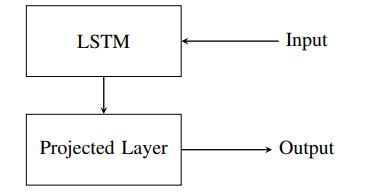
Citation: Budi Suarti, Sukarno, Ardiansyah, Slamet Budijanto. Bio-active compounds, their antioxidant activities, and the physicochemical and pasting properties of both pigmented and non-pigmented fermented de-husked rice flour[J]. AIMS Agriculture and Food, 2021, 6(1): 49-64. doi: 10.3934/agrfood.2021004
[1] | Miao Peng, Rui Lin, Zhengdi Zhang, Lei Huang . The dynamics of a delayed predator-prey model with square root functional response and stage structure. Electronic Research Archive, 2024, 32(5): 3275-3298. doi: 10.3934/era.2024150 |
[2] | Yanhe Qiao, Hui Cao, Guoming Xu . A double time-delay Holling Ⅱ predation model with weak Allee effect and age-structure. Electronic Research Archive, 2024, 32(3): 1749-1769. doi: 10.3934/era.2024080 |
[3] | Mengting Sui, Yanfei Du . Bifurcations, stability switches and chaos in a diffusive predator-prey model with fear response delay. Electronic Research Archive, 2023, 31(9): 5124-5150. doi: 10.3934/era.2023262 |
[4] | Fengrong Zhang, Ruining Chen . Spatiotemporal patterns of a delayed diffusive prey-predator model with prey-taxis. Electronic Research Archive, 2024, 32(7): 4723-4740. doi: 10.3934/era.2024215 |
[5] | San-Xing Wu, Xin-You Meng . Hopf bifurcation analysis of a multiple delays stage-structure predator-prey model with refuge and cooperation. Electronic Research Archive, 2025, 33(2): 995-1036. doi: 10.3934/era.2025045 |
[6] | Xiaowen Zhang, Wufei Huang, Jiaxin Ma, Ruizhi Yang . Hopf bifurcation analysis in a delayed diffusive predator-prey system with nonlocal competition and schooling behavior. Electronic Research Archive, 2022, 30(7): 2510-2523. doi: 10.3934/era.2022128 |
[7] | Zimeng Lv, Jiahong Zeng, Yuting Ding, Xinyu Liu . Stability analysis of time-delayed SAIR model for duration of vaccine in the context of temporary immunity for COVID-19 situation. Electronic Research Archive, 2023, 31(2): 1004-1030. doi: 10.3934/era.2023050 |
[8] | Rina Su . Dynamic analysis for a class of hydrological model with time delay under fire disturbance. Electronic Research Archive, 2022, 30(9): 3290-3319. doi: 10.3934/era.2022167 |
[9] | Ruizhi Yang, Dan Jin . Dynamics in a predator-prey model with memory effect in predator and fear effect in prey. Electronic Research Archive, 2022, 30(4): 1322-1339. doi: 10.3934/era.2022069 |
[10] | Qinghua Zhu, Meng Li, Fang Han . Hopf bifurcation control of the ML neuron model with Hc bifurcation type. Electronic Research Archive, 2022, 30(2): 615-632. doi: 10.3934/era.2022032 |
In modern wireless communication systems, accurate estimation of wireless channels is essential for detecting transmitted signals, decoding them optimally, and recovering data. The OFDM technique is widely used in 5G wireless communication systems to combat the effects of multi-path fading and interference [1,2]. OFDM is a digital modulation technique that divides a wide-band channel into multiple narrow-band sub-channels, each of which is modulated with a different carrier frequency. This helps to mitigate the effects of multi-path fading, which can cause a signal to experience multiple reflections and arrivals at the receiver, leading to distortion and fading [3]. The estimation of wireless channels is typically performed using conventional techniques such as LS and MMSE methods [2,3]. However, the performance of these methods can be limited by non-linearities and imperfections in the system. To address these issues, DNNs have been increasingly used to model wireless channel characteristics [4]. By leveraging the power of machine learning, DNNs can learn the complex mapping between transmitted signals and received signals, and provide more accurate and robust channel estimation. With the growing popularity of DNN-based techniques, they are expected to play a crucial role in the development of advanced wireless communication systems, including 5G and beyond [5,6,7].
