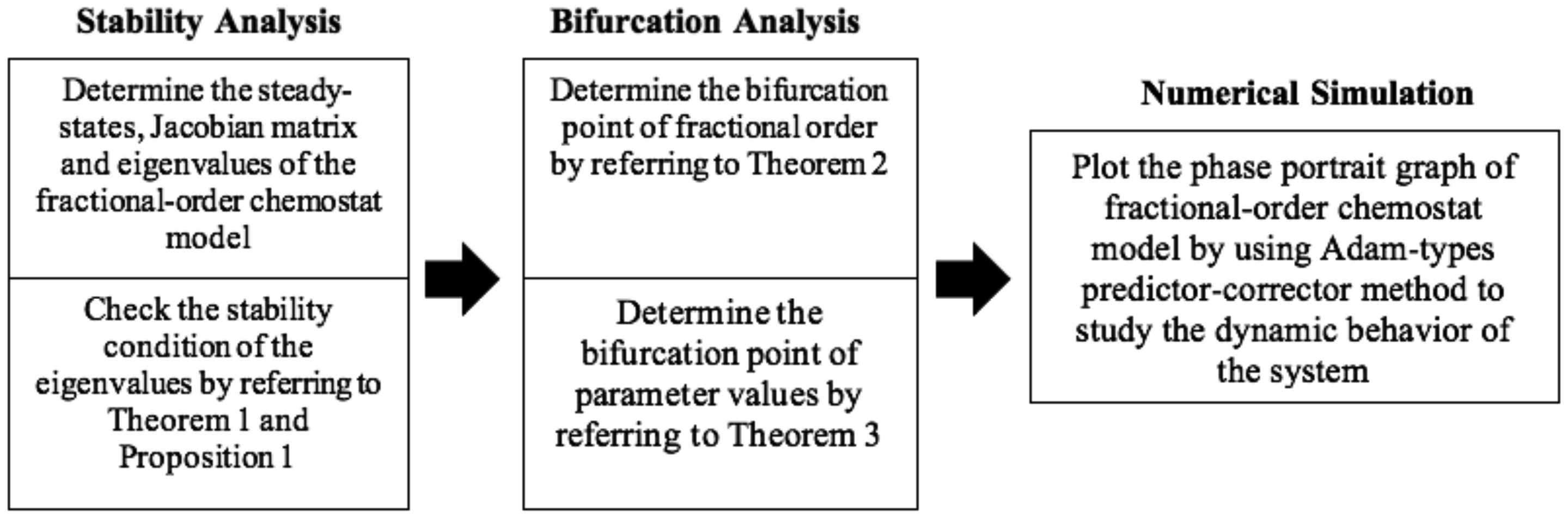
This article investigates the due-window assignment scheduling problem with setup times on a single machine, where setup times of jobs are past-sequence-dependent. Under common, slack and unrestricted due-window assignment methods, the goal is to determine the optimal job sequence and due-window such that the cost function (i.e., the weighted sum of earliness and tardiness, number of early and tardy jobs, due-window starting time and size) is minimized. We solve the problem optimally by introducing a polynomial time algorithm. An extension to the problem with learning and deterioration effects is also studied.
