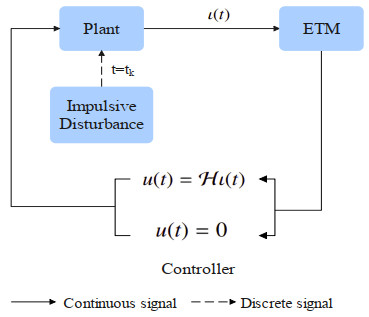
Citation: Anju Usman Singh. Dysbiosis, small intestinal bacterial overgrowth and biofilms in autism and chronic illness[J]. AIMS Molecular Science, 2018, 5(2): 160-165. doi: 10.3934/molsci.2018.2.160
[1] | Tao Xie, Xing Xiong . Finite-time synchronization of fractional-order heterogeneous dynamical networks with impulsive interference via aperiodical intermittent control. AIMS Mathematics, 2025, 10(3): 6291-6317. doi: 10.3934/math.2025287 |
[2] | Huiling Li, Jin-E Zhang, Ailong Wu . Finite-time stabilization of nonlinear systems with partially known states via aperiodic intermittent control and event-triggered impulsive control. AIMS Mathematics, 2025, 10(2): 3269-3290. doi: 10.3934/math.2025152 |
[3] | Linni Li, Jin-E Zhang . Input-to-state stability of nonlinear systems with delayed impulse based on event-triggered impulse control. AIMS Mathematics, 2024, 9(10): 26446-26461. doi: 10.3934/math.20241287 |
[4] | Biwen Li, Yujie Liu . Quasi-synchronization of nonlinear systems with parameter mismatch and time-varying delays via event-triggered impulsive control. AIMS Mathematics, 2025, 10(2): 3759-3778. doi: 10.3934/math.2025174 |
[5] | Jiaojiao Li, Yingying Wang, Jianyu Zhang . Event-triggered sliding mode control for a class of uncertain switching systems. AIMS Mathematics, 2023, 8(12): 29424-29439. doi: 10.3934/math.20231506 |
[6] | Miao Xiao, Zhe Lin, Qian Jiang, Dingcheng Yang, Xiongfeng Deng . Neural network-based adaptive finite-time tracking control for multiple inputs uncertain nonlinear systems with positive odd integer powers and unknown multiple faults. AIMS Mathematics, 2025, 10(3): 4819-4841. doi: 10.3934/math.2025221 |
[7] | Biwen Li, Qiaoping Huang . Synchronization issue of uncertain time-delay systems based on flexible impulsive control. AIMS Mathematics, 2024, 9(10): 26538-26556. doi: 10.3934/math.20241291 |
[8] | Guijun Xing, Huatao Chen, Zahra S. Aghayan, Jingfei Jiang, Juan L. G. Guirao . Tracking control for a class of fractional order uncertain systems with time-delay based on composite nonlinear feedback control. AIMS Mathematics, 2024, 9(5): 13058-13076. doi: 10.3934/math.2024637 |
[9] | Li Liu, Yinfang Song, Hong Yu, Gang Zhang . Almost sure exponential synchronization of multilayer complex networks with Markovian switching via aperiodically intermittent discrete observa- tion noise. AIMS Mathematics, 2024, 9(10): 28828-28849. doi: 10.3934/math.20241399 |
[10] | Aziz Belmiloudi . Time-varying delays in electrophysiological wave propagation along cardiac tissue and minimax control problems associated with uncertain bidomain type models. AIMS Mathematics, 2019, 4(3): 928-983. doi: 10.3934/math.2019.3.928 |
Impulsive systems are a class of dynamical systems characterized by sudden changes in state or input at discrete time points. Such dynamical properties of impulsive systems have generated widespread applications in fields such as electrical engineering [1], digital signal processing [2], and biomedical engineering [3]. In impulsive systems, impulses are typically categorized into stabilizing impulses and disturbance impulses. Stabilizing impulses represent the stabilizing effect and optimal performance achieved by the nonlinear system under specific impulsive control, positively influencing system stability (see, e.g., [4,5]). Conversely, disturbance impulses may occur when the nonlinear system is subjected to external perturbations, negatively affecting system stability and potentially leading to instability (see, e.g., [6,7]). To maintain system stability and performance, corresponding control strategies are required to suppress or compensate for such disturbance impulses.
In certain practical engineering applications, such as wind power management, vehicle control, and spacecraft trajectory adjustments, continuously feeding control signals into the plant system is not realistic. Compared to traditional continuous control methods, intermittent control (IC) allows for inputting control signals into the plant system only at specific control time intervals, making it more suitable for these actual applications. IC can be divided into periodic intermittent control (PIC) (see e.g., [8,9,10]) and aperiodic intermittent control (APIC) (see, e.g., [11,12,13]). PIC inputs control signals into the plant system at fixed control period and control width, while APIC is more flexible, allowing for dynamic adjustments of control strategies as needed. In [14], exponential stability of nonlinear systems under PIC was investigated, and stability criteria based on linear matrix inequalities (LMI) and scalar inequalities were proposed. Given that the control period and width in PIC are fixed and known, the derived conditions may be too conservative. Consequently, there is an urgent need to introduce the APIC to replace PIC. In [15], the stabilization problem of continuous systems achieving exponential input-to-state stability through APIC was studied. In [16], the concept of aperiodic time-triggered IC was introduced, and a lower conservative exponential stability theorem was formulated for the considered aperiodic time-triggered IC system through the construction of a hybrid time-dependent Lyapunov functional. In the light of these reporting-based works, the APIC has become a highly regarded area of interest.
In current research, traditional time-triggered control (TC) and event-triggered control (EC) employ different approaches for control system design. TC involves sending and executing control signals at regular intervals, which, although simple and convenient, may lead to resource wastage and performance degradation due to its fixed-time interval signal transmission. In contrast, EC significantly reduces resource consumption and enhances system performance by updating control signals under specific triggering conditions. Recently, EC has achieved significant progress in achieving the satisfied dynamic performances of control systems (see, e.g., [17,18,19,20]). However, EC is unnecessary and energy-consuming as the event generator and controller continue to keep on running when the control execution is not required. Hence, an event-triggered intermittent control (EIC) strategy combining IC with EC has emerged. This strategy operates within specific time periods, allowing for intermittent updating of control signals, thereby further reducing resource consumption. A dynamic EIC scheme with input delays was introduced to stabilize the delayed dynamical systems in [21]. The time-triggered APIC and event-triggered APIC (EAPIC) schemes were developed to achieve exponential stabilization of continuous-time dynamical systems in [22].
Within a finite-time control framework, two particular stability concepts are under scrutiny: asymptotic stability, where system trajectories converge to equilibrium states (see, e.g., [23,24]), and finite-time stability (FTS), where the system does not exceed a predefined threshold in finite-time. Building upon the FTS concept, finite-time contraction stability (FTCS) further emphasizes the "boundedness" and "contraction" nature of the system itself. Numerous theoretical results exist regarding FTS and FTCS (see, e.g., [25,26,27]). Additionally, some guidelines for FTS and FTCS are also presented for impulsive systems (see, e.g., [28,29,30]). In [31], some sufficient conditions of FTS/FTCS for nonlinear impulsive systems were constructed via the Lyapunov function method. In [32], FTS and FTCS of nonlinear systems subject to impulsive disturbances with parameter uncertainties were obtained by designing APIC. A further exploration about FTS for nonlinear systems involving impulsive disturbances under the EAPIC strategy faces a series of challenges. This includes how to build the relationship between the IC parameters and event-triggering mechanism (ETM) threshold under EAPIC, as well as whether the designing APIC can suppress the effects of impulsive disturbance. These issues constitute the focal points of further study. From the above discussion, the strengths of this paper can be concluded as below:
(1) Based on the Lyapunov method and LMI techniques, this paper combines EC and APIC to investigate the FTS and FTCS of uncertain systems with uncertain impulsive disturbance using EAPIC, where the impulsive instants are generated by the predesigned ETM.
