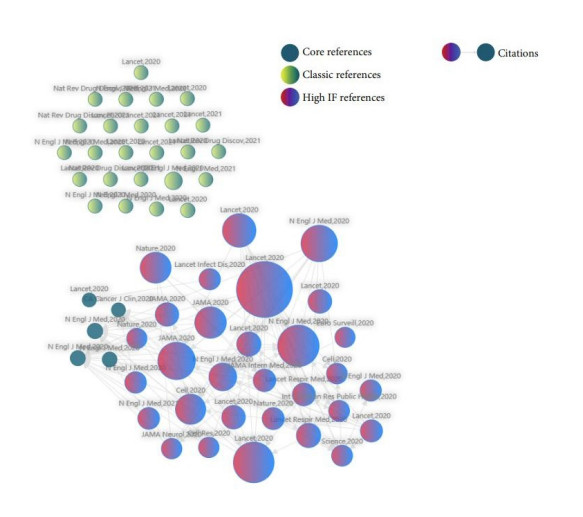
To predict COVID-19 severity by building a prediction model based on the clinical manifestations and radiomic features of the thymus in COVID-19 patients.
We retrospectively analyzed the clinical and radiological data from 217 confirmed cases of COVID-19 admitted to Xiangyang NO.1 People's Hospital and Jiangsu Hospital of Chinese Medicine from December 2019 to April 2022 (including 118 mild cases and 99 severe cases). The data were split into the training and test sets at a 7:3 ratio. The cases in the training set were compared in terms of clinical data and radiomic parameters of the lasso regression model. Several models for severity prediction were established based on the clinical and radiomic features of the COVID-19 patients. The DeLong test and decision curve analysis (DCA) were used to compare the performances of several models. Finally, the prediction results were verified on the test set.
For the training set, the univariate analysis showed that BMI, diarrhea, thymic steatosis, anorexia, headache, findings on the chest CT scan, platelets, LDH, AST and radiomic features of the thymus were significantly different between the two groups of patients (P < 0.05). The combination model based on the clinical and radiomic features of COVID-19 patients had the highest predictive value for COVID-19 severity [AUC: 0.967 (OR 0.0115, 95%CI: 0.925-0.989)] vs. the clinical feature-based model [AUC: 0.772 (OR 0.0387, 95%CI: 0.697-0.836), P < 0.05], laboratory-based model [AUC: 0.687 (OR 0.0423, 95%CI: 0.608-0.760), P < 0.05] and model based on CT radiomics [AUC: 0.895 (OR 0.0261, 95%CI: 0.835-0.938), P < 0.05]. DCA also confirmed the high clinical net benefits of the combination model. The nomogram drawn based on the combination model could help differentiate between the mild and severe cases of COVID-19 at an early stage. The predictions from different models were verified on the test set.
Severe cases of COVID-19 had a higher level of thymic involution. The thymic differentiation in radiomic features was related to disease progression. The combination model based on the radiomic features of the thymus could better promote early clinical intervention of COVID-19 and increase the cure rate.
Citation: Peng An, Xiumei Li, Ping Qin, YingJian Ye, Junyan Zhang, Hongyan Guo, Peng Duan, Zhibing He, Ping Song, Mingqun Li, Jinsong Wang, Yan Hu, Guoyan Feng, Yong Lin. Predicting model of mild and severe types of COVID-19 patients using Thymus CT radiomics model: A preliminary study[J]. Mathematical Biosciences and Engineering, 2023, 20(4): 6612-6629. doi: 10.3934/mbe.2023284
[1] | Yingjian Yang, Wei Li, Yingwei Guo, Nanrong Zeng, Shicong Wang, Ziran Chen, Yang Liu, Huai Chen, Wenxin Duan, Xian Li, Wei Zhao, Rongchang Chen, Yan Kang . Lung radiomics features for characterizing and classifying COPD stage based on feature combination strategy and multi-layer perceptron classifier. Mathematical Biosciences and Engineering, 2022, 19(8): 7826-7855. doi: 10.3934/mbe.2022366 |
[2] | Michael James Horry, Subrata Chakraborty, Biswajeet Pradhan, Maryam Fallahpoor, Hossein Chegeni, Manoranjan Paul . Factors determining generalization in deep learning models for scoring COVID-CT images. Mathematical Biosciences and Engineering, 2021, 18(6): 9264-9293. doi: 10.3934/mbe.2021456 |
[3] | Yingjian Yang, Wei Li, Yan Kang, Yingwei Guo, Kai Yang, Qiang Li, Yang Liu, Chaoran Yang, Rongchang Chen, Huai Chen, Xian Li, Lei Cheng . A novel lung radiomics feature for characterizing resting heart rate and COPD stage evolution based on radiomics feature combination strategy. Mathematical Biosciences and Engineering, 2022, 19(4): 4145-4165. doi: 10.3934/mbe.2022191 |
[4] | Javad Hassannataj Joloudari, Faezeh Azizi, Issa Nodehi, Mohammad Ali Nematollahi, Fateme Kamrannejhad, Edris Hassannatajjeloudari, Roohallah Alizadehsani, Sheikh Mohammed Shariful Islam . Developing a Deep Neural Network model for COVID-19 diagnosis based on CT scan images. Mathematical Biosciences and Engineering, 2023, 20(9): 16236-16258. doi: 10.3934/mbe.2023725 |
[5] | Zhihao Zhang, Ting Zeng, Yijia Wang, Yinxia Su, Xianghua Tian, Guoxiang Ma, Zemin Luan, Fengjun Li . Prediction Model of hospitalization time of COVID-19 patients based on Gradient Boosted Regression Trees. Mathematical Biosciences and Engineering, 2023, 20(6): 10444-10458. doi: 10.3934/mbe.2023459 |
[6] | Zhenwu Xiang, Qi Mao, Jintao Wang, Yi Tian, Yan Zhang, Wenfeng Wang . Dmbg-Net: Dilated multiresidual boundary guidance network for COVID-19 infection segmentation. Mathematical Biosciences and Engineering, 2023, 20(11): 20135-20154. doi: 10.3934/mbe.2023892 |
[7] | XiaoQing Zhang, GuangYu Wang, Shu-Guang Zhao . CapsNet-COVID19: Lung CT image classification method based on CapsNet model. Mathematical Biosciences and Engineering, 2022, 19(5): 5055-5074. doi: 10.3934/mbe.2022236 |
[8] | Yutao Wang, Qian Shao, Shuying Luo, Randi Fu . Development of a nomograph integrating radiomics and deep features based on MRI to predict the prognosis of high grade Gliomas. Mathematical Biosciences and Engineering, 2021, 18(6): 8084-8095. doi: 10.3934/mbe.2021401 |
[9] | Suzan Farhang-Sardroodi, Mohammad Sajjad Ghaemi, Morgan Craig, Hsu Kiang Ooi, Jane M Heffernan . A machine learning approach to differentiate between COVID-19 and influenza infection using synthetic infection and immune response data. Mathematical Biosciences and Engineering, 2022, 19(6): 5813-5831. doi: 10.3934/mbe.2022272 |
[10] | Luca Dedè, Francesco Regazzoni, Christian Vergara, Paolo Zunino, Marco Guglielmo, Roberto Scrofani, Laura Fusini, Chiara Cogliati, Gianluca Pontone, Alfio Quarteroni . Modeling the cardiac response to hemodynamic changes associated with COVID-19: a computational study. Mathematical Biosciences and Engineering, 2021, 18(4): 3364-3383. doi: 10.3934/mbe.2021168 |
To predict COVID-19 severity by building a prediction model based on the clinical manifestations and radiomic features of the thymus in COVID-19 patients.
