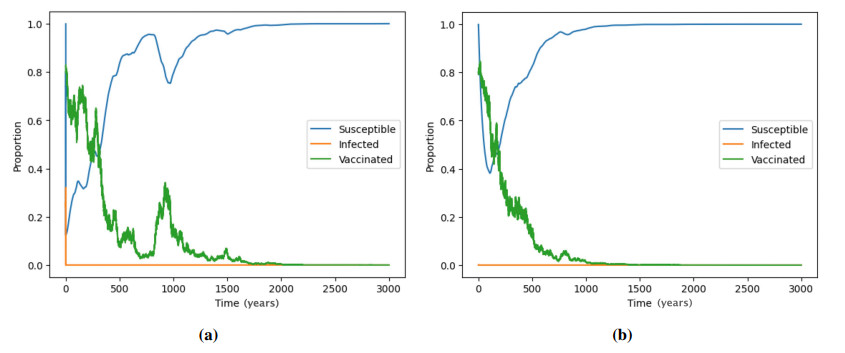
Vaccination is an effective strategy to prevent the spread of diseases. However, hesitancy and rejection of vaccines, particularly in childhood immunizations, pose challenges to vaccination efforts. In that case, according to rational decision-making and classical utility theory, parents weigh the costs of vaccination against the costs of not vaccinating their children. Social norms influence these parental decision-making outcomes, deviating their decisions from rationality. Additionally, variability in values of utilities stemming from stochasticity in parents' perceptions over time can lead to further deviations from rationality. In this paper, we employ independent white noises to represent stochastic fluctuations in parental perceptions of utility functions of the decisions over time, as well as in the disease transmission rates. This approach leads to a system of stochastic differential Eqs of a susceptible-infected-recovered (SIR) model coupled with a stochastic replicator Eq. We explore the dynamics of these Eqs and identify new behaviors emerging from stochastic influences. Interestingly, incorporating stochasticity into the utility functions for vaccination and nonvaccination leads to a decision-making model that reflects the bounded rationality of humans. Noise, like social norms, is a two-sided sword that depends on the degree of bounded rationality of each group. We also perform a stochastic optimal control as a discount to the cost of vaccination to counteract bounded rationality.
Citation: Andras Balogh, Tamer Oraby. Stochastic games of parental vaccination decision making and bounded rationality[J]. Mathematical Biosciences and Engineering, 2025, 22(2): 355-388. doi: 10.3934/mbe.2025014
[1] | Jianjun Long, Hui Huang . Stability of equilibrium production-price in a dynamic duopoly Cournot-Bertrand game with asymmetric information and cluster spillovers. Mathematical Biosciences and Engineering, 2022, 19(12): 14056-14073. doi: 10.3934/mbe.2022654 |
[2] | Bruno Buonomo, Alberto d’Onofrio, Deborah Lacitignola . Rational exemption to vaccination for non-fatal SIS diseases: Globally stable and oscillatory endemicity. Mathematical Biosciences and Engineering, 2010, 7(3): 561-578. doi: 10.3934/mbe.2010.7.561 |
[3] | Anthony Morciglio, R. K. P. Zia, James M. Hyman, Yi Jiang . Understanding the oscillations of an epidemic due to vaccine hesitancy. Mathematical Biosciences and Engineering, 2024, 21(8): 6829-6846. doi: 10.3934/mbe.2024299 |
[4] | Linda J. S. Allen, P. van den Driessche . Stochastic epidemic models with a backward bifurcation. Mathematical Biosciences and Engineering, 2006, 3(3): 445-458. doi: 10.3934/mbe.2006.3.445 |
[5] | Wei Wu, Zhiyi Lin, Ming Wei . Research on a collaborative evolution model of multi-airport route network considering subsidy strategies. Mathematical Biosciences and Engineering, 2023, 20(11): 19808-19838. doi: 10.3934/mbe.2023877 |
[6] | Tingting Xue, Long Zhang, Xiaolin Fan . Dynamic modeling and analysis of Hepatitis B epidemic with general incidence. Mathematical Biosciences and Engineering, 2023, 20(6): 10883-10908. doi: 10.3934/mbe.2023483 |
[7] | Natalie M. Linton, Sung-mok Jung, Hiroshi Nishiura . Not all fun and games: Potential incidence of SARS-CoV-2 infections during the Tokyo 2020 Olympic Games. Mathematical Biosciences and Engineering, 2021, 18(6): 9685-9696. doi: 10.3934/mbe.2021474 |
[8] | Yue Song, Yi Zhang, Song Yang, Na Li . Investigation on stability and controller design for singular bio-economic systems with stochastic fluctuations. Mathematical Biosciences and Engineering, 2021, 18(3): 2991-3005. doi: 10.3934/mbe.2021150 |
[9] | Sheng-I Chen, Chia-Yuan Wu . A stochastic programming model of vaccine preparation and administration for seasonal influenza interventions. Mathematical Biosciences and Engineering, 2020, 17(4): 2984-2997. doi: 10.3934/mbe.2020169 |