(2) Four elements of uncertain systems–FTS/FTCS, APIC, and impulse disturbances–are combined simultaneously. Moreover, under EAPIC, the correlation among finite-time interval, impulsive disturbance, inspection period, and ETM threshold is revealed. Additionally, the adopted EAPIC can exclude the Zeno phenomenon.
(3) You et al. [32] apply time-triggered APIC to stabilize uncertain nonlinear systems with impulse disturbance. This method involves control actions at fixed time intervals, which can lead to unnecessary computations and communications. In contrast, the EAPIC considered in this paper triggers control based on the actual changes in the system state, thus being more universal and flexible.
Notations: $ \mathcal{R} $ and $ \mathcal{R}_+ $ represent the sets of real and non-negative real numbers, respectively. $ \mathcal{Z}_+ $ stands for the set of positive integers. $ \mathcal{R}^n $ denotes the $ n $-dimensional real space with the Euclidean norm $ \vert\vert\cdot\vert\vert $. $ \mathcal{R}^{n\times m} $ indicates the $ n\times m $ dimensional real space. $ Y\geq 0 $ or $ Y\leq 0 $ means that matrix $ Y $ is a positive definite or negative definite symmetric matrix. $ Y^{T} $ and $ Y^{-1} $ are used to describe the transpose and inverse of $ Y $ separately. $ \lambda_{max}(Y) $ and $ \lambda_{min}(Y) $ represent the maximum and minimum eigenvalues of matrix $ Y $, respectively. $ \ast $ stands for a symmetric block within a symmetric matrix. $ \mathcal{I} $ denotes the unit matrix possessing suitable dimension. $ C([-\theta, 0]; \mathcal{R}^n) $ is the family of continuous functions $ \psi: [-\theta, 0]\to\mathcal{R}^n $. A locally Lipschitz function $ \nu: \mathcal{R}^n\to\mathcal{R}_+ $ has the upper right-hand Dini derivative determined by
$ D^+\nu(\iota) = \limsup\limits_{a\to0^+}\frac{\nu(\iota+a)-\nu(\iota)}{a}. $ |
The uncertain system is considered as follows:
$ {˙ι(t)=A(t)ι(t)+B(t)σ(ι(t−θ))+C(t)u(t),t≥0,ι(t)=ς(t),t∈[−θ,0], $
|
(2.1) |
where $ \iota(t)\in\mathcal{R}^n $ is state vector, $ \mathcal{A}(t), \mathcal{B}(t)\in\mathcal{R}^{n\times n} $, as well as $ \mathcal{C}(t)\in\mathcal{R}^{n\times m} $. Assume that $ \mathcal{A}(t) = $ $ \mathcal{A}+\Delta \mathcal{A}(t) $, $ \mathcal{B}(t) = \mathcal{B}+\Delta \mathcal{B}(t) $, and $ \mathcal{C}(t) = \mathcal{C}+\Delta \mathcal{C}(t) $, in which $ \mathcal{A}, \mathcal{B} $, and $ \mathcal{C} $ are known real matrices, whereas $ \Delta \mathcal{A}(t), \Delta \mathcal{B}(t) $, and $ \Delta \mathcal{C}(t) $ are uncertain unknown matrices. $ \sigma(\iota(t)) = \left(\sigma_1(\iota_1(t)), \sigma_2(\iota_2(t)), \cdots, \sigma_n(\iota_n(t))\right)^T\in\mathcal{R}^n $ with $ \sigma(0) = 0 $. $ \theta $ denotes time delay. The APIC $ u(t)\in\mathcal{R}^m $ is given as below
$ u(t)={Hι(t),tk≤t<tk+τk,0,tk+τk≤t<tk+1, $
|
(2.2) |
in which $ \mathcal{H} $ signifies the control strength. $ t_k, \tau_k $ respectively denote the start time and control width of each control period, which satisfy $ 0 < \tau_k < t_{k+1}-t_k $. It can be noted that the controller (2.2) requires operation only on control intervals. That is, the absence of transmitting control input information during the rest interval implies that system (2.1) operates alternately between the open-loop control mode and the closed-loop control mode. Moreover, it is considered that the state of system (2.1) jumps in certain cases due to the activation of the aperiodic interval controller, namely, the generation of impulsive phenomena, and we regard them as disturbance impulses. Thus, system (2.1) with impulses involved can be formulated as
$ {˙ι(t)=A(t)ι(t)+B(t)σ(ι(t−θ))+C(t)u(t),t≠tk,t≥0,ι(t)=D(t)ι(t−),t=tk,ι(t)=ς(t),t∈[−θ,0], $
|
(2.3) |
where $ \mathcal{D}(t) $ represents the impulse gain matrix, which satisfies $ \mathcal{D}(t) = \mathcal{D}+\Delta \mathcal{D}(t) $. $ \mathcal{D} $ is a known matrix, and $ \Delta \mathcal{D}(t) $ is an unknown matrix. Assume system (2.3) is right continuous. Put $ \iota(t, 0, \varsigma) $ to indicate the solution of system (2.3) through $ (0, \varsigma), \varsigma\in \mathcal{R}^n $. The series $ \left\{t_k\right\}_{k = 1}^{+\infty} $ fulfills $ 0 = t_0 < t_1 < \cdots < t_k < \cdots $ and $ \lim_{k\to+\infty} = +\infty $.
It is assumed that the intermittent sequence $ \left\{t_k\right\} $ is created through the ETM as follows:
$ tk+1={inf{t:t∈Γk(tk+τk,tk+ω]},ifΓk(tk+τk,tk+ω]≠∅,tk+ω,ifΓk(tk+τk,tk+ω]=∅, $
|
(2.4) |
where $ \Gamma_k(\varepsilon, t] = \left\{t: t > \varepsilon+\tau_k, \mathcal{U}(\iota(t))\geq e^\zeta \mathcal{U}(\iota(\varepsilon+\tau_k))\right\} $, $ e^\zeta, \zeta > 0 $ denotes the threshold value, $ \omega > 0 $ stands for the inspection period, which is generally devised as a constant large enough, as well as $ \mathcal{U}(\cdot) $ indicates the Lyapunov function with respect to the state of the system (2.3). Figures 1 and 2 describe the EAPIC loop and framework of EAPIC, respectively.
The following describes some assumptions, definitions, and lemmas.
Assumption 2.1 ([29]). Suppose there are positive constants $ \varpi_j $, which make
$ \vert\sigma_j(\beta_1)-\sigma_j(\beta_2)\vert\leq\varpi_j\vert\beta_1-\beta_2\vert,\; \beta_1,\beta_2\in \mathcal{R},\; j = 1,2,\dots,n, $ |
hold.
