox, U-Net: Convolutional Networks for Biomedical Image Segmentation, in International Conference on Medical Image Computing and Computer-Assisted Intervention, (2015), 234-241. https://doi.org/10.1007/978-3-319-24574-4_28 |
[23] |
C. Cho, Y. H. Lee, J. Park, S. Lee, A self-spatial adaptive weighting based u-net for image segmentation, Electronics, 10 (2021), 348-359. https://doi.org/10.3390/electronics10030348 doi: 10.3390/electronics10030348
![]() |
[24] | P. J. R. Prasad, O. J. Elle, F. Lindseth, F. Albregtsen, R. P. Kumar, Modifying u-net for small dataset: a simplified u-net version for liver parenchyma segmentation, in Medical Imaging 2021: Computer-Aided Diagnosis, International Society for Optics and Photonics, 2021, 11579 (2021). https://doi.org/10.1117/12.2582179 |
[25] |
M. Ullah, A. Mohammed, F. Alaya Cheikh, PedNet: A spatio-temporal deep convolutional neural network for pedestrian segmentation, J. Imag., 4 (2018), 107-125. https://doi.org/10.3390/jimaging4090107 doi: 10.3390/jimaging4090107
![]() |
[26] |
Z. A. Khan, A. Beghdadi, M. Kaaniche, F. A. Cheikh, Residual networks based distortion classification and ranking for laparoscopic image quality assessment, in 2020 IEEE International Conference on Image Processing (ICIP), (2021), 176-180. https://doi.org/10.1109/ICIP40778.2020.9191111 doi: 10.1109/ICIP40778.2020.9191111
![]() |
[27] |
J. Hofmanninger, F. Prayer, J. Pan, S. Rohrich, H. Prosch, G. Langs, Automatic lung segmentation in routine imaging is a data diversity problem, not a methodology problem, Eur. Rad. Exp., 4 (2020), 1-13. https://doi.org/10.1186/s41747-020-00173-2 doi: 10.1186/s41747-019-0127-0
![]() |
[28] |
Y. Yang, Q. Li, Y. Guo, Y. Liu, X. Li, J. Guo, et al., Lung parenchyma parameters measure of rats from pulmonary window computed tomography images based on ResU-Net model for medical respiratory researches, Math. Biosci. Eng., 18 (2021), 4193-4211. https://doi.org/10.3934/mbe.2021210 doi: 10.3934/mbe.2021210
![]() |
[29] | Y. Yang, Y. Guo, J. Guo, Y. Gao, Y. Kang, A method of abstracting single pulmonary lobe from computed tomography pulmonary images for locating COPD, in Proceedings of the Fourth International Conference on Biological Information and Biomedical Engineering, (2020), 1-6. https://doi.org/10.1145/3403782.3403805 |
[30] |
G. Z. Yang, D. M. Hansell, CT image enhancement with wavelet analysis for the detection of small airways disease, IEEE Trans. Med. Imaging, 16 (1997), 953-961. https://doi.org/10.1109/42.650893 doi: 10.1109/42.650893
![]() |
[31] |
S. S. Birring, M. D. Peake, Symptoms and the early diagnosis of lung cancer, Thorax, 60 (2005), 268-269. https://doi.org/10.1136/thx.2004.032698 doi: 10.1136/thx.2004.032698
![]() |
[32] |
F. Neycenssac, Contrast enhancement using the laplacian-of-a-gaussian filter, CVGIP: Graph. Models Image Process., 55 (1993), 447-463. https://doi.org/10.1006/cgip.1993.1034 doi: 10.1006/cgip.1993.1034