[10] | Xinyu Bai, Shaojuan Ma . Stochastic dynamical behavior of COVID-19 model based on secondary vaccination. Mathematical Biosciences and Engineering, 2023, 20(2): 2980-2997. doi: 10.3934/mbe.2023141 |
Vaccination is an effective strategy to prevent the spread of diseases. However, hesitancy and rejection of vaccines, particularly in childhood immunizations, pose challenges to vaccination efforts. In that case, according to rational decision-making and classical utility theory, parents weigh the costs of vaccination against the costs of not vaccinating their children. Social norms influence these parental decision-making outcomes, deviating their decisions from rationality. Additionally, variability in values of utilities stemming from stochasticity in parents' perceptions over time can lead to further deviations from rationality. In this paper, we employ independent white noises to represent stochastic fluctuations in parental perceptions of utility functions of the decisions over time, as well as in the disease transmission rates. This approach leads to a system of stochastic differential Eqs of a susceptible-infected-recovered (SIR) model coupled with a stochastic replicator Eq. We explore the dynamics of these Eqs and identify new behaviors emerging from stochastic influences. Interestingly, incorporating stochasticity into the utility functions for vaccination and nonvaccination leads to a decision-making model that reflects the bounded rationality of humans. Noise, like social norms, is a two-sided sword that depends on the degree of bounded rationality of each group. We also perform a stochastic optimal control as a discount to the cost of vaccination to counteract bounded rationality.
In disease modeling, applying game theory to understand human behavior about mitigation choices offers crucial insights into the dynamics of epidemics. Conventional methods often presuppose rational decision-making; however, as the COVID-19 pandemic has demonstrated, human behavior can sometimes hinder public health initiatives to control the spread of the disease. Individual reactions to pharmaceutical interventions such as vaccination and non-pharmaceutical measures, including mask use, are shaped by a complex mixture of factors that include social norms, misinformation, and trust in authorities [1].
Moreover, integrating concepts from behavioral economics and psychology, such as bounded rationality and prospect theory, adds realism to these models. These theories recognize that individuals do not always process information flawlessly or make decisions based solely on maximum utility [2]. Instead, decisions are influenced by limited cognitive resources, biases, and how information is framed.
Kermack and McKendrick (1927) initially laid the groundwork for deterministic models of epidemics, which have since evolved to include stochastic components [3]. In [4], a pioneer in stochastic disease modeling was introduced using stochastic processes like birth-and-death processes. In that study, the authors introduced stochastic elements into mathematical epidemiology, making it possible to model the inherent randomness of disease transmission and study extinction thresholds to better understand and predict the dynamics of infectious diseases. Several researchers have studied stochastic models of the spread of diseases in the form of stochastic differential Eqs (SDEs). SDEs incorporate randomness directly into the model, representing various sources of uncertainty and variability in disease transmission and progression. These models have been particularly beneficial in capturing the random nature of contact patterns and the influence of random events on the spread of infectious diseases. Incorporating SDEs into epidemic modeling provides a more comprehensive understanding of how diseases spread and how they can be controlled under uncertainty.