Citation: Weiguo Liu, Xuyin Wang, Xiaoxiao Wang, Peizhen Zhao. Due-window assignment scheduling with past-sequence-dependent setup times[J]. Mathematical Biosciences and Engineering, 2022, 19(3): 3110-3126. doi: 10.3934/mbe.2022144
[1] | Shaimaa A. M. Abdelmohsen, D. Sh. Mohamed, Haifa A. Alyousef, M. R. Gorji, Amr M. S. Mahdy . Mathematical modeling for solving fractional model cancer bosom malignant growth. AIMS Biophysics, 2023, 10(3): 263-280. doi: 10.3934/biophy.2023018 |
[2] | Mati ur Rahman, Mehmet Yavuz, Muhammad Arfan, Adnan Sami . Theoretical and numerical investigation of a modified ABC fractional operator for the spread of polio under the effect of vaccination. AIMS Biophysics, 2024, 11(1): 97-120. doi: 10.3934/biophy.2024007 |
[3] | Yasir Nadeem Anjam, Mehmet Yavuz, Mati ur Rahman, Amna Batool . Analysis of a fractional pollution model in a system of three interconnecting lakes. AIMS Biophysics, 2023, 10(2): 220-240. doi: 10.3934/biophy.2023014 |
[4] | Marco Menale, Bruno Carbonaro . The mathematical analysis towards the dependence on the initial data for a discrete thermostatted kinetic framework for biological systems composed of interacting entities. AIMS Biophysics, 2020, 7(3): 204-218. doi: 10.3934/biophy.2020016 |
[5] | Mehmet Yavuz, Kübra Akyüz, Naime Büşra Bayraktar, Feyza Nur Özdemir . Hepatitis-B disease modelling of fractional order and parameter calibration using real data from the USA. AIMS Biophysics, 2024, 11(3): 378-402. doi: 10.3934/biophy.2024021 |
[6] | Larisa A. Krasnobaeva, Ludmila V. Yakushevich . On the dimensionless model of the transcription bubble dynamics. AIMS Biophysics, 2023, 10(2): 205-219. doi: 10.3934/biophy.2023013 |
[7] | Carlo Bianca . Differential equations frameworks and models for the physics of biological systems. AIMS Biophysics, 2024, 11(2): 234-238. doi: 10.3934/biophy.2024013 |
[8] | Mohammed Alabedalhadi, Mohammed Shqair, Ibrahim Saleh . Analysis and analytical simulation for a biophysical fractional diffusive cancer model with virotherapy using the Caputo operator. AIMS Biophysics, 2023, 10(4): 503-522. doi: 10.3934/biophy.2023028 |
[9] | Bertrand R. Caré, Pierre-Emmanuel Emeriau, Ruggero Cortini, Jean-Marc Victor . Chromatin epigenomic domain folding: size matters. AIMS Biophysics, 2015, 2(4): 517-530. doi: 10.3934/biophy.2015.4.517 |
[10] | David Gosselin, Maxime Huet, Myriam Cubizolles, David Rabaud, Naceur Belgacem, Didier Chaussy, Jean Berthier . Viscoelastic capillary flow: the case of whole blood. AIMS Biophysics, 2016, 3(3): 340-357. doi: 10.3934/biophy.2016.3.340 |
This article investigates the due-window assignment scheduling problem with setup times on a single machine, where setup times of jobs are past-sequence-dependent. Under common, slack and unrestricted due-window assignment methods, the goal is to determine the optimal job sequence and due-window such that the cost function (i.e., the weighted sum of earliness and tardiness, number of early and tardy jobs, due-window starting time and size) is minimized. We solve the problem optimally by introducing a polynomial time algorithm. An extension to the problem with learning and deterioration effects is also studied.
Numerous mathematical models have been established to predict and study the biological system. In the past four decades, there have been far-reaching research on improving cell mass production in chemical reactors [1]. The chemostat model is used to understand the mechanism of cell mass growth in a chemostat. A chemostat is an apparatus for continuous culture that contains bacterial populations. It can be used to investigate the cell mass production under controlled conditions. This reactor provides a dynamic system for population studies and is suitable to be used in a laboratory. A substrate is continuously added into the reactor containing the cell mass, which grows by consuming the substrate that enters through the inflow chamber. Meanwhile, the mixture of cell mass and substrate is continuously harvested from the reactor through the outflow chamber. The dynamics in the chemostat can be investigated by using the chemostat model [2].
Ordinary differential equations (ODEs) are commonly used for modelling biological systems. However, most biological systems behaviour has memory effects, and ODEs usually neglect such effects. The fractional-order differential equations (FDEs) are taken into account when describing the behaviour of the systems' equations. A FDEs is a generalisation of the ODEs to random nonlinear order [3]. This equation is more effective because of its good memory, among other advantages [4]–[8]. The errors occuring from the disregarded parameters when modelling of phenomena in real-life also can be reduced. FDEs are also used to efficiently replicate the real nature of various systems in the field of engineering and sciences [9]. In the past few decades, FDEs have been used in biological systems for various studies [5], [6], [10]–[17].
