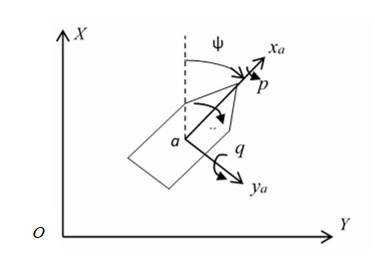
Mycobacterium avium subsp. paratuberculosis (MAP) may play a role in the pathology of human inflammatory bowel disease (IBD). Previously, we found a high frequency (98% in patients with active disease) of MAP DNA detection in the blood of Portuguese Crohn's Disease patients, suggesting this cohort has high exposure to MAP organisms. Water is an important route for MAP dissemination, in this study we therefore aimed to assess MAP contamination within water sources in Porto area (the residential area of our IBD study cohort).
Water and biofilms were collected in a wide variety of locations within the Porto area, including taps connected to domestic water sources and from municipal water distribution systems. Baseline samples were collected in early autumn plus further domestic water samples in early winter, to assess the effect of winter rainfall. DNA was extracted from all 131 samples and IS900-based nested PCR used to assess the frequency of MAP presence.
Our results show high MAP positivity in municipal water sources (20.7% of water samples and 41.4% of biofilm samples) and even higher amongst domestic sources (30.8% of water samples and 50% of biofilm samples). MAP positivity in biofilms correlated with positivity in water samples from the same sources. A significantly higher frequency of MAP-positivity was observed during winter rains as compared with samples collected in autumn prior to the winter rainfall period (61.9% versus 30.8%). We conclude that domestic and municipal water sources of Porto region have a high burden of MAP contamination and this prevalence increases with rainfall. We hypothesize that human exposure to MAP from local water supplies is commonplace and represents a major route for MAP transmission and challenge which, if positively linked to disease pathology, may contribute to the observed high prevalence of IBD in Porto district.
Citation: Telma Sousa, Marta Costa, Pedro Sarmento, Maria Conceição Manso, Cristina Abreu, Tim J. Bull, José Cabeda, Amélia Sarmento. DNA-based detection of Mycobacterium avium subsp. paratuberculosis in domestic and municipal water from Porto (Portugal), an area of high IBD prevalence[J]. AIMS Microbiology, 2021, 7(2): 163-174. doi: 10.3934/microbiol.2021011
[1] | Zhe Zou, Minjie Zheng . Design and stabilization analysis of luxury cruise with dynamic positioning systems based on sampled-data control. Mathematical Biosciences and Engineering, 2023, 20(8): 14026-14045. doi: 10.3934/mbe.2023626 |
[2] | Bin Hang, Beibei Su, Weiwei Deng . Adaptive sliding mode fault-tolerant attitude control for flexible satellites based on T-S fuzzy disturbance modeling. Mathematical Biosciences and Engineering, 2023, 20(7): 12700-12717. doi: 10.3934/mbe.2023566 |
[3] | Shuaiwen Sun, Zhijing Xu . Large kernel convolution YOLO for ship detection in surveillance video. Mathematical Biosciences and Engineering, 2023, 20(8): 15018-15043. doi: 10.3934/mbe.2023673 |
[4] | Xueyan Wang . A fuzzy neural network-based automatic fault diagnosis method for permanent magnet synchronous generators. Mathematical Biosciences and Engineering, 2023, 20(5): 8933-8953. doi: 10.3934/mbe.2023392 |
[5] | Wei Zou, Yanxia Shen, Lei Wang . Design of robust fuzzy iterative learning control for nonlinear batch processes. Mathematical Biosciences and Engineering, 2023, 20(11): 20274-20294. doi: 10.3934/mbe.2023897 |
[6] | Lixiang Zhang, Yian Zhu, Jie Ren, Wei Lu, Ye Yao . A method for detecting abnormal behavior of ships based on multi-dimensional density distance and an abnormal isolation mechanism. Mathematical Biosciences and Engineering, 2023, 20(8): 13921-13946. doi: 10.3934/mbe.2023620 |
[7] | Yue Song, Yi Zhang, Song Yang, Na Li . Investigation on stability and controller design for singular bio-economic systems with stochastic fluctuations. Mathematical Biosciences and Engineering, 2021, 18(3): 2991-3005. doi: 10.3934/mbe.2021150 |
[8] | Qianhong Zhang, Fubiao Lin, Xiaoying Zhong . On discrete time Beverton-Holt population model with fuzzy environment. Mathematical Biosciences and Engineering, 2019, 16(3): 1471-1488. doi: 10.3934/mbe.2019071 |
[9] | Chaoyue Wang, Zhiyao Ma, Shaocheng Tong . Adaptive fuzzy output-feedback event-triggered control for fractional-order nonlinear system. Mathematical Biosciences and Engineering, 2022, 19(12): 12334-12352. doi: 10.3934/mbe.2022575 |
[10] | Jinxin Du, Lei Liu . Adaptive fuzzy fixed time formation control of state constrained nonlinear multi-agent systems against FDI attacks. Mathematical Biosciences and Engineering, 2024, 21(3): 4724-4741. doi: 10.3934/mbe.2024207 |
Mycobacterium avium subsp. paratuberculosis (MAP) may play a role in the pathology of human inflammatory bowel disease (IBD). Previously, we found a high frequency (98% in patients with active disease) of MAP DNA detection in the blood of Portuguese Crohn's Disease patients, suggesting this cohort has high exposure to MAP organisms. Water is an important route for MAP dissemination, in this study we therefore aimed to assess MAP contamination within water sources in Porto area (the residential area of our IBD study cohort).
Water and biofilms were collected in a wide variety of locations within the Porto area, including taps connected to domestic water sources and from municipal water distribution systems. Baseline samples were collected in early autumn plus further domestic water samples in early winter, to assess the effect of winter rainfall. DNA was extracted from all 131 samples and IS900-based nested PCR used to assess the frequency of MAP presence.
