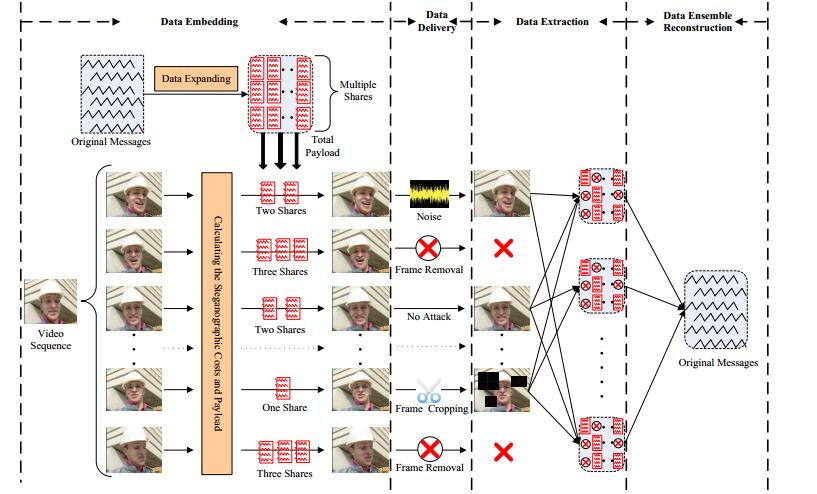
Citation: Jieqiong Yang, Panzhu Luo. Review on international comparison of carbon financial market[J]. Green Finance, 2020, 2(1): 55-74. doi: 10.3934/GF.2020004
[1] | Dingwei Tan, Yuliang Lu, Xuehu Yan, Lintao Liu, Longlong Li . High capacity reversible data hiding in MP3 based on Huffman table transformation. Mathematical Biosciences and Engineering, 2019, 16(4): 3183-3194. doi: 10.3934/mbe.2019158 |
[2] | Yongju Tong, YuLing Liu, Jie Wang, Guojiang Xin . Text steganography on RNN-Generated lyrics. Mathematical Biosciences and Engineering, 2019, 16(5): 5451-5463. doi: 10.3934/mbe.2019271 |
[3] | Kun Zheng, Junjie Shen, Guangmin Sun, Hui Li, Yu Li . Shielding facial physiological information in video. Mathematical Biosciences and Engineering, 2022, 19(5): 5153-5168. doi: 10.3934/mbe.2022241 |
[4] | Wanru Du, Xiaochuan Jing, Quan Zhu, Xiaoyin Wang, Xuan Liu . A cross-modal conditional mechanism based on attention for text-video retrieval. Mathematical Biosciences and Engineering, 2023, 20(11): 20073-20092. doi: 10.3934/mbe.2023889 |
[5] | Xue Li, Huibo Zhou, Ming Zhao . Transformer-based cascade networks with spatial and channel reconstruction convolution for deepfake detection. Mathematical Biosciences and Engineering, 2024, 21(3): 4142-4164. doi: 10.3934/mbe.2024183 |
[6] | Xianyi Chen, Anqi Qiu, Xingming Sun, Shuai Wang, Guo Wei . A high-capacity coverless image steganography method based on double-level index and block matching. Mathematical Biosciences and Engineering, 2019, 16(5): 4708-4722. doi: 10.3934/mbe.2019236 |
[7] | Shanqing Zhang, Xiaoyun Guo, Xianghua Xu, Li Li, Chin-Chen Chang . A video watermark algorithm based on tensor decomposition. Mathematical Biosciences and Engineering, 2019, 16(5): 3435-3449. doi: 10.3934/mbe.2019172 |
[8] | Pedro R. Palos Sánchez, José A. Folgado-Fernández, Mario Alberto Rojas Sánchez . Virtual Reality Technology: Analysis based on text and opinion mining. Mathematical Biosciences and Engineering, 2022, 19(8): 7856-7885. doi: 10.3934/mbe.2022367 |
[9] | Jimmy Ming-Tai Wu, Jerry Chun-Wei Lin, Philippe Fournier-Viger, Youcef Djenouri, Chun-Hao Chen, Zhongcui Li . The density-based clustering method for privacy-preserving data mining. Mathematical Biosciences and Engineering, 2019, 16(3): 1718-1728. doi: 10.3934/mbe.2019082 |
[10] | Qing Ye, Qiaojia Zhang, Sijie Liu, Kaiqiang Chen . A novel chaotic system based on coupled map lattice and its application in HEVC encryption. Mathematical Biosciences and Engineering, 2021, 18(6): 9410-9429. doi: 10.3934/mbe.2021463 |
Steganography is an efficient privacy communication measurement in which secret messages are embedded into digital media, such as digital images, video or audio files, to implement the information delivery [1,2,3,4]. As a countermeasure to steganography, steganalysis [5,6,7,8] is mainly used to detect the presence of hidden data in a digital media. Traditional steganography mainly involves single digital image, and always combines the side-information based distortion function and Syndrome-Trellis Codes (STC) [4] to implement steganography. For example, data hider uses the non-round coefficients from one uncompressed image to measure the steganography costs of each DCT coefficient and then embed messages when saving them as compressed images. This strategy is consistently feasible in the context of modern steganography, because the side-information is not available to the recipient (or steganalyst). However, when the size of secret messages is too big, the steganography for single image does not work. One feasible way is to hide the messages among a batch of images. We usually name this scheme as batch steganography [9,10,11]. Nevertheless, batch steganography is not easy, at least inconvenient, to apply in real world due to the following two reasons. First, traditional distortion function mainly focuses on single image, the distortion definition for batch steganography is not straightforward. Second, batch steganography needs a huge of homogeneous covers, which are hard to be obtained due to diverse social networks.
One natural question arises: Is there a kind of media that can avoid the above two problems to facilitate data hiding? The answer is positive. Video sequences provide this possibility because they usually consist of a number of homogeneous image frames and thus have a higher capacity. Nevertheless, some ones may wonder if video steganography is as successful as traditional side-information based image steganography, because most of videos from different acquisition devices, e.g. cell phones or digital cameras, are always saved as JPEG format, not the uncompressed format. Accordingly, if we use the video to hide the secret messages, it has to answer two key questions: (1) How to design optimal distortion function by using continuous video frames with same (or approximate) scenes? (2) How to design embedding strategy to ensure that stego videos can resist diverse network attacks, such as usual noise attacks, video frame attacks and video compression attacks?
Aiming at the first question, since video always contains compressed image frames, the existing distortion function based on side-information cannot be transplanted directly. Nevertheless, we can get some inspiration from different definition of side-information, for example, designing the steganography cost by using multiple image with the same scene. Actually, several works has been developed in this direction. In [12], the authors proposed a new view to model the differences between the printed image and its scan version. Unfortunately, this scheme is inconvenient due to two pitfalls: (1) this scheme is rather labor-consuming due to requiring a large number of scan versions. (2) the difference among scan images maybe lead to the complication increasing. To remove this weakness, in [13], the authors designed a different type of side-information by multiple compressed images with the same scene. This scheme avoided time-consuming and formed a more secure method even if only two images are used. Although a quite significant increasing for anti-detection can be obtained easily with respect to the case of single image, this scheme is rather difficult to be practical because the image database is hard to build.