Since great strides in the study of FDEs have been developed, the dynamics in the chemostat can be investigated using the mathematical model of the chemostat in the form of FDEs. Moreover, there have been few studies on the expansion of the chemostat model with fractional-order theory. Thus, we deepen and complete the analysis on the integer-order chemostat model with fractional-order theory and discuss the stability of the equilibrium points of the fractional-order chemostat model. Next, the bifurcation analysis for the fractional-order chemostat model is conducted to identify the bifurcation point that can change the stability of the system. The analysis identifies the values of the fractional-order and the system parameters to ensure the operation of the chemostat is well-controlled.
Recently, there are many approaches to define fractional operators such as by Caputo, Riemann-Liouville, Hadamard, and Grunwald-Letnikov [3]. However, Caputo is often used due to its convenience in various applications [18]. Caputo is also useful to encounter an obstacle where the initial condition is done in the differential of integer-order [19]. In this paper, we applied Caputo derivative to define the system of fractional-order. The Caputo derivative for the left-hand side is defined as
where Г denotes the function of gamma, n is an integer, where
The Adams-type predictor-corrector method is one of the technique that have been proposed for fractional-order differential equations [19]–[21]. The Adams-type predictor-corrector method is a analysis of numerical algorithm that involves two basics steps: predictor and corrector. The predictor formula can be described as
meanwhile the corrector formula can be determined by
The predictor-corrector method is also called as the PECE (Predict, Evaluate, Correct, Evaluate) method [22]. The procedure of the predictor-corrector method can be explained as follows
(i) Calculate the predictor step,
(ii) Evaluate
(iii) Calculate the corrector step,
(iv) Evaluate
The procedure repeatedly predicts and corrects the value until the corrected value becomes a converged number [21]. This method is able to maintain the stability of the properties and has good accuracy. Moreover, this method also has lower computational cost than other methods [23]. The algorithm of the Adams-type predictor-corrector method proposed by [22] is shown in Appendix.
The conditions of stability for integer-order differential equations and fractional-order differential equations are different. Both systems could have the same steady-state points but different stability conditions [24], [25]. The stability condition for fractional-order differential equations can be stated by Theorem 1 and the Routh-Hurwitz stability condition as described by Proposition 1.
Theorem 1
Proposition 1
or
The stability theorem on the fractional-order systems and fractional Routh-Hurwitz stability conditions are introduced to analyze the stability of the model. The fractional Routh-Hurwitz stability conditions is specifically introduced for the eigenvalues of the Jacobian matrix that obtained in quadratic form. The proof of this proposition is shown in Appendix.
Bifurcation can be defined as any sudden change that occurs while a parameter value is varied in the differential equation system and it has a significant influence on the solution [27]. An unstable steady-state may becomes stable and vice versa. A slight changes in the parameter value may change the system's stability. Despite the steady-state point and the eigenvalues of the system of fractional-order are similar as the system of integer-order, the discriminant method used for the stability of the steady-state point is different. Accordingly, the Hopf bifurcation condition in the fractional-order system is slightly different as compared with the integer-order system.
Fractional order α can be selected as the bifurcation parameter in a fractional-order system, but this is not allowed in an integer-order system. The existence of Hopf bifurcation can be stated as in Theorem 2.
Theorem 2
(i) 1. The characteristic equation of chemostat system has a pair of complex conjugate roots,
(ii) Critical value
(iii)
Proof. Condition (i) is not easy to obtain due to the selected parameter's value. However, this condition can be managed under some confined conditions. In fact, the washout steady-state solution of the chemostat model has two negative real roots. The remaining two roots depend on the characteristic of the polynomial from the no-washout steady-state solution.