Assumption 2.2 ([32]). The parameter uncertainties $ \Delta \mathcal{A}(t), \Delta \mathcal{B}(t), \Delta \mathcal{C}(t) $, and $ \Delta \mathcal{D}(t) $ satisfy
$ \left(\Delta \mathcal{A}(t), \Delta \mathcal{B}(t), \Delta \mathcal{C}(t)\right) = \mathcal{N} \mathcal{K}_1(t)\left( \mathcal{M}_1, \mathcal{M}_2, \mathcal{M}_3\right),\; \; \Delta \mathcal{D}(t) = \mathcal{F} \mathcal{K}_2(t) \mathcal{M}_4, $ |
where $ \mathcal{N}, \mathcal{F}, \mathcal{M}_1, \mathcal{M}_2, \mathcal{M}_3 $, and $ \mathcal{M}_4 $ are known matrices as well as $ \mathcal{K}_i(t) $ is an uncertain matrix-valued function of suitable dimension fulfilling
$ \mathcal{K}_i^T(t) \mathcal{K}_i(t)\leq \mathcal{I},\; i = 1,2. $ |
Definition 2.1. For specified constants $ \mathcal{T}, \delta_1, \delta_2, \pi, \rho $ with $ 0 < \rho < \delta_1 < \delta_2 $ and $ \pi\in(0, \mathcal{T}) $, along with an arbitrary trajectory $ \iota(t) $, the existence of a controller making $ \vert\vert\iota(0)\vert\vert\leq\delta_1 $ implies that $ \vert\vert\iota(t)\vert\vert\leq\delta_2 $ for $ t\in[0, \mathcal{T}] $, then system (2.3) is called FTS with respect to (w.r.t) $ (\mathcal{T}, \delta_1, \delta_2) $. Moreover, for $ t\in[\mathcal{T}-\pi, \mathcal{T}] $, if $ \vert\vert\iota(t)\vert\vert\leq\rho $, then system (2.3) is called FTCS w.r.t $ (\mathcal{T}, \delta_1, \delta_2, \rho, \pi) $.
Remark 2.1. According to [33], FTS depicts the "boundedness" of the described systems. In contrast, FTCS demonstrates the "boundedness" and "contraction" of the described systems simultaneously. In particular, to attain the "contraction" feature, FTCS requires the additional condition that the reasoned systems can remain in a comparatively smaller boundary than the initial boundary until the end time is implemented. Figure 3 visually depicts the FTS and FTCS, which also consider the possible impact on the system of impulsive disturbance.
Lemma 2.1 ([34]). For matrices $ \mathcal{D}, \mathcal{S}, \mathcal{F}, \mathcal{M} $, and $ \mathcal{K}(t) $ with appropriate dimensions and $ \mathcal{S}>0, \mathcal{K}^{T} \mathcal{K}(t)\leq\mathcal{I} $, scalars $ \kappa>0 $ and $ \mathcal{S}-\kappa \mathcal{F} \mathcal{F}^T>0 $, it follows that
$ ( \mathcal{D}+ \mathcal{F} \mathcal{K}(t) \mathcal{M})^T \mathcal{S}^{-1}( \mathcal{D}+ \mathcal{F} \mathcal{K}(t) \mathcal{M})\leq \mathcal{D}^T( \mathcal{S}-\kappa \mathcal{F} \mathcal{F}^T)^{-1} \mathcal{D}+\kappa^{-1} \mathcal{M}^T \mathcal{M}. $ |
Lemma 2.2 ([35]). For a symmetric and positive matrix $ \mathcal{Q} $, constant $ \varrho > 0 $, one has
$ 2e^Tf\leq\varrho e^T\mathcal{Q}e+\varrho^{-1} f^T\mathcal{Q}^{-1}f,\; \; e,f\in \mathcal{R}^n. $ |
Lemma 2.3 ([36]). The $ \mathcal{N} $ and $ \mathcal{M} $ are matrices with proper dimensions. Let $ \mathcal{K}(t) $ meet $ \mathcal{K}^{T} \mathcal{K}(t)\leq\mathcal{I} $. For any $ \gamma > 0 $, then
$ \mathcal{N} \mathcal{K}(t) \mathcal{M}+ \mathcal{M}^T \mathcal{K}^T(t) \mathcal{N}^T\leq\gamma \mathcal{N} \mathcal{N}^T+\gamma^{-1} \mathcal{M}^T \mathcal{M}. $ |
Lemma 2.4 ([29]). Let $ \iota\in C([\chi-\theta, \infty); [0, \infty)) $ satisfy
$ \dot{\iota}(t)\leq-c\iota(t)+d\max\left\{\iota_t\right\},\; \; t\geq\chi. $ |
If $ c > d > 0 $, then
$ \iota(t)\leq\left[\max\left\{\iota_\chi\right\}\right]\exp\left(-\lambda(t-\chi)\right),\; \; t\geq\chi, $ |
in which $ \max\left\{\iota_t\right\} = \sup_{t-\theta\leq\phi\leq t}\iota(\phi) $, and $ \lambda $ is the smallest positive real root in the following equation
$ c-\lambda-de^{\lambda\theta} = 0. $ |
Lemma 2.5 ([37]). For $ t\in(-\theta, +\infty) $, if function $ \iota\geq 0 $ meets
$ \dot{\iota}(t)\leq a_1\iota(t)+a_2\iota(t-\theta),\; a_1,a_2 > 0,\; t\geq 0, $ |
it follows that
$ \iota(t)\leq\vert\vert\iota(0)\vert\vert \exp\left((a_1+a_2)t\right)\!. $ |
In this section, sufficient conditions for FTS and FTCS of system (2.3) are obtained through EAPIC.
Theorem 3.1. Suppose that Assumptions 2.1 and 2.2 hold, there exists symmetric and positive matrix $ \mathcal{P} $, positive constants $ \lambda, \delta_1, \delta_2, \mathcal{T}, \rho, \pi, \mu_1, \mu_2, \gamma_1, \gamma_2, \gamma_3, \gamma_4, \alpha_1, \alpha_2, \eta_1, \eta_2, \vartheta $, where $ \rho < \delta_1 < \delta_2, \pi\in(0, \mathcal{T}), \mu_1\leq\mu_2, $ and $ \vartheta\geq1 $ to satisfy
$ (A)\; \mu_1\mathcal{I}\leq\mathcal{P}\leq\mu_2\mathcal{I}; $
$ (B)\; (−ϑPDTPMT4∗−P+γ4PFFTP0∗∗−γ4I)
$ (C)\; (˜ψ1PB∗−α1I+γ−12MT2M2)
$ \tilde{\psi}_1 = \mathcal{P} \mathcal{A}+ \mathcal{A}^T\mathcal{P}+\mathcal{P} \mathcal{C} \mathcal{H}+ \mathcal{H}^T \mathcal{C}^T\mathcal{P}+(\gamma_1+\gamma_2+\gamma_3)\mathcal{P} \mathcal{N} \mathcal{N}^T\mathcal{P}+\gamma_1^{-1} \mathcal{M}_1^T \mathcal{M}_1+\gamma_3^{-1} \mathcal{H}^T \mathcal{M}_3^T \mathcal{M}_3 \mathcal{H}+\eta_1\mathcal{P}; $ |
$ (D)\; (˜ψ2PB∗−α2I+γ−12MT2M2)
$ \tilde{\psi}_2 = \mathcal{P} \mathcal{A}+ \mathcal{A}^T\mathcal{P}+(\gamma_1+\gamma_2)\mathcal{P} \mathcal{N} \mathcal{N}^T\mathcal{P}+\gamma_1^{-1} \mathcal{M}_1^T \mathcal{M}_1-\eta_2\mathcal{P}; $ |
$ (E)\; \frac{\zeta}{\lambda}\leq\bar{\tau}\leq\tau_k\leq\omega-\frac{\zeta}{\eta_2+h_2}; $
$ (F)\; n(0, t)\ln\vartheta-\lambda(\sum_{i = 0}^{n(0, t)}\tau_i)+(n(0, t)+1)\zeta\leq\ln(\mu_1\delta_2^2)-\ln(\mu_2\delta_1^2), \; \; t\in[0, \mathcal{T}] $,
in which $ \lambda $ is the smallest positive solution of the formula $ \eta_1-\lambda-h_1e^{\lambda\theta} = 0 $ and $ \eta_1 > h_1 $. $ \bar{\tau} = \liminf\frac{\sum_{i = 0}^{k-1}\tau_i}{k} $ denotes the minimum average control width. $ n(0, t) $ represents the control period count on $ (0, \mathcal{T}], \; \mathcal{T}\neq t_k $. Thus, the Zeno phenomenon is ruled out, and system (2.3) is FTS w.r.t $ (\mathcal{T}, \delta_1, \delta_2) $ under APIC (2.2). Besides, for $ t\in[\mathcal{T}-\pi, \mathcal{T}] $, if the following relation.