Our results show high MAP positivity in municipal water sources (20.7% of water samples and 41.4% of biofilm samples) and even higher amongst domestic sources (30.8% of water samples and 50% of biofilm samples). MAP positivity in biofilms correlated with positivity in water samples from the same sources. A significantly higher frequency of MAP-positivity was observed during winter rains as compared with samples collected in autumn prior to the winter rainfall period (61.9% versus 30.8%). We conclude that domestic and municipal water sources of Porto region have a high burden of MAP contamination and this prevalence increases with rainfall. We hypothesize that human exposure to MAP from local water supplies is commonplace and represents a major route for MAP transmission and challenge which, if positively linked to disease pathology, may contribute to the observed high prevalence of IBD in Porto district.
Ship dynamic positioning is a system used in ships and offshore vessels to automatically maintain the ship's positions and heading by thrusters and propulsion systems [1]. Eliminating the need for traditional anchoring, dynamic positioning ship (DPS) is equipped with a sophisticated system that allows it to stay in a specific location or follow a set track. And DPS has been used in various maritime applications, including offshore oil and gas exploration, subsea construction, cable laying, and so on. Recently, there is a wealth of research in the academic literature on the control for DPS ([2,3,4,5,6,7]), and the control algorithms and strategies include that proportional integral derivative (PID) control, adaptive control, intelligent control, etc. [2]. The research direction includes sensor fusion and position reference systems [3]; robust control [4]; fault tolerance control [5]; cooperative control and networked system [6]; simulation and validation [7] etc. For example, in [3], the technology of sensor fusion and the selection and optimization of position reference systems for DPS are discussed, which emphasizes the requirements of system accuracy and reliability. Reference [4] uses H∞ robust control techniques for DPS to obtain a robust controller with better performance than traditional dynamic positioning systems. Trajectory preservation is achieved through appropriate weighting functions. Reference [5] discusses the asymptotic stability conditions for DPS with partial actuator failure. The saturation proportional differential controller has been designed, and the asymptotic stability conditions are given by using the LKF approaches. In [6], a new event triggered collaborative DP control algorithm is proposed for multiple surface vessels. This algorithm adopts an undirected interactive topology to construct a communication network between surface vessels, so that all followers can obtain leader information. Reference [7] proposes a multi physics modeling method for a comprehensive electric propulsion system used for DPS, which can run in real-time and connect to control hardware.
In recent years, due to the advantages of discreteness, high efficiency, and low communication costs, the sampled-data control system has received widespread attention and it has been widely applicated in various systems, such as the hypersonic vehicle system [8,9], Lur'e system [10,11,12], neural networks system [13,14,15,16,17], Markovian jump system [18,19] and so on [20,21]. It can be applied to practical system such as fast train [22], unmanned aerial vehicle [23], large cruise liner [24,25], small unmanned ships [26], etc. Nowadays, the Takagi-Sugeno fuzzy system (TSFS) has received widespread attention due to their significant effectiveness in describing the complex nonlinear system, and considerable sampled-data control methods are reported for TSFS [27,28]. For example, in [29], the sampled-data control issue for TSFS with random time delay and actuator faults is studied using the input-delay method; In [30], the stability issue for nonlinear sampled-data TSFS is studied using the LKF method, which is validated by a single link robotic arm with an electric motor model. In [31], the stability characteristics of TSFS under sampling-data control are studied. The system is considered as a variable time delay system based on the input delay method. An appropriate LKF candidates and quadratic delay product terms are provided. Reference [32] studies the nonfragile sampling control problem for TSFS with parameter uncertainty. A new augmented LKF with sufficient sample data information has been constructed, which results in less conservative results.
Recently, the research on sampled-data control systems for DPS has attracted widespread attention. In [33], A semi-globally practically asymptotically stable controller is designed using the integration and backstepping technique of the Euler approximation models. In [34], based on the Euler approximation model and nonlinear sampling control theory, a state feedback controller and an output feedback controller based on a reduced order observer are designed for DPS to stabilize the tracking error. In [35], the sampling control system was applied to the dynamic positioning ship, and the input delay method was combined with the Wirtinger integral inequality to obtain results with less conservatism. In [36], the control problem of a sampling system for DPS with actuator failure is discussed, and a sampling controller is designed to ensure the exponential stability of the system and meet the H∞ performance index. In [37], the fuzzy robust stability criterion of sampled-control systems for DPS is studied, and a fuzzy sampling controller is designed using an input time delay method to ensure that the system remains stable under external disturbances. In [38], the reliable tracking control problem for DPS based on nonperiodic measurement information is studied and the mean square exponential stability criterion is obtained.
However, there is still much room for further study for the sampled-data DPS. Firstly, some important characteristics are still overlooked, which include the features of sampling pattern and the effective characteristics of the constructed LKF. Secondly, the positivity limit of the Lyapunov matrix is strict, which will result in the conservatism of the system.
Inspired by the above discussions, we aim to study the stability condition and controller design for sampled-data DPS with the T-S fuzzy model. In addition, we will construct an improved LKF by adding the new useful terms and introducing the reciprocally convex method. The main advantages of this article are be listed that:
(1) To capture the features of sampling pattern fully, some novel terms, like (tk+1−t)t∫tke2αs[x(s)x(tk)]T[R1R2∗R3][x(s)x(tk)]ds are introduced in the constructed LKF.
(2) The tighter technique of reciprocally convex combination is introduced during the differentiation of LKF. Hence, the conservatism of stability criteria can be greatly reduced.
(3) A second-order term (tk+1−t)(t−tk)[xT(tk)N1x(tk)] related to time t is added during the construction of V(t), thereby a less conservative result is obtained.
Notations: Rm denotes m-dimensional Euclidean space, and " T " represents the matrix transposition. "*" is a symmetric block matrix.
