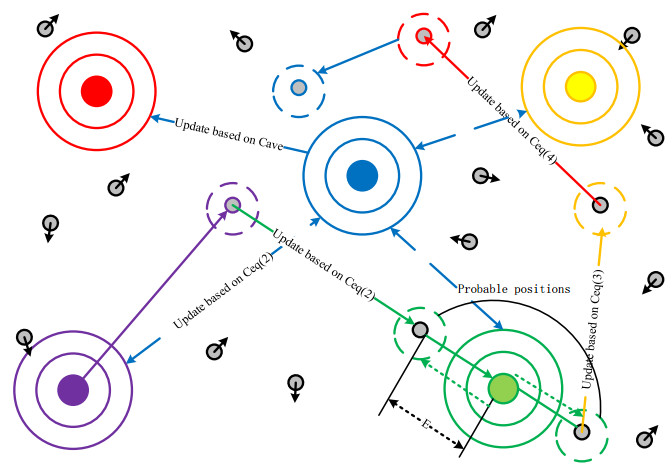
The pandemic has led to notable psychological challenges among healthcare professionals, including nurses.
Our aims of this study were to assess insomnia and nightmare distress levels in nurses and investigate their association with mental resilience.
Nurses participated in an online survey, which included the Nightmare Distress Questionnaire (NDQ), Brief Resilience Scale (BRS) and Athens Insomnia Scale (AIS). Demographic information, such as age, professional experience and gender, was also collected.
The study included 355 female and 78 male nurses. Findings revealed that 61.4% had abnormal AIS scores, 7% had abnormal NDQ scores and 25.4% had low BRS scores. Female nurses had higher AIS and NDQ scores but lower BRS scores compared to males. BRS demonstrated negative correlations with both AIS and NDQ. Multiple regression analysis indicated that NDQ accounted for 24% of the AIS variance, with an additional 6.5% explained by the BRS. BRS acted as a mediator, attenuating the impact of nightmares on insomnia, with gender moderating this relationship.
Nursing staff experienced heightened sleep disturbances during the pandemic, with nightmares and insomnia being prevalent. Nightmares significantly contributed to insomnia, but mental resilience played a vital role in mitigating this effect. Strategies are warranted to address the pandemic's psychological impact on nursing professionals.
Citation: Argyro Pachi, Athanasios Tselebis, Christos Sikaras, Eleni Paraskevi Sideri, Maria Ivanidou, Spyros Baras, Charalampos Milionis, Ioannis Ilias. Nightmare distress, insomnia and resilience of nursing staff in the post-pandemic era[J]. AIMS Public Health, 2024, 11(1): 36-57. doi: 10.3934/publichealth.2024003
[1] | Xiaoxu Peng, Heming Jia, Chunbo Lang . Modified dragonfly algorithm based multilevel thresholding method for color images segmentation. Mathematical Biosciences and Engineering, 2019, 16(6): 6467-6511. doi: 10.3934/mbe.2019324 |
[2] | Shikai Wang, Heming Jia, Xiaoxu Peng . Modified salp swarm algorithm based multilevel thresholding for color image segmentation. Mathematical Biosciences and Engineering, 2020, 17(1): 700-724. doi: 10.3934/mbe.2020036 |
[3] | Jiande Zhang, Chenrong Huang, Ying Huo, Zhan Shi, Tinghuai Ma . Multi-population cooperative evolution-based image segmentation algorithm for complex helical surface image. Mathematical Biosciences and Engineering, 2020, 17(6): 7544-7561. doi: 10.3934/mbe.2020385 |
[4] | Shenghan Li, Linlin Ye . Multi-level thresholding image segmentation for rubber tree secant using improved Otsu's method and snake optimizer. Mathematical Biosciences and Engineering, 2023, 20(6): 9645-9669. doi: 10.3934/mbe.2023423 |
[5] | Wenqi Ji, Xiaoguang He . Kapur's entropy for multilevel thresholding image segmentation based on moth-flame optimization. Mathematical Biosciences and Engineering, 2021, 18(6): 7110-7142. doi: 10.3934/mbe.2021353 |
[6] | Akansha Singh, Krishna Kant Singh, Michal Greguš, Ivan Izonin . CNGOD-An improved convolution neural network with grasshopper optimization for detection of COVID-19. Mathematical Biosciences and Engineering, 2022, 19(12): 12518-12531. doi: 10.3934/mbe.2022584 |
[7] | Mingzhu Li, Ping Li, Yao Liu . IDEFE algorithm: IDE algorithm optimizes the fuzzy entropy for the gland segmentation. Mathematical Biosciences and Engineering, 2023, 20(3): 4896-4911. doi: 10.3934/mbe.2023227 |
[8] | Nilkanth Mukund Deshpande, Shilpa Gite, Biswajeet Pradhan, Ketan Kotecha, Abdullah Alamri . Improved Otsu and Kapur approach for white blood cells segmentation based on LebTLBO optimization for the detection of Leukemia. Mathematical Biosciences and Engineering, 2022, 19(2): 1970-2001. doi: 10.3934/mbe.2022093 |
[9] | Yuting Liu, Hongwei Ding, Zongshan Wang, Gushen Jin, Bo Li, Zhijun Yang, Gaurav Dhiman . A chaos-based adaptive equilibrium optimizer algorithm for solving global optimization problems. Mathematical Biosciences and Engineering, 2023, 20(9): 17242-17271. doi: 10.3934/mbe.2023768 |
[10] | Yuan-Nan Young, Doron Levy . Registration-Based Morphing of Active Contours for Segmentation of CT Scans. Mathematical Biosciences and Engineering, 2005, 2(1): 79-96. doi: 10.3934/mbe.2005.2.79 |
The pandemic has led to notable psychological challenges among healthcare professionals, including nurses.
Our aims of this study were to assess insomnia and nightmare distress levels in nurses and investigate their association with mental resilience.
Nurses participated in an online survey, which included the Nightmare Distress Questionnaire (NDQ), Brief Resilience Scale (BRS) and Athens Insomnia Scale (AIS). Demographic information, such as age, professional experience and gender, was also collected.
The study included 355 female and 78 male nurses. Findings revealed that 61.4% had abnormal AIS scores, 7% had abnormal NDQ scores and 25.4% had low BRS scores. Female nurses had higher AIS and NDQ scores but lower BRS scores compared to males. BRS demonstrated negative correlations with both AIS and NDQ. Multiple regression analysis indicated that NDQ accounted for 24% of the AIS variance, with an additional 6.5% explained by the BRS. BRS acted as a mediator, attenuating the impact of nightmares on insomnia, with gender moderating this relationship.
Nursing staff experienced heightened sleep disturbances during the pandemic, with nightmares and insomnia being prevalent. Nightmares significantly contributed to insomnia, but mental resilience played a vital role in mitigating this effect. Strategies are warranted to address the pandemic's psychological impact on nursing professionals.
Image segmentation is the basic work of image processing research. There are primarily four types of segmentation methods: thresholding [1,2,3], boundary-based [4,5], region-based [6,7,8], and hybrid techniques [9,10,11,12]. Several algorithmic techniques such as Artificial Neural Network [13], Convolutional neural Network [14], and K-nearest Neighbors [15] can also be applied in image segmentation.
Among all the methods of image segmentation, Otsu [16] method is the most popular one. Computational complexity of Otsu methods increases exponentially with the increasing number of thresholds due to exhaustive search. It is a difficult task to study how to improve the segmentation accuracy of Otsu with multi-thresholds method. The expansion of the actual Otsu's thresholding to multilevel thresholding is known as multi-Otsu thresholding [17]. Nevertheless, these methods are unable to obtain effective results for noisy images. To solve this problem, J. Zhuang [18] proposed a 2-D Otsu method that selects the optimal threshold on a 2-D histogram. Jing [19] proposed the maximum between-cluster variance by using a 3-D histogram approach and was named the 3-D Otsu method. In this method, the 3D histogram takes the median value of neighborhood pixels as the third feature of the existing features of the 2D OTSU method, that is, gray information and neighborhood mean value. L. Wang [20] derived a group of recurrence formula for 3-D Otsu's method and eliminated redundancy in the formula by introducing a look-up table. The method reduced the computation of the 3DOtsu. Therefore, in order to optimize the search process, a faster and automatic optimal threshold selection method is needed.
The traditional exhaustive methods take the large amount of computation. In this case, meta-heuristic methods have attracted much attention in recent years. L. Bian proposed a new multi-threshold MRI image segmentation algorithm based on mixed entropy using Curvelet transformation and Multi-Objective Particle Swarm Optimization [21]. This method could deal with the difficulties caused by noise disturbance, intensity inhomogeneity and edge blurring in Magnetic Resonance Imaging image segmentation. N. Muangkote proposed the nature-inspired meta-heuristic named multilevel thresholding moth-flame optimization algorithm (MTMFO) for multilevel thresholding [22]. This algorithm effectively solved the problem of satellite image segmentation. B. Surina proposed a multi-level thresholding model based on gray-level & local-average histogram (GLLA) and Tsallis–Havrda–Charvát entropy for RGB color image [23]. This method had the effectiveness and reasonability. A. Wunnava proposed an adaptive Harris Hawks optimization (AHHO) technique to solve the multi-level image segmentation [24]. The experimental results were beneficial to the segmentation field of image processing. The optimization algorithm is adopted to solve the threshold selection of the multi-threshold image segmentation method, which effectively improves the segmentation precision of the image segmentation method [25,26].
The optimization algorithm can solve practical engineering problems in recent years [27]. Different optimization algorithms adapt to different engineering problems and have different optimization capabilities [28,29]. These nature-inspired optimization algorithms were mainly classified into two classes recently which are evolutionary algorithm (EA) and biology-inspired or bio-inspired algorithms. EA imitated the Darwinian theory of evolution [30]. There were many good algorithms in this class. In 1975, GA was invented by John Holland [31], it used the binary representation of individuals. In 1997, Differential evolution was proposed Rainer stone, the essence was a multi - objective optimization algorithm [32]. The most popular class was the biology-inspired or bio-inspired algorithms right now. One of the most famous algorithms was the Particle Swarm Optimizer (PSO) which was developed based on the swarming behavior of fish and birds [33]. In 2015, the ant lion optimizer was proposed by Mirjalili [34]. In 2016, Askarzadeh proposed the crow search algorithm [35]. In 2017, the killer whale algorithm was proposed by Biyanto [36]. These algorithms were inspired from the predation behavior animal, so as to obtain better searching ability. There also some algorithms inspired from the physics and chemistry, these algorithms usually had simple mathematical models, but had good optimization effect. In 2001, the harmony search algorithm was proposed by Geem [37]. In 2015, Zheng Yu-Jun proposed water wave optimization algorithm [38]. In 2019, A. Faramarzi proposed a novel optimization algorithm, called Equilibrium optimizer (EO), which controlled volume mass balance models used to estimate both dynamic and equilibrium states [39]. No-Free-Lunch [40] proved that no algorithm can solve all optimization problems.
There is no perfect optimization algorithm and the optimization algorithm should be improved to better solve engineering problems. Many scholars study the hybrid optimization algorithm [41]. Pankaj U. proposed a new multistage hybrid optimization algorithm to optimize multilevel threshold [42]. The method had a good performance in test images. Amandeep proposed a fast SAR image segmentation method based on Particle Swarm Optimization-Gravitational Search Algorithm [43]. The method had good segmentation accuracy. D. Kole proposed a new approach to automatic unsupervised efficient image segmentation algorithm using hybrid technique based on Particle Swarm Optimization and Genetic Algorithm [44]. Gao H. presented a learning strategy-based particle swarm optimization algorithm with an exchange method [45]. The experiment shows that the method had a good result using the Berkeley images. So, the hybrid optimization algorithm could use the advantage of the different algorithms to enhance the search ability of the original algorithm.
This paper focuses on the segmentation of wood fiber images. The wood fiber images have the small target, it takes huge challenge for the segmentation method. We use 3DOtsu as a fitness function to segment wood fiber images. The threshold image method can overcome the difficulty of wood fiber image difference channel. The Equilibrium optimizer algorithm can find the threshold of the wood fiber images, however the segmentation accuracy is low and the CPU time is large. In order to improve the optimal ability of EOA, we use the GOA improve the EOA. The HEOA obtains the strong ability find the optimal threshold from the wood fiber images.
In this paper, the HEOA is proposed. The main contribution of this study is that the GOA improves the original EOA for multilevel threshold. Experiments are performed on CEC2015 data, classic images and wood fiber images. The proposed algorithm is compared with the original EOA and other six algorithms include CSA, FPA, PSO, HSOA and HWOA. The GOA algorithms can balance the exploration and exploitation of the EOA. The results show the superiority of the proposed algorithm in terms of the objective function value, image quality measures on both normal and high-level thresholding.
Otsu algorithm is a classical image segmentation method, and its segmentation results are excellent. This method can be extended to multi-threshold Otsu to obtain the maximum variance between two classes, so as to obtain the optimal threshold of the image [46].
Assuming that there are K thresholds, which divide the image into K+1 classes. The extended between-class variance is calculated by
f(t)=K∑i=0σi | (1) |
The sigma terms are determined by Eq. (6) and the mean levels are calculated by Eq. (7):
σ0=ϖ0(μ0−μT)2,σ1=ϖ1(μ1−μT)2,⋯,σK−1=ϖK−1(μK−1−μT)2 | (2) |
μ0=t∑i=1ipi/ϖ0,μ1=t2∑i=t1+1ipi/ϖ1,⋯,μK−1=L∑i=tM−1+1ipi/ϖK−1 | (3) |
The optimum thresholds are found by maximizing the between-class variance by Eq. (8):
t∗=argmax(K−1∑i=0σi) | (4) |
3D-Otsu, the histogram is constructed by taking gray values of pixels along with their spatial information including the neighborhood mean and median [47]. The Otsu only segment the single channel of the image, however the color images have three channels. There are more information in the three channels, so the 3DOtus can use the information of three channels and get the best threshold form the color images. An image I with K gray levels and N number of pixels is considered, where the intensity value of pixel at the location (x,y) denoted by f(x,y). The mean and median value of l×l neighborhood of pixel is denoted by g(x,y) and h(x,y), which is defined by Eq. (9) and Eq. (10).
g(x,y)=1l2l−12∑i=−l−12l−12∑j=−l−12f(x+i,y+j) | (5) |
h(x,y)=med{f(x+i,y+j);i,j=−l−12,...,l−12} | (6) |
Where, the value of l is taken as 3 in this paper. For every pixel in the image I, mean and median values are calculated in the l×l neighborhood.
Let (tf,tg,th) as the optimal threshold of the three histograms, and use the Eq. (8) to calculate the optimal threshold from the three histograms. The optimal threshold is expressed as:
t∗f=argmax{K−1∑i=0σi(tf)} | (7) |
t∗g=argmax{K−1∑i=0σi(tg)} | (8) |
t∗h=argmax{K−1∑i=0σi(th)} | (9) |
The final optimum thresholds are average of the result of t∗f, t∗g and t∗h, and can be defined by Eq.10:
t∗=(t∗f+t∗g+t∗h)/3 | (10) |
The 3DOtsu image segmentation method uses the information of three channels of color images, the optimal threshold can segment the image exactly.
The EO method is inspired by the simple well-mixed dynamic mass balance on the control body, in which the mass balance equation is used to describe the coordination of non-active components in the control body as a function of its various source and aggregation mechanisms. Similar to most meta-heuristic algorithms, EO uses the initial population to start the optimization process. The equilibrium candidates' collaboration can be seen from Figure1. The initial concentrations are constructed based on the number of particles and dimensions with uniform random initialization in the search space as follows:
Cinitiali=Cmin+rand×(Cmax−Cmin) | (11) |
Where, Cinitiali is the initial concentration vector of the ith particle, Cmax and Cmin denote the minimum and maximum values for the dimensions, rand is a random vector in interval of [0, 1].
The equilibrium state is the final convergence state of the algorithm, which is desired to be the global optimum. At the beginning of the optimization process, there is no knowledge about the equilibrium state and only equilibrium candidates are determined to provide a search pattern for the particles. According to the different experiments under different types of case problems, these candidate particles are the four optimal particles determined in the whole optimization process plus another particle. These particles are nominated as equilibrium candidates and are used to construct a vector called the equilibrium pool:
C→eq,pool={C→eq(1),C→eq(2),C→eq(3),C→eq(4),C→eq(ave)} | (12) |
Where, C→eq(1) is the first particle updates all of its concentrations, C→eq(ave)is the average of the particle.
The main concentration updating rule is the exponential term (F). In order to guarantee convergence by slowing down the search speed along with improving the exploration and exploitation ability of the algorithm, the version can be seen as:
→F=a1×sign(r−0.5)×(e−λ×t−1) | (13) |
Where:
t=(1−IterMax_iter)(a2IterMax_iter) | (14) |
Where, Iter and Max_iter present the current and the maximum number of iterations.
The generation rate is one of the most important terms in the proposed algorithm to provide the exact solution by improving the exploitation phase. The final set of generation rate equation is as follows:
G→=G→0e−λ×(t−t0)=G→0−F→ | (15) |
Where:
→G0=→GCP(→Ceq−λ→C) | (16) |
→GCP={0.5r1r2≥GP0r2<GP | (17) |
Where, r1 and r2 are random numbers in [0, 1] and GCP vector is constructed by the repetition of the same value resulted from Eq. (16).
Finally, the updating rule of EO will be as follows:
C→=C→eq+(C→−C→eq)×F→+G→λV(1−F→) | (18) |
Where, F is defined in Eq. (13), and V is considered as unit.
In 2017, Mirjalili Seyedali proposed the grasshopper optimization algorithm [48]. The grasshoppers are a genus of straight fins of insect, they are seen as pests, because they are in crops for food, to cause damage to agriculture. The growth cycle of grasshoppers is shown in Figure 2. The grasshoppers usually exist alone in nature, but they are one of the biggest swarm of all species. The grasshoppers are unique in that they crowd behavior in adults and larvae of between. Millions of larva foraging on the basis of jumping, they feed on almost all plants, and when they reach adulthood, they form a large group in the air, making long migrations, looking for the next food source.
In larvae stage, the main characteristic of grasshopper is moving slowly, small scale food. When they become adult, collective action has became the main activity characteristics of grasshopper. The natureinspired algorithms logically divide the search process into two tendencies: exploration and exploitation. So mathematical model of the gregarious grasshoppers is represented as follows:
Xi=Si+Gi+Ai | (19) |
where Xi defines the position of the i-th grasshopper, Si is the social interaction, Gi is the gravity force on the i-th grasshopper, and Ai shows the wind advection.
{Si=N∑j=1s(dij)→dijs(r)=fe−rl−e−r | (20) |
where dij is the distance between the i-th and the j-th grasshopper, calculated as dij=|xj−xi|, s is a function to define the strength of social forces, →dij is an unit vector from the i-th grasshopper to the j-th grasshopper.
Gi=−g→eg | (21) |
where g is the gravitational constant and →eg shows an unity vector towards the center of earth.
Ai=u→ew | (22) |
Where u is a constant drift and →ew is a unity vector in the direction of wind.
Substituting S, G, and A into Eq. (19), then this equation can be expanded as follows:
Xi=N∑j=1s(|xj−xi|)xj−xidij−g→eg+u→ew | (23) |
However, this mathematical model cannot be used directly to solve optimization problems, mainly because the grasshoppers quickly reach the comfort zone and the swarm does not converge to a specified point. A modified version of this equation is proposed as follows to solve optimization problems:
Xdi=c1(N∑j=1c2ubd−lbd2s(|xj−xi|)xj−xidij)+→Td | (24) |
Among them, ubd and lbd are a type of upper and lower limitation, →Td is the optimal value after each iteration, c1=c2=cmax−lcmax−cminL, c1 balances the global search and local search for the target area, c2 balances the relationship among the attraction between two grasshopper, cmax and cmin can set the maximum and minimum search ability, l represents the current iteration number, L is the largest number of iterations.
In this subsection, we describe the hybrid equilibrium optimizer algorithm in detail. The EOA fall into the local optimal easily. The algorithm cannot balance the exploitation and exploration. In order to solve this problem, we use the advantage of the GOA to improve the optimization ability of the GOA. We use the Eq. (19) to enhance the individual ability of the EOA. The Xi have the strong ability to avoid the EOA drop into the local optimal. The formula can be seen below:
→C=→Ceq+(→C−→Ceq)×→F+→GλV(1−→F)×Xi | (25) |
A comprehensive algorithm step of HEOA based multilevel color image segmentation is given in Algorithm 1.
Algorithm 1 Pseudo-code of HEOA algorithm |
Input: The color image Output: Segmentation color image Read the input and compute the histogram of three channels of color imageInitialize the parameters r1andr2 Initialize the random population Ci while L < Max_iter do Calculate the fitness values of EOA for (each hawk Ci) do Calculate Ceq, Xi Update the C using Eq. (25) Calculate the fitness function using Eq.(10) end for UpdateCbestif there is a better solution L=L+1 end while Get the best solution as the multilevel threshold K According the K segment the three channels of the images Get the segmentation images |
In order to use the information of the three channels of color images, we use the fitness functions Eq. (10) to calculate the histogram of the three channels. And then, we use the HEOA to optimize the fitness function. Finally, we can get the best threshold and segment the color images. The flowchart of the BMPA can be seen from the Figure 3.
The computational complexity of the proposed method depends on the number of each combination (L), the number of generations (g), the number of population (n) and the parameters dimensions (d). So, computational complexity on L combination isO(L). The computational complexity of population location update is O(n*d). The calculation of fitness function values of all seagull populations isO(n∗L). Therefore, the final computational complexity of the proposed method is:
O(MBE)≈O(g∗(n∗d+n∗L)) | (26) |
To verify the optimization performance of HEOA, its performance is compared with five other optimization methods including CSA, FPA, PSO, BA and basic EOA. The optimization algorithm compared in this section tests CEC2015 benchmark test functions. The detailed description of CEC 2015 benchmark test functions [49] is presented in Table 2. For fair comparison, the other parameters of all algorithms are set according to their original papers. The results obtained by the algorithms on benchmark functions are presented in Table 3. All parameters of the comparison optimization algorithm are shown in table 1. Figure 4 show the result of the CEC2015. The population size is 30 and the number of iterations is 100. The proposed DLNN are testing in Matlab 2018b.