Regarding the second question, existing video steganographic methods [14,15,16,17,18,19] can be divided into two categories according to the information embedding domain. One is spatial domain based video steganography, in which the data is embedded directly into raw pixel values, and they usually refer to the processing of image steganography, such as Least Significant Bit (LSB) Matching method [1], Spread Spectrum (SS) method [20], and BCH code [15] et al.. Although spatial methods can embed high capacity messages, it is inevitable to loss the hidden messages once the stego videos are damaged by unexpected network interference, such as noise, compression, or frame losing. Another type is the joint-compression domain video steganography. In this category, most of methods embed data into different types of compressed video, e.g. motion vectors (MVs) methods [17,18], inter/intra prediction methods [19], quantized DCT coefficients methods [14,16] et al.. These compression domain based methods have a similarity, that is, lower embedding capacity. Moreover, although some compression domain based methods can effectively resist double compression attack, the secret messages are very hard to be recovered once the video frames are lost or damaged during delivery. Therefore, the robustness for video steganography needs to be further improved. This paper tries to fill this gap.
Facing the aforementioned problems, we make the following novel contributions in video steganography:
● We propose a robust video steganography scheme by a new distortion function and ensemble reconstruction mechanism. The proposed solution can not only improve the security performance of stego video, but also ensure the completeness of original data even if some video frames are damaged in delivery.
● Proposed scheme investigates another form of side-information by referring the adjacent image frames with the same scene, and then employs the side-information to design distortion function. This distortion function is effective because the message senders do not need to access the uncompressed image frames.
● Vandermonde matrix is used to expand and divide original data to multiple shares, which are embedded in the continuous frames by combining the designed distortion function and STC algorithm. Subsequently, ensemble reconstruction mechanism is designed to ensure the completeness and correctness of original data, even if partial data is damaged during delivery.
● Comprehensive experiments are performed with classical video sequences. The experimental results demonstrate that proposed scheme can significantly improve the overall performance on visual quality, robustness and anti-steganalysis, leading to a superiority for existing video steganographic methods.
The rest of this paper is organized as follows. Section 2 provides the details of proposed scheme by introducing the procedure of distortion function and data ensemble reconstruction. Subsequently, comprehensive experiments are performed to evaluate the performance of proposed scheme. The experimental results and corresponding discussions are presented in Section 3. Finally, Section 4 concludes the paper.
The framework of our proposed robust video steganography scheme is shown in Figure 1. The proposed scheme is mainly comprised of two parts: data embedding and data extraction. In the data embedding stage, we firstly use a multi-ary Vandermonde matrix to expand original data and then divide them into multiple small shares, which are considered as "actual embedding data". Secondly, we consider the continuous adjacent frames with same scene as pre-cover to provide the side-information, and then design an efficient distortion function by referring to the adjacent frames. Finally, the data shares are embedded into each frame by an existing cost-based embedding scheme. In the data extraction stage, we extract the undamaged shares from the received video frames, and then recover the original data by an ensemble reconstruction mechanism, although the video frames might be damaged or intercepted during the delivery. We claim that the original data can be recovered perfectly as long as the recipient can obtain enough undamaged shares.
A video sequence usually contains a lot of image frames with (approximately) same scene, if message sender hopes to deliver secret messages by video sequence, he can spread the messages into continuous image frames. At the receiving end, the receiver extracts the secret messages from these image frames according to a fixed order. Unfortunately, video sequences may be attacked/damaged during transmission, such as the network noise or the warden who might try to remove the video frames. In this case, it is unreasonable to assume that the recipient can receive the information completely and accurately. To improve the robustness of video steganography, in this section, we try to use matrix decomposition mechanism [11,21] to divide the original messages into multiple shares. Since each share only carry a small portion of valid information, partial loss for these shares do not affect the recovery for original messages. The corresponding details can be explained by Figure 2.
Assume that the given secret messages are a binary stream. To expand the original data, we first present the original data into q-ary symbol system, where q is an odd prime. Actually, this procedure is rather simple. The messages are segmented into multiple pieces. Each piece contains L1 bits, which can be converted to L2 q-ary digits according to the following equation.
L1=⌊L2⋅log2q⌋. | (2.1) |
We can provide a simple sample to explain it graphically. Assume that L1=4, L2=2 and the original data is converted into 5-ary notational system. Three binary pieces, (1101 0110 1001), can be converted to six 5-ary digits (23 11 14). Notably, the size reduction from L1 to L2 can be calculated by the following equation.
r=1−L1L2⋅log2q | (2.2) |
Clearly, when L1 and L2 are very large, r is close to 0.
When the original data (binary stream) is converted completely, we integrate all q-ary digits as a sequence and then expand them into multiple shares by the following steps.
Step 1: Segment the q-ary digit sequence into K small blocks. Denote each of them as {dk,1,dk,2,⋯,dk,m}, where m represents the length of a digital block and k∈[1,K].
Step 2: Build Vandermonde matrix A
A=[11⋯1a1a2⋯ana21a22⋯a2n⋮⋮⋯⋮am−11am−12⋯am−1n]modq | (2.3) |
where a1,a2,⋯,an∈[0,q−1] are named as the indices of A and they are different with each other. In addition, m, n, and q must satisfy m≤n≤q.
Step 3: With the following equation, each digital block {dk,1, dk,2, ⋯, dk,m} can be expanded to n shares.
[tk,1tk,2tk,3⋯tk,n]=[dk,1dk,2dk,3⋯dk,m]⋅A, | (2.4) |
where the symbol "⋅" in Equation (2.4) presents the multiplication operator in q-ary notational system.
In order to understand data decomposition mechanism easily, we provide an actual example. Assume that q=7, n=6, m=3, and the original data are three 7-ary digits [2 1 4]. We set the indices a1,a2,⋯,an of Vandermonde matrix as [5 3 1 0 2 4]. So, the Vandermonde matrix can be built easily by Equation (2.3) and the original digit vector [2 1 4] can be expanded as [2 6 0 2 6 0] according to Equation (2.4).
According to the above steps, m q-ary digits from the original data can be expanded easily to n q-ary digits. Obviously, there is some redundancy in these n q-ary digits. We denote the redundancy rate as Re, which can be calculated easily as follows.
Re=1−mn. | (2.5) |
Obviously, as long as the loss (or damaged) rate for tk,1, tk,2, tk,3, ⋯, tk,n is not more than Re, the original digits dk,1, dk,2, dk,3, ⋯, dk,m can be just reconstructed by Equation (2.6).
[dk,1dk,2dk,3⋯dk,m]=[t′k,1t′k,2t′k,3⋯t′k,m]⋅(A′)−1 | (2.6) |
where t′k,1, t′k,2, t′k,3, ⋯, t′k,m are m undamaged digits, which are selected from the received digits (they maybe contain the wrong digits). A′ is m×m Vandermonde matrix built by the indices a′1, a′2, ⋯, a′m (referring to Equation (2.3)). A′−1 is the inversion matrix of A′ in q-ary notational system, whose calculation details can be found in [11].