Mohammed A.S.M et al [8] have shown that DNNs with LSTM layers can outperform conventional channel estimation methods in OFDM systems with different channel models. D Venkata Ratnam et al [9] compared the performance of DNNs with LSTM and Bidirectional LSTM (Bi-LSTM) layers, as well as conventional channel estimation methods, in OFDM systems. Their results showed that the Bi-LSTM based DNN estimator outperformed both the LSTM DNN and the conventional methods in terms of accuracy and robustness. To further improve the performance of OFDM systems, advanced neural network techniques have been proposed, such as the LSTM Projected Layer (LSTM-PL). The LSTM-PL is a recurrent neural network(RNN) that is well-suited for predicting and estimating time-series data, making it an excellent candidate for wireless channel estimation [10]. The LSTM-PL model includes a projection layer that feeds into an LSTM layer, enabling more efficient processing of sequential data. The model can be trained using a vast data set of channel response samples to predict channel responses based on received signals.
Unlike traditional channel estimation methods like LS and MMSE methods, the LSTM-PL model doesn't need statistical information about the channel and can handle imperfections and non-linearities more effectively. Simulations evaluate the performance of the LSTM-PL model for wireless channel estimation, and the results shows that the proposed method outperforms the traditional LS, MMSE, LSTM methods in terms of SER performance. The LSTM-PL model has also been tested in various multipath scenarios, demonstrating its ability to estimate the wireless channel effectively under different channel conditions.
The LSTM-PL's unique architecture and memory mechanisms allow it to effectively learn from past data, capture dependencies between sequences, and accurately predict future outcomes. LSTMs have become popular in the field of deep learning because they are capable of assimilating information and long-term retention of data. In addition, LSTMs have been shown to be highly effective at tasks that involve processing sequences of variable length, due to their ability to adaptively adjust the memory cells in response to the specific characteristics of the input data [11].
The LSTM layer structure and cell state equations are given in [11]. Figure 1 shows the block diagram view of an LSTM projected Layer. In an LSTM projected layer, the memory cells are projected onto an output space through a linear transformation, which allows the network to produce a more interpretable representation of the stored information [12]. This projected representation can then be used to make predictions or decisions based on the input sequence. The projection layer also enables the network to be more easily fine-tuned for specific tasks, as the weights in the projection layer can be adjusted to optimize performance.
LSTM-PL offers several advantages over traditional LSTMs in certain applications. By projecting the memory cells onto an output space through a linear transformation, LSTM projected layers produce a more interpretable representation of the stored information, which can be useful in applications where it is important to understand the reasoning behind the predictions made by the network. Additionally, the projection layer in LSTM-PL can be fine-tuned to optimize performance on specific tasks, making them more flexible and adaptable to different applications [10].
The sizes of the learnable parameters of the layers that come after the projected layer are the same as those in the network that does not have the LSTM projected layer. When an LSTM layer is projected, it reduces the number of learnable parameters instead of reducing the number of hidden units, thus keeping the output size of the layer and downstream layers the same. This approach can lead to better prediction accuracy as it helps the network learn a more compact and expressive representation of the input sequence. In many LSTM-PL have been found to outperform traditional LSTMs due to the projection layer's ability to extract more relevant information from the stored memory. Furthermore, LSTM-PL can be more computationally efficient than traditional LSTMs, as they do not require separate computation for the gating mechanisms [10,12].
The OFDM system with transmitter, receiver and LSTM-PL DNN network shown in Figure 2. This system operates in a similar fashion to conventional OFDM systems. To initiate transmission, symbols with pilots are converted into a parallel data stream on the transmitter side. The stream is then transformed from the frequency domain to the time domain via an inverse discrete Fourier transform (IDFT). To address inter-symbol interference (ISI), a cyclic prefix (CP) is appended, with a length that is at least equal to the maximum delay spread of the channel [13].
