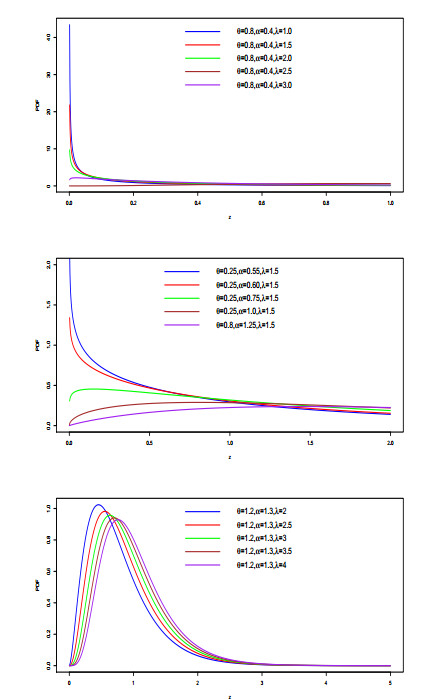
Goal of this paper is to study the following doubly nonlocal equation
(−Δ)su+μu=(Iα∗F(u))F′(u)inRN(P)
in the case of general nonlinearities F∈C1(R) of Berestycki-Lions type, when N≥2 and μ>0 is fixed. Here (−Δ)s, s∈(0,1), denotes the fractional Laplacian, while the Hartree-type term is given by convolution with the Riesz potential Iα, α∈(0,N). We prove existence of ground states of (P). Furthermore we obtain regularity and asymptotic decay of general solutions, extending some results contained in [
Citation: Silvia Cingolani, Marco Gallo, Kazunaga Tanaka. On fractional Schrödinger equations with Hartree type nonlinearities[J]. Mathematics in Engineering, 2022, 4(6): 1-33. doi: 10.3934/mine.2022056
[1] | Qian Lin, Yan Zhu . Unicyclic graphs with extremal exponential Randić index. Mathematical Modelling and Control, 2021, 1(3): 164-171. doi: 10.3934/mmc.2021015 |
[2] | Ihtisham Ul Haq, Nigar Ali, Hijaz Ahmad . Analysis of a chaotic system using fractal-fractional derivatives with exponential decay type kernels. Mathematical Modelling and Control, 2022, 2(4): 185-199. doi: 10.3934/mmc.2022019 |
[3] | Shipeng Li . Impulsive control for stationary oscillation of nonlinear delay systems and applications. Mathematical Modelling and Control, 2023, 3(4): 267-277. doi: 10.3934/mmc.2023023 |
[4] | Xingwen Liu, Shouming Zhong . Stability analysis of delayed switched cascade nonlinear systems with uniform switching signals. Mathematical Modelling and Control, 2021, 1(2): 90-101. doi: 10.3934/mmc.2021007 |
[5] | Pshtiwan Othman Mohammed . Some positive results for exponential-kernel difference operators of Riemann-Liouville type. Mathematical Modelling and Control, 2024, 4(1): 133-140. doi: 10.3934/mmc.2024012 |
[6] | Hongwei Zheng, Yujuan Tian . Exponential stability of time-delay systems with highly nonlinear impulses involving delays. Mathematical Modelling and Control, 2025, 5(1): 103-120. doi: 10.3934/mmc.2025008 |
[7] | Saravanan Shanmugam, R. Vadivel, S. Sabarathinam, P. Hammachukiattikul, Nallappan Gunasekaran . Enhancing synchronization criteria for fractional-order chaotic neural networks via intermittent control: an extended dissipativity approach. Mathematical Modelling and Control, 2025, 5(1): 31-47. doi: 10.3934/mmc.2025003 |
[8] | Hongyu Ma, Dadong Tian, Mei Li, Chao Zhang . Reachable set estimation for 2-D switched nonlinear positive systems with impulsive effects and bounded disturbances described by the Roesser model. Mathematical Modelling and Control, 2024, 4(2): 152-162. doi: 10.3934/mmc.2024014 |
[9] | Gani Stamov, Ekaterina Gospodinova, Ivanka Stamova . Practical exponential stability with respect to h−manifolds of discontinuous delayed Cohen–Grossberg neural networks with variable impulsive perturbations. Mathematical Modelling and Control, 2021, 1(1): 26-34. doi: 10.3934/mmc.2021003 |
[10] | Yuchen Yuan, Ying Fang . Optimal reinsurance design under the VaR risk measure and asymmetric information. Mathematical Modelling and Control, 2022, 2(4): 165-175. doi: 10.3934/mmc.2022017 |
Goal of this paper is to study the following doubly nonlocal equation
(−Δ)su+μu=(Iα∗F(u))F′(u)inRN(P)
in the case of general nonlinearities F∈C1(R) of Berestycki-Lions type, when N≥2 and μ>0 is fixed. Here (−Δ)s, s∈(0,1), denotes the fractional Laplacian, while the Hartree-type term is given by convolution with the Riesz potential Iα, α∈(0,N). We prove existence of ground states of (P). Furthermore we obtain regularity and asymptotic decay of general solutions, extending some results contained in [
Statistical distributions are a fundamental tool for understanding and predicting phenomena in the real world. Many researchers have been interested in developing a novel family of, which have more efficient in modeling numerous kinds of skewed data in different fields, such as environmental, biomedical, finance, and insurance (for example, see Meraou and raqab [1], Hashemi et al. [2], and Abdelghani et al. [3]). For further information on analyzing experimental data, one may refer to Naderi et al. [4], Nadarajah [5], Chakraborty et al. [6], and the references cited therein.
Various statistical distributions have been considered in this context and in the last decades. These new models are generated using various methods, like adding one or more parameters to the already existing model, and it provides great pliancy in fitting asymmetric data in practice. For more information, on may refer to some recently generated distributions, namely, the new version of the beta power transformed family for [7], Marshal-Olkin generated family for [8], alpha power transformed for [9], extended alpha power transformed family for [10], Kumaraswamy Marshal-Olkin family for [11], bivariate Kavya-Manoharan transformation family for [12], new hyperbolic sine-generator for [13], and Kavya-Manoharan Weibull-G family for[14].
Choosing the best statistical distribution for modeling data is a critical and challenging task. There are situations where the modeled probability distributions fail to choose the best fit to the different types of datasets, precisely in risk measurement, economic, financial, actuarial sciences, and insurance losses, there is no claim since of the heavier tails. Indeed, this is a desirable case for the benefit of insurance companies. Some record values from numerous areas such as insurance, actuarial sciences, and economics, exist with observations far from the data's mean. Outliers or heavier tails can cause this. Classical distributions fail to model this type of dataset. Thus, heavy-tailed distributions are needed to describe the datasets appropriately. Heavy-tailed distributions available in the literature are: heavy-tailed log-logistic distribution by [15], heavy-tailed beta-power transformed Weibull distribution by [16], alpha power inverse Weibull distribution by [17], and a heavy-tailed and over dispersed collective risk model by [18]. For more details, see [19,20,21,22,23,24,25,26]. An approach to modeling the number of claims, in this case, is to use the Topp-Leone generated (TL-G) family of distributions. Recently, [27] derived this new version of the generation family. Its cumulative distribution function (CDF) can be expressed as
G(y;η,λ)=(1−(1−H(y;η))2)λ,y∈R,λ>0. | (1.1) |
The associated probability density function (PDF) of TL-G is given by
g(y;η,λ)=2λh(y;η)(1−H(y;η))(1−(1−H(y;η))2)λ−1, | (1.2) |
where h(y;η) and H(y;η) represent PDF and CDF of the parent model. In the literature, The TL-G family has been studied by different authors, for example the TL Weibull distribution has been considered by [28], which he takes the Weibull distribution baseline distribution and studying different statistical properties. [29] has proposed alpha power inverted TL distribution and studied different distributional properties. TL Frèchet distribution is studied by [30]. In the same way, TL modified Weibull distribution is derived by [31], and presents the type Ⅱ TL Bur Ⅻ distribution [32]. They showed that the new distribution is more flexible than other generalizations of the Bur distribution.
It's worth noting that the extended exponential (EE) distribution is the commonly used distribution for analyzing skewed data and complementary risk scenarios. It is as a new generation of an exponential (Exp) distribution, which can be used in various practical cases, including fitting the claim severity in actuarial science, following the work of [33,34,35,36,37,38,39,40,41,42,43,44,45].
The research [46] introduced the EE model. The corresponding PDF and CDF of EE distribution are
ψ(t;α,θ)=αθe−θt(1−e−θt)α−1, t>0 | (1.3) |
and
Ψ(t;α,θ)=(1−e−θt)α, | (1.4) |
where, α>0 is the shape parameter and θ>0 is the scale parameter.
The objective of this work is: Firstly, we define a novel version of the EE distribution which is more efficient to modeling the complex, skewed and asymmetric datasets. The new extension model is the TL extended exponential (TL-EE) model and it has three parameters. It can be positively skewed and unimodal. The unknown parameters of the TL-EE model have been estimated using the maximum likelihood estimation (MLE) technique. Second main objective is devoted to exploring three well-known actuarial measures for the TL-EE model including value at risk (VaR), tail value at risk (TVaR), and tail variance premium (TVP). These indicator risks have been a great potential in portfolio optimization under uncertainty.
The rest of this article is structured as follows. Section 2 introduces the new TL-EE model and derives various distributional properties. Also, different basic statistical properties for the suggested model were presented in Section 3. We discuss the model's parameters for TL-EE distribution in Section 4. Brief simulation experiments have been conducted in Section 5. Further, various actuarial measures from the TL-EE model have been derived in Section 6. Finally, two real datasets representing the insurance losses is performed in Section 7 for examine the potential of the proposed TL-EE model. In the last section, closing remarks are devoted.
Here, the proposed TL-EE model with some distributional properties are derived. Let Z be a random variable that follows a TL-EE with parameters θ, α and λ (Z∼TL-EE(θ,α,λ)). According to Eqs (1.1)–(1.4), the CDF and PDF of the TL-EE model are obtained, respectively, to be
FTL-EE(z;θ,α,λ)=(1−(1−(1−e−θz)α)2)λ, | (2.1) |
whether z>0,θ,α,λ>0, and
fTL-EE(z;θ,α,λ)=2λαθe−θz(1−e−θz)α−1(1−(1−e−θz)α)(1−(1−(1−e−θz)α)2)λ−1. | (2.2) |
It is clear that from Eq (2.1), if α=1, the TL-EE model becomes the TL-Exponential distribution. For various values of the model parameters, Figures 1 and 2 sketched graphs of the PDF and CDF of the TL-EE model, respectively. The PDF of the TL-EE model is decreasing if α<1 and is unimodal if α>1.
Now, the survival with hazard rate functions (SF, HR) of the TL-EE distribution is expressed by
STL-EE(z;θ,α,λ)=1−(1−(1−(1−e−θz)α)2)λ |
and
hTL-EE(z;θ,α,λ)=2λαθe−θz(1−e−θz)α−1(1−(1−e−θz)α)(1−(1−(1−e−θz)α)2). |
Figures 3 depicts the graphs of HR of the TL-EE model using numerous selected records of parameters. From Figures 3, it can be seen that the HR of the TL-EE model is increasing with shape parameter α>1 and decreasing with shape parameter α<1.
In this section, the quantile function, skewness, kurtosis and moment-generating function of the proposed model are established.
Let Z∼TL-EE(θ,α,λ). The quantile function of Z
zu=Q(u)=F−1TL-EE(u), 0<u<1 |
is obtained as follows:
zu=−1θlog{1−[1−(1−u1/λ)1/2]1/α}. | (3.1) |
By using (3.1), a random sample from the TL-EE model is obtained with u follows a uniform random number (0, 1).
By taking
u=14,12,and34 |
in (3.1), we can be illustrated the 1st quantile, median, and 3rd quantile, respectively.
The skewness (Skew) and the kurtosis (Kurt) measures of Z are obtained to be
S=z1/4+z3/4−2z1/2z3/4−z1/4 |
and
K=z7/8−z5/8+z3/8−z1/8z6/8−z2/8, |
The rth moment of Z can be expressed by
μ′r=∫∞0zr fTL-EE(z;θ,α,λ)dz=2αλθ−r∞∑i=0 πi(α,λ) Φi(t;r,α), | (3.2) |
where
πi(α,λ)=(−1)i(α−1i)(λ−1i) |
and
Φi(t;r,α)=∫10(log(t))r ti−1 (1−(1−t)α)2i+1dt. |
Proof.
μ′r=∫∞0zr2λαθe−θz(1−e−θz)α−1(1−(1−e−θz)α)(1−(1−(1−e−θz)α)2)λ−1 dz. |
By taking t=e−θz, then
μ′r=2λαθ−r∫10(log(t))rt(1−t)α−1(1−(1−t)α)(1−(1−(1−t)α)2)λ−1 dt. |
Using the series representation
(1−y)a−1=∞∑i=0(−1)i(a−1i) yi. |
The formula of the rth moment of Z can be written as
μ′r=2λαθ−r∫10∞∑i=0(−1)i(α−1i)(λ−1i)log(t))rti−1(1−(1−t)α) (1−(1−t)α)2i dt=2λαθ−r∫10∞∑i=0(−1)i(α−1i)(λ−1i)log(t))rti−1(1−(1−t)α)2i+1 dt=2αλθ−r∞∑i=0 πi(α,λ) Φi(t;r,α). |
From (3.2), the mean, 2nd moment, and variance of Z can be written as
μ′1=2αλθ∞∑i=0 πi(α,λ) Φi(t;1,α), |
μ′2=2αλθ2∞∑i=0 πi(α,λ) Φi(t;2,α), |
and
Var(Z)=μ′21−μ′2. |
Consequently, the coefficient of variation (CV) of Z is obtained to be
CV(Z)=√Var(Z)μ′1. |
At the end, The moment-generating function of Z can be expressed by
MZ(l)=E[elz]=2αλ∞∑k=0∞∑i=0 πi(α,λ) lkk! θk Φi(t;k,α). | (3.3) |
The proof is completed.