Condition (ii) can be satisfied with the existence of critical value α* and when
The integer system required p = 0 for the bifurcation's operating condition. For the fractional-order system, the operating condition of the system will change into
In studying the dynamic process of chemostat, the parameters such as
Theorem 3
(i) The characteristic equation of chemostat system has a pair of complex conjugate roots,
(ii) Critical value
(iii)
Proof. This theorem can be proved in the same way as Theorem 2. Therefore, condition (i) can be guaranteed. Condition (ii) can be satisfied with the existence of critical value
For condition (iii), the condition of
Firstly, determine the steady-states, Jacobian matrix and eigenvalues of the fractional-order chemostat model. The stability properties of the fractional-order chemostat model were estimated by using the stability and bifurcation analyses with FDEs by referring to Theorem 1 and Proposition 1. Then, determine the bifurcation point of fractional-order by referring to Theorem 2 and determine the bifurcation point of parameter values by referring to Theorem 3. Next, plot the phase portrait of fractional-order chemostat model by using Adam-types predictor-corrector method to study the dynamic behaviour of the system. Figure 1 depicts the flowchart of this research. This flowchart can be applied to all problems with suitable parameter values.
An integer-order chemostat model that considered a variable yield coefficient and the Monod growth model from [1] is studied in this section. The chemostat system can be written as
with the initial value of
Let Eq. (3.2) equal to zero in order to find the steady-state solutions
By solving Eq. (3.4), the following solutions are obtained
From
Hence, the solutions of steady-state for the chemostat model are
(i) Washout:
(ii) No Washout:
where
The steady-state solutions are physically meaningful if their components are positive. Therefore,
The solution of steady-state in Equation (3.8) represents the washout situation, where the cell mass is wholly removed from the reactor and where the substrate concentration is at the same stock as in the beginning. This state must always be unstable in order to ensure that the cell mass is able to grow in the chemostat. This is because the cell mass will be continuously removed from the chemostat if the washout steady-state is stable. The Jacobian matrix for the washout steady-state solution can be written as
The eigenvalues of this matrix are
The eigenvalues in
The eigenvalues of the Jacobian matrix in terms of the characteristic polynomial are
where
and
The eigenvalues of the no-washout steady-state solution were evaluated with Routh-Hurwitz condition in Proposition 1. Based on the eigenvalues in Eq. (3.15) and by referring to the study by [5], the eigenvalues' condition can be simplified as the following two cases
(i) If b>0 or equivalent to
(ii) If b<0 or equivalent to
Then, if
The parameter values of the fractional-order chemostat model are provided in Table 1. The initial substrate concentration, S0 and ρ were assumed as non-negative values to ensure that the steady-state solutions were physically meaningful. The stability diagram of the steady-state solutions is plotted in Figure 2.
Parameters | Description | Values | Units |
k | Saturation constant | 1.75 | gl−1 |
Q | Dilution rate | 0.02 | l2gr−1 |
µ | Maximum growth rate | 0.3 | h−1 |
γ | Constant in yield coefficient | 0.01 | – |
β | Constant in yield coefficient | 5.25 | lg−1 |
S0 | Input concentration of substrate | 1 | gl−1 |
The washout steady-state solution is stable if Q > 0 and
The steady-state solutions of the fractional-order chemostat model for the parameter values given in Table 1 are
(i) Washout:
(ii) No Washout:
The eigenvalues obtained from the washout steady-state solution are
and the eigenvalues from the no-washout steady-state solution are
Based on Eq. (3.22) to Eq. (3.25), these satisfied the first condition of Hopf bifurcation in Theorem 2. There exists a pair of complex conjugate roots and the other eigenvalues are negative real roots. The transversality condition as the third condition is also satisfied. The eigenvalues of the washout steady-state solution based on the chemostat system is not imaginary, and so there is no existence of Hopf bifurcation in the washout steady-state solution. According to Theorem 2, the critical value of the fractional-order as stated in the second condition can be obtained as
where
Value of p and q are obtained from
The running state of the fractional-order chemostat system when fractional order α at the Hopf bifurcation point is shown. The fractional-order chemostat system changed its stability once Hopf bifurcation occurred. Therefore, we conjecture that the system of fractional-order chemostat may be lost or gain its stability when the fractional order α is less than the Hopf bifurcation point, or
The initial concentration of the substrate, S0, was chosen as the control parameter, while fractional order α was fixed. The solutions of steady-state of the fractional-order chemostat model with S0 as the control parameter are
(i) Washout:
(ii) No Washout:
The eigenvalues obtained from the washout steady-state solution are
and the eigenvalues from the no-washout steady-state solution are
These satisfied the first condition of Hopf bifurcation in Theorem 3. There exist a pair of complex conjugate roots in terms of S0, and the other eigenvalues were negative real roots in terms of S0. The transversality condition as the third condition is also satisfied. According to Theorem 3, the critical value of the fractional order as stated in the second condition can be obtained as follows
By referring to the study by [18], Eq. (3.37) can also be calculated as
From the calculations, the critical value of the initial concentration of the substrate is
The change in the running state when the value of the initial substrate concentration passes through the Hopf bifurcation point is shown. The stability of the fractional-order chemostat system changed once Hopf bifurcation occurred. In
The Hopf bifurcation points of system of fractional-order chemostat and system of integer-order chemostat are different.