Let a sample-spaced multi-path channel be denoted by complex random variables with N taps as {h(n)}N−10, where each tap represents a different propagation delay, attenuation, and phase shift associated with the individual paths within the channel. Then the received OFDM signal can be represented as,
y(n)=x(n)⊙h(n)+w(n) | (2.1) |
where x(n) is the transmitted signal, w(n) Additive White Gaussian Noise(AWGN) and ⊙ represents circular convolution. After removing the CP and performing DFT, the received frequency domain signal is
Y(k)=X(k)H(k)+W(k) | (2.2) |
where Y(k), X(k), H(k) and W(k) are the DFT of y(n), x(n), h(n) and w(n) respectively [4].
The first OFDM block of a frame is used for pilot symbols while subsequent blocks are used for data transmission. This allows the channel to be considered constant over both blocks but changing between frames. A two-stage process is used to develop a reliable LSTM-PL DNN model for joint channel estimation and symbol detection. The model is trained during the offline stage using diverse information sequences and various channel conditions, including urban or hilly terrain delay profiles with specific statistical properties. The effectiveness of the LSTM-PL model was assessed for signal detection and channel estimation in OFDM wireless communication systems. The proposed DNN model comprises an input layer, three hidden layers, and an output layer. The input layer contains 256 nodes, and its size is determined by the sum of the real and imaginary parts of two OFDM blocks. Each block includes pilots and transmitted symbols used for channel estimation. The hidden layers are designed to learn the complex temporal dynamics of the wireless channel, while the output layer has four nodes that correspond to the predicted symbols, which correspond to the transmitted symbols. During online deployment, the LSTM-PL DNN model can recover transmitted data without requiring explicit estimation of the wireless channel. This is possible because the DNN can adapt to channel characteristics through training on a large datasets, enabling it to operate in real-time without complex channel estimation algorithms.
The DNN model is trained by utilizing simulation data generated from diverse channel models to effectively learn the channel characteristics [14]. In the proposed LSTM-PL model, the narrow band Rayleigh fading channel model [15,16,17] is employed for simulation. This model comprises of five layers, as shown in Figure 3, starting with the sequence input layer that has an input size of 256. The subsequent layer is the LSTM-PL with fewer input and output projected size. Following that, the four classes are formed through the utilization of a fully connected layer, a softmax layer, and finally, a classification layer which holds an output size of four. The model is trained to minimize the loss function, which serves as the key objective of the training process. In the proposed model, crossentropyex [8] is implemented as the loss function, facilitating the efficient calculation and reduction of the difference between the predicted and actual outputs. The model is trained to minimize the cost function using Adam optimizer and iteration is carried out till the error difference is within the threshold limit or very less.
The potency of the DL LSTM-PL DNN-based channel state estimator is demonstrated in this section. To do this, the LSTM-PL DNN was offline trained on simulated datasets. The conventional as well as DNN-based estimators were compared with the proposed estimator SER at various SNRs. The training options and parameters for the LSTM-PL-based channel estimator can be seen in Table 1, while Table 2 contains the simulation parameters for the OFDM systems. During training, the LSTM-PL DNN is fed with the received signal, which consists of the OFDM pilots and transmission symbols, and the DNN outputs the corresponding symbol type of the transmitted signal. The pilot sequence was interleaved with a portion of the data symbols, and 10, 000 OFDM packets were created. From these packets, 80 percentage were utilized for training, and remaining 20 percentage were utilized for validation and testing. The difference between the estimated and actual channel response is then computed using loss function, and the DNN parameters are updated using back propagation to minimize this loss.