Proof.
MZ(l)=∫∞0elz 2λαθe−θz(1−e−θz)α−1(1−(1−e−θz)α)(1−(1−(1−e−θz)α)2)λ−1dz. |
By using the series representation
elz=∞∑i=0lkzkk! |
and taking
t=e−θz, |
we have
MZ(l)=2λα∫10∞∑k=0∞∑i=0(−1)i(α−1i)(λ−1i)lk log(t))kk!ti−1(1−(1−t)α)2i+1dt=2αλ∞∑k=0∞∑i=0 πi(α,λ) lkk! θk Φi(t;k,α). |
The cumulant generating function (CGF) KZ(l) of the TL-EE distribution can be resulted as
KZ(l)=log{2αλ∞∑k=0∞∑i=0 πi(α,λ) lkk! θk Φi(t;k,α)}. | (3.4) |
The characteristic function ϕZ(l) of the TL-EE model can be concluded from MZ(l) and it is given as
MZ(l)=E[eilz]=2αλ∞∑k=0∞∑i=0 πi(α,λ)ilkk! θk Φi(t;k,α), | (3.5) |
where
i=√−1 |
is the imaginary unit.
The different statistic measures of TL-EE distribution are recorded in Tables 1 and 2, whereas Figures 4 and 5 plotted the 3D curves by applying various selected values of the parameters. From the numerical results of Tables 1 and 2, we have:
θ | μ′1 | Var | CV | S | K | |
α=0.25 | 0.5 | 0.2460 | 0.2550 | 2.0523 | 4.2055 | 25.407 |
1 | 0.1230 | 0.0637 | 2.0523 | 4.2055 | 25.407 | |
1.5 | 0.0820 | 0.0283 | 2.0523 | 4.2055 | 25.407 | |
2 | 0.0615 | 0.0159 | 2.0523 | 4.2055 | 25.407 | |
α=0.5 | 0.5 | 0.6196 | 0.6258 | 1.2768 | 2.5999 | 9.7552 |
1 | 0.3098 | 0.1564 | 1.2768 | 2.5999 | 9.7552 | |
1.5 | 0.2065 | 0.0695 | 1.2768 | 2.5999 | 9.7552 | |
2 | 0.1549 | 0.0391 | 1.2768 | 2.5999 | 9.7552 | |
α=0.75 | 0.5 | 0.9702 | 0.9287 | 0.9932 | 2.0249 | 5.9692 |
1 | 0.4851 | 0.2321 | 0.9932 | 2.0249 | 5.9692 | |
1.5 | 0.3234 | 0.1031 | 0.9932 | 2.0249 | 5.9692 | |
2 | 0.2425 | 0.0580 | 0.9932 | 2.0249 | 5.9692 | |
α=1 | 0.5 | 1.2813 | 1.1606 | 0.8407 | 1.7251 | 4.3798 |
1 | 0.6406 | 0.2901 | 0.8407 | 1.7251 | 4.3798 | |
1.5 | 0.4271 | 0.1289 | 0.8407 | 1.7251 | 4.3798 | |
2 | 0.3203 | 0.0725 | 0.8407 | 1.7251 | 4.3798 |
θ | μ′1 | Var | CV | S | K | |
α=0.25 | 0.5 | 0.4325 | 0.4196 | 1.4976 | 3.1710 | 14.660 |
1 | 0.2162 | 0.1049 | 1.4976 | 3.1710 | 14.660 | |
1.5 | 0.1441 | 0.0466 | 1.4976 | 3.1710 | 14.66 | |
2 | 0.1081 | 0.0262 | 1.4976 | 3.1710 | 14.660 | |
α=0.5 | 0.5 | 0.9848 | 0.8709 | 0.9476 | 2.0392 | 6.1814 |
1 | 0.4924 | 0.2177 | 0.9476 | 2.0392 | 6.1814 | |
1.5 | 0.3282 | 0.0967 | 0.9476 | 2.0392 | 6.1814 | |
2 | 0.2462 | 0.0544 | 0.9476 | 2.0392 | 6.1814 | |
α=0.75 | 0.5 | 1.4485 | 1.1686 | 0.7462 | 1.6458 | 4.1135 |
1 | 0.7242 | 0.2921 | 0.7462 | 1.6458 | 4.1135 | |
1.5 | 0.4828 | 0.1298 | 0.7462 | 1.6458 | 4.1135 | |
2 | 0.3621 | 0.0730 | 0.7462 | 1.6458 | 4.1135 | |
α=1 | 0.5 | 1.8340 | 1.3685 | 0.6378 | 1.4452 | 3.2372 |
1 | 0.9170 | 0.3421 | 0.6378 | 1.4452 | 3.2372 | |
1.5 | 0.6113 | 0.1520 | 0.6378 | 1.4452 | 3.2372 | |
2 | 0.4585 | 0.0855 | 0.6378 | 1.4452 | 3.2372 |
(1) The measures of Mean and Var of Z decrease when θ tends to be increases with fixed α and λ, and CV, S, and K measures have constants values, which indicate that these measures are free of parameter of θ.
(2) If α tends to be increases with θ and λ are fixed, the Mean and Var measures augment, whereas the CV, S and K decrease.
(3) The proposed TL-EE model is more efficient in analyzing more datasets.
Let Z1:n≤Z2:n≤…≤Zn:n denote the order statistic of a random sample of size n Z1,Z2,…,Zn from the TL-EE model. The PDF of kth order statistic of Zk:n can be formulated as
gZk:n(x)=n!(k−1)!(n−k)![F(z)]k−1[1−F(z)]n−kf(z). | (4.1) |
With replacing (4.1), we get
gZk:n(x)=2λαθe−θzn!(k−1)!(n−k)![1−(1−(1−e−θz)α)2]λk−1×[1−(1−(1−(1−e−θz)α)2)λ]n−k×(1−e−θz)α−1(1−(1−e−θz)α). | (4.2) |
Specifically, we can obtain the PDF of the first and latest order statistics
Z1:n=min{Z1,Z2,...,Zn}andZn:n=max{Z1,Z2,...,Zn} |
and they are given, respectively, by
gZ1:n(x)=2nλαθe−θz[1−(1−(1−e−θz)α)2]λ−1×[1−(1−(1−(1−e−θz)α)2)λ]n−1×(1−e−θz)α−1(1−(1−e−θz)α) | (4.3) |
and
gZn:n(x)=2nλαθe−θz[1−(1−(1−e−θz)α)2]λn−1×(1−e−θz)α−1(1−(1−e−θz)α). | (4.4) |
At the end, the rth order moment of Zr:n for the TL-EE model is written as follows
E(Zrk:n)=∫∞0zr⋅gZk:n(z)dz, |
where gZk:n(z) is given in (4.2).
Rényi entropy is a very important tool in information measure. It is introduced as
R(p)=11−plog(∫∞0[f(z;θ,α,λ)]pdz),p≠1,p>0. |
For the TL-EE model, we get
I=∫∞0[f(z;θ,α,λ)]p=(2αλθ)p∫∞0e−pθz(1−e−θz)p(α−1)×(1−(1−e−θz)p)αdz×(1−(1−(1−e−θz)α)2)p(λ−1). |
By taking t=e−θz, we have
I=2pαpλpθp−1∫10tp(1−t)p(α−1)(1−(1−t)p)α×(1−(1−(1−t)α)2)p(λ−1)dt. |
Using the series representation,
(1−y)a−1=∞∑i=0(−1)i(a−1i) yi. |
So, we have
I=2pαpλpθp−1∫10∞∑i=0(−1)i(p(α−1)i) tp+i(1−(1−t)p)α×(1−(1−(1−t)α)2)p(λ−1)dt=2pαpλpθp−11−p∞∑i=0ηi(α,p)Ψi(t,α,λ,p) |
with
ηi(α,p)=(−1)i(p(α−1)i) |
and
Ψi(t,α,λ,p)=∫10tp+i(1−(1−t)p)α(1−(1−(1−t)α)2)p(λ−1)dt. |
Consequently, Rényi entropy of the TL-EE distribution is given by
R(p)=11−plog{2pαpλpθp−11−p∞∑i=0ηi(α,p)Ψi(t,α,λ,p)}. |
Suppose z1,z2,…,zn are n observations from the TL-EE model. Furthermore, the log-likelihood function corresponding to Eq (2.2) can be written as
LL(Ω)=nlog(2λαθ)−θn∑i=1zi+(α−1)n∑i=1log(1−e−θzi)+(λ−1)n∑i=1log(1−(1−(1−e−θzi)α)2)+n∑i=1log(1−(1−e−θzi)α). | (6.1) |
Here, Ω=(θ,α,λ). To get the MLEs of θ, α, and λ, we maximize Eq (6.1) with respect to the unknown parameters and then equate the result to zero. That is,
∂l∂θ=nθ+n∑i=1αzie−θzi(1−e−θzi)(α−1)(1−(1−e−θzi)α)−2n∑i=1(1−(1−e−θzi)α)αzie−θzi(1−e−θzi)(α−1)1−(1−(1−e−θzi)α)2−n∑i=1zi+n∑i=1zie−θzi1−e−θzi=0, | (6.2) |
∂l∂α=nα−n∑i=1(1−e−θzi)αlog(1−e−θzi)(1−(1−e−θzi)α)−2n∑i=1((1−e−θzi)α−1)(1−e−θzi)αlog(1−e−θzi)(1−(1−(1−e−θzi)α)2)+n∑i=1log(1−e−θzi)=0, | (6.3) |
and
∂l∂λ=nλ+∑ni=1log(1−(1−(1−e−θzi)α)2)=0. | (6.4) |
Clearly, from Eqs (6.2)–(6.4) the final estimates cannot be resulted in explicit form. To overcome this problem, numerous approximate techniques such as bisection method, fixed point, and Newton-Raphson are produced to obtain the final estimates of Ω.
Here, we perform an Monte Carlo Markov Chain simulation analysis to examine the potential of the MLEs of the proposed model for different sample sizes n={100,250,500,705,1000} using the quantile function of the proposed model and for different parameter sets
Set1=(θ=0.6,α=0.8,λ=0.5), |
Set2=(θ=0.25,α=0.5,λ=0.7), |
Set3=(θ=0.75,α=1.0,λ=0.9) |
and
Set4=(θ=0.8,α=0.25,λ=1.2). |
The above steps are provided to obtain a random sample from the TL-EE model:
Step 1. Drawn u from uniform with interval [0,1].
Step 2. Obtain z as
z=−1θlog{1−[1−(1−u1/λ)1/2]1/α}. |
We computed the average estimate (AE), the average mean square errors (MSEs), the average biases (AB), and associated mean relative errors (MREs) based on M=1000 times. These values are given as follows:
AE=1MM∑i=1^εε,AB=1MM∑i=1|^εε−εε|,MSE=1MM∑i=1(^εε−εε)2,MRE=1MM∑i=1|^εε−εε|/εε, |
where
εε=(θ,α,λ). |
Tables 3–6 summarize the results of the simulation study of the TL-EE model. It is noted from the results that a the AEs of all parameters converge to the actual values of parameters. Also, the ABs, MSEs, and MREs decrease as n tends to be increase based on MLE technique which ensure that the estimates of unknown parameters are consistent and asymptotically unbiased.