In this section, we describe the design details of a new distortion function when the sender possesses more than two continuous frames with the (approximately) same scene. Since the continuous video frames have strong correlation, when multiple continuous frames are used to carry the given messages, we can consider the adjacent frames as pre-covers, and calculate steganographic cost (distortion) of current cover to provide a better guidance for video steganography.
In the following, we describe the detailed designing procedure of distortion function when the continuous three cover frames are available. These three frames are considered as JPEG version and denoted F(1), F(2) and F(3). We denote the quantized DCT coefficients in three frames as x(1)ij, x(2)ij and x(3)ij, respectively. We then pronounce x(2)ij as cover frame, x(1)ij and x(3)ij as side-information.
When x(1)ij and x(3)ij are considered as side-information, the message sender can calculate the steganographic cost of modifying coefficient x(2)ij by -1 and +1. The corresponding costs are denoted as ρ(2)ij(−1) and ρ(2)ij(+1). In order to ensure that the proposed distortion function can reflect the cost of changing the cover coefficient x(2)ij more accurately, we select the classical embedding schemes, such as J-UNIWARD [3], as the basis of calculating steganographic cost. Since the side-information is used to improve further the accuracy of distortion function, we keep the original costs (the costs calculating by classical steganographic schemes) when x(1)ij = x(2)ij and x(2)ij = x(3)ij, otherwise, re-modulate them. In other words, the values x(1)ij and x(3)ij are only useful when x(1)ij ≠ x(2)ij OR x(2)ij ≠ x(3)ij. Since proposed distortion function refers to two adjacent frames, the new cost ρ′ij(±1) can be explained by the following four-cases procedures:
Case1: When x(1)ij=x(2)ij and x(2)ij=x(3)ij,
ρ′ij(±1)=ρ(2)ij(±1). | (2.7) |
Case2: When x(1)ij≠x(2)ij and x(2)ij=x(3)ij,
ρ′ij(sij)=α(Q)ρ(2)ij(sij)+ρ(2)ij(sij)2, | (2.8) |
where sij=sign(x(1)ij−x(2)ij).
Case3: When x(1)ij=x(2)ij and x(2)ij≠x(3)ij,
ρ′ij(sij)=ρ(2)ij(sij)+β(Q)ρ(2)ij(sij)2, | (2.9) |
where sij=sign(x(3)ij−x(2)ij).
Case4: When x(1)ij≠x(2)ij and x(2)ij≠x(3)ij
ρ′ij(sij)=α(Q)ρ(2)ij(sij)+β(Q)ρ(2)ij(sij)2, | (2.10) |
where sij=sign(x(1)ij−x(2)ij+x(3)ij−x(2)ij).
Clearly, α(Q) and β(Q) are two modulation factors referring to the compression factor Q, where α(Q), β(Q)∈[0,1] and Q∈[1,100]. They are utilized to control the actual cost values calculated from the side-information and will be discussed later.
Notably, in this section, we only design an new distortion function, which is considered to provide a better steganographic cost measurement. An actual steganographic method can be formed by combining the new distortion function and STC algorithm [4]. The corresponding details can be found in following section.
Following data decomposition mechanism and distortion measurement, we design a robust video steganographic scheme.
Algorithm 1: Data Embedding in Video Sequence |
Input:Video frames v1,v2,…,vS, T={t1,t2,⋯,tn}, modulation parameters α(Q) and β(Q), multi-ary parameter q. |
Output: Stego video sequence V′. |
1 for t← 1 to S−2 do |
![]() |
23 end |
24 Integrate all stego frames v′1,v′2,…,v′S to build stego video sequence V′\; |
Denote the given secret messages Mo and video sequence as V. Proposed scheme tries to spread Mo into video frames* and ensure that the recipient can get the complete messages even if the video sequence is damaged during delivery. Therefore, proposed scheme is believed to be able to resist the diverse video attacks, such as noise, frame cropping or removal. The specific embedding procedure can be implemented as follows.
* We do not consider that how the sender informs the recipient of the length of each share, or how many shares correspond to a frame or a video sequence, because it could be solved by hiding the information in the frame or video header or by other secret channel.
Step1: Decompose the video sequence V to a batch of frames v1,v2,…,vS, which are ensured to have the (approximate) same scenes†. We denote the quality factor of video frames as Q, and then get two parameters α(Q) and β(Q) by referring to the modulation parameters table, which will be discussed in the next section.
† In fact, the scene cuts are very common in video and always produce the frames with diversity contents. However, we do not discuss this special case lonely, because when new scene is cut, video frames can be re-extracted from the new scene to ensure they have the (approximate) same contents.
Step2: Convert the original data Mo to binary stream, which is subsequently converted to a K×m q-ary digital matrix D={d1,d2,⋯,dm}.
Step3: Build Vandermonde matrix A by a1,a2,⋯,an and calculate the expanded data by the following equation.
T=D×Amodq | (2.11) |
where T={t1,t2,⋯,tn} is a K×n matrix, ti = (t1,i,t2,i,⋯,tK,i)T is a data vector, i∈[1,n]. Since the indices of Vandermonde matrix must be delivered with the expanded data, we denote (t1,i,t2,i,⋯,tK,i,ai)T as a complete share.
Step4: With the expanded data T and the cover frames v1,v2,…,vS. We can embed these shares into each frame by combining costs ρ′ij and STC algorithm. The detailed procedure can be found in Algorithm 1.
Once stego video is delivered through insecure network channel, it might face to diverse network attacks. According to proposed data reconstruction procedure, the recipient can reconstruct the original information by ensemble decision, even if partial stego frames are removed or intercepted during delivery. Assume that the remaining stego frames can extract m′ complete shares (maybe contain some modified digits), n≥m′≥m. We select m shares from the remaining stego frames, and then extract their data vectors t′1, t′2, t′3, ⋯, t′m and the corresponding indices a′1, a′2, ⋯, a′m. The original information can be recovered correctly by an ensemble reconstruction mechanism whose detailed procedure is shown in Algorithm 2.
We also provide an actual example to explain our ensemble mechanism. According to the example in Section 2.2 and results of data decomposition, the complete expanded data should be [2 6 0 2 6 0]. We assume two digits are modified in delivery, that is, the last digit is lost and the second digit is changed (assuming '6' to '4'). Thus, the digits that are received by recipient are [2 4 0 2 6]. Since the original data have three digits, we can randomly select three digits from [2 4 0 2 6] and repeat four times (corresponding to the parameter en=4 in Algorithm 2). Assume that these four selections are [0 2 6], [4 2 6], [2 0 6] and [2 4 2], respectively, we then calculate their Vandermonde inverse matrix according the method in [11] and obtain four "suspicious" original data, [2 1 4], [2 0 1], [2 1 4] and [2 4 2]. Finally, the majority voting (corresponding to the MaxVoting function in Algorithm 2) is used to give the final decision [2 1 4]. An actual example can be found in Figure 3. Although the recipient does not know which digits are modified in video transmission, the ensemble mechanism probably makes a correct decision by majority voting.
Algorithm 2: Data Ensemble Reconstruction From Attacked Video |
Input:m′ data vectors t′1, t′2, t′3, ⋯, t′m′, the indices a′1, a′2, ⋯, a′m′ for m′ data vectors, multi-ary parameter q, ensemble rounds en. |
Output: D={d1,d2,⋯,dm}. |
1 for i← 1 to en do |
![]() |
6 end |
7 D = MaxVoting(D1,D2,D3,⋯,Den). |
We would like to raise the attention to readers that if too many shares are lost, for example, when m′<m, proposed scheme is not able to recover the original data. Actually, the main principle has been explained by Equation (2.5).