Algorithm | Parameters | Value |
EOA | c1 | 2 |
c2 | 2 | |
CSA [50] | AP | 0.5 |
FPA [51] | P | 0.5 |
PSO [52] | Swam size Cognitive, social acceleration Inertial weight | 2002, 20.95–0.4 |
BA [53] | β | (0, 1) |
HEOA | Levy | 1.5 |
No. | Functions | Related basic functions | Dim | fmin |
CEC-1 | Rotated Bent Cigar Function | Bent Cigar Function | 30 | 100 |
CEC-2 | Rotated Discus Function | Discus Function | 30 | 200 |
CEC-3 | Shifted and Rotated Weierstrass Function | Weierstrass Function | 30 | 300 |
CEC-4 | Shifted and Rotated Schwefel's Function | Schwefel's Function | 30 | 400 |
CEC-5 | Shifted and Rotated Katsuura Function | Katsuura Function | 30 | 500 |
CEC-6 | Shifted and Rotated HappyCat Function | HappyCat Function | 30 | 600 |
CEC-7 | Shifted and Rotated HGBat Function | HGBat Function | 30 | 700 |
CEC-8 | Shifted and Rotated Expanded Griewank's plus Rosenbrock's Function | Griewank's Function Rosenbrock's Function | 30 | 800 |
CEC-9 | Shifted and Rotated Expanded Scaer's F6 Function | Expanded Scaer's F6 Function | 30 | 900 |
CEC-10 | Hybrid Function 1 (N = 3) | Schwefel's FunctionRastrigin's FunctionHigh Conditioned Elliptic Function | 30 | 1000 |
CEC-11 | Hybrid Function 2 (N = 4) | Griewank's Function Weierstrass Function Rosenbrock's Function Scaer's F6 Function | 30 | 1100 |
CEC-12 | Hybrid Function 3 (N = 5) | Katsuura FunctionHappyCat FunctionExpanded Griewank's plus Rosenbrock's Function Schwefel's FunctionAckley's Function | 30 | 1200 |
CEC-13 | Composition Function 1 (N = 5) | Rosenbrock's FunctionHigh Conditioned Elliptic FunctionBent Cigar FunctionDiscus FunctionHigh Conditioned Elliptic Function | 30 | 1300 |
CEC-14 | Composition Function 2 (N = 3) | Schwefel's FunctionRastrigin's FunctionHigh Conditioned Elliptic Function | 30 | 1400 |
CEC-15 | Composition Function 3 (N = 5) | HGBat FunctionRastrigin's FunctionSchwefel's FunctionWeierstrass FunctionHigh Conditioned Elliptic Function | 30 | 1500 |
Func. | HEOA | CSA | PSO | FPA | BA | EOA | ||||||
Mean | Std. | Mean | Std. | Mean | Std. | Mean | Std. | Mean | Std. | Mean | Std. | |
CEC-1 | 1.05E+05 | 1.54E+07 | 4.21E+05 | 1.47E+08 | 2.17E+09 | 3.53E+07 | 1.76E+08 | 7.02E+07 | 4.76E+08 | 2.86E+08 | 1.07E+05 | 3.69E+07 |
CEC-2 | 6.70E+06 | 1.70E+09 | 2.06E+04 | 5.26E+09 | 7.01E+10 | 3.53E+09 | 3.04E+10 | 4.70E+09 | 3.36E+10 | 6.82E+09 | 6.70E+07 | 4.05E+09 |
CEC-3 | 3.20E+02 | 7.12E-02 | 3.20E+02 | 1.51E-01 | 3.22E+02 | 9.57E-02 | 3.21E+02 | 1.43E-01 | 3.21E+02 | 2.10E-01 | 3.55E+02 | 1.47E-01 |
CEC-4 | 4.10E+02 | 1.75E+00 | 4.05E+02 | 9.03E+00 | 5.46E+02 | 1.29E+01 | 5.30E+02 | 1.61E+01 | 5.21E+02 | 9.70E+00 | 4.50E+02 | 2.39E+01 |
CEC-5 | 9.81E+02 | 1.50E+02 | 1.22E+03 | 3.30E+02 | 4.74E+03 | 2.40E+02 | 3.80E+03 | 3.97E+02 | 3.65E+03 | 3.52E+02 | 9.91E+02 | 2.97E+02 |
CEC-6 | 2.05E+03 | 4.75E+06 | 2.14E+03 | 2.33E+07 | 3.79E+09 | 9.03E+06 | 4.52E+09 | 1.14E+07 | 1.28E+08 | 2.71E+07 | 2.10E+03 | 1.37E+07 |
CEC-7 | 7.02E+02 | 1.24E+01 | 7.03E+02 | 4.37E+01 | 1.81E+03 | 1.40E+01 | 1.78E+03 | 2.70E+01 | 1.78E+03 | 4.99E+01 | 8.82E+02 | 2.49E+01 |
CEC-8 | 1.47E+03 | 1.03E+06 | 1.41E+04 | 3.25E+06 | 2.21E+09 | 1.05E+06 | 1.34E+09 | 1.63E+06 | 1.34E+09 | 3.78E+06 | 1.47E+04 | 1.08E+06 |
CEC-9 | 1.00E+03 | 7.30E+00 | 1.00E+03 | 4.77E+01 | 1.33E+03 | 2.45E+01 | 1.33E+03 | 3.46E+01 | 1.62E+03 | 5.15E+01 | 1.00E+04 | 3.38E+01 |
CEC-10 | 1.23E+03 | 4.82E+04 | 2.05E+03 | 3.09E+06 | 2.58E+09 | 4.14E+05 | 2.97E+07 | 8.13E+05 | 4.41E+08 | 4.49E+06 | 1.36E+04 | 7.89E+05 |
CEC-11 | 1.35E+03 | 4.52E+01 | 1.40E+03 | 7.94E+01 | 2.00E+03 | 6.39E+01 | 1.77E+03 | 1.02E+02 | 1.77E+03 | 9.80E+01 | 1.32E+04 | 1.24E+02 |
CEC-12 | 1.30E+03 | 1.03E+01 | 1.30E+03 | 1.75E+01 | 1.76E+03 | 1.22E+01 | 1.49E+03 | 1.28E+01 | 1.51E+03 | 3.25E+01 | 1.34E+05 | 1.58E+01 |
CEC-13 | 1.30E+03 | 8.67E+00 | 1.34E+03 | 1.49E+00 | 5.44E+05 | 1.86E+01 | 1.54E+03 | 3.03E+01 | 1.56E+03 | 2.48E+00 | 1.55E+05 | 3.24E+01 |
CEC-14 | 3.22E+03 | 2.32E+03 | 8.88E+03 | 4.24E+03 | 2.23E+04 | 2.92E+03 | 2.23E+04 | 4.76E+03 | 2.23E+04 | 5.46E+03 | 3.22E+04 | 3.59E+03 |
CEC-15 | 1.60E+03 | 3.36E+02 | 1.60E+03 | 6.49E+02 | 1.28E+04 | 7.10E+02 | 9.01E+03 | 1.34E+03 | 4.09E+03 | 1.05E+03 | 1.80E+03 | 1.27E+03 |
As can be seen from table 3, the results of HEOA algorithm in processing all standard functions are better than those of other comparison algorithms, indicating that the HEOA algorithm can not only solve single-dimensional mathematical functions but also deal with multi-dimensional mathematical functions effectively. It shows that the optimization ability of HEOA algorithm is superior to other comparison algorithms. It can be seen from the Figure 4, the EOA and BA obtains the worst result and the HEOA gets the best result. Above the analysis, HEOA obtains the strong optimal ability, and in the next section we use the HEOA optimize the 3DOtsu.
In this section, HEOA algorithm is applied to optimize the 3DOtsu. In order to better verify the image segmentation ability of proposed algorithm, it is compared with the optimized 3DOtsu algorithm of CSA, PSO, FPA and BA. The color image has three color channels. In this paper, the images of the three channels are segmented, and then the three resulting images are fused to obtain the final segmentation result graph. Firstly, the segmentation effect and precision of HEOA algorithm are analyzed when the threshold value is increased. Then the segmentation ability, statistical analysis and stability analysis of the proposed HEOA algorithm and other optimization algorithms in 3DOtsu image segmentation are analyzed. All parameters of the comparison optimization algorithm are shown in table 3. The test images and the histogram of three channels of color images are as follows Figure 5. The test images included color natural images and satellite images. It can be seen from the histogram, the histogram of three channels has significant different and it take huge challenge for optimization algorithm to find the optimal threshold. Color image segmentation requires a higher threshold level, so it is more complex to use optimization technology to solve the problem. Therefore, the optimization algorithm has the characteristics of randomness.
In order to better observe the performance of the algorithm, we select K = 4, 8, 12 and 15. The evaluation index can better observe the performance of the algorithm. In order to comprehensively analyze the performance of the algorithm, we calculate the CPU time, Uniformity (U), Peak Signal-to-Noise Ratio (PSNR) and Feature Similarity Index (FSIM). The evaluation index of many object optimization methods can be seen table 4.
No. | Measures | Formulation | Reference |
1 | Uniformity measure | U=1−2×(k−1)×∑dj=1∑i∈Rj(fi−μj)2N×(fmax−fmin)2 | [54] |
2 | Peak Signal-to-Noise Ratio (PSNR) | PSNR=10×log10(2552MSE) MSE=1mnm−1∑i=1n−1∑j=1[I(i,j)−K(i,j)]2 |
[55] |
3 | Feature Similarity Index (FSIM) | FSIM=∑x=ηSL(x)⋅PCm(x)∑x=ηPCm(x) | [56] |
In this section, we compare the different segmentation method as fitness function. The EOA and HEOA optimize Otsu and 3DOtsu function. The FSIM of the compared algorithms can be seen from table 5. The Bar chart of the FSIM can be seen in Figure 6.
Image | K | EOA-Otsu | EOA-3DOtsu | HEOA-Otsu | HEOA-3DOtsu |
Test1 | 4 | 0.8628 | 0.8886 | 0.8973 | 0.9106 |
8 | 0.8657 | 0.8864 | 0.8957 | 0.9170 | |
12 | 0.9103 | 0.9332 | 0.9411 | 0.9580 | |
15 | 0.9293 | 0.9479 | 0.9565 | 0.9695 | |
Test2 | 4 | 0.8644 | 0.8799 | 0.8901 | 0.9015 |
8 | 0.8459 | 0.8674 | 0.8736 | 0.9135 | |
12 | 0.9298 | 0.9511 | 0.9569 | 0.9591 | |
15 | 0.9287 | 0.9474 | 0.9562 | 0.9671 | |
Test3 | 4 | 0.8586 | 0.8833 | 0.8919 | 0.9056 |
8 | 0.8599 | 0.8766 | 0.8859 | 0.9246 | |
12 | 0.9207 | 0.9370 | 0.9466 | 0.9661 | |
15 | 0.9049 | 0.9239 | 0.9358 | 0.9688 | |
Test4 | 4 | 0.8518 | 0.8660 | 0.8805 | 0.8963 |
8 | 0.8856 | 0.9060 | 0.9125 | 0.9221 | |
12 | 0.8823 | 0.9034 | 0.9143 | 0.9619 | |
15 | 0.9012 | 0.9260 | 0.9343 | 0.9742 | |
Test5 | 4 | 0.8379 | 0.8545 | 0.8645 | 0.8988 |
8 | 0.8648 | 0.8854 | 0.8992 | 0.9193 | |
12 | 0.9166 | 0.9305 | 0.9446 | 0.9659 | |
15 | 0.9055 | 0.9286 | 0.9345 | 0.9750 | |
Test6 | 4 | 0.8725 | 0.8902 | 0.9025 | 0.9157 |
8 | 0.8854 | 0.9040 | 0.9160 | 0.9189 | |
12 | 0.9267 | 0.9484 | 0.9566 | 0.9585 | |
15 | 0.8904 | 0.9095 | 0.9214 | 0.9663 | |
Test7 | 4 | 0.8708 | 0.8883 | 0.9009 | 0.9147 |
8 | 0.8845 | 0.9037 | 0.9155 | 0.9171 | |
12 | 0.9250 | 0.9478 | 0.9555 | 0.9581 | |
15 | 0.8898 | 0.9087 | 0.9202 | 0.9648 | |
Test8 | 4 | 0.8704 | 0.8871 | 0.9000 | 0.9143 |
8 | 0.8830 | 0.9017 | 0.9142 | 0.9155 | |
12 | 0.9232 | 0.9469 | 0.9535 | 0.9578 | |
15 | 0.8886 | 0.9079 | 0.9201 | 0.9629 | |
Test9 | 4 | 0.8716 | 0.8884 | 0.9011 | 0.9151 |
8 | 0.8844 | 0.9026 | 0.9157 | 0.9163 | |
12 | 0.9237 | 0.9470 | 0.9552 | 0.9596 | |
15 | 0.8897 | 0.9082 | 0.9217 | 0.9633 | |
Test10 | 4 | 0.8720 | 0.8896 | 0.9024 | 0.9171 |
8 | 0.8863 | 0.9027 | 0.9162 | 0.9179 | |
12 | 0.9247 | 0.9482 | 0.9561 | 0.9607 | |
15 | 0.8915 | 0.9089 | 0.9223 | 0.9645 |
It can be seen from table 5, the FSIM value of 3DOtsu is better than Otsu. The 3DOtsu can use the information of color image, and get the best segment result. And the result of HEOA is better than EOA, it means that the Hybrid algorithm improves the optimal ability of EOA and makes the algorithm find the best result. Above analyze, we use the 3DOtsu as the fitness function in the next experiment.
The Figure 6 show the FSIM result of the compared algorithms. The result of 3DOtsu is better than the result of Otsu. The 3DOtsu use the information of three channels and obtains the better threshold than Otsu. At the same time, the result of HEOA is better than EOA, it means that the GOA can improve the optimal ability of the EOA.
In this experiment, to show the merits of proposed technique, the results are compared with CSA, FPA, PSO, BA, HSOA [57] and HWOA [58] using 3DOtsu objective function. The parameters of the compared algorithms are set in the references.
The Figure 7 show the curve of Uniformity. It can be seen from figure, the HEOA obtains the best result in compared algorithms, the HSOA and HWOA gets the better result in compared algorithms, the CSA, FPA, PSO and BA obtains the worst result in all cases. It can be kwon that the hybrid optimization algorithms have the better optimal ability than original optimization algorithm. Most of all, the HEOA has the strongest ability in the compared algorithms.
From Table 6, it can be observed that for all the test images, HEOA is better and more reliable than CSA, FPA, PSO, and BA, because of its precise search capability, at a high threshold level (K). Performance of HSOA and HWOA has closely followed HEOA. The solution update strategy for FPA and PSO may have led to poor results. The comprehensive performance ranking of the comparison algorithm is as follows: HEOA > HSOA > HWOA > PSO > BA > CSA > FPA. So, the HEOA have a better performance than other algorithms.
Image | K | HEOA | HSOA | HWOA | CSA | FPA | PSO | BA |
Test1 | 4 | 0.9571 | 0.9415 | 0.9437 | 0.9245 | 0.9284 | 0.9262 | 0.9243 |
8 | 0.9674 | 0.9525 | 0.9594 | 0.9299 | 0.9309 | 0.9392 | 0.9373 | |
12 | 0.9744 | 0.9641 | 0.9654 | 0.9404 | 0.9456 | 0.9424 | 0.9457 | |
15 | 0.9795 | 0.9684 | 0.9617 | 0.9428 | 0.9441 | 0.9445 | 0.9458 | |
Test2 | 4 | 0.9544 | 0.9460 | 0.9460 | 0.9224 | 0.9167 | 0.9207 | 0.9216 |
8 | 0.9722 | 0.9583 | 0.9624 | 0.9442 | 0.9422 | 0.9425 | 0.9356 | |
12 | 0.9813 | 0.9717 | 0.9639 | 0.9437 | 0.9458 | 0.9526 | 0.9497 | |
15 | 0.9824 | 0.9698 | 0.9697 | 0.9499 | 0.9479 | 0.9468 | 0.9515 | |
Test3 | 4 | 0.9528 | 0.9437 | 0.9427 | 0.9244 | 0.9184 | 0.9184 | 0.9165 |
8 | 0.9626 | 0.9500 | 0.9482 | 0.9255 | 0.9274 | 0.9323 | 0.9290 | |
12 | 0.9782 | 0.9657 | 0.9625 | 0.9464 | 0.9453 | 0.9486 | 0.9406 | |
15 | 0.9812 | 0.9701 | 0.9683 | 0.9467 | 0.9450 | 0.9487 | 0.9513 | |
Test4 | 4 | 0.9518 | 0.9403 | 0.9435 | 0.9201 | 0.9171 | 0.9161 | 0.9187 |
8 | 0.9699 | 0.9589 | 0.9520 | 0.9327 | 0.9350 | 0.9331 | 0.9402 | |
12 | 0.9749 | 0.9623 | 0.9653 | 0.9385 | 0.9398 | 0.9409 | 0.9439 | |
15 | 0.9806 | 0.9717 | 0.9700 | 0.9486 | 0.9490 | 0.9457 | 0.9485 | |
Test5 | 4 | 0.9541 | 0.9410 | 0.9402 | 0.9257 | 0.9167 | 0.9206 | 0.9207 |
8 | 0.9679 | 0.9558 | 0.9560 | 0.9385 | 0.9306 | 0.9399 | 0.9392 | |
12 | 0.9769 | 0.9670 | 0.9618 | 0.9393 | 0.9441 | 0.9404 | 0.9455 | |
15 | 0.9808 | 0.9639 | 0.9678 | 0.9468 | 0.9508 | 0.9519 | 0.9467 | |
Test6 | 4 | 0.9507 | 0.9393 | 0.9373 | 0.9169 | 0.9197 | 0.9138 | 0.9154 |
8 | 0.9666 | 0.9504 | 0.9512 | 0.9383 | 0.9298 | 0.9355 | 0.9352 | |
12 | 0.9787 | 0.9644 | 0.9673 | 0.9492 | 0.9505 | 0.9474 | 0.9431 | |
15 | 0.9824 | 0.9713 | 0.9667 | 0.9470 | 0.9480 | 0.9498 | 0.9472 | |
Test7 | 4 | 0.9514 | 0.9397 | 0.9378 | 0.9183 | 0.9212 | 0.9150 | 0.9167 |
8 | 0.9667 | 0.9524 | 0.9529 | 0.9392 | 0.9300 | 0.9360 | 0.9366 | |
12 | 0.9802 | 0.9648 | 0.9675 | 0.9510 | 0.9521 | 0.9479 | 0.9433 | |
15 | 0.9841 | 0.9725 | 0.9680 | 0.9473 | 0.9483 | 0.9502 | 0.9487 | |
Test8 | 4 | 0.9508 | 0.9377 | 0.9365 | 0.9165 | 0.9196 | 0.9137 | 0.9161 |
8 | 0.9649 | 0.9522 | 0.9520 | 0.9383 | 0.9284 | 0.9349 | 0.9355 | |
12 | 0.9789 | 0.9642 | 0.9663 | 0.9507 | 0.9507 | 0.9479 | 0.9417 | |
15 | 0.9837 | 0.9715 | 0.9670 | 0.9468 | 0.9464 | 0.9497 | 0.9479 | |
Test9 | 4 | 0.9503 | 0.9374 | 0.9355 | 0.9158 | 0.9191 | 0.9118 | 0.9151 |
8 | 0.9633 | 0.9510 | 0.9500 | 0.9363 | 0.9272 | 0.9346 | 0.9351 | |
12 | 0.9772 | 0.9634 | 0.9655 | 0.9497 | 0.9488 | 0.9468 | 0.9402 | |
15 | 0.9820 | 0.9706 | 0.9655 | 0.9462 | 0.9455 | 0.9491 | 0.9460 | |
Test10 | 4 | 0.9505 | 0.9382 | 0.9375 | 0.9171 | 0.9207 | 0.9118 | 0.9167 |
8 | 0.9637 | 0.9519 | 0.9510 | 0.9367 | 0.9277 | 0.9360 | 0.9364 | |
12 | 0.9776 | 0.9653 | 0.9661 | 0.9511 | 0.9493 | 0.9472 | 0.9413 | |
15 | 0.9838 | 0.9710 | 0.9655 | 0.9481 | 0.9464 | 0.9504 | 0.9479 |
Table 7 and table 8 are PSNR and FSIM values of each algorithm respectively. As can be seen from the table, with the increase of the number of threshold values, the PSNR and FSIM values of the image have been significantly improved, indicating that the increase of the number of threshold values significantly improves the segmentation accuracy. The PSNR value of HEOA algorithm is better than other algorithms in all of the 24 groups. So, HEOA have a good competitive than other algorithms. Among all the data of FSIM, HEOA show an improvement of 1.42%, 1.35%, 4.21%, 4.02%, 4.19% and 4.09% over HSOA, HWOA, CSA, FPA, PSO and BA. This means that the segmentation results of HWOA are closer to the original image than other comparison algorithms.