Simple size | Est. | ˆθ | ˆα | ˆλ |
100 | AE | 0.6932 | 1.3583 | 1.0299 |
AB | 0.0932 | 0.5583 | 0.5299 | |
MSE | 0.0664 | 1.4037 | 1.3624 | |
MRE | 0.1553 | 0.6979 | 1.0598 | |
250 | AE | 0.6341 | 1.0291 | 0.6319 |
AB | 0.0341 | 0.2291 | 0.1319 | |
MSE | 0.0225 | 0.4660 | 0.3173 | |
MRE | 0.0569 | 0.2864 | 0.2638 | |
500 | AE | 0.6255 | 0.9456 | 0.5500 |
AB | 0.0255 | 0.1456 | 0.0500 | |
MSE | 0.0130 | 0.2324 | 0.0889 | |
MRE | 0.0426 | 0.1820 | 0.1001 | |
750 | AE | 0.6075 | 0.8852 | 0.5434 |
AB | 0.0075 | 0.0852 | 0.0434 | |
MSE | 0.0054 | 0.1477 | 0.0581 | |
MRE | 0.0125 | 0.1065 | 0.0869 | |
1000 | AE | 0.6047 | 0.8728 | 0.5401 |
AB | 0.0047 | 0.0728 | 0.0401 | |
MSE | 0.0043 | 0.1318 | 0.0519 | |
MRE | 0.0078 | 0.0911 | 0.0803 |
Simple size | Est. | ˆθ | ˆα | ˆλ |
100 | AE | 0.3099 | 1.0043 | 0.8253 |
AB | 0.0599 | 0.5043 | 0.1253 | |
MSE | 0.0143 | 0.8638 | 1.5698 | |
MRE | 0.2396 | 1.0086 | 0.1790 | |
250 | AE | 0.2668 | 0.6964 | 0.8021 |
AB | 0.0168 | 0.1964 | 0.1021 | |
MSE | 0.0052 | 0.2768 | 0.4464 | |
MRE | 0.0674 | 0.3928 | 0.1636 | |
500 | AE | 0.2485 | 0.5278 | 0.8099 |
AB | 0.0014 | 0.0278 | 0.1099 | |
MSE | 0.0019 | 0.0493 | 0.1588 | |
MRE | 0.0056 | 0.0557 | 0.1570 | |
750 | AE | 0.2552 | 0.5480 | 0.7556 |
AB | 0.0052 | 0.04806 | 0.0556 | |
MSE | 0.0016 | 0.0596 | 0.1034 | |
MRE | 0.0208 | 0.0961 | 0.0795 | |
1000 | AE | 0.2553 | 0.5312 | 0.7357 |
AB | 0.0053 | 0.0312 | 0.03574 | |
MSE | 0.0011 | 0.0268 | 0.0693 | |
MRE | 0.0210 | 0.0624 | 0.0510 |
Simple size | Est. | ˆθ | ˆα | ˆλ |
100 | AE | 0.8480 | 2.1978 | 1.9580 |
AB | 0.0980 | 1.1978 | 1.0580 | |
MSE | 0.0665 | 1.2910 | 1.7854 | |
MRE | 0.1307 | 1.1978 | 1.1756 | |
250 | AE | 0.8246 | 1.5339 | 1.3104 |
AB | 0.0346 | 0.5339 | 0.4104 | |
MSE | 0.0302 | 1.0916 | 1.6425 | |
MRE | 0.0861 | 0.5339 | 0.4560 | |
500 | AE | 0.7731 | 1.2048 | 1.1657 |
AB | 0.0231 | 0.2048 | 0.2657 | |
MSE | 0.0130 | 0.6911 | 1.1267 | |
MRE | 0.0275 | 0.2048 | 0.2953 | |
750 | AE | 0.7656 | 1.1924 | 0.9820 |
AB | 0.0156 | 0.1924 | 0.0820 | |
MSE | 0.0087 | 0.4560 | 0.3348 | |
MRE | 0.0208 | 0.1924 | 0.0911 | |
1000 | AE | 0.7653 | 1.1837 | 0.9436 |
AB | 0.0153 | 0.1837 | 0.0436 | |
MSE | 0.0082 | 0.4025 | 0.1537 | |
MRE | 0.0204 | 0.1837 | 0.0485 |
Simple size | Est. | ˆθ | ˆα | ˆλ |
100 | AE | 0.9913 | 0.4293 | 2.4947 |
AB | 0.1913 | 0.1793 | 1.2947 | |
MSE | 0.1833 | 0.2143 | 1.9780 | |
MRE | 0.2391 | 0.7172 | 1.0789 | |
250 | AE | 0.8291 | 0.3066 | 1.5424 |
AB | 0.0291 | 0.0566 | 0.3424 | |
MSE | 0.0882 | 0.06599 | 1.4114 | |
500 | AE | 0.8240 | 0.2643 | 1.3341 |
AB | 0.0240 | 0.0143 | 0.1341 | |
MSE | 0.0217 | 0.0087 | 0.3792 | |
MRE | 0.0300 | 0.0572 | 0.1118 | |
750 | AE | 0.8172 | 0.2658 | 1.2881 |
AB | 0.0172 | 0.0140 | 0.0881 | |
MSE | 0.0163 | 0.0070 | 0.2778 | |
MRE | 0.0208 | 0.0653 | 0.0734 | |
1000 | AE | 0.8163 | 0.2650 | 1.2237 |
AB | 0.0163 | 0.0150 | 0.02376 | |
MSE | 0.0137 | 0.0048 | 0.1518 | |
MRE | 0.0204 | 0.0602 | 0.0198 |
The exposure market risk in a portfolio of instruments is one of the most important tasks of actuarial sciences. In the last decades, various characteristics of risk measures have been considered for example, one may refer to [47,48,49]). This part of the study introduces the final expression of VaR, TVaR, and TVP measures for the TL-EE model.
The VaR at level significance q defined the quantile function of proposed model, which introducing the percentage loss in the portfolio value. Let Z follows the TL-EE distribution. The expression of VaR, denoted by T1, for the TL-EE is given by
VaRq(Z)=inf{z:F(z)>q}=F−1Z(q), |
WHERE 0<q<1. Hence, the VaR of Z is
T1=−1θlog{1−[1−(1−q1/λ)1/2]1/α}. | (8.1) |
The TVaR or conditional tail expectation quantifies the average of losses above the VaR for some given confidence level. The TVaR, denoted by T2 for the proposed TL-EE model, is determined by using the following relation
T2=E[Z∣Z>VaRq(Z)]=11−q∫∞VaRqzfZ(z;θ,α,λ)dz=2αλθ(1−q)∞∑i=0 πi(α,λ) Φi(t;1,α) |
with
Φi(t;1,α)=∫∞VaRqlog(t) ti−1 (1−(1−t)α)2i+1dt. |
The TVP has great importance in the portfolio sector. The expression of TVP, denoted T4, of the TL-EE model is defined by the following equation:
T4=T2+δT3, |
where 0<δ<1 and
T3=1(1−q)∫∞VaRqz2fZ(z;θ,α,λ) dz−(T2)2. |
The numerical experiments for T1, T2, and T3 of the TL-EE model and other well known distributions such as EE and Exp for various parametric values are shown in this part. The parameters were calculated using the ML approach. The three risk metrics were computed using the 1000 replications of process, and the final results were summarized in Tables 7 and 8.
Model | Par | q | T1 | T2 | T4 |
TL-EE | θ=2.5 | 0.60 | 1.6234 | 1.8534 | 1.8810 |
α=4.25 | 0.65 | 1.6583 | 1.8839 | 1.9132 | |
λ=100 | 0.70 | 1.6969 | 1.9183 | 1.9493 | |
0.75 | 1.7408 | 1.9583 | 1.9910 | ||
0.80 | 1.7926 | 2.0064 | 2.0407 | ||
0.85 | 1.8570 | 2.0673 | 2.1032 | ||
0.90 | 1.9449 | 2.1519 | 2.1892 | ||
0.95 | 2.0903 | 2.2941 | 2.3329 | ||
EE | θ=2.5 | 1.3107 | 1.4156 | 1.2374 | 1.4161 |
α=4.25 | 0.65 | 0.9357 | 1.3689 | 1.4812 | |
0.70 | 1.0078 | 1.4353 | 1.5546 | ||
0.75 | 1.0905 | 1.5127 | 1.6391 | ||
0.80 | 1.1891 | 1.6063 | 1.7397 | ||
0.85 | 1.3131 | 1.7256 | 1.8658 | ||
0.90 | 1.4838 | 1.8919 | 2.0388 | ||
0.95 | 1.7692 | 2.1731 | 2.3266 | ||
Exp | θ=2.5 | 0.60 | 0.3665 | 0.7665 | 0.8625 |
0.65 | 0.4199 | 0.8199 | 0.9239 | ||
0.70 | 0.4815 | 0.8815 | 0.9935 | ||
0.75 | 0.5545 | 0.9545 | 1.0745 | ||
0.80 | 0.6437 | 1.0437 | 1.1717 | ||
0.85 | 0.7588 | 1.1588 | 1.2948 | ||
0.90 | 0.9210 | 1.3210 | 1.4650 | ||
0.95 | 1.1982 | 1.5982 | 1.7502 |
Model | Par | q | T1 | T2 | T4 |
TL-EE | θ=4.0 | 0.60 | 1.0991 | 1.2434 | 1.2543 |
α=6.5 | 0.65 | 1.1211 | 1.2625 | 1.2740 | |
λ=85 | 0.70 | 1.1453 | 1.2841 | 1.2963 | |
0.75 | 1.1729 | 1.3091 | 1.3220 | ||
0.80 | 1.2054 | 1.3393 | 1.3527 | ||
0.85 | 1.2458 | 1.3775 | 1.3915 | ||
0.90 | 1.3008 | 1.4304 | 1.4450 | ||
0.95 | 1.3919 | 1.5194 | 1.5346 | ||
EE | θ=4.0 | 0.60 | 0.6456 | 0.9230 | 0.9644 |
α=6.5 | 0.65 | 0.6867 | 0.9598 | 1.0040 | |
0.70 | 0.7325 | 1.00159 | 1.0485 | ||
0.75 | 0.7849 | 1.0502 | 1.0999 | ||
0.80 | 0.8472 | 1.1091 | 1.1614 | ||
0.85 | 0.9253 | 1.1839 | 1.2388 | ||
0.90 | 1.0325 | 1.2881 | 1.3456 | ||
0.95 | 1.2114 | 1.4642 | 1.5242 | ||
Exp | θ=4.0 | 0.60 | 0.2290 | 0.4790 | 0.5165 |
0.65 | 0.2624 | 0.5124 | 0.5530 | ||
0.70 | 0.3009 | 0.5509 | 0.5947 | ||
0.75 | 0.3465 | 0.5965 | 0.6434 | ||
0.80 | 0.4023 | 0.6523 | 0.7023 | ||
0.85 | 0.4742 | 0.7242 | 0.7774 | ||
0.90 | 0.5756 | 0.8256 | 0.8818 | ||
0.95 | 0.7489 | 0.9989 | 1.0583 |
For visual comparisons, we presented the findings visually as shown in Figures 6 and 7. From these results and Figures, we conclude that the TL-EE model is heavier than EE and Exp distributions which make it very suitable to fit heavy tailed datasets.
In this section, we consider the data from fire losses, and it contains records of all the total loss amounts in thousands of Norwegian Krone (NKR) from 1972 to 1992. The recorded datasets are taken from norfire function () containing in the CASdatasets package [50], and it was also studied by [51]. Table 9 reported different characteristic measures of the proposed dataset. The skewness measure refers that the recorded data is positively skewed.
Mean | Q2 | Sd | Q1 | Q3 | Skew | Kurt |
520.0 | 573.5 | 134.7 | 676.0 | 697.1 | 772.5 | 982.0 |
The adaptability of the TL-EE model is made by discussing its efficacy to that of other analogous models like EE, Exp, Poisson Lomax (PL) (see [52]), Poisson exponential (PE) (see [53]), Weibull (Wei), Lindley (Lin), zero truncated Poisson gamma (ZTPGA) (see [3]), Power Lindley (PLin) (see [54]), Exponential geometric (EG) (see [55]), and Two parameters Mira (TPM) (see [56]) distributions. The PDFs of the suggested models are:
(1) PL distribution:
f(z;α,λ,θ)=αθλ(eθ−1) (1+z/λ)α−1 eθ(1−(1+z/λ)−α); |
where z, α,λ,θ>0.
(2) PE distribution:
f(z;λ,θ)=λθeθ−1 e−λz+θ(1−e−λz);wherez>0, λ,θ>0. |
(3) Exp distribution:
f(z;λ)=λe−λz;wherez>0, λ>0. |
(4) Lin distribution:
f(z;λ)=λ2λ+1(1+z)e−λz;wherez>0, λ>0. |
(5) Wei distribution:
f(z;μ,σ)=μσ(zσ)μ−1 e−(zσ)μ;wherez>0, μ,σ>0. |
(6) ZTPGA:
f(z,α,λ,θ)=θλαzα−1e−λz(eθ−1)Γ(α)eθHα,λ(z); |
where zx>0, α,λ,θ>0, and Hα,λ(z) is the CDF of gamma distribution.
(7) PLin:
f(z,α,β)=αβ2β+1(1+zα) zα−1 e−βzα;wherez>0, α,β>0. |
(8) EG:
f(z,λ,p)=pλe−λz(p+(1−p)e−λx)2; |
where z>0, λ>0, 0<p<1.
(9) TPM:
f(z,α,δ)=δ3(αz2+2)e−δz2(α+δ2);wherez>0, α,δ>0. |
The results of the MLEs and the corresponding log-likelihood (ll) function of TL-EE model with proposed compared distributions are reported in Table 10. Now, To determination about the best model for modeling the dataset, numerous measures including Akaike information criterion (A1), correction Akaike information criterion (A2), Hannan Quinn information criterion (A3), Bayesian Information criterion (B1) and Kolmogorov-Smirnov (KS) statistics with associated P-values are computed. The results of all these measures are recorded in Table 11. From these results, we can deduce that the TL-EE model is a more suitable candidate distribution for analyzing the Norwegian fire insurance dataset. The estimated PDF, CDF, and survival function of the TL-EE model with the empirical dataset, the scaled total time on the test (TTT), the probability-probability (PP), and box plots for the Norwegian fire insurance dataset are sketched in Figure 8 and 9. These figures confirms this conclusion.
