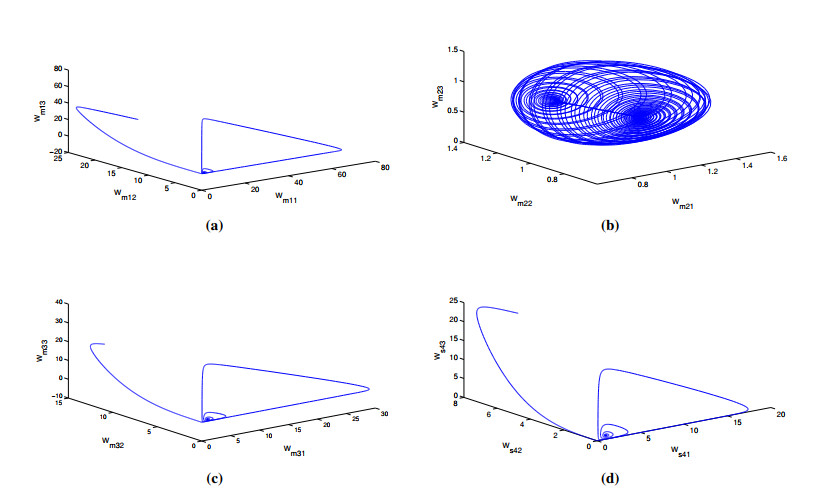
Citation: Chisato Kinoshita, Koji Aoyama, Toshio Nakaki. microRNA as a new agent for regulating neuronal glutathione synthesis and metabolism[J]. AIMS Molecular Science, 2015, 2(2): 124-143. doi: 10.3934/molsci.2015.2.124
[1] | Honglei Wang, Wenliang Zeng, Xiaoling Huang, Zhaoyang Liu, Yanjing Sun, Lin Zhang . MTTLm6A: A multi-task transfer learning approach for base-resolution mRNA m6A site prediction based on an improved transformer. Mathematical Biosciences and Engineering, 2024, 21(1): 272-299. doi: 10.3934/mbe.2024013 |
[2] | Shanzheng Wang, Xinhui Xie, Chao Li, Jun Jia, Changhong Chen . Integrative network analysis of N6 methylation-related genes reveal potential therapeutic targets for spinal cord injury. Mathematical Biosciences and Engineering, 2021, 18(6): 8174-8187. doi: 10.3934/mbe.2021405 |
[3] | Pingping Sun, Yongbing Chen, Bo Liu, Yanxin Gao, Ye Han, Fei He, Jinchao Ji . DeepMRMP: A new predictor for multiple types of RNA modification sites using deep learning. Mathematical Biosciences and Engineering, 2019, 16(6): 6231-6241. doi: 10.3934/mbe.2019310 |
[4] | Yong Zhu, Zhipeng Jiang, Xiaohui Mo, Bo Zhang, Abdullah Al-Dhelaan, Fahad Al-Dhelaan . A study on the design methodology of TAC3 for edge computing. Mathematical Biosciences and Engineering, 2020, 17(5): 4406-4421. doi: 10.3934/mbe.2020243 |
[5] | Atefeh Afsar, Filipe Martins, Bruno M. P. M. Oliveira, Alberto A. Pinto . A fit of CD4+ T cell immune response to an infection by lymphocytic choriomeningitis virus. Mathematical Biosciences and Engineering, 2019, 16(6): 7009-7021. doi: 10.3934/mbe.2019352 |
[6] | Tamás Tekeli, Attila Dénes, Gergely Röst . Adaptive group testing in a compartmental model of COVID-19*. Mathematical Biosciences and Engineering, 2022, 19(11): 11018-11033. doi: 10.3934/mbe.2022513 |
[7] | Wenli Cheng, Jiajia Jiao . An adversarially consensus model of augmented unlabeled data for cardiac image segmentation (CAU+). Mathematical Biosciences and Engineering, 2023, 20(8): 13521-13541. doi: 10.3934/mbe.2023603 |
[8] | Tongmeng Jiang, Pan Jin, Guoxiu Huang, Shi-Cheng Li . The function of guanylate binding protein 3 (GBP3) in human cancers by pan-cancer bioinformatics. Mathematical Biosciences and Engineering, 2023, 20(5): 9511-9529. doi: 10.3934/mbe.2023418 |
[9] | Xin Yu, Jun Liu, Ruiwen Xie, Mengling Chang, Bichun Xu, Yangqing Zhu, Yuancai Xie, Shengli Yang . Construction of a prognostic model for lung squamous cell carcinoma based on seven N6-methylandenosine-related autophagy genes. Mathematical Biosciences and Engineering, 2021, 18(5): 6709-6723. doi: 10.3934/mbe.2021333 |
[10] | Tahir Rasheed, Faran Nabeel, Muhammad Bilal, Yuping Zhao, Muhammad Adeel, Hafiz. M. N. Iqbal . Aqueous monitoring of toxic mercury through a rhodamine-based fluorescent sensor. Mathematical Biosciences and Engineering, 2019, 16(4): 1861-1873. doi: 10.3934/mbe.2019090 |
The constituent members in a system mainly found in nature can be interacting with each other through cooperation and competition. Demonstrations for such systems involve biological species, countries, businesses, and many more. It's very much intriguing to investigate in a comprehensive manner numerous social as well as biological interactions existent in dissimilar species/entities utilizing mathematical modeling. The predation and the competition species are the most famous interactions among all such types of interactions. Importantly, Lotka [1] and Volterra [2] in the 1920s have announced individually the classic equations portraying population dynamics. Such illustrious equations are notably described as predator-prey (PP) equations or Lotka-Volterra (LV) equations. In this structure, PP/LV model represents the most influential model for interacting populations. The interplay between prey and predator together with additional factors has been a prominent topic in mathematical ecology for a long period. Arneodo et al. [3] have established in 1980 that a generalized Lotka-Volterra biological system (GLVBS) would depict chaos phenomena in an ecosystem for some explicitly selected system parameters and initial conditions. Additionally, Samardzija and Greller [4] demonstrated in 1988 that GLVBS would procure chaotic reign from the stabled state via rising fractal torus. LV model was initially developed as a biological concept, yet it is utilized in enormous diversified branches for research [5,6,7,8]. Synchronization essentially is a methodology of having different chaotic systems (non-identical or identical) following exactly a similar trajectory, i.e., the dynamical attributes of the slave system are locked finally into the master system. Specifically, synchronization and control have a wide spectrum for applications in engineering and science, namely, secure communication [9], encryption [10,11], ecological model [12], robotics [13], neural network [14], etc. Recently, numerous types of secure communication approaches have been explored [15,16,17,18] such as chaos modulation [18,19,20,21], chaos shift keying [22,23] and chaos masking [9,17,20,24]. In chaos communication schemes, the typical key idea for transmitting a message through chaotic/hyperchaotic models is that a message signal is nested in the transmitter system/model which originates a chaotic/ disturbed signal. Afterwards, this disturbed signal has been emitted to the receiver through a universal channel. The message signal would finally be recovered by the receiver. A chaotic model has been intrinsically employed both as receiver and transmitter. Consequently, this area of chaotic synchronization & control has sought remarkable considerations among differential research fields.