Consider the dynamic equations for DPS as follows:
M˙υ(t)+Dυ(t)+Gη(t)=u(t)+w(t),˙η(t)=J(ψ(t))υ(t), | (1) |
where
M=[m11000m22m230m32m33],D=[n11000n22n230n32n33],G=[g11000g22000g33],J(ψ(t))=[cos(ψ(t))−sin(ψ(t))0sin(ψ(t))cos(ψ(t))0001]. |
where η(t)=[xa(t)ya(t)ψ(t)]T is the positions and heading angle in the northeast coordinate system respectively. υ(t)=[p(t)v(t)r(t)]T represents the surge, sway, and yaw velocity vector in the attached coordinate system (see Figure 1). J(ψ) is the coordinate rotation matrix. u(t) is the control force vector; M and D denote the inertial matrix and damping matrix, respectively. G is the mooring force matrix, and w(t)=[w1(t),w2(t),w3(t)] is the environmental disturbance including wind, waves, and currents.
Remark 1. During the modelling of the ship, it can be considered that the interaction forces between the various points of action of the ship are ignored, that is, the ship is considered as a rigid body. Hence, the combination of forward, lateral, and turning motions with heave, roll, and pitch motions is neglected, and we only consider the three degrees of freedom plane motion of the DPS.
Define
x(t)=[η(t)υ(t)]T=[xp(t)yp(t)ψ(t)p(t)q(t)r(t)]T, | (2) |
Let
−M−1D=[a11000a22a230a32a33],M−1=[d11000d22d230d32d33],−M−1G=[b11000b22b230b32b33]. | (3) |
Substitute (2) and (3) into (1) such that
˙x(t)=Ax(t)+Bu(t)+Bww(t), | (4) |
where
A=[000cos(ψ(t))−sin(ψ(t))0000sin(ψ(t))cos(ψ(t))0000001b1100a11000b22b230a22a230b32b330a32a33],B=Bw=[000000000d11000d22d230d32d33], |
where a11,a22,a23,a32,a33,d11,d22,d23,d32,d33,b11,b22,b23,b32,b33 are constant.
The global fuzzy models can be described as follows:
Mode Rule i: IF z1(t) is fi1, ⋯zn(t) is fin, THEN
˙x(t)=Aix(t)+Biu(t)+Bwiw(t),i=1,2,…,n | (5) |
where z1(t),z2(t),…,zn(t) represent the premise variables, fij is the fuzzy set, and n is the rules number.
Assume the yaw angle satisfies ψ(t)=[−π/π22,π/π22], then the controller rules are considered such that:
Model Rule 1:
IF ψ(t)=0, THEN
˙x(t)=A1x(t)+B1u(t)+Bw1w(t), | (6) |
Model Rule 2:
IF ψ(t)=π2, THEN
˙x(t)=A2x(t)+B2u(t)+Bw2w(t), | (7) |
Model Rule 3:
IF ψ(t)=−π2, THEN
˙x(t)=A3x(t)+B3u(t)+Bw3w(t), | (8) |
where
A1=[0001−α0000α10000001b1100a11000b22b230a22a230b32b330a32a33],A2=[000β−100001β0000001b1100a11000b22b230a22a230b32b330a32a33],A3=[000β10000−1β0000001b1100a11000b22b230a22a230b32b330a32a33],Bi=Bwi=[000000000d11000d22d230d32d33],i=1,2,3. |
where α=sin(2∘), β=cos(88∘). The global fuzzy models are inferred such that:
˙x(t)=3∑i=1μi(z(t))[Aix(t)+Biu(t)+Bwiw(t)], | (9) |
where
μi(z(t))=ωi(z(t))3∑i=1ωi(z(t))⩾0,i=1,2,3,ωi(z(t))=n∏j=1fij(zj(t)),3∑i=1μi(z(t))=1,z(t)=[z1(t),z2(t),…,zn(t)], |
The membership function of ψ(t) is shown in Figure 2.
Assuming that the states of the DPS are obtained at each sampling instant tk, the sampling time is assumed to be
0=t0<t1<⋯<tk<⋯<limk→∞tk=+∞. |
The structure of the system is depicted in Figure 3.
The system stability process is shown in Figure 4. It can be seen that the DPS fuzzy controller calculates the ship's control force and moment based on the deviation between the desired and actual position and velocity of DPS. Then, the thrust allocation distributes control force and moment for DPS to generate the actual position and velocity under the environmental disturbances, which achieves the stability target of the system.
Let d represent the upper bound of the sampling interval, i.e.,
0<tk+1−tk⩽d,∀k⩾0,d>0, |
then we consider the following sampled-data controller
u(t)=Kx(tk),tk⩽t<tk+1, | (10) |
where K represents the controller matrix. Then the T-S fuzzy controller can be described that:
Controller Rule 1:
IF ψ(t)=0, THEN
u(t)=K1x(tk). | (11) |
Controller Rule 2:
IF ψ(t)=π2, THEN
u(t)=K2x(tk). | (12) |
Controller Rule 3:
IF ψ(t)=−π2, THEN
u(t)=K3x(tk). | (13) |
Hence, the overall fuzzy controller is inferred to be:
u(t)=3∑j=1μj(z(t))Kjx(tk),tk⩽t<tk+1,k=0,1,2,… | (14) |
Substitute (14) into (11)
˙x(t)=3∑i=13∑j=1μi(z(t))μj(z(t))[Aix(t)+BiKjx(tk)+Bwiw(t)] | (15) |
When the system has an uncertainty parameter, the system is converted into
˙x(t)=3∑i=13∑j=1μi(z(t))μj(z(t))[(Ai+ΔA)x(t)+(Bi+ΔB)Kjx(tk)+Bwiw(t)] | (16) |
where ΔA and ΔB are the uncertainty parameter matrix, and satisfy
[ΔA(t)ΔB(t)]=DF(t)[EaEb] | (17) |
D,Ea,Eb are known dimensional constant matrices, and F(t) is unknown time-varying matrices with Lebesgue measurable elements that satisfy
FT(t)F(t)⩽I,∀t | (18) |
For the further deriving process, the two lemmas are given.
