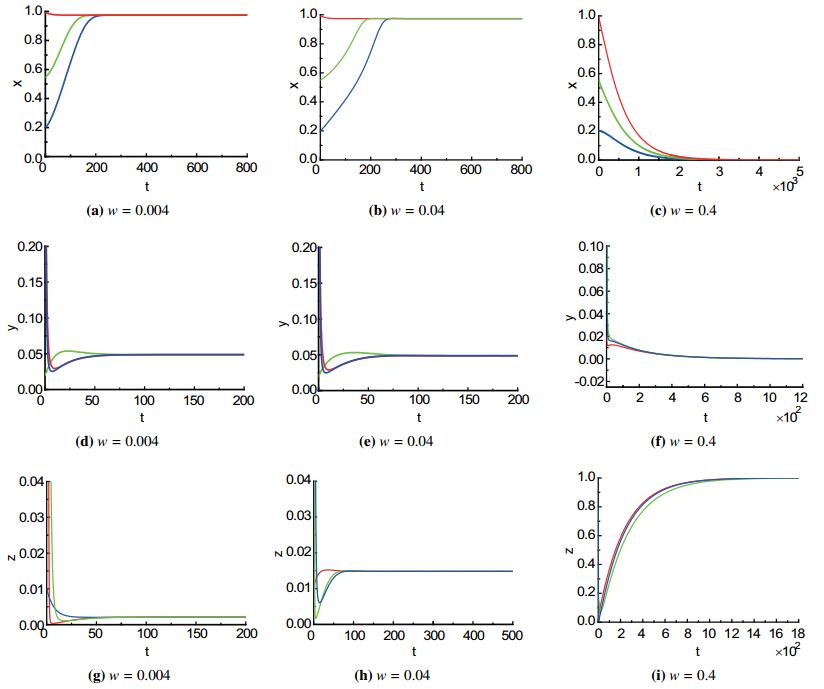
Citation: Jinna Lu, Xiaoguang Zhang. Bifurcation analysis of a pair-wise epidemic model on adaptive networks[J]. Mathematical Biosciences and Engineering, 2019, 16(4): 2973-2989. doi: 10.3934/mbe.2019147
[1] | Linda J. S. Allen, P. van den Driessche . Stochastic epidemic models with a backward bifurcation. Mathematical Biosciences and Engineering, 2006, 3(3): 445-458. doi: 10.3934/mbe.2006.3.445 |
[2] | Hongfan Lu, Yuting Ding, Silin Gong, Shishi Wang . Mathematical modeling and dynamic analysis of SIQR model with delay for pandemic COVID-19. Mathematical Biosciences and Engineering, 2021, 18(4): 3197-3214. doi: 10.3934/mbe.2021159 |
[3] | Xiaoli Wang, Junping Shi, Guohong Zhang . Bifurcation analysis of a wild and sterile mosquito model. Mathematical Biosciences and Engineering, 2019, 16(5): 3215-3234. doi: 10.3934/mbe.2019160 |
[4] | Shishi Wang, Yuting Ding, Hongfan Lu, Silin Gong . Stability and bifurcation analysis of $ SIQR $ for the COVID-19 epidemic model with time delay. Mathematical Biosciences and Engineering, 2021, 18(5): 5505-5524. doi: 10.3934/mbe.2021278 |
[5] | Xiaohong Tian, Rui Xu, Ning Bai, Jiazhe Lin . Bifurcation analysis of an age-structured SIRI epidemic model. Mathematical Biosciences and Engineering, 2020, 17(6): 7130-7150. doi: 10.3934/mbe.2020366 |
[6] | A. Q. Khan, M. Tasneem, M. B. Almatrafi . Discrete-time COVID-19 epidemic model with bifurcation and control. Mathematical Biosciences and Engineering, 2022, 19(2): 1944-1969. doi: 10.3934/mbe.2022092 |
[7] | Baojun Song, Wen Du, Jie Lou . Different types of backward bifurcations due to density-dependent treatments. Mathematical Biosciences and Engineering, 2013, 10(5&6): 1651-1668. doi: 10.3934/mbe.2013.10.1651 |
[8] | Dirk Stiefs, Ezio Venturino, Ulrike Feudel . Evidence of chaos in eco-epidemic models. Mathematical Biosciences and Engineering, 2009, 6(4): 855-871. doi: 10.3934/mbe.2009.6.855 |
[9] | Huan Dai, Yuying Liu, Junjie Wei . Stability analysis and Hopf bifurcation in a diffusive epidemic model with two delays. Mathematical Biosciences and Engineering, 2020, 17(4): 4127-4146. doi: 10.3934/mbe.2020229 |
[10] | Yingzi Liu, Zhong Li, Mengxin He . Bifurcation analysis in a Holling-Tanner predator-prey model with strong Allee effect. Mathematical Biosciences and Engineering, 2023, 20(5): 8632-8665. doi: 10.3934/mbe.2023379 |
Modelling has become an important approach in understanding and control of infectious diseases over recent years. Classical infectious disease model has a long history which can trace back to the study of smallpox in 1760 by Bernoulli [1]. Since then the classical compartmental epidemic models are used more and more to study the epidemic spreading in human population. The homogeneous mixing model in early stage assume that the population are well-mixing, meaning that each pair of individuals has an equal probability of contacting which is idealized obviously. With the rise of complex network, it is found that the complex networks can characterize the contact pattern of people more practical. And increasingly, more and more researchers realize that modeling the spreading of epidemic incorporating complex social network structures is more reasonable. Thus Pastor-Satorras et al. established and studied the susceptible-infective-susceptible(SIS) model based on the node dynamics on static homogeneous and scale-free networks (so called node-based epidemic model), respectively [2]. They proved that there has no the epidemic threshold in scale-free network when the parameters are proper. Furthermore, Pastor-Satorras and Vespignani studied the transmission threshold of epidemic on scale-free networks with the limited scale [3]. Then Boguna et al. gave the critical value of SIS epidemic model with correlations on complex networks [4], and they found that the epidemic threshold inversely proportional to the largest eigenvalue of the connectivity of the average number of contaction with the type of a node with connectivity k linking to the other node with degree k′.