Most prominently, synchronization theory has been in existence for over $ 30 $ years due to the phenomenal research of Pecora and Carroll [25] established in 1990 using drive-response/master-slave/leader-follower configuration. Consequently, many authors and researchers have started introducing and studying numerous control and synchronization methods [9,26,27,28,29,30,31,32,33,34,35,36] etc. to achieve stabilized chaotic systems for possessing stability. In [37], researchers discussed optimal synchronization issues in similar GLVBSs via optimal control methodology. In [38,39], the researchers studied the adaptive control method (ACM) to synchronize chaotic GLVBSs. Also, researchers [40] introduced a combination difference anti-synchronization scheme in similar chaotic GLVBSs via ACM. In addition, authors [41] investigated a combination synchronization scheme to control chaos existing in GLVBSs using active control strategy (ACS). Bai and Lonngren [42] first proposed ACS in 1997 for synchronizing and controlling chaos found in nonlinear dynamical systems. Furthermore, compound synchronization using ACS was first advocated by Sun et al. [43] in 2013. In [44], authors discussed compound difference anti-synchronization scheme in four chaotic systems out of which two chaotic systems are considered as GLVBSs using ACS and ACM along with applications in secure communications of chaos masking type in 2019. Some further research works [45,46] based on ACS have been reported in this direction. The considered chaotic GLVBS offers a generalization that allows higher-order biological terms. As a result, it may be of interest in cases where biological systems experience cataclysmic changes. Unfortunately, some species will be under competitive pressure in the coming years and decades. This work may be comprised as a step toward preserving as many currently living species as possible by using the proposed synchronization approach which is based on master-slave configuration and Lyapunov stability analysis.
In consideration of the aforementioned discussions and observations, our primary focus here is to develop a systematic approach for investigating compound difference anti-synchronization (CDAS) approach in $ 4 $ similar chaotic GLVBSs via ACS. The considered ACS is a very efficient yet theoretically rigorous approach for controlling chaos found in GLVBSs. Additionally, in view of widely known Lyapunov stability analysis (LSA) [47], we discuss actively designed biological control law & convergence for synchronization errors to attain CDAS synchronized states.
The major attributes for our proposed research in the present manuscript are:
● The proposed CDAS methodology considers four chaotic GLVBSs.
● It outlines a robust CDAS approach based active controller to achieve compound difference anti-synchronization in discussed GLVBSs & conducts oscillation in synchronization errors along with extremely fast convergence.
● The construction of the active control inputs has been executed in a much simplified fashion utilizing LSA & master-salve/ drive-response configuration.
● The proposed CDAS approach in four identical chaotic GLVBSs of integer order utilizing ACS has not yet been analyzed up to now. This depicts the novelty of our proposed research work.
This manuscript is outlined as follows: Section 2 presents the problem formulation of the CDAS scheme. Section 3 designs comprehensively the CDAS scheme using ACS. Section 4 consists of a few structural characteristics of considered GLVBS on which CDAS is investigated. Furthermore, the proper active controllers having nonlinear terms are designed to achieve the proposed CDAS strategy. Moreover, in view of Lyapunov's stability analysis (LSA), we have examined comprehensively the biological controlling laws for achieving global asymptotical stability of the error dynamics for the discussed model. In Section 5, numerical simulations through MATLAB are performed for the illustration of the efficacy and superiority of the given scheme. Lastly, we also have presented some conclusions and the future prospects of the discussed research work in Section 6.
We here formulate a methodology to examine compound difference anti-synchronization (CDAS) scheme viewing master-slave framework in four chaotic systems which would be utilized in the coming up sections.
Let the scaling master system be
$ ˙wm1= f1(wm1), $
|
(2.1) |
and the base second master systems be
$ ˙wm2= f2(wm2), $
|
(2.2) |
$ ˙wm3= f3(wm3). $
|
(2.3) |
Corresponding to the aforementioned master systems, let the slave system be
$ ˙ws4= f4(ws4)+U(wm1,wm2,wm3,ws4), $
|
(2.4) |
where $ w_{m1} = (w_{m11}, w_{m12}, ..., w_{m1n})^T\in R^n $, $ w_{m2} = (w_{m21}, w_{m22}, ..., w_{m2n})^T\in R^n $, $ w_{m3} = (w_{m31}, w_{m32}, ..., w_{m3n})^T\in R^n $, $ w_{s4} = (w_{s41}, w_{s42}, ..., w_{s4n})^T\in R^n $ are the state variables of the respective chaotic systems (2.1)–(2.4), $ f_1, f _2, f_3, f_4: R^n \rightarrow R^n $ are four continuous vector functions, $ U = (U_1, U_2, ..., U_n)^T : R^n\times R^n\times R^n\times R^n \rightarrow R^n $ are appropriately constructed active controllers.
Compound difference anti-synchronization error (CDAS) is defined as
$ E = Sw_{s4}+Pw_{m1}(Rw_{m3}-Qw_{m2}), $ |
where $ P = diag(p_1, p_2, ....., p_n), Q = diag(q_1, q_2, ....., q_n), R = diag(r_1, r_2, ....., r_n), S = diag(s_1, s_2, ....., s_n) $ and $ S\neq 0 $.
Definition: The master chaotic systems (2.1)–(2.3) are said to achieve CDAS with slave chaotic system (2.4) if
$ lim_{t \to \infty} \|E(t)\| = lim _{t \to \infty} \|Sw_{s4}(t)+Pw_{m1}(t)(Rw_{m3}(t)-Qw_{m2}(t))\| = 0. $ |
We now present our proposed CDAS approach in three master systems (2.1)–(2.3) and one slave system (2.4). We next construct the controllers based on CDAS approach by
$ Ui= ηisi−(f4)i−KiEisi, $
|
(3.1) |
where $ \eta_i = p_i{(f_1)}_i({r_iw_{m3i}-q_iw_{m2i}})+p_iw_{m1i} ({r_i{(f_{3})_i-q_i{(f_{2}})_i}}) $, for $ i = 1, 2, ..., n. $
Theorem: The systems (2.1)–(2.4) will attain the investigated CDAS approach globally and asymptotically if the active control functions are constructed in accordance with (3.1).
Proof. Considering the error as
$ Ei= siws4i+piwm1i(riwm3i−qiwm2i),fori=1,2,3,.....,n. $
|
Error dynamical system takes the form
$ ˙Ei= si˙ws4i+pi˙wm1i(riwm3i−qiwm2i)+piwm1i(ri˙wm3i−qi˙wm2i)= si((f4)i+Ui)+pi(f1)i(riwm3i−qiwm2i)+piwm1i(ri(f3)i−qi(f2)i)= si((f4)i+Ui)+ηi, $
|
where $ \eta_i = p_i{(f_1)}_i({r_iw_{m3i}-q_iw_{m2i}})+p_iw_{m1i} ({r_i{(f_{3})_i-q_i{(f_{2}})_i}}) $, $ i = 1, 2, 3, ...., n. $ This implies that
$ ˙Ei= si((f4)i−ηisi−(f4)i−KiEisi)+ηi= −KiEi $
|
(3.2) |
The classic Lyapunov function $ V(E(t)) $ is described by
$ V(E(t))= 12ETE= 12ΣE2i $
|
Differentiation of $ V(E(t)) $ gives
$ \dot{V}(E(t)) = \Sigma{E_i\dot{E}_i} $ |
Using Eq (3.2), one finds that
$ ˙V(E(t))=ΣEi(−KiEi)= −ΣKiE2i). $
|
(3.3) |
An appropriate selection of $ (K_1, K_1, ......., K_n) $ makes $ \dot{V}(E(t)) $ of eq (3.3), a negative definite. Consequently, by LSA [47], we obtain
$ lim_{t \to \infty} E_{i}(t) = 0, \quad \quad (i = 1, 2, 3). $ |
Hence, the master systems (2.1)–(2.3) and slave system (2.4) have attained desired CDAS strategy.
We now describe GLVBS as the scaling master system:
$ {˙wm11=wm11−wm11wm12+b3w2m11−b1w2m11wm13,˙wm12=−wm12+wm11wm12,˙wm13=b2wm13+b1w2m11wm13, $
|
(4.1) |
where $ (w_{m11}, w_{m12}, w_{m13})^T\in R^{3} $ is state vector of (4.1). Also, $ w_{m11} $ represents the prey population and $ w_{m12} $, $ w_{m13} $ denote the predator populations. For parameters $ b_1 = 2.9851 $, $ b_2 = 3 $, $ b_3 = 2 $ and initial conditions $ (27.5, 23.1, 11.4) $, scaling master GLVBS displays chaotic/disturbed behaviour as depicted in Figure 1(a).
