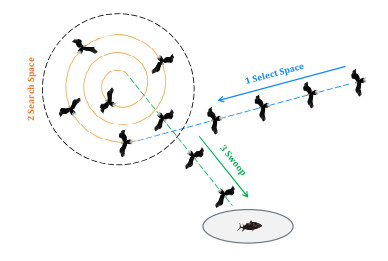
A stochastic Microcystins degradation model with distributed delay is studied in this paper. We first demonstrate the existence and uniqueness of a global positive solution to the stochastic system. Second, we derive a stochastic critical value Rs0 related to the basic reproduction number R0. By constructing suitable Lyapunov function types, we obtain the existence of an ergodic stationary distribution of the stochastic system if Rs0>1. Next, by means of the method developed to solve the general four-dimensional Fokker-Planck equation, the exact expression of the probability density function of the stochastic model around the quasi-endemic equilibrium is derived, which is the key aim of the present paper. In the analysis of statistical significance, the explicit density function can reflect all dynamical properties of a chemostat model. To validate our theoretical conclusions, we present examples and numerical simulations.
Citation: Ying He, Yuting Wei, Junlong Tao, Bo Bi. Stationary distribution and probability density function analysis of a stochastic Microcystins degradation model with distributed delay[J]. Mathematical Biosciences and Engineering, 2024, 21(1): 602-626. doi: 10.3934/mbe.2024026
[1] | Liwei Yang, Lixia Fu, Ping Li, Jianlin Mao, Ning Guo, Linghao Du . LF-ACO: an effective formation path planning for multi-mobile robot. Mathematical Biosciences and Engineering, 2022, 19(1): 225-252. doi: 10.3934/mbe.2022012 |
[2] | Yejun Hu, Liangcai Dong, Lei Xu . Multi-AGV dispatching and routing problem based on a three-stage decomposition method. Mathematical Biosciences and Engineering, 2020, 17(5): 5150-5172. doi: 10.3934/mbe.2020279 |
[3] | Jian Si, Xiaoguang Bao . A novel parallel ant colony optimization algorithm for mobile robot path planning. Mathematical Biosciences and Engineering, 2024, 21(2): 2568-2586. doi: 10.3934/mbe.2024113 |
[4] | Zhenao Yu, Peng Duan, Leilei Meng, Yuyan Han, Fan Ye . Multi-objective path planning for mobile robot with an improved artificial bee colony algorithm. Mathematical Biosciences and Engineering, 2023, 20(2): 2501-2529. doi: 10.3934/mbe.2023117 |
[5] | Li-zhen Du, Shanfu Ke, Zhen Wang, Jing Tao, Lianqing Yu, Hongjun Li . Research on multi-load AGV path planning of weaving workshop based on time priority. Mathematical Biosciences and Engineering, 2019, 16(4): 2277-2292. doi: 10.3934/mbe.2019113 |
[6] | Zhen Zhou, Chenchen Geng, Buhu Qi, Aiwen Meng, Jinzhuang Xiao . Research and experiment on global path planning for indoor AGV via improved ACO and fuzzy DWA. Mathematical Biosciences and Engineering, 2023, 20(11): 19152-19173. doi: 10.3934/mbe.2023846 |
[7] | Tian Xue, Liu Li, Liu Shuang, Du Zhiping, Pang Ming . Path planning of mobile robot based on improved ant colony algorithm for logistics. Mathematical Biosciences and Engineering, 2021, 18(4): 3034-3045. doi: 10.3934/mbe.2021152 |
[8] | Ningning Zhao, Shihao Cui . Study on 4D taxiing path planning of aircraft based on spatio-temporal network. Mathematical Biosciences and Engineering, 2023, 20(3): 4592-4608. doi: 10.3934/mbe.2023213 |
[9] | Zhen Yang, Junli Li, Liwei Yang, Qian Wang, Ping Li, Guofeng Xia . Path planning and collision avoidance methods for distributed multi-robot systems in complex dynamic environments. Mathematical Biosciences and Engineering, 2023, 20(1): 145-178. doi: 10.3934/mbe.2023008 |
[10] | Yuzhuo Shi, Huijie Zhang, Zhisheng Li, Kun Hao, Yonglei Liu, Lu Zhao . Path planning for mobile robots in complex environments based on improved ant colony algorithm. Mathematical Biosciences and Engineering, 2023, 20(9): 15568-15602. doi: 10.3934/mbe.2023695 |
A stochastic Microcystins degradation model with distributed delay is studied in this paper. We first demonstrate the existence and uniqueness of a global positive solution to the stochastic system. Second, we derive a stochastic critical value Rs0 related to the basic reproduction number R0. By constructing suitable Lyapunov function types, we obtain the existence of an ergodic stationary distribution of the stochastic system if Rs0>1. Next, by means of the method developed to solve the general four-dimensional Fokker-Planck equation, the exact expression of the probability density function of the stochastic model around the quasi-endemic equilibrium is derived, which is the key aim of the present paper. In the analysis of statistical significance, the explicit density function can reflect all dynamical properties of a chemostat model. To validate our theoretical conclusions, we present examples and numerical simulations.
The optimization problem refers to the task of finding the maximum (or minimum) value of an objective function under certain constraints [1]. It has extensive applications in various fields such as scientific research, engineering design, economic management, and national defense construction [2]. Currently, optimization methods can be classified into traditional and modern approaches. Traditional optimization methods often rely on precise mathematical models [3] and utilize techniques like linear programming, dynamic programming, integer programming, and branch and bound [4] to solve problems. However, these methods are complex, difficult to implement, and often yield subpar optimization results, making them inadequate for engineering needs. The downside of traditional optimization algorithms is their excessive dependence on precise mathematical models [5]. However, real-life optimization problems are becoming increasingly complex, making it challenging to establish accurate mathematical models. Even in cases where complex mathematical models are constructed, solving them can be difficult. As the complexity of optimization problems continues to rise, traditional optimization algorithms based on precise mathematical models face significant challenges [6].
The metaheuristic algorithm is one of the most widely used methods in the field of modern optimization. It is a computational intelligence-based approach to finding optimal or satisfactory solutions for complex optimization problems [7]. Metaheuristic algorithms are effective in solving NP-hard problems and accurately searching for the best solution to a problem [8]. These algorithms are inspired by the information storage, processing, exchange, adaptation, updating, and evolution mechanisms found in nature. Based on their different sources of inspiration, metaheuristic optimization methods [9] can be categorized into four types: Evolutionary-based methods, such as Genetic Algorithms (GA) [10] and Differential Evolution (DE) [11]; Swarm intelligence-based methods, such as Particle Swarm Optimization (PSO) [12], Ant Colony Optimization (ACO) [13], Whale Optimization Algorithm (WOA) [14], Cuckoo Search (CS) [15], Slime Mold Algorithm (SMA) [16], Marine Predators Algorithm (MPA) [17], African Vultures Optimization Algorithm (AVOA) [18] and Bald Eagle Search (BES) Algorithm [19]; Physics/Chemistry-based methods, such as: Chemical Reaction Optimization (CRO) [20], Archimedes Optimization Algorithm(AOA) [21], and Equilibrium Optimizer (EO) [22]; Human behavior-based methods, such as Teaching-Learning-Based Optimization (TLBO) [23] and Gaining-sharing Knowledge Based Algorithm (GSK) [24]. The metaheuristic algorithm is a powerful tool for solving complex optimization problems and has found applications in various fields. It can provide solutions to complex real-world problems.