Following this idea, a large body of investigations on network epidemiology emerged based on the modeling approach of node-based epidemic model. Mieghem et al. [5] introduced a node-level epidemic model by remoulding a traditional SIS model. Yang et al. [6,7,8] studied the spreading of virus by taking into account heterogeneous, the influence of patch forwarding and bi-virus competing, respectively. Zhang et al. [9] established an SIS model for epidemic spreading on semi-directed networks, which contains the outbreak threshold on undirected networks and directed networks as special cases. Yang et al. [10] incorporated the infection age into on epidemic model on complex networks and studied the effect of which on epidemic spreading. Peng et al. [11] considered the dual influences of dynamical contact networks' structures and heterogeneous spatial constraints. Sun et al. [12] investigated the effect of feedback regulation on vegetation patterns in semi-arid environments on homogeneous networks, and gave a conclusion and compare between the homogeneous mixing models and network-based epidemic models[13]. However, the node-based epidemic models on complex networks neglects the correlation of connections, Keeling et al. [14,15,16] proposed the moment closure epidemic model by taking the different types of edges into the dynamic models (so called edge-based epidemic model). Wang et al. [17,18,19] put forward several different edge-based epidemic models investigating the effect of multiple routes of transmission, infectious force in latent period on random networks and two-stage contact process on complex networks, respectively. Yang et al. [20] proposed a new coumputational approach for the basic reproduction number of epidemic models on complex networks. Li et al. [21,22] studied the spread of several specific infectious diseases through modeling approach of epidemic models on homogeneous complex networks.
Furthermore, modeling epidemic on dynamical networks has attracted tremendous interest recently. With the study of infections disease, people find that adaptive behavior is frequently happening. For example, if the susceptible discover they have infected neighbors, the edges between the susceptibles and infected neighbors can be automatically interrupted to avoid being infected. The edge after disconnecting can sometimes rewire with other non-infected node or don't rewire sometimes. Then most of the researches either mainly focus on the studies of structures of complex networks[23,24] or dynamics of node-based epidemic models on networks [25,26,27,28]. Moreover, Zhang et al. [28] established the epidemic models on complex networks to study the spreading of seasonal-influenza diseases, and they found that that preferential imitation can invalidate targeted subsidy policies epidemic spreading. Gross et al. [29,30]studied a SIS edge-based model in dynamical networks. It's worth mentioning that Zhang et al. [31] gave a rigorously proof that the adaptive behaviors can lead to rich dynamics like degenerate Hopf bifurcation, homoclinic bifurcation and Bogdanov-Takens bifurcation analytical, etc. All of these studies suggested that a simple rewiring rule for the network has a profound impart on the network structure and dynamics behavior. In addition, Shaw et al. studied susceptible-infective-recovered-susceptible(SIRS) model in dynamics network and employ Monte Carlo for stochastic simulation. In this paper, it is assumed that once susceptible node has lost the connection with an infected neighbour, it will re-established connections only with other noninfected node, and there exist threshold and different steady state solutions when rewiring rate is zero. When rewiring rate isn't zero, there have rich dynamics which contains bistable phenomenon and Hopf bifurcation, transcritical bifurcation and saddle-node bifurcation [32]. By contrast, Zanette and Risau-Gusman [33] assumed that the new connections are allowed connected with other nodes randomly whatever their states are. This sort of rewiring is appropriate for asymptomatic diseases. Besides that, Schwarzkopf et al. [34] considered a epidemic model with an discriminate rewiring mechanism, depending on the nodes' degree instead of the infection process. More recently, Rogers et al. study a SIRS pair-wise proxy model by combining random activation-deletion links with 'smart' rewiring, they develop a method to predict the effects of stochasticity in adaptive network [35]. Moreover, Juher et al. [36] studied the epidemic spreading with two extinction scenarios by bifurcation analysis, and they obtained an analytical condition for the occurrence of bistability based on[29]. Zhou et al. revealed the phenomenon of epidemic reemergence in growing network by the dynamics of bistability and oscillation[37].
Make a survey of above studies, the triples approximation formula used to close the moment closure models is based on the Poisson distribution, in which the average degree are often used to stand for the nodes' degrees. However, there are very large difference among degrees of nodes of real world complex networks since which are usually heterogeneous. Thus the triples approximation formula overlooked the degree of center node of triples which has dramatic effects on the accuracy of approximation and the analysis of system. By incorporating the center node's degree as a variable of triples approximation formula, Kiss et al. [38] proposed a more precise approximation formula which can consider the effect of heterogeneous of the complex networks. Above all, in this paper, we study how the topological structures of adaptive networks influences epidemic dynamics using the new triples approximation formula. We firstly close the model and reduce which to a three dimensional system by some reasonable assumptions. Owing to the more nonlinearity of the new formula, the analysis of the corresponding closed model is more difficult. By the qualitative theory and the stability theory of ordinary differential equations, the basic reproduction number is derived, and the rich dynamics of backward bifurcation, saddle-node bifurcation and Hopf bifurcation are analyzed.