The base master systems are the identical chaotic GLVBSs prescribed respectively as:
$ {˙wm21=wm21−wm21wm22+b3w2m21−b1w2m21wm23,˙wm22=−wm22+wm21wm22,˙wm23=b2wm23+b1w2m21wm23, $
|
(4.2) |
where $ (w_{m21}, w_{m22}, w_{m23})^T\in R^{3} $ is state vector of (4.2). For parameter values $ b_1 = 2.9851 $, $ b_2 = 3 $, $ b_3 = 2 $, this base master GLVBS shows chaotic/disturbed behaviour for initial conditions $ (1.2, 1.2, 1.2) $ as displayed in Figure 1(b).
$ {˙wm31=wm31−wm31wm32+b3w2m31−b1w2m31wm33,˙wm32=−wm32+wm31wm32,˙wm33=b2wm33+b1w2m31wm33, $
|
(4.3) |
where $ (w_{m31}, w_{m32}, w_{m33})^T\in R^{3} $ is state vector of (4.3). For parameters $ b_1 = 2.9851 $, $ b_2 = 3 $, $ b_3 = 2 $, this second base master GLVBS displays chaotic/disturbed behaviour for initial conditions $ (2.9, 12.8, 20.3) $ as shown in Figure 1(c).
The slave system, represented by similar GLVBS, is presented by
$ {˙ws41=ws41−ws41ws42+b3w2s41−b1w2s41ws43+U1,˙ws42=−ws42+ws41ws42+U2,˙ws43=b2ws43+b1w2s41ws43+U3, $
|
(4.4) |
where $ (w_{s41}, w_{s42}, w_{s43})^T\in R^{3} $ is state vector of (4.4). For parameter values, $ b_1 = 2.9851 $, $ b_2 = 3 $, $ b_3 = 2 $ and initial conditions $ (5.1, 7.4, 20.8) $, the slave GLVBS exhibits chaotic/disturbed behaviour as mentioned in Figure 1(d).
Moreover, the detailed theoretical study for (4.1)–(4.4) can be found in [4]. Further, $ U_1 $, $ U_2 $ and $ U_3 $ are controllers to be determined.
Next, the CDAS technique has been discussed for synchronizing the states of chaotic GLVBS. Also, LSA-based ACS is explored & the necessary stability criterion is established.
Here, we assume $ P = diag(p_1, p_2, p_3) $, $ Q = diag(q_1, q_2, q_3) $, $ R = diag(r_1, r_2, r_3) $, $ S = diag(s_1, s_2, s_3) $. The scaling factors $ p_i, q_i, r_i, s_i $ for $ i = 1, 2, 3 $ are selected as required and can assume the same or different values.
The error functions $ (E_1, E_2, E_3) $ are defined as:
$ {E1=s1ws41+p1wm11(r1wm31−q1wm21),E2=s2ws42+p2wm12(r2wm32−q2wm22),E3=s3ws43+p3wm13(r3wm33−q3wm23). $
|
(4.5) |
The major objective of the given work is the designing of active control functions $ U_i, (i = 1, 2, 3) $ ensuring that the error functions represented in (4.5) must satisfy
$ lim_{t \to \infty} E_i(t) = 0 \quad for \quad (i = 1, 2, 3). $ |
Therefore, subsequent error dynamics become
$ {˙E1=s1˙ws41+p1˙wm11(r1wm31−q1wm21)+p1wm11(r1˙wm31−q1˙wm21),˙E2=s2˙ws42+p2˙wm12(r2wm32−q2wm22)+p2wm12(r2˙wm32−q2˙wm22),˙E3=s3˙ws43+p3˙wm13(r3wm33−q3wm23)+p3wm13(r3˙wm33−q3˙wm23). $
|
(4.6) |
Using (4.1), (4.2), (4.3), and (4.5) in (4.6), the error dynamics simplifies to
$ {˙E1=s1(ws41−ws41ws42+b3w2s41−b1w2s41ws43+U1)+p1(wm11−wm11wm12+b3w2m11−b1w2m11wm13)(r1wm31−q1wm21)+p1wm11(r1(wm31−wm31wm32+b3w2m31−b1w2m31wm33)−q1(wm21−wm21wm22+b3w2m21−b1w2m21wm23),˙E2=s2(−ws42+ws41ws42+U2)+p2(−wm12+wm11wm12)(r2wm32−q2wm22)+p2wm12(r2(−wm32+wm31wm32)−q2(−wm22+wm21wm22)),˙E3=s3(b2ws43+b1w2s41ws43+U3)+p3(b2wm13+b1w2m11wm13)(r3wm33−q3wm23)+p3wm13(r3(b2wm33+b1w2m31wm33)−q3(b2wm23+b1w2m21wm23)). $
|
(4.7) |
Let us now choose the active controllers:
$ U1= η1s1−(f4)1−K1E1s1, $
|
(4.8) |
where $ \eta_1 = p_1{(f_1)}_1({r_1w_{m31}-q_1w_{m21}})+p_1w_{m11} ({r_1{(f_{3})_1-q_1{(f_{2}})_1}}) $, as described in (3.1).
$ U2= η2s2−(f4)2−K2E2s2, $
|
(4.9) |
where $ \eta_2 = p_2{(f_1)}_2({r_2w_{m32}-q_2w_{m22}})+p_2w_{m12} ({r_2{(f_{3})_2-q_2{(f_{2}})_2}}) $.
$ U3= η3s3−(f4)3−K3E3s3, $
|
(4.10) |
where $ \eta_3 = p_3{(f_1)}_3({r_3w_{m33}-q_3w_{m23}})+p_3w_{m13} ({r_3{(f_{3})_3-q_3{(f_{2}})_3}}) $ and $ K_1 > 0, K_2 > 0, K_3 > 0 $ are gaining constants.
By substituting the controllers (4.8), (4.9) and (4.10) in (4.7), we obtain
$ {˙E1=−K1E1,˙E2=−K2E2,˙E3=−K3E3. $
|
(4.11) |
Lyapunov function $ V(E(t)) $ is now described by
$ V(E(t))= 12[E21+E22+E23]. $
|
(4.12) |
Obviously, the Lyapunov function $ V(E(t)) $ is +ve definite in $ R^3 $. Therefore, the derivative of $ V(E(t)) $ as given in (4.12) can be formulated as:
$ ˙V(E(t))= E1˙E1+E2˙E2+E3˙E3. $
|
(4.13) |
Using (4.11) in (4.13), one finds that
$ ˙V(E(t))= −K1E21−K2E22−K3E23<0, $
|
which displays that $ \dot V(E(t)) $ is -ve definite.
In view of LSA [47], we, therefore, understand that CDAS error dynamics is globally as well as asymptotically stable, i.e., CDAS error $ E(t)\rightarrow 0 $ asymptotically for $ t\rightarrow \infty $ to each initial value $ E(0)\in R^3 $.
This section conducts a few simulation results for illustrating the efficacy of the investigated CDAS scheme in identical chaotic GLVBSs using ACS. We use $ 4^{th} $ order Runge-Kutta algorithm for solving the considered ordinary differential equations. Initial conditions for three master systems (4.1)–(4.3) and slave system (4.4) are $ (27.5, 23.1, 11.4) $, $ (1.2, 1.2, 1.2) $, $ (2.9, 12.8, 20.3) $ and $ (14.5, 3.4, 10.1) $ respectively. We attain the CDAS technique among three masters (4.1)–(4.3) and corresponding one slave system (4.4) by taking $ p_i = q_i = r_i = s_i = 1 $, which implies that the slave system would be entirely anti-synchronized with the compound of three master models for $ i = 1, 2, 3 $. In addition, the control gains $ (K_1, K_2, K_3) $ are taken as 2. Also, Figure 2(a)–(c) indicates the CDAS synchronized trajectories of three master (4.1)–(4.3) & one slave system (4.4) respectively. Moreover, synchronization error functions $ (E_1, E_2, E_3) = (51.85, 275.36, 238.54) $ approach 0 as $ t $ tends to infinity which is exhibited via Figure 2(d). Hence, the proposed CDAS strategy in three masters and one slave models/systems has been demonstrated computationally.