Allen (1994) provided foundational work on stochastic epidemic models, demonstrating how SDEs can model demographic stochasticity and environmental variability in disease dynamics [5]. Gray et al. (2011) extended this approach to the SIS model, exploring the existence of a stationary distribution and derived expressions for its mean and variance [6]. Tornatore et al. (2005) investigated the stability of a stochastic SIR system, providing insights into the conditions under which disease extinction or persistence occurs [7]. Furthermore, Gao et al. (2019) studied a delay differential Eq SIS model with a general non-linear incidence rate, highlighting the impact of delays and non-linearities in disease transmission [8]. Cao et al. (2017) focused on the dynamics of an epidemic model with vaccination in a noisy environment, emphasizing the role of stochastic effects in vaccination strategies [9]. Liu (2023) modeled multiple transmission pathways in a scenario of waterborne pathogens using a stochastic model, illustrating the complexity and variability in disease transmission routes [10]. See also [11] for an introduction to stochastic epidemic models.
Researchers have continued to advance the field by exploring the effects of multiple noise sources on infectious disease models. For example, Jian et al. (2024) examined the impact of multisource noise on disease dynamics, offering insights into how different environmental randomness can influence disease spread [12]. Babaei et al. (2023) applied SDEs to model the COVID-19 pandemic, integrating stochastic elements into SEIAQHR models to capture the variability and uncertainty of real-world scenarios [13]. Similarly, Iddrisu et al. (2023) highlighted the critical role of stochasticity in understanding cholera epidemiology, demonstrating how SDEs can provide more accurate predictions and inform public health interventions [14]. These contributions underscore the importance of stochastic models in epidemiology, as they provide more realistic and flexible frameworks for predicting disease outcomes and formulating effective public health policies.
Rational decision-making theories often disregard information processing constraints, presuming that rational decision-makers always opt for the highest rewards without factoring in the effort or resources needed to determine the best action [15,16,17]. H.A. Simon's bounded rationality theory addresses these deviations, suggesting that decision-makers operate under limited information processing resources [18,19]. Behavioral economics experiments reveal deviations between perfectly rational decision-makers and those constrained by bounded rationality [20].
Behavioral epidemiology has evolved in the last two decades to become a major domain in the research of infectious diseases. The intricate interplay between human behavior and epidemiological outcomes highlights this. Foundational contributions to the literature of behavioral epidemiology, such as by [21,22,23,24,25], have shaped the understanding of the impact of behavioral responses to disease outbreaks and their control. In particular, it gave insight into how parental vaccination decisions affect childhood disease dynamics and public health interventions, especially with vaccine scare [26,27,28]. Free-riding behaviors [29], social networks [30], social learning [31], and economic incentives [32], to name a few factors, have been shown to impact vaccination uptake and thus disease spread. Those research findings emphasize the importance of integrating behavioral and epidemiological modeling approaches to address challenges to disease control [25]. They demonstrate the complex interplay of behavioral, social, cognitive, and economic factors in human behavior in general and vaccine decision-making, in particular, which form the basis for this research.
We seek to add a building block to behavioral epidemiology advances regarding factors influencing parental vaccination decisions, particularly rationality in the decision-making process. We extend the work in [28], where the authors emphasized integrating social factors into behavioral responses and infectious disease models, and [27], where the authors explored the use of prospect theory to enhance replicator dynamics in vaccination decision making. Here, we aim to build on these models by focusing on bounded rationality in decision-making resulting from stochasticity in the perception of the payoff of vaccination, leading to a mutation term in the replicator dynamics. Our results contribute to understanding parental behavior towards childhood immunization by showing how collective deviations in those perceptions can make disease persist. We also found that the consequences of these deviations could be counteracted using an optimal temporal discount in the cost of vaccination.
