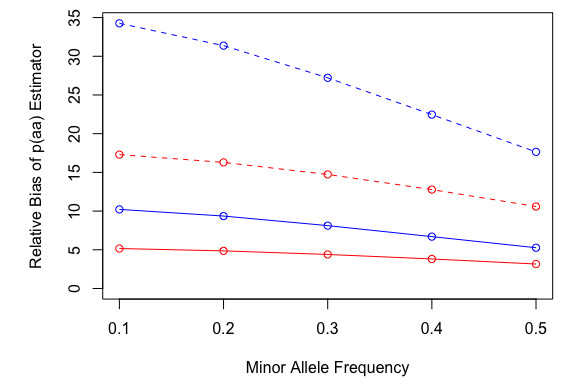
Citation: Virginia L. Ma, Shili Lin. Examining the rare disease assumption used to justify HWE testing with control samples[J]. Mathematical Biosciences and Engineering, 2020, 17(1): 73-91. doi: 10.3934/mbe.2020004
[1] | Yunxiang Zhang, Lvcheng Jin, Bo Wang, Dehong Hu, Leqiang Wang, Pan Li, Junling Zhang, Kai Han, Geng Tian, Dawei Yuan, Jialiang Yang, Wei Tan, Xiaoming Xing, Jidong Lang . DL-CNV: A deep learning method for identifying copy number variations based on next generation target sequencing. Mathematical Biosciences and Engineering, 2020, 17(1): 202-215. doi: 10.3934/mbe.2020011 |
[2] | Yong Zhou, Minrui Guo . Isolation in the control of epidemic. Mathematical Biosciences and Engineering, 2022, 19(11): 10846-10863. doi: 10.3934/mbe.2022507 |
[3] | Luju Liu, Jianhong Wu, Xiao-Qiang Zhao . The impact of migrant workers on the tuberculosis transmission: General models and a case study for China. Mathematical Biosciences and Engineering, 2012, 9(4): 785-807. doi: 10.3934/mbe.2012.9.785 |
[4] | Zakarya Noffel, Hana M. Dobrovolny . Quantifying the effect of defective viral genomes in respiratory syncytial virus infections. Mathematical Biosciences and Engineering, 2023, 20(7): 12666-12681. doi: 10.3934/mbe.2023564 |
[5] | Yi Liu, Long Cheng, Xiangyang Song, Chao Li, Jiantao Zhang, Lei Wang . A TP53-associated immune prognostic signature for the prediction of the overall survival and therapeutic responses in pancreatic cancer. Mathematical Biosciences and Engineering, 2022, 19(1): 191-208. doi: 10.3934/mbe.2022010 |
[6] | Peter Hinow, Edward A. Rietman, Sara Ibrahim Omar, Jack A. Tuszyński . Algebraic and topological indices of molecular pathway networks in human cancers. Mathematical Biosciences and Engineering, 2015, 12(6): 1289-1302. doi: 10.3934/mbe.2015.12.1289 |
[7] | Xiaodong Xia, Manman Wang, Jiao Li, Qiang Chen, Heng Jin, Xue Liang, Lijun Wang . Identification of potential genes associated with immune cell infiltration in atherosclerosis. Mathematical Biosciences and Engineering, 2021, 18(3): 2230-2242. doi: 10.3934/mbe.2021112 |
[8] | Mohammadjavad Hosseinpoor, Hamid Parvin, Samad Nejatian, Vahideh Rezaie, Karamollah Bagherifard, Abdollah Dehzangi, Amin Beheshti, Hamid Alinejad-Rokny . Proposing a novel community detection approach to identify cointeracting genomic regions. Mathematical Biosciences and Engineering, 2020, 17(3): 2193-2217. doi: 10.3934/mbe.2020117 |
[9] | Fen-fen Zhang, Zhen Jin . Effect of travel restrictions, contact tracing and vaccination on control of emerging infectious diseases: transmission of COVID-19 as a case study. Mathematical Biosciences and Engineering, 2022, 19(3): 3177-3201. doi: 10.3934/mbe.2022147 |
[10] | Durward Cator, Qimin Huang, Anirban Mondal, Martial Ndeffo-Mbah, David Gurarie . Individual-based modeling of COVID-19 transmission in college communities. Mathematical Biosciences and Engineering, 2022, 19(12): 13861-13877. doi: 10.3934/mbe.2022646 |
Genome-wide Association Studies (GWAS) are frequently performed to look for genetic variants that are associated with an array of diseases. In such studies, where hundreds of thousands, or even millions, of Single Nucleotide Polymorphisms (SNPs) are generated, the first step of data analysis is often testing for Hardy-Weinberg Equilibrium (HWE) for each of the SNPs [1,2,3]. This is usually framed as a quality control step to help identify possible errors that could have occurred, including systematic genotyping ones [3,4,5,6,7,8,9]. SNPs that do not pass the HWE tests are eliminated before moving on to the next step, as they are deemed to have violated the HWE assumption and to have likely been caused by errors [10,11,12,13,14]. To detect all possible errors, multiple testing procedures are typically not fully implemented, leading to the rejection of a large proportion of the SNPs in some studies [15,16,17,18].
In a case-control study setting, it is known that if a locus is indeed associated with the disease of interest, then HWE will be distorted in the cases [19]; therefore, researchers use controls only to test for HWE [8,20,21]. By doing so, there is an (implicit) assumption that the disease being studied is rare [18,22,23], which is based on the belief that controls under such a setting are similar to the population in terms of their genetic makeup. It is worth pointing out, though, that the rare disease assumption itself is often overlooked in practical applications. On the other hand, it has been recognized in the statistical genetics community that using controls only to test for HWE is not appropriate if the disease is common [3,22,24,25]; thus, a number of tests that use both cases and controls have been proposed [2,18,22,23,26]. Nevertheless, the assertion that, if the disease is rare, the controls may well represent the general population [3] and HWE testing based on controls only is valid [18,22,23,27], has not been seriously scrutinized or vetted.
Many complex diseases, such as hypertension, asthma, and diabetes, have a population prevalence of over 10% [28,29,30]. Although a population prevalence of 0.0005 has been used as a threshold for rare diseases [31], a less stringent threshold of 0.05 has also been used for considering complex diseases [32], which may be more appropriately referred to as uncommon or rarer than common (or simply rarer). In this article, our main simulation study used the 0.05 threshold as we aim to consider complex diseases with moderate relative risks, although we also explore the more stringent threshold of 0.0005.