![]() |
[33] | Z. Shi, J. Bai, L. He, T. Nakamura, Q. Yao, H. Itoh, et al., A method for enhancing lung nodules in chest radiographs by use of LoG Filter, in 2009 2nd International Congress on Image and Signal Processing, (2009), 1-4. https://doi.org/10.1109/CISP.2009.5301319 |
[34] |
J. J. van Griethuysen, A. Fedorov, C. Parmar, A. Hosny, N. Aucoin, V. Narayan, et al., Computational radiomics system to decode the radiographic phenotype, Cancer Res., 77 (2017), 104-107. https://doi.org/10.1158/0008-5472.CAN-17-0339 doi: 10.1158/0008-5472.CAN-17-0339
![]() |
[35] |
R. Tibshirani, Regression shrinkage and selection via the Lasso Robert Tibshirani, J. Roy. Stat. Soc. Series B (Stat. Methodol.), 58 (2007), 267-288. https://doi.org/10.1111/j.2517-6161.1996.tb02080.x doi: 10.1111/j.2517-6161.1996.tb02080.x
![]() |
[36] |
N. Simon, J. Friedman, T. Hastie, R. Tibshirani, Regularization paths for Cox's proportional hazards model via coordinate descent, J. Stat. Softw., 39 (2011), 1-13. https://doi.org/10.18637/jss.v039.i05 doi: 10.18637/jss.v039.i05
![]() |
1. | Yingwei Guo, Yingjian Yang, Fengqiu Cao, Yang Liu, Wei Li, Chaoran Yang, Mengting Feng, Yu Luo, Lei Cheng, Qiang Li, Xueqiang Zeng, Xiaoqiang Miao, Longyu Li, Weiyan Qiu, Yan Kang, Radiomics features of DSC-PWI in time dimension may provide a new chance to identify ischemic stroke, 2022, 13, 1664-2295, 10.3389/fneur.2022.889090 | |
2. | Yingjian Yang, Wei Li, Yingwei Guo, Nanrong Zeng, Shicong Wang, Ziran Chen, Yang Liu, Huai Chen, Wenxin Duan, Xian Li, Wei Zhao, Rongchang Chen, Yan Kang, Lung radiomics features for characterizing and classifying COPD stage based on feature combination strategy and multi-layer perceptron classifier, 2022, 19, 1551-0018, 7826, 10.3934/mbe.2022366 | |
3. | Yingwei Guo, Yingjian Yang, Fengqiu Cao, Mingming Wang, Yu Luo, Jia Guo, Yang Liu, Xueqiang Zeng, Xiaoqiang Miu, Asim Zaman, Jiaxi Lu, Yan Kang, A Focus on the Role of DSC-PWI Dynamic Radiomics Features in Diagnosis and Outcome Prediction of Ischemic Stroke, 2022, 11, 2077-0383, 5364, 10.3390/jcm11185364 | |
4. | Yingwei Guo, Yingjian Yang, Fengqiu Cao, Wei Li, Mingming Wang, Yu Luo, Jia Guo, Asim Zaman, Xueqiang Zeng, Xiaoqiang Miu, Longyu Li, Weiyan Qiu, Yan Kang, Novel Survival Features Generated by Clinical Text Information and Radiomics Features May Improve the Prediction of Ischemic Stroke Outcome, 2022, 12, 2075-4418, 1664, 10.3390/diagnostics12071664 | |
5. | Yingjian Yang, Shicong Wang, Nanrong Zeng, Wenxin Duan, Ziran Chen, Yang Liu, Wei Li, Yingwei Guo, Huai Chen, Xian Li, Rongchang Chen, Yan Kang, Lung Radiomics Features Selection for COPD Stage Classification Based on Auto-Metric Graph Neural Network, 2022, 12, 2075-4418, 2274, 10.3390/diagnostics12102274 | |