Image | K | HEOA | HSOA | HWOA | CSA | FPA | PSO | BA |
Test1 | 4 | 23.3863 | 23.2573 | 23.2526 | 23.0420 | 23.0405 | 23.0397 | 23.0539 |
8 | 29.2231 | 29.1025 | 29.0968 | 28.9021 | 28.9007 | 28.8989 | 28.9020 | |
12 | 32.5692 | 32.4393 | 32.4442 | 32.2300 | 32.2265 | 32.2282 | 32.2414 | |
15 | 33.3295 | 33.1947 | 33.1953 | 33.0002 | 32.9903 | 32.9903 | 32.9923 | |
Test2 | 4 | 24.4702 | 24.3443 | 24.3386 | 24.1472 | 24.1340 | 24.1342 | 24.1432 |
8 | 29.4260 | 29.3020 | 29.2936 | 29.0852 | 29.0987 | 29.0861 | 29.1020 | |
12 | 32.5786 | 32.4454 | 32.4470 | 32.2471 | 32.2464 | 32.2522 | 32.2424 | |
15 | 34.2153 | 34.0865 | 34.0884 | 33.8828 | 33.8824 | 33.8823 | 33.8887 | |
Test3 | 4 | 24.4769 | 24.3474 | 24.3453 | 24.1433 | 24.1406 | 24.1517 | 24.1495 |
8 | 29.9667 | 29.8287 | 29.8366 | 29.6330 | 29.6383 | 29.6298 | 29.6211 | |
12 | 32.6661 | 32.5412 | 32.5392 | 32.3369 | 32.3265 | 32.3288 | 32.3254 | |
15 | 34.7330 | 34.6031 | 34.6015 | 34.3994 | 34.3925 | 34.4084 | 34.3905 | |
Test4 | 4 | 24.7640 | 24.6386 | 24.6369 | 24.4284 | 24.4400 | 24.4423 | 24.4286 |
8 | 29.5467 | 29.4208 | 29.4154 | 29.2214 | 29.2193 | 29.2207 | 29.2104 | |
12 | 33.5823 | 33.4474 | 33.4548 | 33.2561 | 33.2435 | 33.2461 | 33.2509 | |
15 | 34.2159 | 34.0826 | 34.0820 | 33.8787 | 33.8740 | 33.8707 | 33.8853 | |
Test5 | 4 | 24.5319 | 24.3991 | 24.3994 | 24.2048 | 24.1918 | 24.1918 | 24.1924 |
8 | 29.5550 | 29.4198 | 29.4251 | 29.2137 | 29.2181 | 29.2237 | 29.2154 | |
12 | 34.3322 | 34.1972 | 34.1979 | 34.0010 | 33.9935 | 33.9870 | 33.9959 | |
15 | 35.0669 | 34.9409 | 34.9366 | 34.7387 | 34.7254 | 34.7407 | 34.7279 | |
Test6 | 4 | 25.2119 | 25.0871 | 25.0842 | 24.8695 | 24.8803 | 24.8734 | 24.8829 |
8 | 30.9087 | 30.7880 | 30.7821 | 30.5869 | 30.5821 | 30.5735 | 30.5700 | |
12 | 34.1912 | 34.0606 | 34.0591 | 33.8576 | 33.8505 | 33.8585 | 33.8626 | |
15 | 35.7843 | 35.6567 | 35.6607 | 35.4492 | 35.4505 | 35.4438 | 35.4423 | |
Test7 | 4 | 25.4053 | 25.1458 | 25.1450 | 24.8847 | 25.0748 | 24.9284 | 24.9402 |
8 | 31.0914 | 30.8883 | 30.8601 | 30.7788 | 30.5828 | 30.6162 | 30.6718 | |
12 | 34.3507 | 34.1142 | 34.1742 | 34.0164 | 33.9942 | 33.8766 | 33.8983 | |
15 | 35.9362 | 35.6969 | 35.7545 | 35.6007 | 35.4588 | 35.4937 | 35.6024 | |
Test8 | 4 | 25.5670 | 25.2034 | 25.2799 | 24.9384 | 25.1283 | 24.9787 | 25.0445 |
8 | 31.2698 | 31.0054 | 30.9921 | 30.8672 | 30.7368 | 30.6972 | 30.8372 | |
12 | 34.5283 | 34.2658 | 34.2137 | 34.2017 | 34.1031 | 33.9285 | 34.0632 | |
15 | 36.0846 | 35.7585 | 35.9118 | 35.6393 | 35.6021 | 35.4988 | 35.6352 | |
Test9 | 4 | 25.5622 | 25.0597 | 25.1265 | 24.7749 | 24.9583 | 24.9368 | 24.8720 |
8 | 31.1656 | 30.8841 | 30.8166 | 30.7155 | 30.6732 | 30.6568 | 30.8154 | |
12 | 34.4249 | 34.0884 | 34.1830 | 34.1183 | 33.9539 | 33.7553 | 33.9067 | |
15 | 36.0382 | 35.7553 | 35.7737 | 35.5952 | 35.5146 | 35.3593 | 35.5413 | |
Test10 | 4 | 25.4424 | 24.9891 | 25.1108 | 24.7329 | 24.7752 | 24.9286 | 24.8300 |
8 | 31.1315 | 30.7681 | 30.7427 | 30.5414 | 30.6599 | 30.5129 | 30.6245 | |
12 | 34.3961 | 33.9364 | 34.1416 | 33.9835 | 33.8675 | 33.7435 | 33.8129 | |
15 | 35.8866 | 35.7225 | 35.7457 | 35.4554 | 35.4165 | 35.2905 | 35.3448 |
Image | K | HEOA | HSOA | HWOA | CSA | FPA | PSO | BA |
Test1 | 4 | 0.9367 | 0.9236 | 0.9225 | 0.9094 | 0.9039 | 0.8944 | 0.9087 |
8 | 0.9517 | 0.9414 | 0.9390 | 0.9036 | 0.9077 | 0.9157 | 0.9084 | |
12 | 0.9587 | 0.9433 | 0.9473 | 0.9135 | 0.9149 | 0.9172 | 0.9135 | |
15 | 0.9668 | 0.9511 | 0.9540 | 0.9271 | 0.9331 | 0.9287 | 0.9284 | |
Test2 | 4 | 0.9356 | 0.9227 | 0.9262 | 0.9006 | 0.8943 | 0.8936 | 0.9065 |
8 | 0.9584 | 0.9422 | 0.9438 | 0.9221 | 0.9123 | 0.9122 | 0.9230 | |
12 | 0.9632 | 0.9548 | 0.9563 | 0.9283 | 0.9305 | 0.9331 | 0.9233 | |
15 | 0.9633 | 0.9553 | 0.9506 | 0.9242 | 0.9251 | 0.9255 | 0.9202 | |
Test3 | 4 | 0.9325 | 0.9197 | 0.9181 | 0.9003 | 0.9077 | 0.8898 | 0.9078 |
8 | 0.9477 | 0.9333 | 0.9362 | 0.9060 | 0.9062 | 0.9001 | 0.9048 | |
12 | 0.9599 | 0.9482 | 0.9483 | 0.9289 | 0.9289 | 0.9190 | 0.9162 | |
15 | 0.9607 | 0.9487 | 0.9468 | 0.9309 | 0.9251 | 0.9325 | 0.9358 | |
Test4 | 4 | 0.9385 | 0.9213 | 0.9207 | 0.9065 | 0.9063 | 0.8982 | 0.8954 |
8 | 0.9485 | 0.9362 | 0.9349 | 0.9057 | 0.9058 | 0.9115 | 0.9144 | |
12 | 0.9580 | 0.9487 | 0.9498 | 0.9240 | 0.9298 | 0.9154 | 0.9200 | |
15 | 0.9637 | 0.9457 | 0.9532 | 0.9229 | 0.9227 | 0.9321 | 0.9237 | |
Test5 | 4 | 0.9332 | 0.9213 | 0.9264 | 0.8894 | 0.9071 | 0.9082 | 0.8960 |
8 | 0.9469 | 0.9377 | 0.9361 | 0.9085 | 0.9141 | 0.9083 | 0.9106 | |
12 | 0.9642 | 0.9438 | 0.9503 | 0.9210 | 0.9254 | 0.9187 | 0.9270 | |
15 | 0.9687 | 0.9484 | 0.9489 | 0.9240 | 0.9174 | 0.9240 | 0.9267 | |
Test6 | 4 | 0.9304 | 0.9238 | 0.9186 | 0.8868 | 0.9055 | 0.8972 | 0.9054 |
8 | 0.9518 | 0.9317 | 0.9372 | 0.9027 | 0.9099 | 0.9117 | 0.9085 | |
12 | 0.9579 | 0.9492 | 0.9460 | 0.9280 | 0.9270 | 0.9258 | 0.9158 | |
15 | 0.9682 | 0.9517 | 0.9490 | 0.9289 | 0.9204 | 0.9315 | 0.9250 | |
Test7 | 4 | 0.9313 | 0.9252 | 0.9198 | 0.8882 | 0.9057 | 0.8984 | 0.9060 |
8 | 0.9536 | 0.9322 | 0.9384 | 0.9035 | 0.9109 | 0.9127 | 0.9087 | |
12 | 0.9590 | 0.9498 | 0.9477 | 0.9287 | 0.9280 | 0.9273 | 0.9177 | |
15 | 0.9699 | 0.9524 | 0.9510 | 0.9292 | 0.9222 | 0.9335 | 0.9258 | |
Test8 | 4 | 0.9294 | 0.9239 | 0.9181 | 0.8877 | 0.9044 | 0.8970 | 0.9058 |
8 | 0.9518 | 0.9309 | 0.9381 | 0.9029 | 0.9096 | 0.9116 | 0.9070 | |
12 | 0.9573 | 0.9487 | 0.9470 | 0.9276 | 0.9263 | 0.9263 | 0.9175 | |
15 | 0.9682 | 0.9513 | 0.9494 | 0.9280 | 0.9216 | 0.9324 | 0.9240 | |
Test9 | 4 | 0.9292 | 0.9234 | 0.9161 | 0.8866 | 0.9038 | 0.8956 | 0.9052 |
8 | 0.9506 | 0.9296 | 0.9368 | 0.9010 | 0.9089 | 0.9096 | 0.9064 | |
12 | 0.9569 | 0.9469 | 0.9470 | 0.9273 | 0.9250 | 0.9262 | 0.9160 | |
15 | 0.9676 | 0.9501 | 0.9474 | 0.9275 | 0.9216 | 0.9308 | 0.9236 | |
Test10 | 4 | 0.9296 | 0.9246 | 0.9170 | 0.8874 | 0.9046 | 0.8962 | 0.9057 |
8 | 0.9509 | 0.9306 | 0.9378 | 0.9025 | 0.9093 | 0.9098 | 0.9069 | |
12 | 0.9589 | 0.9483 | 0.9490 | 0.9275 | 0.9261 | 0.9277 | 0.9165 | |
15 | 0.9678 | 0.9501 | 0.9479 | 0.9287 | 0.9232 | 0.9316 | 0.9240 |
Table 9 shows the CPU time of each algorithm under different thresholds. When the threshold value K = 4, the results of each optimization algorithm differ little. At this point, the number of thresholds is small, the search space is small, and the optimization capability of each optimization algorithm is basically the same. When K = 15, the computational complexity of image segmentation increases and the CPU time increases absolutely. The average time of each algorithm in the test image is: HEOA < HSOA < HWOA < FPA < CSA < BA < PSO. So, the HEOA algorithm not only has the good performance in image segmentation, but also has the less CPU time than other compared algorithms.Table 6. The Uniformity measure for the comparison algorithms.
Image | K | HEOA | HSOA | HWOA | CSA | FPA | PSO | BA |
Test1 | 4 | 1.2145 | 1.2251 | 1.2247 | 1.2442 | 1.2405 | 1.2406 | 1.2389 |
8 | 1.3154 | 1.3254 | 1.3260 | 1.3452 | 1.3422 | 1.3386 | 1.3385 | |
12 | 1.5172 | 1.5274 | 1.5279 | 1.5448 | 1.5467 | 1.5451 | 1.5466 | |
15 | 1.8157 | 1.8260 | 1.8258 | 1.8359 | 1.8435 | 1.8376 | 1.8439 | |
Test2 | 4 | 1.2149 | 1.2259 | 1.2255 | 1.2363 | 1.2392 | 1.2436 | 1.2365 |
8 | 1.3154 | 1.3262 | 1.3263 | 1.3388 | 1.3411 | 1.3401 | 1.3371 | |
12 | 1.5181 | 1.5286 | 1.5288 | 1.5423 | 1.5449 | 1.5466 | 1.5389 | |
15 | 1.8158 | 1.8266 | 1.8266 | 1.8419 | 1.8398 | 1.8409 | 1.8424 | |
Test3 | 4 | 1.2151 | 1.2257 | 1.2258 | 1.2354 | 1.2367 | 1.2445 | 1.2367 |
8 | 1.3158 | 1.3268 | 1.3265 | 1.3402 | 1.3411 | 1.3456 | 1.3436 | |
12 | 1.5191 | 1.5292 | 1.5292 | 1.5394 | 1.5406 | 1.5474 | 1.5487 | |
15 | 1.8164 | 1.8270 | 1.8273 | 1.8462 | 1.8369 | 1.8437 | 1.8413 | |
Test4 | 4 | 1.2151 | 1.2254 | 1.2253 | 1.2444 | 1.2358 | 1.2358 | 1.2409 |
8 | 1.3166 | 1.3268 | 1.3271 | 1.3432 | 1.3398 | 1.3458 | 1.3461 | |
12 | 1.5192 | 1.5299 | 1.5301 | 1.5421 | 1.5476 | 1.5483 | 1.5465 | |
15 | 1.8166 | 1.8275 | 1.8269 | 1.8390 | 1.8466 | 1.8422 | 1.8425 | |
Test5 | 4 | 1.2161 | 1.2269 | 1.2269 | 1.2388 | 1.2447 | 1.2368 | 1.2401 |
8 | 1.3167 | 1.3273 | 1.3273 | 1.3456 | 1.3396 | 1.3382 | 1.3431 | |
12 | 1.5201 | 1.5304 | 1.5302 | 1.5471 | 1.5428 | 1.5454 | 1.5401 | |
15 | 1.8169 | 1.8274 | 1.8278 | 1.8431 | 1.8379 | 1.8456 | 1.8405 | |
Test6 | 4 | 1.2168 | 1.2271 | 1.2268 | 1.2372 | 1.2413 | 1.2412 | 1.2383 |
8 | 1.3172 | 1.3281 | 1.3273 | 1.3412 | 1.3407 | 1.3448 | 1.3379 | |
12 | 1.5206 | 1.5307 | 1.5313 | 1.5491 | 1.5408 | 1.5445 | 1.5493 | |
15 | 1.8169 | 1.8278 | 1.8270 | 1.8395 | 1.8463 | 1.8410 | 1.8428 | |
Test7 | 4 | 1.2183 | 1.2275 | 1.2282 | 1.2373 | 1.2421 | 1.2429 | 1.2399 |
8 | 1.3179 | 1.3284 | 1.3291 | 1.3429 | 1.3420 | 1.3463 | 1.3391 | |
12 | 1.5219 | 1.5326 | 1.5316 | 1.5510 | 1.5410 | 1.5462 | 1.5506 | |
15 | 1.8179 | 1.8293 | 1.8289 | 1.8408 | 1.8466 | 1.8410 | 1.8440 | |
Test8 | 4 | 1.2173 | 1.2270 | 1.2272 | 1.2371 | 1.2403 | 1.2424 | 1.2393 |
8 | 1.3167 | 1.3278 | 1.3282 | 1.3409 | 1.3401 | 1.3447 | 1.3386 | |
12 | 1.5217 | 1.5308 | 1.5303 | 1.5497 | 1.5398 | 1.5460 | 1.5498 | |
15 | 1.8172 | 1.8273 | 1.8284 | 1.8396 | 1.8446 | 1.8397 | 1.8424 | |
Test9 | 4 | 1.2154 | 1.2265 | 1.2258 | 1.2357 | 1.2391 | 1.2409 | 1.2376 |
8 | 1.3166 | 1.3268 | 1.3277 | 1.3398 | 1.3389 | 1.3428 | 1.3375 | |
12 | 1.5204 | 1.5304 | 1.5291 | 1.5482 | 1.5397 | 1.5453 | 1.5485 | |
15 | 1.8154 | 1.8272 | 1.8281 | 1.8395 | 1.8431 | 1.8385 | 1.8422 | |
Test10 | 4 | 1.2155 | 1.2282 | 1.2270 | 1.2377 | 1.2404 | 1.2419 | 1.2380 |
8 | 1.3172 | 1.3283 | 1.3279 | 1.3401 | 1.3399 | 1.3444 | 1.3391 | |
12 | 1.5207 | 1.5319 | 1.5305 | 1.5494 | 1.5397 | 1.5460 | 1.5495 | |
15 | 1.8160 | 1.8272 | 1.8297 | 1.8400 | 1.8440 | 1.8393 | 1.8442 |
The experimental results of each algorithm are the same, so statistical tests are needed. Parametric statistical tests are based on various assumptions [59]. The well-known non-parametric statistical tests, namely Friedman test [60] and Wilcoxon rank-sum test [61] are used in this section. For the average rank of Friedman's test given in table 10, the method presented in this paper takes the first place in all cases, and as the number of threshold levels increases, the rank value becomes smaller and smaller, which has greater advantages compared with other comparison methods. Through the above analysis, as the dimension of optimization problem increases, the optimization ability of the proposed algorithm HEOA becomes more and more obvious.
K | HEOA | HSOA | HWOA | CSA | FPA | PSO | BA |
4 | 3.1581 | 3.4458 | 3.5147 | 3.4581 | 3.6152 | 3.9951 | 3.8547 |
8 | 2.9315 | 3.5514 | 3.6891 | 3.5125 | 3.9156 | 3.9514 | 3.6984 |
12 | 1.9984 | 2.5589 | 3.6661 | 3.1285 | 3.9991 | 3.9541 | 3.8854 |
15 | 1.7415 | 2.6518 | 3.6581 | 3.1814 | 3.8147 | 3.8574 | 3.7781 |
Overall | 2.4574 | 3.0520 | 3.6320 | 3.3201 | 3.8362 | 3.9395 | 3.8042 |
The experimental statistical results are shown in Table 11 below. The null hypothesis is constructed as: there is no significant difference between the two algorithms. The alternative hypothesis states that there is a significant difference between the two algorithms. In the experiment, HEOA based method produces better result in 36 out of 40 cases when compared with HSOA based method and produces better result in 35 out of 40 cases when compared with HWOA based method and produces better result in 38 out of 40 cases when compared with CSA based method and produces better result in 38 out of 40 cases when compared with FPA based method and produces better result in 40 out of 40 cases when compared with the PSO based method. As can be seen from the results, there are significant differences among the six algorithms. In most cases, HEOA performs better than other algorithms.
Images | K | HSOA | HWOA | CSA | FPA | PSO |
Test1 | 4 | P < 0.05 | P < 0.05 | P < 0.05 | P < 0.05 | P < 0.05 |
8 | P > 0.05 | P < 0.05 | P < 0.05 | P < 0.05 | P < 0.05 | |
12 | P < 0.05 | P < 0.05 | P < 0.05 | P < 0.05 | P < 0.05 | |
15 | P < 0.05 | P > 0.05 | P < 0.05 | P < 0.05 | P < 0.05 | |
Test2 | 4 | P < 0.05 | P < 0.05 | P < 0.05 | P > 0.05 | P < 0.05 |
8 | P < 0.05 | P < 0.05 | P < 0.05 | P < 0.05 | P < 0.05 | |
12 | P < 0.05 | P < 0.05 | P < 0.05 | P < 0.05 | P < 0.05 | |
15 | P < 0.05 | P < 0.05 | P < 0.05 | P < 0.05 | P < 0.05 | |
Test3 | 4 | P < 0.05 | P < 0.05 | P < 0.05 | P < 0.05 | P < 0.05 |
8 | P < 0.05 | P < 0.05 | P < 0.05 | P < 0.05 | P < 0.05 | |
12 | P < 0.05 | P < 0.05 | P < 0.05 | P < 0.05 | P < 0.05 | |
15 | P < 0.05 | P < 0.05 | P < 0.05 | P < 0.05 | P < 0.05 | |
Test4 | 4 | P < 0.05 | P < 0.05 | P < 0.05 | P < 0.05 | P < 0.05 |
8 | P > 0.05 | P < 0.05 | P < 0.05 | P < 0.05 | P < 0.05 | |
12 | P < 0.05 | P > 0.05 | P < 0.05 | P < 0.05 | P < 0.05 | |
15 | P < 0.05 | P < 0.05 | P < 0.05 | P < 0.05 | P < 0.05 | |
Test5 | 4 | P < 0.05 | P < 0.05 | P < 0.05 | P < 0.05 | P < 0.05 |
8 | P < 0.05 | P < 0.05 | P < 0.05 | P < 0.05 | P < 0.05 | |
12 | P < 0.05 | P < 0.05 | P < 0.05 | P < 0.05 | P < 0.05 | |
15 | P < 0.05 | P < 0.05 | P < 0.05 | P < 0.05 | P < 0.05 | |
Test6 | 4 | P > 0.05 | P < 0.05 | P < 0.05 | P < 0.05 | P < 0.05 |
8 | P < 0.05 | P < 0.05 | P < 0.05 | P < 0.05 | P < 0.05 | |
12 | P < 0.05 | P < 0.05 | P < 0.05 | P < 0.05 | P < 0.05 | |
15 | P < 0.05 | P < 0.05 | P < 0.05 | P < 0.05 | P < 0.05 | |
Test7 | 4 | P < 0.05 | P < 0.05 | P > 0.05 | P < 0.05 | P < 0.05 |
8 | P < 0.05 | P < 0.05 | P < 0.05 | P < 0.05 | P < 0.05 | |
12 | P < 0.05 | P < 0.05 | P < 0.05 | P < 0.05 | P < 0.05 | |
15 | P < 0.05 | P < 0.05 | P < 0.05 | P < 0.05 | P < 0.05 | |
Test8 | 4 | P > 0.05 | P < 0.05 | P < 0.05 | P > 0.05 | P < 0.05 |
8 | P < 0.05 | P > 0.05 | P < 0.05 | P < 0.05 | P < 0.05 | |
12 | P < 0.05 | P < 0.05 | P < 0.05 | P < 0.05 | P < 0.05 | |
15 | P < 0.05 | P < 0.05 | P < 0.05 | P < 0.05 | P < 0.05 | |
Test9 | 4 | P < 0.05 | P < 0.05 | P < 0.05 | P < 0.05 | P < 0.05 |
8 | P < 0.05 | P > 0.05 | P < 0.05 | P < 0.05 | P < 0.05 | |
12 | P < 0.05 | P < 0.05 | P < 0.05 | P < 0.05 | P < 0.05 | |
15 | P < 0.05 | P < 0.05 | P < 0.05 | P < 0.05 | P < 0.05 | |
Test10 | 4 | P < 0.05 | P > 0.05 | P > 0.05 | P < 0.05 | P < 0.05 |
8 | P < 0.05 | P < 0.05 | P < 0.05 | P < 0.05 | P < 0.05 | |
12 | P < 0.05 | P < 0.05 | P < 0.05 | P < 0.05 | P < 0.05 | |
15 | P < 0.05 | P < 0.05 | P < 0.05 | P < 0.05 | P < 0.05 |
In this section, we compare with the novel image segmentation methods. The compared algorithms are PCNN [62], fuzzy c-means (FCM) [63], grayscale co-occurrence matrix (GLCM) [64]. In order to test the performance of the color image segmentation algorithms, we select the wood fibers which are taken under a high-powered microscope as the test images. The figures 8–11 show the wood fiber images and the segmentation result.
It can be seen from the figures 4–7, the result of PCNN has the over-segmentation phenomenon and the segmented images are the worst of the compared algorithms. The FCM and GLCM have the under-segmentation phenomenon and the results of the fiber images are not clear. Among the result of the fiber images, the HEOA-3DOtsu get the best result. The table 12 shows the HEOS obtains a large improvement than the others compared image segmentation methods. The recall and precision are 93.19% and 91.28% respectively. The results show that the performance of this method can provide the segmentation result of the wood fiber image. The Figure12 show the segmentation result of the proposed method. It can be seen from figure, the number of wood fiber can be seen clearly. This method obtains independent wood fiber area. So, the proposed method can segment the wood fiber images successfully and have strong robustness.