Due to the lack of adequate work in the literature, the question of whether the rare disease assumption sufficiently justifies the use of controls only in HWE testing has not been settled satisfactorily. In other words, even if it has been shown that the disease is indeed rare, relying on this assumption still raises several concerns. The problem lies in the following question: are the controls actually similar to the general population? For studying maternal and imprinting effect, it was argued that the rare disease assumption is necessary but not sufficient for the premise that controls have similar genetic makeup to the general population [32]. This issue was also touched upon in a simulation study devised to investigate HWE testing [34]. If the controls are not a good representation of the population, then more variants than necessary may be thrown out since the measure of HWE will be distorted [18]. Regardless of the prevalence of the disease being studied, for a SNP that is associated with the disease, not only will HWE be distorted in cases, but it may also be distorted in the controls, even if HWE holds in the general population. Therefore, if one uses controls only to test for HWE, SNPs that are indeed associated with the disease of interest will potentially be screened out. This results in a loss of information that is entirely avoidable, warranting additional studies.
In this paper, we take up this challenge by considering two major issues that differ from those commonly addressed in the literature. First, we investigate if the rare disease assumption itself is sufficient to justify the use of controls only as surrogate for a random sample of the population in the context of HWE testing. We look at the bias incurred when estimating population genotype frequencies based on controls. Even though most of the diseases being studied today are common ones, this outstanding issue regarding the sufficiency of the rare disease assumption needs to be resolved to correct any misconceptions. Secondly, we explore whether there is an inflated Type Ⅰ error when using controls only for testing for HWE, regardless of whether the disease of interest is common or rare, under a variety of disease models. These two issues are addressed comprehensively through analytical derivations, simulation studies, and the use of the genotypes from the 1000 Genomes Project.
In this section, to set things up, we first express the distribution of the genotypes for the cases in terms of the relative risks of the genetic model and the genotypes frequencies in the general population (assumed to be in HWE) from which the cases are drawn (Subsection 2.1). From the expressions, one can easily see that the genotype frequencies in the cases are different from the corresponding frequencies in the general population, a well-known fact. The most salient point of the methodology in this contribution is contained in Subsection 2.2, in which we (a) derive the genotype distribution of the controls, (b) show that the distribution is different from that of the general population just like the cases, and (c) argue that the common notion that controls can be used as a representation of the general population under the rare disease assumption is in fact a fallacy. Taking (b) and (c) together, we further argue in this subsection that the common practice of testing for HWE using controls only may lead to tossing out valuable genetic data. The impact of issues discussed is examined in terms of estimating biases of the genotype frequencies (Subsection 2.3). Finally, in Subsection 2.4, we propose four versions of the Chi-square test to assess the impact of using a case sample or a control sample to test for HWE. Although our focus is on using the controls, we also look into the use of cases as a comparison and for completeness.
Consider a SNP with alleles a and A, whose frequencies are pa and 1−pa, respectively, with a being the minor allele, and thus, pa is referred to as the Minor Allele Frequency (MAF). Assuming that the SNP is from a population under HWE, then the probabilities of the three genotypes, AA,Aa, and aa, in the population are:
pAA=(1−pa)2,pAa=2pa(1−pa),andpaa=p2a. | (2.1) |
Now, suppose that the SNP is associated with a disease and that having one or two copies of the minor allele will lead to increased risks of being affected by the disease (i.e. becoming a case). These increased risks are above the baseline level (when there are no copies of the minor allele) and can be formally defined in terms of the following relative risks:
RR1=P(case∣Aa)P(case∣AA) and RR2=P(case∣aa)P(case∣AA). |
Then, using the Bayes rule, the distribution of the three genotypes in the population of cases can be derived in terms of the genotype distribution in the general population (pAA,pAa,paa) and the two relative risks (RR1,RR2):
pAA∣case≡P(AA∣case)=pAApAA+pAa×RR1+paa×RR2,pAa∣case≡P(Aa∣case)=pAa×RR1pAA+pAa×RR1+paa×RR2,paa∣case≡P(aa∣case)=paa×RR2pAA+pAa×RR1+paa×RR2. | (2.2) |
These probabilities have been derived many times previously in the literature, including the reproduction in Brems [34]. It is easily seen that the genotype distribution for the cases as defined in the set of equations in (2.2) is different from that of the general population as defined in (2.1). To understand their relationship more clearly, we let C=pAA+pAa×RR1+paa×RR2. Then, in order for the three corresponding probabilities to be similar, we need to have C≈1,RR1/C≈1, and RR2/C≈1. These requirements lead to the conclusion that both RR1≈1 and RR2≈1. However, for a SNP that is associated with the disease, these requirements cannot be satisfied. In other words, the cases are not similar to the general population in their genetic makeup. Hence, it has been correctly concluded by researchers that using cases for testing HWE will lead to inflated type Ⅰ error.
Similarly, one can derive the distribution of the three genotypes within the population of controls, noting that the population prevalence of the disease is also needed to completely specify them:
pAA∣cntr≡P(AA∣control)=pAA−pAA∣case×κ1−κ,pAa∣cntr≡P(Aa∣control)=pAa−pAa∣case×κ1−κ,paa∣cntr≡P(aa∣control)=paa−paa∣case×κ1−κ, | (2.3) |
where κ≡P(case) is the population disease prevalence; that is, the probability that a randomly chosen individual from the general population is affected by the disease. Expressing it in terms of the relative risks, we have κ=P(case∣AA)[PAA+PAa×RR1+Paa×RR2]. Then one can find the ratio of the probabilities of each genotype in the controls relative to the general population:
pAA∣cntrPAA=1−P(case∣AA)1−(PAA+PAa×RR1+Paa×RR2)×P(case∣AA)>1,pAa∣cntrPAa=1−RR1×P(case∣AA)1−(PAA+PAa×RR1+Paa×RR2)×P(case∣AA),paa∣cntrPaa=1−RR2×P(case∣AA)1−(PAA+PAa×RR1+Paa×RR2)×P(case∣AA)<1. | (2.4) |
Note that the two inequalities above are strict as long as there is a phenocopy rate (i.e. P(case∣AA)>0), indicating that the aa genotype in the controls is decreased whereas there is an increase in the AA genotypes. This observation holds true for a fixed diseased model and population characteristics, which together determine the degrees of depletion and influx.