6. | TaoHu Zhou, WenTing Tu, Peng Dong, ShaoFeng Duan, XiuXiu Zhou, YanQing Ma, Yun Wang, Tian Liu, HanXiao Zhang, Yan Feng, WenJun Huang, YanMing Ge, Shiyuan Liu, Zhaobin Li, Li Fan, CT-Based Radiomic Nomogram for the Prediction of Chronic Obstructive Pulmonary Disease in Patients with Lung cancer, 2023, 10766332, 10.1016/j.acra.2023.03.021 | |
7. | Xingguang Deng, Wei Li, Yingjian Yang, Shicong Wang, Nanrong Zeng, Jiaxuan Xu, Haseeb Hassan, Ziran Chen, Yang Liu, Xiaoqiang Miao, Yingwei Guo, Rongchang Chen, Yan Kang, COPD stage detection: leveraging the auto-metric graph neural network with inspiratory and expiratory chest CT images, 2024, 62, 0140-0118, 1733, 10.1007/s11517-024-03016-z | |
8. | TaoHu Zhou, Yu Guan, XiaoQing Lin, XiuXiu Zhou, Liang Mao, YanQing Ma, Bing Fan, Jie Li, ShiYuan Liu, Li Fan, CT-based whole lung radiomics nomogram for identification of PRISm from non-COPD subjects, 2024, 25, 1465-993X, 10.1186/s12931-024-02964-2 | |
9. | Tao-Hu Zhou, Xiu-Xiu Zhou, Jiong Ni, Yan-Qing Ma, Fang-Yi Xu, Bing Fan, Yu Guan, Xin-Ang Jiang, Xiao-Qing Lin, Jie Li, Yi Xia, Xiang Wang, Yun Wang, Wen-Jun Huang, Wen-Ting Tu, Peng Dong, Zhao-Bin Li, Shi-Yuan Liu, Li Fan, CT whole lung radiomic nomogram: a potential biomarker for lung function evaluation and identification of COPD, 2024, 11, 2054-9369, 10.1186/s40779-024-00516-9 | |
10. | Yingjian Yang, Jie Zheng, Peng Guo, Tianqi Wu, Qi Gao, Yingwei Guo, Ziran Chen, Chengcheng Liu, Zhanglei Ouyang, Huai Chen, Yan Kang, Automatic cardiothoracic ratio calculation based on lung fields abstracted from chest X-ray images without heart segmentation, 2024, 15, 1664-042X, 10.3389/fphys.2024.1416912 | |
11. | Yingjian Yang, Nanrong Zeng, Ziran Chen, Wei Li, Yingwei Guo, Shicong Wang, Wenxin Duan, Yang Liu, Rongchang Chen, Yan Kang, Weihua Yang, Multi‐Layer Perceptron Classifier with the Proposed Combined Feature Vector of 3D CNN Features and Lung Radiomics Features for COPD Stage Classification, 2023, 2023, 2040-2295, 10.1155/2023/3715603 | |
12. | Shicong Wang, Wei Li, Nanrong Zeng, Jiaxuan Xu, Yingjian Yang, Xingguang Deng, Ziran Chen, Wenxin Duan, Yang Liu, Yingwei Guo, Rongchang Chen, Yan Kang, Acute exacerbation prediction of COPD based on Auto-metric graph neural network with inspiratory and expiratory chest CT images, 2024, 10, 24058440, e28724, 10.1016/j.heliyon.2024.e28724 | |
13. | Fei Shan, Minwen Zheng, 2024, Chapter 9, 978-981-99-8440-4, 153, 10.1007/978-981-99-8441-1_9 |
Detailed implementation process | |
Input | Coefficient array [β1, β2, …., βi] Initialization: preset significant condition (P = 0.005) |
Output | Lung radiomics feature Flung radiomics; N |
Computation | Absolute value operation for the coefficient array [|β1|, |β2|, …., |βi|] Sorting operation for the coefficient array [max {|β1|, |β2|, …., |βi|}, …., min {|β1|, |β2|, …., |βi|}] for N = 2; N ≤ 13; N ← N + 1 do { Form a new coefficient array →βN=[max{|β1|, |β2|, …., |βi|},….,min{|β1|, |β2|, …., |βi|}]⏟N Construct the candidate lung radiomics feature ZN=N∑i=1→βN·xi (4) Calculate the significances of ZN among different COPD stages if all significances < (P = 0.05) Flung radiomics=ZN break from for end if } end for return {Flung radiomics; N } |