We retrospectively analyzed the clinical and radiological data from 217 confirmed cases of COVID-19 admitted to Xiangyang NO.1 People's Hospital and Jiangsu Hospital of Chinese Medicine from December 2019 to April 2022 (including 118 mild cases and 99 severe cases). The data were split into the training and test sets at a 7:3 ratio. The cases in the training set were compared in terms of clinical data and radiomic parameters of the lasso regression model. Several models for severity prediction were established based on the clinical and radiomic features of the COVID-19 patients. The DeLong test and decision curve analysis (DCA) were used to compare the performances of several models. Finally, the prediction results were verified on the test set.
For the training set, the univariate analysis showed that BMI, diarrhea, thymic steatosis, anorexia, headache, findings on the chest CT scan, platelets, LDH, AST and radiomic features of the thymus were significantly different between the two groups of patients (P < 0.05). The combination model based on the clinical and radiomic features of COVID-19 patients had the highest predictive value for COVID-19 severity [AUC: 0.967 (OR 0.0115, 95%CI: 0.925-0.989)] vs. the clinical feature-based model [AUC: 0.772 (OR 0.0387, 95%CI: 0.697-0.836), P < 0.05], laboratory-based model [AUC: 0.687 (OR 0.0423, 95%CI: 0.608-0.760), P < 0.05] and model based on CT radiomics [AUC: 0.895 (OR 0.0261, 95%CI: 0.835-0.938), P < 0.05]. DCA also confirmed the high clinical net benefits of the combination model. The nomogram drawn based on the combination model could help differentiate between the mild and severe cases of COVID-19 at an early stage. The predictions from different models were verified on the test set.
Severe cases of COVID-19 had a higher level of thymic involution. The thymic differentiation in radiomic features was related to disease progression. The combination model based on the radiomic features of the thymus could better promote early clinical intervention of COVID-19 and increase the cure rate.
Since the first outbreak of COVID-19 in Wuhan in December 2019, COVID-19 has been notoriously known for its fast dissemination and high mortality. As of August 14, 2022, WHO announced that COVID-19 has affected 215 countries and districts throughout the world [1,2]. The cumulative number of COVID-19 cases is 587 million, with 6.4 million deaths. COVID-19 has brought unprecedented disasters to the socioeconomic and healthcare systems of nearly every part of the world. We have to admit that this epidemic originating in China has brought huge economic and survival catastrophes to the world. Autopsies have confirmed that patients who have died of COVID-19 have a significant reduction in the number of lymphocytes in immune organs, such as the spleen, which is usually accompanied by focal bleeding and necrosis [3,4]. The thymus is an important lymphatic organ of the human body and closely related to immunity. It is the site where T-cells are differentiated, develop and mature. The thymus can also secrete thymus hormones and hormone-like substances. This organ is also affected by COVID-19. It is reported that thymus α1 and thymopentin secreted by thymic tissue can significantly reduce the severity and mortality of COVID-19 by increasing the percentage of lymphocytes and activating the proliferation of T cells. Thymopeptide also plays an important role in the treatment and improvement of multiple organ failure and systemic infection (bacteremia and toxemia) in the late stage of COVID-19. In addition, normal thymic function plays an important role in supporting the recovery from COVID-19. Therefore, it is of great clinical significance to carry out thymus research in patients with COVID-19. Novelty of this research: We observed significant differences in the CT manifestations of the thymus between severe and mild cases of COVID-19. These findings can inform understanding about the pathological evolution of COVID-19 and the early intervention of COVID-19 to improve the prognosis. Few reports have been published in this respect yet. Also, most of the existing studies are solely based on clinical features or CT scans of lungs and therefore lack systematicity. At the same time, the pulmonary CT manifestations have a certain lag, and some patients have entered a critical state before the pulmonary CT abnormalities. Therefore, there are certain defects in chest CT prediction, and we need to introduce other new predictive factors to improve the prediction performance (Figure 1) [5,6,7]. Contributions: In the present study, first, we tested and verified the difference of thymic CT radiomics between the two groups with eight machine learning methods. Then, we further summarized the clinical, laboratory and chest CT data, and we then added the analysis of thymic CT radiomics data and established four prediction models (clinical data model, laboratory model, CT radiomics model, combined model), which finally confirmed that the combined model had the highest prediction efficiency (AUC: 0.967), which was verified in the test set (AUC: 0.979). This combination model provides supports for COVID-19 management and treatment, or it may further improve the treatment and survival rate of severe cases, which has not been reported before.
We collected clinical data and chest CT scan images from 229 COVID-19 patients who were admitted to Xiangyang NO.1 People's Hospital and Jiangsu Hospital of Chinese Medicine from December 2019 to April 2022, including 131 males and 98 females. They were aged 16 to 45 years old. Eight patients with tumors; endocrine, immunological and hematological diseases; thymic lesions; or a history of surgery, together with four cases lost to the follow-up, were excluded. Finally, 217 cases were recruited, including 118 mild cases and 99 severe cases. Some other data were also considered: smoking history, history of alcohol consumption, BMI, age, white blood cell count, red blood cell count, and liver and kidney functions (Figures 2 and 3) [8].
CT scans were performed using a GE Light Speed 64-row 128-slice spiral CT scanner, with tube voltage of 120 kV, tube current of 50–200 mA, 512 × 512 matrix and slice thickness of 1 mm. The scan was performed from bilateral lung apexes to the pancreas. The CT images were reconstructed at slice thickness of 1.0 mm and slice interval of 1.0 mm. The images were sent to the PACS server in the DICOM format. Two senior radiologists with over 10 years of working experience reviewed the CT images of thymus on the PACS server. An agreement was reached concerning the interpretation of the CT scan results through negotiations between the two radiologists. The window width was set to 300–400 HU, and the window position was -50 to -60 HU [11].
According to Ackman et al., thymi are divided into four types. 1) Fatty: The gland is completely replaced by fatty tissues, and there is no thymic parenchyma recognizable. 2) Predominance of fatty tissues: There is a small amount of thymic parenchyma (10–30%). 3) Fatty tissues and soft tissues each accounting for one half: The ratio of fatty tissues to thymic parenchyma is about 1:1 (about 50%). 4) Predominance of thymic soft tissues (≥60%): The density of the thymus is close to that of muscles. In the present study, thymi where fatty tissues were predominant, where fatty and soft tissues each accounted for half of total tissues, and where soft tissues were predominant were collectively known as non-fatty thymi [9,10,11].
The transverse diameter, anteroposterior diameter, superoinferior diameter and average CT value were measured for the thymi. During CT value measurement, the region of interest (ROI) was delineated to cover the entire thymus, avoiding artifacts and mediastinal fat. Each measurement was repeated three times, and the average was taken [12,13,14]. The ROI was delineated in the entire thymus, and the high-throughput radiographic texture parameters were extracted using 3D Slicer (including morphological features, gray-level size zone matrix, histogram features, gray-level co-occurrence matrix and length matrix). These texture parameters were analyzed using the eight machine learning algorithms (including XGboost, AdaBoost, SVM, Naive Bayes, Random forest, GBDT, KNN, Bagging). Finally, the largest areas under the ROC curve of the mentioned algorithms were compared. CT radiomics was analyzed, and Radscore was extracted using lasso regression after the differences were verified between the two groups of radiomics using machine learning (Figure 4) [15,16,17].