In this algorithm, we use ensemble voting strategy (the function MaxVoting in Algorithm 2) to decide the correct original data. Although the received shares are complete, they maybe contain some modified digits, e.g. digit 3 may be changed to 7 due to the noise interference. Thus, the calculated original data matrices D1,D2,D3,⋯,Den in Algorithm 2 might be different. We can give the correct decision by counting the maximum same occurrences for D1,D2,D3,⋯,Den. Also, we do not set m′=m in data reconstruction, because if m′=m, D1,D2,D3,⋯,Den might be different each other, this result is invalid in the ensemble strategy.
We carry out our experiments on a classical video database [22], e.g. Figure 4, which contains 15 test sequences with 4:2:0 YUV format. These video sequences have the same resolution of 352×288, and belong to diverse categories, including people, architecture, landscape, flowers, and so on. The detailed descriptions are given in Table 1.
Sequence | Resolution | Number of frames |
Bus | 352×288 | 150 |
City | 352×288 | 300 |
Coastguard | 352×288 | 300 |
Crew | 352×288 | 300 |
Flower | 352×288 | 250 |
Football | 352×288 | 260 |
Foreman | 352×288 | 300 |
Harbour | 352×288 | 300 |
Highway | 352×288 | 2000 |
Ice | 352×288 | 240 |
Mobile | 352×288 | 300 |
Paris | 352×288 | 1065 |
Soccer | 352×288 | 300 |
Tempete | 352×288 | 260 |
Waterfall | 352×288 | 260 |
In addition, to verify our proposed method, each video is separated to a number of image frames. Then, all frames are compressed with same quality factor to avoid the influence of different quantization matrices for steganalysis. Since only the luminance contains a lot of non-zero coefficients for video frames, we just hide the messages into Y component. On the other hand, for a given steganographic algorithm, all frames are embedded with random messages embedding and then create new stego videos. Moreover, in order to test the performance of proposed scheme, we select some experimental video to train the corresponding parameters, e.g. α(Q) and β(Q). The ensemble classifier is employed to show the comparable results.
As the main concern of steganography, embedding capacity and anti-steganalysis performance are two important focuses. In our experiments, we measure the embedding capacity by bit per frame (bpf for short), which is explained as follows.
r=ThetotalnumberofembeddingbitsThenumberofframes | (3.1) |
Similarly, the anti-steganalysis performance is evaluated by minimum average classification probability error (PE in short).
PE=minPFA(PFA+PMD)/2 | (3.2) |
where PFA and PMD are the false-alarm and the missing detection rates of a detector, respectively.
We design a series of experiments to test the contribution of two modulation parameters α(Q) and β(Q) and give their optimal values, which are determined when the PE has the minimum.
Two video sequences, Highway and Paris, are used to perform this experiment because they contain more frames (2000+1065=3065). We save these frames with different quality factors and then divide them equally into training and testing sets. Gabor Filter Residual (GFR) feature set [7] and ensemble classifier [23] are used to provide the experimental results because they can effectively detect modern steganography, e.g. J-UNIWARD [3]. We determine the optimal modulation factors experimentally by getting the minimal PE. Six quality factors, 70, 75, 80, 85, 90, 95, are tested to obtain the optimal modulation parameters. In Figure 5, we give the changing trend of two parameters under relative payloads r=0.2Kbpf. As can be seen in this figure, there is only a slight difference between α(Q) and β(Q). This is because the adjacent frames have the same scene (same content). When the continuous adjacent frames are used as the reference (pre-cover) of current frame, there might have a (approximate) same steganographic costs. In addition, we can see that the modulation value becomes significantly bigger with the quality factors increasing. In fact, this interesting phenomenon is mainly related to the calculation procedure of original distortion function.
Table 2 shows the optimal modulation parameters for different payloads by carrying out a series of experiments. It can be observed that the optimal modulation parameter values gradually decrease with the payload increasing. Actually, since the new distortion depends on the original distortion (e.g. ρij(±1) in Section 2.3), with respect to embedding in single frame, the new distortion function referring to the continuous adjacent frames will significantly increase empirical security, especially for the large payloads and small quality factors.
Payload r | Quality factor Q | |||||
70 | 75 | 80 | 85 | 90 | 95 | |
0.1K | (0.087,0.086) | (0.091,0.092) | (0.192,0.201) | (0.284,0.291) | (0.479,0.464) | (0.654,0.662) |
0.2K | (0.095,0.093) | (0.114,0.107) | (0.190,0.181) | (0.286,0.274) | (0.457,0.461) | (0.623,0.619) |
0.3K | (0.094,0.099) | (0.104,0.117) | (0.167,0.163) | (0.243,0.252) | (0.437,0.431) | (0.581,0.597) |
0.4K | (0.085,0.083) | (0.090,0.103) | (0.150,0.144) | (0.248,0.224) | (0.407,0.389) | (0.563,0.542) |
0.5K | (0.076,0.079) | (0.084,0.091) | (0.137,0.129) | (0.226,0.224) | (0.375,0.370) | (0.503,0.489) |
In our proposed scheme, we introduce Vandermonde matrix to divide the original data into multiple shares (e.g. Figure 2), and then hide these shares in a series of video frames by combining new distortion function and STC algorithm. In this section, we analyze the robustness of proposed scheme.
With the Equation (2.4) and Figure 2, we know that m q-ary digits can be expanded to n q-ary digits by Vandermonde matrix. Obviously, n q-ary digits carry m original digits. In other words, for n expanded digits, each of them only carries mn valid original digits. Therefore, there is some redundancy in n expanded digits, which can be calculated by n−mn (referring to Equation (2.5)). As such, as long as the recipient can receive no less than m digits from n expanded digits, he can recover the m original digits. Actually, this procedure can be deduced easily from the Figure 2. For example, we assume that the recipient has received m′ shares, m≤m′≤n. He selects m ones from m′ shares, and then extract m expanded data vectors t′1, t′2, t′3, ⋯, t′m and their corresponding indices a′1, a′2, ⋯, a′m. According to Equation (2.3), a Vandermonde matrix A′ can be built according to m indices.
A′=[11⋯1a′1a′2⋯a′m(a′1)2(a′2)2⋯(a′m)2⋮⋮⋯⋮(a′1)m−1(a′2)m−1⋯(a′m)m−1]modq. | (3.3) |
We can prove that matrix A′ has an inverse matrix in q-ary notational system because it is a full-rank matrix. We omit the actual proof [11] here due to space limitations. Denote A′−1 as the inverse matrix of A′, the original data D={d1,d2,⋯,dm} can be calculated easily by the following equation.
