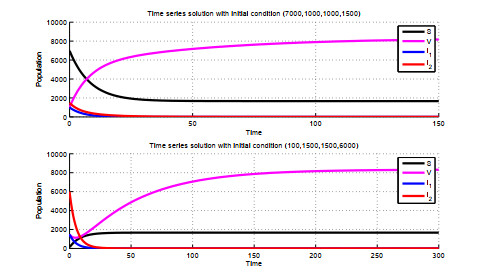
Black Americans (AA) face a confluence of challenges when seeking care including unaffordable costs, negative experiences with providers, racism, and distrust in the healthcare system. This study utilized linear regressions and mediation analysis to explore the interconnectedness of these challenges within a community-based sample of 313 AA women aged 45 and older. Approximately 23% of participants reported affordability problems, while 44% had a negative experience with a provider. In the initial linear regression model excluding perceived racism, higher levels of distrust were observed among women reporting affordability problems (β = 2.66; p = 0.003) or negative experiences with a healthcare provider (β = 3.02; p = <0.001). However, upon including perceived racism in the model, it emerged as a significant predictor of distrust (β = 0.81; p = < 0.001), attenuating the relationships between affordability and distrust (β = 1.74; p = 0.030) and negative experience with a provider and distrust (β = 1.79; p = 0.009). Mediation analysis indicated that perceived racism mediated approximately 35% and 41% of the relationships between affordability and distrust and negative experience with a provider and distrust, respectively. These findings underscore the critical imperative of addressing racism in the efforts to mitigate racial disparities in healthcare. Future research should explore the applicability of these findings to other marginalized populations.
Citation: Jacqueline Wiltshire, Carla Jackie Sampson, Echu Liu, Myra Michelle DeBose, Paul I Musey, Keith Elder. Affordability, negative experiences, perceived racism, and health care system distrust among black American women aged 45 and over[J]. AIMS Public Health, 2024, 11(4): 1030-1048. doi: 10.3934/publichealth.2024053
[1] | Yansong Pei, Bing Liu, Haokun Qi . Extinction and stationary distribution of stochastic predator-prey model with group defense behavior. Mathematical Biosciences and Engineering, 2022, 19(12): 13062-13078. doi: 10.3934/mbe.2022610 |
[2] | Qi Zhou, Huaimin Yuan, Qimin Zhang . Dynamics and approximation of positive solution of the stochastic SIS model affected by air pollutants. Mathematical Biosciences and Engineering, 2022, 19(5): 4481-4505. doi: 10.3934/mbe.2022207 |
[3] | Yajuan Guo, Zijian Liu, Yuanshun Tan, Yawei Liu . Modeling and analysis of a stochastic giving-up-smoking model with quit smoking duration. Mathematical Biosciences and Engineering, 2023, 20(12): 20576-20598. doi: 10.3934/mbe.2023910 |
[4] | Dwi Lestari, Noorma Yulia Megawati, Nanang Susyanto, Fajar Adi-Kusumo . Qualitative behaviour of a stochastic hepatitis C epidemic model in cellular level. Mathematical Biosciences and Engineering, 2022, 19(2): 1515-1535. doi: 10.3934/mbe.2022070 |
[5] | Tingting Xue, Long Zhang, Xiaolin Fan . Dynamic modeling and analysis of Hepatitis B epidemic with general incidence. Mathematical Biosciences and Engineering, 2023, 20(6): 10883-10908. doi: 10.3934/mbe.2023483 |
[6] | Yiping Tan, Yongli Cai, Zhihang Peng, Kaifa Wang, Ruoxia Yao, Weiming Wang . Dynamics of a stochastic HBV infection model with drug therapy and immune response. Mathematical Biosciences and Engineering, 2022, 19(8): 7570-7585. doi: 10.3934/mbe.2022356 |
[7] | Shengnan Zhao, Sanling Yuan . A coral reef benthic system with grazing intensity and immigrated macroalgae in deterministic and stochastic environments. Mathematical Biosciences and Engineering, 2022, 19(4): 3449-3471. doi: 10.3934/mbe.2022159 |
[8] | Ying He, Junlong Tao, Bo Bi . Stationary distribution for a three-dimensional stochastic viral infection model with general distributed delay. Mathematical Biosciences and Engineering, 2023, 20(10): 18018-18029. doi: 10.3934/mbe.2023800 |
[9] | David F. Anderson, Tung D. Nguyen . Results on stochastic reaction networks with non-mass action kinetics. Mathematical Biosciences and Engineering, 2019, 16(4): 2118-2140. doi: 10.3934/mbe.2019103 |
[10] | Ying He, Yuting Wei, Junlong Tao, Bo Bi . Stationary distribution and probability density function analysis of a stochastic Microcystins degradation model with distributed delay. Mathematical Biosciences and Engineering, 2024, 21(1): 602-626. doi: 10.3934/mbe.2024026 |
Black Americans (AA) face a confluence of challenges when seeking care including unaffordable costs, negative experiences with providers, racism, and distrust in the healthcare system. This study utilized linear regressions and mediation analysis to explore the interconnectedness of these challenges within a community-based sample of 313 AA women aged 45 and older. Approximately 23% of participants reported affordability problems, while 44% had a negative experience with a provider. In the initial linear regression model excluding perceived racism, higher levels of distrust were observed among women reporting affordability problems (β = 2.66; p = 0.003) or negative experiences with a healthcare provider (β = 3.02; p = <0.001). However, upon including perceived racism in the model, it emerged as a significant predictor of distrust (β = 0.81; p = < 0.001), attenuating the relationships between affordability and distrust (β = 1.74; p = 0.030) and negative experience with a provider and distrust (β = 1.79; p = 0.009). Mediation analysis indicated that perceived racism mediated approximately 35% and 41% of the relationships between affordability and distrust and negative experience with a provider and distrust, respectively. These findings underscore the critical imperative of addressing racism in the efforts to mitigate racial disparities in healthcare. Future research should explore the applicability of these findings to other marginalized populations.
Seasonal influenza is an acute respiratory infection caused by influenza viruses. Worldwide, these annual epidemics are estimated to result in about 3 to 5 million cases of severe illness, and about 290,000 to 650,000 respiratory deaths [1]. This infection can have an endemic, epidemic or pandemic behavior.
There were, three major flu pandemics during the 20th century, the so-called Spanish flu (H1N1) in 1918 was the most devastating pandemic. It has been estimated that the Spanish flu claimed around 40–50 million deaths (as much as 3% of the total population), and it also infected 20–40% of the whole population. In 1957–1958, the Asian flu or bird flu pandemic (H2N2) caused more than two million deaths [2]. Unlike the Spanish flu, this time the infection-causing virus was detected earlier due to the advancement of science and technology. A vaccine was made available but with limited supply. After a decade (in 1968), a flu pandemic (H3N2) that originated again in Hong Kong hit mankind. That flu pandemic also claimed one million lives. In 2009, the H1N1 swine flu is one of the more publicized pandemics that attracted the attention of all scientists and health professionals in the world and made them very much concerned. However, the pandemic did not result in great casualties like before. As of July 2010, only about 18,000 related deaths had been reported [2]. Besides the 4 influenza pandemics since 1918, annual seasonal influenza epidemics have spread among nations on smaller scales. There are many methods of preventing the spread of infectious disease, one of them is vaccination. Vaccination is the administration of agent-specific, but relatively harmless, antigenic components that in vaccinated individuals can induce protective immunity against the corresponding infectious agent [3].
Influenza causes serious public-health problems around the world, therefore, we need to understand transmission mechanisms and control strategies. Mathematical models also provided insight into the severity of past influenza epidemics. Some models were used to investigate the three most devastating historical pandemics of influenza in the 20th century [4,5,6]. There are a lot of pathogens with several circulating strains.
An important factor when analyzing the dynamics of a disease is the way in which it is transmitted from an infected individual to a healthy one. The incidence rate of a disease is defined as the number of susceptible individuals that become infected per unit of time. It measures the number of new cases of a disease in a period of time. There are different types of incidence functions that have been used in literature in order to model the force of infection of a disease. For example, Rahman and Zou [2] used the bilinear incidence rate βSI. However, there are more realistic incidence rates than the bilinear incidence rate, For instance, Capasso and his co-workers observed in the seventies [7] that the incidence rate may increase more slowly as I increases, so they proposed a saturated incidence rate βIS1+ζI.
Baba and Hincal [8] studied an epidemic model consisting of three strains of influenza (I1, I2, and I3) where we have vaccine for strain 1 (V1) only, and force of infection βSI1+ζS for strain 2. Baba et al. [9] studied an epidemic model consisting of two strains of influenza (I1 and I2) where force of infection βSI21+ζI22 for strain 2. As models with more general incidence functions are considered, the dynamics of the system become more complicated. Models with incidence functions of the form g(I)h(S) have been studied, such as [10]. In the most general case, the transmission of the disease may be given by a non-factorable function of S and I.
In this paper, our purpose is to study model considered in [2] modifying the force of infection in the compartments I1 and I2, by extending the incidence function to a more general form F(S,I), which is based on the incidence rate studied in [11]. Our main aim is to mathematically analyze the effect of the vaccine for strain 1, the general incidence rate of strain 1 (F1(S,I1)), and the general incidence rate of strain 2 (F2(S,I2)) on the dynamics of the model (2.2).
This paper is organized as follows. In section 2.1, we formulate the model. In section 3.1, we investigate the disease dynamics described by the model. In section 3.2, we calculate the basic reproduction number. In section 3.3, we establish the existence of equilibrium points. In section 3.4, we study the stability of the model. In section 3.5, provides some numeric simulations to illustrate our main theoretical results. The paper ends with some remarks.
Rahman and Zou [2] proposed a two-strain model with a single vaccination, namely.
˙S=Λ−(β1I1+β2I2+λ)S˙V1=rS−(μ+kI2)V1˙I1=β1I1S−α1I1˙I2=β2I2S+kI2V1−α2I2˙R=γ1I1+γ2I2−μR. | (2.1) |
where λ=r+μ, α1=γ1+v1+μ, α2=γ2+v2+μ. The compartments are S(t), V1(t), I1(t), I2(t) and R(t) which denote the population of susceptible, vaccine of strain 1, infective with respect to strain 1, infective with respect to strain 2 and removed individuals at time t, respectively. We assume that all the parameters are positive constants that can be interpreted as follows:
● Λ is the birth rate.
● μ is the death rate.
● r is the rate of vaccination with strain 1.
● k is the transmission coefficient of vaccinated individuals to strain 2.
● β1 is the transmission coefficient of susceptible individuals to strain 1.
● β2 is the transmission coefficient of susceptible individuals to strain 2.
● 1γ1 is the average infection period of strain 1.
● 1γ2 is the average infection period of strain 2.
● v1 is the infection-induced death rate of strain 1.
● v2 is the infection-induced death rate of strain 2.