Three-dimensional unmanned aerial vehicle (UAV) path planning involves determining optimal routes for UAVs in three-dimensional space [25]. This process considers factors such as obstacles, mission objectives, and UAV capabilities to plan efficient and collision-free paths. Advanced algorithms, such as A* or Rapidly-exploring Random Trees (RRT), are utilized to generate paths that optimize criteria like energy consumption, time, or safety [26,27]. Three-dimensional UAV path planning finds applications in various fields, including aerial surveillance, package delivery, precision agriculture, and disaster response [28]. By enabling UAVs to navigate complex environments, this technology enhances their autonomy and effectiveness in performing a wide range of tasks. PSO, ACO, WOA, and other classic metaheuristic algorithms can also solve this problem, but these algorithms often have shortcomings such as low optimization accuracy, long search path and slow convergence speed. These algorithms are powerless to solve the path planning problem, especially the complicated three-dimensional path planning problem, and cannot find the optimization path well.
The Bald Eagle Search (BES) Algorithm [19] is a metaheuristic algorithm developed by H.A. Alsattar in 2020. Inspired by the hunting tactics and social behavior of bald eagles when hunting fish, the algorithm comprises three stages: Selection, search, and swooping. The BES algorithm is characterized by its simplicity, few control parameters, and exceptional global search capabilities, making it effective in solving various complex optimization problems [29]. According to the NFL theorem [30], any algorithm has two sides. The BES algorithm also has problems such as a somewhat complex structure, easy to fall into local optimality, and global search accuracy that needs to be improved. Since its inception, the BES Algorithm has attracted numerous researchers who have explored its applications in engineering optimization [29], biomedical science [31], mobile edge computing [32], energy cell [33], forest fire detection [34], active distribution systems [35], face recognition [36], autonomous driving [37], and other fields. Because of these advantages of bald eagle search algorithm, it can solve the problem of three-dimensional path planning efficiently and excellent. In addition, the BES algorithm has not been applied to the three-dimensional path planning problem. In order to broaden the application field of the algorithm, we set up five 3D geographical environment maps to simulate the flight situation of UAVs in real conditions, and creatively apply the recently proposed excellent performance of the bald eagle search algorithm to the 3D path planning problem with complex geographical environments.
There are also some studies on the bald eagle search algorithm in path planning problems. Chen et al. [38] proposed a self-adaptive hybrid bald eagle search (SAHBES) algorithm, which is used to solve the path planning problem of unmanned ships in complex waters. Although the author improved the bald eagle search algorithm and applied it to the path planning problem, the path planning scenario of this article is relatively simple. It solves only simple path planning on a two-dimensional plane without combining it with actual problems. Second, there are also problems such as fewer comparison algorithms, insufficient fitness function, and insufficient comprehensive and complex constraints. In the literature [39], the author combined the search and swoop mechanisms of the bald eagle search algorithm and proposed an improved QPSO algorithm to solve the smooth path planning problem. Although this study has many comparative algorithms and relatively advanced improvement strategies, its path planning application scope is limited to a two-dimensional plane, its obstacles are relatively simple, idealistic and it does not incorporate realistic scenarios. Therefore, to expand the application scope of the bald eagle search algorithm and enable the algorithm to solve problems in three-dimensional space, we combined the geographical environment and the actual situation, designed a relatively objective and comprehensive constraint conditions as the fitness function and a variety of complex terrain obstacles, so as to solve the complex three-dimensional path planning problem in real life.
The paper is organized as follows: The related work Section 2 provides an introduction to the bald eagle search algorithm and the three-dimensional path planning problem. Section 3 presents the experimental results and discussion. Statistical test analysis is covered in Section 4. Section 5 is the conclusions and future works.
The Bald Eagle Search (BES) algorithm, proposed by H.A. Alsattar [28] in 2020. It draws inspiration from the foraging behavior of bald eagles and is known for its robust global search capability. The algorithm is divided into three parts: Select search space, search within the selected search space and swooping. The three predation stages of BES are shown in Figure 1.
In the select stage, the bald eagle begins by identifying and selecting the most promising location within the defined search space. This selection is based on the quantity of available food in each area. The mathematical model for this stage can be expressed as follows:
Pi,new=Pbest+α∗r(Pmean−Pi) | (1) |
where Pi,new is the updated position of the i−th bald eagle. Pbest Indicates the current best position of the bald eagle. The position change parameter α∈(1.5,2) and r is a random number belonging to (0, 1). Moreover, Pmean is the average position of the bald eagle. Pi denotes the i−th position of the bald eagle.
Once the select stage is completed, the BES enters the search stage. During this stage, the algorithm emulates the hunting behavior of bald eagle by systematically searching for prey within the previously identified area. The eagle moves in a circular motion, gradually expanding their search in a spiral shape. The mathematical representation for this stage can be formulated as follows:
Pi,new=Pi+y(i)∗(Pi−Pi+1)+x(i)∗(Pi−Pmean) | (2) |
x(i)=xr(i)max(|xr|) y(i)=yr(i)max(|yr|) | (3) |
xr(i)=r(i)∗sin(θ(i)) yr(i)=r(i)∗cos(θ(i)) | (4) |
θ(i)=a∗π∗rand and r(i)=θ(i)+R∗rand | (5) |
where x(i) and y(i) are the position of bald eagle in polar space, they all have values between −1 and 1. Pi+1 is the next updated position of the i - th bald eagle. θ(i) and r(i) are the polar angle and polar diameter. a and R are spiral parameters, ranging from: (5, 10), (0.5, 2). rand is a random number, its value ranges from 0 to 1.
Finally, during the swooping stage, the bald eagle dives to prey on the locked-in prey in the search space. Eq (6) describes the hunting behavior of bald eagle during this stage.
Pi,new=rand∗Pbest+x1(i)∗(Pi−c1∗Pmean)+y1(i)∗(Pi−c2∗Pbest) | (6) |
x1(i)=xr(i)max(|xr|) y1(i)=yr(i)max(|yr|) | (7) |
xr(i)=r(i)∗sinh(θ(i)) yr(i)=r(i)∗cosh(θ(i)) | (8) |
θ(i)=a∗π∗rand and r(i)=θ(i) | (9) |
The BES includes enhancement coefficients c1 and c2, which each have a value between 1 and 2. For a more comprehensive understanding of the BES algorithm, Algorithm 1 provides the pseudocode, while Figure 2 presents the flow chart.
Algorithm1 Pseudo-code of the BES algorithm |
Input: population size N, dimension dim, maximum iteration number Maxiter, |
upper and lower bounds up, lb |
Output: the optimal solution |
1. Random initialization Point Pi |
2. Calculate the fitness values |
3. While (t < Max_iter) |
Select stage |
4. For (point i) |
5. Update individual position using Eq (6) |
6. If f(Pnew)<f(Pi) |
7. Pi=Pnew |
8. End If |
9. If f(Pnew)<f(Pbest) |
10. Pbest=Pnew |
11. End If |
12. End For |
Search stage |
13. For (point i) |
14. Update individual position using Eq (7) |
15. If f(Pnew)<f(Pi) |
16. Pi=Pnew |
17. End If |
18. If f(Pnew)<f(Pbest) |
19. Pbest=Pnew |
20. End If |
21. End For |
Swoop stage |
22. For (point i) |
23. Update individual position using Eq (11) |
24. If f(Pnew)<f(Pi) |
25. Pi=Pnew |
26. End If |
27. If f(Pnew)<f(Pbest) |
28. Pbest=Pnew |
29. End If |
30. End For |
31. End While |
Three-dimensional path planning refers to the process of determining an optimal path in a three-dimensional space with obstacles, aiming to reach the target location while satisfying various constraints such as distance, altitude, threat zones, flight time, and energy consumption. The objective of this planning is to find a path that is as close as possible to the desired destination while adhering to the mentioned constraints [40].
