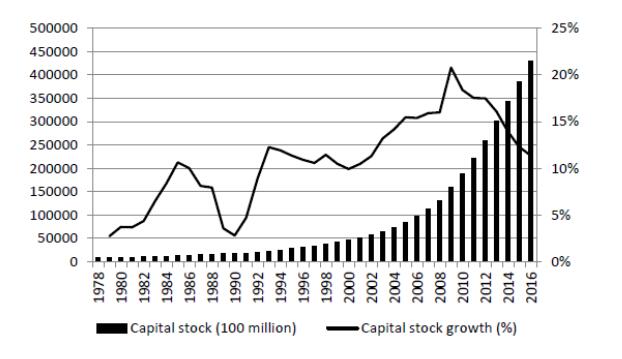
Citation: Tinghui Li, Zimei Huang, Benjamin M Drakeford. Statistical measurement of total factor productivity under resource and environmental constraints[J]. National Accounting Review, 2019, 1(1): 16-27. doi: 10.3934/NAR.2019.1.16
[1] | Giorgio Garau . Total factor productivity and relative prices: the case of Italy. National Accounting Review, 2022, 4(1): 16-37. doi: 10.3934/NAR.2022002 |
[2] | Dina Krasnoselskaya, Venera Timiryanova . Exploring the impact of ecological dimension on municipal investment: empirical evidence from Russia. National Accounting Review, 2023, 5(3): 227-244. doi: 10.3934/NAR.2023014 |
[3] | Shuang Luo, Wenting Lei, Peng Hou . Impact of artificial intelligence technology innovation on total factor productivity: an empirical study based on provincial panel data in China. National Accounting Review, 2024, 6(2): 172-194. doi: 10.3934/NAR.2024008 |
[4] | Tinghui Li, Xue Li . Does structural deceleration happen in China? Evidence from the effect of industrial structure on economic growth quality. National Accounting Review, 2020, 2(2): 155-173. doi: 10.3934/NAR.2020009 |
[5] | Shan Huang, Khor Teik Huat, Zifei Zhou . The studies on Chinese traditional culture and corporate environmental responsibility: literature review and its implications. National Accounting Review, 2022, 4(1): 1-15. doi: 10.3934/NAR.2022001 |
[6] | Rui Xie, Yuanyuan Zhao, Liming Chen . Structural path analysis and its applications: literature review. National Accounting Review, 2020, 2(1): 83-94. doi: 10.3934/NAR.2020005 |
[7] | Andrea Karim El Meligi, Donatella Carboni, Giorgio Garau . Perceived crowding and physical distance rules: a national account perspective. National Accounting Review, 2021, 3(3): 330-341. doi: 10.3934/NAR.2021017 |
[8] | Nataša Stanojević, Katarina Zakić . China and deglobalization of the world economy. National Accounting Review, 2023, 5(1): 67-85. doi: 10.3934/NAR.2023005 |
[9] | Edward Y. Uechi . Determining a proportion of labor and equipment to achieve optimal production: A model supported by evidence of 19 U.S. industries from 2000 to 2020. National Accounting Review, 2024, 6(2): 266-290. doi: 10.3934/NAR.2024012 |
[10] | Nikolay I. Kuryshev, Vladimir R. Tsibulsky . Scarcity of resources as a determining factor of value in input-output models (objectivist concept of capital). National Accounting Review, 2023, 5(3): 208-226. doi: 10.3934/NAR.2023013 |
China's economy has experienced years of sustained growth since Reform and Opening up of the economy, with real GDP growth averaging 9.6 percent in the period 1978–2016, within which six years have a growth rate of more than 13 percent. However, this extensive mode of economic growth has reduced the quality of economic growth and the potential for sustainable growth. The resource and environment are not only the base and support of economic growth, but also the rigid constraint of economic growth quality. As a measure of economic growth, GDP has not taken into account the efficiency of factor inputs and the cost of resource and environmental factors. Therefore, against this background, it is important to consider the resource and environmental constraints in TFP analysis and study the effect of the TFP change on the economic growth quality of a country (or a region) with more scientific measurements.
The existing study with regard to the measurement of TFP mainly focus on the following two aspects. First, based on the perspective of the production function, it is necessary to fit the production function and estimate relative parameters in the process of measuring TFP. The most commonly used methods are the Solow residual method and the stochastic frontier production function. The Solow residual method originated from Solow's growth accounting study, in which he attributed the output increase deducted the output caused by capital and labour to the result of technological progress (Solow, 1957). Kendrick later defined the surplus output as TFP. Some scholars used the Solow Residual method to calculate China's TFP (Jun, 2002; Qingwang and Junxue, 2005). Similarly, Otsuka and Goto (2015) used Solow residual method to measure total factor productivity. Zeng et al. (2015) also employed the Solow residual method to measure China's TFP and the contribution rate of science and technology from 1953 to 2013. They also found that physical capital is the main power of economic growth and the contribution of technological progress to economic growth is declining. Some scholars adopt the stochastic frontier analysis (SFA) method to study the related issues of TFP. Stochastic frontier analysis (SFA) assumes a given functional form for the relationship between inputs and an output. When the functional form is specified then the unknown parameters of the function could be estimated using econometric techniques (Roy et al., 2017). Wang et al. (2006) used the SFA method and drew the conclusion that China's economic efficiency as a whole is not high, and the efficiency of the east of China is higher than that of the west. Coelli et al. (2003) applied a stochastic production frontier model to measure total factor productivity growth in Bangladesh crop agriculture for the 31 observations from 1960 to 1991, using data for 16 regions. Bernini et al. (2017) used SFA to measure total factor productivity.