Distribution | Par | ll |
TL-EE | ˆθ=0.0048 ˆα=2.8201 ˆλ=67.987 | -344.054 |
EE | ˆθ=0.0064 ˆα=55.743 | -349.589 |
PL | ˆα = 470.909 ˆλ=590.227 ˆθ=8.4518 | -348.5498 |
PE | ˆλ=0.0065 ˆθ=59.178 | -349.538 |
Wei | ˆμ=5.5134 ˆσ= 753.625 | -349.229 |
Exp | ˆλ=0.0014 | -415.080 |
Lin | ˆλ=0.0028 | -394.886 |
ZTPGA | ˆα=5.0374 ˆλ=0.0146 ˆθ=21.882 | -344.680 |
PLin | ˆα=0.0786 ˆβ= 2.1419 | -380.426 |
EG | ˆα=0.0043 ˆp= 0.0858 | -380.322 |
TPM | ˆα=0.0043 ˆδ= 0.8077 | -383.885 |
Model | A1 | A2 | A3 | B1 | KS | P-value |
TL-EE | 694.109 | 694.580 | 696.438 | 700.131 | 0.1018 | 0.618 |
EE | 703.178 | 704.409 | 705.731 | 709.193 | 0.1320 | 0.292 |
PL | 703.099 | 703.570 | 705.428 | 709.121 | 0.1190 | 0.417 |
PE | 703.077 | 703.308 | 704.630 | 707.092 | 0.1357 | 0.262 |
Wei | 702.458 | 702.689 | 704.011 | 706.473 | 0.1212 | 0.393 |
Exp | 832.160 | 832.236 | 832.937 | 834.168 | 0.5257 | 1.2×10−13 |
Lin | 791.772 | 791.847 | 792.548 | 793.779 | 0.4396 | 1.1×10−9 |
ZTPGA | 695.360 | 695.831 | 697.689 | 701.382 | 0.1046 | 0.5839 |
PLin | 764.852 | 765.082 | 766.404 | 768.866 | 0.3852 | 2.5×10−06 |
EG | 764.645 | 764.876 | 766.198 | 768.660 | 0.38.26 | 7.5×10−06 |
TPM | 771.770 | 772.001 | 773.323 | 775.785 | 0.3873 | 1.36×10−07 |
Further, we compute the risk measures of the TL-EE, EE, and Exp models using the Norwegian fire insurance dataset. The results are displayed in Table 12, and it can be concluded that the values of actuarial measures of the TL-EE distribution are very close to their corresponding empirical values for the Norwegian fire dataset. The suggested TL-EE model is very suitable to fit and analyze the Norwegian fire dataset. Figure 10 confirms this conclusion.
Model | Par | q | T1 | T2 | T4 |
Empirical | 0.85 | 875.8 | 925.7 | 982.0 | |
0.90 | 917.8 | 939.6 | 1285.2 | ||
0.95 | 933.6 | 955.6 | 1335.8 | ||
0.99 | 1041.1 | 1194.8 | 1571.9 | ||
TL-EE | ˆθ=0.0048 | 0.85 | 825.0 | 932.4 | 10280.6 |
ˆα=2.8201 | 0.90 | 869.9 | 975.6 | 10714.9 | |
ˆλ=67.987 | 0.95 | 944.2 | 1048.2 | 11156.3 | |
0.99 | 1111.6 | 1214.3 | 11601.9 | ||
EE | ˆα=55.743 | 0.85 | 910.8 | 1073.0 | 22573.1 |
ˆθ=0.0064 | 0.90 | 978.3 | 138.4 | 23600.3 | |
0.95 | 1090.5 | 1248.5 | 24654.0 | ||
0.99 | 1344.7 | 1501.1 | 25649.0 | ||
Exp | ˆλ= 0.0014 | 0.85 | 1322.4 | 2019.5 | 415065.0 |
0.90 | 1605.1 | 2302.2 | 439644.4 | ||
0.95 | 2088.2 | 2785.3 | 464424.4 | ||
0.99 | 33210.2 | 3907.3 | 484983.8 |
In this subsection, we presented the dataset from a group medical insurance and it contains values of all the claim amounts exceeding 25,000 USD over the period 1991. The values of dataset were taken from http://www.soa.org. Before progressing further, let us provide some basic statistics of the observed value of dataset, which is provided in Table 13. The skewness measure give first indicator that the proposed data is skewed to the right, and the plot of TTT, PP, and box are displayed in Figure 11.
Mean | Q2 | Sd | Q1 | Q3 | Skew | Kurt |
487.2 | 427.8 | 248.0 | 301.1 | 585.4 | 1.932 | 4.471 |
The TL-EE distribution and the other proposed models are estimated by using the MLE method. Table 14 summarized the results of the MLEs and the corresponding ll function. Furthermore, to select the model which fits the dataset well, we compute various statistical measures such as A1, A2, A3, B1, KS, and P-value, and the are reported in Table 15. From these results, we can deduce that the TL-EE model is a more suitable candidate distribution for analyzing the dataset. The estimated PDF, CDF, and survival function of the TL-EE model with the empirical dataset are drawn in Figure 12. This figure ensures this conclusion.
Distribution | Par | ll |
TL-EE | ˆθ=0.0029 ˆα=0.4591 ˆλ=38.326 | -331.867 |
EE | ˆθ=0.0049 ˆα=5.7608 | -334.355 |
PL | ˆα = 133.818 ˆλ=190.851 ˆθ=3.9591 | -332.767 |
PE | ˆλ=0.0068 ˆθ=14.346 | -333.323 |
Wei | ˆμ=2.1057 ˆσ= 552.523 | -340.802 |
Exp | ˆλ=0.0020 | -359.435 |
Lin | ˆλ=0.0040 | -345.044 |
ZTPGA | ˆα=1.8306 ˆλ=0.0077 ˆθ=6.1417 | -335.129 |
PLin | ˆα=1.5245 ˆβ= 0.0001 | -337.759 |
EG | ˆα=0.0076 ˆp= 0.0338 | -339.999 |
TPM | ˆα=0.0062 ˆδ= 0.3874 | -338.991 |
Model | A1 | A2 | A3 | B1 | KS | P-value |
TL-EE | 669.734 | 670.256 | 671.919 | 675.470 | 0.1089 | 0.5567 |
EE | 672.711 | 672.967 | 674.167 | 676.535 | 0.1470 | 0.2082 |
PL | 671.534 | 672.055 | 673.718 | 677.270 | 0.1124 | 0.5162 |
PE | 670.646 | 670.901 | 672.102 | 674.470 | 0.1181 | 0.4535 |
Wei | 685.604 | 685.859 | 687.060 | 689.428 | 0.1803 | 0.0678 |
Exp | 720.871 | 720.954 | 721.599 | 722.783 | 0.4094 | 4.2×10−08 |
Lin | 692.089 | 692.172 | 692.817 | 694.001 | 0.2846 | 0.0004 |
ZTPGA | 676.258 | 676.780 | 678.442 | 681.994 | 0.1104 | 0.5383 |
PLin | 679.518 | 679.773 | 680.974 | 683.342 | 0.1520 | 0.1784 |
EG | 683.998 | 684.253 | 685.454 | 687.822 | 0.1707 | 0.0961 |
TPM | 681.982 | 682.237 | 683.438 | 685.806 | 0.2147 | 0.0167 |
Finally, we provide the results of risk measures of the TL-EE, EE, and Exp models using the medical insurance claim dataset. The results are given in Table 16, and it can be concluded that the values of actuarial measures of the TL-EE model are very close to their corresponding empirical values for the medical insurance claim dataset. Hence, the proposed TL-EE model is very suitable to fit and analyze the medical insurance claim dataset. Figure 13 ensures this result.
Model | Par | q | T1 | T2 | T4 |
Empirical | 0.85 | 664.3 | 925.8 | 11899.1 | |
0.90 | 726.9 | 1071.7 | 7070.6 | ||
0.95 | 910.2 | 1207.3 | 3901.6 | ||
0.99 | 1347.8 | 1500.3 | 1500.3 | ||
TL-EE | ˆθ=0.0029 | 0.85 | 687.2 | 942.0 | 26230.5 |
ˆα=0.4591 | 0.90 | 759.2 | 1027.0 | 27617.5 | |
ˆλ=38.326 | 0.95 | 879.9 | 1170.6 | 29096.2 | |
0.99 | 1156.6 | 1500.8 | 30579.1 | ||
EE | ˆα=0.0049 | 0.85 | 731.0 | 861.8 | 37517.5 |
ˆθ=5.7608 | 0.90 | 818.4 | 932.3 | 39320.7 | |
0.95 | 964.4 | 1051.9 | 41155.2 | ||
0.99 | 1296.3 | 1328.3 | 42819.2 | ||
Exp | ˆλ= 0.0020 | 0.85 | 948.5 | 1448.5 | 213948.6 |
0.90 | 1151.2 | 1651.2 | 226651.3 | ||
0.95 | 1497.8 | 1997.8 | 239499.0 | ||
0.99 | 2302.5 | 2802.5 | 250302.6 |
This article uses a new class of distributions based on a TL family of distributions. It is named a TL-EE model. Different distributional and mathematical properties of this model are provided including, rth moment, moment generating and characteristic function as well as distribution of order statistic and Reny entropy. The MLE was used to estimate the parameters of the proposed distribution. Various actuarial measures are computed, and brief simulation analyses are illustrated to see the efficiency of the TL-EE in risk insurance. In the end, we have illustrated two financial datasets, and it is checked that the TL-EE model regularly outperforms other competitor distributions. In future work, we will use various censored methods, including progressive censoring shames under different types along with illustration of accelerated life tests with numerous kinds of stress load.
The author declares he has not used Artificial Intelligence (AI) tools in the creation of this article.
The author acknowledges the reviwers for their comments.
The author declares that there are is conflict of interest in this paper.