At present, swarm intelligence algorithm has become a hot topic to solve the path planning problem, such as HG-SMA [41], modified SMOA algorithm [42], ABCL algorithm [43]; improvement adaptive ant colony algorithm [44], adaptive selection mutation constraint differential evolution (CDE) algorithm [45], etc.
The first step in using swarm intelligence algorithms to solve three-dimensional path planning problems is to establish an abstract model of three-dimensional space, that is, to establish a digital geographic environment model. The specific method is to extract and analyze features of the three-dimensional real geographic environment through computers, and convert the real environment into storable and processable map model data [46].
In the environmental modeling, due to the advantages of grid method, such as simple, convenient map creation and maintenance, and easy operation, we use grid method for mathematical modeling in this paper, and discretization the three-dimensional environment model with grid as the basic unit [9]. The methods for spatial modeling are as follows:
1). Use the vertex in front of the left of the three-dimensional map as the coordinate origin of the three-dimensional space, at which a three-dimensional coordinate system is established, where the x-axis increases along the longitude, the y-axis increases along the latitude, and the z-axis is perpendicular to the ground. A three-dimensional cube area constructed with the origin as the vertex, along the x-axis, y-axis, and z-axis is the planned space for the three-dimensional path, as shown in Figure 1.
2). After the three-dimensional planning space is established, the three-dimensional space is divided along the XY plane, XZ plane and YZ plane to get the grid points needed for n planes and three-dimensional paths, and then the planes are intersected to form intersections. After each intersection point in the three-dimensional graph is obtained, the coordinates of each intersection point can be obtained, and then the bald eagle search algorithm is used to optimize the selection of the optimal intersection point as the path point to form the three-dimensional optimal path.
3). When conducting environmental modeling, we have discretized the entire search space into a series of three-dimensional discrete points to obtain the position coordinates of these points. The bald eagle search algorithm is used to optimize these discrete points, so as to iteratively search out the optimal intersection points and find the optimal path when the conditions meet the constraints most.
We transform a continuous space into a set of discrete points by constructing the cuboid shown in Figure 3 to simulate the search area of the UAV. If the actual area searched by the UAV is 20 × 20 × 1 km in length, width and height, then we can construct a 20 × 20 × 2 km cuboid to cover the specified area. Using the above division method, the space is divided into 20 planes, that is, there are 20 waypoints on a path, and the waypoints on each plane are two-dimensional, thus the environment is modeled step by step. A mathematical foundation is created for solving the path planning problem with BES algorithm.
The geographical environment required by UAV three-dimensional path planning needs to be extracted from the geographical model, and a good terrain model can test the performance of the algorithm well. In the geographical environment, a mountain model needs to be established, which simulates the real geographical environment and enables the UAV to find the optimal path in the obstacle area. The mathematical formula of the mountain model [40] is as follows:
Z(x,y)=n∑i=1hiexp[−(x−xixsi)2−(y−yiysi)2] | (10) |
Z is the elevation, n is the number of peaks, hi is the terrain parameter, which controls the terrain height, (xi, yi) represents the central coordinate of the i-th peak, and xsi and ysi are the slopes of the peaks that control the x, y axis.
After establishing the geographical environment, the 3D path planning also needs to consider the constraints of the UAV itself, including the maximum range constraint, the minimum height constraint, the maximum pitch angle constraint, etc. [46].
The first is the maximum range constraint of UAV. Due to the impact of fuel on UAV, the flying distance of UAV is restricted. It is assumed that there are n nodes on a single range of UAV, the maximum range is Lmax, and the distance in the i section of the path node can be expressed as Li. Therefore, the total distance of the UAV along the whole route is [47]:
Flength(xi)=N−1∑i=1‖Pi+1−Pi‖ | (11) |
where Pi represents the coordinates of the i-th layer grid plane path point (xi, yi, zi), ‖Pi+1−Pi‖ is the Euclidean distance between pi and the next path point pi+1, expressed as √(xi+1−xi)2+(yi+1−yi)2+(zi+1−zi)2, N is the total number of waypoints, and under other conditions equal, the shorter the path length, the better the effect.
The second is the minimum altitude constraint. When the UAV flies in the complex terrain, the flight altitude may be reduced. At this time, the UAV needs to properly reduce the flight altitude to complete the task target. However, too low flight altitude can cause the UAV to collide with complex terrain, which is dangerous. Therefore, in order to ensure safety, the flight altitude of the UAV is restricted and constrained, its fitness function can be expressed [48]:
Fmin_height(xi)={hi−ΔHhi≥ΔHkh0<hi<ΔH∞hi≤0 | (12) |
ΔH is the optimal flight altitude; hi is the height of UAV above the ground, the positive number kh is the constraint value.
Among the UAV's own constraints, the adjustment of the maximum pitch angle constraint is an important link in the 3D path planning of UAV. The maximum pitch angle is the angle limiting case from the current flying point to the next point in the vertical direction [48]. Only when the maximum pitch angle is less than or equal to, the UAV can fly safely. The following is the calculation formula [49] of the maximum pitch angle of the UAV:
tanθmax⩾ | (13) |
The formula represents the maximum pitch climb from the current flight point (xi-1, yi-1, zi-1) to the next flight point (xi, yi, zi).
F_{ {angle }}\left(x_i\right) = \begin{cases}0 & \theta \leq \theta_{\max } \\ k & \theta > \theta_{\max }\end{cases} | (14) |
where, θ is the climbing angle of the UAV; θmax is the maximum climbing angle of the UAV; K is the constraint value.
Based on the above objective function, we obtain the fitness function of UAV path planning:
F = \sum\limits_i {({\omega _1}{F_{length}}({x_i}) + {\omega _2}{F_{min\_height}}({x_i}) + {\omega _3}{F_{angle}}({x_i}))} \ \ \ \ \ i = 1, ..., n | (15) |
where, ω1, ω2, and ω3 represent the weights of the three objective functions, and ω1 + ω2 + ω3 = 1. Through multiple experiments, this article adjusts and selects appropriate weight assignments as ω1, ω2, and ω3, all of which are 1/3, so that the planned paths can relatively meet the needs of UAV navigation routes on all three objective functions.
All the experiments in the paper are run in MATLAB R2017 (b) environment, the CPU is Inter (R) Core (TM) i7-97000, the main frequency is 3.00 GHz, a 64-bit operating system, and 16 GB RAM desktop computer.
To verify the performance and effectiveness of the bald eagle search algorithm, we compared it with five algorithms: PSO [50], GWO [51], ACO [52], ChOA [9], and TLBO [53], to solve the three-dimensional path planning problem of UAV. In the experiment, the number of iterations was 500, the population size was 100, and 10 runs were conducted. Table 1 displays the precise parameter configurations.