Second, based on the non-parametric perspective, the measurement of TFP mainly includes two methods: the Malmquist index method and the data envelopment analysis method (DEA). Fare et al. (1994) and Krüger (2001) applied the Malmquist index to measure the productivity among several countries. Li et al. (2018) calculated the total factor energy efficiency (TFEE) of 27 industries in the Jing-Jin-Ji region, then used the Malmquist index to decompose the TFEE of key energy-intensive industries. Yoruk and Zaim (2005) adopted both the ML productivity index and the traditional Malmquist productivity index to analyze the total factor growth from 1983 to 1998 in OECD countries. Zhou et al. (2010) introduced a Malmquist CO2 emission performance index for measuring changes in total factor carbon emission performance over time. Based on the Malmquist TFP index, Chen and Fan (2016) established the transcendental log model of the SFA-Malmquist index to measure the TFP of China. Wang and Fan (2017) adopted the EDA-Malmquist index method to measure the TFP of 30 provinces in China and analyze their change trend, phase characteristics and regional differences. Some scholars used the DEA method to measure the economic growth efficiency. Yuan and Zhong (2010) employed the DEA method to calculate the economic growth efficiency of 29 provinces. They concluded that in the period 1978–2007, the economic development efficiency of China showed the trend of a U-shaped change, and there was a great difference among provinces. Liao and Drakeford (2019) used Data Envelopment analysis (DEA) to evaluate total-factor energy efficiency of 30 province-level divisions in China. Li (2013) used the non-radial DEA method to estimate the economic efficiency and carbon environmental efficiency of 29 provinces in China.
A lager body of existing literature measures and analyzes the TFP with capital and labour as input variables and GDP as the output variable, which ignore the resource and environmental factors and lead to overestimation of the TFP. In order to measure total factor productivity more accurately, more and more scholars have begun to pay close attention to the resource and environmental constraints in the calculation of TFP, when they used DEA method to measure TFP. However, taking environmental variables as input or output factors is controversial. On the one hand, many studies take environmental variables as input factors. Fare et al. (2001) believed that pollution can be incorporated into production process analysis as an input factor, since environmental regulations allocate inputs, which could be used in production to pollution abatement activities. Telle and Larsson (2007) also took emissions as input factors into the measure of productivity. Shadbegian and Gray (2005) believed that the traditional TFP calculation ignores the influence of environmental factors and does not identify the whereabouts of the input of the factors, and the measurement results may lead to the misreading of the meaning of TFP. In the long run, environmental regulations can cause huge social costs, adversely affect the economic development of the country, and hinder the growth of TFP. On the other hand, environmental variables are considered as output variables, along with resource utilization. Jing (2009) used the industrial "three wastes" emissions as the "bad" output and measured the inter-provincial environmental efficiency in China from 1990 to 2006 using the slacks-based measure (SBM) model, and the results show that China's regional average efficiency is decreased obviously under environmental constraints. Zhu et al. (2011) constructed an environmental comprehensive index with environmental indicators like emission and pollution control as output variables to calculate the total factor productivity. Some scholars also taken resource and environmental factors as the output variables to measure the total factor productivity under the resource and environmental constraints (Liu et al., 2012).
In this paper, therefore, to measure the TFP, different from the traditional TFP calculation, we take both resource and environmental factors into the DEA-Malmquist productivity index research framework to build a TFP measurement model. Besides, selecting indicators of environmental governance and pollutant emissions, which can represent environment quality, we build an environmental comprehensive index using principal component analysis. Further, using the adjusted GDP, i.e. the product of the environmental comprehensive index and GDP, and the real GDP respectively as output variables to measure the TFP. We further compare and analyze the results, and show the effect of resource and environmental factors on TFP.
The remainder of the paper is organized as follows. Section 2 presents the measure model of TFP. Section 3 describes variables selection and processing used in the measurement of TFP. Results of the TFP measurement and the characteristic analysis are presented in Section 4. Conclusions are drawn and discussed in Section 5.