Simulation Parameters | Value |
Input data size | 256 |
Hidden Layers | 16 |
LSTM-PL Output projected size | 0.25× Hidden Layer |
Optimization Techniques | Adam |
Loss Function | crossentropyex |
State activation function | tanh |
Gate activation function | sigmoid |
Mini-Batch size | 1000 |
Maximum epochs | 100 |
Momentum | 0.9 |
Parameter | Value |
Type of Modulation | 4-ary Phase Shift Keying(QPSK) |
OFDM Center Frequency | 2.6 GHz |
Channel Taps | 24 |
CP | 18 |
Number of OFDM sub-carries | 64 |
FFT size | 64 |
Pilots | 8 and 4 |
By training the LSTM-PL DNN using received symbols comprising OFDM pilots and data symbols, the DNN can effectively learn the channel characteristics associated with the given pilot, capturing the intricate temporal dynamics of the channel response over time. Once trained, the DNN can be utilized to detect symbols in real-time for new input data.
The performance of the proposed estimator was assessed by training it with the Adam optimization technique under different pilot conditions 8 pilots and 4 pilots. To determine their effectiveness, the proposed estimator was compared in terms of SER with conventional LS, MMSE, and DNN-based estimators like LSTM-DNN and Bi-LSTN DNN estimators. These estimators were tested under identical channel conditions using a fewer number of pilots 8 and 4.
Figure 5 shows the SER performance when only 4 pilots are used. Here, both conventional estimators LS and MMSE lose their estimating power because as SNR increases, SER increases. There is no improvement of SER as SNR increases. However, DNN-based estimators perform much better than conventional LS and MMSE estimators. Additionally, it can be observed that out of all DNN-based estimators, LSTM-PL DNN performs much better than LSTM-DNN and Bi-LSTM-DNN.
Table 3 presents a comparison of the total learnable parameters of LSTM-PL, LSTM, and Bi-LSTM DNN based on simulations. The implementation of LSTM-PL necessitates only 10, 500 learnable parameters, which is significantly lower in comparison to the number of parameters required by other DNNs such as LSTM and Bi-LSTM. Generally, LSTM networks necessitate 17, 500 learnable parameters, while Bi-LSTM networks require up to 35, 000 parameters. The number of learnable parameters in a DNN is directly proportional to its computational complexity [8], and in this case, it is evident that the proposed LSTM-PL DNN has the least computational complexity among all the DNNs mentioned above.
DNN | Total Learnable parameters |
LSTM-PL | 10500 |
LSTM | 17500 |
Bi-LSTM | 35000 |
A novel approach to OFDM channel estimation using the DNN method has been developed, utilizing LSTM-PL neural networks. The proposed LSTM-PL DNN is trained offline and used online to track the channel statistics for reconstructing the transmitted symbols. The performance of the proposed DNN has been evaluated using two different pilot configurations. The experiments conducted using the LSTM-PL DNN estimator trained with Adam optimizer show superior performance compared to the conventional estimators, as well as DNN estimators like LSTM-DNN and Bi-LSTM DNN when pilots of 8 and 4 are used. Specifically, the proposed LSTM-PL DNN based approach performs better than all other estimators when only a small number of pilots are available. Additionally, the proposed method has a significantly lower computational complexity compared to that of the LSTM and Bi-LSTM DNN methods. Moreover, the proposed approach holds immense potential for enhancing communication systems, including 5G and beyond, due to its independence from prior channel knowledge. Future research may entail conducting tests on real devices or utilizing authentic training data.
All authors declare no conflicts of interest in this paper.