One can also easily see that, just like the three case probabilities, the three control probabilities in (2.3) also differ from their corresponding population probabilities in (2.1). In order for the two distributions to be approximately the same, we would require that
pAA−pAA∣case×κ≈(1−κ)×pAA, |
pAa−pAa∣case×κ≈(1−κ)×pAa, |
paa−paa∣case×κ≈(1−κ)×paa. |
Simple algebraic manipulations show that these conditions are equivalent to requiring that the genotype distribution for the cases be approximately the same as the genotype distribution for the general population. It is the interplay between disease allele frequency and the underlying disease model - which together specify the disease prevalence - that determines whether the genotype frequencies under the controls are similar to the corresponding frequencies in the general population. As such, regardless of whether the disease is rare or common, the genotype distribution of the controls may not be similar to the distribution of the general population, depending on the characteristics of the genetic variant and the disease model. Therefore, the commonly invoked "rare disease assumption" is not grounded in theoretical analysis; rather, it appears to be a commonly-held misconception.
To further examine this notion that controls as a whole can be used as a representation of the general population and to show that it is in fact a fallacy, we rewrite the genotype distribution of the controls using a slightly different representation based on the Bayes rule:
P(AA∣control)=[P(control∣AA)pAA]/(1−κ), |
P(Aa∣control)=[P(control∣Aa)pAa]/(1−κ), |
P(aa∣control)=[P(control∣aa)paa]/(1−κ). |
In order for these probabilities to be close to their population counterparts, it is required that
P(control∣Genotype)≈1−κ, |
where Genotype can be AA, Aa, or aa. If a disease is rare, then 1−κ≈1, which implies that P(control∣Genotype) would all have to be close to 1. Clearly, this is not possible if the disease is Mendelian. For example, for a recessive disorder with complete penetrance, P(control∣aa)=0, which is not close to 1. In general, for a SNP associated with the disease, the requirements stated above may not be always satisfied, and the rare disease assumption by itself is not sufficient to guarantee the similarity of the genotype distributions of the controls and the general population, echoing an earlier comment [32]. Therefore, using controls only to test for HWE may be equally as invalid as using cases in many situations. While the latter is a known fact and thus the practice is avoided, the former has not been previously pointed out, and testing for HWE in controls regardless of the disease prevalence is still commonly done in genetic studies. This is a practice that can lead to valuable data tossed out due to distorted HWE in the controls.
We can ascertain the expected degree of deviation when using controls to estimate the genotype frequencies of a population in HWE by calculating the biases and relative biases. For example, the bias for estimating the frequency of aa is,
Biasaa=E[naa∣cntrncntr]−paa=paa∣cntr−paa, |
where ncntr is the number of controls in the sample while naa∣cntr is the number of controls having the aa genotype. Clearly, this bias is not zero based on formulas (2.1) and (2.3) for the population and control frequencies, respectively, and therefore the estimator is biased. Since paa can be quite small, especially for variants with a small MAF, we will consider the absolute relative bias as defined in the first expression in the following:
ARbiasaa=|paa∣cntr−paa|paa=κ|paa−paa∣case|(1−κ)paa. | (2.5) |
To understand the fact that ARbiasaa does not always vanish with a rare disease, we rewrite it as the second expression in (2.5). For a rare disease, κ≈0 and therefore 1−κ≈1. However, note that κ is related to paa, which is usually very small for a rare disease. Suppose paa and κ are of the same order of magnitude; then, ARbiasaa is in the order of |paa−paa∣case|, which can be large, especially for a disease with a high penetrance (large RR2 value). Even for a disease with a moderate penetrance, the relative bias may still be appreciable. For instance, suppose κ=0.05,pa=0.2,RR1=1, and RR2=3; then, κ and paa are of the same degree of magnitude (both are in the order of 10−2). In this case, there is still an appreciable relative bias – the frequency of the aa genotype in the controls decreases by more than 9%. Formulas for calculating the biases and absolute relative biases for the other two genotypes can be similarly derived.
We performed the Chi-square test for HWE to illustrate the potential inflation of type Ⅰ error empirically. The underlying general population is assumed to be under HWE; therefore, a rejection of the null hypothesis H0 that the SNP marker is in HWE is treated as committing a type Ⅰ error. Let nAA,nAa, and naa be the number of individuals with genotypes AA,Aa, and aa, respectively, in a sample with a total size of n=nAA+nAa+naa. Then under the assumption of HWE, the corresponding numbers of expected counts for these three genotypes may be calculated in two ways, depending on whether the underlying population allele frequencies pa and pA=1−pa are known or estimated as ˆpa=(2naa+nAa)/(2n) and ˆpA=1−ˆpa. The former is a rather unrealistic (U) scenario, whereas the latter is realistic (R) and typically done. Nevertheless, we include the U scenario in our study for comparison purposes. These two sets of expected counts for the three genotypes are
(U):ˆnAA=np2A,ˆnAa=2npApa,ˆnaa=np2a; |
(R):ˆnAA=nˆp2A,ˆnAa=2nˆpAˆpa,ˆnaa=nˆp2a. |
Then, the Chi-square test statistic is
T=∑G∈{AA,Aa,aa}(nG−ˆnG)2ˆnG, | (2.6) |
regardless of which scenario is being considered. However, under U, the test statistic has 2 degrees of freedom since the known probabilities are used; whereas, under R, there is only 1 degree of freedom because the probabilities are now estimated based on the sample being tested. For finite samples as in our situation, the Chi-square approximation is reasonable as long as the expected count for each cell is at least 5.
To be comprehensive, we consider several versions of the Chi-square test. Our first two tests (T1 and T2) are for data from the cases. As well documented in the literature, it is expected that a HWE test based on the cases will lead to an inflation of the type Ⅰ error rate. As such, these two tests are used merely as a confirmatory investigation for completeness. The last two tests (T3 and T4) are for data from the controls. This setting is the focus of our study, as we are interested in addressing the question that we have raised: is it justifiable to test for HWE using controls only, especially when the disease is rare?