In this paper, we derive a stochastic differential Eq of RD for vaccination [33]. The stochasticity arises from the perception of the utilities of the choices and deviations from rationality. Using stochastic game theory to model vaccine choice represents a significant step forward in capturing this complexity. In this framework, a collective decision to vaccinate is influenced not only by personal perceptions of risk-benefit but also by the choices made by others in their community and external but common environmental, cultural, informational, and economic factors. These shared factors are essential for understanding collective dynamics and population-level responses under systemic uncertainty. This interdependence of decisions, along with the inherent variability in the way individuals assess risks and benefits under uncertainty, can also be effectively modeled as a stochastic game in which the outcomes depend randomly on the actions of all participants [28,34,35]. To our knowledge, we are the first to introduce and study a stochastic behavioral game theory model in the disease modeling literature.
The paper is summarized as follows. In Section 2, we derive a new stochastic behavioral game theory Eq that models vaccination versus non-vaccination choices. We pair that Eq with a stochastic SIR model in which the transmission rate also evolves randomly. This will be followed in Section 3 by an analytical stability analysis of the model's equilibria. We also use stochastic simulations of stochastic differential Eqs to further study the stability of the model equilibria and corroborate some of the analytical results. Additionally, we use stochastic optimal control of the cost of vaccination to counteract the effect of bounded rationality. We follow that section with a discussion and conclusion section.
Let (Ω,F,{Ft}t≥0,P) be a complete probability space, with filtration {Ft}t≥0, with respect to which all Brownian motions Bi and Wi introduced in the following are defined. We use a stochastic replicator Eq following the methods of establishing a stochastic replicator Eq in [36,37] to guarantee the feasibility of solutions of the stochastic RD Eq. The stochastic RD Eq models the evolution over time of strategies or traits in a population by incorporating random fluctuations due to environmental variability or when the population is finite. For instance, let a population have two strategies or traits, A and B, like vaccination and non-vaccination strategies. If strategy A has a higher payoff than B, then the RD Eq's deterministic component would imply that the adopters of strategy A must increase. However, the stochastic component could cause temporary increases in the adopters of strategy B, while strategy A is more gainful and vice versa.
Let X(t)=(X1(t),X2(t)) be the number of vaccinators and non-vaccinators in a community of a total size of N:=X1+X2 decision makers and let the fractions be denoted by xi=Xi/N. Since x1+x2=1, we will also use the lower case x to denote x1 whenever it does not cause confusion. Let ui(x1,x2)=∑2j=1v(si,sj)xj be the expected payoff for a decision maker adopting strategy si if randomly and uniformly matched with a decision maker adopting strategy sj. The function v(si,sj) is the payoff for a decision maker who adopts strategy si interacting with a decision maker adopting strategy sj.
A deviation from rationality due to external and internal factors is modeled here by adding a white noise term to the payoff v(si,sj) giving a new payoff function v(si,sj)+νi˙Bi(t). The stochastic process ˙B(t)=(˙B1(t),˙B2(t)) is a two-dimensional white noise. Volatility νi>0 is the magnitude of the noise in the perceived utility. We assume here that all vaccinators and all non-vaccinators share the same volatility ν1 and ν2, respectively. Maintaining collective population dynamics, the stochastic RD model postulates that stochastic noise affects payoff perceptions uniformly across adopters of the same strategy. This does not account for individual variability in the perceived payoffs. That assumption reflects the influence of common external factors on risk perceptions, aligning with psychological theories of collective decision-making under uncertainty.
The following stochastic differential Eq then gives the replicator Eq modeling behavioral dynamics,
dXi(t)dt=κXi(t)(ui(x1,x2)+νi˙Bi(t)), | (2.1) |
or
dXi(t)=κXi(t)ui(x1,x2)dt+κνiXi(t)dBi(t), | (2.2) |
where κ>0 is the replication rate. Applying Itô's formula (see Lemma S2 in the Supplementary) to the transformation x1=X1/N, we get
dx1=κx1x2(v(s1,s2)−v(s2,s1)+κν22x2−κν21x1)dt+κx1x2(ν1dB1(t)−ν2dB2(t)), | (2.3) |
Since ν1dB1(t)−ν2dB2(t) has the same distribution as √ν21+ν22dW(t), where dW(t) is also Gaussian with mean zero and variance dt, then Eq (2.3) is equivalent to:
dx1=κx1x2(v(s1,s2)−v(s2,s1)+κν22x2−κν21x1)dt+κx1x2√ν21+ν22dW(t), | (2.4) |
Eq (2.4) guarantees that 0≤x1(t)≤1 for all t>0 if 0≤x1(0)≤1.