The specific meanings of some texture parameters are as follows. 1) Histogram features include gray mean, maximum, minimum, variance and percentile. 2) Texture features: ① Absolute Gradient reflects the degree or suddenness of gray intensity fluctuation in an image. For two adjacent pixels or voxels, if one is black and the other is white, the gradient is the highest; if both pixels are black (or both are white), the gradient at this location is zero. ② Gray Level Co-occurrence Matrix (GLCM) reflects the predefined distance between pixels or voxels in different directions (horizontal, vertical or diagonal of 2D analysis or 13 directions of 3D analysis, and captures the spatial relationship of pixel pairs or voxel pairs with predefined gray intensity. ③ The Gray Level Run Length Matrix (GLRLM) provides information about the two-dimensional or three-dimensional spatial distribution of consecutive pixels of the same gray level in one or more directions. ④ The Gray Level Size Zone Matrix (GLSZM for short) is similar to GLRLM in principle, but the more uniform texture of GLSZM will lead to a wider and flatter matrix, which is helpful for the weight calculation of the system. ⑤ The Neighboring Gray Tone Difference Matrix (NGTDM) quantifies the sum of differences between the gray level of a pixel or voxel and the average gray level of its adjacent pixels or voxels within a predefined distance. ⑥ The Gray Level Dependence Matrix (GLDM) reflects the gray level similarity and gray level dependency in the entire ROI.
Statistical analyses were performed using Jamovi 2.23 software. Categorical data were expressed as percentages (%), and measurement data were expressed as mean ± standard deviation. The categorical data were analyzed by using the chi-square test or Fisher's exact test. The measurement data were analyzed by using the t-test (if obeying a normal distribution) or the rank-sum test (if not obeying a normal distribution). The data were split into the training and test sets at a 7:3 ratio. Statistically significant variables on the training set were subject to logistic regression. Four models were built, namely, the clinical feature-based model, laboratory-based model, model based on CT radiomics of thymus and combination model. The diagnostic efficacies of different models were compared using the DeLong test. The clinical net benefits of the models were verified by DCA. Finally, the above results were verified on the test set, and a nomogram was drawn for most efficient model. A p-value below 0.05 was statistically significant [17,18].
The univariate analysis showed that BMI, diarrhea, findings on the chest CT scan, fatigue and myalgia, headache, limb twitch and the prevalence of anorexia were significantly different between the two groups of patients (P < 0.05). Multivariate logistic regression showed that BMI, diarrhea, anorexia and manifestations on the chest CT scan were independent risk factors predicting the severity of COVID-19 (Table 1).
Clinical data model | Univariate analysis | Multivariate analysis | ||
Factor | P | Hazard ratio | P | Hazard ratio |
Gender | 0.768 | 1.084 (0.634–1.851) | ||
BMI | 0.001* | 1.167 (1.065–1.278) | 0.010* | 1.137 (1.031–1.254) |
Smoking history | 0.747 | 0.916 (0.537–1.560) | ||
Drinking history | 0.443 | 0.811 (0.475–1.381) | ||
Age | 0.225 | 1.035 (0.979–1.090) | ||
cough | 0.106 | 1.566 (0.909–2.698) | ||
diarrhea | 0.002* | 2.451 (1.378–4.358) | 0.008* | 2.358 (1.246–4.463) |
Chest CT findings | 0.002* | 2.402 (1.386–4.163) | 0.009* | 2.217 (1.221–4.029) |
fatigue and myalgia | 0.045* | 0.566 (0.325–0.986) | ||
anorexia | 0.001* | 2.821 (1.609–4.946) | 0.029* | 1.990 (1.075–3.684) |
headache | 0.019* | 0.517 (0.298–0.898) | ||
limb twitch | 0.029* | 0.541 (0.312–0.938) | ||
Social medical insurance | 0.620 | 1.016 (0.953–1.080) | ||
general education | 0.078 | 0.606 (0.348–1.060) |
The equation of the clinical data model is as follows:
![]() |
The univariate analysis confirmed that white blood cell count, platelet count, D-Dimer, LDH and AST were significantly different between the two groups of patients (P < 0.05). Multivariate logistic regression showed that white blood cell count, platelet count, LDH and D-Dimer were independent risk factors predicting the severity of COVID-19 (Table 2).
Laboratory examination model | Univariate analysis | Multivariate analysis | ||
Factor | P | Hazard ratio | P | Hazard ratio |
red blood cell | 0.580 | 1.147 (0.706–1.860) | ||
hemoglobin | 0.132 | 0.990 (0.977–1.001) | ||
white blood cell | 0.001* | 1.242 (1.088–1.418) | 0.001* | 1.271 (1.098–1.471) |
platelet | 0.002* | 0.995 (0.991–0.998) | 0.006* | 0.994 (0.991–1.001) |
ESR | 0.639 | 1.015 (0.953–1.080) | ||
D-Dimer | 0.002* | 1.401 (1.135–1.728) | 0.003* | 1.407 (1.126–1.759) |
LDH | 0.002* | 1.002 (1.001–1.004) | 0.007* | 1.002 (1.001–1.004) |
AST | 0.004* | 1.012 (1.004–1.020) | ||
ALT | 0.549 | 1.003 (0.993–1.010) | ||
uric acid | 0.260 | 1.001 (0.998–1.001) | ||
TC | 0.342 | 0.919 (0.773–1.090) |
The equation of the laboratory examination model is as follows:
![]() |
We used 3D Slicer software to extract the high-throughput texture information from the conventional thymus CT scan images. After removing the confounding factors and lost packets, we obtained 852 groups of texture parameters, including Histogram features, Absolute Gradient, GLCM, GLRLM, GLSZM, NGTDM, GLDM, etc.
The above available texture parameters were analyzed using two groups of methods. ① Eight machine learning algorithms' AUC values (AdaBoost-0.549, bagging-0.460, SVM-0.978, naive Bayes-0.696, random forest-0.991, XGboost-0.994, KNN-0.959, GBDT-0.626): Finally, the XGboost was confirmed to obtain the maximum AUC (P < 0.05). That is to say, CT radiomics is feasible to differentiate COVID-19 severe cases, as confirmed by machine learning. ② Lasso regression method: 17 useful radiomics data were extracted by lasso regression, and then the final weighted radiomics score (Radscore) was calculated (P < 0.05). Subsequently, multivariate regression analysis confirmed that average CT value, transverse diameter, thymus steatosis and Radscore were independent influencing factors for predicting mild and severe groups (Figures 5 and 6, Tables 3 and 4).
Thymus CT feature model | Univariate analysis | Multivariate analysis | ||
Factor | p | Hazard ratio | p | Hazard ratio |
average CT value | 0.001* | 0.784 (0.675–0.911) | 0.002* | 0.743 (0.615–0.897) |
transverse diameter | 0.001* | 0.888 (0.837–0.942) | 0.011* | 0.908 (0.843–0.979) |
axial diameter | 0.392 | 0.874 (0.641–1.190) | ||
Anteroposterior diameter | 0.251 | 1.447 (0.770–2.720) | ||
thymus steatosis | 0.003* | 1.074 (1.025–1.125) | 0.014* | 1.076 (1.015–1.140) |
Lung CT value | 0.368 | 0.594 (0.192–1.840) | ||
Liver CT value | 0.878 | 0.995 (0.933–1.060) | ||
Radscore | 0.001* | 2.356 (1.857–2.989) | 0.001* | 2.345 (1.816–3.028) |
notes: Radscore = -0.20463620+0.08033656*Maximum2DDiameterRow-0.24104915*SurfaceVolumeRatio+0.03263107*Idn...46-0.09277765*DependenceVariance...61-0.05773797*SmallAreaLowGrayLevelEmphasis...100+0.12575685*Maximum...115+0.02108543*ClusterShade...129-0.36639420*Median...211+0.08557059*Idn...232+0.22752327*MCC...239+0.11411019*RunEntropy...359+0.23346851*ClusterShade...408+0.29022507*InverseVariance...421+0.05698528*InverseVariance...514+0.47092109*RunVariance...735-0.03106958*Kurtosis...765 |
The equation of the thymus CT feature model is as follows:
![]() |
We built the combination model based on the potentially significant risk factors. The multivariate logistic analysis suggested that chest CT manifestations, anorexia, white blood cell count, platelet count, D-Dimer, LDH, average CT value of thymus transverse diameter of thymus and Radscore were independent risk factors predicting the severity of COVID-19 (Table 5).