In this work, the investigated CDAS approach in similar four chaotic GLVBSs using ACS has been analyzed. Lyapunov's stability analysis has been used to construct proper active nonlinear controllers. The considered error system, on the evolution of time, converges to zero globally & asymptotically via our appropriately designed simple active controllers. Additionally, numerical simulations via MATLAB suggest that the newly described nonlinear control functions are immensely efficient in synchronizing the chaotic regime found in GLVBSs to fitting set points which exhibit the efficacy and supremacy of our proposed CDAS strategy. Exceptionally, both analytic theory and computational results are in complete agreement. Our proposed approach is simple yet analytically precise. The control and synchronization among the complex GLVBSs with the complex dynamical network would be an open research problem. Also, in this direction, we may extend the considered CDAS technique on chaotic systems that interfered with model uncertainties as well as external disturbances.
The authors gratefully acknowledge Qassim University, represented by the Deanship of Scientific Research, on the financial support for this research under the number 10163-qec-2020-1-3-I during the academic year 1441 AH/2020 AD.
The authors declare there is no conflict of interest.
[1] | Lee RC, Feinbaum RL, Ambros V (1993) The C. elegans heterochronic gene lin-4 encodes small RNAs with antisense complementarity to lin-14. Cell 75: 843-854. |
[2] |
Lagos-Quintana M, Rauhut R, Lendeckel W, et al. (2001) Identification of novel genes coding for small expressed RNAs. Science 294: 853-858. doi: 10.1126/science.1064921
![]() |
[3] |
Lau NC, Lim LP, Weinstein EG, et al. (2001) An abundant class of tiny RNAs with probable regulatory roles in Caenorhabditis elegans. Science 294: 858-862. doi: 10.1126/science.1065062
![]() |
[4] |
Lee RC, Ambros V (2001) An extensive class of small RNAs in Caenorhabditis elegans. Science 294: 862-864. doi: 10.1126/science.1065329
![]() |
[5] |
Pasquinelli AE, Reinhart BJ, Slack F, et al. (2000) Conservation of the sequence and temporal expression of let-7 heterochronic regulatory RNA. Nature 408: 86-89. doi: 10.1038/35040556
![]() |
[6] | Ardekani AM, Naeini MM (2010) The Role of MicroRNAs in Human Diseases. Avicenna J Med Biotechnol 2: 161-179. |
[7] |
Reuter S, Gupta SC, Chaturvedi MM, et al. (2010) Oxidative stress, inflammation, and cancer: how are they linked? Free Radic Biol Med 49: 1603-1616. doi: 10.1016/j.freeradbiomed.2010.09.006
![]() |
[8] |
Magenta A, Greco S, Gaetano C, et al. (2013) Oxidative stress and microRNAs in vascular diseases. Int J Mol Sci 14: 17319-17346. doi: 10.3390/ijms140917319
![]() |
[9] | Rahal A, Kumar A, Singh V, et al. (2014) Oxidative stress, prooxidants, and antioxidants: the interplay. Biomed Res Int 2014: 761264. |
[10] |
Dringen R (2000) Metabolism and functions of glutathione in brain. Prog Neurobiol 62: 649-671. doi: 10.1016/S0301-0082(99)00060-X
![]() |
[11] |
Jomova K, Vondrakova D, Lawson M, et al. (2010) Metals, oxidative stress and neurodegenerative disorders. Mol Cell Biochem 345: 91-104. doi: 10.1007/s11010-010-0563-x
![]() |
[12] |
Meister A (1988) On the discovery of glutathione. Trends Biochem Sci 13: 185-188. doi: 10.1016/0968-0004(88)90148-X
![]() |
[13] |
Maruyama Y, Yasuda R, Kuroda M, et al. (2012) Kokumi substances, enhancers of basic tastes, induce responses in calcium-sensing receptor expressing taste cells. PLoS One 7: e34489. doi: 10.1371/journal.pone.0034489
![]() |
[14] |
Ueda Y, Yonemitsu M, Tsubuku T, et al. (1997) Flavor characteristics of glutathione in raw and cooked foodstuffs. Biosci Biotechnol Biochem 61: 1977-1980. doi: 10.1271/bbb.61.1977
![]() |
[15] |
Aoyama K, Watabe M, Nakaki T (2012) Modulation of neuronal glutathione synthesis by EAAC1 and its interacting protein GTRAP3-18. Amino Acids 42: 163-169. doi: 10.1007/s00726-011-0861-y
![]() |
[16] | Kinoshita C, Aoyama K, Matsumura N, et al. (2014) Rhythmic oscillations of the microRNA miR-96-5p play a neuroprotective role by indirectly regulating glutathione levels. Nat Commun 5: 3823. |
[17] |
Ubhi K, Rockenstein E, Kragh C, et al. (2014) Widespread microRNA dysregulation in multiple system atrophy - disease-related alteration in miR-96. Eur J Neurosci 39: 1026-1041. doi: 10.1111/ejn.12444
![]() |
[18] |
Ha M, Kim VN (2014) Regulation of microRNA biogenesis. Nat Rev Mol Cell Biol 15: 509-524. doi: 10.1038/nrm3838
![]() |
[19] |
Sevignani C, Calin GA, Siracusa LD, et al. (2006) Mammalian microRNAs: a small world for fine-tuning gene expression. Mamm Genome 17: 189-202. doi: 10.1007/s00335-005-0066-3
![]() |
[20] | Cipolla GA (2014) A non-canonical landscape of the microRNA system. Front Genet 5: 337. |
[21] |
Lytle JR, Yario TA, Steitz JA (2007) Target mRNAs are repressed as efficiently by microRNA-binding sites in the 5' UTR as in the 3' UTR. Proc Natl Acad Sci U S A 104: 9667-9672. doi: 10.1073/pnas.0703820104
![]() |
[22] | Henchcliffe C, Beal MF (2008) Mitochondrial biology and oxidative stress in Parkinson disease pathogenesis. Nat Clin Pract Neurol 4: 600-609. |
[23] |
Finkel T, Holbrook NJ (2000) Oxidants, oxidative stress and the biology of ageing. Nature 408: 239-247. doi: 10.1038/35041687
![]() |
[24] |
Ray PD, Huang BW, Tsuji Y (2012) Reactive oxygen species (ROS) homeostasis and redox regulation in cellular signaling. Cell Signal 24: 981-990. doi: 10.1016/j.cellsig.2012.01.008
![]() |
[25] |
Lee S, Choi E, Cha MJ, et al. (2014) Looking into a Conceptual Framework of ROS-miRNA-Atrial Fibrillation. Int J Mol Sci 15: 21754-21776. doi: 10.3390/ijms151221754
![]() |
[26] |
Magenta A, Cencioni C, Fasanaro P, et al. (2011) miR-200c is upregulated by oxidative stress and induces endothelial cell apoptosis and senescence via ZEB1 inhibition. Cell Death Differ 18: 1628-1639. doi: 10.1038/cdd.2011.42
![]() |
[27] |
Brabletz S, Brabletz T (2010) The ZEB/miR-200 feedback loop--a motor of cellular plasticity in development and cancer? EMBO Rep 11: 670-677. doi: 10.1038/embor.2010.117
![]() |
[28] |
Mateescu B, Batista L, Cardon M, et al. (2011) miR-141 and miR-200a act on ovarian tumorigenesis by controlling oxidative stress response. Nat Med 17: 1627-1635. doi: 10.1038/nm.2512