There are some differences and limitations among the methods used to measure the TFP. For example, the Solow residual method, which is based on the production function, and the stochastic frontier model, which required a specific production function, subject to conditions of functional form itself, resulting in a certain gap between assumptions and reality. This will lead to the relatively large error due to inevitable measurement errors. In addition, the Solow residual method and the stochastic frontier production function are not suitable for the situation of multiple inputs and outputs, and they also require large sample data, since measure results of small sample data usually have large errors. A small amount of research adopted the SBM-DDF model and the Luenberger productivity index to measure China's provincial industrial or regional TFP under the restrictions of resource and environment. However, the SBM-DDF model, compared with the DDF model, although considering the slack variable, has many binding conditions, which will lead to infeasible solutions and affect the accuracy of measure result.
The data envelopment analysis method (DEA) does not need to set a production function in advance, and it avoids the subjective weight of setting the evaluation index. The DEA method is an excellent relative efficiency analysis method. Its essence is to estimate the effective efficient frontier of the whole system based on a set of input and output samples of multiple decision making units (DMU), and to further analyze the efficiency of the multiple DMU (Cooper et al., 2000). The Malmquist productivity index is a non-parametric function of measuring the productivity with the ratio of the distance function. The analysis of the correlation of a variety of input and output factors variables, has not considered the constraint of the price and minimized cost. The Malmquist index method, based on DEA, which has been widely used in production efficiency analysis, contains the advantages of both methods (Chen and Jia, 2017). In addition to the ease of calculation, it is applicable for the situation when price information is not fully available, since it requires no price information about input and output factors. According to its principle, it can also be used to measure TFP. Therefore, we choose the Malmquist index method based on DEA to measure the TFP under the constraints of resource and environment from the input and output angle.
This paper employs Malmquist index method based on DEA to measure the TFP under the constraints of resource and environment. In order to solve the problem of multiple input and output in time series, we treat each year as a decision making unit (DMU) to calculate TFP. xi represents the input in the i th DMU, and the input variables are labour, capital, technology, and energy.
x=(x1,x2,……,xm)∈RM+ | (1) |
yi represents the output in the i th DMU, and the output variable is the adjusted GDP.
y=(y1,y2,……,yN)∈RM+ | (2) |
The Malmquist output index of period t can be expressed as:
Mt0(xt+1,yt+1,xt,yt)=Dt0(xt+1,yt+1)Dt0(xt,yt) | (3) |
The Malmquist output index of period t+1 can be expressed as:
Mt+10(xt+1,yt+1,xt,yt)=Dt+10(xt+1,yt+1)Dt+10(xt,yt) | (4) |
where Dt0 andDt+10represent respectively the distance function of period t and t+1.
To avoid confusion caused by the randomness of base period selection, Fare (1996) defined the geometric mean of two periods Mtand Mt+1as the Malmquist productivity index, which can be expressed like this:
Mt0(xt+1,yt+1,xt,yt)=[Dt0(xt+1,yt+1)Dt0(xt,yt)×Dt+10(xt+1,yt+1)Dt+10(xt,yt)]1/2 | (5) |
The change of the TFP could be equal to 1, greater than 1 or less than 1, indicating that TFP and its decomposition components may have no change, be improved or be reversed.
Before using the DEA-Malmquist index model to measure TFP, the input and output indicators should be determined first. Based on the input and output indicators selected in advance, the DEA-Malmquist index model can be either a multi-input model or multi-output model. The selection of input and output indicators has a direct impact on the measurement of the efficiency of the decision-making unit (DMU). According to the research purpose, based on the theory of TFP and the setting of factors of existing literature, this paper selects corresponding input and output variables from the perspective of resource and environmental constraints.
This paper selects four input variables, namely, labour, energy, technology and capital, to measure TFP. For the labour variable, we use the human capital stock as representation, and the total human capital stock is the product of the population on average of education years and the number of employees. The unit is 10, 000 per year and in order to calculate the average number of educated people, the level of education can be divided into four classes: college graduate or above (16 years), high school (12 years), junior high school (9 years), primary school or below (6 years). In addition, the total energy consumption of China is used to represent energy input variable. On the input variable of science and technology, this paper uses scientific and technological innovation scale, which refers to the scale of state funding for science and technology innovation, obtained by weighted R & D investment and the number of researchers. The specific calculation method is: Gj=αRj+(1−α)Nj, where Gj is the scientific and technological innovation scale of the year j, and Rj and Nj represent R & D investment and the number of researchers respectively; the value of weight α is 0.5, which means that R & D investment and the number of researchers contribute the same to the scale of innovation in science and technology. About capital input variables, the economy's capital stock is used as a measure of capital input both at home and abroad. Thus, the capital stock is first estimated in this paper according to the method of perpetual inventory put forward by GoldSmith (1951); then, the amount of capital available for this year is the selected base period plus annual net capital investment.