![]() |
![]() |
![]() |
![]() |
[1] |
C. Argaez, M. Melgaard, Solutions to quasi-relativistic multi-configurative Hartree–Fock equations in quantum chemistry, Nonlinear Anal. Theor., 75 (2012), 384–404. doi: 10.1016/j.na.2011.08.038
![]() |
[2] | D. R. Adams, L. I. Hedberg, Function spaces and potential theory, Berlin Heidelberg: Springer, 1996. |
[3] |
M. Badiale, L. Pisani, S. Rolando, Sum of weighted Lebesgue spaces and nonlinear elliptic equations, Nonlinear Differ. Equ. Appl., 18 (2011), 369–405. doi: 10.1007/s00030-011-0100-y
![]() |
[4] |
P. Belchior, H. Bueno, O. H. Miyagaki, G. A. Pereira, Remarks about a fractional Choquard equation: ground state, regularity and polynomial decay, Nonlinear Anal., 164 (2017), 38–53. doi: 10.1016/j.na.2017.08.005
![]() |
[5] |
H. Berestycki, P.-L. Lions, Nonlinear scalar field equations. I. Existence of a ground state, Arch. Rational Mech. Anal., 82 (1983), 313–345. doi: 10.1007/BF00250555
![]() |
[6] | C. Bucur, E. Valdinoci, Nonlocal diffusion and applications, Springer Nature Switzerland AG, 2016. |
[7] |
J. Byeon, O. Kwon, J. Seok, Nonlinear scalar field equations involving the fractional Laplacian, Nonlinearity, 30 (2017), 1659–1681. doi: 10.1088/1361-6544/aa60b4
![]() |
[8] | H. Brezis, T. Kato, Remarks on the Schrödinger operator with singular complex potential, J. Math. Pure. Appl., 58 (1979), 137–151. |
[9] |
X. Cabré, Y. Sire, Nonlinear equations for fractional Laplacians, I: regularity, maximum principles, and Hamiltonian estimates, Ann. Inst. H. Poincaré Anal. Non Linéaire, 31 (2014), 23–53. doi: 10.1016/j.anihpc.2013.02.001
![]() |
[10] |
L. Caffarelli, L. Silvestre, An extension problem related to the fractional Laplacian, Commun. Part. Diff. Eq., 32 (2007), 1245–1260. doi: 10.1080/03605300600987306
![]() |
[11] |
X. Chang, Z.-Q. Wang, Ground state of scalar field equations involving a fractional Laplacian with general nonlinearities, Nonlinearity, 26 (2013), 479–494. doi: 10.1088/0951-7715/26/2/479
![]() |
[12] |
Y. Cho, G. Hwang, H. Hajaiej, T. Ozawa, On the Cauchy problem of fractional Schrödinger equation with Hartree type nonlinearity, Funkc. Ekvacioj, 56 (2013), 193–224. doi: 10.1619/fesi.56.193
![]() |
[13] |
Y. Cho, M. M. Fall, H. Hajaiej, P.A. Markowich, S. Trabelsi, Orbital stability of standing waves of a class of fractional Schrödinger equations with Hartree-type nonlinearity, Anal. Appl., 15 (2017), 699–729. doi: 10.1142/S0219530516500056
![]() |
[14] |
S. Cingolani, M. Clapp, S. Secchi, Multiple solutions to a magnetic nonlinear Choquard equation, Z. Angew. Math. Phys., 63 (2012), 233–248. doi: 10.1007/s00033-011-0166-8
![]() |
[15] |
S. Cingolani, M. Gallo, On the fractional NLS equation and the effects of the potential well's topology, Adv. Nonlinear Stud., 21 (2021), 1–40. doi: 10.1515/ans-2020-2114
![]() |
[16] |
S. Cingolani, M. Gallo, K. Tanaka, Normalized solutions for fractional nonlinear scalar field equation via Lagrangian formulation, Nonlinearity, 34 (2021), 4017–4056. doi: 10.1088/1361-6544/ac0166
![]() |
[17] |
S. Cingolani, M. Gallo, K. Tanaka, Symmetric ground states for doubly nonlocal equations with mass constraint, Symmetry, 13 (2021), 1199. doi: 10.3390/sym13071199
![]() |
[18] |
S. Cingolani, K. Tanaka, Deformation argument under PSP condition and applications, Anal. Theory Appl., 37 (2021), 191–208. doi: 10.4208/ata.2021.pr80.03
![]() |
[19] | S. Cingolani, M. Gallo, K. Tanaka, Multiple solutions for the nonlinear Choquard equation with even or odd nonlinearities, Calc. Var., in press. |
[20] |
M. Clapp, D. Salazar, Positive and sign changing solutions to a nonlinear Choquard equation, J. Math. Anal. Appl., 407 (2013), 1–15. doi: 10.1016/j.jmaa.2013.04.081
![]() |
[21] |
S. Coleman, V. Glaser, A. Martin, Action minima among solutions to a class of Euclidean scalar field equations, Commun. Math. Phys., 58 (1978), 211–221. doi: 10.1007/BF01609421
![]() |
[22] |
A. Dall'Acqua, T. Ø. Sørensen, E. Stockmeyer, Hartree-Fock theory for pseudorelativistic atoms, Ann. Henri Poincaré, 9 (2008), 711–742. doi: 10.1007/s00023-008-0370-z
![]() |
[23] |
P. D'Avenia, G. Siciliano, M. Squassina, On the fractional Choquard equations, Math. Mod. Meth. Appl. Sci., 25 (2015), 1447–1476. doi: 10.1142/S0218202515500384
![]() |
[24] |
P. D'Avenia, G. Siciliano, M. Squassina, Existence results for a doubly nonlocal equation, São Paulo J. Math. Sci., 9 (2015), 311–324. doi: 10.1007/s40863-015-0023-3
![]() |
[25] |
E. Di Nezza, G. Palatucci, E. Valdinoci, Hitchhiker's guide to the fractional Sobolev spaces, Bull. Sci. Math., 136 (2012), 521–573. doi: 10.1016/j.bulsci.2011.12.004
![]() |
[26] | S. Dipierro, M. Medina, E. Valdinoci, Fractional elliptic problems with critical growth in the whole of Rn, Pisa: Edizioni della Normale, 2017. |
[27] |
L. Dong, D. Liu, W. Qi, L. Wang, H. Zhou, P. Peng, et al., Necklace beams carrying fractional angular momentum in fractional systems with a saturable nonlinearity, Commun. Nonlinear Sci. Numer. Simul., 99 (2021), 105840. doi: 10.1016/j.cnsns.2021.105840
![]() |
[28] |
P. Felmer, A. Quaas, J. Tan, Positive solutions of the nonlinear Schrödinger equations with the fractional Laplacian, P. Roy. Soc. Edinb. A, 142 (2012), 1237–1262. doi: 10.1017/S0308210511000746
![]() |
[29] | R. L. Frank, E. Lenzmann, On ground states for the L2-critical boson star equation, arXiv: 0910.2721. |
[30] |
R. L. Frank, E. Lenzmann, L. Silvestre, Uniqueness of radial solutions for the fractional Laplacian, Commun. Pure Appl. Math., 69 (2016), 1671–1726. doi: 10.1002/cpa.21591
![]() |
[31] |
J. Fröhlich, B. L. G. Jonsson, E. Lenzmann, Boson stars as solitary waves, Commun. Math. Phys., 274 (2007), 1–30. doi: 10.1007/s00220-007-0272-9
![]() |
[32] | J. Fröhlich, E. Lenzmann, Mean-field limit of quantum Bose gases and nonlinear Hartree equation, In: Séminaire Équations aux dérivées partielles (Polytechnique) dit aussi "Séminaire Goulaouic-Schwartz" (2003-2004), talk no. 18, 26. |
[33] |
J. Fröhlich, T.-P. Tsai, H.-T. Yau, On the point-particle (Newtonian) limit of the non-linear Hartree equation, Commun. Math. Phys., 225 (2002), 223–274. doi: 10.1007/s002200100579
![]() |
[34] | M. Gallo, Multiplicity and concentration results for local and fractional NLS equations with critical growth, Adv. Differential Equ., 26 (2021), 397–424. |
[35] |
J. Giacomoni, D. Goel, K. Sreenadh, Regularity results on a class of doubly nonlocal problems, J. Differ. Equations, 268 (2020), 5301–5328. doi: 10.1016/j.jde.2019.11.009
![]() |
[36] |
Q. Guo, S. Zhu, Sharp threshold of blow-up and scattering for the fractional Hartree equation, J. Differ. Equations, 264 (2018), 2802–2832. doi: 10.1016/j.jde.2017.11.001
![]() |
[37] |
C. Hainzl, E. Lenzmann, M. Lewin, B. Schlein, On blowup for time-dependent generalized Hartree–Fock equations, Ann. Henri Poincaré, 11 (2010), 1023–1052. doi: 10.1007/s00023-010-0054-3
![]() |
[38] |
H. Hajaiej, P. A. Markowich, S. Trabelsi, Multiconfiguration Hartree-Fock theory for pseudorelativistic systems: the time-dependent case, Math. Mod. Meth. Appl. Sci., 24 (2014), 599–626. doi: 10.1142/S0218202513500619
![]() |
[39] |
S. Herr, E. Lenzmann, The Boson star equation with initial data of low regularity, Nonlinear Anal. Theor., 97 (2014), 125–137. doi: 10.1016/j.na.2013.11.023
![]() |
[40] |
J. Hirata, K. Tanaka, Nonlinear scalar field equations with L2 constraint: mountain pass and symmetric mountain pass approaches, Adv. Nonlinear Stud., 19 (2019), 263–290. doi: 10.1515/ans-2018-2039
![]() |
[41] |
N. Ikoma, Existence of solutions of scalar field equations with fractional operator, J. Fixed Point Theory Appl., 19 (2017), 649–690. doi: 10.1007/s11784-016-0369-x
![]() |
[42] |
N. Ikoma, Erratum to: Existence of solutions of scalar field equations with fractional operator, J. Fixed Point Theory Appl., 19 (2017), 1649–1652. doi: 10.1007/s11784-017-0427-z
![]() |
[43] | N. Ikoma, K. Tanaka, A note on deformation argument for L2 constraint problems, Adv. Differential Equ., 24 (2019), 609–646. |
[44] | L. Jeanjean, K. Tanaka, A remark on least energy solutions in RN, Proc. Amer. Math. Soc., 131 (2003), 2399–2408. |
[45] |
K. Kirkpatrick, E. Lenzmann, G. Staffilani, On the continuum limit for discrete NLS with long-range lattice interactions, Commun. Math. Phys., 317 (2013), 563–591. doi: 10.1007/s00220-012-1621-x
![]() |
[46] |
C. Klein, C. Sparber, P. Markowich, Numerical study of fractional nonlinear Schrödinger equations, Proc. Royal Soc. A, 470 (2014), 20140364. doi: 10.1098/rspa.2014.0364
![]() |
[47] | N. Laskin, Fractional quantum mechanics and Lévy path integrals, Phys. Rev. A, 268 (2000), 56–108. |