The modification of the model (2.1) is given by the following system:
˙S=Λ−F1(S,I1)−F2(S,I2)−λS˙V1=rS−(μ+kI2)V1˙I1=F1(S,I1)−α1I1˙I2=F2(S,I2)+kI2V1−α2I2˙R=γ1I1+γ2I2−μR. | (2.2) |
Whose state space is R5+={(S,V1,I1,I2,R):S≥0,V1≥0,I1≥0,I2≥0,R≥0} and subject to the initial conditions S(0)=S0≥0, V1(0)=V10≥0, I1(0)=I10≥0, I2(0)=I20≥0 and R(0)=R0≥0.
We make the following hypotheses on Fi, i=1,2.:
H1) Fi(S,Ii)=Iifi(S,Ii) with Fi, fi∈C2(R2+→R+) and F(0,Ii)=F(S,0)=0 for all S,Ii≥0.
H2) ∂fi∂S(S,Ii)>0 and ∂fi∂Ii(S,Ii)≤0 for all S,Ii≥0.
H3) limIi→0+Fi(S,Ii)Ii exists and is positive for all S>0.
The first of this hypotheses is a basic requirement for any biologically feasible incidence rate, since the disease cannot spread when the number of susceptible or infected individuals is zero.
As for (H2), the condition ∂fi∂S(S,Ii)>0 ensures the monotonicity of fi(S,Ii) on S, while ∂fi∂Ii(S,I)≤0 suggests that Fi(S,Ii)Ii is non-increasing with respect to Ii. In the case when fi monotonically increases with respect to both variables and is concave with respect to Ii, the hypothesis (H2) naturally holds. Concave incidence functions have been used to represent the saturation effect in the transmission rate when the number of infected is very high and exposure to the disease is virtually certain.
(H3) is needed only to ensure that the basic reproduction number is well defined. Some examples of incidence functions studied in the literature that satisfy (H1)–(H3) are as follows:
(C1) F(S, I) = βSI [2].
(C2) F(S, I) = βSI1+ζS, where ζ≥0 describes the psychological effect of general public towards the infective [8].
(C3) F(S, I) = βSI1+ζI2, where ζ≥0 measures the psychological or inhibitory effect of the population [9].
A more thorough list can be found in [11]. It should be noted that model (2.2) extends as well as generalizes many special cases.
Lemma 1. Under the initial value (S0,V10,I10,I20,R0)∈R5+ the system (2.2) has a unique positive and bounded solution in R5+ for t>0. All solutions ultimately enter and remain in the following bounded and positively invariant region
Ω={(S,V1,I1,I2,R)∈R5+|N=S+V1+I1+I2+R≤Λμ}. |
Proof. The right hand side of system (2.2) is continuous and satisfies the Lipschitz condition in R5+. Then the system (2.2) has a unique solution (S(t,V1(t),I1(t),I2(t),R(t)) in [0,tm) for some tm>0. Adding all equations in (2.2), the total population N=S+V1+I1+I2+R satisfies:
˙N=˙S+˙V1+˙I1+˙I2+˙R=Λ−μS−μV1−μI1−μI2−μR−v1I1−v2I2≤Λ−μ(S+V1+I1+I2+R)=Λ−μN. |
The comparison theorem implies that limt→∞supN(t)≤Λμ. Hence N(t) is bounded and so are all components S(t), V1(t), I1(t), I2(t) and R(t). This in turn shows that the solution exists globally, i.e. for all t≥0. Consequently, the solutions S(t), V1(t), I1(t), I2(t), R(t) of (2.2) are ultimately bounded in the positively invariant region Ω.
Let (S(t), V1(t), I1(t), I2(t), R(t)) be a solution of system (2.2) with positive initial conditions. Assume by contradiction that there exists t>0 such that S(t)≤0, V1(t)≤0, I1(t)≤0, I2(t)≤0 or R(t)≤0. By continuity of solutions, this implies that there is a minimal t0>0 such that S(t0), V1(t0), I1(t0), I2(t0) or R(t0) is zero.
If S(t0)=0, then ˙S=Λ>0 at t0, so S is increasing in a neighbourhood (t0−ϵ, t0+ϵ) of t0. Thus S(t0−ϵ2)<S(t0)=0, and since S(0)>0 and S(t0−ϵ2)<0, there exists a t1∈(0, t0−ϵ2) with S(t1)=0. But t1<t0, which contradicts the minimality of t0, then S(t0)>0.
If V1(t0)=0, then ˙V1=rS(t0)>0 at t0. So V1 is increasing in a neighbourhood (t0−ϵ, t0+ϵ) of t0. Thus V1(t0−ϵ2)<V1(t0)=0, and since V1(0)>0 and V1(t0−ϵ2)<0, there exists a t1∈(0, t0−ϵ/2) with V1(t1)=0. But t1<t0, which contradicts the minimality of t0, then V1(t0)>0.
If I1(t0)=0, then ˙I1=0 at t0. On the other hand, any solution with I1(0)=0 satisfies I(t)=0 for all t>0. Since I1(0)>0 and I1(t0)=0, this contradicts the uniqueness of solutions. Similar contradictions are obtained if we assume that I2(t0)=0 or R(t0)=0. Thus we conclude that the solutions of (2.2) are positive for all t>0.
Since the equation for ˙R is actually decoupled from the rest in Eq (2.2), we only need to consider dynamics of the following four-dimensional sub-system:
˙S=Λ−F1(S,I1)−F2(S,I2)−λS˙V1=rS−(μ+kI2)V1˙I1=F1(S,I1)−α1I1˙I2=F2(S,I2)+kI2V1−α2I2. | (3.1) |
The basic reproduction number is a dimensionless quantity denoted by R0. It is defined as the expected number of secondary infection cases caused by a single typical infective case during its entire period of infectivity in a wholly susceptible population. Then, referring to the method of [12].
F:=(F1(S,I1)F2(S,I2)+kI2V1). |
V:=(α1I1α2I2). |
Then
F′=(∂F1(S,I1)∂I100∂F2(S,I2)∂I2+kV1)|E0=(∂F1(S0,0)∂I100∂F2(S0,0)∂I2+krΛμλ). |
V′=(α100α2)|E0=(α100α2). |
where E0=(S0,V01,0,0)=(Λλ,rΛμλ,0,0). The matrix F is non-negative and is responsible for new infections (transmission matrix), while the V is invertible and is referred to as the transition matrix for the model (3.1). It follows that,
F′V′−1=(σ1α100σ2α2+krΛα2μλ). |
where σi=∂Fi(S0,0)∂Ii, for i=1,2. Thus, the basic reproduction number can be calculated as
R0=ρ(F′V′−1)=max{σ1α1, σ2α2+krΛα2μλ}. |
where ρ(A) denotes the spectral radius of a matrix A. Let
R1=σ1α1 and R2=σ2α2+krΛα2μλ. |
Then
R0=max{R1,R2}. |
Therefore R1,R2≤R0.
The four possible equilibrium points for the system (3.1) are: Disease-free equilibrium, single-strain (I1)-infection, single-strain (I2)-infection and endemic equilibrium. The system (3.1) has disease-free equilibrium E0=(Λλ,rΛμλ,0,0) for all parameter values. We will now prove the existence of the other equilibrium points. First we will show some lemmas.
Lemma 2. For i = 1, 2.
∂Fi(S,Ii)∂Ii=I∂fi(S,Ii)∂Ii+Fi(S,Ii)Ii. |
Also:
∂Fi(S,Ii)∂Ii≤Fi(S,Ii)Ii. |
Proof. By H1)
Fi(S,Ii)=Iifi(S,Ii) |
Then
∂Fi(S,Ii)∂Ii=Ii∂fi(S,Ii)∂Ii+fi(S,Ii) |
By H2) ∂fi(S,Ii)∂Ii≤0, then:
∂Fi(S,Ii)∂Ii≤f1(S,Ii)=Fi(S,Ii)Ii. |
Lemma 3. For model (3.1), the closed set Ω1={(S,V1,I1,I2)∈Ω|S≤S0 and V1≤V01} is a positively invariant set.
Proof. As Ω is a positively invariant set for model (3.1), it will be enough to show that if S=S0, then ˙S≤0 and if S≤S0 and V1=V01, then ˙V1≤0.
If S=S0, then
˙S=Λ−F1(S0,I1)−F2(S0,I2)−λS0=λS0−F1(S0,I1)−F2(S0,I2)−λS0=−F1(S0,I1)−F2(S0,I2)≤0 |
If S≤S0 and V1=V01, Then
˙V1≤rS0−(μ+kI2)V01=rS0−μV01−kI2V01=−kI2V01≤0 |
Lemma 4. For i = 1, 2.
∂Fi(S,Ii)∂S≥0 |
Proof. By (H1)
Fi(S,Ii)=Iifi(S,Ii) |
Then
∂Fi(S,Ii)∂S=Ii∂fi(S,Ii)∂S≥0 By H2). |
Remark 1. By (H2) given a and b, if S≤a and Ii≥b, then fi(S,Ii)≤fi(a,b), i=1,2.
Theorem 1. (1) The model (3.1) admits a unique single-strain (I1)-infection equilibrium E1=(ˉS,¯V1,¯I1,0) if and only if R1>1.
(2) The model (3.1) admits a unique single-strain (I2)-infection equilibrium E2=(˜S,~V1,0,~I2) if and only if R2>1.
Proof. (1) If I2=0 and R1>1, we consider the system
Λ−F1(ˉS,¯I1)−λˉS=0 | (3.2) |
rˉS−μ¯V1=0 | (3.3) |
F1(ˉS,¯I1)−α1¯I1=0. | (3.4) |
By (3.3) and (3.4)
¯V1=rˉSμ, F1(ˉS,¯I1)=α1¯I1. |
Substituting in (3.2).
Λ−α1¯I1−λˉS=0ˉS=Λ−α1¯I1λ. |
Note that ˉS≥0 if and only if ¯I1≤Λα1. ¯I1 being determined by the positive roots of the equation.
G(¯I1)≡F1(Λ−α1¯I1λ,¯I1)−α1¯I1. | (3.5) |
Then
G′(¯I1)=−α1λ∂F1(Λ−α1¯I1λ,¯I1)∂S+∂F1(Λ−α1¯I1λ,¯I1)∂¯I1−α1. |
And
G(0)=F1(Λλ,0)=0 by H1. |
G′(0)=−α1λ∂F1(Λλ,0)∂S+∂F1(Λλ,0)∂¯I1−α1=∂F1(S0,0)∂¯I1−α1 by H1=α1(σ1α1−1)=α1(R1−1)>0. |
Therefore G(¯I1)>0 by I1 sufficiently small. Also
G(Λα1)=F1(0,¯I1)−Λ=−Λ<0. |
Then Eq (3.5) has a positive root. Also if E1 exists then
f1(ˉS,¯I1)−α1=0. |
Note that ˉS<S0. Then by Lemma 2 and remark 1
0<f1(S0,0)−α1=∂F1(S0,0)∂I1−α1=α1(R1−1). |
Then R1>1.