[1] | de Mira NVM, Massaretto IL, Pascual CDSCI, et al. (2009) Comparative study of phenolic compounds in different Brazilian rice (Oryza sativa L.) genotypes. J Food Compos Anal 22: 405-409. |
[2] | Shao Y, Xu F, Sun X, et al. (2014) Identification and quantification of phenolic acids and anthocyanins as antioxidants in bran, embryo and endosperm of white, red and black rice kernels (Oryza sativa L.). J Cereal Sci 59: 211-218. |
[3] | Gao Y, Guo X, Liu Y, et al. (2018) Comparative assessment of phytochemical profile, antioxidant capacity and antiproliferative activity in different varieties of brown rice (Oryza sativa L.). LWT 96: 19-25. |
[4] | Razak DLA, Abd Rashid NY, Jamaluddin A, et al. (2015) Enhancement of phenolic acid content and antioxidant activity of rice bran fermented with Rhizopus oligosporus and Monascus purpureus. Biocatal Agric Biotechnol 4: 33-38. |
[5] | Noviasari S, Kusnandar F, Setiyono A, et al. (2019) Profile of phenolic compounds, DPPH-scavenging and anti α-amylase activity of black rice bran fermented with Rhizopus oligosporus. Pertanika J Trop Agric Sci 42: 489-501. |
[6] | Bhanja T, Kumari A, Banerjee R (2009) Bioresource technology enrichment of phenolics and free radical scavenging property of wheat koji prepared with two filamentous fungi. Bioresour Technol 100: 2861-2866. |
[7] | Oliveiera M, Cipolatti EP, Badiale-furlong E, et al. (2012) Phenolic compounds and antioxidant activity in fermented rice (Oryza sativa) Phenolic compounds and antioxidant activity in fermented rice (Oryza sativa) bran. Food Sci Technol 32: 531-536. |
[8] | Hayat A, Jahangir TM, Khuhawar MY, et al. (2015) HPLC determination of gamma amino butyric acid (GABA) and some biogenic amines (BAs) in controlled, germinated, and fermented brown rice by pre-column derivatization. J. Cereal Sci 64: 56-62. |
[9] | AOAC (2015) Official methods of analysis of AOAC International 18th edition. AOAC International. |
[10] | Giusti MM, Wrolstad RE (2001) Characterization and Measurement of Anthocyanins by UV-Visible Spectroscopy. Current Protocols in Food Analytical Chemistry. John Wiley & Sons, Inc., New York. DOI: 10.1002/0471142913.faf0102s00. |
[11] | Reddy CKR, Imi LK, Aripriya SH, et al. (2017) Effects of polishing on proximate composition, physico- chemical characteristics, mineral composition and antioxidant properties of pigmented rice. Rice Sci 24: 241-252. |
[12] | Brand-Williams W, Cuvelier ME, Berset C (1995) Use of a free radical method to evaluate antioxidant activity. LWT-Food Sci Technol 28: 25-30. |
[13] | AACC (1999) AACC International Method. 61-03.01: Determination of the pasting properties of rice with the rapid visco analyzer. Minnesota (US): American Association of Cereal Chemists. 2-5. |
[14] | Surojanametakul V, Panthavee W, Satmalee P, et al. (2019) Effect of traditional dried starter culture on morphological, chemical and physicochemical properties of sweet fermented glutinous rice products. J Agric Sci 11: 43-51. |
[15] | Chinsamran K, Piyachomkwan K, Santisopasri V (2005) Effect of lactic acid fermentation on physico-chemical properties of starch derived from cassava, sweet potato and rice effect of lactic acid fermentation on physico-chemical properties of starch derived from cassava, sweet potato and rice. Kasetsart J Nat Sci 39: 76-87. |
[16] | Chu J, Zhao H, Lu Z, et al. (2019) Improved physicochemical and functional properties of dietary fi ber from millet bran fermented by Bacillus natto. Food Chem 294: 79-86. |
[17] | SNI (2009) Tepung Beras. Badan Standardisasi Nasional. Jakarta. Available from: https://bsn.go.id/uploads/download/skema_tepung_%E2%80%93_lampiran_xx_perka_bsn_11_tahun_2019.pdf. |