![]() |
[29] |
Rosati J, Spallotta F, Nanni S, et al. (2011) Smad-interacting protein-1 and microRNA 200 family define a nitric oxide-dependent molecular circuitry involved in embryonic stem cell mesendoderm differentiation. Arterioscler Thromb Vasc Biol 31: 898-907. doi: 10.1161/ATVBAHA.110.214478
![]() |
[30] |
Lin Y, Liu X, Cheng Y, et al. (2009) Involvement of MicroRNAs in hydrogen peroxide-mediated gene regulation and cellular injury response in vascular smooth muscle cells. J Biol Chem 284: 7903-7913. doi: 10.1074/jbc.M806920200
![]() |
[31] |
Lin H, Qian J, Castillo AC, et al. (2011) Effect of miR-23 on oxidant-induced injury in human retinal pigment epithelial cells. Invest Ophthalmol Vis Sci 52: 6308-6314. doi: 10.1167/iovs.10-6632
![]() |
[32] | Papaioannou MD, Lagarrigue M, Vejnar CE, et al. (2011) Loss of Dicer in Sertoli cells has a major impact on the testicular proteome of mice. Mol Cell Proteomics 10: M900587MCP900200. |
[33] |
Wang Q, Wang Y, Minto AW, et al. (2008) MicroRNA-377 is up-regulated and can lead to increased fibronectin production in diabetic nephropathy. FASEB J 22: 4126-4135. doi: 10.1096/fj.08-112326
![]() |
[34] |
Xu Y, Fang F, Zhang J, et al. (2010) miR-17* suppresses tumorigenicity of prostate cancer by inhibiting mitochondrial antioxidant enzymes. PLoS One 5: e14356. doi: 10.1371/journal.pone.0014356
![]() |
[35] |
Zhang X, Ng WL, Wang P, et al. (2012) MicroRNA-21 modulates the levels of reactive oxygen species by targeting SOD3 and TNFalpha. Cancer Res 72: 4707-4713. doi: 10.1158/0008-5472.CAN-12-0639
![]() |
[36] |
Fleissner F, Jazbutyte V, Fiedler J, et al. (2010) Short communication: asymmetric dimethylarginine impairs angiogenic progenitor cell function in patients with coronary artery disease through a microRNA-21-dependent mechanism. Circ Res 107: 138-143. doi: 10.1161/CIRCRESAHA.110.216770
![]() |
[37] |
Zhao H, Tao Z, Wang R, et al. (2014) MicroRNA-23a-3p attenuates oxidative stress injury in a mouse model of focal cerebral ischemia-reperfusion. Brain Res 1592: 65-72. doi: 10.1016/j.brainres.2014.09.055
![]() |
[38] |
Xia XG, Zhou H, Samper E, et al. (2006) Pol II-expressed shRNA knocks down Sod2 gene expression and causes phenotypes of the gene knockout in mice. PLoS Genet 2: e10. doi: 10.1371/journal.pgen.0020010
![]() |
[39] |
Bai XY, Ma Y, Ding R, et al. (2011) miR-335 and miR-34a Promote renal senescence by suppressing mitochondrial antioxidative enzymes. J Am Soc Nephrol 22: 1252-1261. doi: 10.1681/ASN.2010040367
![]() |
[40] | Togliatto G, Trombetta A, Dentelli P, et al. (2014) Unacylated ghrelin (UnAG) induces oxidative stress resistance in a glucose intolerance mouse model and peripheral artery disease by restoring endothelial cell miR-126 expression. Diabetes 64: 1370-82. |
[41] |
Ji G, Lv K, Chen H, et al. (2013) MiR-146a regulates SOD2 expression in H2O2 stimulated PC12 cells. PLoS One 8: e69351. doi: 10.1371/journal.pone.0069351
![]() |
[42] |
Meng X, Wu J, Pan C, et al. (2013) Genetic and epigenetic down-regulation of microRNA-212 promotes colorectal tumor metastasis via dysregulation of MnSOD. Gastroenterology 145: 426-436 e421-426. doi: 10.1053/j.gastro.2013.04.004
![]() |
[43] | Liu X, Yu J, Jiang L, et al. (2009) MicroRNA-222 regulates cell invasion by targeting matrix metalloproteinase 1 (MMP1) and manganese superoxide dismutase 2 (SOD2) in tongue squamous cell carcinoma cell lines. Cancer Genom Proteom 6: 131-139. |
[44] |
Kriegel AJ, Fang Y, Liu Y, et al. (2010) MicroRNA-target pairs in human renal epithelial cells treated with transforming growth factor beta 1: a novel role of miR-382. Nucleic Acids Res 38: 8338-8347. doi: 10.1093/nar/gkq718
![]() |
[45] |
Tu H, Sun H, Lin Y, et al. (2014) Oxidative stress upregulates PDCD4 expression in patients with gastric cancer via miR-21. Curr Pharm Des 20: 1917-1923. doi: 10.2174/13816128113199990547
![]() |
[46] |
Haque R, Chun E, Howell JC, et al. (2012) MicroRNA-30b-mediated regulation of catalase expression in human ARPE-19 cells. PLoS One 7: e42542. doi: 10.1371/journal.pone.0042542
![]() |
[47] |
Wang Q, Chen W, Bai L, et al. (2014) Receptor-interacting protein 1 increases chemoresistance by maintaining inhibitor of apoptosis protein levels and reducing reactive oxygen species through a microRNA-146a-mediated catalase pathway. J Biol Chem 289: 5654-5663. doi: 10.1074/jbc.M113.526152
![]() |
[48] |
Xu X, Wells A, Padilla MT, et al. (2014) A signaling pathway consisting of miR-551b, catalase and MUC1 contributes to acquired apoptosis resistance and chemoresistance. Carcinogenesis 35: 2457-2466. doi: 10.1093/carcin/bgu159
![]() |
[49] | Espinosa-Diez C, Fierro-Fernandez M, Sanchez-Gomez FJ, et al. (2014) Targeting of gamma-glutamyl-cysteine ligase by miR-433 reduces glutathione biosynthesis and promotes TGF-beta-dependent fibrogenesis. Antioxid Redox Signal. |
[50] |
Dong X, Liu H, Chen F, et al. (2014) MiR-214 promotes the alcohol-induced oxidative stress via down-regulation of glutathione reductase and cytochrome P450 oxidoreductase in liver cells. Alcohol Clin Exp Res 38: 68-77. doi: 10.1111/acer.12209
![]() |
[51] | Wang L, Huang H, Fan Y, et al. (2014) Effects of downregulation of microRNA-181a on H2O2-induced H9c2 cell apoptosis via the mitochondrial apoptotic pathway. Oxid Med Cell Longev 2014: 960362. |
[52] |
Maciel-Dominguez A, Swan D, Ford D, et al. (2013) Selenium alters miRNA profile in an intestinal cell line: evidence that miR-185 regulates expression of GPX2 and SEPSH2. Mol Nutr Food Res 57: 2195-2205. doi: 10.1002/mnfr.201300168
![]() |
[53] |
Jee MK, Jung JS, Choi JI, et al. (2012) MicroRNA 486 is a potentially novel target for the treatment of spinal cord injury. Brain 135: 1237-1252. doi: 10.1093/brain/aws047
![]() |
[54] | Mutallip M, Nohata N, Hanazawa T, et al. (2011) Glutathione S-transferase P1 (GSTP1) suppresses cell apoptosis and its regulation by miR-133alpha in head and neck squamous cell carcinoma (HNSCC). Int J Mol Med 27: 345-352. |
[55] |
Moriya Y, Nohata N, Kinoshita T, et al. (2012) Tumor suppressive microRNA-133a regulates novel molecular networks in lung squamous cell carcinoma. J Hum Genet 57: 38-45. doi: 10.1038/jhg.2011.126