[48] | E. Lenzmann, Well-posedness for semi-relativistic Hartree equations of critical type, Math. Phys. Anal. Geom., 2 (2007), 43–64. |
[49] |
E. Lenzmann, Uniqueness of ground states for pseudorelativistic Hartree equations, Anal. PDE, 2 (2009), 1–27. doi: 10.2140/apde.2009.2.1
![]() |
[50] |
E. Lenzmann, M. Lewin, On singularity formation for the L2-critical Boson star equation, Nonlinearity, 24 (2011), 3515–3540. doi: 10.1088/0951-7715/24/6/008
![]() |
[51] |
E. H. Lieb, Existence and uniqueness of the minimizing solution of Choquard's nonlinear equation, Stud. Appl. Math., 57 (1977), 93–105. doi: 10.1002/sapm197757293
![]() |
[52] | E. H. Lieb, M. Loss, Analysis, USA: American Mathematical Society, 2001. |
[53] |
E. H. Lieb, H.-T. Yau, The Chandrasekhar theory of stellar collapse as the limit of quantum mechanics, Commun. Math. Phys., 112 (1987), 147–174. doi: 10.1007/BF01217684
![]() |
[54] |
P.-L. Lions, Symétrie et compacité dans les espaces de Sobolev, J. Funct. Anal., 49 (1982), 315–334. doi: 10.1016/0022-1236(82)90072-6
![]() |
[55] |
S. Longhi, Fractional Schrödinger equation in optics, Optics Lett., 40 (2015), 1117–1120. doi: 10.1364/OL.40.001117
![]() |
[56] |
J. Lu, V. Moroz, C. B. Muratov, Orbital-free density functional theory of out-of-plane charge screening in graphene, J. Nonlinear. Sci., 25 (2015), 1391–1430. doi: 10.1007/s00332-015-9259-4
![]() |
[57] |
H. Luo, Ground state solutions of Pohozaev type for fractional Choquard equations with general nonlinearities, Comput. Math. Appl., 77 (2019), 877–887. doi: 10.1016/j.camwa.2018.10.024
![]() |
[58] |
L. Ma, L. Zhao, Classification of positive solitary solutions of the nonlinear Choquard equation, Arch. Rational Mech. Anal., 195 (2010), 455–467. doi: 10.1007/s00205-008-0208-3
![]() |
[59] |
I. M. Moroz, R. Penrose, P. Tod, Spherically-symmetric solutions of the Schrödinger-Newton equations, Class. Quantum Grav., 15 (1998), 2733–2742. doi: 10.1088/0264-9381/15/9/019
![]() |
[60] |
V. Moroz, J. Van Schaftingen, Groundstates of nonlinear Choquard equations: existence, qualitative properties and decay asymptotics, J. Funct. Anal., 265 (2013), 153–184. doi: 10.1016/j.jfa.2013.04.007
![]() |
[61] | V. Moroz, J. Van Schaftingen, Existence of groundstates for a class of nonlinear Choquard equations, Trans. Amer. Math. Soc., 367 (2015), 6557–6579. |
[62] |
V. Moroz, J. Van Schaftingen, A guide to the Choquard equation, J. Fixed Point Theory Appl., 19 (2017), 773–813. doi: 10.1007/s11784-016-0373-1
![]() |
[63] | S. Pekar, Untersuchung über die Elektronentheorie der Kristalle, Berlin: Akademie Verlag, 1954. |
[64] |
R. Penrose, On gravity's role in quantum state reduction, Gen. Rel. Grav., 28 (1996), 581–600. doi: 10.1007/BF02105068
![]() |
[65] |
R. Penrose, Quantum computation, entanglement and state reduction, Phil. Trans. R. Soc. A, 356 (1998), 1927–1939. doi: 10.1098/rsta.1998.0256
![]() |
[66] | R. Penrose, The road to reality. A complete guide to the laws of the universe, New York: Alfred A. Knopf Inc., 2005. |
[67] |
Z. Shen, F. Gao, M. Yin, Ground state for nonlinear fractional Choquard equations with general nonlinearities, Math. Method. Appl. Sci., 39 (2016), 4082–4098. doi: 10.1002/mma.3849
![]() |
[68] | L. Silvestre, Regularity of the obstacle problem for a fractional power of the Laplace operator, Commun. Pure Appl. Math., 60 (2006), 67–112. |
[69] |
C. Stuart, Existence theory for the {H}artree equation, Arch. Rational Mech. Anal., 51 (1973), 60–69. doi: 10.1007/BF00275993
![]() |
[70] |
P. Tod, The ground state energy of the Schrödinger-Newton equation, Phys. Lett. A, 280 (2001), 173–176. doi: 10.1016/S0375-9601(01)00059-7
![]() |
[71] |
P. Tod, I. M. Moroz, An analytical approach to the Schrödinger-Newton equations, Nonlinearity, 12 (1999), 201–216. doi: 10.1088/0951-7715/12/2/002
![]() |
[72] | Z. Yang, F. Zhao, Multiplicity and concentration behaviour of solutions for a fractional Choquard equation with critical growth, Adv. Nonlinear Anal., 10 (2021), 732–774. |
1. | Zakiah I. Kalantan, Eman M. Swielum, Neama T. AL-Sayed, Abeer A. EL-Helbawy, Gannat R. AL-Dayian, Mervat Abd Elaal, Bayesian and E-Bayesian Estimation for a Modified Topp Leone–Chen Distribution Based on a Progressive Type-II Censoring Scheme, 2024, 16, 2073-8994, 981, 10.3390/sym16080981 | |
2. | Ahmed R. El-Saeed, Badr Aloraini, Safar M. Alghamdi, Statistical modeling of disability: Using a new extended Topp Leone inverse exponential model, 2025, 18, 16878507, 101447, 10.1016/j.jrras.2025.101447 |
θ | μ′1 | Var | CV | S | K | |
α=0.25 | 0.5 | 0.2460 | 0.2550 | 2.0523 | 4.2055 | 25.407 |
1 | 0.1230 | 0.0637 | 2.0523 | 4.2055 | 25.407 | |
1.5 | 0.0820 | 0.0283 | 2.0523 | 4.2055 | 25.407 | |
2 | 0.0615 | 0.0159 | 2.0523 | 4.2055 | 25.407 | |
α=0.5 | 0.5 | 0.6196 | 0.6258 | 1.2768 | 2.5999 | 9.7552 |
1 | 0.3098 | 0.1564 | 1.2768 | 2.5999 | 9.7552 | |
1.5 | 0.2065 | 0.0695 | 1.2768 | 2.5999 | 9.7552 | |
2 | 0.1549 | 0.0391 | 1.2768 | 2.5999 | 9.7552 | |
α=0.75 | 0.5 | 0.9702 | 0.9287 | 0.9932 | 2.0249 | 5.9692 |
1 | 0.4851 | 0.2321 | 0.9932 | 2.0249 | 5.9692 | |
1.5 | 0.3234 | 0.1031 | 0.9932 | 2.0249 | 5.9692 | |
2 | 0.2425 | 0.0580 | 0.9932 | 2.0249 | 5.9692 | |
α=1 | 0.5 | 1.2813 | 1.1606 | 0.8407 | 1.7251 | 4.3798 |
1 | 0.6406 | 0.2901 | 0.8407 | 1.7251 | 4.3798 | |
1.5 | 0.4271 | 0.1289 | 0.8407 | 1.7251 | 4.3798 | |
2 | 0.3203 | 0.0725 | 0.8407 | 1.7251 | 4.3798 |
θ | μ′1 | Var | CV | S | K | |
α=0.25 | 0.5 | 0.4325 | 0.4196 | 1.4976 | 3.1710 | 14.660 |
1 | 0.2162 | 0.1049 | 1.4976 | 3.1710 | 14.660 | |
1.5 | 0.1441 | 0.0466 | 1.4976 | 3.1710 | 14.66 | |
2 | 0.1081 | 0.0262 | 1.4976 | 3.1710 | 14.660 | |
α=0.5 | 0.5 | 0.9848 | 0.8709 | 0.9476 | 2.0392 | 6.1814 |
1 | 0.4924 | 0.2177 | 0.9476 | 2.0392 | 6.1814 | |
1.5 | 0.3282 | 0.0967 | 0.9476 | 2.0392 | 6.1814 | |
2 | 0.2462 | 0.0544 | 0.9476 | 2.0392 | 6.1814 | |
α=0.75 | 0.5 | 1.4485 | 1.1686 | 0.7462 | 1.6458 | 4.1135 |
1 | 0.7242 | 0.2921 | 0.7462 | 1.6458 | 4.1135 | |
1.5 | 0.4828 | 0.1298 | 0.7462 | 1.6458 | 4.1135 | |
2 | 0.3621 | 0.0730 | 0.7462 | 1.6458 | 4.1135 | |
α=1 | 0.5 | 1.8340 | 1.3685 | 0.6378 | 1.4452 | 3.2372 |
1 | 0.9170 | 0.3421 | 0.6378 | 1.4452 | 3.2372 | |
1.5 | 0.6113 | 0.1520 | 0.6378 | 1.4452 | 3.2372 | |
2 | 0.4585 | 0.0855 | 0.6378 | 1.4452 | 3.2372 |
Simple size | Est. | ˆθ | ˆα | ˆλ |
100 | AE | 0.6932 | 1.3583 | 1.0299 |
AB | 0.0932 | 0.5583 | 0.5299 | |
MSE | 0.0664 | 1.4037 | 1.3624 | |
MRE | 0.1553 | 0.6979 | 1.0598 | |
250 | AE | 0.6341 | 1.0291 | 0.6319 |
AB | 0.0341 | 0.2291 | 0.1319 | |
MSE | 0.0225 | 0.4660 | 0.3173 | |
MRE | 0.0569 | 0.2864 | 0.2638 | |
500 | AE | 0.6255 | 0.9456 | 0.5500 |
AB | 0.0255 | 0.1456 | 0.0500 | |
MSE | 0.0130 | 0.2324 | 0.0889 | |
MRE | 0.0426 | 0.1820 | 0.1001 | |
750 | AE | 0.6075 | 0.8852 | 0.5434 |
AB | 0.0075 | 0.0852 | 0.0434 | |
MSE | 0.0054 | 0.1477 | 0.0581 | |
MRE | 0.0125 | 0.1065 | 0.0869 | |
1000 | AE | 0.6047 | 0.8728 | 0.5401 |
AB | 0.0047 | 0.0728 | 0.0401 | |
MSE | 0.0043 | 0.1318 | 0.0519 | |
MRE | 0.0078 | 0.0911 | 0.0803 |
Simple size | Est. | ˆθ | ˆα | ˆλ |
100 | AE | 0.3099 | 1.0043 | 0.8253 |
AB | 0.0599 | 0.5043 | 0.1253 | |
MSE | 0.0143 | 0.8638 | 1.5698 | |
MRE | 0.2396 | 1.0086 | 0.1790 | |
250 | AE | 0.2668 | 0.6964 | 0.8021 |
AB | 0.0168 | 0.1964 | 0.1021 | |
MSE | 0.0052 | 0.2768 | 0.4464 | |
MRE | 0.0674 | 0.3928 | 0.1636 | |
500 | AE | 0.2485 | 0.5278 | 0.8099 |
AB | 0.0014 | 0.0278 | 0.1099 | |
MSE | 0.0019 | 0.0493 | 0.1588 | |
MRE | 0.0056 | 0.0557 | 0.1570 | |
750 | AE | 0.2552 | 0.5480 | 0.7556 |
AB | 0.0052 | 0.04806 | 0.0556 | |
MSE | 0.0016 | 0.0596 | 0.1034 | |
MRE | 0.0208 | 0.0961 | 0.0795 | |
1000 | AE | 0.2553 | 0.5312 | 0.7357 |
AB | 0.0053 | 0.0312 | 0.03574 | |
MSE | 0.0011 | 0.0268 | 0.0693 | |
MRE | 0.0210 | 0.0624 | 0.0510 |
Simple size | Est. | ˆθ | ˆα | ˆλ |
100 | AE | 0.8480 | 2.1978 | 1.9580 |
AB | 0.0980 | 1.1978 | 1.0580 | |
MSE | 0.0665 | 1.2910 | 1.7854 | |
MRE | 0.1307 | 1.1978 | 1.1756 | |
250 | AE | 0.8246 | 1.5339 | 1.3104 |
AB | 0.0346 | 0.5339 | 0.4104 | |
MSE | 0.0302 | 1.0916 | 1.6425 | |
MRE | 0.0861 | 0.5339 | 0.4560 | |
500 | AE | 0.7731 | 1.2048 | 1.1657 |
AB | 0.0231 | 0.2048 | 0.2657 | |
MSE | 0.0130 | 0.6911 | 1.1267 | |
MRE | 0.0275 | 0.2048 | 0.2953 | |
750 | AE | 0.7656 | 1.1924 | 0.9820 |
AB | 0.0156 | 0.1924 | 0.0820 | |
MSE | 0.0087 | 0.4560 | 0.3348 | |
MRE | 0.0208 | 0.1924 | 0.0911 | |
1000 | AE | 0.7653 | 1.1837 | 0.9436 |
AB | 0.0153 | 0.1837 | 0.0436 | |
MSE | 0.0082 | 0.4025 | 0.1537 | |
MRE | 0.0204 | 0.1837 | 0.0485 |
Simple size | Est. | ˆθ | ˆα | ˆλ |
100 | AE | 0.9913 | 0.4293 | 2.4947 |
AB | 0.1913 | 0.1793 | 1.2947 | |
MSE | 0.1833 | 0.2143 | 1.9780 | |