[18] | Liang J, Han BZ, Nout MJR, et al. (2008) Effects of soaking, germination and fermentation on phytic acid, total and in vitro soluble zinc in brown rice. Food Chem 110: 821-828. |
[19] | Liang J, Li Z, Tsuji K, Nakano K, et al. (2008) Milling characteristics and distribution of phytic acid and zinc in long-, medium- and short-grain rice. J. Cereal Sci 48: 83-91. |
[20] | Iwai T, Takahashi M, Oda K, et al. (2014) Dynamic changes in the distribution of minerals in relation to phytic acid accumulation during rice. Plant Physiol 160: 2007-2014. |
[21] | Suresh S, Radha KV (2015) Effect of a mixed substrate on phytase production by Rhizopus oligosporus MTCC 556 using solid state fermentation and determination of dephytinization activities in food grains. Food Sci Biotechnol 24: 551-559. |
[22] | Oduguwa OO, Edema MO, Ayeni A (2008) Physico-chemical and microbiological analyses of fermented corn cob, rice bran and cowpea husk for use in composite rabbit feed. Bioresour Technol 99: 1816-1820. |
[23] | Benabda O, Sana M, Kasmi M, et al. (2019) Optimization of protease and amylase production by Rhizopus oryzae cultivated on bread waste using solid-state Fermentation. J Chem 2019: 1-9. |
[24] | Handoyo T, Morita N, (2006) Structural and functional properties of fermented soybean (tempeh) by using Rhizopus oligosporus. Int J Food Prop 9: 347-355. |
[25] | Verma DK, Srivastav PP (2017) Proximate composition, mineral content and fatty acids analyses of aromatic and non-aromatic indian rice. Rice Sci 24: 21-31. |
[26] | Oliveira S, Feddern V, Kupski L, et al. (2011) Bioresource technology changes in lipid, fatty acids and phospholipids composition of whole rice bran after solid-state fungal fermentation. Bioresour Technol 102: 8335-8338. |
[27] | Oliveira MDS, Feddern V, Kupski L, et al. (2010) Physico-chemical characterization of fermented rice bran biomass[ Caracterización fisico-química de la biomasa del salvado de arroz fermentado]. CyTA-J Food 8: 229-236. |
[28] | Ribeiro AC, Graca CS, Chiattoni ML, et al. (2017) Fermentation process in the availability of nutrients in rice bran. RR: J Microbiol Biotechnol 6: 45-52. |
[29] | Kong EL, Lee BK, Michelle, et al. (2015) DNA damage inhibitory effect and phytochemicals of fermented red brown rice extract. Asian Pacific J Trop Dis 5: 732-736. |
[30] | Schmidt CG, Gonç alves LM, Prietto L, et al. (2014) Antioxidant activity and enzyme inhibition of phenolic acids from fermented rice bran with fungus Rizhopus oryzae. Food Chem 146: 371-377. |
[31] | Kumar P, Prakash KS, Jan K, et al. (2017) Effects of gamma irradiation on starch granule structure and physicochemical properties of brown rice starch. J Cereal Sci 77: 194-200. |
[32] | Zhang MW, Zhang RF, Zhang FX, et al. (2010) Phenolic profiles and antioxidant activity of black rice bran of different commercially available varieties. J Agric Food Chem 58: 7580-7587. |
[33] | Chaiyasut C, Pengkumsri N, Sirilun S, et al. (2017) Assessment of changes in the content of anthocyanins, phenolic acids, and antioxidant property of Saccharomyces cerevisiae mediated fermented black rice bran. AMB Expr 7: 114. |
[34] | Abdel-Aal ESM, Young JC, Rabalski I (2019) Anthocyanin composition in black, blue, pink, purple, and red cereal grains. Agric Food Chem 54: 4696-4704. |
[35] | Maulani RR, Sumardi D, Pancoro A (2019) Total flavonoids and anthocyanins content of pigmented rice. Drug Invent Today 12: 369-373. |
[36] | Luximon-Ramma A, Bahorun T, Soobrattee M, et al. (2002) Antioxidant activities of phenolic, proanthocyanidin, and flavonoid components in extracts of Cassia fistula. J Agric Food Chem 50: 5042-5047. |
[37] | Cao G, Sofic E, Prior RL (1997) Antioxidant and prooxidant behavior of flavonoids: structure-activity relationships. Free Radic Biol Med 22: 749-760. |