In our paper, we develop a new triple approximation formula [38] and make further theoretical analysis on the basis of the previous researches. The new triple approximation formula can involve the dramatic effects of center nodes' degree of triples on the structures, which makes the approximation more accurate. We close the epidemic model on adaptive networks on the basis of new triple approximation formula, and the analytical conditions for rich dynamics like backward bifurcation and saddle-node bifurcation are given. The outline of this paper is as follows. In Section 2, we give the SIS epidemic model based on the adaptive networks, then an equivalent nondimensionalized three dimensional model is derived under the new triple approximation formula. In Section 3, the existence and stabilities of equilibria are studied, and the occurrence of backward bifurcation and saddle-node bifurcation are proven analytically. In Section 4, the normal form of Hopf bifurcation and the first Lyapunov coefficient are derived by a numerical example. What is more, numerical simulations (bifurcation diagrams and time series plots) holding for these results are also provided. Finally a brief conclusion is given in Section 5.
We consider an adaptive network with the constant number of nodes and connections are N and K, respectively. Thus the average degree of the network can be denoted as n=2KN. Since there are no isolated nodes in the network and the network is connected, the degree of every node is larger that 1, i.e. n>1.
The individuals (nodes) of the network are divided into two groups: the susceptibles (S), and the infected (I). In the model, transmission will occur from infected nodes to susceptible nodes along an edge connecting an infected node with a susceptible individual (so called SI link). For every SI link, the susceptible is infected by his or her infective neighbor with fixed probability of τ per unit time. In additional, each infected individual recovers to susceptible at rate γ independently. Furthermore, since the susceptible individuals are likely to protect themselves, so the connections between susceptible and infected individuals are also being rewired adaptively during the spreading of the epidemic. Each SI link is rewired with probability w to connect the susceptible node to another randomly selected susceptible one. Assume that there are no self-loops and multiple connections between nodes to form in the rewiring process.
Let [S] and [I] denote the total number of susceptible and infected individuals in the adaptive network G. Moreover, [AB] and [ABC] represent the number of pairs of type A−B (with one member in state A, and the other member in state B) and the number of triples of type A−B−C (with one edge member in state A, the middle member in state B, and the other edge member in state C), respectively, where A,B,C∈S,I. Then we have the balanced relationships, [S]+[I]=N and [SS]+2[SI]+[II]=2K, with respect to the nodes and edges of the network, respectively. Hence the spreading process on an adaptive network can be described by the following SIS pairwise epidemic model
{˙[S]=γ[I]−τ[SI],˙[I]=τ[SI]−γ[I],˙[SI]=τ([SSI]−[ISI])−(τ+γ+w)[SI]+γ[II],˙[SS]=2γ[SI]+2w[SI]−2τ[SSI],˙[II]=−2γ[II]+2τ[ISI]+2τ[SI]. | (2.1) |
One can verify that ˙[S]+˙[I]=0 and ˙[SS]+2˙[SI]+˙[II]=0, which corresponds to the balance conditions of nodes and edges respectively. However, these equations (2.1) are unclosed and cannot be analyzed. The proceeds of the computation and analysis need to seek approximations that allow us to close these equations at a lower dimension. That is to say, in order to close the equations (2.1) it is necessary to approximate the triples [SSI] and [ISI] that appear. Keeling et. al. [15] proposed an approximate formula in terms of pairs and singles
[ABC]≈n−1n[AB][BC][B], | (2.2) |
where n is the average degree of the network, and A,B,C∈{S,I}. However, since all triples are formed from two pairs sharing a common central node, the approximation of triples depends on the degree of central node. Therefore Kiss et. al. [38] modified the equation (2.2) by replacing the average degree of the whole network with the group of central individuals
[ABC]≈kB−1kB[AB][BC][B], | (2.3) |
where kB denotes the expected average degree of central nodes with type B. In fact, all types of triples [SSI] and [ISI] are susceptible, therefore the average degree of susceptible individuals is only needed, which given by
kS=[SS]+[SI][S]. | (2.4) |
Henceforth, we use equation (2.3) to approximate the triple structures in the network. Furthermore, utilizing the balance conditions of constant number of nodes and connections, the system (2.1) is closed at the level of pairs and singles
{˙[I]=τ[SI]−γ[I],˙[SI]=τ[SS]+[SI]+[I]−N(N−[I])([SS]+[SI])[SI]([SS]−[SI])−(τ+γ+w)[SI]+γ(nN−2[SI]−[SS]),˙[SS]=2(γ+w)[SI]−2τ[SS]+[SI]+[I]−N(N−[I])([SS]+[SI])[SS][SI]. | (2.5) |
in which the derivations are taken with respect to t′. Therefore for biological feasibility, we just consider the system (2.5) in the following domain
Ω0={([I],[SI],[SS])∈R3+|0⩽[I]<N,0⩽2[SI]+[SS]⩽nN}. | (2.6) |
It is not difficult to verify that all of the solutions in the first octant will stay or enter inside the set Ω0.
Moreover, for simplicity of expression and analysis, we will rescale the model (2.5) into a dimensionless system. Let
x=[I]N,y=2[SI]nN,z=[SS]nN,t′=1γt,A1=τγ,A2=wγ, | (2.7) |
therefore system becomes into the following nondimensionalized model
{˙x=−x+A1ny2,˙y=A1y(2−2x−ny−2nz)(y−2z)2(1−x)(y+2z)−(A1+A2+3)y+2(1−z),˙z=y(A2+1+A1(2−2x−ny−2nz)z(1−x)(y+2z)), | (2.8) |
where the dots represent the derivatives with respect to time t.
Since the average degree n>1 holds, and according to (2.7), then one can define the parameter space as following
Λ={(A1,A2,n)|A1>0, A2>0, n>1}. | (2.9) |
Further, due to Ω0 is positive invariant of system (2.5), then we have
Ω={(x,y,z)∈R3+|x,y,z⩾0,x<1,y+z⩽1} | (2.10) |
is positive invariant of system (2.8).
In the rest of the paper, we will study the dynamics and bifurcations of the dimensionless system (2.8) in Ω with parameters in Λ.