Lemma 1 [39] For any matrix H>0, and a vector with appropriate dimension μ(s), the inequality satisfies
−∫t2t1μT(s)Hμ(s)ds⩽(t2−t1)ξTtFTH−1Fξt+2ξTtHT∫t2t1μ(s)ds | (19) |
where, matrix F and vector ξt are not dependent on integral variables and have any dimensions.
Lemma 2 [40] For any matrix J,R=RT>0, constant d1⩽d(t)⩽d2, and vector function ˙x:[d2,d1]→Rn, the following integral inequality holds:
(d1−d2)∫t−d1t−d2˙xT(s)R˙x(s)ds⩽−ϑT(t)[I−I00I−I]TW[I−I00I−I]ϑ(t) | (20) |
where
ϑT(t)=[xT(t−d1)xT(t−d(t))xT(t−d2)],W=[−RJ∗−R]⩽0 |
Lemma 3 [41] Given matrices ΩT=Ω,Γ,X, for any F satisfying FTF⩽L, the inequality is established
Ω+HFE+ETFTHT<0 | (21) |
and only if there is a scalar ε>0, then
Ω+εHHT+ε−1ETLE<0 | (22) |
In the section, a novel LKF is constructed, and the stabilization condition of system (15) is proposed. To begin, the notation is defined as follows:
ξT(t)=[xT(t)˙xT(t)xT(tk)xT(t−d)∫ttkxT(tk)] |
Theorem 1: The system (15) with w(t)=0 is asymptotically stable, if there exist positive-definite matrices X=[XT1XT2XT3XT4XT5]T, P=[P1P2∗P3],Q,Z,U,S,R,N1,N2,M=[M11M12∗M22], and given scale d>0, such that
[ZU∗Z]>0 | (23) |
Ψ1+dΨ3<0 | (24) |
[Ψ1+dΨ2dX∗−dM−111]<0 | (25) |
where
Ψ1=[Ξ11Ξ12Ξ13Ξ14Ξ15∗Ξ22Ξ230P2∗∗Ξ33Ξ34Ξ35∗∗∗Ξ440∗∗∗∗0]Ψ2=−IT3M22I3−IT3N1I3Ψ3=IT23MI23+IT3N1I3Ξ11=G1Ai+ATiGT1+P2+PT2+Q−Z+X1Ξ12=−G1+SRT+ATiG2+P1+XT2Ξ13=G1BiKj−MT12+Z−U+XT3−X1Ξ14=U+XT4Ξ15=PT3+XT5Ξ22=−G2−GT2+d2ZΞ23=G2BiKj−X2Ξ33=M12−2Z+UT+U−X3Ξ34=Z−U−XT4 |
Ξ35=−XT5−PT3Ξ44=−Q−ZI23=[0I000000I000],I3=[00I000],L=diag{l1,l2,⋯ln} |
Proof. Consider the following LKF:
V(t)=5∑i=1Vi(t),t∈[tk,tk+1)V1(t)=[x(t)∫ttkx(s)ds]T[P1P2PT2P3][x(t)∫ttkx(s)ds]V2(t)=∫tt−dx(s)TQx(s)dsV3(t)=(d−(t−tk))∫ttk[˙x(s)x(tk)]TM[˙x(s)x(tk)]dsV4(t)=(tk+1−t)(t−tk)[xT(tk)N1x(tk)]V5(t)=d∫0−d∫tt+θ˙xT(s)Z˙x(s)dsdθ | (26) |
Take the derivative of V(t), which yields
˙V1(t)=2[x(t)∫ttkx(s)ds]T[P1+SRTP2PT2P3][˙x(t)x(t)−x(tk)]=2xT(t)P1˙x(t)+2(∫ttkx(s)ds)TPT2˙x(t)+2xT(t)P2x(t)+2(∫ttkx(s)ds)TP3x(t)+2xT(t)SRT˙x(t)−2(∫ttkx(s)ds)TP3x(tk) | (27) |
˙V2(t)=x(t)TQx(t)−x(t−d)TQx(t−d) | (28) |
˙V3(t)=−∫ttk[˙x(s)x(tk)]TM[˙x(s)x(tk)]ds+(d−(t−tk))[˙x(t)x(tk)]TM[˙x(t)x(tk)]=−∫ttk˙x(s)M11˙x(s)ds−2x(tk)M12[x(t)−x(tk)]−(t−tk)x(tk)M22x(tk)+(d−(t−tk))ς(t)MςT(t) | (29) |
˙V4(t)=(dk−(t−tk))[xT(tk)N1x(tk)]−(t−tk)[xT(tk)N1x(tk)]⩽(d−(t−tk))[xT(tk)N1x(tk)]−(t−tk)[xT(tk)N1x(tk)] | (30) |
˙V5(t)=d2˙xT(t)Z˙x(t)−d∫tt−d˙xT(t)Z˙x(t)ds | (31) |
By Lemma 1, it can be obtained that
−∫ttk˙x(s)M11˙x(s)ds⩽(t−tk)ξT(t)XM−111XTξ(t)+2ξT(t)X[x(t)−x(tk)] | (32) |
Then
˙V3(t)⩽(t−tk)ξT(t)VM−111VTξ(t)+2ξT(t)V[x(t)−x(tk)]−2x(tk)M12[x(t)−x(tk)]−(t−tk)x(tk)M22x(tk)+(d−(t−tk))ς(t)MςT(t) |
By Lemma 2, we have
−d∫tt−d˙xT(s)Z˙x(s)ds⩽ϑT(t)[−ZZ−UU∗−2Z+UT+UZ−U∗∗−Z]ϑ(t) | (33) |
where
ϑ(t)=[xT(t)xT(tk)xT(t−d)]T |
then
˙V5(t)⩽d2˙xT(t)Z˙x(t)−ϑT(t)[Z−Z+U−U∗2Z−UT+U−Z+U∗∗Z]ϑ(t) | (34) |
For any matrixes G1,G2, we have
2[xT(t)G1+˙xT(t)G2]×[−˙x(t)+r∑i=1r∑j=1μi(θ(t))μj(θ(t))[Aix(t)+BiKjx(tk)]]=0 | (35) |
For any diagonal matrix D>0, it can be obtained that
−2[Lx(tk)]TD[−Lx(tk)]⩾0 | (36) |
Then, from (27) to (36), we have
˙V(t)⩽3∑i=13∑j=1μi(θ(t))μj(θ(t))[ξT(t)Ψ(τ(t))ξ(t)] | (37) |
where
τ(t)=t−tkξT(t)=[xT(t)˙xT(t)xT(tk)xT(t−d)∫ttkxT(tk)]Ψ(τ(t))=Ψ(t−tk)=Ψ1+(t−tk)(Ψ2+VTˉM−111V)+(d−(t−tk))Ψ3 |
Ψ1,Ψ2,Ψ3 is defined in Theorem 1.