Algorithms | Parameters | Values | Algorithms | Parameters | Values |
ACO | Pheromone factor α | 4 | ChOA | random number r1, r2 | [0, 1] |
Heuristic function factor β | 5 | Convergence factor f | [2.5, 0] | ||
Pheromone volatilization factor ρ | 0.2 | TLBO | Teaching factor TF | {1, 2} | |
GWO | Convergence factor a | [2, 0] | Learning step ri | [0, 1] | |
PSO | Inertia weight w | 1 | BES | Position change parameterα | 2 |
Cognitive coefficient c1 | 2 | Spiral parameter a, R | 10, 1.5 | ||
Social coefficient c2 | 2 | Enhancement coefficient c1, c2 | 2 | ||
Maximum velocity v | 6 | random number r | [0, 1] |
Since these algorithms are popular algorithms, relatively classic, and widely used, we wrote algorithm programs to implement UAV three-dimensional path planning problems. Taking the implementation process of ACO algorithm as an example, a set of initial paths are randomly generated, which can be regarded as the initial movement trajectories of ants. In each iteration, the pheromone on the path is updated based on the ant's movement trajectory and selection probability. The calculation of the selection probability is usually based on the concentration of the pheromone and the value of the heuristic function. According to the ants' movement rules, constraints, and objective functions, each ant is moved in turn until all ants reach the target point or the preset number of iterations is reached. Among the movement trajectories of all ants, the optimal path is selected as the best path for this iteration. Then, we determine whether the termination conditions are met, such as reaching the preset maximum number of iterations or the length of the optimal path no longer changing significantly, etc. The optimal path is output as the final three-dimensional path planning result [54].
To better test the performance of different algorithms, and use the bald eagle search algorithm to solve the UAV three-dimensional path planning problem, we set five three-dimensional geographic environment maps to simulate the flight situation of the UAV in the real situation. Five three-dimensional maps are gradually developed, from simple to complex, covering various flight environments of unmanned aerial vehicles in real situations, including geographical features, terrain height, mountain peak height, number and density of mountains, the degree of undulation, and so on. These three-dimensional geographic environment maps can be a good test of the ability of the algorithm to deal with path planning problems in various terrain, including extreme environments, and test the limit performance of the algorithm.
Five three-dimensional geographical environment maps are randomly obtained by Eq (1), and the height range of the mountains in the generated map is set from 0 to 1000. The number and height of the mountain peaks and other geographical environments change from simple to complex. Scenario 1 is the simplest terrain in the scene, with flat terrain near the starting point and the endpoint without occlusion of mountains. The number of mountains in the scene is small, the height of the peaks is low, there is a certain interval between the mountains, the distribution is sparse, and the terrain and landform are relatively single, so the UAV path planning problem is less difficult. Scenario 2 has increased the height of the mountain peak, increased the number and density of mountains, and added mountain obstacles near the endpoint, resulting in a more complex shape of the mountain. In Scenario 3, the difficulty is further increased, the mountain density is also expanded while the height of the mountain is increased, the distance between the mountains is shortened, there are a certain number of obstacles near the starting point and the end point, and the terrain in the scene has certain ups and downs, which can test the performance of the algorithm. The terrain and topography of Scenario 4 are more complex, with mountain peaks exceeding 900m in height, and the density and number of peaks becoming extremely dense. There are not only a large number of rolling hills and mountains in the map, but also very small distances between obstacles. The drone needs to leap over extreme terrain and topography from the starting point to the end point, which will pose a great challenge to the performance of the algorithm. In scenario 5, although the height of the peak has decreased, there is no plain in the map, all the terrain is hills, mountains and peaks, and large mountains and small mountains are interlaced, forming the extreme terrain of mountains and mountains, and the terrain near the starting point and the end point is the most complex terrain. This geographical landscape will test the extreme performance of the algorithm in solving UAV path planning problems.
The detailed parameter settings of the five 3D geographic environment maps are shown in Table 2. Through the five three-dimensional geographical environment scenes, the superiority of the algorithm can be tested step by step, the scheme of all the algorithms in solving the three-dimensional path planning problem of UAV can be obtained, the advantages and disadvantages of various algorithms can be judged, and the best performing algorithm can be used to better solve the three-dimensional path planning problem of UAV.
Actual search area | Meshing status | Number of waypoints | Start point | end point | |
Scenario 1 | 20 × 20× 2 (km) | 20 × 20× 2 | 20 | (20, 7) | (6, 5) |
Scenario 2 | 40 × 40× 2 (km) | 40 × 40× 2 | 40 | (40, 14) | (12, 10) |
Scenario 3 | 60 × 60× 2 (km) | 60 × 60× 2 | 60 | (60, 21) | (18, 15) |
Scenario 4 | 80 × 80× 2 (km) | 80 × 80× 2 | 80 | (80, 28) | (24, 20) |
Scenario 5 | 90 × 90× 2 (km) | 90 × 90× 2 | 90 | (90, 35) | (30, 25) |
In Scenario 1 of Figure 4, the first image shows a comprehensive view of paths for different algorithms, and the second image shows a top view of paths for different algorithms, the color bar on the right show the heights of the different landforms. In the simplest terrain scenario 1, as shown in Figure 4, where there are fewer mountains, all six algorithms can find their optimized routes and achieve shorter paths. However, the Choa algorithm is lacking in obstacle avoidance capabilities, as it cannot avoid certain mountains. On the other hand, the BES algorithm exhibits faster convergence and better convergence accuracy, being able to correctly navigate around the mountains and find the optimal route as shown in Figure 5. In Figure 6, with an increased number and height of mountains, the path routes taken by the algorithms are generally consistent. The ChOA algorithm fails to avoid certain mountains. It is evident that the BES algorithm is able to find the shortest path and performs the best among the compared algorithms.
In the more complex terrain of Figure 8, the differences between the algorithms begin to show. The GWO, ChOA, and TLBO algorithms find longer paths but with faster convergence, while the PSO algorithm converges slower but with good accuracy as shown in Figure 9. Both the PSO and ChOA algorithms exhibit cases where they fail to avoid the mountains. The BES algorithm performs the best in terms of both accuracy and speed, making it more competitive in finding optimal paths. In Figures 10 and 11, with the highly challenging terrain scenario 4, the BES algorithm also demonstrates excellent performance by finding the best route even in extremely complex and challenging terrain formations, with the lowest fitness value. On the other hand, the other algorithms find longer routes and perform poorly as they fail to avoid the mountains. In the most challenging scenario 5 as shown in Figure 12, all algorithms perform similarly, with minimal differences in the optimized path lengths. However, as can be seen in Figure 13, the BES algorithm exhibits the best performance by converging to the optimal fitness value in a shorter time period. Algorithms such as ChOA and ACO find longer routes and perform poorly.
These experimental results indicate that, in comparison to the other five intelligent optimization algorithms. PSO and ChOA algorithms perform poorly, not only searching for longer paths, but also unable to safely avoid obstacles, posing a strong security risk. Other algorithms are also inferior to BES in all aspects. The BES algorithm shows outstanding performance in terms of convergence accuracy and speed, as well as obstacle avoidance capabilities. It can find the optimal route in the shortest time, demonstrating stronger competitiveness and providing excellent solutions to three-dimensional path planning problems for unmanned aerial vehicles.