We find equilibria by setting the right hand side of system (2.8) equal to zero. One trivial equilibrium point is the disease-free equilibrium (DFE), which is denoted by E0(0,0,1). The stability of the E0 is determined by the Jacobian,
J=[−112A1n00A1n−2A1−A2−3−20A1+A2+1−A1n0]. | (3.1) |
Evaluating the J on the disease-free equilibrium E0 yields the characteristic polynomial,
P0(λ)=(λ+1){λ2+[2A1+A2+3−A1n]λ+2(A1+A2+1−A1n)}. | (3.2) |
Then we define the reproduction number as
R0=A1nA1+A2+1. | (3.3) |
Then, E0 is stable (unstable) for R0<1 (R0>1), as expected.
Next, we discuss the existence of endemic equilibrium. As mentioned above, the solution according to the endemic equilibrium, x>0, is founded by letting the right side of the equations of the system (2.5) to zero. Then the coordinates of any endemic equilibrium E(x,y,z) satisfy
y(x)=2xA1n,z(x)=(A1+A2+1)(1−x)−x+√Δ12A1n, | (3.4) |
where
Δ1=[(A1+A2)2+4A1]x2−2[(A1+A2)2+3A1+A2]x+(A1+A2+1)2, |
it should be noted that Δ1>0 always holds since whose discriminant is −16A1(A2+1)<0, and we discard the other branch root of z(x)=(A1+A2+1)(1−x)−x−√Δ12A1n since it is negative. Define
f1(x):=A2x+A1n−(A1+A2+1). |
and
f2(x):=a2x2+a1x+a0, |
where
a2=A2(A1+A2+2),a0=(A1+A2+1)2(1−R0),a1=(n−1)A21+(A2(n−3)+2n−3)A1−2A2(A2+1). |
Then the coordinate x is a root of the following cubic polynomial equation
f2(x)=f1(x)√Δ1. | (3.5) |
By a direct calculation, we analyze the roots of following equivalent equation f(x) of (3.5) within the interval (0,1)
f(x)=b3x3+b2x2+b1x+b0=0withf1(x)f2(x)⩾0, | (3.6) |
where
b3=A22>0,b2=A2(2A1n−A1−2A2),b0=A1n(A1+A2+1)(1−R0),b1=(n2−n)A21−(3A2n−A2+1)A1+A2(A2+1). |
In order to obtain the exact solutions of equation (3.6) by utilizing the ShengJin's formula [39], then we have
A=b22−3b3b1,B=b2b1−9b3b0,C=b21−3b2b0, |
δ=√A3b3,h=2A√A27b23,xN=−b23b3,yN=2Ab2−3b3B27b23, |
Δ=y2N−h2=B2−4AC81b23. |
Because of the uncertainty of the sign of Δ, we list all the possible real roots formulas below:
Cases 1. Δ<0 (implies A>0), equation (3.6) has 3 different real roots
x11=xN+2δcosθ3=xN−2δcos(α3+2π3),x12=xN+2δcos(θ3+4π3)=xN−2δcos(α3+4π3),x13=xN+2δcos(θ3+2π3)=xN−2δcosα3, |
where θ=arccos−yNh, α=arccosyNh and x11>x12>x13.
Cases 2. Δ=0, equation (3.6) has 3 real roots,
(ⅰ) If A>0, two of which are repeated roots
x21=xN−23√yN2b3=−b2b3+BA,x22=x23=xN+3√yN2b3=−B2A. |
(ⅱ) If A=0, there are 3 equal roots at x1,2,3=xN.
Cases 3. Δ>0, the unique real root of equation (3.6) is
x31=xN+3√Y1+3√Y2, |
where Y1,2=−yN±√Δ2b3.
We denote the possible endemic equilibria by Eij(xij,y(xij),z(xij)) (i,j=1,2,3 and i⩾j).
For (A1,A2,n)∈Λ, f(1)=A1(n−1)+A2>0, f″(1)=2A2(A1(2n−1)+A2)>0, we have the following three cases about the existence of endemic equilibria.
(1) R0>1: In this case f(0)=b0<0. It is not difficult to derive that b2>A2(A1+2)>0, therefore f″(0)=2b2>0, then f″(x)>0 always holds when x∈(0,1), that is to say, f′(x) is monotone increasing in the interval (0,1). We discuss the roots of f(x) in the following situations:
(a) If b1⩾0, i.e. f′(0)⩾0, thus f′(x)>0 always holds when x∈(0,1), implying that f(x) is monotone increasing in the interval (0,1).
(b) If b1<0, i.e. f′(0)<0, on account of
f′(1)>A1A2n+A1(A2+1)(n−1)+A2>0,f″(0)>0, |
which guarantees x∗1∈(0,1). Therefore it is not difficult to derive that f(x) is decreasing and increasing monotonically in the interval (0,x∗1) and (x∗1,1), respectively.
Hence, system (2.8) has a unique endemic equilibrium whose x-coordinate is the largest real root of f(x).
(ⅰ) If Δ<0, the unique endemic equilibrium is E11(x11,y(x11),z(x11)) since x11>x12>x13;
(ⅱ) If Δ=0, equation (3.6) has 3 real roots, since b2 and b0 have opposite sign, then A=0 and Δ=0 cannot be simultaneously true, namely it is nonexistent of 3 equal roots. Hence, we just consider the case of A>0, i.e. there has 3 real roots, x21, x22=x23, two of which are repeated roots. According to the Vieta theorem
x21+2x22=−b2b3<0,x21x222=−b0b3>0, |
then we can obtain x21>0, x22=x23<0, therefore the unique endemic equilibrium is E21(x21,y(x21),z(x21)).
(ⅲ) If Δ>0, it is obviously that the unique endemic equilibrium is E31(x31,y(x31),z(x31)).