Name of the selected COPD radiomics features | Class | Coefficient | Definition |
original_shape_Elongation | Shape Features | 0.0502 | Radiomics1 |
original_shape_MajorAxisLength | Shape Features | −0.0128 | Radiomics2 |
original_shape_Maximum2DDiameterColumn | Shape Features | −0.0715 | Radiomics3 |
log.sigma.2.0.mm.3D_firstorder_Kurtosis | First Order Features | −0.0024 | Radiomics4 |
log.sigma.2.0.mm.3D_firstorder_Maximum | First Order Features | −0.0038 | Radiomics5 |
log.sigma.2.0.mm.3D_glszm_GrayLevelVariance | GLSZM 1 Features | −0.0231 | Radiomics6 |
log.sigma.4.0.mm.3D_glszm_GrayLevelVariance | GLSZM 1 Features | −0.0132 | Radiomics7 |
log.sigma.5.0.mm.3D_glszm_SizeZoneNonUniformity | GLSZM 1 Features | −0.0149 | Radiomics8 |
wavelet.HLH_firstorder_Skewness | First Order Features | 0.0069 | Radiomics9 |
wavelet.HLH_glcm_MCC | GLCM 2 Features | 0.0094 | Radiomics10 |
wavelet.HLH_glrlm_LongRunLowGrayLevelEmphasis | GLRLM 3 Features | −0.0156 | Radiomics11 |
wavelet.HLH_glszm_LargeAreaLowGrayLevelEmphasis | GLSZM 1 Features | −0.0590 | Radiomics12 |
wavelet.LLL_firstorder_10Percentile | First Order Features | −0.2494 | Radiomics13 |
*Note: 1 Gray Level Size Zone Matrix. 2 Gray Level Cooccurrence Matrix. 3 Gray Level Run Length Matrix. |
COPD stage comparison | Radiomics1 | Radiomics2 | Radiomics3 | Radiomics4 | Radiomics5 |
Stage 0 vs. Stage Ⅰ | 0.0067 | 0.0675(ns) | < 0.0001 | 0.0018 | > 0.9999 (ns) |
Stage 0 vs. Stage Ⅱ | 0.0034 | 0.0951(ns) | 0.0001 | 0.0062 | > 0.9999 (ns) |
Stage 0 vs. Stage Ⅲ & Ⅳ | < 0.0001 | > 0.9999 (ns) | 0.0003 | > 0.9999 (ns) | > 0.9999 (ns) |
Stage Ⅰ vs. Stage Ⅱ | > 0.9999 (ns) | > 0.9999 (ns) | > 0.9999 (ns) | > 0.9999 (ns) | > 0.9999 (ns) |
Stage Ⅰ vs. Stage Ⅲ & Ⅳ | 0.0495 | 0.0700(ns) | > 0.9999 (ns) | 0.0425 | > 0.9999 (ns) |
Stage Ⅱ vs. Stage Ⅲ & Ⅳ | 0.03318 | 0.0987(ns) | > 0.9999 (ns) | 0.1213(ns) | > 0.9999 (ns) |
COPD stage comparison | Radiomics6 | Radiomics7 | Radiomics8 | Radiomics9 | Radiomics10 |
Stage 0 vs. Stage Ⅰ | > 0.9999 (ns) | > 0.9999 (ns) | 0.0295 | > 0.9999 (ns) | > 0.9999 (ns) |
Stage 0 vs. Stage Ⅱ | 0.06115 (ns) | > 0.9999 (ns) | 0.3687 (ns) | > 0.9999 (ns) | > 0.9999 (ns) |
Stage 0 vs. Stage Ⅲ & Ⅳ | > 0.9999 (ns) | > 0.9999 (ns) | > 0.9999 (ns) | 0.0014 | 0.5143 (ns) |
Stage Ⅰ vs. Stage Ⅱ | 0.0508 (ns) | > 0.9999(ns) | > 0.9999 (ns) | > 0.9999 (ns) | > 0.9999 (ns) |
Stage Ⅰ vs. Stage Ⅲ & Ⅳ | > 0.9999 (ns) | > 0.9999 (ns) | 0.3202 (ns) | 0.0070 | 0.8122 (ns) |
Stage Ⅱ vs. Stage Ⅲ & Ⅳ | 0.0480 | 0.8283 (ns) | > 0.9999 (ns) | 0.0125 | > 0.9999 (ns) |
COPD stage comparison | Radiomics11 | Radiomics12 | Radiomics13 | ||
Stage 0 vs. Stage Ⅰ | > 0.9999 (ns) | 0.0002 | < 0.0001 | ||
Stage 0 vs. Stage Ⅱ | > 0.9999 (ns) | 0.0081 (ns) | < 0.0001 | ||