![]() |
[56] |
Uchida Y, Chiyomaru T, Enokida H, et al. (2013) MiR-133a induces apoptosis through direct regulation of GSTP1 in bladder cancer cell lines. Urol Oncol 31: 115-123. doi: 10.1016/j.urolonc.2010.09.017
![]() |
[57] |
Patron JP, Fendler A, Bild M, et al. (2012) MiR-133b targets antiapoptotic genes and enhances death receptor-induced apoptosis. PLoS One 7: e35345. doi: 10.1371/journal.pone.0035345
![]() |
[58] |
Zhang X, Zhu J, Xing R, et al. (2012) miR-513a-3p sensitizes human lung adenocarcinoma cells to chemotherapy by targeting GSTP1. Lung Cancer 77: 488-494. doi: 10.1016/j.lungcan.2012.05.107
![]() |
[59] |
Kraemer A, Barjaktarovic Z, Sarioglu H, et al. (2013) Cell survival following radiation exposure requires miR-525-3p mediated suppression of ARRB1 and TXN1. PLoS One 8: e77484. doi: 10.1371/journal.pone.0077484
![]() |
[60] |
Lerner AG, Upton JP, Praveen PV, et al. (2012) IRE1alpha induces thioredoxin-interacting protein to activate the NLRP3 inflammasome and promote programmed cell death under irremediable ER stress. Cell Metab 16: 250-264. doi: 10.1016/j.cmet.2012.07.007
![]() |
[61] |
Knoll S, Furst K, Kowtharapu B, et al. (2014) E2F1 induces miR-224/452 expression to drive EMT through TXNIP downregulation. EMBO Rep 15: 1315-1329. doi: 10.15252/embr.201439392
![]() |
[62] |
Ragusa M, Statello L, Maugeri M, et al. (2012) Specific alterations of the microRNA transcriptome and global network structure in colorectal cancer after treatment with MAPK/ERK inhibitors. J Mol Med (Berl) 90: 1421-1438. doi: 10.1007/s00109-012-0918-8
![]() |
[63] |
Yan GR, Xu SH, Tan ZL, et al. (2011) Global identification of miR-373-regulated genes in breast cancer by quantitative proteomics. Proteomics 11: 912-920. doi: 10.1002/pmic.201000539
![]() |
[64] |
He HC, Zhu JG, Chen XB, et al. (2012) MicroRNA-23b downregulates peroxiredoxin III in human prostate cancer. FEBS Lett 586: 2451-2458. doi: 10.1016/j.febslet.2012.06.003
![]() |
[65] | Jiang W, Min J, Sui X, et al. (2014) MicroRNA-26a-5p and microRNA-23b-3p up-regulate peroxiredoxin III in acute myeloid leukemia. Leuk Lymphoma: 1-12. |
[66] |
Lee HK, Finniss S, Cazacu S, et al. (2014) Mesenchymal Stem Cells Deliver Exogenous miRNAs to Neural Cells and Induce Their Differentiation and Glutamate Transporter Expression. Stem Cells Dev 23: 2851-2861. doi: 10.1089/scd.2014.0146
![]() |
[67] |
Yang ZB, Zhang Z, Li TB, et al. (2014) Up-regulation of brain-enriched miR-107 promotes excitatory neurotoxicity through down-regulation of glutamate transporter-1 expression following ischaemic stroke. Clin Sci (Lond) 127: 679-689. doi: 10.1042/CS20140084
![]() |
[68] |
Morel L, Regan M, Higashimori H, et al. (2013) Neuronal exosomal miRNA-dependent translational regulation of astroglial glutamate transporter GLT1. J Biol Chem 288: 7105-7116. doi: 10.1074/jbc.M112.410944
![]() |
[69] |
Moon JM, Xu L, Giffard RG (2013) Inhibition of microRNA-181 reduces forebrain ischemia-induced neuronal loss. J Cereb Blood Flow Metab 33: 1976-1982. doi: 10.1038/jcbfm.2013.157
![]() |
[70] |
Kaalund SS, Veno MT, Bak M, et al. (2014) Aberrant expression of miR-218 and miR-204 in human mesial temporal lobe epilepsy and hippocampal sclerosis-Convergence on axonal guidance. Epilepsia 55: 2017-2027. doi: 10.1111/epi.12839
![]() |
[71] |
Fridovich I (1978) The biology of oxygen radicals. Science 201: 875-880. doi: 10.1126/science.210504
![]() |
[72] |
Lu M, Zhang Q, Deng M, et al. (2008) An analysis of human microRNA and disease associations. PLoS One 3: e3420. doi: 10.1371/journal.pone.0003420
![]() |
[73] |
Aoyama K, Watabe M, Nakaki T (2008) Regulation of neuronal glutathione synthesis. J Pharmacol Sci 108: 227-238. doi: 10.1254/jphs.08R01CR
![]() |
[74] | Cooper AJ, Kristal BS (1997) Multiple roles of glutathione in the central nervous system. Biol Chem 378: 793-802. |
[75] |
Janaky R, Varga V, Hermann A, et al. (2000) Mechanisms of L-cysteine neurotoxicity. Neurochem Res 25: 1397-1405. doi: 10.1023/A:1007616817499
![]() |
[76] |
Quintana-Cabrera R, Fernandez-Fernandez S, Bobo-Jimenez V, et al. (2012) gamma-Glutamylcysteine detoxifies reactive oxygen species by acting as glutathione peroxidase-1 cofactor. Nat Commun 3: 718. doi: 10.1038/ncomms1722
![]() |
[77] | Seelig GF, Simondsen RP, Meister A (1984) Reversible dissociation of gamma-glutamylcysteine synthetase into two subunits. J Biol Chem 259: 9345-9347. |
[78] |
Dalton TP, Chen Y, Schneider SN, et al. (2004) Genetically altered mice to evaluate glutathione homeostasis in health and disease. Free Radic Biol Med 37: 1511-1526. doi: 10.1016/j.freeradbiomed.2004.06.040
![]() |
[79] | Yang Y, Dieter MZ, Chen Y, et al. (2002) Initial characterization of the glutamate-cysteine ligase modifier subunit Gclm(-/-) knockout mouse. Novel model system for a severely compromised oxidative stress response. J Biol Chem 277: 49446-49452. |
[80] |
Ristoff E, Larsson A (2007) Inborn errors in the metabolism of glutathione. Orphanet J Rare Dis 2: 1-9. doi: 10.1186/1750-1172-2-1
![]() |
[81] |
Brigelius-Flohe R, Maiorino M (2013) Glutathione peroxidases. Biochim Biophys Acta 1830: 3289-3303. doi: 10.1016/j.bbagen.2012.11.020
![]() |
[82] |
Kryukov GV, Castellano S, Novoselov SV, et al. (2003) Characterization of mammalian selenoproteomes. Science 300: 1439-1443. doi: 10.1126/science.1083516
![]() |
[83] | Arthur JR (2000) The glutathione peroxidases. Cell Mol Life Sci 57: 1825-1835. |
[84] |
Power JH, Blumbergs PC (2009) Cellular glutathione peroxidase in human brain: cellular distribution, and its potential role in the degradation of Lewy bodies in Parkinson's disease and dementia with Lewy bodies. Acta Neuropathol 117: 63-73. doi: 10.1007/s00401-008-0438-3
![]() |
[85] |
Muse KE, Oberley TD, Sempf JM, et al. (1994) Immunolocalization of antioxidant enzymes in adult hamster kidney. Histochem J 26: 734-753. doi: 10.1007/BF00158205
![]() |
[86] | Li S, Yan T, Yang JQ, et al. (2000) The role of cellular glutathione peroxidase redox regulation in the suppression of tumor cell growth by manganese superoxide dismutase. Cancer Res 60: 3927-3939. |
[87] |