D=[d1d2d3⋯dm]=[t′1t′2t′3⋯t′m]⋅(A′)−1 | (3.4) |
In general, the redundancy rate Re depends on the parameters m and n. It can be up very high if we set an extreme gap between m and n. Table 3 shows the relationship between redundancy rate Re and parameters m and n. As can been in this table, when the parameter m is fixed, Re will become higher with n increasing. This conclusion can be also validated theoretically by the trend of lines in Figure 6.
m | n | q | Re |
5 | 9 | 11 | 44.44% |
5 | 13 | 17 | 61.54% |
5 | 29 | 31 | 82.76% |
5 | 71 | 73 | 92.96% |
5 | 251 | 251 | 98.01% |
In this section, we compare the proposed video steganographic scheme with other state-of-the-arts. The performance comparison mainly focuses on three aspects: visual quality, robustness, and anti-steganalysis. We should raise the readers' attention that we do not use the video sequences Highway and Paris in the following experiments, because they are used in Section 3.2 to find the optimal modulation parameters. If these two video sequences are re-used, the corresponding experiments might encounter the over-fitting.
The imperceptibility is very important for video steganography. It is always required that the steganographic method should not cause severe visual quality degradation.
In our experiment, we use J-UNIWARD algorithm to calculate original distortion and then employ proposed new distortion function to further improve the original distortion. Peak signal-to-noise ratio (PSNR for short) is used to evaluate the visual quality of stego video sequences. Since the video frames are compressed format, we calculate the PSNR (dB) by comparing the uncompressed video sequence before data embedding and the decompression reconstructed video sequence after data embedding. We test all video sequences with three payloads 0.1Kbpf, 0.2Kbpf, and 0.3Kbpf. Figure 7 shows the visual quality of proposed scheme with three payloads. As can be seen in this figure, the stego frames and original frames are apparently difficult to distinguish. This demonstrates that proposed steganographic scheme has a high visual quality. Additionally, Table 4 lists the PSNR values of all video sequences by comparing three steganographic methods, Chang's method [14], Liu's method [15], and Mstafa's method [16]. The payload is fixed to 0.1K bpf. We can get the conclusion from this table that proposed scheme has a slight visual quality degradation after data hiding, but, comparison with other methods, it still has a significant superior performance.
Sequence | Cover | Chang et al. [14] | Liu et al.[15] | Mstafa et al.[16] | Proposed Scheme} |
Bus | 33.378 | 32.963 | 33.084 | 33.190 | 33.212 |
City | 34.898 | 34.657 | 34.775 | 34.782 | 34.804 |
Coastguard | 34.562 | 34.157 | 34.321 | 34.379 | 34.483 |
Crew | 37.012 | 36.792 | 36.801 | 36.887 | 36.901 |
Flower | 34.501 | 34.125 | 34.301 | 34.374 | 34.423 |
Football | 35.928 | 35.645 | 35.709 | 35.756 | 35.811 |
Foreman | 36.068 | 35.798 | 35.887 | 35.892 | 35.975 |
Harbour | 34.040 | 33.724 | 33.882 | 33.910 | 33.922 |
Ice | 39.556 | 39.084 | 39.163 | 39.192 | 39.221 |
Mobile | 33.476 | 33.003 | 33.098 | 33.104 | 33.192 |
Soccer | 35.518 | 35.241 | 35.287 | 35.296 | 35.332 |
Tempete | 34.531 | 34.302 | 34.339 | 34.387 | 34.401 |
Waterfall | 34.668 | 34.012 | 34.206 | 34.213 | 34.279 |
We further test the robustness of proposed scheme with a series of experiments. The corresponding experiments can be performed by three attack forms: usual attack, frame attack and video compression attack.
We firstly test the robustness of proposed scheme for usual attack forms. Three usual attack forms, including Salt & Peppers noise, Gaussian noise and Median filtering, are used to provide testing results. In these experiments, we set data decomposition parameter combination as (m=5, n=13, q=17), the redundancy rate is thus Re=61.54%. In other words, as long as the expanded data is lost (or damaged) no more than 61.54%, the original data can be recovered perfectly. In data embedding procedure, we evenly hide the expanded data (multiple shares) in each frame with payload 0.2K bpf. For Salt & Peppers noise attack, the noise intention is fixed I=0.01 and 0.05, and for Gaussian noise attack, we also fix the parameters V=0.01 and 0.05, while for Median filtering, the size of block is set to 3×3. We verify proposed scheme by using and un-using ensemble mechanism, where the ensemble rounds are 11 (corresponding to en=11 in Algorithm 2). Each experiment is run 100 times. Each time, we randomly extract data from the remaining (complete) frames. The results are from average calculation as the times that the original data can be recovered correctly over the total testing times. Table 5 shows the experimental results. It can be observed that, for three usual attack forms, when the ensemble mechanism is not used, the ratio that original data can be recovered perfectly is rather low, only 67% for Salt & Peppers noise with I=0.01, 63% for Gaussian noise with V=0.01 and 79% for Median filtering. This is because the noise might modify the embedding information so that the recovered original data is wrong even if they seem to be complete.
Attacking Forms | Attacking Parameters | Non-Ensemble | Ensemble |
Salt & Peppers | I=0.01 | 67% | 100% |
I=0.05 | 46% | 94% | |
Gaussian noise | V=0.01 | 63% | 98% |
V=0.05 | 42% | 92% | |
Median filtering | 3×3 block | 79% | 100% |
In addition, we also test two video frame attack forms, frame cropping and frame removal. For frame cropping, we set two cases, only one frame cropping and all frames cropping. The cropping scales are fixed 20% and 40%. For frame removal, five removal ratios are tested for all frames, 20%, 40%, 50%, 60% and 70%. The corresponding experimental results are shown in Table 6. As can be seen from this table, when the cropping for all frames is more than 40%, the data reconstruction ability for non-ensemble scheme becomes inferior. When the removal ratio is up to 70%, the original data cannot be recovered because the lost ratio has exceeded the redundancy rate Re=61.54%. Overall, proposed scheme can implement a robust recovery for the original data even if they are lost or damaged during delivery.
Attacking Forms | Attacking Parameters | Non-Ensemble | Ensemble |
Frame Cropping | 20% (One frame) | 100% | 100% |
40% (One frame) | 100% | 100% | |
20% (All frames) | 82% | 98% | |
40% (All frames) | 53% | 90% | |
Frame Removal | 20% frames | 100% | 100% |
40% frames | 100% | 100% | |
50% frames | 68% | 100% | |
60% frames | 15% | 62% | |
70% frames | 0% | 0% |
Moreover, in order to gain more insight, we also test the proposed scheme by using the H.264 video compression with different quantization parameters (QP for short). In this attack from, compression is applied to every macroblock of video frame and QP is used to control the level of compression. Table 7 presents the experimental results. We can observe from this table that the data reconstruction ability significantly becomes inferior with QP value increasing, because lower QP value maybe cause an inferior video quality, leading to a larger data modification. Also, for compression testing with different QP values, QP=10,20,30,40, we further test PSNR for three video steganographic schemes, Chang's scheme [14], Dalal's scheme [24] and proposed scheme. Three video sequences, Bus, Flower and Foreman, are used to give the experimental results, which are shown in Table 8. As can be seen that increasing of QP value results in more compression and leads to a lower video quality.