Stage 0 vs. Stage Ⅲ & Ⅳ | 0.0330 | < 0.0001 | < 0.0001 | ||
Stage Ⅰ vs. Stage Ⅱ | > 0.9999(ns) | > 0.9999 (ns) | 0.4088 (ns) | ||
Stage Ⅰ vs. Stage Ⅲ & Ⅳ | 0.4837(ns) | 0.3096 (ns) | < 0.0001 | ||
Stage Ⅱ vs. Stage Ⅲ & Ⅳ | 0.1819(ns) | 0.0089 | 0.0030 | ||
*Note: ns: no significance. |
COPD stage comparison | Y1 | Y2 | Y3 | Y4 |
Stage 0 vs. Stage Ⅰ | 0.0542 (ns) | 0.0641 (ns) | > 0.9999 (ns) | < 0.0013 |
Stage 0 vs. Stage Ⅱ | < 0.0001 | 0.1686 (ns) | 0.0686 (ns) | < 0.0001 |
Stage 0 vs. Stage Ⅲ & Ⅳ | < 0.0001 | > 0.9999 (ns) | > 0.9999 (ns) | < 0.0001 |
Stage Ⅰ vs. Stage Ⅱ | 0.0718 (ns) | > 0.9999 (ns) | 0.3835 (ns) | 0.1287 (ns) |
Stage Ⅰ vs. Stage Ⅲ & Ⅳ | < 0.0001 | 0.0729 (ns) | > 0.9999 (ns) | < 0.0001 |
Stage Ⅱ vs. Stage Ⅲ & Ⅳ | < 0.0039 | 0.1851(ns) | 0.1143 (ns) | 0.0619 (ns) |
*Note: ns: no significance. |
COPD stage comparison | F1 | F2 |
Stage 0 vs. Stage Ⅰ | 0.0031 | 0.0479 |
Stage 0 vs. Stage Ⅱ | < 0.0001 | < 0.0001 |
Stage 0 vs. Stage Ⅲ & Ⅳ | < 0.0001 | < 0.0001 |
Stage Ⅰ vs. Stage Ⅱ | 0.0646 (ns) | 0.0085 |
Stage Ⅰ vs. Stage Ⅲ & Ⅳ | < 0.0001 | < 0.0001 |
Stage Ⅱ vs. Stage Ⅲ & Ⅳ | < 0.0014 | 0.0342 |
*Note: ns: no significance. |
Detailed implementation process | |
Input | Coefficient array [β1, β2, …., βi] Initialization: preset significant condition (P = 0.005) |
Output | Lung radiomics feature Flung radiomics; N |
Computation | Absolute value operation for the coefficient array [|β1|, |β2|, …., |βi|] Sorting operation for the coefficient array [max {|β1|, |β2|, …., |βi|}, …., min {|β1|, |β2|, …., |βi|}] for N = 2; N ≤ 13; N ← N + 1 do { Form a new coefficient array →βN=[max{|β1|, |β2|, …., |βi|},….,min{|β1|, |β2|, …., |βi|}]⏟N Construct the candidate lung radiomics feature ZN=N∑i=1→βN·xi (4) Calculate the significances of ZN among different COPD stages if all significances < (P = 0.05) Flung radiomics=ZN break from for end if } end for return {Flung radiomics; N } |
Name of the selected COPD radiomics features | Class | Coefficient | Definition |
original_shape_Elongation | Shape Features | 0.0502 | Radiomics1 |
original_shape_MajorAxisLength | Shape Features | −0.0128 | Radiomics2 |
original_shape_Maximum2DDiameterColumn | Shape Features | −0.0715 | Radiomics3 |
log.sigma.2.0.mm.3D_firstorder_Kurtosis | First Order Features | −0.0024 | Radiomics4 |
log.sigma.2.0.mm.3D_firstorder_Maximum | First Order Features | −0.0038 | Radiomics5 |
log.sigma.2.0.mm.3D_glszm_GrayLevelVariance | GLSZM 1 Features | −0.0231 | Radiomics6 |
log.sigma.4.0.mm.3D_glszm_GrayLevelVariance | GLSZM 1 Features | −0.0132 | Radiomics7 |
log.sigma.5.0.mm.3D_glszm_SizeZoneNonUniformity | GLSZM 1 Features | −0.0149 | Radiomics8 |
wavelet.HLH_firstorder_Skewness | First Order Features | 0.0069 | Radiomics9 |
wavelet.HLH_glcm_MCC | GLCM 2 Features | 0.0094 | Radiomics10 |
wavelet.HLH_glrlm_LongRunLowGrayLevelEmphasis | GLRLM 3 Features | −0.0156 | Radiomics11 |