Table 3 displays the best values, worst values, mean values, and standard deviations obtained by the BES algorithm and the other five intelligent optimization algorithms in the five terrain scenarios. It can be observed that the BES algorithm performs the best among these indicators, consistently achieving the best results in the majority of the values. Especially in scenarios 4 and 5 with extremely complex terrain, the best values and average values of BES algorithm are the best, reflecting the excellent performance of BES algorithm. The ACO algorithm follows closely in performance. In the experimental data, it can also be reflected that the performance of PSO and ChOA algorithms in solving this problem is lacking. This demonstrates that the BES algorithm is significantly ahead of the other algorithms in terms of data, showcasing its remarkable competitiveness and stability. It is capable of effectively solving three-dimensional path planning problems for unmanned aerial vehicles.
PSO | GWO | ACO | ChOA | TLBO | BES | ||
Scenario 1 | Best | 98.09 | 102.56 | 100.93 | 104.72 | 84.34 | 76.67 |
Worst | 192.58 | 176.14 | 117.30 | 169.34 | 144.96 | 145.36 | |
Mean | 138.10 | 104.04 | 102.59 | 106.89 | 98.38 | 79.95 | |
Std | 25.38 | 3.56 | 2.51 | 5.52 | 8.80 | 8.07 | |
Scenario 2 | Best | 163.36 | 153.75 | 150.57 | 162.63 | 155.46 | 105.81 |
Worst | 304.71 | 259.47 | 158.89 | 230.32 | 261.42 | 217.77 | |
Mean | 202.77 | 154.87 | 151.83 | 161.07 | 174.50 | 111.25 | |
Std | 27.06 | 4.93 | 2.50 | 10.30 | 12.87 | 14.34 | |
Scenario 3 | Best | 179.71 | 203.68 | 199.36 | 215.94 | 202.28 | 154.89 |
Worst | 368.47 | 354.75 | 291.82 | 364.91 | 298.86 | 288.89 | |
Mean | 254.43 | 206.00 | 205.54 | 216.46 | 228.34 | 161.42 | |
Std | 44.15 | 7.62 | 5.92 | 12.54 | 21.73 | 15.22 | |
Scenario 4 | Best | 316.68 | 300.72 | 311.22 | 313.8 | 291.81 | 271.80 |
Worst | 592.86 | 549.12 | 357.00 | 530.88 | 467.82 | 459.24 | |
Mean | 411.69 | 307.98 | 313.02 | 322.71 | 332.70 | 276.06 | |
Std | 46.14 | 18.27 | 8.37 | 17.94 | 32.08 | 12.06 | |
Scenario 5 | Best | 416.56 | 404.84 | 445.96 | 482.76 | 428.44 | 368.24 |
Worst | 897.32 | 869.08 | 520.56 | 752.76 | 852.92 | 760.12 | |
Mean | 565.12 | 412.6 | 490.16 | 431.40 | 444.36 | 381.60 | |
Std | 94.72 | 21.92 | 6.09 | 35.44 | 30.60 | 41.64 |
Figure 14 shows the running time comparison results of all algorithms. It can be seen from the figure that in the five scenarios, the time spent by the BES algorithm is shorter, which is lower than the running time of other comparison algorithms. The experimental results show that the ChOA algorithm has the longest running time, the TLBO algorithm and the BES algorithm have similar time consumption, but the BES algorithm takes the shortest time. This further proves the excellent performance of the BES algorithm in solving the three-dimensional path planning problem of UAVs, and can find the optimal path of UAVs in a short time.
To further evaluate BES, we used a non-parametric Wilcoxon rank sum statistical test, which allows us to determine whether there are significant differences between data sets. The Wilcoxon rank sum test provides a useful statistical method for assessing differences between samples, and its results can be interpreted through the analysis of p-values [55]. When the P-value falls below the pre-determined significance threshold (typically 0.05), we dismiss the null hypothesis - which posits that the two samples originate from the same population. Furthermore, we deem the medians of the two samples to be notably dissimilar [56]. Conversely, if the P-value exceeds the significance level, we are unable to discard the null hypothesis. In this paper, we conducted statistical tests on 5 terrain scenes, and the statistical results of each comparative algorithm are shown in Table 4. From the experimental results, it can be seen that all Wilcoxon rank sum test results do not exceed 0.05. The statistical analysis results show that BES has significant differences compared to other algorithms and is significantly better than other algorithms.
Algorithms | Scenario 1 | Scenario 2 | Scenario 3 | Scenario 4 | Scenario 5 |
BES vs PSO | 3.76 × 10−5 | 5.57 × 10−11 | 5.03 × 10−4 | 0.0033 | 7.01 × 10−18 |
BES vs GWO | 1.10 × 10−6 | 3.72 × 10−15 | 6.18 × 10−4 | 1.00 × 10−17 | 8.87 × 10−32 |
BES vs ACO | 5.93 × 10−12 | 1.30 × 10−13 | 2.02 × 10−17 | 2.21 × 10−18 | 1.74 × 10−10 |
BES vs ChOA | 2.21 × 10−9 | 5.37 × 10−16 | 1.09 × 10−16 | 4.11 × 10−7 | 3.33 × 10−13 |
BES vs TLBO | 2.76 × 10−11 | 4.46 × 10−16 | 1.52 × 10−27 | 4.80 × 10−5 | 2.51 × 10−25 |
The Friedman Rank Test is a non-parametric statistical test used to compare multiple paired or related samples [57]. The test works by ranking the combined observations from all the groups [58]. In this experiment, we ranked the performance indicators of different algorithms in different testing scenarios. In Table 5, it can be clearly seen that BES has the best average performance in five scenarios and ranks first among all algorithms, further demonstrating the excellent performance of the BES algorithm, which can perfectly solve the three-dimensional path planning problem of unmanned aerial vehicles. These results are consistent with previous experiments.
Algorithms | Scenario 1 | Scenario 2 | Scenario 3 | Scenario 4 | Scenario 5 | |||||
Mean score | Rank | Mean score | Rank | Mean score | Rank | Mean score | Rank | Mean score | Rank | |
PSO | 4.50 | 5 | 4.00 | 5 | 4.75 | 5 | 4.00 | 5 | 3.55 | 5 |
GWO | 4.00 | 4 | 3.50 | 3 | 3.75 | 4 | 3.50 | 3 | 2.75 | 2 |
ACO | 2.25 | 2 | 1.75 | 1 | 2.00 | 2 | 2.25 | 2 | 3.25 | 3 |
ChOA | 5.25 | 6 | 6.00 | 6 | 5.50 | 6 | 6.00 | 6 | 5.50 | 6 |
TLBO | 2.75 | 3 | 3.75 | 4 | 3.25 | 3 | 3.75 | 4 | 3.50 | 4 |
BES | 2.20 | 1 | 2.05 | 2 | 1.75 | 1 | 1.50 | 1 | 2.50 | 1 |
To broaden the application scope of the bald eagle search (BES) algorithm and better test its performance, we utilize the BES algorithm to solve the three-dimensional path planning problem for unmanned aerial vehicle (UAV). We simulate the flight scenarios of UAVs in real-life situations using five three-dimensional geographic environment maps. These maps effectively test the algorithm's ability to handle various terrains, including extreme environments, and evaluate its performance limits in path planning. When comparing the results with five other intelligent optimization algorithms, it is observed that the BES algorithm performs the best in terms of accuracy and speed in regards to fitness functions that include the maximum range constraint, minimum altitude constraint, and maximum pitch climb. The algorithm demonstrates strong convergence, while other intelligent algorithms show significant differences in terms of optimal values, worst values, average values, and standard deviation. This indicates that the BES algorithm has excellent performance, good stability, and the ability to effectively solve complex three-dimensional path planning problems. These achievements are attributed to the BES algorithm's powerful global search capability and unique spiraling predation mechanism, which allow the algorithm to have strong coverage in the search space. Furthermore, the BES algorithm also excels in statistical analysis, achieving remarkable results. These experimental results prove the outstanding performance and competitiveness of the BES algorithm in solving three-dimensional path planning problems quickly, stably, and effectively. Since the Bald Eagle Search algorithm has not undergone any modifications in its original version, further optimization can be conducted in the future to improve the performance of BES. This can be achieved by adding effective strategies, modifying adaptive parameters, simplifying the search stage and swoop stages, and so on. BES can be utilized to solve more complex problems such as: Clustering problem [59], three-dimensional wireless sensor problems [60], job shop scheduling problems [61], and spherical traveling salesman problems [62].