Attacking Forms | Attacking Parameters | Non-Ensemble | Ensemble |
H.264 Compression | QP=40 | 31% | 78% |
QP=30 | 44% | 84% | |
QP=20 | 52% | 95% | |
QP=10 | 73% | 100% |
Schemes | Video | Quantization parameter QP | |||
QP=10 | QP=20 | QP=30 | QP=40 | ||
Chang et al. [14] | Bus | 33.544 | 33.012 | 32.910 | 32.874 |
Flower | 34.890 | 34.576 | 34.250 | 34.011 | |
Foreman | 35.169 | 35.083 | 34.980 | 34.911 | |
Dalal et al. [24] | Bus | 33.788 | 33.654 | 33.540 | 33.217 |
Flower | 35.014 | 34.983 | 34.756 | 34.669 | |
Foreman | 36.701 | 36.542 | 36.102 | 35.818 | |
Proposed | Bus | 34.095 | 34.013 | 33.953 | 33.881 |
Flower | 35.870 | 35.704 | 35.556 | 35.231 | |
Foreman | 37.017 | 36.809 | 36.544 | 36.223 |
As a good video steganographic method, the anti-steganalysis capability is also an important focus. Since video steganographic schemes consistently hide the messages in a batch of video frame, we use three batch steganographic detection schemes (also named steganographer detection methods), hierarchical clustering scheme (HC) [25], local outlier factor detection (LOF) [26], and ensemble clustering scheme (EC) [27], to demonstrate the security performance of different video steganographic schemes against steganalysis.
Table 9 shows the comparison results between proposed scheme and three state-of-the-art schemes with three payloads r = 0.01K, 0.02K, 0.03K bpf, respectively. In this experiment, we regard each video sequence as a cluster (total 13 clusters). Each experiment, we randomly choose 7 clusters (video sequences), and select 50 frames from each one. Then, one cluster is randomly chosen as the guilty who uses three payloads mentioned above to hide messages, respectively. Each experiment is repeated 100 times and the overall identification accuracy rate is used to evaluate anti-steganalysis that is denoted by the number of correctly identification over the total testing number. From Table 9, we can observe that proposed scheme has a lower accuracy rate than that of other schemes. This illustrates that proposed scheme is more secure. In addition, we find that the EC method is conclusively more efficient comparing with HC and LOF methods. This is because EC method uses the ensemble clustering mechanism containing a number of sub-clustering, it can experimentally give a superior detection performance. Actually, this interesting phenomenon has been conclusively verified in [27].
Schemes | Payload r | HC method | LOF method | EC method |
Chang et al. [14] | 0.1K | 82% | 88% | 90% |
0.2K | 84% | 89% | 92% | |
0.3K | 88% | 94% | 97% | |
Liu et al. [15] | 0.1K | 79% | 82% | 87% |
0.2K | 82% | 90% | 91% | |
0.3K | 86% | 90% | 93% | |
Mstafa et al. [16] | 0.1K | 77% | 80% | 84% |
0.2K | 80% | 85% | 90% | |
0.3K | 85% | 86% | 89% | |
Proposed | 0.1K | 73% | 78% | 79% |
0.2K | 78% | 82% | 86% | |
0.3K | 81% | 84% | 88% |
In this paper, we proposed a robust video steganographic scheme. We first expand original data to multiple shares. This mechanism can ensure that the recipient recover the original data successfully even if they only obtain a part of data. Then, a new distortion function is designed by using continuous adjacent video frames as side-information, which can further improve the security performance of steganography. Proposed scheme is robust in the sense that the recipient can recover the hidden data even if some frames are damaged or lost during delivery. Extensive experiments are performed to show that our proposed schemes outperform existing video steganographic schemes in terms of visual quality, robustness and anti-steganalysis.
While proposed scheme has shown a good performance in the diverse tests, we should note that it has an obvious short on the utilization of video sequences because proposed scheme consistently expands the original data to multiple shares, this makes that the actual hidden data become more and more, leading to a significant cover-consuming. Nevertheless, we believe that this is only a small problem because we can easily obtain massive video sequences from the Internet. Moreover, although our method is robust in social networks, if too many shares are damaged or lost, proposed scheme will do not work.
Finally, we believe that there may be some room for further improvement. For example, the distortion function can be designed by involving more adjacent frames, although the complexity may rise sharply. In addition, we should consider to further reduce the computational complexity for inverse matrix in q-ary notation system. The above two issues are left as our future works.
This work was supported by Natural Science Foundation of China under Grants (No.61602295, No.U1736120) and Natural Science Foundation of Shanghai (No.16ZR1413100, No.18ZR1427500) and the Foreign Visiting Scholar Program of Shanghai Municipal Education Commission.
The authors declare that there is no conflict of interests regarding the publication of this article.
[1] | Carlson J, Olivas R, Gentry B, et al. (2012) United States legislative proposals on forest carbon, In: Ashton M, Tyrrell M, Spalding D, Gentry B (eds), Managing forest carbon in a changing climate, Dordrecht: Springer. |
[2] | Cheng K, Xu CH (2018) International experience in carbon finance risk regulation. J Hubei Univ Econ (Humanities and Social Sciences Edition) 15: 44-47. |
[3] | China Carbon Forum (2019) China Carbon Pricing Survey. China Carbon Forum, December 2019. Available from: http://www.chinacarbon.info |
[4] |
Du S, Tang W, Song M (2016) Low-carbon production with low-carbon premium in cap-and-trade regulation. J Clean Prod 134: 652-662. doi: 10.1016/j.jclepro.2016.01.012
![]() |
[5] | Fund A (2009) What is the Amazon Fund? Green financial products and services-current trends and future opportunities in north America: a report of the North American Task Force (NATF) of the United Nations Environment Programme Finance Initiative (2007) UNEP Finance Initiative. Available from: https://max.book118.com/html/2016/0314/37640902.shtm. |