wavelet.HLH_glszm_LargeAreaLowGrayLevelEmphasis | GLSZM 1 Features | −0.0590 | Radiomics12 |
wavelet.LLL_firstorder_10Percentile | First Order Features | −0.2494 | Radiomics13 |
*Note: 1 Gray Level Size Zone Matrix. 2 Gray Level Cooccurrence Matrix. 3 Gray Level Run Length Matrix. |
COPD stage comparison | Radiomics1 | Radiomics2 | Radiomics3 | Radiomics4 | Radiomics5 |
Stage 0 vs. Stage Ⅰ | 0.0067 | 0.0675(ns) | < 0.0001 | 0.0018 | > 0.9999 (ns) |
Stage 0 vs. Stage Ⅱ | 0.0034 | 0.0951(ns) | 0.0001 | 0.0062 | > 0.9999 (ns) |
Stage 0 vs. Stage Ⅲ & Ⅳ | < 0.0001 | > 0.9999 (ns) | 0.0003 | > 0.9999 (ns) | > 0.9999 (ns) |
Stage Ⅰ vs. Stage Ⅱ | > 0.9999 (ns) | > 0.9999 (ns) | > 0.9999 (ns) | > 0.9999 (ns) | > 0.9999 (ns) |
Stage Ⅰ vs. Stage Ⅲ & Ⅳ | 0.0495 | 0.0700(ns) | > 0.9999 (ns) | 0.0425 | > 0.9999 (ns) |
Stage Ⅱ vs. Stage Ⅲ & Ⅳ | 0.03318 | 0.0987(ns) | > 0.9999 (ns) | 0.1213(ns) | > 0.9999 (ns) |
COPD stage comparison | Radiomics6 | Radiomics7 | Radiomics8 | Radiomics9 | Radiomics10 |
Stage 0 vs. Stage Ⅰ | > 0.9999 (ns) | > 0.9999 (ns) | 0.0295 | > 0.9999 (ns) | > 0.9999 (ns) |
Stage 0 vs. Stage Ⅱ | 0.06115 (ns) | > 0.9999 (ns) | 0.3687 (ns) | > 0.9999 (ns) | > 0.9999 (ns) |
Stage 0 vs. Stage Ⅲ & Ⅳ | > 0.9999 (ns) | > 0.9999 (ns) | > 0.9999 (ns) | 0.0014 | 0.5143 (ns) |
Stage Ⅰ vs. Stage Ⅱ | 0.0508 (ns) | > 0.9999(ns) | > 0.9999 (ns) | > 0.9999 (ns) | > 0.9999 (ns) |
Stage Ⅰ vs. Stage Ⅲ & Ⅳ | > 0.9999 (ns) | > 0.9999 (ns) | 0.3202 (ns) | 0.0070 | 0.8122 (ns) |
Stage Ⅱ vs. Stage Ⅲ & Ⅳ | 0.0480 | 0.8283 (ns) | > 0.9999 (ns) | 0.0125 | > 0.9999 (ns) |
COPD stage comparison | Radiomics11 | Radiomics12 | Radiomics13 | ||
Stage 0 vs. Stage Ⅰ | > 0.9999 (ns) | 0.0002 | < 0.0001 | ||
Stage 0 vs. Stage Ⅱ | > 0.9999 (ns) | 0.0081 (ns) | < 0.0001 | ||
Stage 0 vs. Stage Ⅲ & Ⅳ | 0.0330 | < 0.0001 | < 0.0001 | ||
Stage Ⅰ vs. Stage Ⅱ | > 0.9999(ns) | > 0.9999 (ns) | 0.4088 (ns) | ||
Stage Ⅰ vs. Stage Ⅲ & Ⅳ | 0.4837(ns) | 0.3096 (ns) | < 0.0001 | ||
Stage Ⅱ vs. Stage Ⅲ & Ⅳ | 0.1819(ns) | 0.0089 | 0.0030 | ||
*Note: ns: no significance. |
COPD stage comparison | Y1 | Y2 | Y3 | Y4 |
Stage 0 vs. Stage Ⅰ | 0.0542 (ns) | 0.0641 (ns) | > 0.9999 (ns) | < 0.0013 |
Stage 0 vs. Stage Ⅱ | < 0.0001 | 0.1686 (ns) | 0.0686 (ns) | < 0.0001 |
Stage 0 vs. Stage Ⅲ & Ⅳ | < 0.0001 | > 0.9999 (ns) | > 0.9999 (ns) | < 0.0001 |
Stage Ⅰ vs. Stage Ⅱ | 0.0718 (ns) | > 0.9999 (ns) | 0.3835 (ns) | 0.1287 (ns) |
Stage Ⅰ vs. Stage Ⅲ & Ⅳ | < 0.0001 | 0.0729 (ns) | > 0.9999 (ns) | < 0.0001 |
Stage Ⅱ vs. Stage Ⅲ & Ⅳ | < 0.0039 | 0.1851(ns) | 0.1143 (ns) | 0.0619 (ns) |
*Note: ns: no significance. |
COPD stage comparison | F1 | F2 |
Stage 0 vs. Stage Ⅰ | 0.0031 | 0.0479 |
Stage 0 vs. Stage Ⅱ | < 0.0001 | < 0.0001 |
Stage 0 vs. Stage Ⅲ & Ⅳ | < 0.0001 | < 0.0001 |
Stage Ⅰ vs. Stage Ⅱ | 0.0646 (ns) | 0.0085 |
Stage Ⅰ vs. Stage Ⅲ & Ⅳ | < 0.0001 | < 0.0001 |
Stage Ⅱ vs. Stage Ⅲ & Ⅳ | < 0.0014 | 0.0342 |
*Note: ns: no significance. |