Combined model | Univariate analysis | Multivariate analysis | ||
Factors | p | Hazard ratio | p | Hazard ratio |
BMI | 0.001* | 1.167 (1.065–1.278) | ||
diarrhea | 0.002* | 2.451 (1.378–4.358) | ||
Chest CT findings | 0.002* | 2.402 (1.386–4.163) | 0.015* | 3.586 (1.285–10.007) |
fatigue and myalgia | 0.045* | 0.566 (0.325–0.986) | ||
anorexia | 0.001* | 2.821 (1.609–4.946) | 0.025* | 3.450 (1.164–10.226) |
headache | 0.019* | 0.517 (0.298–0.898) | ||
limb twitch | 0.029* | 0.541 (0.312–0.938) | ||
white blood cell | 0.001* | 1.242 (1.088–1.418) | 0.001* | 1.608 (1.226–2.109) |
platelet | 0.002* | 0.995 (0.991–0.998) | 0.002* | 0.989 (0.983–0.997) |
D-Dimer | 0.002* | 1.401 (1.135–1.728) | 0.001* | 1.866 (1.315–2.649) |
LDH | 0.002* | 1.002 (1.001–1.004) | 0.001* | 1.005 (1.002–1.008) |
AST | 0.004* | 1.012 (1.004–1.020) | ||
average CT value | 0.001* | 0.784 (0.675–0.911) | 0.001* | 0.544 (0.402–0.737) |
transverse diameter | 0.001* | 0.888 (0.837–0.942) | 0.002* | 0.830 (0.740–0.931) |
thymus steatosis | 0.003* | 1.074 (1.025–1.125) | ||
Radscore | 0.001* | 2.356 (1.857–2.989) | 0.001* | 2.748 (1.863–4.052) |
The equation of the Combined model is as follows:
The DeLong test was performed for all of the 4 prediction models on the training set using MedCalc 20.144. It was found that the combination model had the highest predictive efficiency [AUC: 0.967 (OR 0.0115, 95%CI: 0.925-0.989)]. The difference was significant compared with the clinical feature-based model [AUC: 0.772 (OR 0.0387, 95%CI: 0.697-0.836), P < 0.05], laboratory-based model [AUC: 0.687 (OR 0.0423, 95%CI: 0.608-0.760), P < 0.05] and model based on the CT radiomics [AUC: 0.895 (OR 0.0261, 95%CI: 0.835-0.938), P < 0.05]. The combination model had a much higher predictive performance on the test set [AUC: 0.979 (OR 0.0199, 95%CI: 0.908-0.999)] compared with the clinical feature-based model [AUC: 0.801 (OR 0.0554, 95%CI: 0.682-0.890), P < 0.05], laboratory-based model [AUC: 0.829 (OR 0.0506, 95%CI: 0.714-0.911), P < 0.05] and model based on the CT radiomics [AUC: 0.796 (OR 0.0571, 95%CI: 0.677-0.887), P < 0.05]. The subsequent DCA also confirmed the high clinical net benefits of the combination model on the training and test sets. Finally, we drew the nomograms from the combined model, which proved to have an excellent clinical performance (Figures 7–9).
The worldwide spread of COVID-19 has caused huge health burdens and economic catastrophe [19,20]. The spread of COVID-19 has been curbed to a certain degree along with the emergence of vaccines and targeted agents, increase in the positive rate of nucleic acid detection and tightening of regional COVID-19 control measures. Nevertheless, the incidence of critically ill COVID-19 cases has been the main concern for WHO. It was reported that COVID-19 progression can be predicted based on clinical and biochemical indicators or lung CT scans. An early prediction of severe COVID-19 can help with disease management and survival improvement. However, there may be significant individual differences as long as COVID-19 is concerned. The above method may not predict all severe cases in the target population. Although the thymus is an important immune organ, its crucial role in the immune resistance against viruses may have been neglected before [21,22,23,24]. It is important that we introduced CT-based radiomics of the thymus into the prediction model, which adequately compensates for the defects of the previous models.
We searched the PubMed database and found few studies that combined clinical and radiomic features for predicting severe COVID-19 (fewer than 30 studies). COVID-19 studies based on the radiomics of the thymus are even more rare. It has been reported that thymic steatosis and volume shrinkage are closely related to age [24,25]. In an aging thymus, there is usually a reduction in the lymphocyte count and density and an increase in the content of fatty tissues. This fact explains the generally poor immunity of the elderly people. To reduce biases, we eliminated those aged above 55 and having thymic involution. Our subjects were only young and middle-aged adults. The thymus is an important lymphoid organ and the site for T-cell production. T-cells play a key role in cell-mediated immunity. Thymic involution can cause a reduction in the number of T-cells and the cell-mediated immunity. In addition, obesity, infections and abnormal renal and liver functions will cause premature thymic involution and fat accumulation. As a result, there will be fewer thymus glands to produce juvenile T-cells, causing platelet disorders, which further increases the risk of infections [26,27,28]. In the present study, severe cases of COVID-19 were more commonly associated with abnormalities in the level of thymic steatosis, thymic volume, thymic density, CT value, BMI and white blood cell count. Such abnormalities had a critical impact on the patients' immune functions, which may be induced by COVID-19 related damage to the thymus [29,30,31]. In addition, the thymus degeneration and thymus steatosis in the severe group were as high as 76.5% and 79.6%, which objectively confirmed the important role of the thymus in severe COVID-19.
Radiographic texture analysis was first proposed by the Dutch researcher Lambin in 2012 [32,33] and initially used for grayscale image recognition. It was soon found that computer vision outperformed the naked eyes in texture recognition. 3D Slicer and ITK-snap, jointly developed by several universities, including Princeton University and Cornell University, have extended radiomics to radiology and pathology. Such an advance is beneficial for upgrading clinical treatment and diagnosis. John McCarthy started the Intelligent System Science and Engineering Project in 1955, which later evolved into artificial intelligence and machine learning, the latter being a subdiscipline of the former. Both have greatly fueled the development in data optimization and processing, which further contributes to the overall human progress [32,33,34]. In addition, our team, together with the National Institutes of Health of the United States (NIH), previously reported on the research of lung CT deep learning/machine learning aided diagnosis COVID-19 in Nature Communications, which has had a great impact and provides support for data mining of subsequent COVID-19 diagnoses. Machine learning and radiomics have great advantages in data extraction and analysis of COVID-19 lung CT images. It can accurately segment images to extract different two-dimensional voxel values and conduct extensive analysis in a wide area to further discover subtle lesions that cannot be recognized by the human eye. In short, the result of image data processed by computer is obviously better than that of human eye analysis, which will greatly benefit patients and improve the diagnostic efficiency of many diseases. To predict severe COVID-19, we combined radiographic textures with machine learning and achieved satisfactory efficacy. From 852 groups of texture parameters, we extracted 17 groups of reliable data using the lasso regression and generated the Radscore. The above data represented signal intensity, density and grayscale difference of the thymus. Such information could be used to characterize minor structural and functional changes of the thymus in the two groups [35,36,37]. In addition, the XGboost algorithm has more advantages. 1) When searching for the best segmentation point, considering the traditional greedy method of enumerating all possible segmentation points of each feature is too inefficient, and XGboost implemented an approximate algorithm. The general idea is to list several candidates which may become segmentation points according to the percentile method and then calculate the best segmentation point from the candidates according to the above formula for segmentation points. 2) XGboost considers the situation that the training data is sparse at some times and can specify the default direction of branches for missing values or specified values, which can greatly improve the efficiency of the algorithm. 3) XGboost feature columns are sorted and stored in memory in blocks, which can be reused during iteration. Although the boosting algorithm iteration must be serial, it can be parallel when processing each feature column using XGboost. 4) XGboost also considers how to use the disk effectively when the amount of data is large, and the memory is not enough. It mainly combines multithreading, data compression and sharding methods to improve the efficiency of the algorithm as much as possible. Therefore, the XGboost algorithm is superior to other machine learning algorithms to some extent in this study. We constructed four models based on all of the identified risk factors, using data from the training set. The predictions from the four models were then verified on the test set. The DeLong test and DCA showed that the combination model had higher predictive performance and clinical net benefits than other models. The nomograph based on the combination model also achieved a good clinical performance. This was probably because the nomogram based on the combination model integrated the clinical features and radiomic features of the thymus upon the CT scan. Thus, from the nomogram based on the combination model, we could obtain parameters fully representing individual differences of the thymi between the mild and severe cases. Therefore, the predictions were more accurate using the combination model [38,39].