Next, we shall show that ¯I1 is unique. From (3.4), it follows that
α1=f1(ˉS,¯I1) |
Using (H2) and Lemma 2, we have that −α1λ∂F1(ˉS,¯I1)∂S<0 and ¯I1∂f1(ˉS,¯I1)∂I1≤0. Furthermore, it can be found that
G′(¯I1)=−α1λ∂F1(Λ−α1¯I1λ,¯I1)∂S+∂F1(Λ−α1¯I1λ,¯I1)∂¯I1−α1.=−α1λ∂F1(Λ−α1¯I1λ,¯I1)∂S+¯I1∂f1(ˉS,¯I1)∂¯I1+f1(ˉS,¯I1)−f1(¯I1,¯I1)=−α1λ∂F1(Λ−α1¯I1λ,¯I1)∂S+¯I1∂f1(ˉS,¯I1)∂¯I1<0. |
Which implies that G(¯I1) strictly decreases at any of the zero points of (3.5). Let us suppose that (3.5) has more than one positive root. Without loss of generality, we choose the one, denoted by ¯I1∗, that is the nearest to ¯I1. Because of the continuity of G(¯I1), we must have G′(¯I1∗)≥0, which results in a contradiction with the strictly decreasing property of G(¯I1) at all the zero points.
(2) If I1=0 and R2>1, we consider the system
Λ−F2(˜S,~I2)−λ˜S=0 | (3.6) |
r˜S−(μ+k~I2)~V1=0 | (3.7) |
F2(˜S,~I2)+k~I2~V1−α2~I2=0. | (3.8) |
By (3.7) and (3.8)
~V1=r˜Sμ+k~I2, F2(˜S,~I2)=−k~I2~V1+α2~I2. |
Substituting in (3.6).
Λ−α2~I2+k~I2~V1−λ˜S=0(λ−kr~I2μ+k~I2)˜S=Λ−α2~I2.(λ(μ+k~I2)−kr~I2μ+k~I2)˜S=Λ−α2~I2(λμ+(μ+r)k~I2−kr~I2μ+k~I2)˜S=Λ−α2~I2˜S=(Λ−α2~I2)(μ+k~I2λμ+μk~I2). |
Note that ˜S≥0 if and only if ~I2≤Λα2. ~I2 being determined by the positive roots of the equation.
H(~I2)≡F2((Λ−α2~I2)(μ+k~I2)λμ+kμ~I2,¯I2)+k~I2~V1−α2~I2=F2(Λμ+(Λk−α2μ)~I2−kα2~I22λμ+kμ~I2,~I2)+(Λrk~I2−α2rk~I22λμ+kμ~I2)−α2~I2. | (3.9) |
Then
H′(~I2)=(kμ)(−kα2~I22−Λμ)+λμ(Λk−α2μ−2kα2~I2)(λμ+μk~I2)2×∂F2(Λμ+(Λk−α2μ)~I2−kα2~I22λμ+μk~I2,~I2)∂S+∂F2(Λμ+(Λk−α2μ)~I2−kα2~I22λμ+μk~I2,~I2)∂~I2+(λμ(Λrk−α2rk~I2)−(kμ)α2rk~I22(λμ+μk~I2)2)−α2. |
And
H(0)=F2(Λλ,0)=0 by H1. |
H′(0)=∂F2(S0,0)∂I2+Λrkλμ−α2 by H1=α2(σ2α2+Λrkα2λμ−1)=α2(R2−1)>0. |
Therefore H(~I2)>0 by ~I2 sufficiently small. Also
H(Λα2)=F2(0,Λα2)−Λ=−Λ<0. |
Then Eq (3.9) has a positive root. Also if E2 exists then
Λ−F2(˜S,~I2)−λ˜S=0f2(˜S,~I2)+k~V1−α2=0. |
Note that by H1 we have −F2(˜S,~I2)<0, then Λ−λ˜S>0, therefore ˜S<S0 and ~V1<V01. Then by Lemma 2 and remark 1
0<f2(S0,0)+kV01−α2=∂F2(S0,0)∂I2+kV01−α2=α2(R2−1). |
Then R2>1.
Next, we shall show that ~I2 is unique. From (3.8), it follows that
α2−k~V1=f2(˜S,~I2). |
Furthermore, it can be found that
H′(~I2)=−α2rμ−α2μ2−2α2μk~I2−2α2kr~I2−α2k2~I22+kΛrμ(λ+k~I2)2×∂F2(˜S,~I2)∂S+∂F2(˜S,~I2)∂~I2+k~V1−kr(α2λ+kΛ)~I2μ(λ+k~I2)2−α2=−α2rμ−α2μ2−2α2μk~I2−2α2kr~I2−α2k2~I22+kΛrμ(λ+k~I2)2×∂F2(˜S,~I2)∂S+~I2∂f2(˜S,~I2)∂~I2−kr(α2λ+kΛ)~I2μ(λ+k~I2)2. |
If −α2rμ−α2μ2+kΛr≤0, then H′(~I2)<0 which implies that H(~I2) strictly decreases at any of the zero points of (3.9). Let us suppose that (3.9) has more than one positive root. Without loss of generality, we choose the one, denoted by ~I2∗, that is the nearest to ~I2. Because of the continuity of H(~I2), we must have H′(~I2∗)≥0, which results in a contradiction with the strictly decreasing property of H(~I2) at all the zero points.
If −α2rμ−α2μ2+kΛr>0. Next, we show that ~I2∉[0,−rα2−α2μ+√rα2(rα2+α2μ+kΛ)α2k). Note that
˜S(~I2)=(Λ−α2~I2)(μ+k~I2λμ+μk~I2). |
Then
˜S′(~I2)=−α2rμ−α2μ2−2α2μk~I2−2α2kr~I2−α2k2~I22+kΛrμ(λ+k~I2)2. |
If ~I2∈[0,−rα2−α2μ+√rα2(rα2+α2μ+kΛ)α2k), then ˜S′(~I2)>0, therefore ˜S≥S0, which results in a contradiction, since ˜S<S0.
Thus ~I2∈[−rα2−α2μ+√rα2(rα2+α2μ+kΛ)α2k,Λα2], which implies that H(~I2) strictly decreases at any of the zero points of (3.9). Let us suppose that (3.9) has more than one positive root in [−rα2−α2μ+√rα2(rα2+α2μ+kΛ)α2k,Λα2]. Without loss of generality, we choose the one, denoted by ~I2∗, that is the nearest to ~I2. Note that H′(~I2∗)<0 and H′(~I2)<0. Because of the continuity of H(~I2), we must have H′(~I2∗)≥0, which results in a contradiction.
The model (3.1) can have endemic infection equilibrium E3=(S∗,V∗1,I∗1,I∗2). To find E3, we consider the system
Λ−F1(S∗,I∗1)−F2(S∗,I∗2)−λS∗=0 | (3.10) |
rS∗−(μ+kI∗2)V∗1=0 | (3.11) |
F1(S∗,I∗1)−α1I∗1=0 | (3.12) |
F2(S∗,I∗2)+kI∗2V∗1−α2I∗2=0. | (3.13) |
By (3.11), (3.12) and (3.13)
V∗1=rS∗μ+kI∗2, F1(S∗,I∗1)=α1I∗1, F2(S∗,I∗2)=−kI∗2V∗1+α2I∗2. |
Substituting in (3.10).
Λ−α1I∗1−α2I∗2+kI∗2V∗1−λS∗=0(λ−krI∗2μ+kI∗2)S∗=Λ−α1I∗1−α2I∗2(λμ+(μ+r)kI∗2−krI∗2μ+kI∗2)S∗=Λ−α1I∗1−α2I∗2S∗=(Λ−α1I∗1−α2I∗2)(μ+kI∗2λμ+μkI∗2). |
Note that S∗≥0 if and only if I∗1≤Λ−α2I∗2α1 and I∗2≤Λ−α1I∗1α2. ¯I2 being determined by the positive roots of the equation.
G2(I∗2)≡f2((Λ−α1I∗1−α2I∗2)(μ+kI∗2)λμ+kμI∗2,I∗2)+kV∗1−α2. |
I∗1 being determined by the positive roots of the equation.
G1(I∗1)≡f1((Λ−α1I∗1−α2I∗2)(μ+kI∗2)λμ+kμI∗2,I∗1)−α1. |
In this section we will study the local and global stability of the equilibrium points.
Theorem 2. The disease-free equilibrium E0=(Λλ,rΛμλ,0,0) is unstable if R0>1 while it is locally asymptotically stable if R0<1.
Proof. The Jacobian matrix of the model we get is the following one
J:=(−∂F1∂S−∂F2∂S−λ0−∂F1∂I1−∂F2∂I2r−μ−kI20−kV1∂F1∂S0∂F1∂I1−α10∂F2∂SkI20∂F2∂I2+kV1−α2). | (3.14) |
Then Eq (3.14) at the disease-free equilibrium E0 is
JE0=(−∂F1(S0,0)∂S−∂F2(S0,0)∂S−λ0−∂F1(S0,0)∂I1−∂F2(S0,0)∂I2r−μ0−kV01∂F1(S0,0)∂S0∂F1(S0,0)∂I1−α10∂F2(S0,0)∂S00∂F2(S0,0)∂I2+kV01−α2)=(−λ0−∂F1(S0,0)∂I1−∂F2(S0,0)∂I2r−μ0−kV0100∂F1(S0,0)∂I1−α10000∂F2(S0,0)∂I2+krΛμλ−α2)=(−λ0−σ1−σ2r−μ0−kV0100α1(σ1α1−1)0000α2(σ2α2+krΛμλα2−1))=(−λ0−σ1−σ2r−μ0−kV0100α1(R1−1)0000α2(R2−1)). | (3.15) |
Thus the eigenvalues of the above Eq (3.15) are
λ1=−λ, λ2=−μ, λ3=α1(R1−1), λ4=α2(R2−1). | (3.16) |
From (3.16), if R0<1, then λ3,λ4<0 and we obtain that the disease-free equilibrium E0 of Model (3.1) is locally asymptotically stable. If R0>1, then the disease-free equilibrium loses its stability.
Theorem 3. Let ¯R2=1α2∂F2(ˉS,0)∂I2+k¯V1α2. The equilibrium E1 is unstable if ¯R2>1 while it is locally asymptotically stable if ¯R2<1.