MRE | 0.2391 | 0.7172 | 1.0789 | |
250 | AE | 0.8291 | 0.3066 | 1.5424 |
AB | 0.0291 | 0.0566 | 0.3424 | |
MSE | 0.0882 | 0.06599 | 1.4114 | |
500 | AE | 0.8240 | 0.2643 | 1.3341 |
AB | 0.0240 | 0.0143 | 0.1341 | |
MSE | 0.0217 | 0.0087 | 0.3792 | |
MRE | 0.0300 | 0.0572 | 0.1118 | |
750 | AE | 0.8172 | 0.2658 | 1.2881 |
AB | 0.0172 | 0.0140 | 0.0881 | |
MSE | 0.0163 | 0.0070 | 0.2778 | |
MRE | 0.0208 | 0.0653 | 0.0734 | |
1000 | AE | 0.8163 | 0.2650 | 1.2237 |
AB | 0.0163 | 0.0150 | 0.02376 | |
MSE | 0.0137 | 0.0048 | 0.1518 | |
MRE | 0.0204 | 0.0602 | 0.0198 |
Model | Par | q | T1 | T2 | T4 |
TL-EE | θ=2.5 | 0.60 | 1.6234 | 1.8534 | 1.8810 |
α=4.25 | 0.65 | 1.6583 | 1.8839 | 1.9132 | |
λ=100 | 0.70 | 1.6969 | 1.9183 | 1.9493 | |
0.75 | 1.7408 | 1.9583 | 1.9910 | ||
0.80 | 1.7926 | 2.0064 | 2.0407 | ||
0.85 | 1.8570 | 2.0673 | 2.1032 | ||
0.90 | 1.9449 | 2.1519 | 2.1892 | ||
0.95 | 2.0903 | 2.2941 | 2.3329 | ||
EE | θ=2.5 | 1.3107 | 1.4156 | 1.2374 | 1.4161 |
α=4.25 | 0.65 | 0.9357 | 1.3689 | 1.4812 | |
0.70 | 1.0078 | 1.4353 | 1.5546 | ||
0.75 | 1.0905 | 1.5127 | 1.6391 | ||
0.80 | 1.1891 | 1.6063 | 1.7397 | ||
0.85 | 1.3131 | 1.7256 | 1.8658 | ||
0.90 | 1.4838 | 1.8919 | 2.0388 | ||
0.95 | 1.7692 | 2.1731 | 2.3266 | ||
Exp | θ=2.5 | 0.60 | 0.3665 | 0.7665 | 0.8625 |
0.65 | 0.4199 | 0.8199 | 0.9239 | ||
0.70 | 0.4815 | 0.8815 | 0.9935 | ||
0.75 | 0.5545 | 0.9545 | 1.0745 | ||
0.80 | 0.6437 | 1.0437 | 1.1717 | ||
0.85 | 0.7588 | 1.1588 | 1.2948 | ||
0.90 | 0.9210 | 1.3210 | 1.4650 | ||
0.95 | 1.1982 | 1.5982 | 1.7502 |
Model | Par | q | T1 | T2 | T4 |
TL-EE | θ=4.0 | 0.60 | 1.0991 | 1.2434 | 1.2543 |
α=6.5 | 0.65 | 1.1211 | 1.2625 | 1.2740 | |
λ=85 | 0.70 | 1.1453 | 1.2841 | 1.2963 | |
0.75 | 1.1729 | 1.3091 | 1.3220 | ||
0.80 | 1.2054 | 1.3393 | 1.3527 | ||
0.85 | 1.2458 | 1.3775 | 1.3915 | ||
0.90 | 1.3008 | 1.4304 | 1.4450 | ||
0.95 | 1.3919 | 1.5194 | 1.5346 | ||
EE | θ=4.0 | 0.60 | 0.6456 | 0.9230 | 0.9644 |
α=6.5 | 0.65 | 0.6867 | 0.9598 | 1.0040 | |
0.70 | 0.7325 | 1.00159 | 1.0485 | ||
0.75 | 0.7849 | 1.0502 | 1.0999 | ||
0.80 | 0.8472 | 1.1091 | 1.1614 | ||
0.85 | 0.9253 | 1.1839 | 1.2388 | ||
0.90 | 1.0325 | 1.2881 | 1.3456 | ||
0.95 | 1.2114 | 1.4642 | 1.5242 | ||
Exp | θ=4.0 | 0.60 | 0.2290 | 0.4790 | 0.5165 |
0.65 | 0.2624 | 0.5124 | 0.5530 | ||
0.70 | 0.3009 | 0.5509 | 0.5947 | ||
0.75 | 0.3465 | 0.5965 | 0.6434 | ||
0.80 | 0.4023 | 0.6523 | 0.7023 | ||
0.85 | 0.4742 | 0.7242 | 0.7774 | ||
0.90 | 0.5756 | 0.8256 | 0.8818 | ||
0.95 | 0.7489 | 0.9989 | 1.0583 |
Mean | Q2 | Sd | Q1 | Q3 | Skew | Kurt |
520.0 | 573.5 | 134.7 | 676.0 | 697.1 | 772.5 | 982.0 |
Distribution | Par | ll |
TL-EE | ˆθ=0.0048 ˆα=2.8201 ˆλ=67.987 | -344.054 |
EE | ˆθ=0.0064 ˆα=55.743 | -349.589 |
PL | ˆα = 470.909 ˆλ=590.227 ˆθ=8.4518 | -348.5498 |
PE | ˆλ=0.0065 ˆθ=59.178 | -349.538 |
Wei | ˆμ=5.5134 ˆσ= 753.625 | -349.229 |
Exp | ˆλ=0.0014 | -415.080 |
Lin | ˆλ=0.0028 | -394.886 |
ZTPGA | ˆα=5.0374 ˆλ=0.0146 ˆθ=21.882 | -344.680 |
PLin | ˆα=0.0786 ˆβ= 2.1419 | -380.426 |
EG | ˆα=0.0043 ˆp= 0.0858 | -380.322 |
TPM | ˆα=0.0043 ˆδ= 0.8077 | -383.885 |
Model | A1 | A2 | A3 | B1 | KS | P-value |
TL-EE | 694.109 | 694.580 | 696.438 | 700.131 | 0.1018 | 0.618 |
EE | 703.178 | 704.409 | 705.731 | 709.193 | 0.1320 | 0.292 |
PL | 703.099 | 703.570 | 705.428 | 709.121 | 0.1190 | 0.417 |
PE | 703.077 | 703.308 | 704.630 | 707.092 | 0.1357 | 0.262 |
Wei | 702.458 | 702.689 | 704.011 | 706.473 | 0.1212 | 0.393 |
Exp | 832.160 | 832.236 | 832.937 | 834.168 | 0.5257 | 1.2×10−13 |
Lin | 791.772 | 791.847 | 792.548 | 793.779 | 0.4396 | 1.1×10−9 |
ZTPGA | 695.360 | 695.831 | 697.689 | 701.382 | 0.1046 | 0.5839 |
PLin | 764.852 | 765.082 | 766.404 | 768.866 | 0.3852 | 2.5×10−06 |
EG | 764.645 | 764.876 | 766.198 | 768.660 | 0.38.26 | 7.5×10−06 |
TPM | 771.770 | 772.001 | 773.323 | 775.785 | 0.3873 | 1.36×10−07 |
Model | Par | q | T1 | T2 | T4 |
Empirical | 0.85 | 875.8 | 925.7 | 982.0 | |
0.90 | 917.8 | 939.6 | 1285.2 | ||
0.95 | 933.6 | 955.6 | 1335.8 | ||
0.99 | 1041.1 | 1194.8 | 1571.9 | ||
TL-EE | ˆθ=0.0048 | 0.85 | 825.0 | 932.4 | 10280.6 |
ˆα=2.8201 | 0.90 | 869.9 | 975.6 | 10714.9 | |
ˆλ=67.987 | 0.95 | 944.2 | 1048.2 | 11156.3 | |
0.99 | 1111.6 | 1214.3 | 11601.9 | ||
EE | ˆα=55.743 | 0.85 | 910.8 | 1073.0 | 22573.1 |
ˆθ=0.0064 | 0.90 | 978.3 | 138.4 | 23600.3 | |
0.95 | 1090.5 | 1248.5 | 24654.0 | ||
0.99 | 1344.7 | 1501.1 | 25649.0 | ||
Exp | ˆλ= 0.0014 | 0.85 | 1322.4 | 2019.5 | 415065.0 |
0.90 | 1605.1 | 2302.2 | 439644.4 | ||
0.95 | 2088.2 | 2785.3 | 464424.4 | ||
0.99 | 33210.2 | 3907.3 | 484983.8 |
Mean | Q2 | Sd | Q1 | Q3 | Skew | Kurt |
487.2 | 427.8 | 248.0 | 301.1 | 585.4 | 1.932 | 4.471 |
Distribution | Par | ll |
TL-EE | ˆθ=0.0029 ˆα=0.4591 ˆλ=38.326 | -331.867 |
EE | ˆθ=0.0049 ˆα=5.7608 | -334.355 |
PL | ˆα = 133.818 ˆλ=190.851 ˆθ=3.9591 | -332.767 |
PE | ˆλ=0.0068 ˆθ=14.346 | -333.323 |
Wei | ˆμ=2.1057 ˆσ= 552.523 | -340.802 |
Exp | ˆλ=0.0020 | -359.435 |
Lin | ˆλ=0.0040 | -345.044 |
ZTPGA | ˆα=1.8306 ˆλ=0.0077 ˆθ=6.1417 | -335.129 |
PLin | ˆα=1.5245 ˆβ= 0.0001 | -337.759 |
EG | ˆα=0.0076 ˆp= 0.0338 | -339.999 |
TPM | ˆα=0.0062 ˆδ= 0.3874 | -338.991 |
Model | A1 | A2 | A3 | B1 | KS | P-value |
TL-EE | 669.734 | 670.256 | 671.919 | 675.470 | 0.1089 | 0.5567 |
EE | 672.711 | 672.967 | 674.167 | 676.535 | 0.1470 | 0.2082 |
PL | 671.534 | 672.055 | 673.718 | 677.270 | 0.1124 | 0.5162 |
PE | 670.646 | 670.901 | 672.102 | 674.470 | 0.1181 | 0.4535 |
Wei | 685.604 | 685.859 | 687.060 | 689.428 | 0.1803 | 0.0678 |
Exp | 720.871 | 720.954 | 721.599 | 722.783 | 0.4094 | 4.2×10−08 |
Lin | 692.089 | 692.172 | 692.817 | 694.001 | 0.2846 | 0.0004 |
ZTPGA | 676.258 | 676.780 | 678.442 | 681.994 | 0.1104 | 0.5383 |
PLin | 679.518 | 679.773 | 680.974 | 683.342 | 0.1520 | 0.1784 |
EG | 683.998 | 684.253 | 685.454 | 687.822 | 0.1707 | 0.0961 |
TPM | 681.982 | 682.237 | 683.438 | 685.806 | 0.2147 | 0.0167 |
Model | Par | q | T1 | T2 | T4 |
Empirical | 0.85 | 664.3 | 925.8 | 11899.1 | |
0.90 | 726.9 | 1071.7 | 7070.6 | ||
0.95 | 910.2 | 1207.3 | 3901.6 | ||
0.99 | 1347.8 | 1500.3 | 1500.3 | ||
TL-EE | ˆθ=0.0029 | 0.85 | 687.2 | 942.0 | 26230.5 |
ˆα=0.4591 | 0.90 | 759.2 | 1027.0 | 27617.5 | |
ˆλ=38.326 | 0.95 | 879.9 | 1170.6 | 29096.2 | |
0.99 | 1156.6 | 1500.8 | 30579.1 | ||
EE | ˆα=0.0049 | 0.85 | 731.0 | 861.8 | 37517.5 |
ˆθ=5.7608 | 0.90 | 818.4 | 932.3 | 39320.7 | |
0.95 | 964.4 | 1051.9 | 41155.2 | ||
0.99 | 1296.3 | 1328.3 | 42819.2 | ||
Exp | ˆλ= 0.0020 | 0.85 | 948.5 | 1448.5 | 213948.6 |
0.90 | 1151.2 | 1651.2 | 226651.3 | ||
0.95 | 1497.8 | 1997.8 | 239499.0 | ||
0.99 | 2302.5 | 2802.5 | 250302.6 |
θ | μ′1 | Var | CV | S | K | |
α=0.25 | 0.5 | 0.2460 | 0.2550 | 2.0523 | 4.2055 | 25.407 |
1 | 0.1230 | 0.0637 | 2.0523 | 4.2055 | 25.407 | |
1.5 | 0.0820 | 0.0283 | 2.0523 | 4.2055 | 25.407 | |
2 | 0.0615 | 0.0159 | 2.0523 | 4.2055 | 25.407 | |
α=0.5 | 0.5 | 0.6196 | 0.6258 | 1.2768 | 2.5999 | 9.7552 |
1 | 0.3098 | 0.1564 | 1.2768 | 2.5999 | 9.7552 | |
1.5 | 0.2065 | 0.0695 | 1.2768 | 2.5999 | 9.7552 | |
2 | 0.1549 | 0.0391 | 1.2768 | 2.5999 | 9.7552 | |
α=0.75 | 0.5 | 0.9702 | 0.9287 | 0.9932 | 2.0249 | 5.9692 |
1 | 0.4851 | 0.2321 | 0.9932 | 2.0249 | 5.9692 | |
1.5 | 0.3234 | 0.1031 | 0.9932 | 2.0249 | 5.9692 | |
2 | 0.2425 | 0.0580 | 0.9932 | 2.0249 | 5.9692 | |
α=1 | 0.5 | 1.2813 | 1.1606 | 0.8407 | 1.7251 | 4.3798 |
1 | 0.6406 | 0.2901 | 0.8407 | 1.7251 | 4.3798 | |
1.5 | 0.4271 | 0.1289 | 0.8407 | 1.7251 | 4.3798 | |
2 | 0.3203 | 0.0725 | 0.8407 | 1.7251 | 4.3798 |
θ | μ′1 | Var | CV | S | K | |
α=0.25 | 0.5 | 0.4325 | 0.4196 | 1.4976 | 3.1710 | 14.660 |
1 | 0.2162 | 0.1049 | 1.4976 | 3.1710 | 14.660 | |
1.5 | 0.1441 | 0.0466 | 1.4976 | 3.1710 | 14.66 | |
2 | 0.1081 | 0.0262 | 1.4976 | 3.1710 | 14.660 | |
α=0.5 | 0.5 | 0.9848 | 0.8709 | 0.9476 | 2.0392 | 6.1814 |
1 | 0.4924 | 0.2177 | 0.9476 | 2.0392 | 6.1814 | |
1.5 | 0.3282 | 0.0967 | 0.9476 | 2.0392 | 6.1814 | |
2 | 0.2462 | 0.0544 | 0.9476 | 2.0392 | 6.1814 | |
α=0.75 | 0.5 | 1.4485 | 1.1686 | 0.7462 | 1.6458 | 4.1135 |
1 | 0.7242 | 0.2921 | 0.7462 | 1.6458 | 4.1135 | |
1.5 | 0.4828 | 0.1298 | 0.7462 | 1.6458 | 4.1135 | |
2 | 0.3621 | 0.0730 | 0.7462 | 1.6458 | 4.1135 | |
α=1 | 0.5 | 1.8340 | 1.3685 | 0.6378 | 1.4452 | 3.2372 |
1 | 0.9170 | 0.3421 | 0.6378 | 1.4452 | 3.2372 | |
1.5 | 0.6113 | 0.1520 | 0.6378 | 1.4452 | 3.2372 | |
2 | 0.4585 | 0.0855 | 0.6378 | 1.4452 | 3.2372 |
Simple size | Est. | ˆθ | ˆα | ˆλ |
100 | AE | 0.6932 | 1.3583 | 1.0299 |
AB | 0.0932 | 0.5583 | 0.5299 | |
MSE | 0.0664 | 1.4037 | 1.3624 | |
MRE | 0.1553 | 0.6979 | 1.0598 | |
250 | AE | 0.6341 | 1.0291 | 0.6319 |
AB | 0.0341 | 0.2291 | 0.1319 | |
MSE | 0.0225 | 0.4660 | 0.3173 | |
MRE | 0.0569 | 0.2864 | 0.2638 | |