Kt=(1−δt)×Kt−1+It/Pt | (6) |
In Equation 6, Kt represents the capital stock in year t; Kt−1 represents the capital stock in year t−1; It represents the amount of investment in year t; δt represents fixed capital depreciation rate, and Pt represents fixed asset investment price index. In terms of determining the base year capital stock, generally, year 1952 or year 1978 is used. Since the China statistical yearbook does not have an early fixed stock, the capital stock in 1978 is calculated using the extrapolation method. Followed by Zhang and Zhang (2003), this paper obtains the capital stock in 1978, that is, 123.96 billion yuan, from the base period of 1952, and converts fixed asset investment price index to the current price of year 1978. With regard to the selection of the fixed capital depreciation rate δt, different scholars have different preferences. For example, Perkins (1998), Wang et al. (2000), Wang and Yao (2003) adopted a 5% depreciation rate; Hall and Jones (1999), Young (2000), Yu (2013) used a 6% depreciation rate; while Liu (2002), Gong et al. (2004) adopted a 10% depreciation rate. This paper refers to a 10.96% depreciation rate adopted by Shan (2008). For fixed assets investment price index Pt, we use a complete price index of investment in fixed assets by combining the sequence of fixed assets investment price index adopted by Guo and Jia (2003) with the national price index of investment in fixed assets released by the Bureau of Statistics after 2003. In terms of investment, this paper uses the total fixed capital formation. Capital stock and its growth rate are calculated and shown in Figure 1.
Considering the resource and environmental factors, the adjusted GDP is selected as the output variable, i.e. the product of the environmental composite index and the real GDP. In addition, in order to analyze the influence of the resource and environmental factors on TFP, the real GDP is also selected as the output variable.
The environmental comprehensive index (ECI) can summarize the impact of environmental factors on economic development. In other words, the ECI can reflect the effectiveness of environmental governance. The greater the value of the ECI, the smaller the environmental cost of economic development. Conversely, the smaller the value of ECI, the greater the environmental cost. When building the ECI, both environmental pollution and the environmental pollution control should be considered. From the perspective of environmental input and output, we select nine indicators, including three absolute and six relative indicators. The input indicators include the total investment in environmental pollution control and the total investment of industrial pollution control, while the output indicators are mainly based on the data of industrial waste discharge and handling. The data are obtained from the "China environmental statistics report" over 1978–2016. Specific environmental input and output indicators and their definitions are shown in Table 1.
Variable | Indicator | Description |
Input | Total investment in industrial pollution control (10, 000 yuan) | Total investment in industrial pollution control |
Total investment in environmental governance (10, 000 yuan) | Total investment in environmental governance | |
Output | Standard meeting rate of industrial waste water discharge (%) | Standard-meeting industrial waste water discharge/total industrial waste water discharge × 100% |
Sulfur dioxide removal rate (%) | Sulfur dioxide removal/sulfur dioxide emissions × 100% | |
Industrial dust removal rate (%) | Industrial dust removal/industrial dust emissions × 100% | |
Industrial smog removal rate (%) | Industrial smog removal/industrial smog emission × 100% | |
Solid waste disposal rate (%) | Industrial solid waste disposal/total industrial solid waste × 100% | |
Comprehensive utilization of solid waste (%) | Comprehensive utilization of industrial solid waste/total industrial solid waste × 100% | |
Product value of comprehensively used "three wastes" (10, 000 yuan) | Product value of comprehensively used industrial "three wastes" |
When calculating the ECI, the dimension reduction methods must be adopted to simplify distracting factors. The commonly used ones are the factor analysis and the principal component analysis (PCA). Followed the method adopted by Fan et al. (2003), we use the factor analysis to concentrate the data and the PCA method to determine the factor weight. Principal component analysis is a multi-indicator statistical method, which can transform multi-indicators into a few comprehensive indicators (i.e. the main components), and most of the original information can be embodied in the main components (Abdi and Williams, 2010). This method reduces the index dimensions and simplifies the complex problems to the maximum extent. The main advantage of the PCA is its objectivity, that is, the determination of the weight is dependent on the characteristics of the data itself, not subjective judgment, thus avoiding subjective arbitrariness.
In order to eliminate the possible impact of different dimensions, we first standardize data processing, and then use SPSS 20.0 to carry out the KMO Test and the Bartlett Sphericity Test on the data. According to the results, the KMO value is close to 1, and the P value of the Bartlett's Sphericity Test is less than 0.05, which rejected the null hypothesis, i.e. the correlation coefficient matrix is the unit matrix, and all data passed the test. Therefore, the data used in this paper is suitable for factor analysis. When the cumulative variance contribution rate of the principal components reaches 80%, the number of factors is determined. After calculating the score of comprehensive factors, we follow the data processing method adopted by Zhu et al. (2011) to transfer the data into values between [0, 1], according to the following Equation 7. The adjusted data is the environmental comprehensive Index (ECI).
ECli=siMax(Si)−Min(Si)×0.4+0.6 | (7) |
where Si represents the comprehensive factor score in year i; Max(Si) and Min(Si) represent the maximum and minimum values of the corresponding comprehensive factors scores. Figure 2 shows the results of the ECI.