Knopp EA, Arndt TL, Eng KL, et al. (1999) Murine phospholipid hydroperoxide glutathione peroxidase: cDNA sequence, tissue expression, and mapping. Mamm Genome 10: 601-605. doi: 10.1007/s003359901053
![]() |
[88] | Hall L, Williams K, Perry AC, et al. (1998) The majority of human glutathione peroxidase type 5 (GPX5) transcripts are incorrectly spliced: implications for the role of GPX5 in the male reproductive tract. Biochem J 333: 5-9. |
[89] |
Nguyen VD, Saaranen MJ, Karala AR, et al. (2011) Two endoplasmic reticulum PDI peroxidases increase the efficiency of the use of peroxide during disulfide bond formation. J Mol Biol 406: 503-515. doi: 10.1016/j.jmb.2010.12.039
![]() |
[90] |
Lindenau J, Noack H, Asayama K, et al. (1998) Enhanced cellular glutathione peroxidase immunoreactivity in activated astrocytes and in microglia during excitotoxin induced neurodegeneration. Glia 24: 252-256. doi: 10.1002/(SICI)1098-1136(199810)24:2<252::AID-GLIA10>3.0.CO;2-Z
![]() |
[91] | Satoh J, Yamamoto Y, Asahina N, et al. (2014) RNA-Seq Data Mining: Downregulation of NeuroD6 Serves as a Possible Biomarker for Alzheimer's Disease Brains. Dis Markers 2014: 123165. |
[92] |
Deponte M (2013) Glutathione catalysis and the reaction mechanisms of glutathione-dependent enzymes. Biochim Biophys Acta 1830: 3217-3266. doi: 10.1016/j.bbagen.2012.09.018
![]() |
[93] | Johnson JA, el Barbary A, Kornguth SE, et al. (1993) Glutathione S-transferase isoenzymes in ratbrain neurons and glia. J Neurosci 13: 2013-2023. |
[94] |
Martin HL, Teismann P (2009) Glutathione--a review on its role and significance in Parkinson's disease. FASEB J 23: 3263-3272. doi: 10.1096/fj.08-125443
![]() |
[95] |
Zeevalk GD, Razmpour R, Bernard LP (2008) Glutathione and Parkinson's disease: is this the elephant in the room? Biomed Pharmacother 62: 236-249. doi: 10.1016/j.biopha.2008.01.017
![]() |
[96] | Holmgren A (1989) Thioredoxin and glutaredoxin systems. J Biol Chem 264: 13963-13966. |
[97] | Kenchappa RS, Ravindranath V (2003) Glutaredoxin is essential for maintenance of brain mitochondrial complex I: studies with MPTP. FASEB J 17: 717-719. |
[98] |
Lovell MA, Xie C, Gabbita SP, et al. (2000) Decreased thioredoxin and increased thioredoxin reductase levels in Alzheimer's disease brain. Free Radic Biol Med 28: 418-427. doi: 10.1016/S0891-5849(99)00258-0
![]() |
[99] | Yoshihara E, Masaki S, Matsuo Y, et al. (2014) Thioredoxin/Txnip: redoxisome, as a redox switch for the pathogenesis of diseases. Front Immunol 4: 514. |
[100] | Dringen R, Pfeiffer B, Hamprecht B (1999) Synthesis of the antioxidant glutathione in neurons: supply by astrocytes of CysGly as precursor for neuronal glutathione. J Neurosci 19: 562-569. |
[101] |
Shanker G, Allen JW, Mutkus LA, et al. (2001) The uptake of cysteine in cultured primary astrocytes and neurons. Brain Res 902: 156-163. doi: 10.1016/S0006-8993(01)02342-3
![]() |
[102] |
Chen Y, Swanson RA (2003) The glutamate transporters EAAT2 and EAAT3 mediate cysteine uptake in cortical neuron cultures. J Neurochem 84: 1332-1339. doi: 10.1046/j.1471-4159.2003.01630.x
![]() |
[103] |
Himi T, Ikeda M, Yasuhara T, et al. (2003) Role of neuronal glutamate transporter in the cysteine uptake and intracellular glutathione levels in cultured cortical neurons. J Neural Transm 110: 1337-1348. doi: 10.1007/s00702-003-0049-z
![]() |
[104] |
Kanai Y, Hediger MA (2003) The glutamate and neutral amino acid transporter family: physiological and pharmacological implications. Eur J Pharmacol 479: 237-247. doi: 10.1016/j.ejphar.2003.08.073
![]() |
[105] |
Maragakis NJ, Rothstein JD (2004) Glutamate transporters: animal models to neurologic disease. Neurobiol Dis 15: 461-473. doi: 10.1016/j.nbd.2003.12.007
![]() |
[106] |
Zerangue N, Kavanaugh MP (1996) Interaction of L-cysteine with a human excitatory amino acid transporter. J Physiol 493: 419-423. doi: 10.1113/jphysiol.1996.sp021393
![]() |
[107] |
Aoyama K, Suh SW, Hamby AM, et al. (2006) Neuronal glutathione deficiency and age-dependent neurodegeneration in the EAAC1 deficient mouse. Nat Neurosci 9: 119-126. doi: 10.1038/nn1609
![]() |
[108] |
Lin CI, Orlov I, Ruggiero AM, et al. (2001) Modulation of the neuronal glutamate transporter EAAC1 by the interacting protein GTRAP3-18. Nature 410: 84-88. doi: 10.1038/35065084
![]() |
[109] |
Abdul-Ghani M, Gougeon PY, Prosser DC, et al. (2001) PRA isoforms are targeted to distinct membrane compartments. J Biol Chem 276: 6225-6233. doi: 10.1074/jbc.M009073200
![]() |
[110] |
Ruggiero AM, Liu Y, Vidensky S, et al. (2008) The endoplasmic reticulum exit of glutamate transporter is regulated by the inducible mammalian Yip6b/GTRAP3-18 protein. J Biol Chem 283: 6175-6183. doi: 10.1074/jbc.M701008200
![]() |
[111] |
Aoyama K, Wang F, Matsumura N, et al. (2012) Increased neuronal glutathione and neuroprotection in GTRAP3-18-deficient mice. Neurobiol Dis 45: 973-982. doi: 10.1016/j.nbd.2011.12.016
![]() |
[112] |
Andersen JK (2004) Oxidative stress in neurodegeneration: cause or consequence? Nat Med 10: S18-25. doi: 10.1038/nrn1434
![]() |
[113] | Selkoe DJ (2001) Alzheimer's disease: genes, proteins, and therapy. Physiol Rev 81: 741-766. |
[114] | Maciotta S, Meregalli M, Torrente Y (2013) The involvement of microRNAs in neurodegenerative diseases. Front Cell Neurosci 7: 265. |
[115] | Schipper HM, Maes OC, Chertkow HM, et al. (2007) MicroRNA expression in Alzheimer blood mononuclear cells. Gene Regul Syst Bio 1: 263-274. |
[116] | Cogswell JP, Ward J, Taylor IA, et al. (2008) Identification of miRNA changes in Alzheimer's disease brain and CSF yields putative biomarkers and insights into disease pathways. J Alzheimers Dis 14: 27-41. |
[117] |
Tanner CM, Goldman SM (1996) Epidemiology of Parkinson's disease. Neurol Clin 14: 317-335. doi: 10.1016/S0733-8619(05)70259-0
![]() |
[118] | Fearnley JM, Lees AJ (1991) Ageing and Parkinson's disease: substantia nigra regional selectivity. Brain 114 : 2283-2301. |
[119] | Baba M, Nakajo S, Tu PH, et al. (1998) Aggregation of alpha-synuclein in Lewy bodies of sporadic Parkinson's disease and dementia with Lewy bodies. Am J Pathol 152: 879-884. |
[120] |