Proof. Then Eq (3.14) at the equilibrium E1 is
JE1=(A110A13A14r−μ0A24A310A330000A44). | (3.17) |
where
A11=−∂F1(ˉS,¯I1)∂S−λ<0A13=−∂F1(ˉS,¯I1)∂I1<0A14=−∂F2(ˉS,0)∂I2A24=−k¯V1<0A31=∂F1(ˉS,¯I1)∂S>0A33=∂F1(ˉS,¯I1)∂I1−α1=¯I1∂f1(ˉS,¯I1)∂I1+f1(ˉS,¯I1)−α1=¯I1∂f1(ˉS,¯I1)∂I1≤0A44=∂F2(ˉS,0)∂I2+k¯V1−α2=α(¯R2−1). |
The last equality regarding A33, is because Eq (3.4) implies that f1(ˉS,¯I1)−α1=0. The corresponding characteristic polynomial is
p(x)=−(A44−x)(x3+a2x2+a1x+a0). |
Then an eigenvalue is A44 and the remaining ones satisfy
(x3+a2x2+a1x+a0)=0. |
where
a2=−(A11−μ+A33)>0a1=−μA11−μA33+A11A33−A13A31a0=μA11A33−μA13A31. |
Note that
A11A33−A13A31=(−∂F1(ˉS,¯I1)∂S−λ)(∂F1(ˉS,¯I1)∂I1−α1)+∂F1(ˉS,¯I1)∂I1∂F1(ˉS,¯I1)∂S=−λ(∂F1(ˉS,¯I1)∂I1−α1)+α1∂F1(ˉS,¯I1)∂S>0. |
Then a1, a0>0 and
a2a1−a0=−(A11+A33)a1+μ(−μA11−μA33)+μ(A11A33−A13A31)−a0=−(A11+A33)a1+μ(−μA11−μA33)>0. |
Applying the Routh–Hurwitz criterion, we see that all roots of x3+a2x2+a1x+a0 have negative real parts. If ¯R2>1, then A44>0 therefore E1 is unstable and if ¯R2<1, then A44<0 therefore E1 is stable.
Remark 2. ˉS≤S0 and ¯V1≤V01, then ¯R2≤R2, therefore if R2<1 then ¯R2<1.
Theorem 4. Let ~R1=1α1∂F1(˜S,0)∂I1. If ∂F2(˜S,~I2)∂I2≤0 the equilibrium E2 is unstable if ~R1>1 while it is locally asymptotically stable if ~R1<1.
Proof. Then Eq (3.14) at the equilibrium E1 is
JE2=(B110B13B14rB220B2400B330B41B420B44). | (3.18) |
Where
B11=−∂F2(˜S,~I2)∂S−λ<0B13=−∂F1(˜S,0)∂I1B14=−∂F2(˜S,~I2)∂I2B22=−μ−k~I2<0B24=−k~V1<0B33=∂F1(˜S,0)∂I1−α1=α1(~R1−1)B41=∂F2(˜S,~I2)∂S>0B42=k~I2>0B44=∂F2(ˉS,¯I2)∂I2+k~V1−α2=~I2∂f2(˜S,~I2)∂I2<0. |
The last equality regarding B44, is because Eq (3.8) implies that k~V1−α2=−f2(˜S,~I2). The corresponding characteristic polynomial is
p(x)=−(B33−x)(x3+b2x2+b1x+b0) |
Then (3.18) has an eigenvalue equal to B33 and the remaining ones satisfy
(x3+b2x2+b1x+b0)=0. |
where
b2=−(B11+B22+B44)>0.b1=B22B11+B22B44+B11B44−B14B41−B24B42b0=−B22B11B44−rB14B42+B14B22B41+B11B24B42. |
Note that
B11B44−B14B41=−λ(∂F2(ˉS,¯I2)∂I2+k~V1−α2)+(−∂F2(˜S,~I2)∂S)(k~V1−α2)>0. |
And
−B22B11B44−rB14B42+B14B22B41=(∂F2(˜S,~I2)∂S+λ)(k~V1−α2)(−μ−k~I2)−(−μ)(∂F2(ˉS,¯I2)∂I2)(−μ−k~I2)−(−r)(∂F2(ˉS,¯I2)∂I2)(−μ)>0. |
Then b1, b0>0. Also
b2b1−b0=−B44b1−B22(B22B11+B22B44−B24B42)−B22(B11B44−B14B41)−B11(B22B11+B22B44+B11B44−B14B41)+B11B24B42+B22B11B44+rB14B42−B14B22B41−B11B24B42=−B44b1−B22(B22B11+B22B44−B24B42)−B11(B22B11+B22B44+B11B44−B14B41)+rB14B42>0. |
Applying the Routh–Hurwitz criterion, we see that all roots of x3+b2x2+b1x+b0 have negative real parts. If ~R1>1, then B33>0 therefore E2 is unstable and if ~R1<1, then B33<0 therefore E2 is stable.
Remark 3. ˜S≤S0, then ~R1≤R1, therefore if R1<1 then ~R1<1.
Remark 4. If ∂F2(˜S,~I2)∂I2>0, then bi>0 i=0,1,2.
Remark 5. The Theorem 4 is valid for ∂F2(˜S,~I2)∂I2>0 if b2b1−b0>0.
Theorem 5. If ¯R2>1 and ~R1>1 then system (3.1) is uniformly persistent.
Proof. The result follows from an application of Theorem 4.6 in [13], with X1=int(R4+) and X2=bd(R4+) this choice is in accordance with the conditions stated in the theorem. Now, note that by of Lemma 1 there exists a compact set Ω in which all solution of system (3.1) initiated in R4+ ultimately enter and remain forever after. The condition (C4.2) is easily verified for this set Ω1. On other hand, we denote the omega limit set of the solution x(t,x0) of system (3.1) starting in x0∈R4+ by w(x0). Note that w(x0) is bounded (Lemma 1), we need to determine the following set:
Ω2=⋃y∈Y2w(y), where Y2={x0∈X2|x(t,x0)∈X2,∀t>0}. |
From the system equations (3.1) it follows that all solutions starting in bd(R4+) but not on the I1 axis or on the I2 axis leave bd(R4+). This implies that
Y2={(S,V1,I1,I2)∈bd(R4+)|I1=0 or I2=0}. |
Furthermore, we see that Ω2={E0,E1,E2}, then ⋃3i=1{Ei} is a covering of Ω2, which is isolated (since Ei (i=1,2,3) is a saddle point) and acyclic. Finally we need to prove that Ei (i = 1, 2, 3) is a weak repeller for X1 to end the prove.
By definition Ei is a weak repeller for X1 if for every solution (S(t),V1(t),I1(t),I2(t)) starting in (S0,V10,I10,I20)∈X1
lim supt→+∞‖(S(t),V1(t),I1(t),I2(t))−Ei‖>0. |
We will first show that E0 is a weak repeller for X1. Since ¯R2>1 and ~R1>1, then R2=1α2(f2(S0,0)+kV0)>1 and R1=1α1(f1(S0,0))>1, therefore f2(S0,0)+kV0−α2>0 and f1(S0,0)−α1>0. Because of the continuity of f2(S,I2)+kV1−α2 and f1(S,I1)−α1, there exists a sufficiently small constant η2>0, such that f1(S0−η2,η2)−α1>0 and f2(S0−η2,η2)+k(V01−η2)−α1>0.
Now, we suppose that E0 is not a weak repeller for X1, i.e., there exists a solution (S(t),V1(t),I1(t),I2(t)) starting in (S0,V10,I10,I20)∈X1 such that
lim supt→+∞‖(S(t),V1(t),I1(t),I2(t))−E0‖=0. |
Then exists T1>0 such that for every η1>0
S0−η1<S(t), V01−η1<V1(t), 0<I1(t)<η1 and 0<I2(t)<η1 ∀t≥T1. |
Let η1=η2, then for t≥T1.
˙I1=I1(f1(S,I1)−α1)≥I1(f1(S0−η2,η2)−α1). |
and
˙I2=I2(f2(S,I2)+kV1−α2)≥I2(f2(S0−η2,η2)+k(V01−η2)−α2). |
By comparison principle, we have
I1(t)≥I1(T1)e(f1(S0−η2,η2)−α1)(t−T1) and I2(t)≥I2(T1)e(f2(S0−η2,η2)+k(V01−η2)−α2)(t−T1), ∀t≥T1. |
Note that f1(S0−η2,η2)−α1>0, f2(S0−η2,η2)+k(V01−η2)−α1>0, I1(T1)>0 and I2(T1)>0, which implies that limt→∞I1=limt→∞I2=∞, this gives a contradiction. Then E0 is a weak repeller for X1.
Similarly it is shown that E1 and E2 are weak repeller for X1. Then we conclude that system (3.1) is uniformly persistent.
Further, it is proved in [14] uniform persistence implies the existence of an interior equilibrium point. Therefore, we have established the following.
Theorem 6. The model (3.1) admits a endemic equilibrium E3=(S∗,V∗1,I∗1,I∗2) if ¯R2>1 and ~R1>1.
Theorem 7. If c1c2−c3>0 and c1c2c3−c23−c21c4>0, where
c1=−C44−C33−C22−C11c2=−C41C14−C42C24+C44C33+C44C22+C44C11−C31C13+C33C22+C33C11+C22C11c3=−rC42C14+C41C14C33+C41C14C22+C42C24C33+C42C24C11+C44C31C13−C44C33C22−C44C33C11−C44C22C11+C31C13C22−C33C22C11c4=rC42C14C33−C41C14C33C22+C42C24C31C13−C42C24C33C11−C44C31C13C22+C44C33C22C11. |
Then E3 is locally asymptotically stable.
Proof. Then Eq (3.14) at the equilibrium E3 is
JE3=(C110C13C14rC220C24C310C330C41C420C44). |
Where
C11=−∂F1(S∗,I∗1)∂S−∂F2(S∗,I∗2)∂S−λ<0C13=−∂F1(S∗,I∗1)∂I1C14=−∂F2(S∗,I∗2)∂I2C22=−μ−kI∗2<0C24=−kV∗1<0C31=∂F1(S,I∗1)∂S>0C33=∂F1(S∗,I∗1)∂I1−α1=I∗1∂f1(S∗,I∗1)∂I1+f1(S∗,I∗1)−α1=I∗1∂f1(S∗,I∗1)∂I1≤0C41=∂F2(S∗,I∗2)∂S>0.C42=kI∗2>0.C44=∂F2(S∗,I∗2)∂I2+kV∗1−α2=I∗2∂f2(S∗,I∗2)∂I2≤0. |
The corresponding characteristic polynomial is
p(x)=x4+c1x3+c2x2+c3x+c4. |
Note that c1>0,
−C41C14+C44C11=C44(C11+C41)−C41(kV∗1−α2)>0C33C11−C31C13=C33(C11+C31)−C31(−α1)>0. |
then c2>0, If C14≥0 then c3>0 and c4>0, while if C14<0 we have that
C41C14C33+C44C31C13−C44C33C11=−C44C33(C11+C41+C31)−C44(α1)(C31)−(kV∗1−α2)C33(−C41)>0−rC42C14−C44C22C11+C41C14C22=−C44C22(C11+C41+C31+r)−(C14)(μ)(r)+(kV∗1−α2)C22(C41+r)+C44C22C31>0. |
and let
⋆=rC42C14C33+C44C33C22C11−C41C14C33C22−C44C31C13C22. |
then
⋆=−C33(−C44C22(C11+C41+C31+r)−(C14)(μ)(r)+(kV∗1−α2)C22(C41+r)+C44C22C31)−C44C31C13C22=−C33(−C44C22(C11+C41+C31+r)−(C14)(μ)(r)+(kV∗1−α2)C22(C41+r))+C44C22C31(α1)>0. |
Then c3>0 and c4>0. If c1c2−c3>0 and c1c2c3−c23−c21c4>0 by Routh–Hurwitz criterion, we see that all roots of x4+c1x3+c2x2+c3x+c4 have negative real parts, then E3 is locally asymptotically stable.