Method | R (%) | P (%) | AP (%) | VAL (%) |
FCM | 84.94 | 90.88 | 91.23 | 88.47 |
PCNN | 80.46 | 90.69 | 81.46 | 79.46 |
DLA | 70.12 | 92.95 | 78.32 | 77.57 |
BMPA | 93.19 | 91.28 | 91.45 | 90.38 |
The analysis of wood fiber graphics can understand the state of the fiber, so as to ensure the production of wood or paper that meets industrial requirements. The scholar Mainly analyze the shape and thickness of individual wood fibers. However, the wood fibers in the collected graphics are more responsible and similar to the background. Traditional segmentation methods cannot solve this problem. So, study the novel image segmentation method is necessary.
In the field of optimization algorithm, the proposed method has a good optimal ability solve the CEC2015. And the proposed method has a good competitiveness with the CSA, FPA, PSO, BA and basic EOA. Because of the hybrid optimization algorithm use the advantage of the two optimization algorithms to optimize the complex benchmark function. At the same time, the proposed method solves the threshold image segmentation and find the best threshold from the 3DOtsu. The algorithm gets the best result among CSA, FPA, PSO, BA, HSOA and HWOA. The hybrid optimization algorithms enhance the optimal ability of the original optimization algorithm.
In the field of the fiber wood image segmentation, the proposed method obtains the highest segmentation accuracy with PCNN, FCN and GLCM. The PCNN obtains the worst result in compared algorithms, the performance of PCNN rely on the set of parameters. The FCN and GLCM has strong ability to solve the grey image, however, these two methods take huge challenge to segment color image. The proposed method can overcome the difficult of color image. Finally, the proposed method obtains the wood fiber area exactly.
The limitations of the proposed method can be divided in two contents. First, similar to other optimization algorithms, this algorithm takes time to iterate to find the best solution, which is time-consuming. Second, this method has strong performance in the field of wood fiber image segmentation. In order to make the proposed method can adopt more real area, we will study the novel optimization algorithm.
The proposed method can solve the other problem of optimization. At the same time, it can be used in the field of medical image segmentation, forest fire image segmentation and so on. In the future, we will continue to study many object multi-level threshold methods and different optimization algorithms, so as to improve the image segmentation accuracy.
In this paper, the HEOA algorithm is used to optimize the 3DOtsu algorithm to obtain the optimal multi-threshold image. We use GOA to improve EOA algorithm and enhance the optimization ability of the algorithm. The CEC2015 is selected as benchmark function to test the performance of the hybrid optimization algorithms. The result shows that the hybrid algorithm can enhance the optimal ability of the EOA. And then, the HEOA is compared with other optimization algorithms to jointly optimize 3DOtsu algorithm for classic images and wood fiber images segmentation experiments. From U measure, PSNR and FSIM values, it can be seen that 3DOtsu-HEOA algorithm has the best segmentation accuracy. Finally, we compare the 3DOtsu-HEOA algorithm with the novel image segmentation method. It can be seen from the segmented result that HEOA can get the best segmented result. So, the 3DOtsu-HEOA algorithm proposed in this paper has better image segmentation accuracy and better stability.
This work was supported by the Fundamental Research Funds for the Central Universities under Grant No. 2572018BH08.
The authors have no conflict of interest.
[1] |
Wise J (2023) Covid-19: WHO declares end of global health emergency. BMJ 381: 1041. https://doi.org/10.1136/bmj.p1041 ![]() |
[2] | Harris E (2023) WHO declares end of COVID-19 global health emergency. JAMA 329: 1817. https://doi.org/10.1001/jama.2023.8656 |
[3] | World Health OrganizationCoronavirus disease (COVID-2019) situation reports (2020). Available from: https://www.who.int/emergencies/diseases/novel-coronavirus-2019/situation-reports/ |
[4] |
Nkengasong JN (2021) COVID-19: Unprecedented but expected. Nat Med 27: 363-366. https://doi.org/10.1038/s41591-021-01269-x ![]() |
[5] |
Huremović D (2019) Brief history of pandemics (pandemics throughout history). Psychiatry of Pandemics 16: 7-35. https://doi.org/10.1007/978-3-030-15346-5_2 ![]() |
[6] |
Van Damme W, Dahake R, Delamou A, et al. (2020) The COVID-19 pandemic: Diverse contexts; different epidemics—How and why?. BMJ Global Health 5: e003098. https://doi.org/10.1136/bmjgh-2020-003098 ![]() |
[7] |
Milionis C, Ilias I, Tselebis A, et al. (2023) Psychological and social aspects of vaccination hesitancy—implications for travel medicine in the aftermath of the COVID-19 crisis: A narrative review. Medicina 59: 1744. https://doi.org/10.3390/medicina59101744 ![]() |
[8] | Ke R, Sanche S, Romero-Severson E, et al. (2020) Fast spread of COVID-19 in Europe and the US suggests the necessity of early, strong and comprehensive interventions. medRxiv [Preprint] : 2020.04.04.20050427. https://doi.org/10.1101/2020.04.04.20050427 |
[9] |
Read J M, Bridgen J R, Cummings D A, et al. (2021) Novel coronavirus 2019-nCoV (COVID-19): early estimation of epidemiological parameters and epidemic size estimates. Philos T Roy Soc B 376: 20200265. https://doi.org/10.1098/rstb.2020.0265 ![]() |
[10] |
Syangtan G, Bista S, Dawadi P, et al. (2021) Asymptomatic SARS-CoV-2 carriers: A systematic review and meta-analysis. Front Public Health 8: 587374. https://doi.org/10.3389/fpubh.2020.587374 ![]() |
[11] |
Litchfield I, Shukla D, Greenfield S (2021) Impact of COVID-19 on the digital divide: A rapid review. BMJ Open 11: e053440. https://doi.org/10.1136/bmjopen-2021-053440 ![]() |
[12] | Tselebis A, Sikaras C, Milionis C, et al. (2023) A moderated mediation model of the influence of cynical distrust, medical mistrust, and anger on vaccination hesitancy in nursing staff. Eur J Investig Health Psychol Educ 13: 2373-2387. https://doi.org/10.3390/ejihpe13110167 |
[13] |
Park E, Kim WH, Kim SB (2022) How does COVID-19 differ from previous crises? A comparative study of health-related crisis research in the tourism and hospitality context. Int J Hosp Manag 103: 103199. https://doi.org/10.1016/j.ijhm.2022.103199 ![]() |
[14] | Contreras GW, Burcescu B, Dang T, et al. (2021) Drawing parallels among past public health crises and COVID-19. Disaster Med Public Health Prep 18: 1-7. https://doi.org/10.1017/dmp.2021.202 |
[15] |
Dubey S, Biswas P, Ghosh R, et al. (2020) Psychosocial impact of COVID-19. Diabetes Metab Syndr 14: 779-788. https://doi.org/10.1016/j.dsx.2020.05.035 ![]() |
[16] |
Tselebis A, Pachi A (2022) Primary mental health care in a new era. Healthcare 10: 2025. https://doi.org/10.3390/healthcare10102025 ![]() |
[17] |
Marvaldi M, Mallet J, Dubertret C, et al. (2021) Anxiety, depression, trauma-related, and sleep disorders among healthcare workers during the COVID-19 pandemic: A systematic review and meta-analysis. Neurosci Biobehav Rev 126: 252-264. https://doi.org/10.1016/j.neubiorev.2021.03.024 ![]() |
[18] |
Varghese A, George G, Kondaguli SV, et al. (2021) Decline in the mental health of nurses across the globe during COVID-19: A systematic review and meta-analysis. J Glob Health 11: 05009. https://doi.org/10.7189/jogh.11.05009 ![]() |
[19] |
Pachi A, Sikaras C, Ilias I, et al. (2022) Burnout, depression and sense of coherence in nurses during the pandemic crisis. Healthcare 10: 134. https://doi.org/10.3390/healthcare10010134 ![]() |
[20] |
Chávez Sosa JV, Mego Gonzales FM, Aliaga Ramirez ZE, et al. (2022) Depression associated with caregiver quality of life in post-COVID-19 patients in two regions of Peru. Healthcare 10: 1219. https://doi.org/10.3390/healthcare10071219 ![]() |
[21] |
Sikaras C, Zyga S, Tsironi M, et al. (2023) The mediating role of depression and of state anxiety οn the relationship between trait anxiety and fatigue in nurses during the pandemic crisis. Healthcare 11: 367. https://doi.org/10.3390/healthcare11030367 ![]() |
[22] |
Jahrami H A, Alhaj O A, Humood A M, et al. (2022) Sleep disturbances during the COVID-19 pandemic: A systematic review, meta-analysis, and meta-regression. Sleep Med Rev 62: 101591. https://doi.org/10.1016/j.smrv.2022.101591 ![]() |
[23] |
Tselebis A, Lekka D, Sikaras C, et al. (2020) Insomnia, perceived stress, and family support among nursing staff during the pandemic crisis. Healthcare 8: 434. https://doi.org/10.3390/healthcare8040434 ![]() |
[24] |
Sikaras C, Tsironi M, Zyga S, et al. (2023) Anxiety, insomnia and family support in nurses, two years after the onset of the pandemic crisis. AIMS Public Health 10: 252-267. https://doi.org/10.3934/publichealth.2023019 ![]() |
[25] |
Yazdi Z, Sadeghniiat-Haghighi K, Javadi AR, et al. (2014) Sleep quality and insomnia in nurses with different circadian chronotypes: Morningness and eveningness orientation. Work 47: 561-567. https://doi.org/10.3233/WOR-131664 ![]() |
[26] |
Jessica R Dietch, Daniel J Taylor, Kristi Pruiksma, et al. (2021) The nightmare disorder index: Development and initial validation in a sample of nurses. Sleep 44: zsaa254. https://doi.org/10.1093/sleep/zsaa254 ![]() |
[27] |
Kelly W E (2022) Bad dreams and bad sleep: Relationships between nightmare frequency, insomnia, and nightmare proneness. Dreaming 32: 194-205. https://doi.org/10.1037/drm0000203 ![]() |
[28] |
Lin YQ, Lin ZX, Wu YX, et al. (2020) Reduced sleep duration and sleep efficiency were independently associated with frequent nightmares in Chinese frontline medical workers during the coronavirus disease 2019 outbreak. Front Neurosci 14: 631025. https://doi.org/10.3389/fnins.2020.631025 ![]() |
[29] |
Hublin C, Kaprio J, Partinen M, et al. (1999) Nightmares: Familial aggregation and association with psychiatric disorders in a nationwide twin cohort. Am J Med Genet 88: 329-336. https://doi.org/10.1002/(SICI)1096-8628(19990820)88:4<329::AID-AJMG8>3.0.CO;2-E ![]() |
[30] |
Ohayon MM, Guilleminault C, Priest RG (1999) Night terrors, sleepwalking and confusional arousals in the general population: Their frequency and relationship to other sleep and mental disorders. J Clin Psychiatry 60: 268-276. https://doi.org/10.4088/jcp.v60n0413 ![]() |
[31] |
Bjorvatn B, Magerøy N, Moen BE, et al. (2015) Parasomnias are more frequent in shift workers than in day workers. Chronobiol Int 32: 1352-1358. https://doi.org/10.3109/07420528.2015.1091354 ![]() |
[32] | Acker KH (1993) Do critical care nurses face burnout, PTSD, or is it something else?: getting help for the helpers. AACN Clin Issues Crit Care Nurs 4: 558-565. |
[33] |
Kaubisch LT, Reck C, von Tettenborn A, et al. (2022) The COVID-19 pandemic as a traumatic event and the associated psychological impact on families-A systematic review. J Affect Disord 319: 27-39. https://doi.org/10.1016/j.jad.2022.08.109 ![]() |
[34] |
Li Y, Abbas Q, Manthar S, et al. (2022) Fear of COVID-19 and secondary trauma: Moderating role of self-efficacy. Front Psychol 13: 838451. https://doi.org/10.3389/fpsyg.2022.838451 ![]() |
[35] |
Qiu D, Li Y, Li L, et al. (2021) Prevalence of post-traumatic stress symptoms among people influenced by coronavirus disease 2019 outbreak: A meta-analysis. Eur Psychiatry 64: e30. https://doi.org/10.1192/j.eurpsy.2021.24 ![]() |
[36] |
Secrist ME, John SG, Harper SL, et al. (2020) Nightmares in treatment-seeking youth: The role of cumulative trauma exposure. J Child Adolesc Trauma 13: 249-256. https://doi.org/10.1007/s40653-019-00268-y ![]() |
[37] |
Milanak ME, Zuromski KL, Cero I, et al. (2019) Traumatic event exposure, posttraumatic stress disorder, and sleep disturbances in a national sample of U.S. adults. J Trauma Stress 32: 14-22. https://doi.org/10.1002/jts.22360 ![]() |
[38] |
Stewart NH, Koza A, Dhaon S, et al. (2021) Sleep disturbances in frontline health care workers during the COVID-19 pandemic: Social media survey study. J Med Internet Res 23: e27331. https://doi.org/10.2196/27331 ![]() |
[39] |
Sateia M J (2014) International classification of sleep disorders-third edition. Chest 146: 1387-1394. https://doi.org/10.1378/chest.14-0970 ![]() |
[40] |
Brock MS, Powell TA, Creamer JL, et al. (2019) Trauma associated sleep disorder: Clinical developments 5 years after discovery. Curr Psychiatry Rep 21: 80. https://doi.org/10.1007/s11920-019-1066-4 ![]() |
[41] |
Mysliwiec V, Brock MS, Creamer JL, et al. (2018) Trauma associated sleep disorder: A parasomnia induced by trauma. Sleep Med Rev 37: 94-104. https://doi.org/10.1016/j.smrv.2017.01.004 ![]() |
[42] |
Kim Cohen J, Turkewitz R (2012) Resilience and measured gene–environment interactions. Dev Psychopathol 24: 1297-1306. https://doi.org/10.1017/S0954579412000715 ![]() |
[43] |
Pietrzak R H, Southwick S M (2011) Psychological resilience in OEF–OIF Veterans: Application of a novel classification approach and examination of demographic and psychosocial correlates. J Affect Disord 133: 560-568. https://doi.org/10.1016/j.jad.2011.04.028 ![]() |
[44] | Southwick SM, Bonanno GA, Masten AS, et al. (2014) Resilience definitions, theory, and challenges: Interdisciplinary perspectives. Eur J Psychotraumatol 1: 5. https://doi.org/10.3402/ejpt.v5.25338 |
[45] |
Cheng M Y, Wang M J, Chang M Y, et al. (2020) Relationship between resilience and insomnia among the middle-aged and elderly: Mediating role of maladaptive emotion regulation strategies. Psychol Health Med 25: 1266-1277. https://doi.org/10.1080/13548506.2020.1734637 ![]() |
[46] |
Palagini L, Moretto U, Novi M, et al. (2018) Lack of resilience is related to stress-related sleep reactivity, hyperarousal, and emotion dysregulation in insomnia disorder. J Clin Sleep Med 14: 759-766. https://doi.org/10.5664/jcsm.7100 ![]() |
[47] |
Cooper A L, Brown J A, Leslie G D (2021) Nurse resilience for clinical practice: An integrative review. J Adv Nurs 77: 2623-2640. https://doi.org/10.1111/jan.14763 ![]() |
[48] |
Pachi A, Kavourgia E, Bratis D, et al. (2023) Anger and aggression in relation to psychological resilience and alcohol abuse among health professionals during the first pandemic wave. Healthcare 11: 2031. https://doi.org/10.3390/healthcare11142031 ![]() |
[49] |
Pachi A, Anagnostopoulou M, Antoniou A, et al. (2023) Family support, anger and aggression in health workers during the first wave of the pandemic. AIMS Public Health 10: 524-537. https://doi.org/10.3934/publichealth.2023037 ![]() |
[50] |
Jérémie Potvin, Laura Ramos Socarras, Geneviève Forest (2021) 669 increased nightmares during the COVID-19 pandemic: Exploring the role of resilience and emotions. Sleep 44: A261-A262. https://doi.org/10.1093/sleep/zsab072.667 ![]() |
[51] |
Faul F, Erdfelder E, Lang AG, et al. (2009) Statistical power analyses using G*Power 3.1: Tests for correlation and regression analyses. Behav Res Methods 41: 1149-1160. https://doi.org/10.3758/BRM.41.4.1149 ![]() |
[52] |