According to the theoretical model built above, we use software DEAP2.1 to measure the TFP index from 1978 to 2016. The results are shown in Table 2.
Year | Index value | Year | Index value | Year | Index value |
1978 | 1.000 | 1991 | 1.16 | 2004 | 1.103 |
1979 | 1.285 | 1992 | 1.189 | 2005 | 1.079 |
1980 | 0.934 | 1993 | 1.266 | 2006 | 1.108 |
1981 | 1.253 | 1994 | 1.332 | 2007 | 1.189 |
1982 | 1.061 | 1995 | 1.198 | 2008 | 1.107 |
1983 | 1.085 | 1996 | 1.128 | 2009 | 1.051 |
1984 | 1.151 | 1997 | 1.06 | 2010 | 1.104 |
1985 | 1.038 | 1998 | 1.037 | 2011 | 1.097 |
1986 | 1.224 | 1999 | 1.029 | 2012 | 1.049 |
1987 | 1.141 | 2000 | 1.074 | 2013 | 1.070 |
1988 | 1.225 | 2001 | 1.081 | 2014 | 1.044 |
1989 | 1.007 | 2002 | 1.042 | 2015 | 1.052 |
1990 | 1.026 | 2003 | 1.042 | 2016 | 1.073 |
The TFP indexes of China are larger than 1 except for 1980. The TFP index represents the rate of change of efficiency. The benchmark year is year 1978, and the index value is 1. When the index is bigger than 1, it indicates that the efficiency increased compared to the previous year; whereas when the index is less than 1, it means that compared to the previous year, efficiency decreased. The size of the efficiency change rate is reflected in the index value. As can be seen from Table 2, there is no significant fluctuation in the TFP index from 1978 to 2016. The results show that the TFP is only less than 1 in 1980, i .e. 0.934, and the rest all are greater than 1 (the maximum value is 1.332 in 1994). TFP is basically in a slow rising state, which indicating that environment quality is improved.
In order to further obtain the fluctuation of the TFP, descriptive statistical analysis was carried out. The results are shown in Table 3. The mean and median of the TFP index are 1.108 and 1.081 respectively, and there is little difference between mean and median, indicating that the TFP has an increasing trend on average. In addition, the standard deviation and variance are 0.087 and 0.008 respectively. These quite small values indicate that the TFP fluctuates little between 1978 and 2016, which means the overall trend is stable. The kurtosis coefficient and the skewness coefficient of the TFP index are 0.195 and 0.760 respectively, indicating that the distribution pattern is close to the normal distribution, but it shows a slight flat peak and left-deviation.
Mean | Median | Std. Dev. | Variance | Kurtosis | Skewness | Min | Max | |
TFP | 1.108 | 1.081 | 0.087 | 0.008 | 0.195 | 0.760 | 0.934 | 1.332 |
Based on the data in Table 2, and combined with GDP growth rate, the time sequence diagram of TFP is plotted, which is shown in Figure 3.
From Figure 3, it can be seen that although the volatility range of TFP index is higher than that of GDP growth rate, the trend of which is in line with the macroeconomic fluctuation, especially after 1988. There exists a significant positive relationship between the TFP index and the GDP growth rate at the 1% level, and both the Pearson correlation coefficient and Spearman's correlation coefficient are close to 0.8 during the period 1988 to 2016, indicating that China's TFP change relates closely to macroeconomic fluctuation. The TFP index during 1978–1979 rises considerably and reaches a peak of 1.285. During 1979–1980, the TFP index had a fall of 27.3%, reaching the lowest point 0.934, but it quickly recovered and rose to 1.253 in 1981. During 1981–1988, TFP experienced a fluctuation, with the basic level remaining at around 1.13. It then fell 17.8% in 1988–1989, followed by a steady state in 1989–1990. The GDP growth rate in 1978–1983 was in a stable condition, with slightly small float, then it rose substantially in 1984 and reached a peak of 15.2%. During 1985–1990, the GDP growth rate fluctuated in a certain range, whereas from 1989 to 1990, it reached a trough of 3.9%. In the period of 1989–1994, both the TFP and the GDP growth rate ascended continuously and reached the peak values, 1.332 and 13.9% respectively, in 1994; then both fell dramatically in 1998. During the period of 1998–2007, both were in a steady rise and reached to peaks 1.189 and 14.2%, but they then declined consistently in 2009, achieving troughs of 1.051 and 9.4% respectively. The TFP index reminded stable in 2009–2016, whereas the GDP growth rate experienced a small rise and fell in 2009–2012, followed by a steady decline in 2016.