These four versions of the test are elaborated as follows:
T1. Data are generated under the genotype distribution for cases. Chi-square test is performed assuming that the expected counts for the three genotypes are calculated using known population frequencies from the underlying population under H0 (the U scenario).
T2. Data are generated under the genotype distribution for cases. Chi-square test is performed assuming that the expected counts for the three genotypes are estimated from the data under H0 (the R scenario).
T3. Data are generated under the genotype distribution for controls. Chi-square test is performed assuming that the expected counts for the three genotypes are calculated using known population frequencies from the underlying population under H0 (the U scenario).
T4. Data are generated under the genotype distribution for controls. Chi-square test is performed assuming that the expected counts for the three genotypes are estimated from the data under H0 (the R scenario).
To illustrate that controls may not be representative of the general population in terms of the genetic makeup, we computed the relative bias of using the genotype probabilities from the controls as estimates of the corresponding population frequencies. We considered a recessive disease model in which only those with two copies of the minor allele have an elevated risk over the baseline; that is, RR1=1 and RR2>1. The relative bias was computed over a range of minor allele frequencies: MAF = 0.1, 0.2, 0.3, 0.4, and 0.5. We studied two values for RR2: 2 and 3. Furthermore, we entertained two scenarios of disease prevalence: 0.15 and 0.05; these two levels of prevalence are chosen as the former is typically regarded as a common disease whereas the latter is treated as rarer [32]. For ease of comparison and visualization of the results, the absolute relative biases for all settings studied are plotted in Figure 1. As we can see from the figure, regardless of the combination of the parameters, using controls to estimate the general population will result in bias. The degree of relative bias does depend on the underlying setting. For a disease with a larger effect size (RR2=3), the relative bias is larger than the corresponding counterpart with a smaller effect size (RR2=2). In addition, a more common disease (κ=0.15) will also lead to greater relative bias compared to a rarer disease (κ=0.05). Finally, as the minor allele frequency gets larger toward a more common SNP, the relative bias decreases.
We consider four sets of genetic models, which differ in their relative risks with one or two copies of the minor alleles --- recessive: RR1=1,RR2>1; dominant: RR1=RR2>1; multiplicative: RR2=RR21; and additive: RR1=(1+RR2)/2. Under each model, multiple scenarios are considered; full details of the parameter values are given in Table 1, which includes the MAF values, pa, as well as the population disease prevalence, κ. In particular, we considered two disease prevalences, κ=0.15 and κ=0.05. We also varied pa at two levels, 0.1 and 0.3, although not all combinations of κ and pa are considered. For each setting under a model, we simulated data for 10,000 cases and 10,000 controls according to the distributions of probabilities provided in equation sets (2.2) and (2.3), respectively, which assume that the underlying general population is in HWE. Tests T1 and T2 were then applied to the case data, while tests T3 and T4 were applied to the control data. This process was repeated for 10,000 simulated data sets.
Model | Parameter Setting | κ | pa | RR1 | RR2 |
Recessive | R1 | 0.15 | 0.3 | 1 | 1.4 |
R2 | 0.05 | 0.1 | 1 | 4 | |
R3 | 0.15 | 0.1 | 1 | 2 | |
Dominant | D1 | 0.15 | 0.3 | 1.4 | 1.4 |
D2 | 0.15 | 0.1 | 2 | 2 | |
D3 | 0.15 | 0.3 | 2 | 2 | |
Multiplicative | M1 | 0.15 | 0.1 | 1.8 | 3.2 |
M2 | 0.15 | 0.1 | 1.6 | 2.6 | |
M3 | 0.15 | 0.3 | 1.8 | 3.2 | |
Additive | A1 | 0.15 | 0.3 | 1.1 | 1.2 |
A2 | 0.05 | 0.1 | 2 | 3 | |
A3 | 0.15 | 0.1 | 2 | 3 | |
*For each of the four types of models, κ denotes the population prevalence; pa is the minor allele frequency; RR1 and RR2 are the relative risks with one or two copies of the minor alleles, respectively, over the baseline disease risk of having no copy of the minor allele. Note that dominant and multiplicative models are less likely to be associated with a rare disease and therefore κ = 0.05 was not investigated for these two models. |
It has been shown in the literature that testing for HWE for a SNP associated with a disease using the cases will lead to an inflation of type Ⅰ error. Nevertheless, we included tests T1 and T2 for confirmatory purposes and as a comparison with results from T3 and T4 to ascertain the degree of discrepancy. Figure 2 shows the QQ plots of the quantiles of the p-values (over 10,000 simulated data sets) against the quantiles of the uniform(0, 1) distribution for the recessive model R1 in the −log10 scale. Not surprisingly, the results from T1 and T2 (tests using the cases; Figure 2(a) and (b)) have severely inflated type Ⅰ error, as both curves are far above the 95% confidence bands (portrayed by the pair of dashed lines). On the other hand, since the data were all simulated under the null hypothesis (H0: HWE), one would expect the p-values to follow a uniform distribution when tests T3 and T4 are used, if it is indeed justifiable to use the controls to test for HWE. However, as can be seen from the curves in Figures 2(c) and (d), there is also severe inflation of type Ⅰ error when testing for HWE using controls only, although the degree of severity is less than when testing with cases. These results cast great doubts on the common practice in the scientific community of using controls to test for HWE.
In the other two recessive models, R2 and R3, the inflation of type Ⅰ error is also clearly seen when testing using the controls, as shown in Supplementary Figures S1 and S2. Note that model R2 has κ=0.05, a rarer disease scenario than when κ=0.15 (R1 and R3), but the inflation of type Ⅰ error is still clearly seen, demonstrating the fallacy of the rare disease assumption. These observations also apply to the dominant and multiplicative models, which are shown in Supplementary Figures S3 - S8.
Finally, for additive model A1, the inflation of type Ⅰ error is still clearly seen when the expected genotype frequencies in the Chi-square test are calculated using the population probabilities; that is, when either T1 or T3 are applied (Supplementary Figure S9(a) and (c)). When the expected frequencies are estimated, either based on the cases or the controls, then the QQ plot curve is almost entirely within the 95% confidence band. On the other hand, for the other two additive models, using the cases and estimated frequencies will lead to inflation of type Ⅰ error, whereas using the controls and estimated frequencies will not (Supplementary Figures S10 and S11).