Schoemann AM, Boulton AJ, Short SD (2017) Determining power and sample size for simple and complex mediation models. Soc Psychol. Personal Sci 8: 379-386. https://doi.org/10.1177/1948550617715068 ![]() |
[53] |
Belicki K (1992) The relationship of nightmare frequency to nightmare suffering with implications for treatment and research. Dreaming 2: 143-148. https://doi.org/10.1037/h0094355 ![]() |
[54] |
Belicki K (1992) Nightmare frequency versus nightmare distress: Relations to psychopathology and cognitive style. J Abnorm Psychol 101: 592-597. https://doi.org/10.1037/0021-843X.101.3.592 ![]() |
[55] |
Böckermann M, Gieselmann A, Pietrowsky R (2014) What does nightmare distress mean? Factorial structure and psychometric properties of the Nightmare Distress Questionnaire (NDQ). Dreaming 24: 279-289. https://doi.org/10.1037/a0037749 ![]() |
[56] |
Smith BW, Dalen J, Wiggins K, et al. (2008) The brief resilience scale: Assessing the ability to bounce back. Int J Behav Med 15: 194-200. https://doi.org/10.1080/10705500802222972 ![]() |
[57] |
Kyriazos TA, Stalikas A, Prassa K, et al. (2018) Psychometric evidence of the Brief Resilience Scale (BRS) and modeling distinctiveness of resilience from depression and stress. Psychology 9: 1828-1857. https://doi.org/21527180-201807-201808160034-201808160034-1828-1857 ![]() |
[58] |
Soldatos CR, Dikeos DG, Paparrigopoulos TJ (2003) The diagnostic validity of the Athens Insomnia Scale. J Psychosom Res 55: 263-267. https://doi.org/10.1016/s0022-3999(02)00604-9 ![]() |
[59] |
Soldatos CR, Dikeos DG, Paparrigopoulos TJ (2000) Athens Insomnia Scale: Validation of an instrument based on ICD-10 criteria. J Psychosom Res 48: 555-560. https://doi.org/10.1016/s0022-3999(00)00095-7 ![]() |
[60] |
Hayes A F, Rockwood N J (2020) Conditional process analysis: Concepts, computation, and advances in the modeling of the contingencies of mechanisms. Am Behav Sci 64: 19-54. https://doi.org/10.1177/0002764219859633 ![]() |
[61] |
Hayes AF (2015) An index and test of linear moderated mediation. Multivariate Behav Res 50: 1-22. https://doi.org/10.1080/00273171.2014.962683 ![]() |
[62] |
Sikaras C, Ilias I, Tselebis A, et al. (2021) Nursing staff fatigue and burnout during the COVID-19 pandemic in Greece. AIMS Public Health 9: 94-105. https://doi.org/10.3934/publichealth.2022008 ![]() |
[63] | Tziallas D, Goutzias E, Konstantinidou E, et al. (2018) Quantitative and qualitative assessment of nurse staffing indicators across NHS public hospitals in Greece. Hell J Nurs 57: 420-449. |
[64] |
Tselebis A, Gournas G, Tzitzanidou G, et al. (2006) Anxiety and depression in Greek nursing and medical personnel. Psychol Rep 99: 93-96. https://doi.org/10.2466/pr0.99.1.93-96 ![]() |
[65] |
Papathanasiou IV, Damigos D, Mavreas V (2011) Higher levels of psychiatric symptomatology reported by health professionals working in medical settings in Greece. Ann Gen Psychiatry 10: 28. https://doi.org/10.1186/1744-859X-10-28 ![]() |
[66] |
Cheung T, Yip PS (2015) Depression, anxiety and symptoms of stress among Hong Kong nurses: A cross-sectional study. Int J Environ Res Public Health 12: 11072-11100. https://doi.org/10.3390/ijerph120911072 ![]() |
[67] |
Scarpelli S, Alfonsi V, Mangiaruga A, et al. (2021) Pandemic nightmares: Effects on dream activity of the COVID-19 lockdown in Italy. J Sleep Res 30: e13300. https://doi.org/10.1111/jsr.13300 ![]() |
[68] |
Gorgoni M, Scarpelli S, Alfonsi V, et al. (2021) Pandemic dreams: Quantitative and qualitative features of the oneiric activity during the lockdown due to COVID-19 in Italy. Sleep Med 81: 20-32. https://doi.org/10.1016/j.sleep.2021.02.006 ![]() |
[69] |
Kilius E, Abbas NH, McKinnon L, et al. (2021) Pandemic nightmares: COVID-19 lockdown associated with increased aggression in female university students' dreams. Front Psychol 12: 644636. https://doi.org/10.3389/fpsyg.2021.644636 ![]() |
[70] |
Scarpelli S, Gorgoni M, Alfonsi V, et al. (2022) The impact of the end of COVID confinement on pandemic dreams, as assessed by a weekly sleep diary: A longitudinal investigation in Italy. J Sleep Res 31: e13429. https://doi.org/10.1111/jsr.13429 ![]() |
[71] |
Picchioni D, Goeltzenleucher B, Green DN, et al. (2002) Nightmares as a coping mechanism for Stress. Dreaming 12: 155-169. https://doi.org/10.1023/A:1020118425588 ![]() |
[72] |
Nielsen T, Levin R (2007) Nightmares: A new neurocognitive model. Sleep Med Rev 11: 295-310. https://doi.org/10.1016/j.smrv.2007.03.004 ![]() |
[73] |
Tselebis A, Zoumakis E, Ilias I (2021) Dream recall/affect and the hypothalamic-pituitary-adrenal axis. Clocks Sleep 3: 403-408. https://doi.org/10.3390/clockssleep3030027 ![]() |
[74] |
Woodward S H, Stegman W K, Pavao J R, et al. (2007) Self-selection bias in sleep and psychophysiological studies of posttraumatic stress disorder. J Trauma Stress: Off Publication Int Soc Trauma Stress Stud 20: 619-623. ![]() |
[75] |
Schredl M (2003) Effects of state and trait factors on nightmare frequency. Eur Arch Psychiatry Clin Neurosci 253: 241-247. https://doi.org/10.1007/s00406-003-0438-1 ![]() |
[76] |
Carr M, Nielsen T (2017) A novel differential susceptibility framework for the study of nightmares: Evidence for trait sensory processing sensitivity. Clin Psychol Rev 58: 86-96. https://doi.org/10.1016/j.cpr.2017.10.002 ![]() |
[77] |
Schredl M, Gilles M, Wolf I, et al. (2019) Nightmares and stress: A longitudinal study. J Clin Sleep Med 15: 1209-1215. https://doi.org/10.5664/jcsm.7904 ![]() |
[78] |
Gieselmann A, Ait Aoudia M, Carr M, et al. (2019) Aetiology and treatment of nightmare disorder: State of the art and future perspectives. J Sleep Res 28: e12820. https://doi.org/10.1111/jsr.12820 ![]() |
[79] |
Musse FCC, Castro LdS, Sousa KMM, et al. (2020) Mental violence: The COVID-19 nightmare. Front Psychiatry 11: 579289. https://doi.org/10.3389/fpsyt.2020.579289 ![]() |
[80] |
Riemann D, Spiegelhalder K, Feige B, et al. (2010) The hyperarousal model of insomnia: A review of the concept and its evidence. Sleep Med Rev 14: 19-31. https://doi.org/10.1016/j.smrv.2009.04.002 ![]() |
[81] |
Feige B, Al-Shajlawi A, Nissen C, et al. (2008) Does REM sleep con-tribute to subjective wake time in primary insomnia? A com-parison of polysomnographic and subjective sleep in 100 patients. J Sleep Res 17: 180-190. https://doi. org/10.1111/j.1365-2869.2008.00651.x ![]() |
[82] |
Feige B, Baglioni C, Spiegelhalder K, et al. (2013) The microstructure of sleep in primary insomnia: An overview and extension. Int J Psychophysiol 89: 171-180. https://doi.org/10.1016/j.ijpsycho.2013.04.002 ![]() |
[83] |
Perlis M L, Giles D E, Mendelson W B, et al. (1997) Psychophysiological insomnia: The behavioural model and a neurocognitive perspective. J Sleep Res 6: 179-188. https://doi.org/10.1046/j.1365-2869.1997.00045.x ![]() |
[84] | van Liempt S (2012) Sleep disturbances and PTSD: A perpetual circle?. Eur J Psychotraumato 2012: 3. https://doi.org/10.3402/ejpt.v3i0.19142 |
[85] |
Barzilay R, Moore T, Greenberg D, et al. (2020) Resilience, COVID-19-related stress, anxiety and depression during the pandemic in a large population enriched for healthcare providers. Transl Psychiat 10: 1-8. https://doi.org/10.1038/s41398-020-00982-4 ![]() |
[86] |
Baskin RG, Bartlett R (2021) Healthcare worker resilience during the COVID-19 pandemic: An integrative review. J Nurs Manag 29: 2329-2342. https://doi.org/10.1111/jonm.13395 ![]() |
[87] |
Labrague LJ (2021) Pandemic fatigue and clinical nurses' mental health, sleep quality and job contentment during the covid-19 pandemic: The mediating role of resilience. J Nurs Manag 29: 1992-2001. https://doi.org/10.1111/jonm.13383 ![]() |
[88] |
Pachi A, Tselebis A, Ilias I, et al. (2022) Aggression, alexithymia and sense of coherence in a sample of schizophrenic outpatients. In Healthcare 10: 1078. https://doi.org/10.3390/healthcare10061078 ![]() |
[89] |
Tselebis A, Moulou A, Ilias I (2001) Burnout versus depression and sense of coherence: Study of Greek nursing staff. Nurs Health Sci 3: 69-71. https://doi.org/10.1046/j.1442-2018.2001.00074.x ![]() |
[90] |
Tselebis A, Anagnostopoulou T, Bratis D, et al. (2011) The 13 item family support scale: Reliability and validity of the Greek translation in a sample of Greek health care professionals. Asia Pacific Family Med 10: 1-4. https://doi.org/10.1186/1447-056X-10-3 ![]() |
[91] | Tselebis A, Bratis D, Pachi A, et al. (2013) Chronic obstructive pulmonary disease: Sense of coherence and family support versus anxiety and depression. Psychiatriki 24: 109-116. |
[92] |
Ungar M (2008) Resilience across cultures. Brit J Soc Work 38: 218-235. https://doi.org/10.1093/bjsw/bcl343 ![]() |
[93] |
Kuldas S, Foody M (2022) Neither resiliency-trait nor resilience-state: Transactional Resiliency/e. Youth Soc 54: 1352-1376. https://doi.org/10.1177/0044118X211029309 ![]() |
[94] | Pollock A, Campbell P, Cheyne J, et al. (2020) Interventions to support the resilience and mental health of frontline health and social care professionals during and after a disease outbreak, epidemic or pandemic: A mixed methods systematic review. Cochrane Database Syst Rev 11: CD013779. https://doi.org/10.1002/14651858.CD013779 |
[95] | McCallum L (2022) Supporting resilience and well-being in health and social care professionals during pandemics. Evid Based Nurs 25: 104. https://doi.org/10.1136/ebnurs-2020-103382 |
[96] |
Zeng LN, Zong QQ, Yang Y, et al. (2020) Gender difference in the prevalence of insomnia: A meta-analysis of observational studies. Front Psychiatry 11: 577429. https://doi.org/10.3389/fpsyt.2020.577429 ![]() |
[97] |
La YK, Choi YH, Chu MK, et al. (2020) Gender differences influence over insomnia in Korean population: A cross-sectional study. PLoS One 15: e0227190. https://doi.org/10.1371/journal.pone.0227190 ![]() |
[98] |
Mong JA, Cusmano DM (2016) Gender differences in sleep: Impact of biological gender and gender steroids. Phil Trans R Soc B 371: 20150110. https://doi.org/10.1098/rstb.2015.0110 ![]() |
[99] |
Moline ML, Broch L, Zak R (2004) Sleep in women across the life cycle from adulthood through menopause. Med Clin N Am 88: 705-36. https://doi.org/10.1016/j.mcna.2004.01.009 ![]() |
[100] |
Polo Kantola P, Erkkola R, Helenius H, et al. (1998) When does estrogen replacement therapy improve sleep quality?. Am J Obst Gynec 178: 1002-1009. https://doi.org/10.1016/S0002-9378(98)70539-3 ![]() |
[101] |
Schredl M, Reinhard I (2001) Gender differences in nightmare frequency: A meta-analysis. Sleep Med Rev 15: 115-121. https://doi.org/10.1016/j.smrv.2010.06.002 ![]() |
[102] |
Schredl M (2014) Explaining the gender difference in nightmare frequency. Am J Psychol 127: 205-213. https://doi.org/10.5406/amerjpsyc.127.2.0205 ![]() |
[103] | Cappadona R, De Giorgi A, Di Simone E, et al. (2021) Sleep, dreams, nightmares, and sex-related differences: A narrative review. Eur Rev Med Pharmacol Sci 25: 3054-3065. https://doi.org/10.26355/eurrev_202104_25559 |
[104] |
Resuehr D, Wu G, Johnson RL, et al. (2019) Shift work disrupts circadian regulation of the transcriptome in hospital nurses. J Biol Rhythms 34: 167-177. https://doi.org/10.1177/0748730419826694 ![]() |
[105] |
Sardella A, Lenzo V, Basile G, et al. (2022) Gender and psychosocial differences in psychological resilience among a community of older adults during the COVID-19 pandemic. J Pers Med 12: 1414. Https://doi.org/10.3390/jpm12091414 ![]() |
[106] |
Peyer KL, Hathaway ED, Doyle K (2022) Gender differences in stress, resilience, and physical activity during the COVID-19 pandemic. J Am Coll Health 24: 1-8. https://doi.org/10.1080/07448481.2022.2052075 ![]() |
[107] |
Zhang M, Zhang J, Zhang F, et al. (2018) Prevalence of psychological distress and the effects of resilience and perceived social support among Chinese college students: Does gender make a difference?. Psychiatry Res 267: 409-413. https://doi.org/10.1016/j.psychres.2018.06.038 ![]() |
[108] |
Croghan IT, Chesak SS, Adusumalli J, et al. (2021) Stress, resilience, and coping of healthcare workers during the COVID-19 pandemic. J Prim Care Community Health 12: 21501327211008448. https://doi.org/10.1177/21501327211008448 ![]() |
[109] |
Alessandri G, Eisenberg N, Vecchione M, et al. (2016) Ego-resiliency development from late adolescence to emerging adulthood: A ten-year longitudinal study. J Adolesc 50: 91-102. https://doi.org/10.1016/j.adolescence.2016.05.004 ![]() |
[110] |
Gooding PA, Hurst A, Johnson J, et al. (2012) Psychological resilience in young and older adults. Int J Geriatr Psychiatry 27: 262-270. https://doi.org/10.1002/gps.2712 ![]() |
[111] |
Tselebis A, Bratis D, Karkanias A, et al. (2008) Associations on dimensions of burnout and family support for a sample of Greek nurses. Psychol Rep 103: 63-66. https://doi.org/10.2466/pr0.103.1.63-66 ![]() |
[112] |
Suran M (2023) Overworked and Understaffed, More Than 1 in 4 US Nurses Say They Plan to Leave the Profession. JAMA 330: 1512-1514. https://doi.org/10.1001/jama.2023.10055 ![]() |
[113] |
Christianson J, Johnson N, Nelson A, et al. (2023) Work-related burnout, compassion fatigue, and nurse intention to leave the profession during COVID-19. Nurse Leader 21: 244-251. https://doi.org/10.1016/j.mnl.2022.06.007 ![]() |
[114] | Chen Y, Zhang Y, Jin R (2020) Professional identity of male nursing students in 3-year colleges and junior male nurses in China. Am J Men's Health 14: 1557988320936583. https://doi.org/10.1177/1557988320936583 |
[115] |
Kim S O, Moon S H (2021) Factors infuencing turnover intention among male nurses in Korea. Int J Environ Re Public Health 18: 9862. https://doi.org/10.3390/ijerph18189862 ![]() |
[116] |
Nadorff MR, Nadorff DK, Germain A (2015) Nightmares: under-reported, undetected, and therefore untreated. J Clin Sleep Med 11: 747-750. https://doi.org/10.5664/jcsm.4850 ![]() |
[117] | Ayşe GÖK, Koğar EY (2021) A meta-analysis study on gender differences in psychological resilience levels. Cyprus Turk J Psychiatry Psychol 3: 132-143. |
[118] |
Boardman JD, Blalock CL, Button TM (2008) Sex differences in the heritability of resilience. Twin Res Hum Genet 11: 12-27. https://doi.org/10.1375/twin.11.1.12 ![]() |
[119] |
Hirani S, Lasiuk G, Hegadoren K (2016) The intersection of gender and resilience. J Psychiatr Ment Health Nurs 23: 455-467. https://doi.org/10.1111/jpm.12313 ![]() |
![]() |
![]() |
1. | K. Balakrishnan, R. Dhanalakshmi, M. Akila, Bam Bahadur Sinha, Improved equilibrium optimization based on Levy flight approach for feature selection, 2022, 1868-6478, 10.1007/s12530-022-09461-1 | |
2. | Krishna Gopal Dhal, Swarnajit Ray, Rebika Rai, Arunita Das, Archimedes Optimizer: Theory, Analysis, Improvements, and Applications, 2023, 1134-3060, 10.1007/s11831-022-09876-8 | |
3. | Jie Xing, Xinsen Zhou, Hanli Zhao, Huiling Chen, Ali Asghar Heidari, Elite levy spreading differential evolution via ABC shrink-wrap for multi-threshold segmentation of breast cancer images, 2023, 82, 17468094, 104592, 10.1016/j.bspc.2023.104592 | |