(2) R0=1: In this case f(0)=b0=0, b2=A2(A1+2)>0 and f(x)=x(b3x2+b2x+b1), recall that f(1)>0, therefore system (2.8) has a unique endemic equilibrium if and only if b1<0. Actually it is not difficult to prove that Δ<0 holds in this case, and the 3 different real roots are
x11=−b2+√b22−4b3b12b3>0,x12=0,x13=−b2−√b22−4b3b12b3<0 |
respectively. Thus the unique endemic equilibrium is E11(x11,y(x11),z(x11)).
(3) R0<1: In this case f(0)=b0>0, thus f(x)=0 has at least one negative real root, namely system (2.8) has up to two endemic equilibria. It is obvious that system (2.8) has no endemic equilibrium if b1⩾0 and b2⩾0 due to all of the coefficients of equation (3.6) are non-negative in this case. Therefore we just need to consider the existence of positive roots of f(x)=0 in (0,1) when b1<0 or b2<0. It is not difficult to derive that x∗1>0.
(a) Δ<0 implies A>0, f(x)=0 has 3 different roots which satisfy x13<x12<x11, then x13<0. Since f(x) is monotonically decrease and increase at the interval (x∗2,x∗1) and (x∗1,+∞) respectively, thus x12<x∗1<x11. According to the Vieta theorem
x11x12x13=−b0b3<0, |
then x11 and x12 have the same sign, we have 0<x12<x11. On the other hand, 1∈[x12,x11] is false due to f(1)>0, that is to say either 1<x12<x∗1<x11 or x12<x∗1<x11<1, then it is not difficult to derive that the latter case holds if and only if x∗1<1 which is equivalent to f′(1)>0 since f″(1)>0. Above all, f(x)=0 has 2 different roots satisfying 0<x12<x11<1 if and only if x∗1<1 (i.e. f′(1)>0), otherwise there are no roots in the interval (0,1). System (2.8) has 2 endemic equilibria E11(x11,y(x11),z(x11)) and E12(x12,y(x12),z(x12)).
(b) Δ=0 implies A>0 (otherwise one can get a contradiction b1>0 and b2>0). f(x)=0 has 3 roots x21<0 and x22=x23=x∗1>0. To sum up, f(x)=0 has 2 roots satisfying 0<x22=x23<1 if and only if x∗1<1 (i.e. f′(1)>0), otherwise there are no roots in the interval (0,1). System (2.8) has 2 identical endemic equilibria E22(x22,y(x22),z(x22)) which x22=x23=x∗1>0.
(c) Δ>0. It is obviously that f(x)=0 has a unique real root x31<0, there are no roots in the interval (0,1).
Note that if b1<0 or b2<0 and A>0, one have f(x∗2)⩾f(0)>0 since x∗1>0 and f(x) is monotonically increase and decrease at the interval (−∞,x∗2) and (x∗2,x∗1) respectively. Therefore the sign of Δ is completely consistent with f(x∗1) because of Δ=f(x∗2)f(x∗1).
We summarize the result above in the following theorem.
Theorem 3.1. For system (2.8) with (A1,A2,n)∈Λ and (x,y,z)∈Ω, f(1)=A1(n−1)+A2>0, f″(1)=2A2(A1(2n−1)+A2)>0,
(1) The disease-free equilibrium E0 always exists.
(2) When R0>1, there exists a unique endemic equilibrium which is (a) E11 when Δ<0; (b) E21 when Δ=0; (c) E31 when Δ>0.
(3) When R0=1, there exists a unique endemic equilibrium E11 if and only if b1<0.
(4) When R0<1, and
(a) If b1⩾0 and b2⩾0 or f′(1)⩽0, there is no endemic equilibrium.
(b) If b1<0 or b2<0 and f′(1)>0, system (2.8) has two endemic equilibria E11 and E12 if and only if Δ<0(implies A>0), and these two equilibria coalesce into one endemic equilibrium E11=E12=E22 if and only if Δ=0(implies A>0). Otherwise, system (2.8) has no endemic equilibrium.
We primarily study the stability of disease-free equilibrium E0 in this subsection. By considering the distribution of the roots of the characteristic equation (3.2) of Jacobian at disease-free equilibrium E0, one can obtain E0 is stable (unstable) for A1+A2+1>A1n (A1+A2+1<A1n). Then the disease-free equilibrium E0 is locally asymptotically stable (resp. unstable) for R0<1 (resp. R0>1).
We determine the types of disease-free equilibrium E0(1,0,1) in the following.
Theorem 3.2. For the system (2.8), the disease free equilibrium E0(1,0,1) is
(a) R0<1: an attracting node;
(b) R0>1: an hyperbolic saddle;
(c) R0=1 and
(ⅰ) A2(A1+A2)>1: a saddle-node of codimension 1;
(ⅱ) A2(A1+A2)<1: a saddle-node of codimension 1;
(ⅲ) A2(A1+A2)=1: an attracting semi-hyperbolic node of codimension 2.
Proof. For the system (2.8), form the characteristic equation (3.2), it is not difficult to obtain that E0 is an attracting node if R0<1, and E0 is an hyperbolic saddle if R0>1.
If R0=1, the eigenvalues of Jacobian at E0 are −1, −(A1+2) and 0. To determine the type of E0, we linearize the system (2.8) at E0 and diagonalize the linear part, then we obtain the following system
˙X=−2(A22+A1A2−1)(A1+2)2X2+X⋅O(∣Y,Z∣)+O(∣Y,Z∣2)+O(∣X,Y,Z∣3),˙Y=−Y+O(∣X,Y,Z∣2),˙Z=−(A1+2)Z+O(∣X,Y,Z∣2), | (3.7) |
Therefore if A2(A1+A2)≠1, it is unnecessary to calculate the center manifold, and E0 is a saddle-node of codimension 1.