In this section, we study the global properties of the equilibria. We use Lyapunov function to show the global stabilities. Such Lyapunov functions all take advantage of the properties of the function.
g(x)=x−1−ln(x). |
which is positive in R+ except at x=1, where it vanishes.
Theorem 8. The DFE E0 is globally asymptotically stable if,
R0<1. |
Proof. Consider the Lyapunov function
V(S,V1,I1,I2)=I1+I2, |
Since I1,I2>0, then V(S,V1,I1,I2)≥0 and V(S,V1,I1,I2) attains zero at I1=I2=0.
Now, we need to show ˙V≤0.
˙V=˙I1+˙I2=F1(S,I1)−α1I1+F2(S,I2)+kI2V1−α2I2.=I1(f1(S,I1)−α1)+I2(f2(S,I2)+kV1−α2). |
For S≤S0 and V1≤V01
˙V≤I1(f1(S0,0)−α1)+I2(f2(S0,0)+kV01−α2).=I1(∂F1(S0,0)∂I1−α1)+I2(∂F2(S0,0)∂I2+kV01−α2)=α1I1(R1−1)+α2I2(R2−1)≤0. |
Furthermore, dVdt=0 if and only if I1=I2=0, so the largest invariant set contained in {(S,V1,I1,I2)∈Ω1|dVdt=0} is the hyperplane I1=I2=0, By LaSalle's invariant principle, this implies that all solution in Ω1 approach the hyperplane I1=I2=0 as t→∞. Also, All solution of (3.1) contained in such plane satisfy ˙S=Λ−λS, ˙V1=rS−μV1, which implies that S→Λλ and V1→rΛμλ as t→∞, that is, all of these solution approach E0. Therefore we conclude that E0 is globally asymptotically stable in Ω1.
Now we will show that every solution (S(t),V1(t),I1(t),I2(t))∈R4+, where t→∞ (S(t),V1(t),I1(t),I2(t))∈Ω1, let (S(t),V1(t),I1(t),I2(t))∈R4+. Then
˙S≤Λ−λS |
By the comparison principle limt→∞supS(t)≤Λλ=S0. Then S(t)≤S0 for t sufficiently large.
Also if S(t)≤S0.
˙V1≤rS0−(μ+kI2)V1≤rS0−μV1 |
By the comparison principle limt→∞supV(t)≤rS0μ=V01. Therefore E0 is globally asymptotically stable.
From now on, we assume that
H4) For i=1,2. fi(S,Ii)=Sgi(S,Ii).
Lemma 5. Let a>0 be a constant, for i=1,2 if ∂Fi(S,Ii)∂Ii≥0 for all Ii, then
(Iia−Fi(S,Ii)Fi(S,a))(Fi(S,a)Fi(S,Ii)−1)≤0 |
Proof. Note that
(Iia−Fi(S,Ii)Fi(S,a))(Fi(S,a)Fi(S,Ii)−1)=Iia(1−fi(S,Ii)fi(S,a))(Fi(S,a)Fi(S,Ii)−1) |
If a≥Ii, then
fi(S,Ii)fi(S,a)≥1 and Fi(S,a)Fi(S,Ii)≥1. |
If a≤Ii, then
fi(S,Ii)fi(S,a)≤1 and Fi(S,a)Fi(S,Ii)≤1. |
Therefore
(Iia−Fi(S,Ii)Fi(S,a))(Fi(S,a)Fi(S,Ii)−1)≤0. |
Theorem 9. Suppose that ∂F1(S,I1)∂I1≥0 for all I1, then E1 is globally asymptotically stable if,
R2<1. |
Proof. Consider the Lyapunov function
V(S,V1,I1,I2)=I2, |
Since I2>0, then V(S,V1,I1,I2)≥0 and V(S,V1,I1,I2) attains zero at I2=0. Now, we need to show ˙V≤0.
˙V=˙I2=F2(S,I2)+kI2V1−α2I2.=I2(f2(S,I2)+kV1−α2). |
For S≤S0 and V1≤V01
˙V≤I2(f2(S0,0)+kV01−α2).=I2(∂F2(S0,0)∂I2+kV01−α2)=α2I2(R2−1)≤0. |
Furthermore, dVdt=0 if and only if I2=0. Suppose that (S(t),V1(t),I1(t),I2(t)) is a solution of (3.1) contained entirely in the set M={(S(t),V1(t),I1(t),I2(t))∈Ω1|˙V=0}. Then, ˙I2=0 and, from the above inequalities, we have I2=0. Thus, the largest positively invariant set contained in M is the plane I2=0. By LaSalle’s invariance principle, this implies that all solutions in approach the plane I2=0 as t→∞. On the other hand, solutions of (3.1) contained in such plane satisfy
˙S=Λ−F1(S,I1)−λS˙V1=rS−(μ)V1˙I1=F1(S,I1)−α1I1. |
Now we will show that S(t)→ˉS, V1(t)→¯V1 and I1(t)→¯I1 Consider the Lyapunov function
V(S,V1,I1)=∫SˉS(1−F1(ˉS,¯I1)F1(χ,¯I1))dχ+¯I1g(I1¯I1). |
Note that 1−F1(ˉS,¯I1)F1(χ,¯I1)=¯I1(f1(χ,¯I1)−f1(ˉS,¯I1))F1(χ,¯I1), by H2) f1(S,¯I1)−f1(ˉS,¯I1)≥0 if S≥ˉS and f1(S,¯I1)−f1(ˉS,¯I1)≤0 if S≤ˉS, then ∫SˉS(1−F1(ˉS,¯I1)F1(χ,¯I1))dχ≥0 for all S. Therefore, V(S,V1,I1)≥0 and V(S,V1,I1) attains zero at S(t)=ˉS, and I1(t)=¯I1.
Now, we need to show ˙V≤0.
˙V=(1−F1(ˉS,¯I1)F1(S,¯I1))˙S+(1−¯I1I1)˙I1=(1−F1(ˉS,¯I1)F1(S,¯I1))(Λ−F1(S,I1)−λS)+(1−¯I1I1)(F1(S,I1)−α1I1)=(1−F1(ˉS,¯I1)F1(S,¯I1))(λˉS+F1(ˉS,¯I1)−F1(S,I1)−λS)+F1(S,I1)−α1I1−¯I1f1(S,I1)+α1¯I1=λ(ˉS−S)(1−F1(ˉS,¯I1)F1(S,¯I1))+(1−F1(ˉS,¯I1)F1(S,¯I1))F1(ˉS,¯I1)−F1(S,I1)+F1(ˉS,¯I1)F1(S,¯I1)F1(S,I1)+F1(S,I1)−I1F1(ˉS,¯I1)¯I1−¯I1f1(S,I1)+F1(ˉS,¯I1)=(2−F1(ˉS,¯I1)F1(S,¯I1)+F1(S,I1)F1(S,¯I1)−I1¯I1−¯I1f1(S,I1)F1(ˉS,¯I1))F1(ˉS,¯I1)+λ(ˉS−S)(1−F1(ˉS,¯I1)F1(S,¯I1)). |
Note that
λ(ˉS−S)(1−F1(ˉS,¯I1)F1(S,¯I1))=λ(ˉS−S)(1−f1(ˉS,¯I1)f1(S,¯I1))≤0. |
and
2−F1(ˉS,¯I1)F1(S,¯I1)+F1(S,I1)F1(S,¯I1)−I1¯I1−¯I1f1(S,I1)F1(ˉS,¯I1)=2−F1(ˉS,¯I1)F1(S,¯I1)+F1(S,I1)F1(S,¯I1)−I1¯I1−¯I1F1(S,I1)I1F1(ˉS,¯I1)+1−F1(S,I1)F1(S,¯I1)F1(S,I1)F1(S,¯I1)+IF1(S,¯I1)¯I1F1(S,I1)−IF1(S,¯I1)¯I1F1(S,I1)=3−F1(ˉS,¯I1)F1(S,¯I1)−¯I1F1(S,I1)I1F1(ˉS,¯I1)−IF1(S,¯I1)¯I1F1(S,I1)+(I1¯I1−F1(S,I1)F1(S,¯I1))(F1(S,¯I1)F1(S,I1)−1)≤0. | (3.19) |
The last inequality is due to the Lemma 5 and the relation of the geometric and arithmetic means, then ˙V≤0. Furthermore, dVdt=0 if and only if S=ˉS and I1=¯I1, which implies that S→ˉS, I1→¯I1 and I2→0 as t→∞. By LaSalle's invariant principle, this implies that all solutions in Ω1 approach the plane S=ˉS, I1=¯I1 and I2=0 as t→∞. Also, All solutions of (3.1) contained in such plane satisfy ˙V1=rˉS−μV1, which implies that V1→rˉSμ=¯V1 as t→∞, that is, all of these solution approach E1. Therefore we conclude that E1 is globally asymptotically stable in Ω1.
Corollary 1. If 2−F1(ˉS,¯I1)F1(S,¯I1)+F1(S,I1)F1(S,¯I1)−I1¯I1−¯I1f1(S,I1)F1(ˉS,¯I1)≤0 and R2<1 then E1 is globally asymptotically stable.
Theorem 10. Suppose that ∂F2(S,I2)∂I2≥0 for all I2, then E2 is globally asymptotically stable if,
R1<1 and 2−F2(˜S,~I2)F2(S,~I2)+SF2(˜S,~I2)˜SF2(S,~I2)−V1~V1−S~V1˜SV1≤0. |
Proof. Consider the Lyapunov function
V(S,V1,I1,I2)=I1. |
Since I1>0, then V(S,V1,I1,I2)≥0 and V(S,V1,I1,I2) attains zero at I1=0. Now, we need to show ˙V≤0.
˙V=˙I1=F1(S,I1)−α1I1=I1(f1(S,I1)−α1) |
For S≤S0
˙V≤I1(f1(S0,0)−α1)=I1(∂F1(S0,0)∂I1−α1)=α1I1(R1−1)≤0. |
Furthermore, dVdt=0 if and only if I1=0. Suppose that (S(t),V1(t),I1(t),I2(t)) is a solution of (3.1) contained entirely in the set M={(S(t),V1(t),I1(t),I2(t))∈Ω1|˙V=0}. Then, ˙I1=0 and, from the above inequalities, we have I1=0. Thus, the largest positively invariant set contained in M is the plane I1=0. By LaSalle’s invariance principle, this implies that all solutions in approach the plane I1=0 as t→∞. On the other hand, solutions of (3.1) contained in such plane satisfy.
˙S=Λ−F2(S,I2)−λS˙V1=rS−(μ+kI2)V1˙I2=F2(S,I2)+kV1I2−α2I2. |
Now we will show that S(t)→˜S, V1(t)→~V1 and I1(t)→~I1 Consider the Lyapunov function
V(S,V1,I2)=∫S˜S(1−F2(˜S,~I2)F2(χ,~I2))dχ+~V1g(V1~V1)+~I2g(I2~I2). |
Now, we need to show ˙V≤0.