The present case control study had certain limitations. For example, there were some intrinsic defects in data collection, and the follow-up was not long enough to observe the subsequent changes in the thymus. Biases are inevitable with double-center studies, so it is necessary to increase the sample size and perform a multi-center study to verify the findings in the present study [40,41]. Regression model has a wide range of clinical application scenarios, but there are also many defects, such as high standard data differentiation (P ˂ 0.05), which may filter out some valuable data. In the future, multiple deep learning models are required to verify the results.
To conclude, Chest CT findings, anorexia, white blood cell, platelet, D-Dimer, LDH, average CT value, transverse diameter and radscore were risk factors predicting severe COVID-19. The combination model with maximum prediction efficiency [AUC: 0.967 (OR 0.0115, 95%CI: 0.925-0.989)] could more effectively predict severe COVID-19 at an early stage and allow for an early adjustment of the therapeutic regimen, thereby improving patients' life quality and survival rate. The combination model may be worthy of wider clinical applications.
The experimental protocol was established according to the ethical guidelines of the Helsinki Declaration and was approved by the Human Ethics Committee of Xiangyang NO.1 People's Hospital affiliated to Hubei University of Medicine (Issue No. 3012 [2021]). Written informed consent was obtained from individual or guardian participants.
Peng An, Guoyan Feng and Yong Lin conceived and drafted the manuscript. Peng An, Junyan Zhang, Ping Qin and Ping Song contributed to the literature review. YingJian Ye, Hongyan Guo, Mingqun Li, Peng Duan were responsible for the quality control of article statistics. Guoyan Feng, Zhibing He, Jingsong Wang revised the manuscript critically for important intellectual content. Yong Lin, Guoyan Feng, and Yan Hu approved the final version to be published and agreed to act as guarantors of the work. Peng An, Xiumei Li and Ping Qin contributed equally to this work.
2021 Science and Technology Innovation Project of Xiangyang No.1 People's Hospital: Research on Risk Stratification Decision and Key Problems of Prostate Cancer Based on In-Depth Learning of MRI/Ultrasound Radiomics (XYY2021Q16, PENG AN). This work was supported by research grants from the National Natural Science Foundation of China (81901567 to Peng Duan), the Natural Science Foundation of Hubei Provincial Department of Education (Q20202105 to Peng Duan), the Scientific and Technological Project of Xiangyang City of Hubei Province (2021YL29 to Peng Duan) and the Innovative Research Program of Xiangyang No.1 People's Hospital (XYY2021M02 to Peng Duan). The "323" Public Health Project of the Hubei health commission and the Xiangyang No.1 People's Hospital (XYY2022-323). The value of digital breast tomosynthesis (DBT) and dynamic contrast-enhanced magnetic resonance image (DCE-MRI) in that diagnosis of early breast cancer (Zhibing He). Graduate Education Research Project Fund of Hubei University of Medicine (YJ2022013) (YingJian Ye), Xiangyang Science and Technology Plan Key Project Fund (2022YL34A) (YingJian Ye).
The authors declare there is no conflict of interest.
[1] |
M. Herrero-Montes, C. Fernández-de-Las-Peñas, D. Ferrer-Pargada, S. Tello-Mena, I. Cancela-Cilleruelo, J. Rodríguez-Jiménez, et al., Prevalence of neuropathic component in post-COVID pain symptoms in previously hospitalized COVID-19 survivors, Int. J. Clin. Pract., 2022 (2022), 3532917. https://doi:10.1155/2022/3532917 doi: 10.1155/2022/3532917
![]() |
[2] |
S. Abuhammad, O. F. Khabour, K. H. Alzoubi, F. El-Zubi, S. H. Hamaieh, Respiratory infectious diseases and adherence to nonpharmacological interventions for overcoming COVID-19 pandemic: A self-reported study, Int. J. Clin. Pract., 2022 (2022), 4495806. https://doi:10.1155/2022/4495806 doi: 10.1155/2022/4495806
![]() |
[3] |
N. Demir, B. Yüzbasıoglu, T. Calhan, S. Ozturk, Prevalence and prognostic importance of high fibrosis-4 index in COVID-19 patients, Int. J. Clin. Pract., 2022 (2022), 1734896. https://doi:10.1155/2022/1734896 doi: 10.1155/2022/1734896
![]() |
[4] |
S. Tharwat, H. A. Abdelsalam, A. Abdelsalam, M. K. Nassar, COVID-19 vaccination intention and vaccine hesitancy among patients with autoimmune and autoinflammatory rheumatological diseases: A survey, Int. J. Clin. Pract., 2022 (2022), 5931506. https://doi:10.1155/2022/5931506 doi: 10.1155/2022/5931506
![]() |
[5] |
Y. Liu, Y. Pan, Z. Hu, M. Wu, C. Wang, Z. Feng, et al., Thymosin Alpha 1 reduces the mortality of severe coronavirus disease 2019 by restoration of lymphocytopenia and reversion of exhausted T cells, Clin. Infect. Dis., 71 (2020), 2150–2157. https://doi:10.1093/cid/ciaa630 doi: 10.1093/cid/ciaa630
![]() |
[6] |
V. J. Sharmila, D. Jemi Florinabel, Deep learning algorithm for COVID-19 classification using chest X-ray images, Comput. Math. Methods Med., 2021 (2021), 9269173. https://doi:10.1155/2021/9269173 doi: 10.1155/2021/9269173
![]() |
[7] |
W. C. Serena Low, J. H. Chuah, C. A. T. H. Tee, S. Anis, M. A. Shoaib, A. Faisal, et al., An overview of deep learning techniques on chest X-ray and CT scan identification of COVID-19, Comput. Math. Methods Med., 2021 (2021), 5528144. https://doi:10.1155/2021/5528144 doi: 10.1155/2021/5528144
![]() |
[8] |
M. Nakhaeizadeh, M. Chegeni, M. Adhami, H. Sharifi, M. A. Gohari, A. Iranpour, et al., Estimating the number of COVID-19 cases and impact of new COVID-19 variants and vaccination on the population in Kerman, Iran: A mathematical modeling study, Comput. Math. Methods Med., 2022 (2022), 6624471. https://doi:10.1155/2022/6624471 doi: 10.1155/2022/6624471
![]() |
[9] |