To better understand the degree of severity in discarding valuable data, we also summarize the p-values for all models, each over 10,000 replicates, using boxplots. If the use of controls is justifiable, then one would expect only 5% of the p-values to be smaller than 0.05 (or equivalently, greater than 1.3 on the −log10 scale). Shown in Figure 3 are the boxplots of the p-values in the -log10 scale. For the results from T3 (using population frequencies to estimate expected counts; Figure 3(a)), we can see that, among all 12 models (4 types and 3 scenarios of each) considered, at least 20% of the tests have p-values less than 0.05, far exceeding the expected 5% if the tests based on controls were appropriate. In fact, for the multiplicative models, as one can see from Figure 3, the -log10(p-values) for all the three M models are above the solid line marking the -log10 transformation of the 0.05, indicating that all p-values are smaller than 0.05. As such, had this been a real data analysis, none of the SNPs would have passed the HWE test and gone on to be tested for their association with the disease. This scenario is perhaps extreme (and some form of multiple testing procedure would likely be implemented), but is used to illustrate how much perfectly fine and valuable data could be erroneously tossed out by carrying out the usual HWE test in current practice.
For the results based on T4 (Figure 3(b)), we observed that the inflation of type Ⅰ error is less severe compared to when the expected genotype frequencies are calculated from the population parameters. These results are not entirely surprising. Under T4, the genotype frequencies and allele frequencies (which are then used to form expected genotype frequencies under HWE) are estimated based on the same dataset; therefore, they are closer than when only the genotype frequencies are estimated while the expected frequencies are calculated using the theoretical values as in T3. This fact is reflected in the adjustment for degrees of freedom (df) (df = 1 in T4 versus df = 2 in T3), but this theoretically correct adjustment for T4 may still not be sufficient in this situation, leading to T4 having fewer rejections compared to T3. Nevertheless, other than the three additive models, at least 20% of the tests still have p-values less than 0.05 for the rest of the 9 models under T4.
To further validate our results in a real data context, we used the genotype data of 697 unrelated individuals for 24,487 SNPs from the 1000 Genomes Project as described in the Genetic Analysis Workshop 17 [33]. By using the real genotype data across the genome, we preserve the linkage disequilibrium (LD) structure among the SNPs in a genome-wide analysis. For this study, we only considered common variants with minor allele frequencies of at least 0.1, due to the relatively small sample size, leading to a total of 2,209 SNPs. This selection criterion was placed for consideration of the validity of the aysmptotic Chi-square distribution of the test statistic defined in (2.6), as we would like to have sufficiently large expected counts. As such, even though it is also of interest to consider variants with frequencies smaller than 0.1, the limited sample size of 697 renders the analysis of rare variants impractical. For the remaining set of 2,209 SNPs, we carried out the HWE test using the Chi-square statistic (T4 test). Using the Bonferroni criterion to adjust for multiple testing, we obtained a total of 1,264 SNPs that are deemed to be in HWE. The 1000 Genomes data for each of the retained SNPs was then treated as constituting a population under HWE.
After these preliminary filtering steps to ensure that our population is under HWE and our sample size is sufficiently large to ensure the appropriateness of the Chi-square test, we proceeded to devise a study to investigate whether testing based on controls only for HWE, especially for rarer diseases, would lead to discarding of valuable SNPs for real genotype data.
Based on each of the four disease models, all with a population prevalence of κ=0.05 as specified in Table 2 (the same four types of disease models as in our earlier simulation studies), we divided the "population" of 697 people from the real 1000 Genomes SNP data into "cases" and "controls" according to their respective probabilities. Specifically, for each SNP and each of the four disease models given in Table 2, we calculated the probability that each individual is a case (case probability) according to his/her genotype at the SNP. A random number is then drawn to determine whether the individual is a case/control depending on the case probability/complement of the case probability. Then, we proceeded to test for HWE using the controls only for each of the SNPs. In order to better visualize our results, we ran ten replicates of HWE testing on the 1,264 SNPs through setting different random seeds for each run.
Inflation ratio with nominal level | ||||
Model | RR1 | RR2 | p=0.05 | p=0.01 |
Recessive | 1 | 1.4 | 10.3 ± 0.206 | 34.4 ± 0.657 |
Dominant | 1.4 | 1.4 | 10.6 ± 0.213 | 35.3 ± 0.804 |
Multiplicative | 1.3 | 1.7 | 11.3 ± 0.179 | 38.0 ± 1.14 |
Additive | 1.2 | 1.4 | 11.0 ± 0.190 | 36.7 ± 1.27 |
∗All four models have a population prevalence of 0.05, reflecting a rarer disease. For each of the four types of models, RR1 and RR2 are the relative risks with one or two copies of the minor alleles, respectively, over the baseline disease risk of having no copy of the minor allele. Each of the entries in the fourth and fifth columns shows the mean and standard deviation of the ratios over 10 replicates. |
Figure 4 shows that, for each of the four models specified in Table 2 that realistically reflect moderate risks for relatively rarer diseases, testing for HWE on controls leads to the rejection of HWE for a much larger number of SNPs than one would expect, contrary to common believe that usual HWE testing procedure can work well for rare diseases. Specifically, we can see from the plots that, although all SNPs were under HWE in the population, using the controls only to test for HWE has resulted in many SNPs that are now deemed to be in violation of HWE for all four types of models considered. This conclusion is reached despite the use of a very conservative genome-wide threshold of 0.05/22,090 even though only 12,630 (1,263 x 10) tests were actually performed. Using this more stringent threshold (derived based on the SNP set of 2,209 and marked by the horizontal genome-wide line in each of the plots), we can see that there are many SNPs that have reached the level of genome-wide significance among the controls while the same threshold failed to be reached with all individuals in the population. In other words, the SNPs exceeding the genome-wide significance threshold when only controls were tested have smaller p-values than the corresponding ones when the entire population was tested for HWE. In fact, we do not expect any SNPs to be above the horizontal red line marking genome-wide significance in the -log10 scale. However, the fact that we see many of them regardless of the underlying model is indicative of the severely inflated type Ⅰ error rate. If this were a real analysis, then the SNPs above the red lines, which were all under HWE in the population, would have been tossed out erroneously, resulting in loss of valuable data and loss of power of discovering variants that are associated with the disease.