$ (G)\; n(0, t)\ln\vartheta-\lambda(\sum_{i = 0}^{n(0, t)}\tau_i)+(n(0, t)+1)\zeta\leq\ln(\mu_1\rho^2)-\ln(\mu_2\delta_1^2) $ holds, then system (2.3) is FTCS w.r.t $ (\mathcal{T}, \delta_1, \delta_2, \rho, \pi) $ under APIC (2.2).
Proof. For $ 0 < \vert\vert\iota(0)\vert\vert\leq\delta_1 $, assume that $ \iota(t) = \iota(t, 0, \varsigma) $ is a solution of system (2.3) via $ (0, \varsigma) $. Consider the Lyapunov function as follows:
$ \mathcal{U}(\iota(t)) = \iota^T(t) \mathcal{P}\iota(t). $ |
From $ (B) $ in Theorem 3.1, it follows that
$ -\vartheta \mathcal{P}+ \mathcal{D}^T( \mathcal{P}^{-1}-\gamma_4 \mathcal{F} \mathcal{F}^T)^{-1} \mathcal{D}+\gamma_4^{-1} \mathcal{M}_4^T \mathcal{M}_4\leq 0. $ |
For $ t = t_k $, by using Lemma 2.1, we obtain
$ U(ι(tk))=ιT(tk)Pι(tk)=ιT(t−k)DT(tk)PD(tk)ι(t−k)=ιT(t−k)(D+ΔD(tk))TP(D+ΔD(tk))ι(t−k)=ιT(t−k)(D+FK2(t)M4)TP(D+FK2(t)M4)ι(t−k)≤ιT(t−k)[DT(P−1−γ4FFT)−1D+γ−14MT4M4]ι(t−k)≤ϑU(ι(t−k)). $
|
(3.1) |
For $ t\neq t_k $, when $ t\in[t_k, t_k+\tau_k) $, we obtain
$ D+U(ι(t))=2ιT(t)P˙ι(t)=2ιT(t)P((A(t)+C(t)H)ι(t)+B(t)σ(ι(t−θ)))=2ιT(t)P((A+ΔA(t)+CH+ΔC(t)H)ι(t)+(B+ΔB(t))σ(ι(t−θ)))=ιT(t)(PA+ATP+PCH+HTCTP)ι(t)+2ιT(t)PΔA(t)ι(t)+2ιT(t)PΔC(t)Hι(t)+2ιT(t)PBσ(ι(t−θ))+2ιT(t)PΔB(t)σ(ι(t−θ)). $
|
(3.2) |
Next, together with Assumption 2.2, Lemma 2.2, and Lemma 2.3 to obtain
$ 2ιT(t)PΔA(t)ι(t)=2ιT(t)PNK1(t)M1ι(t)=ιT(t)(PNK1(t)M1+MT1KT1(t)NTPT)ι(t)≤ιT(t)(γ1PNNTP+γ−11MT1M1)ι(t), $
|
(3.3) |
$ 2ιT(t)PΔB(t)σ(ι(t−θ))=2ιT(t)PNK1(t)M2σ(ι(t−θ))≤ιT(t)γ2PNNTPι(t)+σT(ι(t−θ))γ−12MT2M2σ(ι(t−θ)), $
|
(3.4) |
$ 2ιT(t)PΔC(t)Hι(t)=2ιT(t)PNK1(t)M3Hι(t)=ιT(t)(PNK1(t)M3H+HTMT3KT1(t)NTPT)ι(t)≤ιT(t)(γ3PNNTP+γ−13HTMT3M3H)ι(t). $
|
(3.5) |
Bringing (3.3)–(3.5) into (3.2), we have, according to $ (C) $ in Theorem 3.1, that
$ D+U(ι(t))≤ιT(t)[PA+ATP+PCH+HTCTP+(γ1+γ2+γ3)PNNTP+γ−11MT1M1+γ−13HTMT1M1H]ι(t)+2ιT(t)PBσ(ι(t−θ))+σT(ι(t−θ))γ−12MT2M2σ(ι(t−θ))≤(ιT(t)σT(ι(t−θ)))(˜ψ1PB∗−α1I+γ−12MT2M2)(ι(t)σ(ι(t−θ)))−η1ιT(t)Pι(t)+α1σT(ι(t−θ)σ(ι(t−θ)≤−η1U(ι(t))+h1U(ι(t−θ)), $
|
where $ h_1 = \alpha_1\varpi_{\sigma}\lambda_{max}(\mathcal{P}^{-1}) $ with $ \varpi_{\sigma} = \max\left\{\varpi_1^2, \varpi_2^2, \dots, \varpi_n^2\right\} $. For $ t\in[t_k, t_k+\tau_k) $, by Lemma 2.4, one has
$ U(ι(t))≤U(ι(tk))exp(−λ(t−tk)). $
|
(3.6) |
Similarly, for $ t\in[t_k+\tau_k, t_{k+1}) $, it follows that
$ D+U(ι(t))≤ιT(t)[PA+ATP+(γ1+γ2)PNNTP+γ−11MT1M1]ι(t)+2ιT(t)PBσ(ι(t−θ))+σT(ι(t−θ))γ−12MT2M2σ(ι(t−θ))≤(ιT(t)σT(ι(t−θ)))(˜ψ2PB∗−α2I+γ−12MT2M2)(ι(t)σ(ι(t−θ)))+η2ιT(t)Pι(t)+α2σT(ι(t−θ))σ(ι(t−θ))≤η2U(ι(t))+h2U(ι(t−θ)), $
|
where $ h_2 = \alpha_2\varpi_{\sigma}\lambda_{max}(\mathcal{P}^{-1}) $. Based on Lemma 2.5, when $ t\in[t_k+\tau_k, t_{k+1}) $, we obtain
$ U(ι(t))≤U(ι(tk+τk))exp((η2+h2)(t−tk−τk)). $
|
(3.7) |
Thus,
$ U(ι(tk+1))≤U(ι(tk+τk))exp((η2+h2)(tk+1−tk−τk)). $
|
(3.8) |
If $ \Gamma_k(t_k+\tau_k, t_k+\omega]\neq\emptyset $, then $ t_{k+1}-t_k\leq\omega $ and only when the event occurrence function to be zero will trigger the next event, namely
$ U(ι(tk+1))=eζU(ι(tk+τk)). $
|
(3.9) |
Consequently, together with (3.8) and (3.9), we derive
$ τk+ζη2+h2≤tk+1−tk≤ω. $
|
(3.10) |
If $ \Gamma_k(t_k+\tau_k, t_k+\omega] = \emptyset $, then $ t_{k+1}-t_k = \omega $, (3.10) still stands. Because $ \frac{\zeta}{\eta_2+h_2} > 0 $, combining (3.10) with $ (E) $ in Theorem 3.1, it follows that
$ 0<(1λ+1η2+h2)ζ≤tk+1−tk≤ω. $
|
(3.11) |
Therefore, the Zeno behavior of system (2.3) is excluded.