˙V=(1−F2(˜S,~I2)F2(S,~I2))˙S+(1−~V1V1)˙V1+(1−~I2I2)˙I2=(1−F2(˜S,~I2)F2(S,~I2))(Λ−F2(S,I2)−λS)+(1−~V1V1)(rS−(μ+kI2)V1)+(1−~I2I2)(F2(S,I2)+kI2V1−α2I2)=(1−F2(˜S,~I2)F2(S,¯I2))(λˉS+F2(˜S,~I2)−F2(S,I2)−λS)+rS−(μ+kI2)V1−rS~V1V1+(μ+kI2)~V1+F2(S,I2)+kI2V1−α2I2−~I2f2(S,I2)−k~I2V1+α2~I2=μ(˜S−S)(1−F2(˜S,~I2)F2(S,~I2))+r(˜S−˜SF2(˜S,~I2)F2(S,~I2)−S+SF2(˜S,~I2)F2(S,~I2))+(1−F2(˜S,~I2)F2(S,~I2))F2(˜S,~I2)+F2(˜S,~I2)F2(S,~I2)F2(S,I2)+rS−r˜S~V1V1−rS~V1V1+r˜S−I2F2(˜S,~I2)~I2−~I2f2(S,I2)+F2(˜S,~I2)=μ(˜S−S)(1−F2(˜S,~I2)F2(S,~I2))+r˜S(2−F2(˜S,~I2)F2(S,~I2)+SF2(˜S,~I2)˜SF2(S,~I2)−V1~V1−S~V1˜SV1)+(2−F2(˜S,~I2)F2(S,~I2)+F2(S,I2)F2(S,~I2)−I2~I2−~I2f2(S,I2)F2(˜S,~I2))F2(˜S,~I2). |
Note that
μ(˜S−S)(1−F2(˜S,~I2)F2(S,~I2))≤0. |
2−F2(˜S,~I2)F2(S,~I2)+F2(S,I2)F2(S,~I2)−I2~I2−~I2f2(S,I2)F2(˜S,~I2)≤0 |
The last inequality is due to the Lemma 5 and the relation of the geometric and arithmetic means, then ˙V≤0. Furthermore, ˙V=0 if and only if S=˜S, I2=~I2 and V1=~V1. Therefore E2 is globally asymptotically stable.
Remark 6. Note that if ∂g2(S,I2)∂S≥0, then
2−F2(˜S,~I2)F2(S,~I2)+SF2(˜S,~I2)˜SF2(S,~I2)−V1~V1−S~V1˜SV1=3−V1~V1−S~V1˜SV1−˜SS+(−1+˜SS)(1−g2(˜S,~I2)g2(S,~I2))≤0. |
Corollary 2. If 2−F2(˜S,~I2)F2(S,~I2)+F2(S,I2)F2(S,~I2)−I2~I2−~I2f2(S,I2)F2(˜S,~I2)≤0, R1<1 and 2−F2(˜S,~I2)F2(S,~I2)+SF2(˜S,~I2)˜SF2(S,~I2)−V1~V1−S~V1˜SV1≤0 then E2 is globally asymptotically stable.
Theorem 11. E3 is globally asymptotically stable if
F1(S∗,I∗1)(2−S∗S−Sg1(S,I1)S∗g1(S∗,I∗1))+F2(S∗,I∗2)(2−S∗S−Sg2(S,I2)S∗g2(S∗,I∗2))+rS∗(3−S∗S−V1V∗1−SV∗1S∗V1)+μS∗(2−S∗S−SS∗)+I1(S∗g1(S,I1)−α1)+I2(S∗g2(S,I2)+kV1∗−α2)<0. |
Proof. Assume E3 exists. Consider the Lyapunov function
V(S,V1,I1,I2)=S∗g(SS∗)+V1∗g(V1V∗1)+I∗1g(I1I∗1)+I∗2g(I2I∗2). |
Where g(x)=x−1−ln(x). Then V(S,V1,I1,I2)≥0 and V(S,V1,I1,I2) attains zero at E3.
Now, we need to show ˙V≤0.
˙V=(1−S∗S)˙S+(1−V∗1V1)˙V1+(1−I∗1I1)˙I1+(1−I∗2I2)˙I2=(1−S∗S)(Λ−F1(S,I1)−F2(S,I2)−λS)+(1−V1∗V1)(rS−(μ+kI2)V1)+(1−I∗1I1)(F1(S,I1)−α1I1)+(1−I∗2I2)(F2(S,I2)+kI2V1−α2I2)=Λ−F1(S,I1)−F2(S,I1)−λS−ΛS∗S+I1S∗g1(S,I1)+I2S∗g2(S,I2)+λS∗+rS−μV1−kI2V1−rSV∗1V1+μV∗1+kI2V∗1+F1(S,I1)−α1I1−I∗1f1(S,I1)+α1I∗1+F2(S,I2)+kI2V1−α2I2−I∗2f2(S,I2)−kI∗2V1+α2I∗2=(F1(S∗,I∗1)+F2(S∗,I∗2)+λS∗)−λS−(F1(S∗,I∗1)+F2(S∗,I∗2)+λS∗)S∗S+I1S∗g1(S,I1)+I2S∗g2(S,I2)+λS∗+rS−μV1−rSV∗1V1+μV∗1+kI2V∗1−α1I1−I∗1f1(S,I1)+F1(S∗,I∗1)−α2I2−I∗2f2(S,I2)−kI∗2V1+F2(S∗,I∗2)+kI∗2V∗1=(2F1(S∗,I∗1)−F1(S∗,I∗1)S∗S−I∗1f1(S,I1))+(2F2(S∗,I∗2)−F2(S∗,I∗2)S∗S)−I∗2f2(S,I2)+(2λS∗−λS∗S∗S−λS+rS−rSV∗1V1+rS∗−rS∗V1V∗1)+(I1S∗g1(S,I1)−α1I1)+(I2S∗g2(S,I2)+kI2V∗1−α2I2)=F1(S∗,I∗1)(2−S∗S−Sg1(S,I1)S∗g1(S∗,I∗1))+F2(S∗,I∗2)(2−S∗S−Sg2(S,I2)S∗g2(S∗,I∗2))+rS∗(3−S∗S−V1V∗1−SV∗1S∗V1)+μS∗(2−S∗S−SS∗)+I1(S∗g1(S,I1)−α1)+I2(S∗g2(S,I2)+kV∗1−α2). |
By the relation of geometric and arithmetic means, we conclude ˙V≤0, with equality holding only at the equilibrium E3. Therefore E3 is globally asymptotically stable.
In this section, we present some numerical simulations of the solutions for system (3.1) to verify the results obtained in section 3.3 and give examples to illustrate theorems in section 3.4. In system (3.1), we set:
F1(S,I1)=β1SI11+ζ1I21,F2(S,I2)=β2SI21+ζ2S,Λ=200,γ1=0.07,γ2=0.09,μ=0.02,v1=0.1,v2=0.1and k=0.00002. |
In this case
g1(S,I1)=β11+ζ1I21, g2(S,I1)=β21+ζ2S, R1=β1Λα1λ and R2=β2Λα2(λ+ζΛ)+krΛα2μλ. |
Parameters and units are arbitrary and have been used for illustration purposes only. Anyway, when considering a realistic scenario such values could be derived from statistical data.
• Example 6.1. In system (3.1), we set β1=0.00003, r=0.1, β2=0.0002, ζ1=0.7 and ζ2=0.9. Then S0≈1667, V0≈8333, R1≈0.2632 R2≈0.7947. By Theorem 8, we see that the disease-free equilibrium E0 is globally asymptotically stable. Numerical simulation illustrates our result (see Figure 1).
• Example 6.2. In system (3.1), we set β1=0.0002, r=0.1, β2=0.0002, ζ1=0 and ζ2=0.9. Then ˉS≈950, ¯V1≈4750, ¯I1≈453, R1≈1.7544, R2≈0.7947. By Theorem 9, we see that the E1 is globally asymptotically stable. Numerical simulation illustrates our result (see Figure 2).
• Example 6.3. In system (3.1), we set β1=0.00003, r=0.1, β2=0.0002, ζ1=0.7 and ζ2=0.001. Then ˜S≈1317, ~V1≈4814, ~I2≈368, R1≈0.2632, R2≈1.3889 and 2−F2(˜S,~I2)F2(S,~I2)+SF2(˜S,~I2)˜SF2(S,~I2)−V1~V1−S~V1˜SV1≤0 (see Figure 3). By corollary 2, we see that the E2 is globally asymptotically stable. Numerical simulation illustrates our result (see Figure 4).
• Example 6.4. In system (3.1), we set β1=0.0002, r=0.01, β2=0.0002, ζ1=0.0001 and ζ2=0.0001. Then R1≈7.0175, R2≈4.1270, ˜S≈1134, ˉS≈5310, ¯V1≈2655, ¯R2≈3.555 and ~R1≈1.194. Then by Theorem 6, E3=(S∗,V∗1,I∗1,I∗2) exists (S∗≈1133, V∗1≈320, I∗1≈44, I∗2≈774), Also c1≈0.2501 c2≈0.0171 c3≈3.4759×10−04 c4≈3.4759×3.924210−06, c1c2−c23≈0.0043 and c1c2c3−c23−c21c4≈1.1218e×10−06 by Theorem 7, E3 is locally asymptotically stable. Also E3 satisfies F1(S∗,I∗1)(2−S∗S−Sg1(S,I1)S∗g1(S∗,I∗1))+F2(S∗,I∗2)(2−S∗S−Sg2(S,I2)S∗g2(S∗,I∗2))+μS∗(2−S∗S−SS∗)+I1(S∗g1(S,I1)−α1)+I2(S∗g2(S,I2)+kV1∗−α2)<0. By Theorem 11, we see that the E3 is globally asymptotically stable. Numerical simulation illustrates our result (see Figure 5).
In this paper, we studied a system of ordinary differential equations to model the disease dynamics of two strains of influenza with only one vaccination for strain 1 being implemented, and general incidence rate for strain 1 and strain 2. We obtained four equilibrium points:
● E0 disease-free equilibrium, I1 and I2 are both zero.
● E1 single-strain-infection equilibria, I2 are zero.
● E2 single-strain-infection-equilibria, I1 are zero.
● E3 double-strain-infection equilibrium, I1 and I2 are both positive.
We have investigated the topics of existence and non-existence of equilibrium points and their stabilities. We also used the next-generation matrix method to obtain two threshold quantities R1 and R2, called the basic reproduction ratios for strain 1 and 2 respectively. It was shown that the global stability of each of the equilibrium points depends on the magnitude of these threshold quantities. More precisely, we have proved the following:
● If R0<1 the disease free equilibrium E0 is globally asymptotically stable and if R0>1, then E0 is unstable.