By Lemma 2, we can derive a linear convex combination about τ(t), for all τ(t)∈[0,d], if
Ψ(0)=Ψ1+dΨ3<0 | (38) |
Ψ(d)=Ψ1+d(Ψ2+VTˉM−11V)<0 | (39) |
Then, Ψ(τ(t)) is negative definite.
Following the Schur complement, (24) and (25) imply (38) and (39), which means that ˙V(t)<0. This proof is completed.
Remark 1. In the constructed LKF, V1(t) and V2(t) are the general energy function of the system state, and it represents the energy changes of the system state and derivative from t−d to t. It is the foundation of the entire LKF. V5(t) symbolizes the relationship between state derivatives and time delay, and it is the foundation of time delay dependent stability standards. Additionally, V3(t) and V4(t) include the characteristic of time delay, and they play an important role in obtaining the final solution.
Remark 2. Compared with literature [42], two (tk,tk+1) dependent terms like (tk+1−t)t∫tke2αs[x(s)x(tk)]T[R1R2∗R3][x(s)x(tk)]ds and (tk+1−t)(t−tk)[xT(tk)N1x(tk)] are added in the LKF, which means that the available characteristic of sampling patterns are fully captured, and the LKF has a more general form.
Remark 3. In some previously published papers (such as [43,44]), they used overly relaxed delimitation techniques when estimating some integral terms such as −d∫tkt−d˙xT(s)Z˙x(s)ds. However, this paper directly uses a tighter technique by reciprocally convex combination in Lemma 2. Besides, free matrices G and G1 are proposed during the derivation procedure of LKF, which improves the design flexibility. Therefore, the conservatism of stability criteria can be greatly reduced.
To prove the robustness of the system, the following H∞ performance function is proposed
Jyw=∫t0[yT(s)y(s)−γ2wT(s)w(s)]ds,γ>0 | (40) |
where w(t)≠0 and w(t)∈L2[0,∞), and y(t) denotes the system output, and satisfies that
y(t)=3∑i=13∑j=1μi(z(t))μj(z(t))Cix(t), | (41) |
Then, based on the Theorem 2, the system (15) can be proved to satisfy the robust performance with disturbances.
Theorem 2: the system (15) is asymptotically stable, if there exist positive-definite matrices X=[XT1XT2XT3XT4XT5]T, P=[P1P2∗P3],Q,Z,U,S,R,N1,N2,M=[M11M12∗M22], and given scale d>0, such that
[ZU∗Z]>0 | (42) |
Ψ1+dΨ3<0 | (43) |
[Ψ1+dΨ2dX∗−dM−111]<0 | (44) |
where
˜Ψ1=[˜Ξ11Ξ12Ξ13Ξ14Ξ150∗Ξ22Ξ230P20∗∗Ξ33Ξ34Ξ350∗∗∗Ξ4400∗∗∗∗00∗∗∗∗∗−γ2I]˜Ξ11=G1Ai+ATiGT1+P2+PT2+Q−Z+X1+CTC |
Ξ12,Ξ13,Ξ14,Ξ15,Ξ22,Ξ23,Ξ33,Ξ34,Ξ35,Ξ44 are defined in Theorem 1.
Prove:
Jyw=∫t0[yT(s)y(s)−γ2wT(s)w(s)]ds,=∫t0[yT(s)y(s)−γ2wT(s)w(s)+˙V(s)]ds−V(t)⩽∫t0[yT(s)y(s)−γ2wT(s)w(s)+˙V(s)]ds⩽3∑i=13∑j=1μi(θ(t))μj(θ(t))[ζT(t)Θ(τ(t))ζ(t)] | (45) |
where
τ(t)=t−tkζT(t)=[xT(t)˙xT(t)xT(tk)xT(t−d)∫ttkxT(tk)wT(t)]Ψ(τ(t))=Ψ(t−tk)=˜Ψ1+(t−tk)(Ψ2+VTˉM−111V)+(d−(t−tk))Ψ3 |
The proof is finished.
Next, Theorem 3 provides the stability conditions for systems with norm bounded uncertainty.
Theorem 3: the system (15) is asymptotically stable, if there exist positive-definite matrices X=[XT1XT2XT3XT4XT5]T, P=[P1P2∗P3],Q,Z,U,S,R,N1,N2,M=[M11M12∗M22], and given scale d>0,ε>0, such that
[ZU∗Z]>0 | (46) |
Ψ1+dΨ3<0 | (47) |
[Ψ1+dΨ2dX∗−dM−111]<0 | (48) |
where
ˆΨ1=[Ξ11+εETaEaΞ12Ξ13+εETaEbΞ14Ξ15G1D∗Ξ22Ξ230P2G2D∗∗Ξ33+εETbEbΞ34Ξ350∗∗∗Ξ4400∗∗∗∗00∗∗∗∗∗−εI] |
Ξ12,Ξ13,Ξ14,Ξ15,Ξ22,Ξ23,Ξ33,Ξ34,Ξ35,Ξ44 are defined in Theorem 1.