If A2(A1+A2)=1, according to the center manifold theorem, there exists a center manifold for system (3.7), which have the following form
Y=A22(A2+1)2(A22−A2−1)(A22−2A2−1)2X2+O(∣X∣3),Z=2A32(A2+1)(A22−2A2−1)3X2+O(∣X∣3), |
then one can obtain
˙X=−2A22(A2+1)(A22−2A2−1)2X3+O(∣X∣4), | (3.8) |
so E0 is an attracting semi-hyperbolic node of codimension 2.
Owing to the complexity of the expressions of the equilibria, in this section we launch into a detailed discussion of the effect of adaptive behavior on the epidemic spreading through numerical simulations, including stabilities, backward bifurcation, saddle-node bifurcation and Hopf bifurcation, etc. In order to study the effect of topology of adaptive networks aroused by rewiring rate w and average degree n, infection rate τ and recovery rate γ on disease transmission respectively, we perform the numerical simulation of the system (2.9) based on the original parameter instead of A1 and A2. From the analysis (2.7) and (3.3) in Sections 2 and 3, the basic reproduction number can be represented as
R0=A1nA1+A2+1=τnτ+w+γ. | (4.1) |
First, we simulate the time series of total densities of infected individuals on adaptive networks based on the different values of rewiring rate w by fixing the other three parameters τ=0.008, γ=0.002 and n=10. In Figure 1(a), (d) and (g), we choose w=0.004, which corresponding to A1=4 and A2=2, in this case the basic reproduction number R0=5.714; In Figure 1(b), (e) and (h), we choose w=0.04, which corresponding to A1=4 and A2=20, in this case the basic reproduction number R0=1.600; In Figure 1(c), (f) and (i), we choose w=0.4, which corresponding to A1=4 and A2=200, in this case the basic reproduction number R0=0.195; We can see that if R0<1, the disease will disappear (see Figure 1(c)), otherwise R0>1 the endemic equilibrium will stable (see Figures 1(a), (b)). Furthermore from Figures 1(a) and (b), we can see that the infected individuals tends to the same level even though the different initial values x(0)=0.96 (red line), x(0)=0.55 (green line) and x(0)=0.20 (blue line) are selected, from which the infected individuals tends to zero, namely the disease extinction is determined by the basic reproduction number R0 in this case. In the other hand, the disease will persist or extinct when the rewiring rate w is lower or higher, respectively.
Next we show the backward bifurcation, saddle-node bifurcation and Hopf bifurcation can really happen by plotting the relations of infected individuals versus parameters τ and w respectively, as shown in Figure 2. One can see that if R0>1, there is an unique endemic equilibrium, however 2 endemic equilibria appear at R0=1 and disappear when coalesce into one endemic equilibrium with multiplicity 2, namely backward bifurcation occurs when R0=1 and disappears at the critical point LP of saddle-node bifurcation. Since R0 is monotonically decrease (or increase) with the increasing of w (or τ), the backward bifurcation curves are opening left and right in Figures 2(a) and 2(b) respectively. Comparing to Figure 2(a) and 2(b), it is different that there is a unique endemic equilibrium when the rewiring rate w is small or the infection rate τ is large. In addition 2 endemic equilibria appear at R0=1 in common, the larger one is stable initially (red solid line)and the lower one is always a saddle node(blue dashed line).
We continue to explore the the bistability and oscillations of the system. In Figure 2(a), we can also see that there have a critical point HP across which the stability of endemic equilibrium with larger epidemicity is changed, then we can observe Hopf bifurcation bifurcating from critical point HP and disappears when w is large (Figure 2(a)) or τ is small (Figure 2(b)). Since the disease-free equilibrium E0 is stable when R0<1, then the bistability occurs from the R0=1 and disappears at the critical point HP, namely both the larger endemic equilibrium and disease-free equilibrium are stable. Furthermore, limit cycle will arise near the critical point HP, i.e. the periodic oscillations can occurs for the system (2.8). To conform above conjectures, we fix two parameters γ=0.002 and τ=0.008, and choose w=0.3044 close to the HP point in Figure 2(a), the phase portrait of system is shown in Figure 3. In this case the disease-free equilibrium E0 is stable node and the larger endemic equilibrium is an unstable focus. Both the orbits of blue and green curve with initial conditions P1(0.8,0.04,0.15) and P3(0.7,0.12,0.15) spiral outward (or inward) to a same stable limit cycle. The orbit (red curve) with initial condition P2(0.85,0.05,0.10) spirals outward to the disease-free equilibrium E0. There this example have proved that the system has a stable limit cycle, see Figure 3.
Furthermore we show the Hopf bifurcation will really happen through a concrete example in this Subsection. In additional, the type of Hopf bifurcation(supercritical or subcritical) is also determined by calculating the first Liapunov coefficient. By selecting the parameters γ=0.002, τ=0.008 and n=10, by a direct calculation one can obtain the critical value of rewiring rate at HP point is w=0.3008852. In this case the larger endemic equilibrium is E1(0.9687103,0.04843551,0.1210720).