● If R1>1 the model (3.1) admits a unique single-strain-infection-equilibria E1. Also if R2<1 then E1 is globally asymptotically stable and if ¯R2>1, then E1 is unstable.
● If R2>1 the model (3.1) admits a unique single-strain-infection equilibria E2. Also if R1<1 and 2−F2(˜S,~I2)F2(S,~I2)+SF2(˜S,~I2)˜SF2(S,~I2)−V1~V1−S~V1˜SV1<0, then E2 is globally asymptotically stable and if ~R1>1, then E2 is unstable.
● If ¯R2>1 and ~R1>1 the model (3.1) admits a double strain infection equilibrium E3. Also if F1(S∗,I∗1)(2−S∗S−Sg1(S,I1)S∗g1(S∗,I∗1))+F2(S∗,I∗2)(2−S∗S−Sg2(S,I2)S∗g2(S∗,I∗2)) +rS∗(3−S∗S−V1V∗1−SV∗1S∗V1)+μS∗(2−S∗S−SS∗)+I1(S∗g1(S,I1)−α1)+I2(S∗ g2(S,I2)+kV1∗−α2)<0. Then E3 is globally asymptotically stable.
In order to discuss the meaning of our mathematical results, let us rewrite the two key indirect parameters R1 and R2 in terms of the rate of vaccination (r), the incidence rate of strain 1 (F1(S,I1)) and the incidence rate of strain 2 (F2(S,I2)) as shown below:
R1=f1(Λr+μ,0)α1, R2=f2(Λr+μ,0)α2+krΛα2μ(r+μ) |
Also, the derivative of R2 with respect to r is,
Λα2(r+μ)2(−∂f2(Λr+μ,0)∂S+k) |
Note that R1(r) is decreasing and R2(r) depends on ∂f2(Λμ,0)∂S. Now we will analyse some cases of incidence rate.
(C1) Fi(S,I)=βiSIi, then ∂f2(Λμ,0)∂S=βi.
(C2) Fi(S,I)=βiSIi1+ζiS, then ∂f2(Λμ,0)∂S=βi1+ζi(Λr+μ).
(C3) Fi(S,I)=βiSIi1+ζiI2i, then ∂f2(Λμ,0)∂S=βi.
Note that for (C1) and (C3), R2(r) is increasing if βi<k, R2(r) is decreasing if βi>k and R2(r) is constant if βi=k. For (C2), R2(r) is increasing if βi≤k (ζ≠0). If βi>k R2(r) is increasing if ζikΛβi−k−μ<r and decreasing if ζikΛβi−k−μ>r.
Furthermore, if the force of infection of strain 1 is (C2), then R1=β1α1(1+ζ1S0), note that R1 is decreasing in ζ1. If the force of infection of strain 2 is (C2), then R2=β2Λα2(λ+ζ2Λ)+krΛα2μλ, note that R2 is decreasing in ζ2.
With the above information and the results in section 3.4, we conclude that the vaccination is always beneficial for controlling strain 1, its impact on strain 2 depends on the force of infection of strain 2. For example, if the force of infection of strain 2 is (C2), the impact of vaccination depends on values of β2, k and ζ2. If ζ2=0 and β2>k it plays a positive role and if ζ2=0 and β2<k, it has a negative impact in controlling strain 2. This is reasonable because larger k (than β2) means that vaccinated individuals are more likely to be infected by strain 2 than those who are not vaccinated, and thus, is helpful to strain 2. Smaller k (than β2) implies the opposite. If ζ2≠0 and β2≤k, it plays a negative role and if ζ2≠0 and β2>k, not necessarily has a positivity impact in controlling strain 2. This is reasonable because larger k (than β2) means that vaccinated individuals are more likely to be infected by strain 2 than those who are not vaccinated, but if ζ2 is large it means that the population is taking precautions to avoid the infection of strain 2. Also, we conclude that ζ1 (of the force of infection (C2)) is always beneficial for controlling strain 1 and ζ2 (of the force of infection (C2)) is always beneficial for controlling strain 2, it means that it is very important that people are taking precautions not to get infected.
This work was supported by Sistema Nacional de Investigadores (15284) and Conacyt-Becas.
No conflict of interest.
[1] |
Egede LE, Ellis C (2008) Development and testing of the multidimensional trust in health care systems scale. J Gen Intern Med 23: 808-815. https://doi.org/10.1007/s11606-008-0613-1 ![]() |
[2] |
Khullar D (2019) Building trust in health care—why, where, and how. JAMA 322: 507-509. https://doi.org/10.1001/jama.2019.4892 ![]() |
[3] | Lewis C, Abrams MK, Seervai S Listening to low-income patients: obstacles to the care we need, when we need it (2017). Available from: https://doi.org/10.26099/g58d-bv09 |
[4] |
Himmelstein DU, Thorne D, Woolhandler S (2011) Medical bankruptcy in Massachusetts: Has health reform made a difference?. Am J Med 124: 224-228. https://doi.org/10.1016/j.amjmed.2010.11.009 ![]() |
[5] |
Kluender R, Mahoney N, Wong F, et al. (2021) Medical debt in the US, 2009–2020. Jama 326: 250-256. https://doi.org/10.1001/jama.2021.8694 ![]() |
[6] |
Wiltshire JC, Elder K, Kiefe C, et al. (2016) Medical debt and related financial consequences among older African American and white adults. Am J Public Health 106: 1086-1091. https://doi.org/10.2105/AJPH.2016.303137 ![]() |
[7] |
Armstrong K, McMurphy S, Dean LT, et al. (2008) Differences in the patterns of health care system distrust between blacks and whites. J Gen Intern Med 23: 827-833. https://doi.org/10.1007/s11606-008-0561-9 ![]() |
[8] |
Khullar D, Darien G, Ness DL (2020) Patient consumerism, healing relationships, and rebuilding trust in health care. JAMA 324: 2359-2360. https://doi.org/10.1001/jama.2020.12938 ![]() |
[9] |
LaVeist TA, Nickerson KJ, Bowie JV (2000) Attitudes about racism, medical mistrust, and satisfaction with care among African American and white cardiac patients. Med Care Res Rev 57 Suppl 1: 146-161. https://doi.org/10.1177/1077558700057001S07 ![]() |
[10] |
Nguyen TC, Gathecha E, Kauffman R, et al. (2022) Healthcare distrust among hospitalised black patients during the COVID-19 pandemic. Postgrad Med J 98: 539-543. https://doi.org/10.1136/postgradmedj-2021-140824 ![]() |
[11] |
Zambrana RE, Williams DR (2022) The intellectual roots of current knowledge on racism and health: Relevance to policy and the national equity discourse: Article examines the roots of current knowledge on racism and health and relevance to policy and the national equity discourse. Health Affairs 41: 163-170. https://doi.org/10.1377/hlthaff.2021.01439 ![]() |
[12] |
Hoffman KM, Trawalter S, Axt JR, et al. (2016) Racial bias in pain assessment and treatment recommendations, and false beliefs about biological differences between blacks and whites. Proc Natl Acad Sci 113: 4296-4301. https://doi.org/10.1073/pnas.1516047113 ![]() |
[13] | Feagin J (2013) Systemic racism: A theory of oppression. New York: Routledge 386. https://doi.org/10.4324/9781315880938 |
[14] |
Swanson ML, Whetstone S, Illangasekare T, et al. (2021) Obstetrics and gynecology and reparations: The debt we owe (and continue to accumulate). Health Equity 5: 353-355. https://doi.org/10.1089/heq.2021.0015 ![]() |
[15] | Hamel L, Lopes L, Muñana C, et al. (2020) Race, health, and COVID-19: The views and experiences of black Americans. Key Findings from the KFF/Undefeated Survey on Race and Health Kaiser Family Foundation . Available from: https://files.kff.org/attachment/Report-Race-Health-and-COVID-19-The-Views-and-Experiences-of-Black-Americans.pdf. |
[16] | Pew Research CenterBlack Americans views of and engagement with science (2022). Available from: https://www.pewresearch.org/wp-content/uploads/sites/20/2022/04/PS_2022.04.07_Black-Americans-and-science_REPORT.pdf. |
[17] |
Martin KJ, Stanton AL, Johnson KL (2022) Current health care experiences, medical trust, and COVID-19 vaccination intention and uptake in black and white Americans. Health Psychol 42: 541-550. https://doi.org/10.1037/hea0001240 ![]() |
[18] | Lopes l MA, Presiado M, Hamel L Americans' challenges with health care costs (2024). Available from: https://www.kff.org/health-costs/issue-brief/americans-challenges-with-health-care-costs/. |
[19] | Ehlers N, Hinkson LR (2017) Subprime health: Debt and race in US medicine. USA: University of Minnesota Press 256. https://doi.org/10.5749/j.ctt1pwt65v |
[20] | Schumacher S HL, Artiga S, Hamel L, et al. Five facts about Black women's experiences in health care (2024). Available from: https://www.kff.org/racial-equity-and-health-policy/issue-brief/five-facts-about-black-womens-experiences-in-health-care/#:~:text=About%20one%20in%20three%20(34,them%20switching%20providers%20(27%25). |
[21] |
Manchikanti L, Helm Ii S, Benyamin RM, et al. (2017) A critical analysis of Obamacare: Affordable care or insurance for many and coverage for few?. Pain Physician 20: 111-138. https://doi.org/10.36076/ppj.2017.138 ![]() |
[22] | Vasquez Reyes M (2020) The disproportional impact of COVID-19 on African Americans. Health Hum Rights 22: 299-307. |
[23] |
Lin JS, Hoffman L, Bean SI, et al. (2021) Addressing racism in preventive services: Methods report to support the US preventive services task force. JAMA 326: 2412-2420. https://doi.org/10.1001/jama.2021.17579 ![]() |
[24] |
Shelton RC, Adsul P, Oh A (2021) Recommendations for addressing structural racism in implementation science: A call to the field. Ethn Dis 31: 357-364. https://doi.org/10.18865/ed.31.S1.357 ![]() |
[25] | Pew Research CenterBlack Americans have a clear vision for reducing racism but little hope It will happen (2022). Available from: https://www.pewresearch.org/wp-content/uploads/sites/20/2022/08/RE_2022.08.30_Black-Voices-Politics_REPORT.pdf. |
[26] |
Williams DR, Lawrence JA, Davis BA (2019) Racism and health: Evidence and needed research. Annu Rev Public Health 40: 105-125. https://doi.org/10.1146/annurev-publhealth-040218-043750 ![]() |
[27] |
Musa D, Schulz R, Harris R, et al. (2009) Trust in the health care system and the use of preventive health services by older black and white adults. Am J Public Health 99: 1293-1299. https://doi.org/10.2105/AJPH.2007.123927 ![]() |
[28] |
Ferraro KF, Kemp BR, Williams MM (2017) Diverse aging and health inequality by race and ethnicity. Innovation Aging 1: igx002. https://doi.org/10.1093/geroni/igx002 ![]() |
[29] | Galama TJ, Van Kippersluis H (2019) A theory of socio-economic disparities in health over the life cycle. Econ J (London) 129: 338-374. https://doi.org/10.1111/ecoj.12577 |
[30] |