[38] | Chen M, Meng H, Zhao Y, et al. (2015) Antioxidant and in vitro anticancer activities of phenolics isolated from sugar beet molasses. BMC Complementary Altern Med 15: 313. |
[39] | Anggraini T, Novelina, Limber U, et al. (2015) Antioxidant activities of some red, black and white rice cultivar from West Sumatra, Indonesia. Pak J Nutr 14: 112-117. |
[40] | Butsat S, Siriamornpun S (2010) Antioxidant capacities and phenolic compounds of the husk, bran and endosperm of Thai rice. Food Chem 119: 606-613. |
[41] | Pang Y, Ahmed S, Xu Y, et al. (2018) Bound phenolic compounds and antioxidant properties of whole grain and bran of white, red and black rice. Food Chem 240: 212-221. |
[42] | Juhász R, Salgó A (2008) Pasting behavior of amylose, amylopectin and their mixtures as determined by rva curves and First Derivatives. Starch-Stärke 60: 70-78. |
[43] | Patindol J, Wang YJ, Jane JL (2005) Structure-functionality changes in starch following rough rice storage. Starch-Stärke 57: 197-207. |
[44] | Olanipekun BF, Otunola ET, Adelakun OE, et al. (2009) Effect of fermentation with Rhizopus oligosporus on some physico-chemical properties of starch extracts from soybean flour. Food Chem Toxicol 47: 1401-1405. |
[45] | Balogun IO, Olatidoye OP, Otunola ET (2019) Effect of fermentation with R. oligosporus and R. stolonifer on some physicochemical properties of starch extracts from dehulled and undehulled. Int Res J Appl Sci 1: 71-75. |
[46] | Ikegwu OJ, Okechukwu PE, Ekumankana EO (2010) Physico-chemical and pasting characteristic of flour and starch from achi Brachytegia eurycoms seed. J Food Technol 8: 58-66. |
[47] | Varavinit S, Shobsngob S, Varanyanond W, et al. (2003) Effect of amylose content on gelatinization, retrogradation and pasting properties of flours from different cultivars of thai rice. Starch-Stärke 55: 410-415. |
[48] | Oloyede OO, James S, Ocheme OB, et al. (2015) Effects of fermentation time on the functional and pasting properties of defatted Moringa oleifera seed flour. Food Sci Nutr 4: 89-95. |
1. | Liping Wang, Xinyu Wang, Dajun Liu, Xuekang Zhang, Peng Wu, Dynamical analysis of a heterogeneous spatial diffusion Zika model with vector-bias and environmental transmission, 2024, 32, 2688-1594, 1308, 10.3934/era.2024061 | |
2. | Yiming Tang, Hairong Lian, Shiwen Jing, Zhaohai Ma, Hopf Bifurcation of an Age-Structured Hand–Foot–Mouth Disease Model in a Contaminated Environment, 2024, 34, 0218-1274, 10.1142/S0218127424501967 |
Simulation Parameters | Value |
Input data size | 256 |
Hidden Layers | 16 |
LSTM-PL Output projected size | 0.25× Hidden Layer |
Optimization Techniques | Adam |
Loss Function | crossentropyex |
State activation function | tanh |
Gate activation function | sigmoid |
Mini-Batch size | 1000 |
Maximum epochs | 100 |
Momentum | 0.9 |
Parameter | Value |
Type of Modulation | 4-ary Phase Shift Keying(QPSK) |
OFDM Center Frequency | 2.6 GHz |
Channel Taps | 24 |
CP | 18 |
Number of OFDM sub-carries | 64 |
FFT size | 64 |
Pilots | 8 and 4 |
DNN | Total Learnable parameters |
LSTM-PL | 10500 |
LSTM | 17500 |
Bi-LSTM | 35000 |
Simulation Parameters | Value |
Input data size | 256 |
Hidden Layers | 16 |
LSTM-PL Output projected size | 0.25× Hidden Layer |
Optimization Techniques | Adam |
Loss Function | crossentropyex |
State activation function | tanh |
Gate activation function | sigmoid |
Mini-Batch size | 1000 |
Maximum epochs | 100 |
Momentum | 0.9 |
Parameter | Value |
Type of Modulation | 4-ary Phase Shift Keying(QPSK) |
OFDM Center Frequency | 2.6 GHz |
Channel Taps | 24 |
CP | 18 |
Number of OFDM sub-carries | 64 |
FFT size | 64 |
Pilots | 8 and 4 |
DNN | Total Learnable parameters |
LSTM-PL | 10500 |
LSTM | 17500 |
Bi-LSTM | 35000 |