In general, these changes can be seen in the context of economic development. China's TFP experienced a relatively frequent fluctuation before 1994, and it grew quite fast during the early stage of the Reform and Opening up of the economy. The reason lies in the fact that during the transformation from a planned economy to a market economy, a series of system innovations, such as the household contract responsibility system, which relaxed restrictions on non-state sectors, released the potential of China's factors efficiency. This improved the overall production efficiency, and effectively promoted the rapid growth of production efficiency, thus improved the rapid growth of TFP. From 1994 to 1998, China's TFP had fallen slightly, because of China's excessive investment, which caused the decline of production capacity. The deflation that started in 1998 led to a situation of excess capacity. The decline in TFP has been alleviated to a certain extent, and the TFP rebounded in 2001 due to a proactive fiscal policy, which the state implemented to promote the overall economic situation. Therefore, the direction of macro regulation and economic system reform in China greatly affect the trend and scope of TFP.
The main objective of this study is to measure the TFP. To achieve this objective, we employed the DEA-Malmquist index method to measure the TFP of China in the period 1978–2016.
Based on the empirical results, several important conclusions are drawn, as follows. First, the TFP indexes of China are larger than 1 except for year 1980, which indicated that the efficiency increased compared to the previous year. Furthermore, the results indicate that the volatility range of TFP index is higher than that of GDP growth rate, the trend of which is in line with the macroeconomic fluctuation, especially after 1988. In other words, TFP is closely related to the macroeconomic fluctuations, and the direction of macro-control and economic system reform greatly affect the change trend and fluctuation range of TFP.
Authors would like to thank Guangzhou University for sponsoring this research.
The authors declare no conflict of interest.
[1] |
Abdi H, Williams LJ (2010) Principal component analysis. Wiley Interdiscip Rev Comput Stat 2: 433–459. doi: 10.1002/wics.101
![]() |
[2] |
Bernini C, Cerqua A, Pellegrini G (2017) Public subsidies, TFP and efficiency: A tale of complex relationships. Res Policy 46: 751–767. doi: 10.1016/j.respol.2017.02.001
![]() |
[3] | Chen H, Fan S (2016) The Impact of the Belt and Road Initiative on the total factor productivity of countries along the line. Stat Decis 23: 116–119. |
[4] |
Chen L, Jia G (2017) Environmental efficiency analysis of China's regional industry: a data envelopment analysis (DEA) based approach. J Clean Prod 142: 846–853. doi: 10.1016/j.jclepro.2016.01.045
![]() |
[5] | Coelli T, Rahman S, Thirtle C (2003) A stochastic frontier approach to total factor productivity measurement in Bangladesh crop agriculture, 1961–1992. J Int Dev: J Dev Stud Assoc 15: 321–333. |
[6] | Cooper WW, Seiford LM, Tone K (2000) Data envelopment analysis, Handbook on Data Envelopment Analysis, 1st ed.; Cooper, WW, Seiford, LM, Zhu, J., Eds, 1–40. |
[7] | Fare R, Grosskopf S, Norrism M (1994) Productivity growth, technical progress, and efficiency change in industrialized countries. Am Econ Rev 87: 1040–1044. |
[8] |
Fare R, Grosskopf S, Pasurka C (2001) Accounting for air pollution emissions in measuring state manufacturing productivity growth. J Regl Sci 41: 381–409. doi: 10.1111/0022-4146.00223
![]() |
[9] | Jing CDRL (2009) Eco-efficiency Differences across Provinces in China in the Presence of Environmental Constraints: 1990–2006. Financ Trade Res, 5. |
[10] | Jun Z (2002) Capital Formation, Industrialization and Economic Growth: Understanding China's Economic Reform. Econ Res J 6: 3–13. |
[11] | Krüger J (2001) The global trends of total factor productivity: evidence from the nonparametric malmquist index approach. Oxford Econ Pap 55: 265–286. |
[12] |
Li J, Xiang Y, Jia H, et al. (2018) Analysis of total factor energy efficiency and its influencing factors on key energy-intensive industries in the Beijing-Tianjin-Hebei Region. Sustainability 10: 111. doi: 10.3390/su10010111
![]() |
[13] | Li T (2013) Measuring the win-win performance of China's carbon reduction and economic growth with energy constraints: a rang-adjusted measure of non-radial DEA. China Econ Q, 667–692. |