The authors declare that they have not used Artificial Intelligence (AI) tools in the creation of this article.
This research is funded by the 2023 General Scientific Research Project of the Zhejiang Provincial Department of Education, grant number Y202351454; and the 2023 General Planning Project (Universities) of the Zhejiang Provincial Education Science Planning, grant number 2023SCG076.
All authors declare no conflicts of interest in this paper.
[1] |
J. Li, R. Li, J. Li, Current research scenario for microcystins biodegradation—a review on fundamental knowledge application prospects and challenges, Sci. Total Environ., 595 (2017), 615–632. https://doi.org/10.1016/j.scitotenv.2017.03.285 doi: 10.1016/j.scitotenv.2017.03.285
![]() |
[2] |
J. Li, K. Shimizu, H. Maseda, Z. Lu, M. Utsumi, Z. Zhang, et al., Investigations into the biodegradation of microcystin-LR mediated by the biofilm in wintertime from a biological treatment facility in a drinking-water treatment plant, Bioresour. Technol., 106 (2012), 27–35. https://doi.org/10.1016/j.biortech.2011.11.099 doi: 10.1016/j.biortech.2011.11.099
![]() |
[3] |
K. Shimizu, H. Maseda, K. Okano, T. Kurashima, Y. Kawauchi, Q. Xue, et al., Enzymatic pathway for biodegrading microcystin LR in sphingopyxis sp. C-1, J. Biosci. Bioeng., 114 (2012), 630–634. https://doi.org/10.1016/j.jbiosc.2012.07.004 doi: 10.1016/j.jbiosc.2012.07.004
![]() |
[4] |
K. Song, W. Ma, K. Guo, Global behavior of a dynamic model with biodegradation of microcystins, J. Appl. Anal. Comput., 9 (2019), 1261–1276. https://doi.org/10.11948/2156-907X.20180215 doi: 10.11948/2156-907X.20180215
![]() |
[5] |
K. Ge, K. Song, W. Ma, Existence of positive periodic solutions of a delayed periodic Microcystins degradation model with nonlinear functional responses, Appl. Math. Lett., 131 (2022), 108056. https://doi.org/10.1016/j.aml.2022.108056 doi: 10.1016/j.aml.2022.108056
![]() |
[6] |
J. Caperon, Time lag in population growth response of isochrysis galbana to a variable nitrate environment, Ecology, 50 (1969), 188–192. https://doi.org/10.2307/1934845 doi: 10.2307/1934845
![]() |
[7] |
M. Droop, Vitamin B12 and marine ecology. IV. The kinetics of uptake, growth and inhibition in monochrysis lutheri, J. Mar. Biol. Assoc. UK, 48 (1968), 689–733. https://doi.org/10.1017/S0025315400019238 doi: 10.1017/S0025315400019238
![]() |
[8] |
B. Li, G. Wolkowicz, Y. Kuang, Global asymptotic behavior of a chemostat model with two perfectly complementary resources and distributed delay, SIAM J. Appl. Math., 60 (2000), 2058–2086. https://doi.org/10.1137/S0036139999359756 doi: 10.1137/S0036139999359756
![]() |
[9] |
K. Song, W. Ma, Z. Jiang, Bifurcation analysis of modeling biodegradation of microcystins, Int. J. Biomath., 12 (2019), 1950028. https://doi.org/10.1142/S1793524519500281 doi: 10.1142/S1793524519500281
![]() |
[10] | N. Macdonald, Time lags in biological models, in Lecture Notes in Biomathematics, Springer-Verlag, Heidelberg, 1978. https://doi.org/10.1007/978-3-642-93107-9 |
[11] |
T. C. Gard, Persistence in stochastic food web models, Bull. Math. Biol., 46 (1984), 357–370. https://doi.org/10.1007/bf02462011 doi: 10.1007/bf02462011
![]() |
[12] |
M. Bandyopadhyay, J. Chattopadhyay, Ratio-dependent predator–prey model: Effect of environmental fluctuation and stability, Nonlinearity, 18 (2005), 913–936. https://doi.org/10.1088/0951-7715/18/2/022 doi: 10.1088/0951-7715/18/2/022
![]() |
[13] |
I. Addition, Extinction and persistence in mean of a novel delay impulsive stochastic infected predator–prey system with jumps, Complexity, 2017 (2017), 1950970. https://doi.org/10.1155/2017/1950970 doi: 10.1155/2017/1950970
![]() |
[14] |
X. Meng, S. Zhao, T. Feng, T. Zhang, Dynamics of a novel nonlinear stochastic SIS epidemic model with double epidemic hypothesis, J. Math. Anal. Appl., 433 (2016), 227–242. https://doi.org/10.1016/j.jmaa.2015.07.056 doi: 10.1016/j.jmaa.2015.07.056
![]() |
[15] |
X. Leng, T. Feng, X. Meng, Stochastic inequalities and applications to dynamics analysis of a novel SIVS epidemic model with jumps, J. Inequal. Appl., 2017 (2017), 138. https://doi.org/10.1186/s13660-017-1418-8 doi: 10.1186/s13660-017-1418-8
![]() |
[16] | R. M. May, Stability and Complexity in Model Ecosystems, Princeton University Press, 2001. |
[17] |
B. Han, D. Jiang, T. Hayat, A. Alsaedi, B. Ahmad, Stationary distribution and extinction of a stochastic staged progression AIDS model with staged treatment and second-order perturbation, Chaos Solitons Fractals, 140 (2020), 110238. https://doi.org/10.1016/j.chaos.2020.110238 doi: 10.1016/j.chaos.2020.110238
![]() |
[18] |
B. Zhou, B. Han, D. Jiang, Ergodic property, extinction and density function of a stochastic SIR epidemic model with nonlinear incidence and general stochastic perturbations, Chaos Solitons Fractals, 152 (2021), 111338. https://doi.org/10.1016/j.chaos.2021.111338 doi: 10.1016/j.chaos.2021.111338