The above observation is further dissected numerically in Table 2, where the last two columns show the inflation ratios of observed over expected number of p-values less than nominal values of 0.05 and 0.01, respectively. Together with the mean ratio over 10 replicates, the standard deviation of the ratios is also provided in the table, showing a great deal of consistency from replicate to replicate across all disease models considered. These results once again substantiate our observation that many SNPs (many more times than one would expect by chance) that are in HWE in the general population would be tossed out erroneously for failing the HWE test based only on the controls, even when the disease is rare. For example, for the recessive model with moderate relative risks, we would expect only about 13 SNPs to have been declared to violate the HWE with a nominal p-value of 0.01, but we, in fact, observed an average of over 450 SNPs.
It is common-practice to test for Hardy-Weinberg Equilibrium on controls in a genome-wide association study with a case-control design as a quality control step to detect genotyping and other errors. However, this practice has been called into question when the disease being studied is common, and, as a consequence, numerous methods have been proposed to remedy this (see [3] and references therein). On the other hand, the use of controls for testing for HWE has gotten multiple "seals of approval" for rare diseases; yet, its appropriateness is rarely discussed other than the assertion that controls would be similar to the general population. As such, its validity has not been seriously scrutinized and challenged in general. Although Brems [34] discussed potential problems of HWE testing when using controls even when the disease is rare, the study was limited in scope as the author only considered the setting in which the expected genotype frequencies were calculated using the known population allele frequency, which deviates from the usual practical usage. Further, bias was never discussed; and, thus, the author did not weigh in on the notion that controls as a whole may not be a good representation of the population.
In this article, a much more comprehensive study than Brems [34] was carried out. We used theoretical analysis of the bias when using genotype estimators based on controls for a general population in HWE to show that the assertion of controls being "similar" to the general population in terms of genetic makeup is in fact a myth rather than the truth, regardless of whether the disease is common or rare. In fact, the bias and relative bias are not only controlled by the disease prevalence, but are also dependent on the disease gene frequency and the relative risks of the disease model; note that these quantities are inter-dependent. Although the relative bias is seen in Figure 1 to have a larger magnitude for a common disease (with prevalence of 0.15) than for a rarer disease (with prevalence of 0.05) with the same relative risks, the bias is obviously apparent for both scenarios. In this theoretical investigation of bias and the simulation study, we used a prevalence of 0.05 to represent a rarer disease, but it appears to be larger than what one might consider to be rare. As a brief illustration to show that bias may still exist for much rarer diseases, we further consider a recessive model in which the disease prevalence is 0.003, which is commensurate with the incidence rate of cancer as provided by the National Cancer Institute and numerous studies [35,36]. For rarer diseases, the disease gene frequency also tends to be smaller, and we can see that the relative bias is particularly severe for the disease gene frequency of 0.05 (Supplementary Figure S12).
Armed with the evidence that controls may not truly represent the general population, we carried out a simulation study to investigate the degree of inflated type Ⅰ error when controls are used to test for HWE. Using a variety of disease models and parameter settings, we show that there is frequently severe inflation of type Ⅰ error, regardless of whether known population frequencies or estimated frequencies based on controls are used as the expected in the Chi-square test. It is seen that the inflation of type Ⅰ error may be smaller for a rarer disease than a more common one when the relative risks are the same, but even for the former, HWE testing in the disease-free controls may still lead to severe inflation of type Ⅰ error. To illustrate the theoretical results in Subsection 2.2 that the inflation of type Ⅰ error does not vanish with a rare Mendelian disease with high relative risks, we also carried out a simulation considering a recessive model with prevalence of 0.0005, which is widely accepted as a definition for a rare disease [31]. The results show that there remain severely inflated type Ⅰ error rates for both the T3 and the T4 tests (Supplementary Figure S13).
To further corroborate the observations seen in the simulation study, we carried out an additional analysis using the 1000 Genomes data. We created populations that are in HWE for over 1,200 SNPs. Then, based on several disease models with realistically moderate relative risks for rare diseases, we divided a population in HWE into cases and controls, and tested for HWE using the controls only. Once again, we observed severely inflated type Ⅰ error rates, with inflation ratios as high as more than 38. These results, based on the real genotype data, substantiate the concern that a considerable amount of valuable data would be discarded erroneously, even if the disease is rare, if the practice of testing on controls only continues. Instead, if the prevalence of a disease is known, for simplicity, we would echo the suggestion in the literature that a mixture of cases and controls, with the appropriate mixing proportions based on the population prevalence, be used to test for HWE to avoid bias and to maximize the usage of available data. This suggested procedure is in fact the inspiration for our 1000 Genomes analysis, where the rationale was reversed: we divided the population in HWE into cases and controls according to the disease model. However, we note that information contained in the disease model (including the disease gene frequency) can be used to determine the disease prevalence, whereas the converse is not true. Therefore, additional studies are needed to evaluate this suggested procedure, although it is not within the scope of the current study.
The authors would like to thank the Editor and two anonymous reviewers for their constructive comments, which we believe have led to improved and clearer presentation of the material. This work was supported in part by the National Science Foundation grant DMS-1208968. Preparation of the Genetic Analysis Workshop 17 Simulated Exome Data Set using sequencing data from the 1000 Genomes Project (www.1000genomes.org) was supported in part by NIH R01 MH059490.
The authors declare there is no conflicts of interest.