The stability analysis of the fractional-order chemostat model was conducted based on the stability theory of FDEs. The integer-order chemostat model was extended to the FDEs. There are two steady-state solutions obtained, which are washout and no-washout steady-state solutions. The Hopf bifurcation of the order of α occured at the solutions of steady-state when the Hopf bifurcation conditions is fulfilled. The results show that the increasing or decreasing the value of α may stabilise the unstable state of the chemostat system. Therefore, the running state of the fractional-order chemostat system is affected by the value of α. The Hopf bifurcation of the initial concentration of the substrate, S0, also occurred when the Hopf bifurcation condition is fulfilled. As the evidence from the phase portrait plots, increase the value of the initial substrate concentration may destabilise the stable state of the chemostat system. The value of the initial substrate should remain at
[1] |
C. Koulamas, G. J. Kyparisis, Single-machine scheduling problems with past-sequence-dependent setup times, Eur. J. Oper. Res., 187 (2008), 1045–1049. https://doi.org/10.1016/j.ejor.2006.03.066 doi: 10.1016/j.ejor.2006.03.066
![]() |
[2] |
D. Biskup, J. Herrmann, Single-machine scheduling against due dates with past-sequence-dependent setup times, Eur. J. Oper. Res., 191 (2008), 587–592. https://doi.org/10.1016/j.ejor.2007.08.028 doi: 10.1016/j.ejor.2007.08.028
![]() |
[3] |
C. Koulamas, G. J. Kyparisis, New results for single-machine scheduling with past-sequence-dependent setup times and due date-related objectives, Eur. J. Oper. Res., 278 (2019), 149–159. https://doi.org/10.1016/j.ejor.2019.04.022 doi: 10.1016/j.ejor.2019.04.022
![]() |
[4] |
W. H. Kuo, D. L. Yang, Single machine scheduling with past-sequence-dependent setup times and learning effects, Inf. Process. Lett., 102 (2007), 22–26. https://doi.org/10.1016/j.ipl.2006.11.002 doi: 10.1016/j.ipl.2006.11.002
![]() |
[5] |
X. R. Wang, J. B. Wang, W. J. Gao, X. Huang, Scheduling with past-sequence-dependent setup times and learning effects on a single machine, Int. J. Adv. Manuf. Technol., 48 (2010), 739–746. https://doi.org/10.1007/s00170-009-2308-0 doi: 10.1007/s00170-009-2308-0
![]() |
[6] |
V. Mani, P. C. Chang, S. H. Chen, Single-machine scheduling with past-sequence-dependent setup times and learning effects: a parametric analysis, Int. J. Syst. Sci., 42 (2011), 2097–2102. https://doi.org/10.1080/00207721003718436 doi: 10.1080/00207721003718436
![]() |
[7] |
H. M. Soroush, Scheduling in bicriteria single machine systems with past-sequence-dependent setup times and learning effects, J. Oper. Res. Soc., 65 (2014), 1017–1036. https://doi.org/10.1057/jors.2013.43 doi: 10.1057/jors.2013.43
![]() |
[8] | L. Y. Wang, X. Huang, W. W. Liu, Y. B. Wu, J. B. Wang, Scheduling with position-dependent weights, due-date assignment and past-sequence-dependent setup times, RAIRO-Oper. Res., 55 (2021), 2747–2758. https://doi.org/10.1051/ro/2020117 |
[9] |
A. Allahverdi, The third comprehensive survey on scheduling problems with setup times/costs, Eur. J. Oper. Res., 246 (2015), 345–378. https://doi.org/10.1016/j.ejor.2015.04.004 doi: 10.1016/j.ejor.2015.04.004