![]() |
[19] |
Z. Shi, D. Jiang, N. Shi, A. Alsaedi, Virus infection model under nonlinear perturbation: Ergodic stationary distribution and extinction, J. Franklin. Inst., 359 (2022), 11039–11067. https://doi.org/10.1016/j.jfranklin.2022.03.035 doi: 10.1016/j.jfranklin.2022.03.035
![]() |
[20] |
S. Zhang, X. Meng, X. Wang, Application of stochastic inequalities to global analysis of a nonlinear stochastic SIRS epidemic model with saturated treatment function, Adv. Differ. Equations, 2018 (2018), 50. https://doi.org/10.1186/s13662-018-1508-z doi: 10.1186/s13662-018-1508-z
![]() |
[21] |
Z. Shi, X. Zhang, D. Jiang, Dynamics of an avian influenza model with half-saturated incidence, Appl. Math. Comput., 355 (2019), 399–416. https://doi.org/10.1016/j.amc.2019.02.070 doi: 10.1016/j.amc.2019.02.070
![]() |
[22] | R. Z. Khas'minskii, Stochastic Stability of Differential Equations, Sijthoff Noordhoff, Alphen ana den Rijn, Netherlands, 1980. https://doi.org/10.1007/978-94-009-9121-7 |
[23] |
D. Xu, Y. Huang, Z. Yang, Existence theorems for periodic Markov process and stochastic functional differential equations, Discrete Contin. Dyn. Syst., 24 (2009), 1005–1023. https://doi.org/10.3934/dcds.2009.24.1005 doi: 10.3934/dcds.2009.24.1005
![]() |
[24] |
J. Ge, W. Zuo, D. Jiang, Stationary distribution and density function analysis of a stochastic epidemic HBV model, Math. Comput. Simul., 191 (2022), 232–255. https://doi.org/10.1016/j.matcom.2021.08.003 doi: 10.1016/j.matcom.2021.08.003
![]() |
[25] |
Y. Shang, Lie algebraic discussion for affinity based information diffusion in social networks, Open Phys., 15 (2017), 705–711. https://doi.org/10.1515/phys-2017-0083 doi: 10.1515/phys-2017-0083
![]() |
[26] |
Y. Shang, Analytical solution for an in-host viral infection model with time-inhomogeneous rates, Acta Phys. Pol. B, 46 (2015), 1567–1577. https://doi.org/10.5506/aphyspolb.46.1567 doi: 10.5506/aphyspolb.46.1567
![]() |
[27] | C. W. Gardiner, Handbook of Stochastic Methods for Physics, Springer, 1983. |
1. | Mohammed A. El-Shorbagy, Anas Bouaouda, Hossam A. Nabwey, Laith Abualigah, Fatma A. Hashim, Bald eagle search algorithm: a comprehensive review with its variants and applications, 2024, 12, 2164-2583, 10.1080/21642583.2024.2385310 | |
2. | Chuan Li, Yanjie Zhu, A hybrid butterfly and Newton–Raphson swarm intelligence algorithm based on opposition-based learning, 2024, 27, 1386-7857, 14469, 10.1007/s10586-024-04678-y | |
3. | Santosh Kumar Chhotray, Debahuti Mishra, Sarada Prasanna Pati, Sashikala Mishra, An Optimized Cascaded CNN Approach for Feature Extraction From Brain MRIs for Tumor Classification, 2025, 13, 2169-3536, 32681, 10.1109/ACCESS.2025.3543214 | |
4. | Lian Lian, Network Traffic Prediction Based on Decomposition and Combination Model, 2025, 38, 1074-5351, 10.1002/dac.70056 |
Algorithms | Parameters | Values | Algorithms | Parameters | Values |
ACO | Pheromone factor α | 4 | ChOA | random number r1, r2 | [0, 1] |
Heuristic function factor β | 5 | Convergence factor f | [2.5, 0] | ||
Pheromone volatilization factor ρ | 0.2 | TLBO | Teaching factor TF | {1, 2} | |
GWO | Convergence factor a | [2, 0] | Learning step ri | [0, 1] | |
PSO | Inertia weight w | 1 | BES | Position change parameterα | 2 |
Cognitive coefficient c1 | 2 | Spiral parameter a, R | 10, 1.5 | ||
Social coefficient c2 | 2 | Enhancement coefficient c1, c2 | 2 | ||
Maximum velocity v | 6 | random number r | [0, 1] |
Actual search area | Meshing status | Number of waypoints | Start point | end point | |
Scenario 1 | 20 × 20× 2 (km) | 20 × 20× 2 | 20 | (20, 7) | (6, 5) |
Scenario 2 | 40 × 40× 2 (km) | 40 × 40× 2 | 40 | (40, 14) | (12, 10) |
Scenario 3 | 60 × 60× 2 (km) | 60 × 60× 2 | 60 | (60, 21) | (18, 15) |
Scenario 4 | 80 × 80× 2 (km) | 80 × 80× 2 | 80 | (80, 28) | (24, 20) |
Scenario 5 | 90 × 90× 2 (km) | 90 × 90× 2 | 90 | (90, 35) | (30, 25) |
PSO | GWO | ACO | ChOA | TLBO | BES | ||
Scenario 1 | Best | 98.09 | 102.56 | 100.93 | 104.72 | 84.34 | 76.67 |
Worst | 192.58 | 176.14 | 117.30 | 169.34 | 144.96 | 145.36 | |
Mean | 138.10 | 104.04 | 102.59 | 106.89 | 98.38 | 79.95 | |
Std | 25.38 | 3.56 | 2.51 | 5.52 | 8.80 | 8.07 | |
Scenario 2 | Best | 163.36 | 153.75 | 150.57 | 162.63 | 155.46 | 105.81 |
Worst | 304.71 | 259.47 | 158.89 | 230.32 | 261.42 | 217.77 | |
Mean | 202.77 | 154.87 | 151.83 | 161.07 | 174.50 | 111.25 | |
Std | 27.06 | 4.93 | 2.50 | 10.30 | 12.87 | 14.34 | |
Scenario 3 | Best | 179.71 | 203.68 | 199.36 | 215.94 | 202.28 | 154.89 |
Worst | 368.47 | 354.75 | 291.82 | 364.91 | 298.86 | 288.89 | |
Mean | 254.43 | 206.00 | 205.54 | 216.46 | 228.34 | 161.42 | |
Std | 44.15 | 7.62 | 5.92 | 12.54 | 21.73 | 15.22 | |
Scenario 4 | Best | 316.68 | 300.72 | 311.22 | 313.8 | 291.81 | 271.80 |
Worst | 592.86 | 549.12 | 357.00 | 530.88 | 467.82 | 459.24 | |