For $ t\in[t_k+\tau_k, t_{k+1}) $, by ETM (2.4), we obtain
$ U(ι(t))≤eζU(ι(tk+τk)). $
|
(3.12) |
When $ t\in[0, \tau_0) $, by (3.6), it is obtained that
$ \mathcal{U}(\iota(t))\leq \mathcal{U}(\iota(0))\exp\left(-\lambda t\right)\! $ |
and
$ \mathcal{U}(\iota(\tau_0))\leq \mathcal{U}(\iota(0))\exp\left(-\lambda \tau_0\right)\!. $ |
When $ t\in[\tau_0, t_1) $, based on (3.12), one obtains
$ \mathcal{U}(\iota(t))\leq e^{\zeta} \mathcal{U}(\iota(\tau_0))\leq \mathcal{U}(\iota(0))\exp(\zeta-\lambda\tau_0), $ |
and
$ \mathcal{U}(\iota(t_1^-))\leq \mathcal{U}(\iota(0))\exp(\zeta-\lambda\tau_0). $ |
When $ t\in[t_1, t_1+\tau_1) $, it follows from (3.1) and (3.6) that
$ \mathcal{U}(\iota(t))\leq \mathcal{U}(\iota(t_1))\exp\left(-\lambda(t-t_1)\right)\leq\vartheta \mathcal{U}(\iota(t_1^-))\exp\left(-\lambda(t-t_1)\right)\leq\vartheta \mathcal{U}(\iota(0))\exp\left(\zeta-\lambda(\tau_0+t-t_1)\right)\!, $ |
and
$ \mathcal{U}(\iota(t_1+\tau_1))\leq\vartheta \mathcal{U}(\iota(0))\exp\left(\zeta-\lambda(\tau_0+\tau_1)\right)\!. $ |
When $ t\in[t_1+\tau_1, t_2) $, we obtain
$ \mathcal{U}(\iota(t))\leq e^\zeta \mathcal{U}(\iota(t_1+\tau_1))\leq\vartheta \mathcal{U}(\iota(0))\exp\left(2\zeta-\lambda(\tau_0+\tau_1)\right)\!, $ |
and
$ \mathcal{U}(\iota(t_2^-))\leq\vartheta \mathcal{U}(\iota(0))\exp\left(2\zeta-\lambda(\tau_0+\tau_1)\right)\!. $ |
When $ t\in[t_2, t_2+\tau_2) $, one has
$ U(ι(t))≤U(ι(t2))exp(−λ(t−t2))≤ϑU(ι(t−2))exp(−λ(t−t2))≤ϑ2U(ι(0))exp(2ζ−λ(τ0+τ1+t−t2)), $
|
and
$ \mathcal{U}(\iota(t_2+\tau_2))\leq\vartheta^2 \mathcal{U}(\iota(0))\exp\left(2\zeta-\lambda(\tau_0+\tau_1+t-t_2)\right)\!. $ |
When $ t\in[t_2+\tau_2, t_3) $, there holds
$ \mathcal{U}(\iota(t))\leq e^\zeta \mathcal{U}(\iota(t_2+\tau_2))\leq\vartheta^2 \mathcal{U}(\iota(0))\exp\left(3\zeta-\lambda(\tau_0+\tau_1+\tau_2)\right)\!. $ |
By mathematical induction, when $ t\in[t_k+\tau_k, t_{k+1}) $, one obtains
$ \mathcal{U}(\iota(t))\leq\vartheta^k \mathcal{U}(\iota(0))\exp\left((k+1)\zeta-\lambda(\sum\limits_{i = 0}^{k}\tau_i)\right) = \mathcal{U}(\iota(0))\exp\left(k\ln\vartheta-\lambda(\sum\limits_{i = 0}^{k}\tau_i)+(k+1)\zeta\right), $ |
which means
$ U(ι(t))≤U(ι(0))exp(n(0,t)lnϑ−λ(n(0,t)∑i=0τi)+(n(0,t)+1)ζ),t∈[0,T]. $
|
(3.13) |
Using $ (A) $ and $ (F) $ in Theorem 3.1, we derive
$ μ1||ι(t)||2≤U(ι(t))≤μ2||ι(0)||2U(ι(0))exp(n(0,t)lnϑ−λ(n(0,t)∑i=0τi)+(n(0,t)+1)ζ)≤μ2δ21exp(n(0,t)lnϑ−λ(n(0,t)∑i=0τi)+(n(0,t)+1)ζ)≤μ1δ22, $
|
which implies $ \vert\vert\iota(t)\vert\vert\leq\delta_2 $, and hence, $ \vert\vert\iota(t)\vert\vert\leq\delta_2 $ holds on $ [0, \mathcal{T}] $. So, system (2.3) can achieve FTS under APIC (2.2). When $ t\in[\mathcal{T}-\pi, \mathcal{T}] $, if $ (G) $ in Theorem 3.1 is satisfied, it follows from (3.13) that
$ μ1||ι(t)||2≤U(ι(t))≤μ2||ι(0)||2U(ι(0))exp(n(0,t)lnϑ−λ(n(0,t)∑i=0τi)+(n(0,t)+1)ζ)≤μ2δ21exp(n(0,t)lnϑ−λ(n(0,t)∑i=0τi)+(n(0,t)+1)ζ)≤μ1ρ2, $
|
which shows that $ \vert\vert\iota(t)\vert\vert\leq\rho $ for all $ t\in[\mathcal{T}-\pi, \mathcal{T}] $. Then, system (2.3) can achieve FTCS under APIC (2.2).
Remark 3.1. It is important to note that conditions $ (C) $ and $ (D) $ in Theorem 3.1 regulate the continuous dynamics so that (3.6) and (3.7) hold, where the parameters $ \eta_1 $ and $ \eta_2 $ imply the decay rate and potential growth for system (2.3), respectively. This means that system (2.3) shows a decreasing trend in the control intervals, which makes system (2.3) to some extent divergent in the resting intervals. Furthermore, $ \vartheta\geq 1 $ in condition $ (B) $ of Theorem 3.1 suggests that impulses may have destabilizing effects.
As a matter of fact, when $ \tau_k = \tau, t_{k+1}-t_k-\tau = c $ with $ \tau, c > 0 $, APIC (2.2) can be turned into PIC as follows:
$ u(t)={Hι(t),kJ≤t<kJ+τ,0,kJ+τ≤t<(k+1)J, $
|
(3.14) |
where $ \mathcal{J} > 0 $ denotes the control period as well as $ 0 < \tau < \mathcal{J} $ indicates the control width, then the following corollary is obvious.
Corollary 3.1. Suppose that Assumptions 2.1 and 2.2 hold, there exists symmetric and positive matrix $ \mathcal{P} $, positive constants $ \lambda, \delta_1, \delta_2, \mathcal{T}, \rho, \pi, \mu_1, \mu_2, \gamma_1, \gamma_2, \gamma_3, \gamma_4, \alpha_1, \alpha_2, \eta_1, \eta_2, \vartheta $, where $ \rho < \delta_1 < \delta_2, \pi\in(0, \mathcal{T}), \mu_1\leq\mu_2, $ and $ \vartheta\geq1 $ to satisfy $ (A)-(D) $ in Theorem 3.1, and for any $ t\in[0, \mathcal{T}] $, if the following relation
$ n(0,t)\left(\ln\vartheta+(\eta_2+h_2)c-\lambda c\right)\leq\ln(\mu_1\delta_2^2)-\ln(\mu_2\delta_1^2)-(\eta_2+h_2)c $ |
holds, then system (2.3) is FTS w.r.t $ (\mathcal{T}, \delta_1, \delta_2) $ under PIC (3.14). Besides, for $ t\in[\mathcal{T}-\pi, \mathcal{T}] $, if
$ n(0,t)\left(\ln\vartheta+(\eta_2+h_2)c-\lambda c\right)\leq\ln(\mu_1\rho^2)-\ln(\mu_2\delta_1^2)-(\eta_2+h_2)c $ |
holds, then system (2.3) is FTCS w.r.t $ (\mathcal{T}, \delta_1, \delta_2, \rho, \pi) $ under PIC (3.14).