We model the payoffs of vaccination to be v(s1,s2)=−ω+δx1 and of vaccination to be v(s2,s1)=−I+δx2, see [28]. In terms of the vaccination choice model, κ is the social learning rate, ω is the cost of vaccination, and δ is the group pressure. Social norms of vaccination and non-vaccination enforced by vaccinators and non-vaccinators add δx1 and δx2 to the payoffs of vaccination and non-vaccination strategies, respectively.
The noise in perceived payoff results in the introduction of a new mutation term κ2x1x2(ν22x2−ν21x1) to replicator dynamics; see, e.g., [36,38]. A mutation term induces irrational choices of a strategy that might not be optimal. In terms of vaccination, for certain large values of ν1, the mutation term is a bounded rational term that can cause agents to adopt the non-vaccination strategy while it has a smaller payoff; that is v(s2,s1)<v(s1,s2).
The transmission dynamics are assumed to follow a susceptible-infected-recovered (SIR) compartmental model. Putting x1=x and x2=1−x in Eq (2.4) and coupling it with the SIR model yields the system of Eqs:
dSdt=μ(1−x)−βSI−μS−σ1SI˙W1dIdt=βSI−(μ+γ)I+σ1SI˙W1dRdt=μx+γI−μRdxdt=κx(1−x)[−ω+I+δ(2x−1)+κ(σ23−(σ22+σ23)x)+√σ22+σ23˙W2], | (2.5) |
where S, I, and R are the proportion of susceptible, infected, and recovered individuals in the population at time t, such that S+I+R=1. The parameter μ is the per capita birth/death rate, β is the transmission rate, γ is the recovery rate. Recall that κ is the social learning rate, ω is the cost of vaccination, and δ is the group pressure. The term μ(1−x) is the recruitment rate of non-vaccinated children to the susceptible compartment due to parental choice.
Let σ1 be the magnitude of noise in disease transmission. The last Eq in (2.5), a stochastic replicator Eq, follows the replicator model introduced by [37], where it is shown to have solutions x between zero and one; see above. Notice that we changed the notation ν1 to σ2 and ν2 to σ3, to be in order within the coupled model. If σ22=σ23=δκ then, δ(2x−1)+κ(σ23−(σ22+σ23)x)=0. In this case, the collective noise would cancel out the group pressure in the stochastic replicator Eq. Hence, it seems that bounded rationality could nullify or counteract social norms.
Since S+I+R=1, then the third Eq of R in Eq (2.5) is redundant, so henceforth we will consider the model (2.5) without it and stop using the recovered compartment. Define the set of solutions for the model in Eq (2.5) as S={(s,i,x)∈R3+:0≤s+i≤1 and 0≤x≤1}. In the following subsections, let (S(t),I(t),x(t)) be a solution of the model (2.5) with initial state (S(0),I(0),x(0))∈S. The proofs of existence, uniqueness, and boundedness of the solution of the model (2.5) are given in S1.2.
In this section, we discuss the different equilibria of the model in (2.5) and their stability. We note that some specific states of the three stochastic processes S, I, and x are absorbing states. The states 0 and 1 are absorbing states for x and 0 is an absorbing state for I.
We will support the results in this section with simulations using the Milstein algorithm for the numerical simulation of SDEs [39] implemented using Python. We will use parameter values from [28], such as μ=1/50 year−1, γ=365/22 year−1, and κ=1.69 year−1. We have to mention here that most of the simulated processes exhibit slow mixing rates, and hence they take a very long time to converge to equilibrium.