J. B. Ackman, B. Kovacina, B. W. Carter, C. C. Wu, A. Sharma, J. O. Shepard, et al., Sex difference in normal thymic appearance in adults 20–30 years of age, Radiology, 268 (2013), 245–253. https://doi:10.1148/radiol.13121104 doi: 10.1148/radiol.13121104
![]() |
[10] |
M. Takesh, S. Adams, Imaging comparison between (18) F-FDG-PET/CT and (18) F-Flouroethyl choline PET/CT in rare case of Thymus Carcinoma exhibiting a positive choline uptake, Case Rep. Oncol. Med., 2013 (2013), 464396. https://doi:10.1155/2013/464396 doi: 10.1155/2013/464396
![]() |
[11] |
N. Simanovsky, N. Hiller, N. Loubashevsky, K. Rozovsky, Normal CT characteristics of the thymus in adults, Eur. J. Radiol., 81 (2012), 3581–3586. https://doi:10.1016/j.ejrad.2011.12.015 doi: 10.1016/j.ejrad.2011.12.015
![]() |
[12] |
T. Araki, M. Nishino, W. Gao, J. Dupuis, G. M. Hunninghake, T. Murakami, et al., Normal thymus in adults: appearance on CT and associations with age, sex, BMI and smoking, Eur. Radiol., 26 (2016), 15–24. https://doi:10.1007/s00330-015-3796-y doi: 10.1007/s00330-015-3796-y
![]() |
[13] |
H. Zhou, R. Xu, H. Mei, L. Zhang, Q. Yu, R. Liu, et al., Application of enhanced T1WI of MRI Radiomics in Glioma grading, Int. J. Clin. Pract., 2022 (2022), 3252574. https://doi:10.1155/2022/3252574 doi: 10.1155/2022/3252574
![]() |
[14] |
J. Wang, J. Zeng, H. Li, X. Yu, A deep learning radiomics analysis for survival prediction in Esophageal cancer, J. Healthcare Eng., 2022 (2022), 4034404. https://doi:10.1155/2022/4034404 doi: 10.1155/2022/4034404
![]() |
[15] |
Y. Wang, G. Feng, J. Wang, P. An, P. Duan, Y. Hu, et al., Contrast-enhanced ultrasound-magnetic resonance imaging radiomics based model for predicting the biochemical recurrence of prostate cancer: A feasibility study, Comput. Math. Methods Med., 2022 (2022), 8090529. https://doi:10.1155/2022/8090529 doi: 10.1155/2022/8090529
![]() |
[16] |
I. Malinauskaite, J. Hofmeister, S. Burgermeister, A. Neroladaki, M. Hamard, X. Montet, et al., Radiomics and machine learning differentiate soft-tissue lipoma and liposarcoma better than musculoskeletal radiologists, Sarcoma, 2020 (2022), 7163453. https://doi:10.1155/2020/7163453 doi: 10.1155/2020/7163453
![]() |
[17] |
P. An, J. Zhang, Y. Li, P. Duan, Y. Hu, X. Li, et al., Clinical and imaging data-based model for predicting Reversible Posterior Leukoencephalopathy Syndrome (RPLS) in pregnant women with severe preeclampsia or eclampsia and analysis of perinatal outcomes, Int. J. Clin. Pract., 2022 (2022), 6990974. https://doi:10.1155/2022/6990974 doi: 10.1155/2022/6990974
![]() |
[18] |
P. An, J. Zhang, F. Yang, Z. Wang, Y. Hu, X. Li, USMRI features and clinical data-based model for predicting the degree of placenta accreta spectrum disorders and developing prediction models, Int. J. Clin. Pract., 2022 (2022), 9527412. https://doi:10.1155/2022/9527412 doi: 10.1155/2022/9527412
![]() |
[19] |
P. An, W. Gu, S. Luo, M. Zhang, Y. Wang, Q. X. Li, Radiological changes on chest CT following COVID-19 infection, Ann. Acad. Med. Singapore, 50 (2021), 346–348. https://doi:10.47102/annals-acadmedsg.2020208 doi: 10.47102/annals-acadmedsg.2020208
![]() |
[20] |
P. An, P. Song, Y. Wang, B. Liu, Asymptomatic patients with novel coronavirus disease (COVID-19), Balkan Med. J., 37 (2020), 229–230. https://doi:10.4274/balkanmedj.galenos.2020.2020.4.20 doi: 10.4274/balkanmedj.galenos.2020.2020.4.20
![]() |
[21] |
P. An, P. Song, K. Lian, Y. Wang, CT manifestations of novel coronavirus pneumonia: A case report, Balkan Med. J., 37 (2020), 163–165. https://doi:10.4274/balkanmedj.galenos.2020.2020.2.15 doi: 10.4274/balkanmedj.galenos.2020.2020.2.15
![]() |
[22] |
P. An, B. J. Wood, W. Li, M. Zhang, Y. Ye, Postpartum exacerbation of antenatal COVID-19 pneumonia in 3 women, CMAJ, 192 (2020), E603–E606. https://doi:10.1503/cmaj.200553 doi: 10.1503/cmaj.200553
![]() |
[23] |
P. An, Y. Ye, M. Chen, Y. Chen, W. Fan, Y. Wang, Management strategy of novel coronavirus (COVID-19) pneumonia in the radiology department: a Chinese experience, Diagn. Interv. Radiol., 26 (2020), 200–203. https://doi:10.5152/dir.2020.20167 doi: 10.5152/dir.2020.20167
![]() |
[24] |
C. Kellogg, O. Equils, The role of the thymus in COVID-19 disease severity: implications for antibody treatment and immunization, Hum. Vaccines Immunother., 17 (2021), 638–643. https://doi:10.1080/21645515.2020.1818519 doi: 10.1080/21645515.2020.1818519
![]() |
[25] |
P. Cuvelier, H. Roux, A. Couëdel-Courteille, J. Dutrieux, C. Naudin, B. C. de Muylder, et al., Protective reactive thymus hyperplasia in COVID-19 acute respiratory distress syndrome, Crit. Care., 25 (2021), 4. https://doi:10.1186/s13054-020-03440-1 doi: 10.1186/s13054-020-03440-1
![]() |
[26] |
R. Thomas, W. Wang, D. M. Su, Contributions of age-related thymic involution to immunosenescence and inflammaging, Immun. Ageing, 17 (2020), 2. https://doi:10.1186/s12979-020-0173-8 doi: 10.1186/s12979-020-0173-8
![]() |
[27] |
W. Wang, R. Thomas, J. Oh, D. M. Su, Thymic aging may be associated with COVID-19 pathophysiology in the elderly, Cells, 10 (2021), 628. https://doi:10.3390/cells10030628 doi: 10.3390/cells10030628
![]() |
[28] |
S. Rehman, T. Majeed, M. A. Ansari, U. Ali, H. Sabit, E. A. Al-Suhaimi, Current scenario of COVID-19 in pediatric age group and physiology of immune and thymus response, Saudi J. Biol. Sci., 27 (2020), 2567–2573. https://doi:10.1016/j.sjbs.2020.05.024 doi: 10.1016/j.sjbs.2020.05.024
![]() |
[29] |
K. A. Harrington, D. S. Kennedy, B. Tang, C. Hickie, E. Phelan, W. Torreggiani, et al., Computed tomographic evaluation of the thymus-does obesity affect thymic fatty involution in a healthy young adult population, Br. J. Radiol., 91 (2018), 20170609. https://doi:10.1259/bjr.20170609 doi: 10.1259/bjr.20170609
![]() |
[30] |
F. Nasseri, F. Eftekhari, Clinical and radiologic review of the normal and abnormal thymus: pearls and pitfalls, Radiographics, 30 (2010), 413–428. https://doi:10.1148/rg.302095131 doi: 10.1148/rg.302095131