[6] | Guo MX (2016) On the problems and the innovative countermeasures existing in the development of carbon finance in China. New Econ 2016: 67. |
[7] | Hao XM (2014) International comparative study of carbon finance system. Shanxi University. |
[8] | Hong RC (2019) The latest development of global carbon pricing mechanism and policy suggestions for the development of carbon pricing mechanism in China. Available from: https://www.huanbao-world.com/green/lsjr/108457.html. |
[9] | Kuch D (2015) Governing carbon emissions: NSW GGAS, In: The rise and fall of carbon emissions trading, Springer. |
[10] |
Lewis JI (2010) The evolving role of carbon finance in promoting renewable energy development in China. Energy Policy 38: 2875-2886. doi: 10.1016/j.enpol.2010.01.020
![]() |
[11] | Li NX, Zhao Y (2010) Some Considerations on the Low-carbon Economy. Energy Technol Econ 8: 1-3. |
[12] | Li YB, Lv RW (2019) Research Progress of China's Carbon Trading System. J Shanghai LiXin Univ Accounting Financ 2019: 42-52. |
[13] |
Liu C (2011) Low-carbon economy: theoretical study and development path choice in China. Energy Procedia 5: 487-493. doi: 10.1016/j.egypro.2011.03.085
![]() |
[14] |
Liu Z (2016) China's carbon emissions report 2016. Working Paper. doi: 10.35188/UNU-WIDER/2016/120-8
![]() |
[15] | Ottinger RL, Robinson N, Tafur V (2005) Directive 2003/87/EC of the European Parliament and of the Council of 13 October 2003 establishing a scheme for greenhouse gas emission allowance trading within the Community and amending Council Directive 96/61/EC, Cambridge University Press, 379-393. |
[16] | Principles E (2019) The equator principles: official website. |
[17] | Reich H, Wienges S (2009) Germany's international climate initiative-a bilateral fund as innovative financing mechanism, The 2009 Amsterdam Conference on the Human Dimensions of Global Environmental Change-Earth System Governance: People, Places and the Planet. |
[18] | State and Trends of Carbon Pricing (2019) The World Bank. Available from: http://documents.worldbank.org/curated/en/191801559846379845/State-and-Trends-of-Carbon-Pricing-2019. |
[19] |
Sun L, Wang M (1996) Global warming and global dioxide emission: an empirical study. J Environ Manage 46: 327-343. doi: 10.1006/jema.1996.0025
![]() |
[20] | Sun ZK (2019) Understanding and Thinking on the current development of carbon finance in China. Manage Obs 2019: 58-59. |
[21] | Wang WJ, Zhao Y (2019) Global carbon market research and its enlightenment to China's carbon market construction. Northeast Asia Forum 2019: 97-112. |
[22] |
Weng Q, Xu H (2018) A review of China's carbon trading market. Renew Sust Energ Rev 91: 613-619. doi: 10.1016/j.rser.2018.04.026
![]() |
[23] | Wu YY (2009) Carbon Finance Development and Carbon Finance Mechanism Innovation Strategy in China. Shanghai Financ 10: 26-29. |
[24] | Xiao F (2017) Heterogeneity of the function of the international carbon fund system and its implications for China. Int Bus (J Univ Int Bus Econ) 2017: 139-149. |
[25] | Yang BW (2019) The legal path of carbon finance regulation in the post-paris era from the perspective of climate finance. Int Bus Res 40: 57-70. |
[26] | Yi L, Li CP, Xu Y (2014) Research and development of carbon financial products: international experience and China's practice. J Humanit 2014: 47-54. |
[27] | Zeng G, Wan Z (2009) The Status Quo, Problems and Prospects of the International Carbon Financial Market. Stud Int Financ 10: 19-25. |
[28] |
Zeng S, Zhang S (2011) Literature review of carbon finance and low carbon economy for constructing low carbon society in China. Low Carbon Econ 2: 15. doi: 10.4236/lce.2011.21003
![]() |
[29] |
Zhang YJ (2016) Research on carbon emission trading mechanisms: current status and future possibilities. Int J Global Energy Issues 39: 89-107. doi: 10.1504/IJGEI.2016.073965
![]() |
[30] | Zhou Z (2018) The current situation and prospect of global carbon pricing mechanism. Futures Daily, 2018-08-21(3). |
1. | Mukesh Dalal, Mamta Juneja, A secure and robust video steganography scheme for covert communication in H.264/AVC, 2021, 1380-7501, 10.1007/s11042-020-10364-z |
Sequence | Resolution | Number of frames |
Bus | 352×288 | 150 |
City | 352×288 | 300 |
Coastguard | 352×288 | 300 |
Crew | 352×288 | 300 |
Flower | 352×288 | 250 |
Football | 352×288 | 260 |
Foreman | 352×288 | 300 |
Harbour | 352×288 | 300 |
Highway | 352×288 | 2000 |
Ice | 352×288 | 240 |
Mobile | 352×288 | 300 |
Paris | 352×288 | 1065 |
Soccer | 352×288 | 300 |
Tempete | 352×288 | 260 |
Waterfall | 352×288 | 260 |
Payload r | Quality factor Q | |||||
70 | 75 | 80 | 85 | 90 | 95 | |
0.1K | (0.087,0.086) | (0.091,0.092) | (0.192,0.201) | (0.284,0.291) | (0.479,0.464) | (0.654,0.662) |
0.2K | (0.095,0.093) | (0.114,0.107) | (0.190,0.181) | (0.286,0.274) | (0.457,0.461) | (0.623,0.619) |
0.3K | (0.094,0.099) | (0.104,0.117) | (0.167,0.163) | (0.243,0.252) | (0.437,0.431) | (0.581,0.597) |
0.4K | (0.085,0.083) | (0.090,0.103) | (0.150,0.144) | (0.248,0.224) | (0.407,0.389) | (0.563,0.542) |
0.5K | (0.076,0.079) | (0.084,0.091) | (0.137,0.129) | (0.226,0.224) | (0.375,0.370) | (0.503,0.489) |
m | n | q | Re |
5 | 9 | 11 | 44.44% |
5 | 13 | 17 | 61.54% |
5 | 29 | 31 | 82.76% |
5 | 71 | 73 | 92.96% |
5 | 251 | 251 | 98.01% |
Sequence | Cover | Chang et al. [14] | Liu et al.[15] | Mstafa et al.[16] | Proposed Scheme} |
Bus | 33.378 | 32.963 | 33.084 | 33.190 | 33.212 |
City | 34.898 | 34.657 | 34.775 | 34.782 | 34.804 |
Coastguard | 34.562 | 34.157 | 34.321 | 34.379 | 34.483 |
Crew | 37.012 | 36.792 | 36.801 | 36.887 | 36.901 |
Flower | 34.501 | 34.125 | 34.301 | 34.374 | 34.423 |
Football | 35.928 | 35.645 | 35.709 | 35.756 | 35.811 |