4. | Yudong Zhang, Juan Manuel Gorriz, Deepak Ranjan Nayak, Optimization Algorithms and Machine Learning Techniques in Medical Image Analysis, 2023, 20, 1551-0018, 5917, 10.3934/mbe.2023255 | |
5. | Rebika Rai, Krishna Gopal Dhal, Recent Developments in Equilibrium Optimizer Algorithm: Its Variants and Applications, 2023, 1134-3060, 10.1007/s11831-023-09923-y | |
6. | Heming Jia, Honghua Rao, Changsheng Wen, Seyedali Mirjalili, Crayfish optimization algorithm, 2023, 56, 0269-2821, 1919, 10.1007/s10462-023-10567-4 | |
7. | Leena Samantaray, Tanmaya Tapaswini Tripathy, Rutuparna Panda, A novel 3D differential entropy-based multi-class segmentation technique for brain MR image analysis, 2025, 100, 17468094, 107064, 10.1016/j.bspc.2024.107064 | |
8. | M. A. JiMing, Duan HongYu, Wang YuFan, Wang LiNa, Khan Bahadar Khan, Medical image segmentation based on simulated annealing and opposition-based learning island algorithm, 2024, 19, 1932-6203, e0307278, 10.1371/journal.pone.0307278 | |
9. | Mohammed Azmi Al-Betar, Iyad Abu Doush, Sharif Naser Makhadmeh, Ghazi Al-Naymat, Osama Ahmad Alomari, Mohammed A. Awadallah, Equilibrium optimizer: a comprehensive survey, 2023, 83, 1573-7721, 29617, 10.1007/s11042-023-16764-1 | |
10. | Huy-Du Dao, Thanh-Tung Nguyen, Ngoc-Kien Vu, Tuan-Linh Nguyen, 2024, Chapter 32, 978-3-031-62237-3, 284, 10.1007/978-3-031-62238-0_32 | |
11. | Huiying Jia, Design of fruit fly optimization algorithm based on Gaussian distribution and its application to image processing, 2024, 6, 27729419, 200090, 10.1016/j.sasc.2024.200090 |
Algorithm 1 Pseudo-code of HEOA algorithm |
Input: The color image Output: Segmentation color image Read the input and compute the histogram of three channels of color imageInitialize the parameters r1andr2 Initialize the random population Ci while L < Max_iter do Calculate the fitness values of EOA for (each hawk Ci) do Calculate Ceq, Xi Update the C using Eq. (25) Calculate the fitness function using Eq.(10) end for UpdateCbestif there is a better solution L=L+1 end while Get the best solution as the multilevel threshold K According the K segment the three channels of the images Get the segmentation images |
No. | Functions | Related basic functions | Dim | fmin |
CEC-1 | Rotated Bent Cigar Function | Bent Cigar Function | 30 | 100 |
CEC-2 | Rotated Discus Function | Discus Function | 30 | 200 |
CEC-3 | Shifted and Rotated Weierstrass Function | Weierstrass Function | 30 | 300 |
CEC-4 | Shifted and Rotated Schwefel's Function | Schwefel's Function | 30 | 400 |
CEC-5 | Shifted and Rotated Katsuura Function | Katsuura Function | 30 | 500 |
CEC-6 | Shifted and Rotated HappyCat Function | HappyCat Function | 30 | 600 |
CEC-7 | Shifted and Rotated HGBat Function | HGBat Function | 30 | 700 |
CEC-8 | Shifted and Rotated Expanded Griewank's plus Rosenbrock's Function | Griewank's Function Rosenbrock's Function | 30 | 800 |
CEC-9 | Shifted and Rotated Expanded Scaer's F6 Function | Expanded Scaer's F6 Function | 30 | 900 |
CEC-10 | Hybrid Function 1 (N = 3) | Schwefel's FunctionRastrigin's FunctionHigh Conditioned Elliptic Function | 30 | 1000 |
CEC-11 | Hybrid Function 2 (N = 4) | Griewank's Function Weierstrass Function Rosenbrock's Function Scaer's F6 Function | 30 | 1100 |
CEC-12 | Hybrid Function 3 (N = 5) | Katsuura FunctionHappyCat FunctionExpanded Griewank's plus Rosenbrock's Function Schwefel's FunctionAckley's Function | 30 | 1200 |
CEC-13 | Composition Function 1 (N = 5) | Rosenbrock's FunctionHigh Conditioned Elliptic FunctionBent Cigar FunctionDiscus FunctionHigh Conditioned Elliptic Function | 30 | 1300 |
CEC-14 | Composition Function 2 (N = 3) | Schwefel's FunctionRastrigin's FunctionHigh Conditioned Elliptic Function | 30 | 1400 |
CEC-15 | Composition Function 3 (N = 5) | HGBat FunctionRastrigin's FunctionSchwefel's FunctionWeierstrass FunctionHigh Conditioned Elliptic Function | 30 | 1500 |
Func. | HEOA | CSA | PSO | FPA | BA | EOA | ||||||
Mean | Std. | Mean | Std. | Mean | Std. | Mean | Std. | Mean | Std. | Mean | Std. | |
CEC-1 | 1.05E+05 | 1.54E+07 | 4.21E+05 | 1.47E+08 | 2.17E+09 | 3.53E+07 | 1.76E+08 | 7.02E+07 | 4.76E+08 | 2.86E+08 | 1.07E+05 | 3.69E+07 |
CEC-2 | 6.70E+06 | 1.70E+09 | 2.06E+04 | 5.26E+09 | 7.01E+10 | 3.53E+09 | 3.04E+10 | 4.70E+09 | 3.36E+10 | 6.82E+09 | 6.70E+07 | 4.05E+09 |
CEC-3 | 3.20E+02 | 7.12E-02 | 3.20E+02 | 1.51E-01 | 3.22E+02 | 9.57E-02 | 3.21E+02 | 1.43E-01 | 3.21E+02 | 2.10E-01 | 3.55E+02 | 1.47E-01 |
CEC-4 | 4.10E+02 | 1.75E+00 | 4.05E+02 | 9.03E+00 | 5.46E+02 | 1.29E+01 | 5.30E+02 | 1.61E+01 | 5.21E+02 | 9.70E+00 | 4.50E+02 | 2.39E+01 |
CEC-5 | 9.81E+02 | 1.50E+02 | 1.22E+03 | 3.30E+02 | 4.74E+03 | 2.40E+02 | 3.80E+03 | 3.97E+02 | 3.65E+03 | 3.52E+02 | 9.91E+02 | 2.97E+02 |
CEC-6 | 2.05E+03 | 4.75E+06 | 2.14E+03 | 2.33E+07 | 3.79E+09 | 9.03E+06 | 4.52E+09 | 1.14E+07 | 1.28E+08 | 2.71E+07 | 2.10E+03 | 1.37E+07 |
CEC-7 | 7.02E+02 | 1.24E+01 | 7.03E+02 | 4.37E+01 | 1.81E+03 | 1.40E+01 | 1.78E+03 | 2.70E+01 | 1.78E+03 | 4.99E+01 | 8.82E+02 | 2.49E+01 |
CEC-8 | 1.47E+03 | 1.03E+06 | 1.41E+04 | 3.25E+06 | 2.21E+09 | 1.05E+06 | 1.34E+09 | 1.63E+06 | 1.34E+09 | 3.78E+06 | 1.47E+04 | 1.08E+06 |
CEC-9 | 1.00E+03 | 7.30E+00 | 1.00E+03 | 4.77E+01 | 1.33E+03 | 2.45E+01 | 1.33E+03 | 3.46E+01 | 1.62E+03 | 5.15E+01 | 1.00E+04 | 3.38E+01 |
CEC-10 | 1.23E+03 | 4.82E+04 | 2.05E+03 | 3.09E+06 | 2.58E+09 | 4.14E+05 | 2.97E+07 | 8.13E+05 | 4.41E+08 | 4.49E+06 | 1.36E+04 | 7.89E+05 |
CEC-11 | 1.35E+03 | 4.52E+01 | 1.40E+03 | 7.94E+01 | 2.00E+03 | 6.39E+01 | 1.77E+03 | 1.02E+02 | 1.77E+03 | 9.80E+01 | 1.32E+04 | 1.24E+02 |
CEC-12 | 1.30E+03 | 1.03E+01 | 1.30E+03 | 1.75E+01 | 1.76E+03 | 1.22E+01 | 1.49E+03 | 1.28E+01 | 1.51E+03 | 3.25E+01 | 1.34E+05 | 1.58E+01 |
CEC-13 | 1.30E+03 | 8.67E+00 | 1.34E+03 | 1.49E+00 | 5.44E+05 | 1.86E+01 | 1.54E+03 | 3.03E+01 | 1.56E+03 | 2.48E+00 | 1.55E+05 | 3.24E+01 |
CEC-14 | 3.22E+03 | 2.32E+03 | 8.88E+03 | 4.24E+03 | 2.23E+04 | 2.92E+03 | 2.23E+04 | 4.76E+03 | 2.23E+04 | 5.46E+03 | 3.22E+04 | 3.59E+03 |
CEC-15 | 1.60E+03 | 3.36E+02 | 1.60E+03 | 6.49E+02 | 1.28E+04 | 7.10E+02 | 9.01E+03 | 1.34E+03 | 4.09E+03 | 1.05E+03 | 1.80E+03 | 1.27E+03 |
No. | Measures | Formulation | Reference |
1 | Uniformity measure | U=1−2×(k−1)×∑dj=1∑i∈Rj(fi−μj)2N×(fmax−fmin)2 | [54] |
2 | Peak Signal-to-Noise Ratio (PSNR) | PSNR=10×log10(2552MSE) MSE=1mnm−1∑i=1n−1∑j=1[I(i,j)−K(i,j)]2 |
[55] |
3 | Feature Similarity Index (FSIM) | FSIM=∑x=ηSL(x)⋅PCm(x)∑x=ηPCm(x) | [56] |
Image | K | EOA-Otsu | EOA-3DOtsu | HEOA-Otsu | HEOA-3DOtsu |
Test1 | 4 | 0.8628 | 0.8886 | 0.8973 | 0.9106 |
8 | 0.8657 | 0.8864 | 0.8957 | 0.9170 | |
12 | 0.9103 | 0.9332 | 0.9411 | 0.9580 | |
15 | 0.9293 | 0.9479 | 0.9565 | 0.9695 | |
Test2 | 4 | 0.8644 | 0.8799 | 0.8901 | 0.9015 |
8 | 0.8459 | 0.8674 | 0.8736 | 0.9135 | |
12 | 0.9298 | 0.9511 | 0.9569 | 0.9591 | |
15 | 0.9287 | 0.9474 | 0.9562 | 0.9671 | |
Test3 | 4 | 0.8586 | 0.8833 | 0.8919 | 0.9056 |
8 | 0.8599 | 0.8766 | 0.8859 | 0.9246 | |
12 | 0.9207 | 0.9370 | 0.9466 | 0.9661 | |
15 | 0.9049 | 0.9239 | 0.9358 | 0.9688 | |
Test4 | 4 | 0.8518 | 0.8660 | 0.8805 | 0.8963 |
8 | 0.8856 | 0.9060 | 0.9125 | 0.9221 | |
12 | 0.8823 | 0.9034 | 0.9143 | 0.9619 | |
15 | 0.9012 | 0.9260 | 0.9343 | 0.9742 | |
Test5 | 4 | 0.8379 | 0.8545 | 0.8645 | 0.8988 |
8 | 0.8648 | 0.8854 | 0.8992 | 0.9193 | |
12 | 0.9166 | 0.9305 | 0.9446 | 0.9659 | |
15 | 0.9055 | 0.9286 | 0.9345 | 0.9750 | |
Test6 | 4 | 0.8725 | 0.8902 | 0.9025 | 0.9157 |
8 | 0.8854 | 0.9040 | 0.9160 | 0.9189 | |
12 | 0.9267 | 0.9484 | 0.9566 | 0.9585 | |
15 | 0.8904 | 0.9095 | 0.9214 | 0.9663 | |
Test7 | 4 | 0.8708 | 0.8883 | 0.9009 | 0.9147 |
8 | 0.8845 | 0.9037 | 0.9155 | 0.9171 | |
12 | 0.9250 | 0.9478 | 0.9555 | 0.9581 | |
15 | 0.8898 | 0.9087 | 0.9202 | 0.9648 | |
Test8 | 4 | 0.8704 | 0.8871 | 0.9000 | 0.9143 |
8 | 0.8830 | 0.9017 | 0.9142 | 0.9155 | |
12 | 0.9232 | 0.9469 | 0.9535 | 0.9578 | |
15 | 0.8886 | 0.9079 | 0.9201 | 0.9629 | |
Test9 | 4 | 0.8716 | 0.8884 | 0.9011 | 0.9151 |
8 | 0.8844 | 0.9026 | 0.9157 | 0.9163 | |
12 | 0.9237 | 0.9470 | 0.9552 | 0.9596 | |
15 | 0.8897 | 0.9082 | 0.9217 | 0.9633 | |
Test10 | 4 | 0.8720 | 0.8896 | 0.9024 | 0.9171 |
8 | 0.8863 | 0.9027 | 0.9162 | 0.9179 | |
12 | 0.9247 | 0.9482 | 0.9561 | 0.9607 | |
15 | 0.8915 | 0.9089 | 0.9223 | 0.9645 |
Image | K | HEOA | HSOA | HWOA | CSA | FPA | PSO | BA |
Test1 | 4 | 0.9571 | 0.9415 | 0.9437 | 0.9245 | 0.9284 | 0.9262 | 0.9243 |
8 | 0.9674 | 0.9525 | 0.9594 | 0.9299 | 0.9309 | 0.9392 | 0.9373 | |
12 | 0.9744 | 0.9641 | 0.9654 | 0.9404 | 0.9456 | 0.9424 | 0.9457 | |
15 | 0.9795 | 0.9684 | 0.9617 | 0.9428 | 0.9441 | 0.9445 | 0.9458 | |
Test2 | 4 | 0.9544 | 0.9460 | 0.9460 | 0.9224 | 0.9167 | 0.9207 | 0.9216 |
8 | 0.9722 | 0.9583 | 0.9624 | 0.9442 | 0.9422 | 0.9425 | 0.9356 | |
12 | 0.9813 | 0.9717 | 0.9639 | 0.9437 | 0.9458 | 0.9526 | 0.9497 | |
15 | 0.9824 | 0.9698 | 0.9697 | 0.9499 | 0.9479 | 0.9468 | 0.9515 | |
Test3 | 4 | 0.9528 | 0.9437 | 0.9427 | 0.9244 | 0.9184 | 0.9184 | 0.9165 |
8 | 0.9626 | 0.9500 | 0.9482 | 0.9255 | 0.9274 | 0.9323 | 0.9290 | |
12 | 0.9782 | 0.9657 | 0.9625 | 0.9464 | 0.9453 | 0.9486 | 0.9406 | |
15 | 0.9812 | 0.9701 | 0.9683 | 0.9467 | 0.9450 | 0.9487 | 0.9513 | |
Test4 | 4 | 0.9518 | 0.9403 | 0.9435 | 0.9201 | 0.9171 | 0.9161 | 0.9187 |
8 | 0.9699 | 0.9589 | 0.9520 | 0.9327 | 0.9350 | 0.9331 | 0.9402 | |
12 | 0.9749 | 0.9623 | 0.9653 | 0.9385 | 0.9398 | 0.9409 | 0.9439 | |
15 | 0.9806 | 0.9717 | 0.9700 | 0.9486 | 0.9490 | 0.9457 | 0.9485 | |
Test5 | 4 | 0.9541 | 0.9410 | 0.9402 | 0.9257 | 0.9167 | 0.9206 | 0.9207 |
8 | 0.9679 | 0.9558 | 0.9560 | 0.9385 | 0.9306 | 0.9399 | 0.9392 | |
12 | 0.9769 | 0.9670 | 0.9618 | 0.9393 | 0.9441 | 0.9404 | 0.9455 | |
15 | 0.9808 | 0.9639 | 0.9678 | 0.9468 | 0.9508 | 0.9519 | 0.9467 | |
Test6 | 4 | 0.9507 | 0.9393 | 0.9373 | 0.9169 | 0.9197 | 0.9138 | 0.9154 |
8 | 0.9666 | 0.9504 | 0.9512 | 0.9383 | 0.9298 | 0.9355 | 0.9352 | |
12 | 0.9787 | 0.9644 | 0.9673 | 0.9492 | 0.9505 | 0.9474 | 0.9431 | |
15 | 0.9824 | 0.9713 | 0.9667 | 0.9470 | 0.9480 | 0.9498 | 0.9472 | |
Test7 | 4 | 0.9514 | 0.9397 | 0.9378 | 0.9183 | 0.9212 | 0.9150 | 0.9167 |
8 | 0.9667 | 0.9524 | 0.9529 | 0.9392 | 0.9300 | 0.9360 | 0.9366 | |
12 | 0.9802 | 0.9648 | 0.9675 | 0.9510 | 0.9521 | 0.9479 | 0.9433 | |
15 | 0.9841 | 0.9725 | 0.9680 | 0.9473 | 0.9483 | 0.9502 | 0.9487 | |
Test8 | 4 | 0.9508 | 0.9377 | 0.9365 | 0.9165 | 0.9196 | 0.9137 | 0.9161 |
8 | 0.9649 | 0.9522 | 0.9520 | 0.9383 | 0.9284 | 0.9349 | 0.9355 | |
12 | 0.9789 | 0.9642 | 0.9663 | 0.9507 | 0.9507 | 0.9479 | 0.9417 | |
15 | 0.9837 | 0.9715 | 0.9670 | 0.9468 | 0.9464 | 0.9497 | 0.9479 | |
Test9 | 4 | 0.9503 | 0.9374 | 0.9355 | 0.9158 | 0.9191 | 0.9118 | 0.9151 |
8 | 0.9633 | 0.9510 | 0.9500 | 0.9363 | 0.9272 | 0.9346 | 0.9351 | |
12 | 0.9772 | 0.9634 | 0.9655 | 0.9497 | 0.9488 | 0.9468 | 0.9402 | |
15 | 0.9820 | 0.9706 | 0.9655 | 0.9462 | 0.9455 | 0.9491 | 0.9460 | |
Test10 | 4 | 0.9505 | 0.9382 | 0.9375 | 0.9171 | 0.9207 | 0.9118 | 0.9167 |
8 | 0.9637 | 0.9519 | 0.9510 | 0.9367 | 0.9277 | 0.9360 | 0.9364 | |
12 | 0.9776 | 0.9653 | 0.9661 | 0.9511 | 0.9493 | 0.9472 | 0.9413 | |
15 | 0.9838 | 0.9710 | 0.9655 | 0.9481 | 0.9464 | 0.9504 | 0.9479 |
Image | K | HEOA | HSOA | HWOA | CSA | FPA | PSO | BA |
Test1 | 4 | 23.3863 | 23.2573 | 23.2526 | 23.0420 | 23.0405 | 23.0397 | 23.0539 |
8 | 29.2231 | 29.1025 | 29.0968 | 28.9021 | 28.9007 | 28.8989 | 28.9020 | |
12 | 32.5692 | 32.4393 | 32.4442 | 32.2300 | 32.2265 | 32.2282 | 32.2414 | |
15 | 33.3295 | 33.1947 | 33.1953 | 33.0002 | 32.9903 | 32.9903 | 32.9923 | |
Test2 | 4 | 24.4702 | 24.3443 | 24.3386 | 24.1472 | 24.1340 | 24.1342 | 24.1432 |
8 | 29.4260 | 29.3020 | 29.2936 | 29.0852 | 29.0987 | 29.0861 | 29.1020 | |
12 | 32.5786 | 32.4454 | 32.4470 | 32.2471 | 32.2464 | 32.2522 | 32.2424 | |
15 | 34.2153 | 34.0865 | 34.0884 | 33.8828 | 33.8824 | 33.8823 | 33.8887 | |
Test3 | 4 | 24.4769 | 24.3474 | 24.3453 | 24.1433 | 24.1406 | 24.1517 | 24.1495 |
8 | 29.9667 | 29.8287 | 29.8366 | 29.6330 | 29.6383 | 29.6298 | 29.6211 | |
12 | 32.6661 | 32.5412 | 32.5392 | 32.3369 | 32.3265 | 32.3288 | 32.3254 | |
15 | 34.7330 | 34.6031 | 34.6015 | 34.3994 | 34.3925 | 34.4084 | 34.3905 | |
Test4 | 4 | 24.7640 | 24.6386 | 24.6369 | 24.4284 | 24.4400 | 24.4423 | 24.4286 |
8 | 29.5467 | 29.4208 | 29.4154 | 29.2214 | 29.2193 | 29.2207 | 29.2104 | |
12 | 33.5823 | 33.4474 | 33.4548 | 33.2561 | 33.2435 | 33.2461 | 33.2509 | |
15 | 34.2159 | 34.0826 | 34.0820 | 33.8787 | 33.8740 | 33.8707 | 33.8853 | |
Test5 | 4 | 24.5319 | 24.3991 | 24.3994 | 24.2048 | 24.1918 | 24.1918 | 24.1924 |
8 | 29.5550 | 29.4198 | 29.4251 | 29.2137 | 29.2181 | 29.2237 | 29.2154 | |
12 | 34.3322 | 34.1972 | 34.1979 | 34.0010 | 33.9935 | 33.9870 | 33.9959 | |
15 | 35.0669 | 34.9409 | 34.9366 | 34.7387 | 34.7254 | 34.7407 | 34.7279 | |
Test6 | 4 | 25.2119 | 25.0871 | 25.0842 | 24.8695 | 24.8803 | 24.8734 | 24.8829 |
8 | 30.9087 | 30.7880 | 30.7821 | 30.5869 | 30.5821 | 30.5735 | 30.5700 | |
12 | 34.1912 | 34.0606 | 34.0591 | 33.8576 | 33.8505 | 33.8585 | 33.8626 | |
15 | 35.7843 | 35.6567 | 35.6607 | 35.4492 | 35.4505 | 35.4438 | 35.4423 | |
Test7 | 4 | 25.4053 | 25.1458 | 25.1450 | 24.8847 | 25.0748 | 24.9284 | 24.9402 |
8 | 31.0914 | 30.8883 | 30.8601 | 30.7788 | 30.5828 | 30.6162 | 30.6718 | |
12 | 34.3507 | 34.1142 | 34.1742 | 34.0164 | 33.9942 | 33.8766 | 33.8983 | |
15 | 35.9362 | 35.6969 | 35.7545 | 35.6007 | 35.4588 | 35.4937 | 35.6024 | |
Test8 | 4 | 25.5670 | 25.2034 | 25.2799 | 24.9384 | 25.1283 | 24.9787 | 25.0445 |
8 | 31.2698 | 31.0054 | 30.9921 | 30.8672 | 30.7368 | 30.6972 | 30.8372 | |
12 | 34.5283 | 34.2658 | 34.2137 | 34.2017 | 34.1031 | 33.9285 | 34.0632 | |
15 | 36.0846 | 35.7585 | 35.9118 | 35.6393 | 35.6021 | 35.4988 | 35.6352 | |
Test9 | 4 | 25.5622 | 25.0597 | 25.1265 | 24.7749 | 24.9583 | 24.9368 | 24.8720 |
8 | 31.1656 | 30.8841 | 30.8166 | 30.7155 | 30.6732 | 30.6568 | 30.8154 | |
12 | 34.4249 | 34.0884 | 34.1830 | 34.1183 | 33.9539 | 33.7553 | 33.9067 | |
15 | 36.0382 | 35.7553 | 35.7737 | 35.5952 | 35.5146 | 35.3593 | 35.5413 | |
Test10 | 4 | 25.4424 | 24.9891 | 25.1108 | 24.7329 | 24.7752 | 24.9286 | 24.8300 |
8 | 31.1315 | 30.7681 | 30.7427 | 30.5414 | 30.6599 | 30.5129 | 30.6245 | |
12 | 34.3961 | 33.9364 | 34.1416 | 33.9835 | 33.8675 | 33.7435 | 33.8129 | |
15 | 35.8866 | 35.7225 | 35.7457 | 35.4554 | 35.4165 | 35.2905 | 35.3448 |
Image | K | HEOA | HSOA | HWOA | CSA | FPA | PSO | BA |
Test1 | 4 | 0.9367 | 0.9236 | 0.9225 | 0.9094 | 0.9039 | 0.8944 | 0.9087 |
8 | 0.9517 | 0.9414 | 0.9390 | 0.9036 | 0.9077 | 0.9157 | 0.9084 | |
12 | 0.9587 | 0.9433 | 0.9473 | 0.9135 | 0.9149 | 0.9172 | 0.9135 | |
15 | 0.9668 | 0.9511 | 0.9540 | 0.9271 | 0.9331 | 0.9287 | 0.9284 | |
Test2 | 4 | 0.9356 | 0.9227 | 0.9262 | 0.9006 | 0.8943 | 0.8936 | 0.9065 |
8 | 0.9584 | 0.9422 | 0.9438 | 0.9221 | 0.9123 | 0.9122 | 0.9230 | |
12 | 0.9632 | 0.9548 | 0.9563 | 0.9283 | 0.9305 | 0.9331 | 0.9233 | |
15 | 0.9633 | 0.9553 | 0.9506 | 0.9242 | 0.9251 | 0.9255 | 0.9202 | |
Test3 | 4 | 0.9325 | 0.9197 | 0.9181 | 0.9003 | 0.9077 | 0.8898 | 0.9078 |
8 | 0.9477 | 0.9333 | 0.9362 | 0.9060 | 0.9062 | 0.9001 | 0.9048 | |