![]() |
[31] |
J. L. Zhang, Y. H. Li, L. L. Wang, H. Q. Liu, S. Y. Lu, Y. Liu, et al., Azvudine is a thymus-homing anti-SARS-CoV-2 drug effective in treating COVID-19 patients, Signal Transduction Targeted Ther., 6 (2021), 414. https://doi:10.1038/s41392-021-00835-6 doi: 10.1038/s41392-021-00835-6
![]() |
[32] |
M. E. Mayerhoefer, A. Materka, G. Langs, I. Häggström, P. Szczypiński, P. Gibbs, et al., Introduction to radiomics, J. Nucl. Med., 61 (2020), 488–495. https://doi:10.2967/jnumed.118.222893 doi: 10.2967/jnumed.118.222893
![]() |
[33] |
M. R. Chetan, F. V. Gleeson, Radiomics in predicting treatment response in non-small-cell lung cancer: current status, challenges and future perspectives, Eur. Radiol., 31 (2021), 1049–1058. https://doi:10.1007/s00330-020-07141-9 doi: 10.1007/s00330-020-07141-9
![]() |
[34] |
M. Seyit, E. Avci, A. Yilmaz, H. Senol, M. Ozen, A. Oskay, Predictive values of coagulation parameters to monitor COVID-19 patients, Int. J. Clin. Pract., 2022 (2022), 8436248. https://doi:10.1155/2022/8436248 doi: 10.1155/2022/8436248
![]() |
[35] |
I. Tsougos, A. Vamvakas, C. Kappas, I. Fezoulidis, K. Vassiou, Application of radiomics and decision support systems for breast mr differential diagnosis, Comput. Math. Methods Med., 2018 (2018), 7417126. https://doi:10.1155/2018/7417126 doi: 10.1155/2018/7417126
![]() |
[36] |
M. Umesh Pai, A. A. Ardakani, A. Kamath, U. Raghavendra, A. Gudigar, N. Venkatesh, et al., Novel radiomics features for automated detection of cardiac abnormality in patients with pacemaker, Comput. Math. Methods Med., 2022 (2022), 1279749. https://doi:10.1155/2022/1279749 doi: 10.1155/2022/1279749
![]() |
[37] |
S. A. Harmon, T. H. Sanford, S. Xu, E. B. Turkbey, H. Roth, Z. Xu, et al., Artificial intelligence for the detection of COVID-19 pneumonia on chest CT using multinational datasets, Nat. Commun., 11 (2020), 4080. https://doi:10.1038/s41467-020-17971-2 doi: 10.1038/s41467-020-17971-2
![]() |
[38] |
S. Cournane, R. Conway, D. Byrne, D. O'Riordan, B. Silke, Predicting outcomes in emergency medical admissions using a laboratory only nomogram, Comput. Math. Methods Med., 2017 (2017), 5267864. https://doi:10.1155/2017/5267864 doi: 10.1155/2017/5267864
![]() |
[39] |
S. Tian, Y. Guo, J. Fu, Z. Li, J. Li, X. Tian, Prognostic value of immunotyping combined with targeted therapy in patients with non-small-cell lung cancer and establishment of nomogram model, Comput. Math. Methods Med., 2022 (2022), 3049619. https://doi:10.1155/2022/3049619 doi: 10.1155/2022/3049619
![]() |
[40] |
R. Qin, H. Zhang, L. Jiang, K. Qiao, J. Hai, J. Chen, et al., Multicenter computer-aided diagnosis for lymph nodes using unsupervised domain-adaptation networks based on cross-domain confounding representations, Comput. Math. Methods Med., 2020 (2020), 3709873. https://doi:10.1155/2020/3709873 doi: 10.1155/2020/3709873
![]() |
[41] |
M. Brambilla, R. Matheoud, C. Basile, C. Bracco, I. Castiglioni, C. Cavedon, et al., An adaptive thresholding method for BTV estimation incorporating PET reconstruction parameters: A multicenter study of the robustness and the reliability, Comput. Math. Methods Med., 2015 (2015), 571473. https://doi:10.1155/2015/571473 doi: 10.1155/2015/571473
![]() |
1. | Zhishan Zheng, Lin Zhou, Han Wu, Lihong Zhou, Construction cost prediction system based on Random Forest optimized by the Bird Swarm Algorithm, 2023, 20, 1551-0018, 15044, 10.3934/mbe.2023674 | |
2. | Hélène M. Roux, Amira Marouf, Jacques Dutrieux, Bénédicte Charmeteau-De Muylder, Suzanne Figueiredo-Morgado, Véronique Avettand-Fenoel, Pelagia Cuvelier, Cécile Naudin, Fatma Bouaziz, Guillaume Geri, Anne Couëdel-Courteille, Pierre Squara, Stefano Marullo, Rémi Cheynier, Genetically determined thymic function affects strength and duration of immune response in COVID patients with pneumonia, 2023, 9, 2375-2548, 10.1126/sciadv.adh7969 |
Clinical data model | Univariate analysis | Multivariate analysis | ||
Factor | P | Hazard ratio | P | Hazard ratio |
Gender | 0.768 | 1.084 (0.634–1.851) | ||
BMI | 0.001* | 1.167 (1.065–1.278) | 0.010* | 1.137 (1.031–1.254) |
Smoking history | 0.747 | 0.916 (0.537–1.560) | ||
Drinking history | 0.443 | 0.811 (0.475–1.381) | ||
Age | 0.225 | 1.035 (0.979–1.090) | ||
cough | 0.106 | 1.566 (0.909–2.698) | ||
diarrhea | 0.002* | 2.451 (1.378–4.358) | 0.008* | 2.358 (1.246–4.463) |
Chest CT findings | 0.002* | 2.402 (1.386–4.163) | 0.009* | 2.217 (1.221–4.029) |
fatigue and myalgia | 0.045* | 0.566 (0.325–0.986) | ||
anorexia | 0.001* | 2.821 (1.609–4.946) | 0.029* | 1.990 (1.075–3.684) |
headache | 0.019* | 0.517 (0.298–0.898) | ||
limb twitch | 0.029* | 0.541 (0.312–0.938) | ||
Social medical insurance | 0.620 | 1.016 (0.953–1.080) | ||
general education | 0.078 | 0.606 (0.348–1.060) |
Laboratory examination model | Univariate analysis | Multivariate analysis | ||
Factor | P | Hazard ratio | P | Hazard ratio |
red blood cell | 0.580 | 1.147 (0.706–1.860) | ||
hemoglobin | 0.132 | 0.990 (0.977–1.001) | ||
white blood cell | 0.001* | 1.242 (1.088–1.418) | 0.001* | 1.271 (1.098–1.471) |
platelet | 0.002* | 0.995 (0.991–0.998) | 0.006* | 0.994 (0.991–1.001) |
ESR | 0.639 | 1.015 (0.953–1.080) | ||
D-Dimer | 0.002* | 1.401 (1.135–1.728) | 0.003* | 1.407 (1.126–1.759) |
LDH | 0.002* | 1.002 (1.001–1.004) | 0.007* | 1.002 (1.001–1.004) |
AST | 0.004* | 1.012 (1.004–1.020) | ||
ALT | 0.549 | 1.003 (0.993–1.010) | ||
uric acid | 0.260 | 1.001 (0.998–1.001) | ||
TC | 0.342 | 0.919 (0.773–1.090) |
Thymus CT feature model | Univariate analysis | Multivariate analysis | ||
Factor | p | Hazard ratio | p | Hazard ratio |
average CT value | 0.001* | 0.784 (0.675–0.911) | 0.002* | 0.743 (0.615–0.897) |
transverse diameter | 0.001* | 0.888 (0.837–0.942) | 0.011* | 0.908 (0.843–0.979) |