In order to derive the norm form of Hopf bifurcation, we draw into the following affine transformation,
(xyz)=(0.96871030.048435510.1210720)+[−0.0020.0400.3833287−0.13228180.1189486−0.4791774−0.001111213−0.1233932](uvw), | (4.2) |
where the columns of the nonsingular transformation matrix are the eigenvectors of Jacobian matrix of system (2.8) at E1 with selected parameters, corresponding to the eigenvectors of λ1,2=±0.0404113i and λ3=−0.2576751, respectively. We obtain the following normal form dynamical system,
{˙u=−0.0404113v+∑i+j+k=2aijkuivjwk,˙v=0.0404113u+∑i+j+k=2bijkuivjwk,˙w=−0.2576751w+∑i+j+k=2cijkuivjwk, | (4.3) |
where
a002=−2.135144,a101=1.141062,a011=0.8192872,a200=−0.06860938,a110=0.09381623,a020=0.2130411,b002=0.6924218,b101=−0.2467929,b011=−0.03582535,b200=−0.034729964,b110=−0.2051875,b020=−0.1968319,c002=1.580789,c101=−1.224142,c011=−1.314055,c200=0.2261677,c110=0.4684255,c020=0.2354379, |
A direct calculation to obtain the center manifold which is
w=h(u,v)=−0.1653248u2+0.6436124uv+0.7258436v2+o(ρ2),ρ=√u2+v2, |
then the system restricted to the center manifold is given by
{˙u=−0.04041138v+F1(u,v)+O(∣u,v∣4),˙v=0.04041138u+F2(u,v)+O(∣u,v∣4), | (4.4) |
where
F1(u,v)=0.3072829u3+0.4645974v3+1.508608u2v+1.820306uv2−0.03472996u2−0.2051875uv−0.1968319v2,F2(u,v)=−1.539753u3−1.550427v3−4.755158u2v−4.737570uv2+0.2261677u2+0.4684255uv+0.2354379v2, |
Thus from the Ref [40,41] we can calculate the first Liapunov coefficient of system L1 which is
L1=116[∂3F1∂u3+∂3F1∂u∂v2+∂3F2∂u2∂v+∂3F2∂v3]+116√|Im(λ1,2)|[∂2F1∂u∂v(∂2F1∂u2+∂2F1∂v2)−∂2F2∂u∂v(∂2F2∂u2+∂2F2∂v2)−∂2F1∂u2∂2F2∂u2+∂2F1∂v2∂2F2∂v2]|u=v=0=−1.592995<0, | (4.5) |
Therefore supercritical Hopf bifurcation occurs near the critical point HB which is consistent with the numerical simulation in Figure 3.
For the purpose of assessing the impact of adaptive behavior on the epidemic spreading, a new triples approximation formula incorporating the effect of center nodes' degree of triples is proposed, by which the SIS epidemic model on adaptive complex networks is closed. We first transform the model into an equivalent nondimensionalized three dimensional system, and the existence of equilibria is derived. Then the basic reproduction number R0 is obtained through the analysis of the stabilities of disease-free equilibrium E0. In addition, the conditions of the existence of endemic equilibria are analyzed strictly. By the qualitative theory and the stability theory of ordinary differential equations, the local stabilities of the endemic equilibria are proved. Moreover, using the methods of bifurcation theory, we show that the model exhibits rich and complicated dynamics such as transcritical forward bifurcation, backward bifurcation, saddle-node bifurcation. Besides, owing to the complexity, we calculate the normal form of Hopf bifurcation numerically, and the first Lyapunov coefficient is derived to determine the type of Hopf bifurcation (supercritical or subcritical). Then we show that a stable limit cycle can bifurcate from the endemic equilibrium with larger epidemicity, and the numerical simulations which is consistent with the analytical results are given.
The topological structure of adaptive network has obvious significance on epidemic spreading in theory, i.e. adaptive behavior can lead to rich dynamics. At the point of the application, on account of that backward bifurcation implies that the the basic reproduction number R0<1 is not enough to control the spread of infectious diseases any more, and Hopf bifurcation means the oscillations will occur, therefore adaptive behavior raises the difficulty of prevention and control of infectious diseases. Keeling et al. [14,16] studied the spread of the childhood epidemics and sexually transmitted diseases by moment closure epidemic model without adaptive behaviors, and they show that the models have no rich dynamics. Therefore, this paper show that adaptive topology is an important reason of periodic outbreaks of epidemic as well as the nonlinear incidence rate and recovery rate in well-mixing epidemic models, which expands people's understanding on the epidemic oscillation in theory. And in application, by controlling the parameters in a 'safe' region to break the occurrence the bifurcations, the research on this paper can provide a new guidance to prevention and control of diseases.
This work is supported by the National Natural Science Foundation of China under Grant Nos.11671241, 11601294, 61803242, 11501340, 11801340, the Youth Science Fund of Shanxi Province under Grant Nos. 201801D221001, 201801D221011, 201601D021012, 201801D221007 and Shanxi Scholarship Council of China under Grant No. 2016-011.
All authors declare no conflicts of interest in this paper.