Prove: replace A and Ad in (25) with A+DF(t)Ea and B+DF(t)Eb, respectively. It can be obtained that:
Ψ+ΓF(t)Ω+ΩTFT(t)ΓT<0 | (49) |
where
Γ=[G1DG2D000],Ω=[Ea0Eb00] |
According to Lemma 3, if there exist a scale ε>0, the following inequality holds
Ψ+εΩTΩ+ε−1ΓΓT<0 | (50) |
According to Schu's complement, Eq (48) is equivalent to Eq (50). The proof is completed.
Then, the controller method can be obtained according to Theorem 4.
Theorem 4: for a given scale d>0, if there exist positive-definite matrices X=[XT1XT2XT3XT4XT5]T, P=[P1P2∗P3],Q,Z,U,S,R,N1,N2,M=[M11M12∗M22], such that
[ZU∗Z]>0 | (51) |
ˉΨ1+dˉΨ3<0 | (52) |
[ˉΨ1+dˉΨ2dˉX∗−dˉM−111]<0 | (53) |
where
ˉΨ1=[ˉΞ11ˉΞ12ˉΞ13ˉΞ14ˉΞ15∗ˉΞ22ˉΞ230ˉP2∗∗ˉΞ33ˉΞ34ˉΞ35∗∗∗ˉΞ440∗∗∗∗0]ˉΨ2=−IT3ˉM22I3−IT3ˉN1I3ˉΨ3=IT23ˉMI23+IT3ˉN1I3ˉΞ11=AiG+GTATi+ˉP2+ˉPT2+ˉQ−Z+ˉX1ˉΞ12=−G+εGATi+SˉRT+ˉP1+ˉXT2ˉΞ13=BiˉKj−ˉMT12+ˉZ−ˉU+ˉXT3−ˉX1ˉΞ14=ˉUE+ˉXT4ˉΞ15=ˉPT3+ˉXT5ˉΞ22=−εG−εGT+d2ˉZˉΞ23=εBiˉKj−ˉX2ˉΞ33=ˉM12−2ˉZ+ˉUT+ˉU−ˉX3ˉΞ34=Z−U−ˉXT4ˉΞ35=−ˉXT5−ˉPT3ˉΞ44=−ˉQ−ˉZI23=[0I000000I000]I3=[00I000]L=diag{l1,l2,⋯ln}ξT(t)=[xT(t)˙xT(t)xT(tk)xT(t−d)∫ttkxT(tk)] |
then the system (15) with w(t)=0 is asymptotically stable. Moreover, the controller gain is
Kj=ˉKjG−1 | (54) |
Proof: Define
G1=G−1,G2=εG−1,ˉK=KG,ˉP=diag{ G,G}Pdiag{ G,G},ˉM=diag{ G,G,G}Mdiag{ G,G,G},ˉD=GDG,ˉQ=GQG,ˉN1=GN1G,ˉN2=GN2G,ˉR=GRG,ˉU=GUG,ˉZ=GZG. |
Let diag{ G,G,G,G,G,G}T and diag{ G,G,G,G,G,G}. By pre- and post-multiplying (24) respectively, (52) can be obtained. Let diag{ G,G,G,G,G,G,G}T and diag{ G,G,G,G,G,G,G}, pre- and post-multiplying (25) respectively, (53) can be obtained. This completes the proof.
Remark 4. The system (15) can obtain less conservativeness than that in [45], which is mainly due to two results. First, during the construction of V(t), a second-order term (tk+1−t)(t−tk)[xT(tk)N1x(tk)] related to time t is also added, which can reduce the conservatism of the results to some extent. Second, during the derivative of the LKF, the reciprocally convex combination method is used, which can obtain a more stringent derivative upper limit than Jensen's inequality, thereby less conservativeness will be achieved.
To demonstrate the merits of the method, a practical example is carried out. The M and D is considered as follows [46].
M=[1.08520002.0575−0.40870−0.40870.2153],D=[0.08650000.07620.151000.01510.0031],G=[0.03890000.02660000]. |
Let α=sin20 and β=cos880, then
A1=[0001.0000−0.034900000.03491.00000000001.0000−0.035800−0.0797000−0.020800−0.0818−0.12240−0.039400−0.2254−0.2468], |
A2=[0000.0349−1.000000001.00000.03490000001.0000−0.035800−0.0797000−0.020800−0.0818−0.12240−0.039400−0.2254−0.2468],A3=[0000.03491.00000000−1.00000.03490000001.0000−0.035800−0.0797000−0.020800−0.0818−0.12240−0.039400−0.2254−0.2468], |
Bi=Bwi=[0000000000.92150000.78021.481101.48117.4562],i=1,2,3. |
The allowable maximum sampling interval is an important control performance index of the sampled-data system. A longer sampling period indicates a lower communication burden and fewer time consumption of the actuator, which helps to save energy, time and so on. Table 1 lists the results which uses different methods to obtain the maximum sampling internal. From Table 1, it can be seen that the sampling internal in Theorem1 improves [36,37,38] about 189.2%, 173.86%, and 35.9%, respectively, which means that the designed fuzzy controller is beneficial to obtain a larger maximum sampling interval.
Assume the initial value of the system that xs(t)=[15m15m0.2∘0m/s0m/s0∘/s].