![]() |
[10] |
J. Pei, X. Liu, P. M. Pardalos, W. Fan, S. Yang, Scheduling deteriorating jobs on a single serial-batching machine with multiple job types and sequence-dependent setup times, Ann. Oper. Res., 249 (2017), 175–195. https://doi.org/10.1007/s10479-015-1824-6 doi: 10.1007/s10479-015-1824-6
![]() |
[11] |
S. Muştu, T. Eren. The single machine scheduling problem with setup times under an extension of the general learning and forgetting effects, Optim. Lett., 15 (2020), 1327–1343. https://doi.org/10.1007/s11590-020-01641-9 doi: 10.1007/s11590-020-01641-9
![]() |
[12] |
M. D. Weerdt, R. Baart, L. He, Single-machine scheduling with release times, deadlines, setup times, and rejection, Eur. J. Oper. Res., 291 (2021), 629–639. https://doi.org/10.1016/j.ejor.2020.09.042 doi: 10.1016/j.ejor.2020.09.042
![]() |
[13] |
W. Wang, Single-machine due-date assignment scheduling with generalized earliness-tardiness penalties including proportional setup times, J. Appl. Math. Comput., 2021 (2021), 1–19. https://doi.org/10.1007/s12190-021-01555-4 doi: 10.1007/s12190-021-01555-4
![]() |
[14] |
A. Janiak, W. A. Janiak, T. Krysiak, T. Kwiatkowski, A survey on scheduling problems with due windows, Eur. J. Oper. Res., 242 (2015), 347–357. https://doi.org/10.1016/j.ejor.2014.09.043 doi: 10.1016/j.ejor.2014.09.043
![]() |
[15] |
G. A. Rolim, M. S. Nagano, Structural properties and algorithms for earliness and tardiness scheduling against common due dates and windows: A review, Comput. Ind. Eng., 149 (2020), 106803. https://doi.org/10.1016/j.cie.2020.106803 doi: 10.1016/j.cie.2020.106803
![]() |
[16] |
S. D. Liman, S. S. Panwalkar, S. Thongmee, Common due window size and location determination in a single machine scheduling problem, J. Oper. Res. Soc., 49 (1998), 1007–1010. https://doi.org/10.1057/palgrave.jors.2600601 doi: 10.1057/palgrave.jors.2600601
![]() |
[17] |
X. Huang, N. Yin, W. W. Liu, J. B. Wang, Common due window assignment scheduling with proportional linear deterioration effects, Asia-Pac. J. Oper. Res., 37 (2020), 1950031. https://doi.org/10.1142/S0217595919500313 doi: 10.1142/S0217595919500313
![]() |
[18] |
J. B. Wang, Y. Hu, B. Zhang, Common due-window assignment for single-machine scheduling with generalized earliness/tardiness penalties and a rate-modifying activity, Eng. Optim., 53 (2021), 496–512. https://doi.org/10.1080/0305215X.2020.1740921 doi: 10.1080/0305215X.2020.1740921
![]() |
[19] |
J. B. Wang, B. Zhang, L. Li, D. Bai, Y. B. Feng, Due window assignment scheduling problems with position-dependent weights on a single machine, Eng. Optim., 52 (2020), 185–193. https://doi.org/10.1080/0305215X.2019.1577411 doi: 10.1080/0305215X.2019.1577411
![]() |
[20] |
D. Wang, Y. Yin, T. C. E. Cheng, A bicriterion approach to common flow allowances due window assignment and scheduling with controllable processing times, Nav. Res. Logist., 64 (2017), 41–63. https://doi.org/10.1002/nav.21731 doi: 10.1002/nav.21731
![]() |
[21] |