axial diameter | 0.392 | 0.874 (0.641–1.190) | ||
Anteroposterior diameter | 0.251 | 1.447 (0.770–2.720) | ||
thymus steatosis | 0.003* | 1.074 (1.025–1.125) | 0.014* | 1.076 (1.015–1.140) |
Lung CT value | 0.368 | 0.594 (0.192–1.840) | ||
Liver CT value | 0.878 | 0.995 (0.933–1.060) | ||
Radscore | 0.001* | 2.356 (1.857–2.989) | 0.001* | 2.345 (1.816–3.028) |
notes: Radscore = -0.20463620+0.08033656*Maximum2DDiameterRow-0.24104915*SurfaceVolumeRatio+0.03263107*Idn...46-0.09277765*DependenceVariance...61-0.05773797*SmallAreaLowGrayLevelEmphasis...100+0.12575685*Maximum...115+0.02108543*ClusterShade...129-0.36639420*Median...211+0.08557059*Idn...232+0.22752327*MCC...239+0.11411019*RunEntropy...359+0.23346851*ClusterShade...408+0.29022507*InverseVariance...421+0.05698528*InverseVariance...514+0.47092109*RunVariance...735-0.03106958*Kurtosis...765 |
Combined model | Univariate analysis | Multivariate analysis | ||
Factors | p | Hazard ratio | p | Hazard ratio |
BMI | 0.001* | 1.167 (1.065–1.278) | ||
diarrhea | 0.002* | 2.451 (1.378–4.358) | ||
Chest CT findings | 0.002* | 2.402 (1.386–4.163) | 0.015* | 3.586 (1.285–10.007) |
fatigue and myalgia | 0.045* | 0.566 (0.325–0.986) | ||
anorexia | 0.001* | 2.821 (1.609–4.946) | 0.025* | 3.450 (1.164–10.226) |
headache | 0.019* | 0.517 (0.298–0.898) | ||
limb twitch | 0.029* | 0.541 (0.312–0.938) | ||
white blood cell | 0.001* | 1.242 (1.088–1.418) | 0.001* | 1.608 (1.226–2.109) |
platelet | 0.002* | 0.995 (0.991–0.998) | 0.002* | 0.989 (0.983–0.997) |
D-Dimer | 0.002* | 1.401 (1.135–1.728) | 0.001* | 1.866 (1.315–2.649) |
LDH | 0.002* | 1.002 (1.001–1.004) | 0.001* | 1.005 (1.002–1.008) |
AST | 0.004* | 1.012 (1.004–1.020) | ||
average CT value | 0.001* | 0.784 (0.675–0.911) | 0.001* | 0.544 (0.402–0.737) |
transverse diameter | 0.001* | 0.888 (0.837–0.942) | 0.002* | 0.830 (0.740–0.931) |
thymus steatosis | 0.003* | 1.074 (1.025–1.125) | ||
Radscore | 0.001* | 2.356 (1.857–2.989) | 0.001* | 2.748 (1.863–4.052) |
Clinical data model | Univariate analysis | Multivariate analysis | ||
Factor | P | Hazard ratio | P | Hazard ratio |
Gender | 0.768 | 1.084 (0.634–1.851) | ||
BMI | 0.001* | 1.167 (1.065–1.278) | 0.010* | 1.137 (1.031–1.254) |
Smoking history | 0.747 | 0.916 (0.537–1.560) | ||
Drinking history | 0.443 | 0.811 (0.475–1.381) | ||
Age | 0.225 | 1.035 (0.979–1.090) | ||
cough | 0.106 | 1.566 (0.909–2.698) | ||
diarrhea | 0.002* | 2.451 (1.378–4.358) | 0.008* | 2.358 (1.246–4.463) |
Chest CT findings | 0.002* | 2.402 (1.386–4.163) | 0.009* | 2.217 (1.221–4.029) |
fatigue and myalgia | 0.045* | 0.566 (0.325–0.986) | ||
anorexia | 0.001* | 2.821 (1.609–4.946) | 0.029* | 1.990 (1.075–3.684) |
headache | 0.019* | 0.517 (0.298–0.898) | ||
limb twitch | 0.029* | 0.541 (0.312–0.938) | ||
Social medical insurance | 0.620 | 1.016 (0.953–1.080) | ||
general education | 0.078 | 0.606 (0.348–1.060) |
Laboratory examination model | Univariate analysis | Multivariate analysis | ||
Factor | P | Hazard ratio | P | Hazard ratio |
red blood cell | 0.580 | 1.147 (0.706–1.860) | ||
hemoglobin | 0.132 | 0.990 (0.977–1.001) | ||
white blood cell | 0.001* | 1.242 (1.088–1.418) | 0.001* | 1.271 (1.098–1.471) |
platelet | 0.002* | 0.995 (0.991–0.998) | 0.006* | 0.994 (0.991–1.001) |
ESR | 0.639 | 1.015 (0.953–1.080) | ||
D-Dimer | 0.002* | 1.401 (1.135–1.728) | 0.003* | 1.407 (1.126–1.759) |
LDH | 0.002* | 1.002 (1.001–1.004) | 0.007* | 1.002 (1.001–1.004) |
AST | 0.004* | 1.012 (1.004–1.020) | ||
ALT | 0.549 | 1.003 (0.993–1.010) | ||
uric acid | 0.260 | 1.001 (0.998–1.001) | ||
TC | 0.342 | 0.919 (0.773–1.090) |
Thymus CT feature model | Univariate analysis | Multivariate analysis | ||
Factor | p | Hazard ratio | p | Hazard ratio |
average CT value | 0.001* | 0.784 (0.675–0.911) | 0.002* | 0.743 (0.615–0.897) |
transverse diameter | 0.001* | 0.888 (0.837–0.942) | 0.011* | 0.908 (0.843–0.979) |
axial diameter | 0.392 | 0.874 (0.641–1.190) | ||
Anteroposterior diameter | 0.251 | 1.447 (0.770–2.720) | ||
thymus steatosis | 0.003* | 1.074 (1.025–1.125) | 0.014* | 1.076 (1.015–1.140) |
Lung CT value | 0.368 | 0.594 (0.192–1.840) | ||
Liver CT value | 0.878 | 0.995 (0.933–1.060) | ||
Radscore | 0.001* | 2.356 (1.857–2.989) | 0.001* | 2.345 (1.816–3.028) |
notes: Radscore = -0.20463620+0.08033656*Maximum2DDiameterRow-0.24104915*SurfaceVolumeRatio+0.03263107*Idn...46-0.09277765*DependenceVariance...61-0.05773797*SmallAreaLowGrayLevelEmphasis...100+0.12575685*Maximum...115+0.02108543*ClusterShade...129-0.36639420*Median...211+0.08557059*Idn...232+0.22752327*MCC...239+0.11411019*RunEntropy...359+0.23346851*ClusterShade...408+0.29022507*InverseVariance...421+0.05698528*InverseVariance...514+0.47092109*RunVariance...735-0.03106958*Kurtosis...765 |
Combined model | Univariate analysis | Multivariate analysis | ||
Factors | p | Hazard ratio | p | Hazard ratio |
BMI | 0.001* | 1.167 (1.065–1.278) | ||
diarrhea | 0.002* | 2.451 (1.378–4.358) | ||
Chest CT findings | 0.002* | 2.402 (1.386–4.163) | 0.015* | 3.586 (1.285–10.007) |
fatigue and myalgia | 0.045* | 0.566 (0.325–0.986) | ||
anorexia | 0.001* | 2.821 (1.609–4.946) | 0.025* | 3.450 (1.164–10.226) |
headache | 0.019* | 0.517 (0.298–0.898) | ||
limb twitch | 0.029* | 0.541 (0.312–0.938) | ||
white blood cell | 0.001* | 1.242 (1.088–1.418) | 0.001* | 1.608 (1.226–2.109) |
platelet | 0.002* | 0.995 (0.991–0.998) | 0.002* | 0.989 (0.983–0.997) |
D-Dimer | 0.002* | 1.401 (1.135–1.728) | 0.001* | 1.866 (1.315–2.649) |
LDH | 0.002* | 1.002 (1.001–1.004) | 0.001* | 1.005 (1.002–1.008) |
AST | 0.004* | 1.012 (1.004–1.020) | ||
average CT value | 0.001* | 0.784 (0.675–0.911) | 0.001* | 0.544 (0.402–0.737) |
transverse diameter | 0.001* | 0.888 (0.837–0.942) | 0.002* | 0.830 (0.740–0.931) |
thymus steatosis | 0.003* | 1.074 (1.025–1.125) | ||
Radscore | 0.001* | 2.356 (1.857–2.989) | 0.001* | 2.748 (1.863–4.052) |