[14] | Liao G, Drakeford B (2019) An analysis of financial support, technological progress and energy efficiency: evidence from China. Green Financ 1: 174–187. |
[15] | Liu R, An T (2012) Trend and factor analysis of Chinese economic growth performance under restrictions of resource and environment-a research based on a new method of productivity index's construction and decomposition. Econ Res J, 34–47. |
[16] |
Otsuka A, Goto M (2015) Agglomeration economies in Japanese industries: the Solow residual approach. Ann Reg Sci 54: 401–416. doi: 10.1007/s00168-015-0659-z
![]() |
[17] | Qingwang G, Junxue J (2005) Estimating Total Factor Productivity in China. Econ Res J 6: 51–60. |
[18] |
Roy PK, Das PS, Pal MK (2017) Decomposition of Total Factor Productivity Growth of the 2-Digit Manufacturing Industries in West Bengal: A Stochastic Frontier Approach. Arthaniti-J Econ Theory Pract 16: 101–124. doi: 10.1177/0976747920170104
![]() |
[19] |
Shadbegian RJ, Gray WB (2005) Pollution abatement expenditures and plant-level productivity: a production function approach. Ecol Econ 54: 196–208. doi: 10.1016/j.ecolecon.2004.12.029
![]() |
[20] | Solow RM (1957) Technical change and the aggregate production function. Rev Econ Stat, 312–320. |
[21] |
Solveig G, Wei T (2016) The effects of energy efficiency improvement in China with global interaction. AIMS Energy 4: 37–51. doi: 10.3934/energy.2016.1.37
![]() |
[22] | Telle K, Larsson J (2007) Do environmental regulations hamper productivity growth? How accounting for improvements of plants' environmental performance can change the conclusion. Ecol Econ 61: 438–445. |
[23] | Wang Z, Gong L, Chen Y (2006) China's regional differences in technical efficiency and the decomposition of total factor productivity growth (1978–2003). Soc Sci China, 55–66. |
[24] |
Yörük BK, Zaim O (2005) Productivity growth in OECD countries: A comparison with Malmquist indices. J Comp Econ 33: 401–420. doi: 10.1016/j.jce.2005.03.011
![]() |
[25] | Yuan X, Zhong Y (2010) The empirical analysis of the time-space change and influencing factors about China's regional economic development efficiency based on the super-efficiency DEA model. J Bus Econ, 81–90. |
[26] | Zeng G, Wang L, Wang W (2015) Contribution rate on progress of science and technology in China: 1953–2013. Forum Sci Tech China, 22–27. |
[27] |
Zhou P, Ang BW, Han JY (2010) Total factor carbon emission performance: a Malmquist index analysis. Energy Econ 32: 194–201. doi: 10.1016/j.eneco.2009.10.003
![]() |
[28] | Zhu C, Yue H, Shi Q (2011) Empirical study on China's economic growth efficiency under the binding of environment. J Quant Tech Econ 5: 3–20. |
1. | Tinghui Li, Jiehua Ma, Bin Mo, Does the Land Market Have an Impact on Green Total Factor Productivity? A Case Study on China, 2021, 10, 2073-445X, 595, 10.3390/land10060595 | |
2. | Tinghui Li, Jiehua Ma, Bin Mo, Does Environmental Policy Affect Green Total Factor Productivity? Quasi-Natural Experiment Based on China’s Air Pollution Control and Prevention Action Plan, 2021, 18, 1660-4601, 8216, 10.3390/ijerph18158216 | |
3. | Shuai Wang, Cunyi Yang, Zhenghui Li, Spatio-Temporal Evolution Characteristics and Spatial Interaction Spillover Effects of New-Urbanization and Green Land Utilization Efficiency, 2021, 10, 2073-445X, 1105, 10.3390/land10101105 | |
4. | Cunyi Yang, Tinghui Li, Khaldoon Albitar, Does Energy Efficiency Affect Ambient PM2.5? The Moderating Role of Energy Investment, 2021, 9, 2296-665X, 10.3389/fenvs.2021.707751 | |
5. | Jiehua Ma, Zhenghui Li, Does Digital Financial Inclusion Affect Agricultural Eco-Efficiency? A Case Study on China, 2021, 11, 2073-4395, 1949, 10.3390/agronomy11101949 | |
6. | Yaya Su, Zhenghui Li, Cunyi Yang, Spatial Interaction Spillover Effects between Digital Financial Technology and Urban Ecological Efficiency in China: An Empirical Study Based on Spatial Simultaneous Equations, 2021, 18, 1660-4601, 8535, 10.3390/ijerph18168535 | |
7. | T Kuznietsova, Y Krasovska, O Lesniak, O Podlevska, O Harnaha, Assessment of multifactor productivity based on empirical data in the agricultural sector of the economy of Ukraine, 2023, 1126, 1755-1307, 012018, 10.1088/1755-1315/1126/1/012018 | |
8. | Minglong Zhang, Yin Liu, Influence of digital finance and green technology innovation on China's carbon emission efficiency: Empirical analysis based on spatial metrology, 2022, 838, 00489697, 156463, 10.1016/j.scitotenv.2022.156463 | |