The model has five equilibria of the drift term (the deterministic part) in Eq (2.5). Those five equilibria and their existence conditions are found to be those of [28] when σ21=σ22=σ23=0. Three of those are disease-free equilibria:
1. full vaccine uptake and no susceptibility, E1≡(S1,I1,x1)=(0,0,1),
2. no vaccine uptake and full susceptibility, E2=(1,0,0), and
3. partial vaccine uptake and partial susceptibility, E3≡(1−x3,0,x3), where
x3=κσ23−δ−ωκσ22+κσ23−2δ., | (3.1) |
The equilibrium E3 exists in two regions:
R3,1={(δ,ω)∈R2+:δ−κσ22<ω<κσ23−δ} |
and
R3,2={(δ,ω)∈R2+:κσ23−δ<ω<δ−κσ22}. |
The two boundaries of either region intersect at (κ2(σ22+σ23),κ2(σ23−σ22)).
The other two are endemic equilibria that exist when the basic reproduction number R0=βμ+γ>1.
They are:
1. no vaccine coverage, E4≡(1R0,μμ+γ(1−1R0),0), and
2. partial vaccine coverage, E5≡(1R0,μμ+γ(1−1R0−x5),x5), where
x5=μ(1−1R0)+(κσ23−δ−ω)(μ+γ)μ+(κσ22+κσ23−2δ)(μ+γ). |
The equilibrium E5 exists in two regions
R5,1={(δ,ω)∈R2+:−κσ22+1R0κ(σ22+σ23)+δ(1−2R0)<ω<−δ+μμ+γ(1−1R0)+κσ23} |
and
R5,2={(δ,ω)∈R2+:−κσ22+1R0κ(σ22+σ23)+δ(1−2R0)>ω>−δ+μμ+γ(1−1R0)+κσ23} |
The two boundaries of either region intersect at (12μμ+γ+κ2(σ22+σ23),12μμ+γ(1−2R0)+κ2(σ23−σ22)).
The disease-endemic equilibria appear in the next stability analyses as types of behavior and not as exact values. The equilibria E3 and E5 represent the partial acceptance of the vaccine. However, in the latter, partial acceptance of the vaccine does not lead to the eradication of the disease.
Define the mean-value function ¯Y(T) of any function Y(t) over [0,T] to be
¯Y(T)=1T∫T0Y(t)dt |
and let its limit be defined as
Y0=limT→∞¯Y(T). |
Let Y∗=lim inft→∞Y(t) and Y∗=lim supt→∞Y(t).
Next, we define modes of stability, such as exponential stability, and introduce a new mode of stability that we call logistic stability. Logistic stability helps in studying the impermanence of dynamic processes that take value in the unit interval [0,1]. To our knowledge, this concept has not been used before in dynamical system analyses.
Definition 1 (Almost Sure Exponential Stability). We say that a process Y(t)∈[0,∞) has an almost sure exponentially stable equilibrium at y=0 if for some c>0,
lim supt→∞1tlogY(t)≤−c |
almost surely.
Definition 2 (Almost Sure Logistic Stability). We say that a process Y(t)∈[0,1] has an almost sure logistically stable equilibrium at y=0 and 1 if for some c>0,
limt→∞1tlogY(t)1−Y(t)=(−1)y+1c |
almost surely.
The unit interval represents a natural domain for many real-life phenomena, such as proportions, probabilities, or normalized populations constrained to the unit interval [0,1]. The concept of logistic stability serves the need to characterize the transient dynamics of those types of processes as well as their behavior in convergence towards stability. Other convergence behaviors like exponential or asymptotic stability provide some types of convergence to equilibria. Those types may not capture other systems where the dynamics of the processes are bound and/or demonstrate logistic-like growth or decay which tend to be slower than the exponential ones. In infectious disease modeling, logistic stability could capture the impermanence of disease states within the unit interval, like prevalence, when epidemics go through transient phenomena. Logistic stability could distinguish those transient dynamics from other forms, emphasizing steady-state behavior. The limiting behavior of 1tlogY(t)1−Y(t) can quantify the rate at which a disease dies out or persists, providing a new metric for control measures.