Foreman | 36.068 | 35.798 | 35.887 | 35.892 | 35.975 |
Harbour | 34.040 | 33.724 | 33.882 | 33.910 | 33.922 |
Ice | 39.556 | 39.084 | 39.163 | 39.192 | 39.221 |
Mobile | 33.476 | 33.003 | 33.098 | 33.104 | 33.192 |
Soccer | 35.518 | 35.241 | 35.287 | 35.296 | 35.332 |
Tempete | 34.531 | 34.302 | 34.339 | 34.387 | 34.401 |
Waterfall | 34.668 | 34.012 | 34.206 | 34.213 | 34.279 |
Attacking Forms | Attacking Parameters | Non-Ensemble | Ensemble |
Salt & Peppers | I=0.01 | 67% | 100% |
I=0.05 | 46% | 94% | |
Gaussian noise | V=0.01 | 63% | 98% |
V=0.05 | 42% | 92% | |
Median filtering | 3×3 block | 79% | 100% |
Attacking Forms | Attacking Parameters | Non-Ensemble | Ensemble |
Frame Cropping | 20% (One frame) | 100% | 100% |
40% (One frame) | 100% | 100% | |
20% (All frames) | 82% | 98% | |
40% (All frames) | 53% | 90% | |
Frame Removal | 20% frames | 100% | 100% |
40% frames | 100% | 100% | |
50% frames | 68% | 100% | |
60% frames | 15% | 62% | |
70% frames | 0% | 0% |
Attacking Forms | Attacking Parameters | Non-Ensemble | Ensemble |
H.264 Compression | QP=40 | 31% | 78% |
QP=30 | 44% | 84% | |
QP=20 | 52% | 95% | |
QP=10 | 73% | 100% |
Schemes | Video | Quantization parameter QP | |||
QP=10 | QP=20 | QP=30 | QP=40 | ||
Chang et al. [14] | Bus | 33.544 | 33.012 | 32.910 | 32.874 |
Flower | 34.890 | 34.576 | 34.250 | 34.011 | |
Foreman | 35.169 | 35.083 | 34.980 | 34.911 | |
Dalal et al. [24] | Bus | 33.788 | 33.654 | 33.540 | 33.217 |
Flower | 35.014 | 34.983 | 34.756 | 34.669 | |
Foreman | 36.701 | 36.542 | 36.102 | 35.818 | |
Proposed | Bus | 34.095 | 34.013 | 33.953 | 33.881 |
Flower | 35.870 | 35.704 | 35.556 | 35.231 | |
Foreman | 37.017 | 36.809 | 36.544 | 36.223 |
Schemes | Payload r | HC method | LOF method | EC method |
Chang et al. [14] | 0.1K | 82% | 88% | 90% |
0.2K | 84% | 89% | 92% | |
0.3K | 88% | 94% | 97% | |
Liu et al. [15] | 0.1K | 79% | 82% | 87% |
0.2K | 82% | 90% | 91% | |
0.3K | 86% | 90% | 93% | |
Mstafa et al. [16] | 0.1K | 77% | 80% | 84% |
0.2K | 80% | 85% | 90% | |
0.3K | 85% | 86% | 89% | |
Proposed | 0.1K | 73% | 78% | 79% |
0.2K | 78% | 82% | 86% | |
0.3K | 81% | 84% | 88% |
Sequence | Resolution | Number of frames |
Bus | 352×288 | 150 |
City | 352×288 | 300 |
Coastguard | 352×288 | 300 |
Crew | 352×288 | 300 |
Flower | 352×288 | 250 |
Football | 352×288 | 260 |
Foreman | 352×288 | 300 |
Harbour | 352×288 | 300 |
Highway | 352×288 | 2000 |
Ice | 352×288 | 240 |
Mobile | 352×288 | 300 |
Paris | 352×288 | 1065 |
Soccer | 352×288 | 300 |
Tempete | 352×288 | 260 |
Waterfall | 352×288 | 260 |
Payload r | Quality factor Q | |||||
70 | 75 | 80 | 85 | 90 | 95 | |
0.1K | (0.087,0.086) | (0.091,0.092) | (0.192,0.201) | (0.284,0.291) | (0.479,0.464) | (0.654,0.662) |
0.2K | (0.095,0.093) | (0.114,0.107) | (0.190,0.181) | (0.286,0.274) | (0.457,0.461) | (0.623,0.619) |
0.3K | (0.094,0.099) | (0.104,0.117) | (0.167,0.163) | (0.243,0.252) | (0.437,0.431) | (0.581,0.597) |
0.4K | (0.085,0.083) | (0.090,0.103) | (0.150,0.144) | (0.248,0.224) | (0.407,0.389) | (0.563,0.542) |
0.5K | (0.076,0.079) | (0.084,0.091) | (0.137,0.129) | (0.226,0.224) | (0.375,0.370) | (0.503,0.489) |
m | n | q | Re |
5 | 9 | 11 | 44.44% |
5 | 13 | 17 | 61.54% |
5 | 29 | 31 | 82.76% |
5 | 71 | 73 | 92.96% |
5 | 251 | 251 | 98.01% |
Sequence | Cover | Chang et al. [14] | Liu et al.[15] | Mstafa et al.[16] | Proposed Scheme} |
Bus | 33.378 | 32.963 | 33.084 | 33.190 | 33.212 |
City | 34.898 | 34.657 | 34.775 | 34.782 | 34.804 |
Coastguard | 34.562 | 34.157 | 34.321 | 34.379 | 34.483 |
Crew | 37.012 | 36.792 | 36.801 | 36.887 | 36.901 |
Flower | 34.501 | 34.125 | 34.301 | 34.374 | 34.423 |
Football | 35.928 | 35.645 | 35.709 | 35.756 | 35.811 |
Foreman | 36.068 | 35.798 | 35.887 | 35.892 | 35.975 |
Harbour | 34.040 | 33.724 | 33.882 | 33.910 | 33.922 |
Ice | 39.556 | 39.084 | 39.163 | 39.192 | 39.221 |
Mobile | 33.476 | 33.003 | 33.098 | 33.104 | 33.192 |
Soccer | 35.518 | 35.241 | 35.287 | 35.296 | 35.332 |
Tempete | 34.531 | 34.302 | 34.339 | 34.387 | 34.401 |
Waterfall | 34.668 | 34.012 | 34.206 | 34.213 | 34.279 |
Attacking Forms | Attacking Parameters | Non-Ensemble | Ensemble |
Salt & Peppers | I=0.01 | 67% | 100% |
I=0.05 | 46% | 94% | |
Gaussian noise | V=0.01 | 63% | 98% |
V=0.05 | 42% | 92% | |
Median filtering | 3×3 block | 79% | 100% |
Attacking Forms | Attacking Parameters | Non-Ensemble | Ensemble |
Frame Cropping | 20% (One frame) | 100% | 100% |
40% (One frame) | 100% | 100% | |
20% (All frames) | 82% | 98% | |
40% (All frames) | 53% | 90% | |
Frame Removal | 20% frames | 100% | 100% |
40% frames | 100% | 100% | |
50% frames | 68% | 100% | |
60% frames | 15% | 62% | |
70% frames | 0% | 0% |
Attacking Forms | Attacking Parameters | Non-Ensemble | Ensemble |
H.264 Compression | QP=40 | 31% | 78% |
QP=30 | 44% | 84% | |
QP=20 | 52% | 95% | |
QP=10 | 73% | 100% |
Schemes | Video | Quantization parameter QP | |||
QP=10 | QP=20 | QP=30 | QP=40 | ||
Chang et al. [14] | Bus | 33.544 | 33.012 | 32.910 | 32.874 |
Flower | 34.890 | 34.576 | 34.250 | 34.011 | |
Foreman | 35.169 | 35.083 | 34.980 | 34.911 | |
Dalal et al. [24] | Bus | 33.788 | 33.654 | 33.540 | 33.217 |
Flower | 35.014 | 34.983 | 34.756 | 34.669 | |
Foreman | 36.701 | 36.542 | 36.102 | 35.818 | |
Proposed | Bus | 34.095 | 34.013 | 33.953 | 33.881 |
Flower | 35.870 | 35.704 | 35.556 | 35.231 | |
Foreman | 37.017 | 36.809 | 36.544 | 36.223 |
Schemes | Payload r | HC method | LOF method | EC method |
Chang et al. [14] | 0.1K | 82% | 88% | 90% |
0.2K | 84% | 89% | 92% | |
0.3K | 88% | 94% | 97% | |
Liu et al. [15] | 0.1K | 79% | 82% | 87% |
0.2K | 82% | 90% | 91% | |
0.3K | 86% | 90% | 93% | |
Mstafa et al. [16] | 0.1K | 77% | 80% | 84% |
0.2K | 80% | 85% | 90% | |
0.3K | 85% | 86% | 89% | |
Proposed | 0.1K | 73% | 78% | 79% |
0.2K | 78% | 82% | 86% | |
0.3K | 81% | 84% | 88% |