Remark 3.2. It should be noted that (3.14), as a special case of (2.2), is time-triggered, meanwhile, both $ \mathcal{J} $ and $ \tau $ are fixed.
Next, when parameter uncertainties $ \Delta \mathcal{A}(t) = \Delta \mathcal{B}(t) = \Delta \mathcal{C}(t) = \Delta \mathcal{D}(t) = 0 $, the uncertain system (2.3) is transformed into the following deterministic system:
$ {˙ι(t)=Aι(t)+Bσ(ι(t−τ))+Cu(t),t≠tk,t≥0,ι(t)=Dι(t−),t=tk,ι(t)=ς(t),t∈[−θ,0]. $
|
(3.15) |
Corollary 3.2. Suppose that Assumption 2.1 holds and there exists symmetric positive matrices $ \mathcal{P} $, positive constants $ \lambda, \delta_1, \delta_2, \mathcal{T}, \rho, \pi, \mu_1, \mu_2, \alpha_1, \alpha_2, \eta_1, \eta_2, \vartheta $, where $ \rho < \delta_1 < \delta_2, \pi\in(0, \mathcal{T}), \mu_1\leq\mu_2, $ and $ \vartheta\geq1 $ such that $ (A) $ in Theorem 3.1 and
$ (B')\; (−ϑPDTP∗−P)
$ (C')\; (˜ψ1PB∗−α1I)
$ (D')\; (˜ψ2PB∗−α2I)
$ (E')\; \frac{\zeta}{\lambda}\leq\bar{\tau}\leq\tau_k\leq\omega-\frac{\zeta}{\eta_2+h_2}; $
hold, then system (3.15) is FTS w.r.t $ (\mathcal{T}, \delta_1, \delta_2) $ under APIC (2.2) if $ (F) $ in Theorem 3.1 is true and the Zeno phenomenon is excluded. Furthermore, system (3.15) is FTCS w.r.t $ (\mathcal{T}, \delta_1, \delta_2, \rho, \pi) $ under APIC (2.2) if $ (G) $ in Theorem 3.1 is satisfied when $ t\in[\mathcal{T}-\pi, \mathcal{T}] $.
The validity of the theoretical results will be verified by two numerical examples.
Example 4.1. Consider the uncertain system as follows:
$ ˙ι(t)=A(t)ι(t)+B(t)σ(ι(t−θ)) $
|
(4.1) |
on $ t\geq 0 $, where
$ A=(−0.700−0.8),B=(1.8002), $
|
$ σ(ι(t−θ))=(0.3(|0.85ι1(t)+2|−|0.85ι1(t)−2|)0.3(|0.85ι2(t−θ)+2|−|0.85ι2(t−θ)−2|)), $
|
$ θ=0.5,ΔA(t)=ΔB(t)=(0.1sin(0.5t)000.1sin(0.5t)). $
|
Consider the initial condition $ \iota(0) = (1.05, 0.6)^{T} $, parameters $ \delta_1 = 1.21, \delta_2 = 2 $, and $ \mathcal{T} = 9.5 $. Based on the state trajectories of system (4.1) shown in Figure 4, we know that system (4.1) is not FTS w.r.t (9.5, 1.21, 2).
When APIC $ u(t) $ and impulsive disturbance are accounted for, system (4.1) can be rewritten as
$ {˙ι(t)=A(t)ι(t)+B(t)σ(ι(t−τ))+C(t)u(t),t≠tk,t≥0,ι(t)=D(t)ι(t−),t=tk,ι(t)=ς(t),t∈[−θ,0], $
|
(4.2) |
where
$ \mathcal{C} = (0.10.1) , \; \; \mathcal{D} = (1.20.10.11.2) ,\; \; \Delta\mathcal{C}(t) = (0.1sin(0.5t)0) , $
|
$ \Delta\mathcal{D}(t) = (0.1sin(0.5t)000.1sin(0.5t)) $
|
and
$ u(t)={Hι(t),tk≤t<tk+τk,0,tk+τk≤t<tk+1. $
|
(4.3) |
Let $ \varpi_1 = \varpi_2 = 0.51, \mathcal{N} = \mathcal{F} = \mathcal{I}_2, \mathcal{M}_1 = \mathcal{M}_2 = \mathcal{M}_4 = 0.1\mathcal{I}_2, \mathcal{M}_3 = (0.1;0), \mathcal{K}_i(t) = (\sin(0.5t), 0;0, \sin(0.5t)) $ and $ \mathcal{K}_i^{T}(t) \mathcal{K}_i(t)\leq\mathcal{I}_2\; (i = 1, 2) $, in which case Assumptions 2.1 and 2.2 hold.
Assume that $ \mu_1 = 5.2, \mu_2 = 5.5, \vartheta = 2, \gamma_1 = 0.0425, \gamma_2 = 0.043, \gamma_3 = 0.0358$, $\gamma_4 = 0.018, \alpha_1 = 1.8266, \alpha_2 = 2.3013, \eta_1 = 0.3665, \eta_2 = 1.1 $. Under conditions $ (A)-(D) $ of Theorem 3.1, it can be obtained by using the MATLAB toolbox
$ \mathcal{P} = (5.35−0.0018−0.00185.35) ,\; \; \mathcal{H} = (−1.864−1.864) \!. $
|
Additionally, by calculating, we can obtain $ h_1 = 0.0888, h_2 = 0.1119 $, and $ \lambda = 0.2651 $.
Design $ \omega = 5, \zeta = 0.1 $ as well as $ \tau_k = 3.5 $, then the intermittent sequence $ \left\{t_k\right\} $ is decided by the following ETM
$ tk+1={inf{t:t∈Γk(tk+3.5,tk+5]},ifΓk(tk+3.5,tk+5]≠∅,tk+5,ifΓk(tk+3.5,tk+5]=∅, $
|
(4.4) |
where
$ \Gamma_k(\varepsilon,t] = \left\{t: t > \varepsilon+3.5, \mathcal{U}(\iota(t))\geq e^{0.1} \mathcal{U}(\iota(\varepsilon+3.5))\right\}, $ |
and $ \mathcal{U}(\iota(t)) = \iota^{T}(t) \mathcal{P}\iota(t) $. Obviously, the condition $ (E) $ in Theorem 3.1 is satisfied. According to Figure 5, we can obtain the intermittent times as $ t_1 = 3.6359, t_2 = 7.4563 $. Furthermore, when $ t\in[0, 9.5] $, we have that the intermittent sequences $ \left\{t_k\right\} $ and control span $ \tau_k $ meet
$ 0.6931k-0.9279k+0.1(k+1)\leq0.949,\quad k = 0,1,2. $ |
By Theorem 3.1, we can conclude that system (4.2) is FTS w.r.t (9.5, 1.21, 2) under APIC (4.3). Moreover, take $ \pi = 2, \rho = 1.15 $. When $ t\in\left[7.5, 9.5\right] $, the intermittent sequences $ \left\{t_k\right\} $ and control span $ \tau_k $ satisfy
$ 0.6931k-0.9279k+0.1(k+1)\leq-0.1578,\quad k = 2. $ |
Based on Theorem 3.1, we can deduce that system (4.2) is FTCS w.r.t (9.5, 1.21, 2, 1.15, 2) under APIC (4.3). The relevant simulation results for $ \iota(0) = (1.05, 0.6)^T $ are displayed in Figure 5, where Figure 5(a) portrays the trajectories of $ \iota_1, \iota_2 $; Figure 5(b, c) shows the trajectories of $ \vert\vert\iota(t)\vert\vert $ and $ u(t) $, respectively.