Mean | 411.69 | 307.98 | 313.02 | 322.71 | 332.70 | 276.06 | |
Std | 46.14 | 18.27 | 8.37 | 17.94 | 32.08 | 12.06 | |
Scenario 5 | Best | 416.56 | 404.84 | 445.96 | 482.76 | 428.44 | 368.24 |
Worst | 897.32 | 869.08 | 520.56 | 752.76 | 852.92 | 760.12 | |
Mean | 565.12 | 412.6 | 490.16 | 431.40 | 444.36 | 381.60 | |
Std | 94.72 | 21.92 | 6.09 | 35.44 | 30.60 | 41.64 |
Algorithms | Scenario 1 | Scenario 2 | Scenario 3 | Scenario 4 | Scenario 5 |
BES vs PSO | 3.76 × 10−5 | 5.57 × 10−11 | 5.03 × 10−4 | 0.0033 | 7.01 × 10−18 |
BES vs GWO | 1.10 × 10−6 | 3.72 × 10−15 | 6.18 × 10−4 | 1.00 × 10−17 | 8.87 × 10−32 |
BES vs ACO | 5.93 × 10−12 | 1.30 × 10−13 | 2.02 × 10−17 | 2.21 × 10−18 | 1.74 × 10−10 |
BES vs ChOA | 2.21 × 10−9 | 5.37 × 10−16 | 1.09 × 10−16 | 4.11 × 10−7 | 3.33 × 10−13 |
BES vs TLBO | 2.76 × 10−11 | 4.46 × 10−16 | 1.52 × 10−27 | 4.80 × 10−5 | 2.51 × 10−25 |
Algorithms | Scenario 1 | Scenario 2 | Scenario 3 | Scenario 4 | Scenario 5 | |||||
Mean score | Rank | Mean score | Rank | Mean score | Rank | Mean score | Rank | Mean score | Rank | |
PSO | 4.50 | 5 | 4.00 | 5 | 4.75 | 5 | 4.00 | 5 | 3.55 | 5 |
GWO | 4.00 | 4 | 3.50 | 3 | 3.75 | 4 | 3.50 | 3 | 2.75 | 2 |
ACO | 2.25 | 2 | 1.75 | 1 | 2.00 | 2 | 2.25 | 2 | 3.25 | 3 |
ChOA | 5.25 | 6 | 6.00 | 6 | 5.50 | 6 | 6.00 | 6 | 5.50 | 6 |
TLBO | 2.75 | 3 | 3.75 | 4 | 3.25 | 3 | 3.75 | 4 | 3.50 | 4 |
BES | 2.20 | 1 | 2.05 | 2 | 1.75 | 1 | 1.50 | 1 | 2.50 | 1 |
Algorithms | Parameters | Values | Algorithms | Parameters | Values |
ACO | Pheromone factor α | 4 | ChOA | random number r1, r2 | [0, 1] |
Heuristic function factor β | 5 | Convergence factor f | [2.5, 0] | ||
Pheromone volatilization factor ρ | 0.2 | TLBO | Teaching factor TF | {1, 2} | |
GWO | Convergence factor a | [2, 0] | Learning step ri | [0, 1] | |
PSO | Inertia weight w | 1 | BES | Position change parameterα | 2 |
Cognitive coefficient c1 | 2 | Spiral parameter a, R | 10, 1.5 | ||
Social coefficient c2 | 2 | Enhancement coefficient c1, c2 | 2 | ||
Maximum velocity v | 6 | random number r | [0, 1] |
Actual search area | Meshing status | Number of waypoints | Start point | end point | |
Scenario 1 | 20 × 20× 2 (km) | 20 × 20× 2 | 20 | (20, 7) | (6, 5) |
Scenario 2 | 40 × 40× 2 (km) | 40 × 40× 2 | 40 | (40, 14) | (12, 10) |
Scenario 3 | 60 × 60× 2 (km) | 60 × 60× 2 | 60 | (60, 21) | (18, 15) |
Scenario 4 | 80 × 80× 2 (km) | 80 × 80× 2 | 80 | (80, 28) | (24, 20) |
Scenario 5 | 90 × 90× 2 (km) | 90 × 90× 2 | 90 | (90, 35) | (30, 25) |
PSO | GWO | ACO | ChOA | TLBO | BES | ||
Scenario 1 | Best | 98.09 | 102.56 | 100.93 | 104.72 | 84.34 | 76.67 |
Worst | 192.58 | 176.14 | 117.30 | 169.34 | 144.96 | 145.36 | |
Mean | 138.10 | 104.04 | 102.59 | 106.89 | 98.38 | 79.95 | |
Std | 25.38 | 3.56 | 2.51 | 5.52 | 8.80 | 8.07 | |
Scenario 2 | Best | 163.36 | 153.75 | 150.57 | 162.63 | 155.46 | 105.81 |
Worst | 304.71 | 259.47 | 158.89 | 230.32 | 261.42 | 217.77 | |
Mean | 202.77 | 154.87 | 151.83 | 161.07 | 174.50 | 111.25 | |
Std | 27.06 | 4.93 | 2.50 | 10.30 | 12.87 | 14.34 | |
Scenario 3 | Best | 179.71 | 203.68 | 199.36 | 215.94 | 202.28 | 154.89 |
Worst | 368.47 | 354.75 | 291.82 | 364.91 | 298.86 | 288.89 | |
Mean | 254.43 | 206.00 | 205.54 | 216.46 | 228.34 | 161.42 | |
Std | 44.15 | 7.62 | 5.92 | 12.54 | 21.73 | 15.22 | |
Scenario 4 | Best | 316.68 | 300.72 | 311.22 | 313.8 | 291.81 | 271.80 |
Worst | 592.86 | 549.12 | 357.00 | 530.88 | 467.82 | 459.24 | |
Mean | 411.69 | 307.98 | 313.02 | 322.71 | 332.70 | 276.06 | |
Std | 46.14 | 18.27 | 8.37 | 17.94 | 32.08 | 12.06 | |
Scenario 5 | Best | 416.56 | 404.84 | 445.96 | 482.76 | 428.44 | 368.24 |
Worst | 897.32 | 869.08 | 520.56 | 752.76 | 852.92 | 760.12 | |
Mean | 565.12 | 412.6 | 490.16 | 431.40 | 444.36 | 381.60 | |
Std | 94.72 | 21.92 | 6.09 | 35.44 | 30.60 | 41.64 |
Algorithms | Scenario 1 | Scenario 2 | Scenario 3 | Scenario 4 | Scenario 5 |
BES vs PSO | 3.76 × 10−5 | 5.57 × 10−11 | 5.03 × 10−4 | 0.0033 | 7.01 × 10−18 |
BES vs GWO | 1.10 × 10−6 | 3.72 × 10−15 | 6.18 × 10−4 | 1.00 × 10−17 | 8.87 × 10−32 |
BES vs ACO | 5.93 × 10−12 | 1.30 × 10−13 | 2.02 × 10−17 | 2.21 × 10−18 | 1.74 × 10−10 |
BES vs ChOA | 2.21 × 10−9 | 5.37 × 10−16 | 1.09 × 10−16 | 4.11 × 10−7 | 3.33 × 10−13 |
BES vs TLBO | 2.76 × 10−11 | 4.46 × 10−16 | 1.52 × 10−27 | 4.80 × 10−5 | 2.51 × 10−25 |
Algorithms | Scenario 1 | Scenario 2 | Scenario 3 | Scenario 4 | Scenario 5 | |||||
Mean score | Rank | Mean score | Rank | Mean score | Rank | Mean score | Rank | Mean score | Rank | |
PSO | 4.50 | 5 | 4.00 | 5 | 4.75 | 5 | 4.00 | 5 | 3.55 | 5 |
GWO | 4.00 | 4 | 3.50 | 3 | 3.75 | 4 | 3.50 | 3 | 2.75 | 2 |
ACO | 2.25 | 2 | 1.75 | 1 | 2.00 | 2 | 2.25 | 2 | 3.25 | 3 |
ChOA | 5.25 | 6 | 6.00 | 6 | 5.50 | 6 | 6.00 | 6 | 5.50 | 6 |
TLBO | 2.75 | 3 | 3.75 | 4 | 3.25 | 3 | 3.75 | 4 | 3.50 | 4 |
BES | 2.20 | 1 | 2.05 | 2 | 1.75 | 1 | 1.50 | 1 | 2.50 | 1 |