[1] | F. Brauer and C. Castillo-Chavez, Mathematical models in population biology and epidemiology, New York: Springer, 2001. |
[2] | R. Pastor-Satorras and A. Vespignani, Epidemic dynamics and endemic states in complex networks, Physic. Rev. E, 63 (2001), 649–667. |
[3] | R. Pastor-Satorras and A. Vespignani, Epidemic dynamics in finite size scale-free networks, Physic. Rev. E, 65 (2002), 035108. |
[4] | M. Boguñá and R. Pastor-Satorras, Epidemic spreading in correlated complex networks, Physic. Rev. E, 66 (2002), 047104. |
[5] | P. V. Mieghem, J. Omic and R. Kooij, Virus spread in networks, IEEE ACM T. Network, 17 (2009), 1–14. |
[6] | L. X. Yang, M. Draief and X. F. Yang, Heterogeneous virus propagation in networks: a theoretical study, Math. Method Appl. Sci , 40 (2017), 1396–1413. |
[7] | L. X. Yang, X. F. Yang and Y. Wu, The impact of patch forwarding on the prevalence of computer virus: A theoretical assessment approach, Appl. Math. Model, 43 (2017), 110–125. |
[8] | L. X. Yang, X. F. Yang and Y. Y. Tang, A Bi-Virus Competing Spreading Model with Generic Infection Rates, IEEE Transact. Netw. Sci. Eng., 5 (2018), 2–13. |
[9] | X. Zhang, G. Q. Sun, Y. X. Zhu, et al., Epidemic dynamics on semi-directed complex networks, Math. Biosci., 246 (2013), 242–251. |
[10] | J. Y. Yang, Y. M. Chen and F. Xu, Efect of infection age on an SIS epidemic model on complex networks, J. Math. Biol., 73 (2016), 1227–1249. |
[11] | X. L. Peng, Z. Q. Zhang, J. Y. Yang, et al., An SIS epidemic model with vaccination in a dynamical contact network of mobile individuals with heterogeneous spatial constraints, Commun. Nonlinear Sci., 73 (2019), 52–73. |
[12] | G. Q. Sun, C. H. Wang, L. L. Chang, et al., Efects of feedback regulation on vegetation patterns in semi-arid environments, Appl. Math. Model., 61 (2018), 200–215. |
[13] | G. Q. Sun, M. Jusup, Z. Jin, et al., Pattern transitions in spatial epidemics: Mechanisms and emergent properties, Phys. Life Rev., 19 (2016), 43–73. |
[14] | M. J. Keeling, D. A. Rand and A. J. Morris, Correlation models for childhood epidemics, P. Roy. Soc. B-Biol. Sci., 264 (1385), 1149–1156. |
[15] | M. J. Keeling, The efects of local spatial structure on epidemiological invasions, Proc. R. Soc. Lond. B., 266 (1999), 859–867. |
[16] | K. T. D. Eames and M. J. Keeling, Monogamous networks and the spread of sexually transmitted diseases, Math. Biosci., 189 (2004), 115–130. |
[17] | Y.Wang, J. D. Cao, A. Alsaedi, et al., Edge-based SEIR dynamics with or without infectious force in latent period on random networks, Commun. Nonlinear Sci., 45 (2017), 35–54. |
[18] | Y. Wang, J. D. Cao, X. D. Li, et al., Edge-based epidemic dynamics with multiple routes of transmission on random networks, Nonlinear Dynam., 91 (2018), 403–420. |
[19] | Y.Wang, J. D. Cao and M. Q. Li, et al., Global behavior of a two-stage contact process on complex networks, J. Franklin I., In Press. |
[20] | J. Y. Yang and F. Xu, The coumputational approach for the basic reproduction number of epidemic models on complex networks, IEEE Access, 2019, 10.1109/ACESS2019.2898639. |
[21] | L. Li, C. H. Wang, S. F. Wang, et al., Hemorrhagic fever with renal syndrome in China: Mechanisms on two distinct annual peaks and control measures, Int. J. Biomath., 11 (2018), 1850030. |
[22] | L. Li, J. Zhang, C. Liu, et al., Analysis of transmission dynamics for Zika virus on networks, Appl. Math. Comput., 347 (2019), 566–577. |
[23] | D. J.Watts and S. H. Strogatz, Collective dynamics of 'small-world' networks, Nature, 393 (1998), 440–442. |
[24] | A. L. Barabási and R. Albert, Emergence of scaling in random networks, Science, 286 (1999), 509–512. |
[25] | R. M. May and A. L. Lloyd, Infection dynamics on scale-free networks, Physic. Rev. E, 64 (2001), 066112. |
[26] | V. M. Eguiluz and K. Klemm, Epidemic threshold in structured scale-free networks, Physic. Rev. Lett., 89 (2002), 108701. |
[27] | M. Boguna, R. Pastor-Satorras, A. Vespignani, Absence of epidemic threshold in scale-free networks with degree correlations, Physic. Rev. Lett., 90 (2003), 028701. |
[28] | H. Zhang, P. Shu, Z.Wang, et al., Preferential imitation can invalidate targeted subsidy policies on seasonal-influenza diseases, Appl. Math. Comput., 294 (2017), 332–342. |
[29] | T. Gross, C. J. D. D'Lima and B. Blasius, Epidemic dynamics on an adaptive network, Physic. Rev. Lett., 96 (2006), 208701. |
[30] | T. Gross and B. Blasius, Adaptive coevolutionary networks: a review, J. R. Soc. Inter., 5 (2008), 259–271. |
[31] | X. Zhang, C. Shan, Z. Jin, et al., Complex dynamics of epidemic models on adaptive networks, J. Difer. Equa., 266 (2019), 803–832. |
[32] | L. B. Shaw and I. B. Schwartz, Fluctuating epidemics on adaptive networks, Physic. Rev. E, 77 (2008), 066101. |
[33] | D. H. Zanette and S. Risau-Gusman, Infection Spreading in a Population with Evolving Contacts, J. Biol. Phys., 34 (2008), 135–148. |
[34] | Y. Schwarzkopf, A. Ráos and D. Mukamel, Epidemic spreading in evolving networks, Physic. Rev. E, 82 (2010), 036112. |
[35] | T. Rogers, W. Cliford-Brown, C. Mills, et al., Stochastic oscillations of adaptive networks: application to epidemic modelling, J. Stat. Mech. Theory. E, 8 (2012), 1–15. |
[36] | D. Juher, J. Ripoll and J. Saldaña, Outbreak analysis of an SIS epidemic model with rewiring, J. Math. Biol., 67 (2013), 411–432. |
[37] | J. Zhou, G. Xiao, S. A. Cheong, et al., Epidemic reemergence in adaptive complex networks, Physic. Rev. E, 85 (2012), 036107. |
[38] | A. Szabó-Solticzky, L. Berthouze, I. Z. Kiss, et al., Oscillating epidemics in a dynamic network model: stochastic and mean-field analysis, J. Math. Biol., 72 (2016), 1153–1176. |
[39] | S.J. Fan, A new extracting formula and a new distinguishing means on the one variable cubic equation, Nat. Sci. J. Hainan Teacheres Coll., 2 (1989), 91–98 (in Chinese). |
[40] | S. N. Chow and J. K. Hale, Methods of bifurcation theory, Springer Science & Business Media, 2012. |
[41] | L. Perko, Diferential equations and dynamical systems, Springer Science & Business Media, 2013. |