[1] | J. Wittke-Thompson, A. Pluzhnikov and N. Cox, Rational inferences about departures from HardyWeinberg equilibrium, Am. J. Hum. Genet., 76 (2005), 967-986. |
[2] | C. Yu, S. Zhang, C. Zhou, et al., A Likelihood Ratio Test of Population Hardy-Weinberg Equilibrium for Case-Control Studies, Genet. Epidemiol., 33 (2009), 275-280. |
[3] | J. Wang and S. Shete, Testing Departure from Hardy-Weinberg Proportions, in Statistical Human Genetics: Methods and Protocols, 2nd Edition (ed. Elston, RC), vol. 1666 of Methods in Molecular Biology, Humana Press, 2017, 83-115. |
[4] | I. Gomes, A. Collins, C. Lonjou, et al., Hardy-Weinberg quality control, Ann. Hum. Genet., 63 (1999), 535-538. |
[5] | S. Weiss, E. Silverman and L. Palmer, Case-control association studies in pharmacogenetics, Pharmacogenomics J., 1 (2001), 157-158. |
[6] | J. Xu, A. Turner, J. Little, et al., Positive results in association studies are associated with departure from Hardy-Weinberg equilibrium: hint for genotyping error? Hum. Genet., 111 (2002), 573-574. |
[7] | L. Hosking, S. Lumsden, K. Lewis, et al., Detection of genotyping errors by Hardy-Weinberg equilibrium testing, Eur. J. Hum. Genet., 12 (2004), 395-399. |
[8] | G. Salanti, G. Amountza, E. Ntzani, et al., Hardy-Weinberg equilibrium in genetic association studies: an empirical evaluation of reporting, deviations, and power, Eur. J. Hum. Genet., 13 (2005), 840-848. |
[9] | R. Moonesinghe, A. Yesupriya, M.-h. Chang, et al., A Hardy-Weinberg Equilibrium Test for Analyzing Population Genetic Surveys With Complex Sample Designs, Am. J. Epidemiol., 171 (2010), 932-941. |
[10] | S. Leal, Detection of genotyping errors and pseudo-SNPs via deviations from Hardy-Weinberg equilibrium, Genet. Epidemiol., 29 (2005), 204-214. |
[11] | D. Cox and P. Kraft, Quantification of the power of Hardy-Weinberg equilibrium testing to detect genotyping error, Hum. Hered., 61 (2006), 10-14. |
[12] | Y. Y. Teo, A. E. Fry, T. G. Clark, et al., On the Usage of HWE for Identifying Genotyping Errors, Ann. Hum. Genet., 71 (2007), 701-703. |
[13] | G. Y. Zou and A. Donner, The merits of testing Hardy-Weinberg equilibrium in the analysis of unmatched case-control data: A cautionary note, Ann. Hum. Genet., 70 (2006), 923-933. |
[14] | M. I. McCarthy, G. R. Abecasis, L. R. Cardon, et al., Genome-wide association studies for complex traits: consensus, uncertainty and challenges, Nat. Rev. Genet., 9 (2008), 356-369. |
[15] | C. Healey, A. Dunning, M. Teare, et al., A common variant in BRCA2 is associated with both breast cancer risk and prenatal viability, Nat. Genet., 26 (2000), 362-364. |
[16] | P. R. Burton, D. G. Clayton, L. R. Cardon, et al., Genome-wide association study of 14,000 cases of seven common diseases and 3,000 shared controls, Nature, 447 (2007), 661-678. |
[17] | J. A. Phillips III, J. S. Poling, C. A. Phillips, et al., Synergistic heterozygosity for TGF beta 1 SNPs and BMPR2 mutations modulates the age at diagnosis and penetrance of familial pulmonary arterial hypertension, Genet. Med., 10 (2008), 359-365. |
[18] | J. Wang and S. Shete, Using Both Cases and Controls for Testing Hardy-Weinberg Proportions in a Genetic Association Study, Hum. Hered., 69 (2010), 212-218. |
[19] | D. Nielsen, M. Ehm and B. Weir, Detecting marker-disease association by testing for HardyWeinberg disequilibrium at a marker locus, Am J Hum Genet., 63 (1998), 1531-1540. |
[20] | J. Graffelman and B. S. Weir, Testing for Hardy-Weinberg equilibrium at biallelic genetic markers on the X chromosome, Heredity, 116 (2016), 558-568. |
[21] | C. C. Reyes-Gibby, J. Wang, S.-C. J. Yeung, et al., Genome-wide association study identifies genes associated with neuropathy in patients with head and neck cancer, Sci. Rep., 8. |
[22] | M. Li and C. Li, Assessing Departure from Hardy-Weinberg Equilibrium in the Presence of Disease Association, Genet. Epidemiol., 32 (2008), 589-599. |
[23] | J. Wang and S. Shete, Testing Hardy-Weinberg Proportions in a Frequency-Matched Case-Control Genetic Association Study, PLoS One, 6. |
[24] | N. Chatterjee, Y.-H. Chen, S. Luo, et al., Analysis of Case-Control Association Studies: SNPs, Imputation and Haplotypes, Stat. Sci., 24 (2009), 489-502. |
[25] | J. Wang, R. Yu and S. Shete, X-Chromosome Genetic Association Test Accounting for XInactivation, Skewed X-Inactivation, and Escape from X-Inactivation, Genet. Epidemiol., 38 (2014), 483-493. |
[26] | Y. Zhang and Y. Yuan, A Shrinkage Method for Testing the Hardy-Weinberg Equilibrium in CaseControl Studies, Genet. Epidemiol., 37 (2013), 743-750. |
[27] | M. Epstein and G. Satten, Inference on haplotype effects in case-control studies using unphased genotype data, Am. J. Hum. Genet., 73 (2003), 1316-1329. |
[28] | D. G. Torgerson, E. J. Ampleford, G. Y. Chiu, et al., Meta-analysis of genome-wide association studies of asthma in ethnically diverse North American populations, Nat. Genet., 43 (2011), 887-892. |
[29] | P. K. Whelton, J. He and P. Muntner, Prevalence, awareness, treatment and control of hypertension in North America, North Africa and Asia, J. Hum. Hypertens., 18 (2004), 545-551. |
[30] | S. Wild, G. Roglic, A. Green, et al., Global Prevalence of Diabetes, Am. Diabetes Assoc. Diabetes Care., 27 (2004), 1047-1053. |