9. | Rongwu Zhang, Lan Luo, Jianjun Du, The influence of fiscal policy uncertainty on corporate total factor productivity: Evidence from Chinese public companies, 2023, 1074-3529, 10.1111/coep.12606 | |
10. | Zhenghui Li, Fanqi Zou, Yong Tan, Jinhui Zhu, Does Financial Excess Support Land Urbanization—An Empirical Study of Cities in China, 2021, 10, 2073-445X, 635, 10.3390/land10060635 | |
11. | Mengxin Wang, Yanling Li, Gaoke Liao, Research on the Impact of Green Technology Innovation on Energy Total Factor Productivity, Based on Provincial Data of China, 2021, 9, 2296-665X, 10.3389/fenvs.2021.710931 |
Variable | Indicator | Description |
Input | Total investment in industrial pollution control (10, 000 yuan) | Total investment in industrial pollution control |
Total investment in environmental governance (10, 000 yuan) | Total investment in environmental governance | |
Output | Standard meeting rate of industrial waste water discharge (%) | Standard-meeting industrial waste water discharge/total industrial waste water discharge × 100% |
Sulfur dioxide removal rate (%) | Sulfur dioxide removal/sulfur dioxide emissions × 100% | |
Industrial dust removal rate (%) | Industrial dust removal/industrial dust emissions × 100% | |
Industrial smog removal rate (%) | Industrial smog removal/industrial smog emission × 100% | |
Solid waste disposal rate (%) | Industrial solid waste disposal/total industrial solid waste × 100% | |
Comprehensive utilization of solid waste (%) | Comprehensive utilization of industrial solid waste/total industrial solid waste × 100% | |
Product value of comprehensively used "three wastes" (10, 000 yuan) | Product value of comprehensively used industrial "three wastes" |
Year | Index value | Year | Index value | Year | Index value |
1978 | 1.000 | 1991 | 1.16 | 2004 | 1.103 |
1979 | 1.285 | 1992 | 1.189 | 2005 | 1.079 |
1980 | 0.934 | 1993 | 1.266 | 2006 | 1.108 |
1981 | 1.253 | 1994 | 1.332 | 2007 | 1.189 |
1982 | 1.061 | 1995 | 1.198 | 2008 | 1.107 |
1983 | 1.085 | 1996 | 1.128 | 2009 | 1.051 |
1984 | 1.151 | 1997 | 1.06 | 2010 | 1.104 |
1985 | 1.038 | 1998 | 1.037 | 2011 | 1.097 |
1986 | 1.224 | 1999 | 1.029 | 2012 | 1.049 |
1987 | 1.141 | 2000 | 1.074 | 2013 | 1.070 |
1988 | 1.225 | 2001 | 1.081 | 2014 | 1.044 |
1989 | 1.007 | 2002 | 1.042 | 2015 | 1.052 |
1990 | 1.026 | 2003 | 1.042 | 2016 | 1.073 |
Mean | Median | Std. Dev. | Variance | Kurtosis | Skewness | Min | Max | |
TFP | 1.108 | 1.081 | 0.087 | 0.008 | 0.195 | 0.760 | 0.934 | 1.332 |
Variable | Indicator | Description |
Input | Total investment in industrial pollution control (10, 000 yuan) | Total investment in industrial pollution control |
Total investment in environmental governance (10, 000 yuan) | Total investment in environmental governance | |
Output | Standard meeting rate of industrial waste water discharge (%) | Standard-meeting industrial waste water discharge/total industrial waste water discharge × 100% |
Sulfur dioxide removal rate (%) | Sulfur dioxide removal/sulfur dioxide emissions × 100% | |
Industrial dust removal rate (%) | Industrial dust removal/industrial dust emissions × 100% | |
Industrial smog removal rate (%) | Industrial smog removal/industrial smog emission × 100% | |
Solid waste disposal rate (%) | Industrial solid waste disposal/total industrial solid waste × 100% | |
Comprehensive utilization of solid waste (%) | Comprehensive utilization of industrial solid waste/total industrial solid waste × 100% | |
Product value of comprehensively used "three wastes" (10, 000 yuan) | Product value of comprehensively used industrial "three wastes" |
Year | Index value | Year | Index value | Year | Index value |
1978 | 1.000 | 1991 | 1.16 | 2004 | 1.103 |
1979 | 1.285 | 1992 | 1.189 | 2005 | 1.079 |
1980 | 0.934 | 1993 | 1.266 | 2006 | 1.108 |
1981 | 1.253 | 1994 | 1.332 | 2007 | 1.189 |
1982 | 1.061 | 1995 | 1.198 | 2008 | 1.107 |
1983 | 1.085 | 1996 | 1.128 | 2009 | 1.051 |
1984 | 1.151 | 1997 | 1.06 | 2010 | 1.104 |
1985 | 1.038 | 1998 | 1.037 | 2011 | 1.097 |
1986 | 1.224 | 1999 | 1.029 | 2012 | 1.049 |
1987 | 1.141 | 2000 | 1.074 | 2013 | 1.070 |
1988 | 1.225 | 2001 | 1.081 | 2014 | 1.044 |
1989 | 1.007 | 2002 | 1.042 | 2015 | 1.052 |
1990 | 1.026 | 2003 | 1.042 | 2016 | 1.073 |
Mean | Median | Std. Dev. | Variance | Kurtosis | Skewness | Min | Max | |
TFP | 1.108 | 1.081 | 0.087 | 0.008 | 0.195 | 0.760 | 0.934 | 1.332 |