Then, the gain can be computed such that
K1=[−0.0281−0.0444−0.0339−0.7195−0.0002−0.00030.0708−0.00850.04570.0004−1.38770.2656−0.01420.00740.0088−0.00010.2795−0.1371]K2=[−0.01230.01660.0170−0.7473−0.00020.0000−0.02280.02810.07900.0002−1.44020.27450.00470.00020.0029−0.00010.2900−0.1421]K3=[−0.01230.01660.0170−0.7473−0.00020.0000−0.02280.02810.07900.0002−1.44020.27450.00470.00020.0029−0.00010.2900−0.1421] |
To demonstrate the control performance of the proposed method, Figures 5–10 present the comparison results with LKF in [36]. From Figures 5–10, it illustrates that the system state xs(t) reaches the expected values within approximately 10 seconds, while [36] achieves the desired target and requires a longer time. This indicates that the response speed in the proposed method is faster. Besides, the system's oscillation amplitude is smaller, which indicates that our result has good anti-interference ability under environment disturbance. In addition, Figures 5–10 further illustrate that the proposed method has more preferable control performance.
The stabilization issue for fuzzy DPS with sampled-data is studied. To reduce the conservative and improve the sampling interval, a novel LKF is constructed by adding some integral terms, which can utilize the information of actual sampling modes fully. Then a fuzzy sampling control scheme is proposed. By introducing free matrices and reciprocally convex approach, the conservatism of the system is reduced. Simulation research demonstrates that the proposed method can achieve better control performance. However, the proposed methodologies didn't consider the lower bound of the sampling interval, and some matrix in the LKF are still required to be positive definite. Additionally, how to avoid the emergence of hierarchical phenomena in the system is an urgent problem to be solved. Furthermore, we will improve the LKF and discuss the aperiodic sampled-data control problem for DPS, which both considers the lower and upper bound of the sampling interval.
The authors declare they have not used Artificial Intelligence (AI) tools in the creation of this article.
This study was funded by National Natural Science Foundation of China (52371369); the Key Projects of National Key R & D Program (No.2021YFB390150), the National Science Project of Fujian Province (No. 2020J01659, 2022J01323, 2021J01822, 2020J01660, 20230019), the Fuzhou-Xiamen-Quanzhou In-dependent Innovation Region Cooperated Special Foundation (No: 3502ZCQXT2021007); Funds of Fujian Provincial for Promoting High Quality Development of Marine and Fisheries Industry (No. FJHYF-ZH-2023-10).
The authors declare there is no conflict of interest.
[1] |
McClure HM, Chiodini RJ, Anderson DC, et al. (1987) Mycobacterium paratuberculosis infection in a colony of stumptail macaques (Macaca arctoides). The J Infect Dis 155: 1011-1019. doi: 10.1093/infdis/155.5.1011
![]() |
[2] |
Judge J, Kyriazakis I, Greig A, et al. (2005) Clustering of Mycobacterium avium subsp. paratuberculosis in rabbits and the environment: how hot is a hot spot? Appl Environ Microb 71: 6033-6038. doi: 10.1128/AEM.71.10.6033-6038.2005
![]() |
[3] |
Good M, Clegg T, Sheridan H, et al. (2009) Prevalence and distribution of paratuberculosis (Johne's disease) in cattle herds in Ireland. Irish Vet J 62: 597-606. doi: 10.1186/2046-0481-62-9-597
![]() |
[4] |
Nielsen SS, Toft N (2009) A review of prevalences of paratuberculosis in farmed animals in Europe. Prev Vet Med 88: 1-14. doi: 10.1016/j.prevetmed.2008.07.003
![]() |
[5] |
Manning EJB, Collins MT (2001) Mycobacterium avium subsp. paratuberculosis: pathogen, pathogenesis and diagnosis. Rev Sci Tech Off Int Epiz 20: 133-150. doi: 10.20506/rst.20.1.1275
![]() |
[6] |
Waddell LA, Rajic A, Stark KDC, et al. (2015) The zoonotic potencial of Mycobacterium avium ssp. paratuberculosis: a systematic review and meta-analysis of the evidence. Epidemiol Infect 143: 3135-3157. doi: 10.1017/S095026881500076X
![]() |
[7] |
Zamani S, Zali MR, Aghdaei HA, et al. (2016) Mycobacterium avium subsp. paratuberculosis and associated risk factors for inflammatory bowel disease in Iranian patients. Gut Pathog 9: 1-10. doi: 10.1186/s13099-016-0151-z
![]() |
[8] |
Kuenstner JT, Potula R, Bull TJ, et al. (2020) Presence of infection by Mycobacterium avium subsp. paratuberculosis in the blood of patients with Crohn's disease and control subjects shown by multiple laboratory culture and antibody methods. Microorg 8: 2054. doi: 10.3390/microorganisms8122054
![]() |
[9] |
Annese V (2020) Genetics and epigenetics of IBD. Pharmacol Res 159: 104892. doi: 10.1016/j.phrs.2020.104892
![]() |
[10] |
Turpin W, Goethel A, Bedrani L, et al. (2018) Determinants of IBD heritability: genes, bugs, and more. Inflamm Bowel Dis 24: 1133-1148. doi: 10.1093/ibd/izy085
![]() |
[11] |
Alam MT, Amos GCA, Murphy ARJ, et al. (2020) Microbial imbalance in inflammatory bowel disease patients at different taxonomic levels. Gut Pathog 12: 1-8. doi: 10.1186/s13099-019-0341-6
![]() |
[12] |
Iacob DG (2019) Infectious threats, the intestinal barrier, and its trojan horse: dysbiosis. Front Microbiol 10: 1676. doi: 10.3389/fmicb.2019.01676
![]() |
[13] |