[31] | T. Richter, S. Nestler-Parr, R. Babela, et al., Rare Disease Terminology and Definitions-A Systematic Global Review: Report of the ISPOR Rare Disease Special Interest Group, Value in Health, 18 (2015), 906-914. |
[32] | J. Yang and S. Lin, Robust Partial Likelihood Approach for Detecting Imprinting and Maternal Effects Using Case-Control Families, Ann. Appl. Stat., 7 (2013), 249-268. |
[33] | A. Ziegler, S. Ghosh, T. D. Dyer, et al., Introduction to genetic analysis workshop 17 summaries, Genet. Epidemiol., 35 (2011), S1-S4. |
[34] | M. W. Brems, The Rare Disease Assumption: The Good, The Bad, and The Ugly, Master's thesis, The Ohio State University, 2015. |
[35] |
L. A. Torre, R. L. Siegel, E. M. Ward, et al., Global Cancer Incidence and Mortality Rates and Trends-An Update, Cancer Epidemiol. Biomark. Prev., 25 (2016), 16-27. doi: 10.1158/1055-9965.EPI-15-0578
![]() |
[36] | National Cancer Institute, Cancer Statistics, Natl Cancer Inst., 2019. Available from: https://www.cancer.gov/about-cancer/understanding/statistics. |
1. | Wen-li Zeng, Shi-kun Yang, Na Song, Fen-fen Chu, The impact of angiotensin converting enzyme insertion/deletion gene polymorphism on diabetic kidney disease: A debatable issue, 2022, 42, 02116995, 415, 10.1016/j.nefro.2021.07.008 | |
2. | Wen-li Zeng, Shi-kun Yang, Na Song, Fen-fen Chu, The impact of angiotensin converting enzyme insertion/deletion gene polymorphism on diabetic kidney disease: A debatable issue, 2022, 42, 20132514, 415, 10.1016/j.nefroe.2022.09.004 | |
3. | Awad Elsid Osman, Sahar Alharbi, Atif Ali Ahmed, Asim Ali Elbagir, Single nucleotide polymorphism within chromosome 8q24 is associated with prostate cancer development in Saudi Arabia, 2022, 22143882, 10.1016/j.ajur.2022.03.012 | |
4. | Hendri SUSILO, Budi S. PIKIR, Nathanael J. TJIPTA, Mochamad Y. ALSAGAFF, Mochammad THAHA, Satriyo D. SURYANTORO, Citrawati D.K. WUNGU, Mochamad AMIN, Derren D. RAMPENGAN, Roy N. RAMADHAN, Platon PAPAGEORGIOU, Ryan GAUCI, Rebeka TÓTHOVÁ, Analysis of angiotensin II type 1 receptor and osteoprotegerin gene polymorphism on the risk of cardiovascular mortality risk and progressivity of chronic kidney disease, 2024, 115, 00264806, 10.23736/S0026-4806.24.09435-7 |
Model | Parameter Setting | κ | pa | RR1 | RR2 |
Recessive | R1 | 0.15 | 0.3 | 1 | 1.4 |
R2 | 0.05 | 0.1 | 1 | 4 | |
R3 | 0.15 | 0.1 | 1 | 2 | |
Dominant | D1 | 0.15 | 0.3 | 1.4 | 1.4 |
D2 | 0.15 | 0.1 | 2 | 2 | |
D3 | 0.15 | 0.3 | 2 | 2 | |
Multiplicative | M1 | 0.15 | 0.1 | 1.8 | 3.2 |
M2 | 0.15 | 0.1 | 1.6 | 2.6 | |
M3 | 0.15 | 0.3 | 1.8 | 3.2 | |
Additive | A1 | 0.15 | 0.3 | 1.1 | 1.2 |
A2 | 0.05 | 0.1 | 2 | 3 | |
A3 | 0.15 | 0.1 | 2 | 3 | |
*For each of the four types of models, κ denotes the population prevalence; pa is the minor allele frequency; RR1 and RR2 are the relative risks with one or two copies of the minor alleles, respectively, over the baseline disease risk of having no copy of the minor allele. Note that dominant and multiplicative models are less likely to be associated with a rare disease and therefore κ = 0.05 was not investigated for these two models. |
Inflation ratio with nominal level | ||||
Model | RR1 | RR2 | p=0.05 | p=0.01 |
Recessive | 1 | 1.4 | 10.3 ± 0.206 | 34.4 ± 0.657 |
Dominant | 1.4 | 1.4 | 10.6 ± 0.213 | 35.3 ± 0.804 |
Multiplicative | 1.3 | 1.7 | 11.3 ± 0.179 | 38.0 ± 1.14 |
Additive | 1.2 | 1.4 | 11.0 ± 0.190 | 36.7 ± 1.27 |
∗All four models have a population prevalence of 0.05, reflecting a rarer disease. For each of the four types of models, RR1 and RR2 are the relative risks with one or two copies of the minor alleles, respectively, over the baseline disease risk of having no copy of the minor allele. Each of the entries in the fourth and fifth columns shows the mean and standard deviation of the ratios over 10 replicates. |
Model | Parameter Setting | κ | pa | RR1 | RR2 |
Recessive | R1 | 0.15 | 0.3 | 1 | 1.4 |
R2 | 0.05 | 0.1 | 1 | 4 | |
R3 | 0.15 | 0.1 | 1 | 2 | |
Dominant | D1 | 0.15 | 0.3 | 1.4 | 1.4 |
D2 | 0.15 | 0.1 | 2 | 2 | |
D3 | 0.15 | 0.3 | 2 | 2 | |
Multiplicative | M1 | 0.15 | 0.1 | 1.8 | 3.2 |
M2 | 0.15 | 0.1 | 1.6 | 2.6 | |
M3 | 0.15 | 0.3 | 1.8 | 3.2 | |
Additive | A1 | 0.15 | 0.3 | 1.1 | 1.2 |
A2 | 0.05 | 0.1 | 2 | 3 | |
A3 | 0.15 | 0.1 | 2 | 3 | |
*For each of the four types of models, κ denotes the population prevalence; pa is the minor allele frequency; RR1 and RR2 are the relative risks with one or two copies of the minor alleles, respectively, over the baseline disease risk of having no copy of the minor allele. Note that dominant and multiplicative models are less likely to be associated with a rare disease and therefore κ = 0.05 was not investigated for these two models. |
Inflation ratio with nominal level | ||||
Model | RR1 | RR2 | p=0.05 | p=0.01 |
Recessive | 1 | 1.4 | 10.3 ± 0.206 | 34.4 ± 0.657 |
Dominant | 1.4 | 1.4 | 10.6 ± 0.213 | 35.3 ± 0.804 |
Multiplicative | 1.3 | 1.7 | 11.3 ± 0.179 | 38.0 ± 1.14 |
Additive | 1.2 | 1.4 | 11.0 ± 0.190 | 36.7 ± 1.27 |
∗All four models have a population prevalence of 0.05, reflecting a rarer disease. For each of the four types of models, RR1 and RR2 are the relative risks with one or two copies of the minor alleles, respectively, over the baseline disease risk of having no copy of the minor allele. Each of the entries in the fourth and fifth columns shows the mean and standard deviation of the ratios over 10 replicates. |