Parsons R (2021) Interpreting the American Caste System as racialized economic performance. Am Sociol 52: 367-389. https://doi.org/10.1007/s12108-021-09498-w ![]() |
[31] | Gonzalez D, Kenney GM, McDaniel M, et al. (2021) Perceptions of unfair treatment or judgment due to race or ethnicity in five settings. Washington, DC: Urban Institute. Available from: https://www.urban.org/sites/default/files/publication/104565/perceptions-of-unfair-treatment-or-judgment-due-to-race-or-ethnicity-in-five-settings_0.pdf. |
[32] |
Bowleg L (2012) The problem with the phrase women and minorities: Intersectionality—an important theoretical framework for public health. Am J Public Health 102: 1267-1273. https://doi.org/10.2105/AJPH.2012.300750 ![]() |
[33] |
Cho S, Crenshaw KW, McCall L (2013) Toward a field of intersectionality studies: Theory, applications, and praxis. Signs 38: 785-810. https://doi.org/10.1086/669608 ![]() |
[34] |
Elliott S, Powell R, Brenton J (2015) Being a good mom: Low-income, black single mothers negotiate intensive mothering. J Fam Issues 36: 351-370. https://doi.org/10.1177/0192513X13490279 ![]() |
[35] | Bird CE, Rieker PP (2008) Gender and health: the effects of constrained choices and social policies. New York: Cambridge University Press. https://doi.org/10.1017/CBO9780511807305 |
[36] |
Williams DR, Priest N, Anderson NB (2016) Understanding associations among race, socioeconomic status, and health: Patterns and prospects. Health Psychol 35: 407. https://doi.org/10.1037/hea0000242 ![]() |
[37] |
Shuey KM, Willson AE (2008) Cumulative disadvantage and black-white disparities in life-course health trajectories. Res Aging 30: 200-225. https://doi.org/10.1177/0164027507311151 ![]() |
[38] |
Pfeffer FT, Killewald A (2019) Intergenerational wealth mobility and racial inequality. Socius 5: 2378023119831799. https://doi.org/10.1177/2378023119831799 ![]() |
[39] |
Williams DR (1997) Race and health: Basic questions, emerging directions. Ann Epidemiol 7: 322-333. https://doi.org/10.1016/S1047-2797(97)00051-3 ![]() |
[40] |
Williams DR, Mohammed SA (2013) Racism and health I: Pathways and scientific evidence. Am Behav Sci 57: 1152-1173. https://doi.org/10.1177/0002764213487340 ![]() |
[41] | LaVeist TA (1994) Beyond dummy variables and sample selection: What health services researchers ought to know about race as a variable. Health Serv Res 29: 1-16. |
[42] |
Nuru-Jeter AM, Michaels EK, Thomas MD, et al. (2018) Relative roles of race versus socioeconomic position in studies of health inequalities: A matter of interpretation. Annu Rev Public Health 39: 169-188. https://doi.org/10.1146/annurev-publhealth-040617-014230 ![]() |
[43] |
Cabral DN, Nápoles-Springer AM, Miike R, et al. (2003) Population- and community-based recruitment of African Americans and Latinos: The San Francisco Bay area lung cancer study. Am J Epidemiol 158: 272-279. https://doi.org/10.1093/aje/kwg138 ![]() |
[44] |
Serdar CC, Cihan M, Yucel D, et al. (2021) Sample size, power and effect size revisited: Simplified and practical approaches in pre-clinical, clinical and laboratory studies. Biochem Med (Zagreb) 31: 010502. https://doi.org/10.11613/BM.2021.010502 ![]() |
[45] |
Ozawa S, Sripad P (2013) How do you measure trust in the health system? A systematic review of the literature. Soc Sci Med 91: 10-14. https://doi.org/10.1016/j.socscimed.2013.05.005 ![]() |
[46] |
Rose A, Peters N, Shea JA, et al. (2004) Development and testing of the health care system distrust scale. J Gen Intern Med 19: 57-63. https://doi.org/10.1111/j.1525-1497.2004.21146.x ![]() |
[47] |
Hall MA, Dugan E, Zheng B, et al. (2001) Trust in physicians and medical institutions: What is it, can it be measured, and does it matter?. Milbank Q 79: 613-639. https://doi.org/10.1111/1468-0009.00223 ![]() |
[48] | Geronimus AT (2001) Understanding and eliminating racial inequalities in women's health in the United States: The role of the weathering conceptual framework. J Am Med Womens Assoc (1972) 56: 133-136, 149–150. |
[49] | Mehmetoglu M (2018) Medsem: A Stata package for statistical mediation analysis. Int J Comput Econ Ec 8: 63-78. https://doi.org/10.1504/IJCEE.2018.10007883 |
[50] |
Zhao X, Lynch Jr JG, Chen Q (2010) Reconsidering Baron and Kenny: Myths and truths about mediation analysis. J Consum Res 37: 197-206. https://doi.org/10.1086/651257 ![]() |
[51] | Jacobucci R, Brandmaier AM, Kievit RA (2019) A practical guide to variable selection in structural equation modeling by using regularized multiple-indicators, multiple-causes models. Adv Meth Pract Psych 2: 55-76. https://doi.org/10.1177/2515245919826527 |
[52] |
Stepanikova I, Oates GR (2017) Perceived discrimination and privilege in health care: The role of socioeconomic status and race. Am J Prev Med 52: S86-S94. https://doi.org/10.1016/j.amepre.2016.09.024 ![]() |
[53] |
Daw JR, Winkelman TNA, Dalton VK, et al. (2020) Medicaid expansion improved perinatal insurance continuity for low-income women. Health Aff (Millwood) 39: 1531-1539. https://doi.org/10.1377/hlthaff.2019.01835 ![]() |
[54] |
Graves JA, Hatfield LA, Blot W, et al. (2020) Medicaid expansion slowed rates of health decline for low-income adults in Southern States. Health Aff (Millwood) 39: 67-76. https://doi.org/10.1377/hlthaff.2019.00929 ![]() |
[55] |
De Marchis EH, Doekhie K, Willard-Grace R, et al. (2019) The impact of the patient-centered medical home on health care disparities: Exploring stakeholder perspectives on current standards and future directions. Popul Health Manag 22: 99-107. https://doi.org/10.1089/pop.2018.0055 ![]() |
[56] |
Swietek KE, Gaynes BN, Jackson GL, et al. (2020) Effect of the patient-centered medical home on racial disparities in quality of care. J Gen Intern Med 35: 2304-2313. https://doi.org/10.1007/s11606-020-05729-x ![]() |
[57] |
Reibling N, Rosenthal MB (2016) The (missed) potential of the patient-centered medical home for disparities. Med Care 54: 9-16. https://doi.org/10.1097/MLR.0000000000000451 ![]() |
[58] |
Leung LB, Steers WN, Hoggatt KJ, et al. (2020) Explaining racial-ethnic differences in hypertension and diabetes control among veterans before and after patient-centered medical home implementation. PLoS One 15: e0240306. https://doi.org/10.1371/journal.pone.0240306 ![]() |
[59] |
Hausmann LR, Hannon MJ, Kresevic DM, et al. (2011) Impact of perceived discrimination in healthcare on patient-provider communication. Med Care 49: 626-633. https://doi.org/10.1097/MLR.0b013e318215d93c ![]() |
[60] |
Sim W, Lim WH, Ng CH, et al. (2021) The perspectives of health professionals and patients on racism in healthcare: A qualitative systematic review. PLoS One 16: e0255936. https://doi.org/10.1371/journal.pone.0255936 ![]() |
[61] |
Drossman DA, Ruddy J (2020) Improving patient-provider relationships to improve health care. Clin Gastroenterol Hepatol 18: 1417-1426. https://doi.org/10.1016/j.cgh.2019.12.007 ![]() |
[62] |
Elder K, Ramamonjiarivelo Z, Wiltshire J, et al. (2012) Trust, medication adherence, and hypertension control in Southern African American men. Am J Public Health 102: 2242-2245. https://doi.org/10.2105/AJPH.2012.300777 ![]() |
[63] |
Hagiwara N, Slatcher RB, Eggly S, et al. (2017) Physician racial bias and word use during racially discordant medical interactions. Health Commun 32: 401-408. https://doi.org/10.1080/10410236.2016.1138389 ![]() |
[64] |
Sims M, Diez-Roux AV, Gebreab SY, et al. (2016) Perceived discrimination is associated with health behaviours among African-Americans in the Jackson Heart Study. J Epidemiol Community Health 70: 187-194. https://doi.org/10.1136/jech-2015-206390 ![]() |
[65] |
Williams DR, Cooper LA (2019) Reducing racial inequities in health: Using what we already know to take action. Int J Environ Res Public Health 16: 606. https://doi.org/10.3390/ijerph16040606 ![]() |
[66] | Williams DR, Rucker TD (2000) Understanding and addressing racial disparities in health care. Health Care Financ Rev 21: 75-90. |
[67] |
Cole DA, Maxwell SE (2003) Testing mediational models with longitudinal data: Questions and tips in the use of structural equation modeling. J Abnorm Psychol 112: 558-577. https://doi.org/10.1037/0021-843X.112.4.558 ![]() |
[68] |
Grimes DA, Schulz KF (2002) Bias and causal associations in observational research. Lancet 359: 248-252. https://doi.org/10.1016/S0140-6736(02)07451-2 ![]() |
[69] |
Albert MA, Churchwell K, Desai N, et al. (2024) Addressing structural racism through public policy advocacy: A policy statement from the American Heart Association. Circulation 149: e312-e329. https://doi.org/10.1161/CIR.0000000000001203 ![]() |
[70] | Radley DC SA, Collins SR, Powe NR, et al. Advancing racial equity in U.S. healthc: The Commonwealth Fund 2024 State Health Disparities Report (2024). Available from: https://doi.org/10.26099/vw02-fa96. |
[71] | Hostetter M, Klein S (2021) Understanding and ameliorating medical mistrust among Black Americans. USA: The Commonwealth Fund. Available from: https://www.commonwealthfund.org/publications/newsletter-article/2021/jan/medical-mistrust-among-black-americans. |
[72] |
Doolan DM, Froelicher ES (2009) Using an existing data set to answer new research questions: A methodological review. Res Theory Nurs Pract 23: 203-215. https://doi.org/10.1891/1541-6577.23.3.203 ![]() |
[73] | Wispelwey BP, Marsh RH, Wilson M, et al. (2022) Leveraging clinical decision support for racial equity: A sociotechnical innovation. NEJM Catal 3. https://doi.org/10.1056/CAT.22.0076 |
![]() |
![]() |
1. | Tiancai Liao, Hengguo Yu, Chuanjun Dai, Min Zhao, Impact of Cell Size Effect on Nutrient-Phytoplankton Dynamics, 2019, 2019, 1076-2787, 1, 10.1155/2019/8205696 |