Y. Yin, T. C. E. Cheng, C. C. Wu, S. R. Cheng, Single-machine due window assignment and scheduling with a common flow allowance and controllable job processing time, J. Oper. Res. Soc., 65 (2014), 1–13. https://doi.org/10.1057/jors.2012.161 doi: 10.1057/jors.2012.161
![]() |
[22] |
Y. Yin, D. Wang, T. C. E. Cheng, C. C. Wu, Bi-criterion single-machine scheduling and due-window assignment with common flow allowances and resource-dependent processing times, J. Oper. Res. Soc., 67 (2016), 1169–1183. https://doi.org/10.1057/jors.2016.14 doi: 10.1057/jors.2016.14
![]() |
[23] | G. H. Hardy, J. E. Littlewood, G. Polya, Inequalities, Cambridge University Press, 1988. https://doi.org/10.1017/s0025557200143451 |
[24] |
J. B. Wang, A note on scheduling problems with learning effect and deteriorating jobs, Int. J. Syst. Sci., 37 (2006), 827–833. https://doi.org/10.1080/00207720600879260 doi: 10.1080/00207720600879260
![]() |
[25] |
D. L. Yang, W. H. Kuo, Some scheduling problems with deteriorating jobs and learning effects, Comput. Ind. Eng., 58 (2010), 25–28. https://doi.org/10.1016/j.cie.2009.06.016 doi: 10.1016/j.cie.2009.06.016
![]() |
[26] | S. Gawiejnowicz, Models and algorithms of time-dependent scheduling, Berlin: Springer, 2020. https://doi.org/10.1007/978-3-662-59362-2 |
[27] |
A. Azzouz, M. Ennigrou, L. B. Said, Scheduling problems under learning effects: classification and cartography, Int. J. Prod. Res., 56 (2018), 1642–1661. https://doi.org/10.1080/00207543.2017.1355576 doi: 10.1080/00207543.2017.1355576
![]() |
[28] |
J. B. Wang, J. Xu, J. Yang, Bi-criterion optimization for flow shop with a learning effect subject to release dates, Complexity, 2018 (2018), 9149510. https://doi.org/10.1155/2018/9149510 doi: 10.1155/2018/9149510
![]() |
[29] |
B. Cheng, J. Duan, X. Zhu, M. Zhou, Optimizing batch operations with batch-position-dependent learning effect and aging effect, Comput. Ind. Eng., 157 (2021), 107325. https://doi.org/10.1016/j.cie.2021.107325 doi: 10.1016/j.cie.2021.107325
![]() |
[30] |
Y. Ma, C. Chu, C. Zuo, A survey of scheduling with deterministic machine availability constraints, Comput. Ind. Eng., 58 (2010), 199–211. https://doi.org/10.1016/j.cie.2009.04.014 doi: 10.1016/j.cie.2009.04.014
![]() |
[31] |
J. B. Wang, L. Li, Machine scheduling with deteriorating jobs and modifying maintenance activities, Comput. J., 61 (2018), 47–53. https://doi.org/10.1093/comjnl/bxx032 doi: 10.1093/comjnl/bxx032
![]() |
[32] |
J. B. Wang, Y. Hu, B. Zhang, Common due-window assignment for single-machine scheduling with generalized earliness/tardiness penalties and a rate-modifying activity, Eng. Optim., 53 (2021), 496–512. https://doi.org/10.1080/0305215X.2020.1740921 doi: 10.1080/0305215X.2020.1740921
![]() |
1. | Xiaomeng Ma, Zhanbing Bai, Sujing Sun, Stability and bifurcation control for a fractional-order chemostat model with time delays and incommensurate orders, 2022, 20, 1551-0018, 437, 10.3934/mbe.2023020 |
Parameters | Description | Values | Units |
k | Saturation constant | 1.75 | gl−1 |
Q | Dilution rate | 0.02 | l2gr−1 |
µ | Maximum growth rate | 0.3 | h−1 |
γ | Constant in yield coefficient | 0.01 | – |
β | Constant in yield coefficient | 5.25 | lg−1 |
S0 | Input concentration of substrate | 1 | gl−1 |