Lee A, Griffiths TA, Parab RS, et al. (2011) Association of Mycobacterium avium subspecies paratuberculosis with Crohn disease in pediatric patients. J Pediatr Gastroenterol Nutr 52: 170-174. doi: 10.1097/MPG.0b013e3181ef37ba
![]() |
[14] | Renouf MJ, Cho YH, McPhee JB (2018) Emergent behavior of IBD-associated Escherichia coli during disease. Inflammatory Bowel Dis 387: 96-12. |
[15] |
Agrawal G, Clancy A, Huynh R, et al. (2020) Profound remission in Crohn's disease requiring no further treatment for 3–23 years: a case series. Gut Pathog 12: 16. doi: 10.1186/s13099-020-00355-8
![]() |
[16] |
Waddell L, Rajić A, Stärk K, et al. (2016) Mycobacterium avium ssp. paratuberculosis detection in animals, food, water and other sources or vehicles of human exposure: A scoping review of the existing evidence. Prev Vet Med 132: 32-48. doi: 10.1016/j.prevetmed.2016.08.003
![]() |
[17] |
Pierce ES (2018) Where are all the Mycobacterium avium subspecies paratuberculosis in patients with Crohn's disease? Plos Pathog 5: e1000234. doi: 10.1371/journal.ppat.1000234
![]() |
[18] |
Falkinham JO (2003) Mycobacterial aerosols and respiratory disease. Emerg Infect Dis 9: 763-767. doi: 10.3201/eid0907.020415
![]() |
[19] |
Angenent LT, Kelley ST, Amand ASt, et al. (2005) Molecular identification of potential pathogens in water and air of a hospital therapy pool. P Natl Acad Sci Usa 102: 4860-4865. doi: 10.1073/pnas.0501235102
![]() |
[20] |
Falkinham J (2018) Mycobacterium avium complex: adherence as a way of life. AIMS Microbiol 4: 428-438. doi: 10.3934/microbiol.2018.3.428
![]() |
[21] |
Pickup RW, Rhodes G, Bull TJ, et al. (2006) Mycobacterium avium subsp. paratuberculosis in lake catchments, in river water abstracted for domestic use, and in effluent from domestic sewage treatment works: diverse opportunities for environmental cycling and human exposure. Appl Environ Microb 72: 4067-4077. doi: 10.1128/AEM.02490-05
![]() |
[22] |
Samba-Louaka A, Robino E, Cochard T, et al. (2018) Environmental Mycobacterium avium subsp. paratuberculosis hosted by free-living amoebae. Front Cell Infect Microbiol 8: 28. doi: 10.3389/fcimb.2018.00028
![]() |
[23] |
Azevedo LF, Magro F, Portela F, et al. (2010) Estimating the prevalence of inflammatory bowel disease in Portugal using a pharmaco-epidemiological approach. Pharmacoepidemiol Drug Saf 19: 499-510. doi: 10.1002/pds.1930
![]() |
[24] |
Nazareth N, Magro F, Machado E, et al. (2015) Prevalence of Mycobacterium avium subsp. paratuberculosis and Escherichia coli in blood samples from patients with inflammatory bowel disease. Med Microbiol Immunol 204: 681-692. doi: 10.1007/s00430-015-0420-3
![]() |
[25] |
Pickup RW, Rhodes G, Arnott S, et al. (2005) Mycobacterium avium subsp. paratuberculosis in the catchment area and water of the River Taff in South Wales, United Kingdom, and its potential relationship to clustering of Crohn's disease cases in the city of Cardiff. Appl Environ Microbiol 71: 2130-2139. doi: 10.1128/AEM.71.4.2130-2139.2005
![]() |
[26] | Rhodes G, Henrys P, Thomson BC, et al. (2013) Mycobacterium avium subspecies paratuberculosis is widely distributed in British soils and waters: implications for animal and human health. Environ Microbiol 15: 2761-2774. |
[27] |
Cunha MV, Rosalino LM, Leão C, et al. (2020) Ecological drivers of Mycobacterium avium subsp. paratuberculosis detection in mongoose (Herpestes ichneumon) using IS900 as proxy. Sci Rep-uk 10: 860. doi: 10.1038/s41598-020-57679-3
![]() |
[28] |
Beumer A, King D, Donohue M, et al. (2010) Detection of Mycobacterium avium subsp. paratuberculosis in drinking water and biofilms by quantitative PCR. Appl Environl Microbiol 76: 7367-7370. doi: 10.1128/AEM.00730-10
![]() |
[29] |
Bull TJ, McMinn EJ, Sidi-Boumedine K, et al. (2003) Detection and verification of Mycobacterium avium subsp. paratuberculosis in fresh ileocolonic mucosal biopsy specimens from individuals with and without Crohn's disease. J Clin Microbiol 41: 2915-2923. doi: 10.1128/JCM.41.7.2915-2923.2003
![]() |
[30] |
Aboagye G, Rowe MT (2011) Occurrence of Mycobacterium avium subsp. paratuberculosis in raw water and water treatment operations for the production of potable water. Water Res 45: 3271-3278. doi: 10.1016/j.watres.2011.03.029
![]() |
[31] |
Taylor RH, Falkinham JO, Norton CD, et al. (2000) Chlorine, chloramine, chlorine dioxide, and ozone susceptibility of Mycobacterium avium. Appl Environ Microb 66: 1702-1705. doi: 10.1128/AEM.66.4.1702-1705.2000
![]() |
[32] |
Esteban J, García-Coca M (2018) Mycobacterium biofilms. Front Microbiol 8: 414-418. doi: 10.3389/fmicb.2017.02651
![]() |
[33] |
Pistone D, Marone P, Pajoro M, et al. (2012) Mycobacterium avium paratuberculosis in Italy: commensal or emerging human pathogen? Digest Liver Dis 44: 461-465. doi: 10.1016/j.dld.2011.12.022
![]() |
[34] |
Coelho AC, Pinto ML, Silva S, et al. (2007) Seroprevalence of ovine paratuberculosis infection in the Northeast of Portugal. Small Ruminant Res 71: 298-303. doi: 10.1016/j.smallrumres.2006.07.009
![]() |
[35] | Leão C, Amaro A, Santos-Sanches I, et al. (2015) Paratuberculosis asymptomatic cattle as plillovers of Mycobacterium avium subsp. paratuberculosis: consequences for disease control. Rev Port Cien Vet 110: 69-73. |