12 | 0.9599 | 0.9482 | 0.9483 | 0.9289 | 0.9289 | 0.9190 | 0.9162 | |
15 | 0.9607 | 0.9487 | 0.9468 | 0.9309 | 0.9251 | 0.9325 | 0.9358 | |
Test4 | 4 | 0.9385 | 0.9213 | 0.9207 | 0.9065 | 0.9063 | 0.8982 | 0.8954 |
8 | 0.9485 | 0.9362 | 0.9349 | 0.9057 | 0.9058 | 0.9115 | 0.9144 | |
12 | 0.9580 | 0.9487 | 0.9498 | 0.9240 | 0.9298 | 0.9154 | 0.9200 | |
15 | 0.9637 | 0.9457 | 0.9532 | 0.9229 | 0.9227 | 0.9321 | 0.9237 | |
Test5 | 4 | 0.9332 | 0.9213 | 0.9264 | 0.8894 | 0.9071 | 0.9082 | 0.8960 |
8 | 0.9469 | 0.9377 | 0.9361 | 0.9085 | 0.9141 | 0.9083 | 0.9106 | |
12 | 0.9642 | 0.9438 | 0.9503 | 0.9210 | 0.9254 | 0.9187 | 0.9270 | |
15 | 0.9687 | 0.9484 | 0.9489 | 0.9240 | 0.9174 | 0.9240 | 0.9267 | |
Test6 | 4 | 0.9304 | 0.9238 | 0.9186 | 0.8868 | 0.9055 | 0.8972 | 0.9054 |
8 | 0.9518 | 0.9317 | 0.9372 | 0.9027 | 0.9099 | 0.9117 | 0.9085 | |
12 | 0.9579 | 0.9492 | 0.9460 | 0.9280 | 0.9270 | 0.9258 | 0.9158 | |
15 | 0.9682 | 0.9517 | 0.9490 | 0.9289 | 0.9204 | 0.9315 | 0.9250 | |
Test7 | 4 | 0.9313 | 0.9252 | 0.9198 | 0.8882 | 0.9057 | 0.8984 | 0.9060 |
8 | 0.9536 | 0.9322 | 0.9384 | 0.9035 | 0.9109 | 0.9127 | 0.9087 | |
12 | 0.9590 | 0.9498 | 0.9477 | 0.9287 | 0.9280 | 0.9273 | 0.9177 | |
15 | 0.9699 | 0.9524 | 0.9510 | 0.9292 | 0.9222 | 0.9335 | 0.9258 | |
Test8 | 4 | 0.9294 | 0.9239 | 0.9181 | 0.8877 | 0.9044 | 0.8970 | 0.9058 |
8 | 0.9518 | 0.9309 | 0.9381 | 0.9029 | 0.9096 | 0.9116 | 0.9070 | |
12 | 0.9573 | 0.9487 | 0.9470 | 0.9276 | 0.9263 | 0.9263 | 0.9175 | |
15 | 0.9682 | 0.9513 | 0.9494 | 0.9280 | 0.9216 | 0.9324 | 0.9240 | |
Test9 | 4 | 0.9292 | 0.9234 | 0.9161 | 0.8866 | 0.9038 | 0.8956 | 0.9052 |
8 | 0.9506 | 0.9296 | 0.9368 | 0.9010 | 0.9089 | 0.9096 | 0.9064 | |
12 | 0.9569 | 0.9469 | 0.9470 | 0.9273 | 0.9250 | 0.9262 | 0.9160 | |
15 | 0.9676 | 0.9501 | 0.9474 | 0.9275 | 0.9216 | 0.9308 | 0.9236 | |
Test10 | 4 | 0.9296 | 0.9246 | 0.9170 | 0.8874 | 0.9046 | 0.8962 | 0.9057 |
8 | 0.9509 | 0.9306 | 0.9378 | 0.9025 | 0.9093 | 0.9098 | 0.9069 | |
12 | 0.9589 | 0.9483 | 0.9490 | 0.9275 | 0.9261 | 0.9277 | 0.9165 | |
15 | 0.9678 | 0.9501 | 0.9479 | 0.9287 | 0.9232 | 0.9316 | 0.9240 |
Image | K | HEOA | HSOA | HWOA | CSA | FPA | PSO | BA |
Test1 | 4 | 1.2145 | 1.2251 | 1.2247 | 1.2442 | 1.2405 | 1.2406 | 1.2389 |
8 | 1.3154 | 1.3254 | 1.3260 | 1.3452 | 1.3422 | 1.3386 | 1.3385 | |
12 | 1.5172 | 1.5274 | 1.5279 | 1.5448 | 1.5467 | 1.5451 | 1.5466 | |
15 | 1.8157 | 1.8260 | 1.8258 | 1.8359 | 1.8435 | 1.8376 | 1.8439 | |
Test2 | 4 | 1.2149 | 1.2259 | 1.2255 | 1.2363 | 1.2392 | 1.2436 | 1.2365 |
8 | 1.3154 | 1.3262 | 1.3263 | 1.3388 | 1.3411 | 1.3401 | 1.3371 | |
12 | 1.5181 | 1.5286 | 1.5288 | 1.5423 | 1.5449 | 1.5466 | 1.5389 | |
15 | 1.8158 | 1.8266 | 1.8266 | 1.8419 | 1.8398 | 1.8409 | 1.8424 | |
Test3 | 4 | 1.2151 | 1.2257 | 1.2258 | 1.2354 | 1.2367 | 1.2445 | 1.2367 |
8 | 1.3158 | 1.3268 | 1.3265 | 1.3402 | 1.3411 | 1.3456 | 1.3436 | |
12 | 1.5191 | 1.5292 | 1.5292 | 1.5394 | 1.5406 | 1.5474 | 1.5487 | |
15 | 1.8164 | 1.8270 | 1.8273 | 1.8462 | 1.8369 | 1.8437 | 1.8413 | |
Test4 | 4 | 1.2151 | 1.2254 | 1.2253 | 1.2444 | 1.2358 | 1.2358 | 1.2409 |
8 | 1.3166 | 1.3268 | 1.3271 | 1.3432 | 1.3398 | 1.3458 | 1.3461 | |
12 | 1.5192 | 1.5299 | 1.5301 | 1.5421 | 1.5476 | 1.5483 | 1.5465 | |
15 | 1.8166 | 1.8275 | 1.8269 | 1.8390 | 1.8466 | 1.8422 | 1.8425 | |
Test5 | 4 | 1.2161 | 1.2269 | 1.2269 | 1.2388 | 1.2447 | 1.2368 | 1.2401 |
8 | 1.3167 | 1.3273 | 1.3273 | 1.3456 | 1.3396 | 1.3382 | 1.3431 | |
12 | 1.5201 | 1.5304 | 1.5302 | 1.5471 | 1.5428 | 1.5454 | 1.5401 | |
15 | 1.8169 | 1.8274 | 1.8278 | 1.8431 | 1.8379 | 1.8456 | 1.8405 | |
Test6 | 4 | 1.2168 | 1.2271 | 1.2268 | 1.2372 | 1.2413 | 1.2412 | 1.2383 |
8 | 1.3172 | 1.3281 | 1.3273 | 1.3412 | 1.3407 | 1.3448 | 1.3379 | |
12 | 1.5206 | 1.5307 | 1.5313 | 1.5491 | 1.5408 | 1.5445 | 1.5493 | |
15 | 1.8169 | 1.8278 | 1.8270 | 1.8395 | 1.8463 | 1.8410 | 1.8428 | |
Test7 | 4 | 1.2183 | 1.2275 | 1.2282 | 1.2373 | 1.2421 | 1.2429 | 1.2399 |
8 | 1.3179 | 1.3284 | 1.3291 | 1.3429 | 1.3420 | 1.3463 | 1.3391 | |
12 | 1.5219 | 1.5326 | 1.5316 | 1.5510 | 1.5410 | 1.5462 | 1.5506 | |
15 | 1.8179 | 1.8293 | 1.8289 | 1.8408 | 1.8466 | 1.8410 | 1.8440 | |
Test8 | 4 | 1.2173 | 1.2270 | 1.2272 | 1.2371 | 1.2403 | 1.2424 | 1.2393 |
8 | 1.3167 | 1.3278 | 1.3282 | 1.3409 | 1.3401 | 1.3447 | 1.3386 | |
12 | 1.5217 | 1.5308 | 1.5303 | 1.5497 | 1.5398 | 1.5460 | 1.5498 | |
15 | 1.8172 | 1.8273 | 1.8284 | 1.8396 | 1.8446 | 1.8397 | 1.8424 | |
Test9 | 4 | 1.2154 | 1.2265 | 1.2258 | 1.2357 | 1.2391 | 1.2409 | 1.2376 |
8 | 1.3166 | 1.3268 | 1.3277 | 1.3398 | 1.3389 | 1.3428 | 1.3375 | |
12 | 1.5204 | 1.5304 | 1.5291 | 1.5482 | 1.5397 | 1.5453 | 1.5485 | |
15 | 1.8154 | 1.8272 | 1.8281 | 1.8395 | 1.8431 | 1.8385 | 1.8422 | |
Test10 | 4 | 1.2155 | 1.2282 | 1.2270 | 1.2377 | 1.2404 | 1.2419 | 1.2380 |
8 | 1.3172 | 1.3283 | 1.3279 | 1.3401 | 1.3399 | 1.3444 | 1.3391 | |
12 | 1.5207 | 1.5319 | 1.5305 | 1.5494 | 1.5397 | 1.5460 | 1.5495 | |
15 | 1.8160 | 1.8272 | 1.8297 | 1.8400 | 1.8440 | 1.8393 | 1.8442 |
K | HEOA | HSOA | HWOA | CSA | FPA | PSO | BA |
4 | 3.1581 | 3.4458 | 3.5147 | 3.4581 | 3.6152 | 3.9951 | 3.8547 |
8 | 2.9315 | 3.5514 | 3.6891 | 3.5125 | 3.9156 | 3.9514 | 3.6984 |
12 | 1.9984 | 2.5589 | 3.6661 | 3.1285 | 3.9991 | 3.9541 | 3.8854 |
15 | 1.7415 | 2.6518 | 3.6581 | 3.1814 | 3.8147 | 3.8574 | 3.7781 |
Overall | 2.4574 | 3.0520 | 3.6320 | 3.3201 | 3.8362 | 3.9395 | 3.8042 |
Images | K | HSOA | HWOA | CSA | FPA | PSO |
Test1 | 4 | P < 0.05 | P < 0.05 | P < 0.05 | P < 0.05 | P < 0.05 |
8 | P > 0.05 | P < 0.05 | P < 0.05 | P < 0.05 | P < 0.05 | |
12 | P < 0.05 | P < 0.05 | P < 0.05 | P < 0.05 | P < 0.05 | |
15 | P < 0.05 | P > 0.05 | P < 0.05 | P < 0.05 | P < 0.05 | |
Test2 | 4 | P < 0.05 | P < 0.05 | P < 0.05 | P > 0.05 | P < 0.05 |
8 | P < 0.05 | P < 0.05 | P < 0.05 | P < 0.05 | P < 0.05 | |
12 | P < 0.05 | P < 0.05 | P < 0.05 | P < 0.05 | P < 0.05 | |
15 | P < 0.05 | P < 0.05 | P < 0.05 | P < 0.05 | P < 0.05 | |
Test3 | 4 | P < 0.05 | P < 0.05 | P < 0.05 | P < 0.05 | P < 0.05 |
8 | P < 0.05 | P < 0.05 | P < 0.05 | P < 0.05 | P < 0.05 | |
12 | P < 0.05 | P < 0.05 | P < 0.05 | P < 0.05 | P < 0.05 | |
15 | P < 0.05 | P < 0.05 | P < 0.05 | P < 0.05 | P < 0.05 | |
Test4 | 4 | P < 0.05 | P < 0.05 | P < 0.05 | P < 0.05 | P < 0.05 |
8 | P > 0.05 | P < 0.05 | P < 0.05 | P < 0.05 | P < 0.05 | |
12 | P < 0.05 | P > 0.05 | P < 0.05 | P < 0.05 | P < 0.05 | |
15 | P < 0.05 | P < 0.05 | P < 0.05 | P < 0.05 | P < 0.05 | |
Test5 | 4 | P < 0.05 | P < 0.05 | P < 0.05 | P < 0.05 | P < 0.05 |
8 | P < 0.05 | P < 0.05 | P < 0.05 | P < 0.05 | P < 0.05 | |
12 | P < 0.05 | P < 0.05 | P < 0.05 | P < 0.05 | P < 0.05 | |
15 | P < 0.05 | P < 0.05 | P < 0.05 | P < 0.05 | P < 0.05 | |
Test6 | 4 | P > 0.05 | P < 0.05 | P < 0.05 | P < 0.05 | P < 0.05 |
8 | P < 0.05 | P < 0.05 | P < 0.05 | P < 0.05 | P < 0.05 | |
12 | P < 0.05 | P < 0.05 | P < 0.05 | P < 0.05 | P < 0.05 | |
15 | P < 0.05 | P < 0.05 | P < 0.05 | P < 0.05 | P < 0.05 | |
Test7 | 4 | P < 0.05 | P < 0.05 | P > 0.05 | P < 0.05 | P < 0.05 |
8 | P < 0.05 | P < 0.05 | P < 0.05 | P < 0.05 | P < 0.05 | |
12 | P < 0.05 | P < 0.05 | P < 0.05 | P < 0.05 | P < 0.05 | |
15 | P < 0.05 | P < 0.05 | P < 0.05 | P < 0.05 | P < 0.05 | |
Test8 | 4 | P > 0.05 | P < 0.05 | P < 0.05 | P > 0.05 | P < 0.05 |
8 | P < 0.05 | P > 0.05 | P < 0.05 | P < 0.05 | P < 0.05 | |
12 | P < 0.05 | P < 0.05 | P < 0.05 | P < 0.05 | P < 0.05 | |
15 | P < 0.05 | P < 0.05 | P < 0.05 | P < 0.05 | P < 0.05 | |
Test9 | 4 | P < 0.05 | P < 0.05 | P < 0.05 | P < 0.05 | P < 0.05 |
8 | P < 0.05 | P > 0.05 | P < 0.05 | P < 0.05 | P < 0.05 | |
12 | P < 0.05 | P < 0.05 | P < 0.05 | P < 0.05 | P < 0.05 | |
15 | P < 0.05 | P < 0.05 | P < 0.05 | P < 0.05 | P < 0.05 | |
Test10 | 4 | P < 0.05 | P > 0.05 | P > 0.05 | P < 0.05 | P < 0.05 |
8 | P < 0.05 | P < 0.05 | P < 0.05 | P < 0.05 | P < 0.05 | |
12 | P < 0.05 | P < 0.05 | P < 0.05 | P < 0.05 | P < 0.05 | |
15 | P < 0.05 | P < 0.05 | P < 0.05 | P < 0.05 | P < 0.05 |
Method | R (%) | P (%) | AP (%) | VAL (%) |
FCM | 84.94 | 90.88 | 91.23 | 88.47 |
PCNN | 80.46 | 90.69 | 81.46 | 79.46 |
DLA | 70.12 | 92.95 | 78.32 | 77.57 |
BMPA | 93.19 | 91.28 | 91.45 | 90.38 |
Algorithm 1 Pseudo-code of HEOA algorithm |
Input: The color image Output: Segmentation color image Read the input and compute the histogram of three channels of color imageInitialize the parameters r1andr2 Initialize the random population Ci while L < Max_iter do Calculate the fitness values of EOA for (each hawk Ci) do Calculate Ceq, Xi Update the C using Eq. (25) Calculate the fitness function using Eq.(10) end for UpdateCbestif there is a better solution L=L+1 end while Get the best solution as the multilevel threshold K According the K segment the three channels of the images Get the segmentation images |
Algorithm | Parameters | Value |
EOA | c1 | 2 |
c2 | 2 | |
CSA [50] | AP | 0.5 |
FPA [51] | P | 0.5 |
PSO [52] | Swam size Cognitive, social acceleration Inertial weight | 2002, 20.95–0.4 |
BA [53] | β | (0, 1) |
HEOA | Levy | 1.5 |
No. | Functions | Related basic functions | Dim | fmin |
CEC-1 | Rotated Bent Cigar Function | Bent Cigar Function | 30 | 100 |
CEC-2 | Rotated Discus Function | Discus Function | 30 | 200 |
CEC-3 | Shifted and Rotated Weierstrass Function | Weierstrass Function | 30 | 300 |
CEC-4 | Shifted and Rotated Schwefel's Function | Schwefel's Function | 30 | 400 |
CEC-5 | Shifted and Rotated Katsuura Function | Katsuura Function | 30 | 500 |
CEC-6 | Shifted and Rotated HappyCat Function | HappyCat Function | 30 | 600 |
CEC-7 | Shifted and Rotated HGBat Function | HGBat Function | 30 | 700 |
CEC-8 | Shifted and Rotated Expanded Griewank's plus Rosenbrock's Function | Griewank's Function Rosenbrock's Function | 30 | 800 |
CEC-9 | Shifted and Rotated Expanded Scaer's F6 Function | Expanded Scaer's F6 Function | 30 | 900 |
CEC-10 | Hybrid Function 1 (N = 3) | Schwefel's FunctionRastrigin's FunctionHigh Conditioned Elliptic Function | 30 | 1000 |
CEC-11 | Hybrid Function 2 (N = 4) | Griewank's Function Weierstrass Function Rosenbrock's Function Scaer's F6 Function | 30 | 1100 |
CEC-12 | Hybrid Function 3 (N = 5) | Katsuura FunctionHappyCat FunctionExpanded Griewank's plus Rosenbrock's Function Schwefel's FunctionAckley's Function | 30 | 1200 |
CEC-13 | Composition Function 1 (N = 5) | Rosenbrock's FunctionHigh Conditioned Elliptic FunctionBent Cigar FunctionDiscus FunctionHigh Conditioned Elliptic Function | 30 | 1300 |
CEC-14 | Composition Function 2 (N = 3) | Schwefel's FunctionRastrigin's FunctionHigh Conditioned Elliptic Function | 30 | 1400 |
CEC-15 | Composition Function 3 (N = 5) | HGBat FunctionRastrigin's FunctionSchwefel's FunctionWeierstrass FunctionHigh Conditioned Elliptic Function | 30 | 1500 |
Func. | HEOA | CSA | PSO | FPA | BA | EOA | ||||||
Mean | Std. | Mean | Std. | Mean | Std. | Mean | Std. | Mean | Std. | Mean | Std. | |
CEC-1 | 1.05E+05 | 1.54E+07 | 4.21E+05 | 1.47E+08 | 2.17E+09 | 3.53E+07 | 1.76E+08 | 7.02E+07 | 4.76E+08 | 2.86E+08 | 1.07E+05 | 3.69E+07 |
CEC-2 | 6.70E+06 | 1.70E+09 | 2.06E+04 | 5.26E+09 | 7.01E+10 | 3.53E+09 | 3.04E+10 | 4.70E+09 | 3.36E+10 | 6.82E+09 | 6.70E+07 | 4.05E+09 |
CEC-3 | 3.20E+02 | 7.12E-02 | 3.20E+02 | 1.51E-01 | 3.22E+02 | 9.57E-02 | 3.21E+02 | 1.43E-01 | 3.21E+02 | 2.10E-01 | 3.55E+02 | 1.47E-01 |
CEC-4 | 4.10E+02 | 1.75E+00 | 4.05E+02 | 9.03E+00 | 5.46E+02 | 1.29E+01 | 5.30E+02 | 1.61E+01 | 5.21E+02 | 9.70E+00 | 4.50E+02 | 2.39E+01 |
CEC-5 | 9.81E+02 | 1.50E+02 | 1.22E+03 | 3.30E+02 | 4.74E+03 | 2.40E+02 | 3.80E+03 | 3.97E+02 | 3.65E+03 | 3.52E+02 | 9.91E+02 | 2.97E+02 |
CEC-6 | 2.05E+03 | 4.75E+06 | 2.14E+03 | 2.33E+07 | 3.79E+09 | 9.03E+06 | 4.52E+09 | 1.14E+07 | 1.28E+08 | 2.71E+07 | 2.10E+03 | 1.37E+07 |
CEC-7 | 7.02E+02 | 1.24E+01 | 7.03E+02 | 4.37E+01 | 1.81E+03 | 1.40E+01 | 1.78E+03 | 2.70E+01 | 1.78E+03 | 4.99E+01 | 8.82E+02 | 2.49E+01 |
CEC-8 | 1.47E+03 | 1.03E+06 | 1.41E+04 | 3.25E+06 | 2.21E+09 | 1.05E+06 | 1.34E+09 | 1.63E+06 | 1.34E+09 | 3.78E+06 | 1.47E+04 | 1.08E+06 |
CEC-9 | 1.00E+03 | 7.30E+00 | 1.00E+03 | 4.77E+01 | 1.33E+03 | 2.45E+01 | 1.33E+03 | 3.46E+01 | 1.62E+03 | 5.15E+01 | 1.00E+04 | 3.38E+01 |
CEC-10 | 1.23E+03 | 4.82E+04 | 2.05E+03 | 3.09E+06 | 2.58E+09 | 4.14E+05 | 2.97E+07 | 8.13E+05 | 4.41E+08 | 4.49E+06 | 1.36E+04 | 7.89E+05 |
CEC-11 | 1.35E+03 | 4.52E+01 | 1.40E+03 | 7.94E+01 | 2.00E+03 | 6.39E+01 | 1.77E+03 | 1.02E+02 | 1.77E+03 | 9.80E+01 | 1.32E+04 | 1.24E+02 |
CEC-12 | 1.30E+03 | 1.03E+01 | 1.30E+03 | 1.75E+01 | 1.76E+03 | 1.22E+01 | 1.49E+03 | 1.28E+01 | 1.51E+03 | 3.25E+01 | 1.34E+05 | 1.58E+01 |
CEC-13 | 1.30E+03 | 8.67E+00 | 1.34E+03 | 1.49E+00 | 5.44E+05 | 1.86E+01 | 1.54E+03 | 3.03E+01 | 1.56E+03 | 2.48E+00 | 1.55E+05 | 3.24E+01 |
CEC-14 | 3.22E+03 | 2.32E+03 | 8.88E+03 | 4.24E+03 | 2.23E+04 | 2.92E+03 | 2.23E+04 | 4.76E+03 | 2.23E+04 | 5.46E+03 | 3.22E+04 | 3.59E+03 |
CEC-15 | 1.60E+03 | 3.36E+02 | 1.60E+03 | 6.49E+02 | 1.28E+04 | 7.10E+02 | 9.01E+03 | 1.34E+03 | 4.09E+03 | 1.05E+03 | 1.80E+03 | 1.27E+03 |
No. | Measures | Formulation | Reference |
1 | Uniformity measure | U=1−2×(k−1)×∑dj=1∑i∈Rj(fi−μj)2N×(fmax−fmin)2 | [54] |
2 | Peak Signal-to-Noise Ratio (PSNR) | PSNR=10×log10(2552MSE) MSE=1mnm−1∑i=1n−1∑j=1[I(i,j)−K(i,j)]2 |
[55] |
3 | Feature Similarity Index (FSIM) | FSIM=∑x=ηSL(x)⋅PCm(x)∑x=ηPCm(x) | [56] |
Image | K | EOA-Otsu | EOA-3DOtsu | HEOA-Otsu | HEOA-3DOtsu |
Test1 | 4 | 0.8628 | 0.8886 | 0.8973 | 0.9106 |
8 | 0.8657 | 0.8864 | 0.8957 | 0.9170 | |
12 | 0.9103 | 0.9332 | 0.9411 | 0.9580 | |
15 | 0.9293 | 0.9479 | 0.9565 | 0.9695 | |
Test2 | 4 | 0.8644 | 0.8799 | 0.8901 | 0.9015 |
8 | 0.8459 | 0.8674 | 0.8736 | 0.9135 | |
12 | 0.9298 | 0.9511 | 0.9569 | 0.9591 | |
15 | 0.9287 | 0.9474 | 0.9562 | 0.9671 | |
Test3 | 4 | 0.8586 | 0.8833 | 0.8919 | 0.9056 |
8 | 0.8599 | 0.8766 | 0.8859 | 0.9246 | |
12 | 0.9207 | 0.9370 | 0.9466 | 0.9661 | |
15 | 0.9049 | 0.9239 | 0.9358 | 0.9688 | |
Test4 | 4 | 0.8518 | 0.8660 | 0.8805 | 0.8963 |
8 | 0.8856 | 0.9060 | 0.9125 | 0.9221 | |
12 | 0.8823 | 0.9034 | 0.9143 | 0.9619 | |
15 | 0.9012 | 0.9260 | 0.9343 | 0.9742 | |
Test5 | 4 | 0.8379 | 0.8545 | 0.8645 | 0.8988 |
8 | 0.8648 | 0.8854 | 0.8992 | 0.9193 | |
12 | 0.9166 | 0.9305 | 0.9446 | 0.9659 | |
15 | 0.9055 | 0.9286 | 0.9345 | 0.9750 | |
Test6 | 4 | 0.8725 | 0.8902 | 0.9025 | 0.9157 |
8 | 0.8854 | 0.9040 | 0.9160 | 0.9189 | |
12 | 0.9267 | 0.9484 | 0.9566 | 0.9585 | |
15 | 0.8904 | 0.9095 | 0.9214 | 0.9663 | |
Test7 | 4 | 0.8708 | 0.8883 | 0.9009 | 0.9147 |
8 | 0.8845 | 0.9037 | 0.9155 | 0.9171 | |
12 | 0.9250 | 0.9478 | 0.9555 | 0.9581 | |
15 | 0.8898 | 0.9087 | 0.9202 | 0.9648 | |
Test8 | 4 | 0.8704 | 0.8871 | 0.9000 | 0.9143 |
8 | 0.8830 | 0.9017 | 0.9142 | 0.9155 | |
12 | 0.9232 | 0.9469 | 0.9535 | 0.9578 | |
15 | 0.8886 | 0.9079 | 0.9201 | 0.9629 | |
Test9 | 4 | 0.8716 | 0.8884 | 0.9011 | 0.9151 |
8 | 0.8844 | 0.9026 | 0.9157 | 0.9163 | |
12 | 0.9237 | 0.9470 | 0.9552 | 0.9596 | |
15 | 0.8897 | 0.9082 | 0.9217 | 0.9633 | |
Test10 | 4 | 0.8720 | 0.8896 | 0.9024 | 0.9171 |
8 | 0.8863 | 0.9027 | 0.9162 | 0.9179 | |
12 | 0.9247 | 0.9482 | 0.9561 | 0.9607 | |