Kim J, Inoue K, Ishii J, et al. (2007) A MicroRNA feedback circuit in midbrain dopamine neurons. Science 317: 1220-1224. doi: 10.1126/science.1140481
![]() |
[121] |
Gusella JF, MacDonald ME, Ambrose CM, et al. (1993) Molecular genetics of Huntington's disease. Arch Neurol 50: 1157-1163. doi: 10.1001/archneur.1993.00540110037003
![]() |
[122] |
Vonsattel JP, DiFiglia M (1998) Huntington disease. J Neuropathol Exp Neurol 57: 369-384. doi: 10.1097/00005072-199805000-00001
![]() |
[123] |
Novak MJ, Tabrizi SJ (2010) Huntington's disease. BMJ 340: c3109. doi: 10.1136/bmj.c3109
![]() |
[124] | Browne SE, Ferrante RJ, Beal MF (1999) Oxidative stress in Huntington's disease. Brain Pathol 9: 147-163. |
[125] |
Petr GT, Bakradze E, Frederick NM, et al. (2013) Glutamate transporter expression and function in a striatal neuronal model of Huntington's disease. Neurochem Int 62: 973-981. doi: 10.1016/j.neuint.2013.02.026
![]() |
[126] |
Packer AN, Xing Y, Harper SQ, et al. (2008) The bifunctional microRNA miR-9/miR-9* regulates REST and CoREST and is downregulated in Huntington's disease. J Neurosci 28: 14341-14346. doi: 10.1523/JNEUROSCI.2390-08.2008
![]() |
[127] |
Marti E, Pantano L, Banez-Coronel M, et al. (2010) A myriad of miRNA variants in control and Huntington's disease brain regions detected by massively parallel sequencing. Nucleic Acids Res 38: 7219-7235. doi: 10.1093/nar/gkq575
![]() |
[128] |
Mason RP, Casu M, Butler N, et al. (2013) Glutathione peroxidase activity is neuroprotective in models of Huntington's disease. Nat Genet 45: 1249-1254. doi: 10.1038/ng.2732
![]() |
[129] |
Kim C, Lee HC, Sung JJ (2014) Amyotrophic lateral sclerosis - cell based therapy and novel therapeutic development. Exp Neurobiol 23: 207-214. doi: 10.5607/en.2014.23.3.207
![]() |
[130] |
Sreedharan J, Brown RH, Jr. (2013) Amyotrophic lateral sclerosis: Problems and prospects. Ann Neurol 74: 309-316. doi: 10.1002/ana.24012
![]() |
[131] |
Synofzik M, Ronchi D, Keskin I, et al. (2012) Mutant superoxide dismutase-1 indistinguishable from wild-type causes ALS. Hum Mol Genet 21: 3568-3574. doi: 10.1093/hmg/dds188
![]() |
[132] |
Butovsky O, Siddiqui S, Gabriely G, et al. (2012) Modulating inflammatory monocytes with a unique microRNA gene signature ameliorates murine ALS. J Clin Invest 122: 3063-3087. doi: 10.1172/JCI62636
![]() |
[133] |
Koval ED, Shaner C, Zhang P, et al. (2013) Method for widespread microRNA-155 inhibition prolongs survival in ALS-model mice. Hum Mol Genet 22: 4127-4135. doi: 10.1093/hmg/ddt261
![]() |
[134] |
Baillet A, Chanteperdrix V, Trocme C, et al. (2010) The role of oxidative stress in amyotrophic lateral sclerosis and Parkinson's disease. Neurochem Res 35: 1530-1537. doi: 10.1007/s11064-010-0212-5
![]() |
[135] |
Golenia A, Leskiewicz M, Regulska M, et al. (2014) Catalase activity in blood fractions of patients with sporadic ALS. Pharmacol Rep 66: 704-707. doi: 10.1016/j.pharep.2014.02.021
![]() |
[136] |
Sturm E, Stefanova N (2014) Multiple system atrophy: genetic or epigenetic? Exp Neurobiol 23: 277-291. doi: 10.5607/en.2014.23.4.277
![]() |
[137] |
Wenning GK, Tison F, Ben Shlomo Y, et al. (1997) Multiple system atrophy: a review of 203 pathologically proven cases. Mov Disord 12: 133-147. doi: 10.1002/mds.870120203
![]() |
[138] |
Dallmann R, Brown SA, Gachon F (2014) Chronopharmacology: new insights and therapeutic implications. Annu Rev Pharmacol Toxicol 54: 339-361. doi: 10.1146/annurev-pharmtox-011613-135923
![]() |
[139] |
Bass J (2012) Circadian topology of metabolism. Nature 491: 348-356. doi: 10.1038/nature11704
![]() |
[140] |
Kondratov RV (2007) A role of the circadian system and circadian proteins in aging. Ageing Res Rev 6: 12-27. doi: 10.1016/j.arr.2007.02.003
![]() |
[141] |
Kondratov RV, Kondratova AA, Gorbacheva VY, et al. (2006) Early aging and age-related pathologies in mice deficient in BMAL1, the core componentof the circadian clock. Genes Dev 20: 1868-1873. doi: 10.1101/gad.1432206
![]() |
[142] |
Willison LD, Kudo T, Loh DH, et al. (2013) Circadian dysfunction may be a key component of the non-motor symptoms of Parkinson's disease: insights from a transgenic mouse model. Exp Neurol 243: 57-66. doi: 10.1016/j.expneurol.2013.01.014
![]() |
[143] |
Beaver LM, Klichko VI, Chow ES, et al. (2012) Circadian regulation of glutathione levels and biosynthesis in Drosophila melanogaster. PLoS One 7: e50454. doi: 10.1371/journal.pone.0050454
![]() |
[144] |
Xu YQ, Zhang D, Jin T, et al. (2012) Diurnal variation of hepatic antioxidant gene expression in mice. PLoS One 7: e44237. doi: 10.1371/journal.pone.0044237
![]() |
[145] |
Krishnan N, Davis AJ, Giebultowicz JM (2008) Circadian regulation of response to oxidative stress in Drosophila melanogaster. Biochem Biophys Res Commun 374: 299-303. doi: 10.1016/j.bbrc.2008.07.011
![]() |
[146] |
Pablos MI, Reiter RJ, Ortiz GG, et al. (1998) Rhythms of glutathione peroxidase and glutathione reductase in brain of chick and their inhibition by light. Neurochem Int 32: 69-75. doi: 10.1016/S0197-0186(97)00043-0
![]() |
[147] |
Filipski E, King VM, Etienne MC, et al. (2004) Persistent twenty-four hour changes in liver and bone marrow despite suprachiasmatic nuclei ablation in mice. Am J Physiol Regul Integr Comp Physiol 287: R844-851. doi: 10.1152/ajpregu.00085.2004
![]() |
[148] |
Arjona A, Sarkar DK (2005) Circadian oscillations of clock genes, cytolytic factors, and cytokines in rat NK cells. J Immunol 174: 7618-7624. doi: 10.4049/jimmunol.174.12.7618
![]() |
[149] |
Kochman LJ, Weber ET, Fornal CA, et al. (2006) Circadian variation in mouse hippocampal cell proliferation. Neurosci Lett 406: 256-259. doi: 10.1016/j.neulet.2006.07.058
![]() |
[150] |
Ma D, Panda S, Lin JD (2011) Temporal orchestration of circadian autophagy rhythm by C/EBPbeta. EMBO J 30: 4642-4651. doi: 10.1038/emboj.2011.322
![]() |
[151] | Kondratova AA, Dubrovsky YV, Antoch MP, et al. (2010) Circadian clock proteins control adaptation to novel environment and memory formation. Aging (Albany NY) 2: 285-297. |
1. | Muhammad Zubair Mehboob, Arslan Hamid, Jeevotham Senthil Kumar, Xia Lei, Comprehensive characterization of pathogenic missense CTRP6 variants and their association with cancer, 2025, 25, 1471-2407, 10.1186/s12885-025-13685-0 |