Example 4.2. Let us consider the two degrees of freedom damped dual-mass spring system illustrated in Figure 6, which has the following mathematical expression:
$ {m1¨ι1(t)=σ1+u+k2(ι2−ι1)+c2(˙ι2−˙ι1)−k1ι1−c1˙ι1,m2¨ι2(t)=σ2−k2(ι2−ι1)−c2(˙ι2−˙ι1), $
|
(4.5) |
where $ m_1, m_2\in \mathcal{R}_+ $ denote two masses, $ \iota_1, \iota_2\in \mathcal{R}_+ $ represent displacements of masses $ m_1, m_2 $, $ k_1, k_2\in \mathcal{R}_+ $ indicate elasticity coefficients of two springs, $ c_1, c_2\in \mathcal{R}_+ $ signify damping coefficients of two dampers, as well as $ u $ stands for the control input as shown in (2.2). Setting $ \dot{\iota}_1 = \iota_3, \dot{\iota}_2 = \iota_4 $, and $ \iota = (\iota_1, \iota_2, \iota_3, \iota_4)^T $, then we obtain
$ ˙ι=(00100001−k1+k2m1k2m1−c1+c2m1c2m1k2m2−k2m2c2m2−c2m2)ι+(00000000001m100001m2)(00σ1σ2)+(001m10)u. $
|
(4.6) |
In real applications, the damped dual-mass spring system may experience transient disturbances in the control mass $ m_1 $ caused by input noise and uncertainty. We treat such transient disturbances as impulsive disturbances that induce an instantaneous change in velocity, namely, $ \iota(t_k) = \mathcal{D}\iota(t_k^-) $, where $ \mathcal{D} = diag\left\{1, 1, d, 1\right\} $. Moreover, the sequence of impulsive moments is given by ETM (4.4). Assume that $ \sigma_1 = \sin(0.1\iota_1(t)), \sigma_2 = \sin(0.1\iota_2(t-\theta)) $, where $ \theta = 0.2 $. Let $ \mu_1 = 1, \mu_2 = 3, \eta_1 = 0.6513, \eta_2 = 1.961, \vartheta = 1.8$, $\alpha_1 = 1.6371, \alpha_2 = 1.5088, k_1 = 2.5, k_2 = 2$, $m_1 = 3.5, m_2 = 1.2, c_1 = 0.5, c_2 = 3, d = 1.3. $ According to $ (A), (C')-(D') $ in Corollary 3.2, using the MATLAB toolbox, one has
$ \mathcal{P} = (1.992−0.14150.7554−0.2287−0.14151.67−0.017540.56890.7554−0.017541.684−0.1489−0.22870.5689−0.14891.59) , \mathcal{H} = (−2.005−4.391−5.702−9.21) . $
|
By further calculations, it follows that $ h_1 = 0.0159, h_2 = 0.0147, $ as well as $ \lambda = 0.6333 $. Therefore, $ (E') $ in Corollary 3.2 holds. As can be seen from Figure 7, the impulsive instants are $ t_1 = 4.6267, t_2 = 9.6267 $. Let $ \mathcal{T} = 6, \delta_1 = 2.17, \delta_2 = 4, \rho = 1.85, \pi = 1.2 $, and the initial value is $ \iota_0 = (1.8, 0.8, -0.1, -0.9) $. When $ t\in[0, 6] $, we have that the impulsive sequences $ \left\{t_k\right\} $ and control span $ \tau_k $ meet
$ 0.5789k-2.2165k+0.1(k+1)\leq0.1245,\; \; \; \; k = 0,1. $ |
From Corollary 3.2, we can deduce that under APIC (2.2), system (4.6) is FTS w.r.t (6, 2.17, 4). Moreover, when $ t\in\left[4.8, 6\right] $, the impulsive sequences $ \left\{t_k\right\} $ and control span $ \tau_k $ satisfy
$ 0.5789k-2.2165k+0.1(k+1)\leq-1.4176,\; \; \; \; k = 1. $ |
According to Corollary 3.2, we can infer that system (4.6) is FTCS w.r.t (6, 2.17, 4, 1.85, 1.2) under APIC (2.2). The associated simulation results are exhibited in Figure 7.
Remark 4.1. Without considering the control input $ u $ and impulsive disturbance, it can be seen from Figure 8 that system (4.6) is not FTCS w.r.t (6, 2.17, 4, 1.85, 1.2).
Due to traditional PIC methods, which execute control actions at fixed time intervals regardless of system state changes, inefficiencies may arise. To overcome these restrictions, this paper proposes the EAPIC method by combining APIC with EC. Through this approach, the FTS and FTCS of parameter-uncertain systems with impulsive disturbance are investigated. With the aid of Lyapunov methods and LMI techniques, criteria for FTS and FTCS of parameter uncertain systems are derived. Additionally, under EAPIC conditions, relationships among impulsive disturbance, intermittent control parameters, and ETM threshold are established. The sequence of impulsive instants is determined by predefined ETM, and the Zeno phenomenon is ruled out. In future work, we will focus on how to incorporate external disturbances, such as random noise, into the EAPIC system. We plan to adapt the existing event-triggered control strategy to enhance the robustness of the system to these disturbances and ensure that the control strategy remains effective under various disturbance conditions.
Tian Xu: Writing-original draft; Jin-E Zhang: Supervision, writing-review & editing. Both authors have read and approved the final version of the manuscript for publication.
The authors would like to thank the editors and referees for their very helpful comments and suggestions.
The authors declare that there are no conflicts of interest.