500 | AE | 0.6255 | 0.9456 | 0.5500 |
AB | 0.0255 | 0.1456 | 0.0500 | |
MSE | 0.0130 | 0.2324 | 0.0889 | |
MRE | 0.0426 | 0.1820 | 0.1001 | |
750 | AE | 0.6075 | 0.8852 | 0.5434 |
AB | 0.0075 | 0.0852 | 0.0434 | |
MSE | 0.0054 | 0.1477 | 0.0581 | |
MRE | 0.0125 | 0.1065 | 0.0869 | |
1000 | AE | 0.6047 | 0.8728 | 0.5401 |
AB | 0.0047 | 0.0728 | 0.0401 | |
MSE | 0.0043 | 0.1318 | 0.0519 | |
MRE | 0.0078 | 0.0911 | 0.0803 |
Simple size | Est. | ˆθ | ˆα | ˆλ |
100 | AE | 0.3099 | 1.0043 | 0.8253 |
AB | 0.0599 | 0.5043 | 0.1253 | |
MSE | 0.0143 | 0.8638 | 1.5698 | |
MRE | 0.2396 | 1.0086 | 0.1790 | |
250 | AE | 0.2668 | 0.6964 | 0.8021 |
AB | 0.0168 | 0.1964 | 0.1021 | |
MSE | 0.0052 | 0.2768 | 0.4464 | |
MRE | 0.0674 | 0.3928 | 0.1636 | |
500 | AE | 0.2485 | 0.5278 | 0.8099 |
AB | 0.0014 | 0.0278 | 0.1099 | |
MSE | 0.0019 | 0.0493 | 0.1588 | |
MRE | 0.0056 | 0.0557 | 0.1570 | |
750 | AE | 0.2552 | 0.5480 | 0.7556 |
AB | 0.0052 | 0.04806 | 0.0556 | |
MSE | 0.0016 | 0.0596 | 0.1034 | |
MRE | 0.0208 | 0.0961 | 0.0795 | |
1000 | AE | 0.2553 | 0.5312 | 0.7357 |
AB | 0.0053 | 0.0312 | 0.03574 | |
MSE | 0.0011 | 0.0268 | 0.0693 | |
MRE | 0.0210 | 0.0624 | 0.0510 |
Simple size | Est. | ˆθ | ˆα | ˆλ |
100 | AE | 0.8480 | 2.1978 | 1.9580 |
AB | 0.0980 | 1.1978 | 1.0580 | |
MSE | 0.0665 | 1.2910 | 1.7854 | |
MRE | 0.1307 | 1.1978 | 1.1756 | |
250 | AE | 0.8246 | 1.5339 | 1.3104 |
AB | 0.0346 | 0.5339 | 0.4104 | |
MSE | 0.0302 | 1.0916 | 1.6425 | |
MRE | 0.0861 | 0.5339 | 0.4560 | |
500 | AE | 0.7731 | 1.2048 | 1.1657 |
AB | 0.0231 | 0.2048 | 0.2657 | |
MSE | 0.0130 | 0.6911 | 1.1267 | |
MRE | 0.0275 | 0.2048 | 0.2953 | |
750 | AE | 0.7656 | 1.1924 | 0.9820 |
AB | 0.0156 | 0.1924 | 0.0820 | |
MSE | 0.0087 | 0.4560 | 0.3348 | |
MRE | 0.0208 | 0.1924 | 0.0911 | |
1000 | AE | 0.7653 | 1.1837 | 0.9436 |
AB | 0.0153 | 0.1837 | 0.0436 | |
MSE | 0.0082 | 0.4025 | 0.1537 | |
MRE | 0.0204 | 0.1837 | 0.0485 |
Simple size | Est. | ˆθ | ˆα | ˆλ |
100 | AE | 0.9913 | 0.4293 | 2.4947 |
AB | 0.1913 | 0.1793 | 1.2947 | |
MSE | 0.1833 | 0.2143 | 1.9780 | |
MRE | 0.2391 | 0.7172 | 1.0789 | |
250 | AE | 0.8291 | 0.3066 | 1.5424 |
AB | 0.0291 | 0.0566 | 0.3424 | |
MSE | 0.0882 | 0.06599 | 1.4114 | |
500 | AE | 0.8240 | 0.2643 | 1.3341 |
AB | 0.0240 | 0.0143 | 0.1341 | |
MSE | 0.0217 | 0.0087 | 0.3792 | |
MRE | 0.0300 | 0.0572 | 0.1118 | |
750 | AE | 0.8172 | 0.2658 | 1.2881 |
AB | 0.0172 | 0.0140 | 0.0881 | |
MSE | 0.0163 | 0.0070 | 0.2778 | |
MRE | 0.0208 | 0.0653 | 0.0734 | |
1000 | AE | 0.8163 | 0.2650 | 1.2237 |
AB | 0.0163 | 0.0150 | 0.02376 | |
MSE | 0.0137 | 0.0048 | 0.1518 | |
MRE | 0.0204 | 0.0602 | 0.0198 |
Model | Par | q | T1 | T2 | T4 |
TL-EE | θ=2.5 | 0.60 | 1.6234 | 1.8534 | 1.8810 |
α=4.25 | 0.65 | 1.6583 | 1.8839 | 1.9132 | |
λ=100 | 0.70 | 1.6969 | 1.9183 | 1.9493 | |
0.75 | 1.7408 | 1.9583 | 1.9910 | ||
0.80 | 1.7926 | 2.0064 | 2.0407 | ||
0.85 | 1.8570 | 2.0673 | 2.1032 | ||
0.90 | 1.9449 | 2.1519 | 2.1892 | ||
0.95 | 2.0903 | 2.2941 | 2.3329 | ||
EE | θ=2.5 | 1.3107 | 1.4156 | 1.2374 | 1.4161 |
α=4.25 | 0.65 | 0.9357 | 1.3689 | 1.4812 | |
0.70 | 1.0078 | 1.4353 | 1.5546 | ||
0.75 | 1.0905 | 1.5127 | 1.6391 | ||
0.80 | 1.1891 | 1.6063 | 1.7397 | ||
0.85 | 1.3131 | 1.7256 | 1.8658 | ||
0.90 | 1.4838 | 1.8919 | 2.0388 | ||
0.95 | 1.7692 | 2.1731 | 2.3266 | ||
Exp | θ=2.5 | 0.60 | 0.3665 | 0.7665 | 0.8625 |
0.65 | 0.4199 | 0.8199 | 0.9239 | ||
0.70 | 0.4815 | 0.8815 | 0.9935 | ||
0.75 | 0.5545 | 0.9545 | 1.0745 | ||
0.80 | 0.6437 | 1.0437 | 1.1717 | ||
0.85 | 0.7588 | 1.1588 | 1.2948 | ||
0.90 | 0.9210 | 1.3210 | 1.4650 | ||
0.95 | 1.1982 | 1.5982 | 1.7502 |
Model | Par | q | T1 | T2 | T4 |
TL-EE | θ=4.0 | 0.60 | 1.0991 | 1.2434 | 1.2543 |
α=6.5 | 0.65 | 1.1211 | 1.2625 | 1.2740 | |
λ=85 | 0.70 | 1.1453 | 1.2841 | 1.2963 | |
0.75 | 1.1729 | 1.3091 | 1.3220 | ||
0.80 | 1.2054 | 1.3393 | 1.3527 | ||
0.85 | 1.2458 | 1.3775 | 1.3915 | ||
0.90 | 1.3008 | 1.4304 | 1.4450 | ||
0.95 | 1.3919 | 1.5194 | 1.5346 | ||
EE | θ=4.0 | 0.60 | 0.6456 | 0.9230 | 0.9644 |
α=6.5 | 0.65 | 0.6867 | 0.9598 | 1.0040 | |
0.70 | 0.7325 | 1.00159 | 1.0485 | ||
0.75 | 0.7849 | 1.0502 | 1.0999 | ||
0.80 | 0.8472 | 1.1091 | 1.1614 | ||
0.85 | 0.9253 | 1.1839 | 1.2388 | ||
0.90 | 1.0325 | 1.2881 | 1.3456 | ||
0.95 | 1.2114 | 1.4642 | 1.5242 | ||
Exp | θ=4.0 | 0.60 | 0.2290 | 0.4790 | 0.5165 |
0.65 | 0.2624 | 0.5124 | 0.5530 | ||
0.70 | 0.3009 | 0.5509 | 0.5947 | ||
0.75 | 0.3465 | 0.5965 | 0.6434 | ||
0.80 | 0.4023 | 0.6523 | 0.7023 | ||
0.85 | 0.4742 | 0.7242 | 0.7774 | ||
0.90 | 0.5756 | 0.8256 | 0.8818 | ||
0.95 | 0.7489 | 0.9989 | 1.0583 |
Mean | Q2 | Sd | Q1 | Q3 | Skew | Kurt |
520.0 | 573.5 | 134.7 | 676.0 | 697.1 | 772.5 | 982.0 |
Distribution | Par | ll |
TL-EE | ˆθ=0.0048 ˆα=2.8201 ˆλ=67.987 | -344.054 |
EE | ˆθ=0.0064 ˆα=55.743 | -349.589 |
PL | ˆα = 470.909 ˆλ=590.227 ˆθ=8.4518 | -348.5498 |
PE | ˆλ=0.0065 ˆθ=59.178 | -349.538 |
Wei | ˆμ=5.5134 ˆσ= 753.625 | -349.229 |
Exp | ˆλ=0.0014 | -415.080 |
Lin | ˆλ=0.0028 | -394.886 |
ZTPGA | ˆα=5.0374 ˆλ=0.0146 ˆθ=21.882 | -344.680 |
PLin | ˆα=0.0786 ˆβ= 2.1419 | -380.426 |
EG | ˆα=0.0043 ˆp= 0.0858 | -380.322 |
TPM | ˆα=0.0043 ˆδ= 0.8077 | -383.885 |
Model | A1 | A2 | A3 | B1 | KS | P-value |
TL-EE | 694.109 | 694.580 | 696.438 | 700.131 | 0.1018 | 0.618 |
EE | 703.178 | 704.409 | 705.731 | 709.193 | 0.1320 | 0.292 |
PL | 703.099 | 703.570 | 705.428 | 709.121 | 0.1190 | 0.417 |
PE | 703.077 | 703.308 | 704.630 | 707.092 | 0.1357 | 0.262 |
Wei | 702.458 | 702.689 | 704.011 | 706.473 | 0.1212 | 0.393 |
Exp | 832.160 | 832.236 | 832.937 | 834.168 | 0.5257 | 1.2×10−13 |
Lin | 791.772 | 791.847 | 792.548 | 793.779 | 0.4396 | 1.1×10−9 |
ZTPGA | 695.360 | 695.831 | 697.689 | 701.382 | 0.1046 | 0.5839 |
PLin | 764.852 | 765.082 | 766.404 | 768.866 | 0.3852 | 2.5×10−06 |
EG | 764.645 | 764.876 | 766.198 | 768.660 | 0.38.26 | 7.5×10−06 |
TPM | 771.770 | 772.001 | 773.323 | 775.785 | 0.3873 | 1.36×10−07 |
Model | Par | q | T1 | T2 | T4 |
Empirical | 0.85 | 875.8 | 925.7 | 982.0 | |
0.90 | 917.8 | 939.6 | 1285.2 | ||
0.95 | 933.6 | 955.6 | 1335.8 | ||
0.99 | 1041.1 | 1194.8 | 1571.9 | ||
TL-EE | ˆθ=0.0048 | 0.85 | 825.0 | 932.4 | 10280.6 |
ˆα=2.8201 | 0.90 | 869.9 | 975.6 | 10714.9 | |
ˆλ=67.987 | 0.95 | 944.2 | 1048.2 | 11156.3 | |
0.99 | 1111.6 | 1214.3 | 11601.9 | ||
EE | ˆα=55.743 | 0.85 | 910.8 | 1073.0 | 22573.1 |
ˆθ=0.0064 | 0.90 | 978.3 | 138.4 | 23600.3 | |
0.95 | 1090.5 | 1248.5 | 24654.0 | ||
0.99 | 1344.7 | 1501.1 | 25649.0 | ||
Exp | ˆλ= 0.0014 | 0.85 | 1322.4 | 2019.5 | 415065.0 |
0.90 | 1605.1 | 2302.2 | 439644.4 | ||
0.95 | 2088.2 | 2785.3 | 464424.4 | ||
0.99 | 33210.2 | 3907.3 | 484983.8 |
Mean | Q2 | Sd | Q1 | Q3 | Skew | Kurt |
487.2 | 427.8 | 248.0 | 301.1 | 585.4 | 1.932 | 4.471 |
Distribution | Par | ll |
TL-EE | ˆθ=0.0029 ˆα=0.4591 ˆλ=38.326 | -331.867 |
EE | ˆθ=0.0049 ˆα=5.7608 | -334.355 |
PL | ˆα = 133.818 ˆλ=190.851 ˆθ=3.9591 | -332.767 |
PE | ˆλ=0.0068 ˆθ=14.346 | -333.323 |
Wei | ˆμ=2.1057 ˆσ= 552.523 | -340.802 |
Exp | ˆλ=0.0020 | -359.435 |
Lin | ˆλ=0.0040 | -345.044 |
ZTPGA | ˆα=1.8306 ˆλ=0.0077 ˆθ=6.1417 | -335.129 |
PLin | ˆα=1.5245 ˆβ= 0.0001 | -337.759 |
EG | ˆα=0.0076 ˆp= 0.0338 | -339.999 |
TPM | ˆα=0.0062 ˆδ= 0.3874 | -338.991 |
Model | A1 | A2 | A3 | B1 | KS | P-value |
TL-EE | 669.734 | 670.256 | 671.919 | 675.470 | 0.1089 | 0.5567 |
EE | 672.711 | 672.967 | 674.167 | 676.535 | 0.1470 | 0.2082 |
PL | 671.534 | 672.055 | 673.718 | 677.270 | 0.1124 | 0.5162 |
PE | 670.646 | 670.901 | 672.102 | 674.470 | 0.1181 | 0.4535 |
Wei | 685.604 | 685.859 | 687.060 | 689.428 | 0.1803 | 0.0678 |
Exp | 720.871 | 720.954 | 721.599 | 722.783 | 0.4094 | 4.2×10−08 |
Lin | 692.089 | 692.172 | 692.817 | 694.001 | 0.2846 | 0.0004 |
ZTPGA | 676.258 | 676.780 | 678.442 | 681.994 | 0.1104 | 0.5383 |
PLin | 679.518 | 679.773 | 680.974 | 683.342 | 0.1520 | 0.1784 |
EG | 683.998 | 684.253 | 685.454 | 687.822 | 0.1707 | 0.0961 |
TPM | 681.982 | 682.237 | 683.438 | 685.806 | 0.2147 | 0.0167 |
Model | Par | q | T1 | T2 | T4 |
Empirical | 0.85 | 664.3 | 925.8 | 11899.1 | |
0.90 | 726.9 | 1071.7 | 7070.6 | ||
0.95 | 910.2 | 1207.3 | 3901.6 | ||
0.99 | 1347.8 | 1500.3 | 1500.3 | ||
TL-EE | ˆθ=0.0029 | 0.85 | 687.2 | 942.0 | 26230.5 |
ˆα=0.4591 | 0.90 | 759.2 | 1027.0 | 27617.5 | |
ˆλ=38.326 | 0.95 | 879.9 | 1170.6 | 29096.2 | |
0.99 | 1156.6 | 1500.8 | 30579.1 | ||
EE | ˆα=0.0049 | 0.85 | 731.0 | 861.8 | 37517.5 |
ˆθ=5.7608 | 0.90 | 818.4 | 932.3 | 39320.7 | |
0.95 | 964.4 | 1051.9 | 41155.2 | ||
0.99 | 1296.3 | 1328.3 | 42819.2 | ||
Exp | ˆλ= 0.0020 | 0.85 | 948.5 | 1448.5 | 213948.6 |
0.90 | 1151.2 | 1651.2 | 226651.3 | ||
0.95 | 1497.8 | 1997.8 | 239499.0 | ||
0.99 | 2302.5 | 2802.5 | 250302.6 |