15 | 0.8915 | 0.9089 | 0.9223 | 0.9645 |
Image | K | HEOA | HSOA | HWOA | CSA | FPA | PSO | BA |
Test1 | 4 | 0.9571 | 0.9415 | 0.9437 | 0.9245 | 0.9284 | 0.9262 | 0.9243 |
8 | 0.9674 | 0.9525 | 0.9594 | 0.9299 | 0.9309 | 0.9392 | 0.9373 | |
12 | 0.9744 | 0.9641 | 0.9654 | 0.9404 | 0.9456 | 0.9424 | 0.9457 | |
15 | 0.9795 | 0.9684 | 0.9617 | 0.9428 | 0.9441 | 0.9445 | 0.9458 | |
Test2 | 4 | 0.9544 | 0.9460 | 0.9460 | 0.9224 | 0.9167 | 0.9207 | 0.9216 |
8 | 0.9722 | 0.9583 | 0.9624 | 0.9442 | 0.9422 | 0.9425 | 0.9356 | |
12 | 0.9813 | 0.9717 | 0.9639 | 0.9437 | 0.9458 | 0.9526 | 0.9497 | |
15 | 0.9824 | 0.9698 | 0.9697 | 0.9499 | 0.9479 | 0.9468 | 0.9515 | |
Test3 | 4 | 0.9528 | 0.9437 | 0.9427 | 0.9244 | 0.9184 | 0.9184 | 0.9165 |
8 | 0.9626 | 0.9500 | 0.9482 | 0.9255 | 0.9274 | 0.9323 | 0.9290 | |
12 | 0.9782 | 0.9657 | 0.9625 | 0.9464 | 0.9453 | 0.9486 | 0.9406 | |
15 | 0.9812 | 0.9701 | 0.9683 | 0.9467 | 0.9450 | 0.9487 | 0.9513 | |
Test4 | 4 | 0.9518 | 0.9403 | 0.9435 | 0.9201 | 0.9171 | 0.9161 | 0.9187 |
8 | 0.9699 | 0.9589 | 0.9520 | 0.9327 | 0.9350 | 0.9331 | 0.9402 | |
12 | 0.9749 | 0.9623 | 0.9653 | 0.9385 | 0.9398 | 0.9409 | 0.9439 | |
15 | 0.9806 | 0.9717 | 0.9700 | 0.9486 | 0.9490 | 0.9457 | 0.9485 | |
Test5 | 4 | 0.9541 | 0.9410 | 0.9402 | 0.9257 | 0.9167 | 0.9206 | 0.9207 |
8 | 0.9679 | 0.9558 | 0.9560 | 0.9385 | 0.9306 | 0.9399 | 0.9392 | |
12 | 0.9769 | 0.9670 | 0.9618 | 0.9393 | 0.9441 | 0.9404 | 0.9455 | |
15 | 0.9808 | 0.9639 | 0.9678 | 0.9468 | 0.9508 | 0.9519 | 0.9467 | |
Test6 | 4 | 0.9507 | 0.9393 | 0.9373 | 0.9169 | 0.9197 | 0.9138 | 0.9154 |
8 | 0.9666 | 0.9504 | 0.9512 | 0.9383 | 0.9298 | 0.9355 | 0.9352 | |
12 | 0.9787 | 0.9644 | 0.9673 | 0.9492 | 0.9505 | 0.9474 | 0.9431 | |
15 | 0.9824 | 0.9713 | 0.9667 | 0.9470 | 0.9480 | 0.9498 | 0.9472 | |
Test7 | 4 | 0.9514 | 0.9397 | 0.9378 | 0.9183 | 0.9212 | 0.9150 | 0.9167 |
8 | 0.9667 | 0.9524 | 0.9529 | 0.9392 | 0.9300 | 0.9360 | 0.9366 | |
12 | 0.9802 | 0.9648 | 0.9675 | 0.9510 | 0.9521 | 0.9479 | 0.9433 | |
15 | 0.9841 | 0.9725 | 0.9680 | 0.9473 | 0.9483 | 0.9502 | 0.9487 | |
Test8 | 4 | 0.9508 | 0.9377 | 0.9365 | 0.9165 | 0.9196 | 0.9137 | 0.9161 |
8 | 0.9649 | 0.9522 | 0.9520 | 0.9383 | 0.9284 | 0.9349 | 0.9355 | |
12 | 0.9789 | 0.9642 | 0.9663 | 0.9507 | 0.9507 | 0.9479 | 0.9417 | |
15 | 0.9837 | 0.9715 | 0.9670 | 0.9468 | 0.9464 | 0.9497 | 0.9479 | |
Test9 | 4 | 0.9503 | 0.9374 | 0.9355 | 0.9158 | 0.9191 | 0.9118 | 0.9151 |
8 | 0.9633 | 0.9510 | 0.9500 | 0.9363 | 0.9272 | 0.9346 | 0.9351 | |
12 | 0.9772 | 0.9634 | 0.9655 | 0.9497 | 0.9488 | 0.9468 | 0.9402 | |
15 | 0.9820 | 0.9706 | 0.9655 | 0.9462 | 0.9455 | 0.9491 | 0.9460 | |
Test10 | 4 | 0.9505 | 0.9382 | 0.9375 | 0.9171 | 0.9207 | 0.9118 | 0.9167 |
8 | 0.9637 | 0.9519 | 0.9510 | 0.9367 | 0.9277 | 0.9360 | 0.9364 | |
12 | 0.9776 | 0.9653 | 0.9661 | 0.9511 | 0.9493 | 0.9472 | 0.9413 | |
15 | 0.9838 | 0.9710 | 0.9655 | 0.9481 | 0.9464 | 0.9504 | 0.9479 |
Image | K | HEOA | HSOA | HWOA | CSA | FPA | PSO | BA |
Test1 | 4 | 23.3863 | 23.2573 | 23.2526 | 23.0420 | 23.0405 | 23.0397 | 23.0539 |
8 | 29.2231 | 29.1025 | 29.0968 | 28.9021 | 28.9007 | 28.8989 | 28.9020 | |
12 | 32.5692 | 32.4393 | 32.4442 | 32.2300 | 32.2265 | 32.2282 | 32.2414 | |
15 | 33.3295 | 33.1947 | 33.1953 | 33.0002 | 32.9903 | 32.9903 | 32.9923 | |
Test2 | 4 | 24.4702 | 24.3443 | 24.3386 | 24.1472 | 24.1340 | 24.1342 | 24.1432 |
8 | 29.4260 | 29.3020 | 29.2936 | 29.0852 | 29.0987 | 29.0861 | 29.1020 | |
12 | 32.5786 | 32.4454 | 32.4470 | 32.2471 | 32.2464 | 32.2522 | 32.2424 | |
15 | 34.2153 | 34.0865 | 34.0884 | 33.8828 | 33.8824 | 33.8823 | 33.8887 | |
Test3 | 4 | 24.4769 | 24.3474 | 24.3453 | 24.1433 | 24.1406 | 24.1517 | 24.1495 |
8 | 29.9667 | 29.8287 | 29.8366 | 29.6330 | 29.6383 | 29.6298 | 29.6211 | |
12 | 32.6661 | 32.5412 | 32.5392 | 32.3369 | 32.3265 | 32.3288 | 32.3254 | |
15 | 34.7330 | 34.6031 | 34.6015 | 34.3994 | 34.3925 | 34.4084 | 34.3905 | |
Test4 | 4 | 24.7640 | 24.6386 | 24.6369 | 24.4284 | 24.4400 | 24.4423 | 24.4286 |
8 | 29.5467 | 29.4208 | 29.4154 | 29.2214 | 29.2193 | 29.2207 | 29.2104 | |
12 | 33.5823 | 33.4474 | 33.4548 | 33.2561 | 33.2435 | 33.2461 | 33.2509 | |
15 | 34.2159 | 34.0826 | 34.0820 | 33.8787 | 33.8740 | 33.8707 | 33.8853 | |
Test5 | 4 | 24.5319 | 24.3991 | 24.3994 | 24.2048 | 24.1918 | 24.1918 | 24.1924 |
8 | 29.5550 | 29.4198 | 29.4251 | 29.2137 | 29.2181 | 29.2237 | 29.2154 | |
12 | 34.3322 | 34.1972 | 34.1979 | 34.0010 | 33.9935 | 33.9870 | 33.9959 | |
15 | 35.0669 | 34.9409 | 34.9366 | 34.7387 | 34.7254 | 34.7407 | 34.7279 | |
Test6 | 4 | 25.2119 | 25.0871 | 25.0842 | 24.8695 | 24.8803 | 24.8734 | 24.8829 |
8 | 30.9087 | 30.7880 | 30.7821 | 30.5869 | 30.5821 | 30.5735 | 30.5700 | |
12 | 34.1912 | 34.0606 | 34.0591 | 33.8576 | 33.8505 | 33.8585 | 33.8626 | |
15 | 35.7843 | 35.6567 | 35.6607 | 35.4492 | 35.4505 | 35.4438 | 35.4423 | |
Test7 | 4 | 25.4053 | 25.1458 | 25.1450 | 24.8847 | 25.0748 | 24.9284 | 24.9402 |
8 | 31.0914 | 30.8883 | 30.8601 | 30.7788 | 30.5828 | 30.6162 | 30.6718 | |
12 | 34.3507 | 34.1142 | 34.1742 | 34.0164 | 33.9942 | 33.8766 | 33.8983 | |
15 | 35.9362 | 35.6969 | 35.7545 | 35.6007 | 35.4588 | 35.4937 | 35.6024 | |
Test8 | 4 | 25.5670 | 25.2034 | 25.2799 | 24.9384 | 25.1283 | 24.9787 | 25.0445 |
8 | 31.2698 | 31.0054 | 30.9921 | 30.8672 | 30.7368 | 30.6972 | 30.8372 | |
12 | 34.5283 | 34.2658 | 34.2137 | 34.2017 | 34.1031 | 33.9285 | 34.0632 | |
15 | 36.0846 | 35.7585 | 35.9118 | 35.6393 | 35.6021 | 35.4988 | 35.6352 | |
Test9 | 4 | 25.5622 | 25.0597 | 25.1265 | 24.7749 | 24.9583 | 24.9368 | 24.8720 |
8 | 31.1656 | 30.8841 | 30.8166 | 30.7155 | 30.6732 | 30.6568 | 30.8154 | |
12 | 34.4249 | 34.0884 | 34.1830 | 34.1183 | 33.9539 | 33.7553 | 33.9067 | |
15 | 36.0382 | 35.7553 | 35.7737 | 35.5952 | 35.5146 | 35.3593 | 35.5413 | |
Test10 | 4 | 25.4424 | 24.9891 | 25.1108 | 24.7329 | 24.7752 | 24.9286 | 24.8300 |
8 | 31.1315 | 30.7681 | 30.7427 | 30.5414 | 30.6599 | 30.5129 | 30.6245 | |
12 | 34.3961 | 33.9364 | 34.1416 | 33.9835 | 33.8675 | 33.7435 | 33.8129 | |
15 | 35.8866 | 35.7225 | 35.7457 | 35.4554 | 35.4165 | 35.2905 | 35.3448 |
Image | K | HEOA | HSOA | HWOA | CSA | FPA | PSO | BA |
Test1 | 4 | 0.9367 | 0.9236 | 0.9225 | 0.9094 | 0.9039 | 0.8944 | 0.9087 |
8 | 0.9517 | 0.9414 | 0.9390 | 0.9036 | 0.9077 | 0.9157 | 0.9084 | |
12 | 0.9587 | 0.9433 | 0.9473 | 0.9135 | 0.9149 | 0.9172 | 0.9135 | |
15 | 0.9668 | 0.9511 | 0.9540 | 0.9271 | 0.9331 | 0.9287 | 0.9284 | |
Test2 | 4 | 0.9356 | 0.9227 | 0.9262 | 0.9006 | 0.8943 | 0.8936 | 0.9065 |
8 | 0.9584 | 0.9422 | 0.9438 | 0.9221 | 0.9123 | 0.9122 | 0.9230 | |
12 | 0.9632 | 0.9548 | 0.9563 | 0.9283 | 0.9305 | 0.9331 | 0.9233 | |
15 | 0.9633 | 0.9553 | 0.9506 | 0.9242 | 0.9251 | 0.9255 | 0.9202 | |
Test3 | 4 | 0.9325 | 0.9197 | 0.9181 | 0.9003 | 0.9077 | 0.8898 | 0.9078 |
8 | 0.9477 | 0.9333 | 0.9362 | 0.9060 | 0.9062 | 0.9001 | 0.9048 | |
12 | 0.9599 | 0.9482 | 0.9483 | 0.9289 | 0.9289 | 0.9190 | 0.9162 | |
15 | 0.9607 | 0.9487 | 0.9468 | 0.9309 | 0.9251 | 0.9325 | 0.9358 | |
Test4 | 4 | 0.9385 | 0.9213 | 0.9207 | 0.9065 | 0.9063 | 0.8982 | 0.8954 |
8 | 0.9485 | 0.9362 | 0.9349 | 0.9057 | 0.9058 | 0.9115 | 0.9144 | |
12 | 0.9580 | 0.9487 | 0.9498 | 0.9240 | 0.9298 | 0.9154 | 0.9200 | |
15 | 0.9637 | 0.9457 | 0.9532 | 0.9229 | 0.9227 | 0.9321 | 0.9237 | |
Test5 | 4 | 0.9332 | 0.9213 | 0.9264 | 0.8894 | 0.9071 | 0.9082 | 0.8960 |
8 | 0.9469 | 0.9377 | 0.9361 | 0.9085 | 0.9141 | 0.9083 | 0.9106 | |
12 | 0.9642 | 0.9438 | 0.9503 | 0.9210 | 0.9254 | 0.9187 | 0.9270 | |
15 | 0.9687 | 0.9484 | 0.9489 | 0.9240 | 0.9174 | 0.9240 | 0.9267 | |
Test6 | 4 | 0.9304 | 0.9238 | 0.9186 | 0.8868 | 0.9055 | 0.8972 | 0.9054 |
8 | 0.9518 | 0.9317 | 0.9372 | 0.9027 | 0.9099 | 0.9117 | 0.9085 | |
12 | 0.9579 | 0.9492 | 0.9460 | 0.9280 | 0.9270 | 0.9258 | 0.9158 | |
15 | 0.9682 | 0.9517 | 0.9490 | 0.9289 | 0.9204 | 0.9315 | 0.9250 | |
Test7 | 4 | 0.9313 | 0.9252 | 0.9198 | 0.8882 | 0.9057 | 0.8984 | 0.9060 |
8 | 0.9536 | 0.9322 | 0.9384 | 0.9035 | 0.9109 | 0.9127 | 0.9087 | |
12 | 0.9590 | 0.9498 | 0.9477 | 0.9287 | 0.9280 | 0.9273 | 0.9177 | |
15 | 0.9699 | 0.9524 | 0.9510 | 0.9292 | 0.9222 | 0.9335 | 0.9258 | |
Test8 | 4 | 0.9294 | 0.9239 | 0.9181 | 0.8877 | 0.9044 | 0.8970 | 0.9058 |
8 | 0.9518 | 0.9309 | 0.9381 | 0.9029 | 0.9096 | 0.9116 | 0.9070 | |
12 | 0.9573 | 0.9487 | 0.9470 | 0.9276 | 0.9263 | 0.9263 | 0.9175 | |
15 | 0.9682 | 0.9513 | 0.9494 | 0.9280 | 0.9216 | 0.9324 | 0.9240 | |
Test9 | 4 | 0.9292 | 0.9234 | 0.9161 | 0.8866 | 0.9038 | 0.8956 | 0.9052 |
8 | 0.9506 | 0.9296 | 0.9368 | 0.9010 | 0.9089 | 0.9096 | 0.9064 | |
12 | 0.9569 | 0.9469 | 0.9470 | 0.9273 | 0.9250 | 0.9262 | 0.9160 | |
15 | 0.9676 | 0.9501 | 0.9474 | 0.9275 | 0.9216 | 0.9308 | 0.9236 | |
Test10 | 4 | 0.9296 | 0.9246 | 0.9170 | 0.8874 | 0.9046 | 0.8962 | 0.9057 |
8 | 0.9509 | 0.9306 | 0.9378 | 0.9025 | 0.9093 | 0.9098 | 0.9069 | |
12 | 0.9589 | 0.9483 | 0.9490 | 0.9275 | 0.9261 | 0.9277 | 0.9165 | |
15 | 0.9678 | 0.9501 | 0.9479 | 0.9287 | 0.9232 | 0.9316 | 0.9240 |
Image | K | HEOA | HSOA | HWOA | CSA | FPA | PSO | BA |
Test1 | 4 | 1.2145 | 1.2251 | 1.2247 | 1.2442 | 1.2405 | 1.2406 | 1.2389 |
8 | 1.3154 | 1.3254 | 1.3260 | 1.3452 | 1.3422 | 1.3386 | 1.3385 | |
12 | 1.5172 | 1.5274 | 1.5279 | 1.5448 | 1.5467 | 1.5451 | 1.5466 | |
15 | 1.8157 | 1.8260 | 1.8258 | 1.8359 | 1.8435 | 1.8376 | 1.8439 | |
Test2 | 4 | 1.2149 | 1.2259 | 1.2255 | 1.2363 | 1.2392 | 1.2436 | 1.2365 |
8 | 1.3154 | 1.3262 | 1.3263 | 1.3388 | 1.3411 | 1.3401 | 1.3371 | |
12 | 1.5181 | 1.5286 | 1.5288 | 1.5423 | 1.5449 | 1.5466 | 1.5389 | |
15 | 1.8158 | 1.8266 | 1.8266 | 1.8419 | 1.8398 | 1.8409 | 1.8424 | |
Test3 | 4 | 1.2151 | 1.2257 | 1.2258 | 1.2354 | 1.2367 | 1.2445 | 1.2367 |
8 | 1.3158 | 1.3268 | 1.3265 | 1.3402 | 1.3411 | 1.3456 | 1.3436 | |
12 | 1.5191 | 1.5292 | 1.5292 | 1.5394 | 1.5406 | 1.5474 | 1.5487 | |
15 | 1.8164 | 1.8270 | 1.8273 | 1.8462 | 1.8369 | 1.8437 | 1.8413 | |
Test4 | 4 | 1.2151 | 1.2254 | 1.2253 | 1.2444 | 1.2358 | 1.2358 | 1.2409 |
8 | 1.3166 | 1.3268 | 1.3271 | 1.3432 | 1.3398 | 1.3458 | 1.3461 | |
12 | 1.5192 | 1.5299 | 1.5301 | 1.5421 | 1.5476 | 1.5483 | 1.5465 | |
15 | 1.8166 | 1.8275 | 1.8269 | 1.8390 | 1.8466 | 1.8422 | 1.8425 | |
Test5 | 4 | 1.2161 | 1.2269 | 1.2269 | 1.2388 | 1.2447 | 1.2368 | 1.2401 |
8 | 1.3167 | 1.3273 | 1.3273 | 1.3456 | 1.3396 | 1.3382 | 1.3431 | |
12 | 1.5201 | 1.5304 | 1.5302 | 1.5471 | 1.5428 | 1.5454 | 1.5401 | |
15 | 1.8169 | 1.8274 | 1.8278 | 1.8431 | 1.8379 | 1.8456 | 1.8405 | |
Test6 | 4 | 1.2168 | 1.2271 | 1.2268 | 1.2372 | 1.2413 | 1.2412 | 1.2383 |
8 | 1.3172 | 1.3281 | 1.3273 | 1.3412 | 1.3407 | 1.3448 | 1.3379 | |
12 | 1.5206 | 1.5307 | 1.5313 | 1.5491 | 1.5408 | 1.5445 | 1.5493 | |
15 | 1.8169 | 1.8278 | 1.8270 | 1.8395 | 1.8463 | 1.8410 | 1.8428 | |
Test7 | 4 | 1.2183 | 1.2275 | 1.2282 | 1.2373 | 1.2421 | 1.2429 | 1.2399 |
8 | 1.3179 | 1.3284 | 1.3291 | 1.3429 | 1.3420 | 1.3463 | 1.3391 | |
12 | 1.5219 | 1.5326 | 1.5316 | 1.5510 | 1.5410 | 1.5462 | 1.5506 | |
15 | 1.8179 | 1.8293 | 1.8289 | 1.8408 | 1.8466 | 1.8410 | 1.8440 | |
Test8 | 4 | 1.2173 | 1.2270 | 1.2272 | 1.2371 | 1.2403 | 1.2424 | 1.2393 |
8 | 1.3167 | 1.3278 | 1.3282 | 1.3409 | 1.3401 | 1.3447 | 1.3386 | |
12 | 1.5217 | 1.5308 | 1.5303 | 1.5497 | 1.5398 | 1.5460 | 1.5498 | |
15 | 1.8172 | 1.8273 | 1.8284 | 1.8396 | 1.8446 | 1.8397 | 1.8424 | |
Test9 | 4 | 1.2154 | 1.2265 | 1.2258 | 1.2357 | 1.2391 | 1.2409 | 1.2376 |
8 | 1.3166 | 1.3268 | 1.3277 | 1.3398 | 1.3389 | 1.3428 | 1.3375 | |
12 | 1.5204 | 1.5304 | 1.5291 | 1.5482 | 1.5397 | 1.5453 | 1.5485 | |
15 | 1.8154 | 1.8272 | 1.8281 | 1.8395 | 1.8431 | 1.8385 | 1.8422 | |
Test10 | 4 | 1.2155 | 1.2282 | 1.2270 | 1.2377 | 1.2404 | 1.2419 | 1.2380 |
8 | 1.3172 | 1.3283 | 1.3279 | 1.3401 | 1.3399 | 1.3444 | 1.3391 | |
12 | 1.5207 | 1.5319 | 1.5305 | 1.5494 | 1.5397 | 1.5460 | 1.5495 | |
15 | 1.8160 | 1.8272 | 1.8297 | 1.8400 | 1.8440 | 1.8393 | 1.8442 |
K | HEOA | HSOA | HWOA | CSA | FPA | PSO | BA |
4 | 3.1581 | 3.4458 | 3.5147 | 3.4581 | 3.6152 | 3.9951 | 3.8547 |
8 | 2.9315 | 3.5514 | 3.6891 | 3.5125 | 3.9156 | 3.9514 | 3.6984 |
12 | 1.9984 | 2.5589 | 3.6661 | 3.1285 | 3.9991 | 3.9541 | 3.8854 |
15 | 1.7415 | 2.6518 | 3.6581 | 3.1814 | 3.8147 | 3.8574 | 3.7781 |
Overall | 2.4574 | 3.0520 | 3.6320 | 3.3201 | 3.8362 | 3.9395 | 3.8042 |
Images | K | HSOA | HWOA | CSA | FPA | PSO |
Test1 | 4 | P < 0.05 | P < 0.05 | P < 0.05 | P < 0.05 | P < 0.05 |
8 | P > 0.05 | P < 0.05 | P < 0.05 | P < 0.05 | P < 0.05 | |
12 | P < 0.05 | P < 0.05 | P < 0.05 | P < 0.05 | P < 0.05 | |
15 | P < 0.05 | P > 0.05 | P < 0.05 | P < 0.05 | P < 0.05 | |
Test2 | 4 | P < 0.05 | P < 0.05 | P < 0.05 | P > 0.05 | P < 0.05 |
8 | P < 0.05 | P < 0.05 | P < 0.05 | P < 0.05 | P < 0.05 | |
12 | P < 0.05 | P < 0.05 | P < 0.05 | P < 0.05 | P < 0.05 | |
15 | P < 0.05 | P < 0.05 | P < 0.05 | P < 0.05 | P < 0.05 | |
Test3 | 4 | P < 0.05 | P < 0.05 | P < 0.05 | P < 0.05 | P < 0.05 |
8 | P < 0.05 | P < 0.05 | P < 0.05 | P < 0.05 | P < 0.05 | |
12 | P < 0.05 | P < 0.05 | P < 0.05 | P < 0.05 | P < 0.05 | |
15 | P < 0.05 | P < 0.05 | P < 0.05 | P < 0.05 | P < 0.05 | |
Test4 | 4 | P < 0.05 | P < 0.05 | P < 0.05 | P < 0.05 | P < 0.05 |
8 | P > 0.05 | P < 0.05 | P < 0.05 | P < 0.05 | P < 0.05 | |
12 | P < 0.05 | P > 0.05 | P < 0.05 | P < 0.05 | P < 0.05 | |
15 | P < 0.05 | P < 0.05 | P < 0.05 | P < 0.05 | P < 0.05 | |
Test5 | 4 | P < 0.05 | P < 0.05 | P < 0.05 | P < 0.05 | P < 0.05 |
8 | P < 0.05 | P < 0.05 | P < 0.05 | P < 0.05 | P < 0.05 | |
12 | P < 0.05 | P < 0.05 | P < 0.05 | P < 0.05 | P < 0.05 | |
15 | P < 0.05 | P < 0.05 | P < 0.05 | P < 0.05 | P < 0.05 | |
Test6 | 4 | P > 0.05 | P < 0.05 | P < 0.05 | P < 0.05 | P < 0.05 |
8 | P < 0.05 | P < 0.05 | P < 0.05 | P < 0.05 | P < 0.05 | |
12 | P < 0.05 | P < 0.05 | P < 0.05 | P < 0.05 | P < 0.05 | |
15 | P < 0.05 | P < 0.05 | P < 0.05 | P < 0.05 | P < 0.05 | |
Test7 | 4 | P < 0.05 | P < 0.05 | P > 0.05 | P < 0.05 | P < 0.05 |
8 | P < 0.05 | P < 0.05 | P < 0.05 | P < 0.05 | P < 0.05 | |
12 | P < 0.05 | P < 0.05 | P < 0.05 | P < 0.05 | P < 0.05 | |
15 | P < 0.05 | P < 0.05 | P < 0.05 | P < 0.05 | P < 0.05 | |
Test8 | 4 | P > 0.05 | P < 0.05 | P < 0.05 | P > 0.05 | P < 0.05 |
8 | P < 0.05 | P > 0.05 | P < 0.05 | P < 0.05 | P < 0.05 | |
12 | P < 0.05 | P < 0.05 | P < 0.05 | P < 0.05 | P < 0.05 | |
15 | P < 0.05 | P < 0.05 | P < 0.05 | P < 0.05 | P < 0.05 | |
Test9 | 4 | P < 0.05 | P < 0.05 | P < 0.05 | P < 0.05 | P < 0.05 |
8 | P < 0.05 | P > 0.05 | P < 0.05 | P < 0.05 | P < 0.05 | |
12 | P < 0.05 | P < 0.05 | P < 0.05 | P < 0.05 | P < 0.05 | |
15 | P < 0.05 | P < 0.05 | P < 0.05 | P < 0.05 | P < 0.05 | |
Test10 | 4 | P < 0.05 | P > 0.05 | P > 0.05 | P < 0.05 | P < 0.05 |
8 | P < 0.05 | P < 0.05 | P < 0.05 | P < 0.05 | P < 0.05 | |
12 | P < 0.05 | P < 0.05 | P < 0.05 | P < 0.05 | P < 0.05 | |
15 | P < 0.05 | P < 0.05 | P < 0.05 | P < 0.05 | P < 0.05 |
Method | R (%) | P (%) | AP (%) | VAL (%) |
FCM | 84.94 | 90.88 | 91.23 | 88.47 |
PCNN | 80.46 | 90.69 | 81.46 | 79.46 |
DLA | 70.12 | 92.95 | 78.32 | 77.57 |
BMPA | 93.19 | 91.28 | 91.45 | 90.38 |