[1] |
Cryan JF, Dinan TG (2012) Mind-altering microorganisms: The impact of the gut microbiota on brain and behaviour. Nat Rev Neurosci 13: 701–712. doi: 10.1038/nrn3346
![]() |
[2] |
Borre YE, Moloney RD, Clarke G, et al. (2014) The impact of microbiota on brain and behavior: Mechanisms & therapeutic potential. Adv Exp Med Biol 817: 373–403. doi: 10.1007/978-1-4939-0897-4_17
![]() |
[3] | Diagnostic and Statistical Manual of Mental Disorders (DSM-V), 2013. American Psychiatric Association, 189: 4189. |
[4] |
Siniscalco D, Cirillo A, Bradstreet JJ, et al. (2013) Epigenetic findings in autism: New perspectives for therapy. Int J Environ Res Public Health 10: 4261–4273. doi: 10.3390/ijerph10094261
![]() |
[5] | Frye RE, Slattery J, Macfabe DF, et al. (2015) Approaches to studying and manipulating the enteric microbiome to improve autism symptoms. Microb Ecol Health Dis 26: 26878. |
[6] | De Magistris L, Picardi A, Siniscalco D, et al. (2013) Antibodies against food antigens in patients with autistic spectrum disorders. BioMed Res Int 2013: 729349. |
[7] |
Mcelhanon BO, Mccracken C, Karpen S, et al. (2014) Gastrointestinal symptoms in autism spectrum disorder: A meta-analysis. Pediatrics 133: 872–883. doi: 10.1542/peds.2013-3995
![]() |
[8] |
Adams JB, Johansen LJ, Powell LD, et al. (2011) Gastrointestinal flora and gastrointestinal status in children with autism-comparisons to typical children and correlation with autism severity. BMC Gastroenterol 11: 22. doi: 10.1186/1471-230X-11-22
![]() |
[9] |
Nikolov RN, Bearss KE, Lettinga J, et al. (2009) Gastrointestinal symptoms in a sample of children with pervasive developmental disorders. J Autism Dev Disord 39: 405–413. doi: 10.1007/s10803-008-0637-8
![]() |
[10] |
Iovene MR, Bombace F, Maresca R, et al. (2017) Intestinal dysbiosis and Yeast Isolation in Stool of Subjects with Autism Spectrum Disorders. Mycopathologia 182: 349–363. doi: 10.1007/s11046-016-0068-6
![]() |
[11] | Siniscalco D (2014) Gut bacteria-brain axis in autism. Autism 4: e124. |
[12] |
Kranich J, Maslowski KM, Mackay CR (2011) Commensal flora and the regulation of inflammatory and autoimmune responses. Semin Immunol 23: 139–145. doi: 10.1016/j.smim.2011.01.011
![]() |
[13] |
Macfarlane S, Macfarlane GT (2006) Composition and metabolic activities of bacterial biofilms colonizing food residues in the human gut. Appl Environ Microbiol 72: 6204–6211. doi: 10.1128/AEM.00754-06
![]() |
[14] |
Liu Y, Fatheree NY, Mangalat N, et al. (2010) Human-derived probiotic Lactobacillus reuteri strains differentially reduce intestinal inflammation. Am J Physiol Gastrointest Liver Physiol 299: G1087–G1096. doi: 10.1152/ajpgi.00124.2010
![]() |
[15] | Fateh R, Iravani S, Frootan M, et al. (2011) Synbiotic preparation in men suffering from functional constipation: A randomised controlled trial. Swiss Med Wkly 141: w13239. |
[16] |
Vuong HE, Hsiao EY (2017) Emerging Roles for the Gut Microbiome in Autism Spectrum Disorder. Biol Psychiatry 81: 411–423. doi: 10.1016/j.biopsych.2016.08.024
![]() |
[17] |
Strati F, Cavalieri D, Albanese D, et al. (2017) New evidences on the altered gut microbiota in autism spectrum disorders. Microbiome 5: 24. doi: 10.1186/s40168-017-0242-1
![]() |
[18] |
Finegold SM, Molitoris D, Song Y, et al. (2002) Gastrointestinal microflora studies in late-onset autism. Clin Infect Dis 35: S6–S16. doi: 10.1086/341914
![]() |
[19] | Wang L, Christophersen CT, Sorich MJ, et al. (2013) Increased abundance of Sutterella spp. and Ruminococcus torques in feces of children with autism spectrum disorder. Mol Autism 4: 42. |
[20] | Williams BL, Hornig M, Parekh T, et al. (2012) Application of novel PCR-based methods for detection, quantitation, and phylogenetic characterization of Sutterella species in intestinal biopsy samples from children with autism and gastrointestinal disturbances. MBio 3: 54–64. |
[21] |
Kang DW, Park JG, Ilhan ZE, et al. (2013) Reduced incidence of Prevotella and other fermenters in intestinal microflora of autistic children. PLoS One 8: e68322. doi: 10.1371/journal.pone.0068322
![]() |
[22] |
Ding HT, Taur Y, Walkup JT (2017) Gut microbiota and autism: Key concepts and findings. J Autism Dev Disord 47: 480–489. doi: 10.1007/s10803-016-2960-9
![]() |
[23] |
Frye RE, Nankova B, Bhattacharyya S, et al. (2017) Modulation of immunological pathways in autistic and neurotypical lymphoblastoid cell lines by the enteric microbiome metabolite propionic acid. Front Immunol 8: 1670. doi: 10.3389/fimmu.2017.01670
![]() |
[24] | Shaw W (2010) Increased urinary excretion of a 3-(3-hydroxyphenyl)-3-hydroxypropionic acid (HPHPA), an abnormal phenylalanine metabolite of Clostridia spp. in the gastrointestinal tract, in urine samples from patients with autism and schizophrenia. Nutr Neurosci 13: 135–143. |
[25] |
Wang L, Yu YM, Zhang YQ, et al. (2018) Hydrogen breath test to detect small intestinal bacterial overgrowth: A prevalence case-control study in autism. Eur Child Adolesc Psychiatry 27: 233–240. doi: 10.1007/s00787-017-1039-2
![]() |
[26] |
Adike A, DiBaise JK (2018) Small Intestinal Bacterial Overgrowth: Nutritional Implications, Diagnosis, and Management. Gastroenterol Clin North Am 47: 193–208. doi: 10.1016/j.gtc.2017.09.008
![]() |
[27] |
House SA, Goodman DC, Weinstein SJ, et al. (2016) Prescription use among children with autism spectrum disorders in Northern New England: Intensity and small area variation. J Pediatr 169: 277–283. doi: 10.1016/j.jpeds.2015.10.027
![]() |
[28] |
Rana SV, Bhardwaj SB (2008) Small intestinal bacterial overgrowth. Scand J Gastroenterol 43: 1030–1037. doi: 10.1080/00365520801947074
![]() |
[29] | Quera PR, Quigley EM, Madrid SAM (2005) Small intestinal bacterial overgrowth. An update. Rev Med Chile 133: 1361–1370. |
[30] |
Gatta L, Scarpignato C (2017) Systematic review with meta-analysis: Rifaximin is effective and safe for the treatment of small intestine bacterial overgrowth. Aliment Pharmacol Ther 45: 604–616. doi: 10.1111/apt.13928
![]() |
[31] | Kwiatkowski L, Rice E, Langland J (2017) Integrative treatment of chronic abdominal bloating and pain associated with overgrowth of small intestinal bacteria: A case report. Altern Ther Health Med 23: 56–61. |
[32] |
Kernien JF, Snarr BD, Sheppard DC, et al. (2018) The interface between fungal biofilms and innate immunity. Front Immunol 8: 1968. doi: 10.3389/fimmu.2017.01968
![]() |
[33] |
Anwar H, Dasgupta MK, Costerton JW (1990) Testing the susceptibility of bacteria in biofilms to antibacterial agents. Antimicrob Agents Chemother 34: 2043–2046. doi: 10.1128/AAC.34.11.2043
![]() |
[34] | Høiby N, Bjarnsholt T, Moser C, et al. (2015) ESCMID guideline for the diagnosis and treatment of biofilm infections 2014. Clin Microbiol Infect 1: 1–25. |
[35] |
Smith A, Buchinsky FJ, Post JC (2011) Eradicating chronic ear, nose, and throat infections: A systematically conducted literature review of advances in biofilm treatment. Otolaryngol Head Neck Surg 144: 338–347. doi: 10.1177/0194599810391620
![]() |
[36] |
Slobodníková L, Fialová S, Rendeková K, et al. (2016) Antibiofilm activity of plant polyphenols. Molecules 21: 1717. doi: 10.3390/molecules21121717
![]() |
[37] |
Li XH, Lee JH (2017) Antibiofilm agents: A new perspective for antimicrobial strategy. J Microbiol 55: 753–766. doi: 10.1007/s12275-017-7274-x
![]() |
[38] |
de Magistris L, Familiari V, Pascotto A, et al. (2010) Alterations of the intestinal barrier in patients with autism spectrum disorders and in their first-degree relatives. J Pediatr Gastroenterol Nutr 51: 418–424. doi: 10.1097/MPG.0b013e3181dcc4a5
![]() |
[39] | Wan MLY, Ling KH, El-Nezami H, et al. (2018) Influence of functional food components on gut health. Crit Rev Food Sci Nutr 2018: 1–10. |
[40] |
Laparra JM, Sanz Y (2010) Interactions of gut microbiota with functional food components and nutraceuticals. Pharmacol Res 61: 219–225. doi: 10.1016/j.phrs.2009.11.001
![]() |