Define the stochastic basic reproduction number
Rs0:=βμ+γ+12σ21. | (3.2) |
which is always smaller than or equal to R0.
First, we will study the global stability of a disease-free equilibrium. There are several cases in which the disease-free equilibrium is stable. The first case depends on the stochastic basic reproduction number and the magnitude of the noise in transmission.
Theorem 1. If Rs0<1 and σ21≤β or if σ21β>max(1,R02), then a disease-free equilibrium is exponentially globally stable almost surely.
Proof. We will prove the theorem in two parts, by showing that the following two inequalities hold:
(C.Ⅰ) If σ21β>max(1,R02), then
lim supt→∞logI(t)t≤−β(μ+γ)σ21(σ21β−R02)<0 a.s., | (3.3) |
(C.Ⅱ) If Rs0<1 and σ21≤β, then
lim supt→∞logI(t)t≤−(μ+γ+12σ21)(1−Rs0)<0 a.s., | (3.4) |
Let V(t,I)=log(I), then by Itô's formula (see Lemma S2 in the Supplementary),
dV=dlog(I)=Vtdt+VI(βSI−(μ+γ)I)dt+12(σ1SI)2VIIdt+(σ1SI)VIdW1(t)=(βS−(μ+γ)−12σ21S2)dt+σ1SdW1(t) |
which by integration over the interval (0,t) gives
log(I(t))−log(I(0))=∫t0(βS(u)−(μ+γ)−12σ21(S(u))2)du+σ1∫t0S(u)dW1(u)=∫t0βS(u)(1−σ212βS(u))du−(μ+γ)t+M(t) |
where M(t)=σ1∫t0S(u)dW1(u) is a local continuous martingale with M(0)=0. Since
lim supt→∞E(M(t)2)t=lim supt→∞σ21∫t0(S(u))2dut≤lim supt→∞σ21tt=σ21<∞, | (3.5) |
then by the Strong Law of Large Numbers, lim supt→∞M(t)t=0 almost surely, , see [40] and Lemma S3 in the Supplementary.
Now to prove (C.Ⅰ), when σ21β>max(1,R02), note that the quadratic function
f(S)=βS(1−σ212βS)=σ212S(2βσ21−S), | (3.6) |
attains its maximum value of β22σ21 when S=βσ21, which is in the interval (0,1) if σ21>β, in which case
log(I(t))−log(I(0))t=∫t0βS(u)(1−σ212βS(u))du−(μ+γ)t+M(t)t≤β22σ21−(μ+γ)+M(t)t |
and so
lim supt→∞log(I(t))−log(I(0))t≤β22σ21−(μ+γ) a.s. |
From this (C.Ⅰ) follows as
lim supt→∞log(I(t))t≤β22σ21−(μ+γ)=−β(μ+γ)σ21(σ21β−β2(μ+γ))=−β(μ+γ)σ21(σ21β−R02)<0 a.s. |
To prove (C.Ⅱ), when σ21≤β, consider the function
f(S)=βS(1−σ212βS)=σ212S(2βσ21−S), | (3.7) |
which attains a maximum value on the interval [0,1] at S=1 with maximum value
maxS∈[0,1]f(S)=β−σ212, | (3.8) |
Thus,
log(I(t))−log(I(0))t=∫t0βS(u)(1−σ212βS(u))du−(μ+γ)t+M(t)t≤β−12σ21−(μ+γ)+M(t)t |
and so
lim supt→∞log(I(t))−log(I(0))t≤β−12σ21−(μ+γ) a.s., |
From this (C.Ⅱ) follows as
lim supt→∞log(I(t))t≤β−12σ21−(μ+γ)=−(μ+γ+12σ21)(1−βμ+γ+12σ21)=−(μ+γ+12σ21)(1−Rs0)<0 a.s. |
Theorem 1 seems to be a classic conclusion; see, e.g., [41]; but it is shown here to hold even with the presence of a stochastic process x(t) in the first Eq. It shows that disease extinction could still be achieved here even when R0>1 as long as Rs0<1, see Figure 1(a). Another case in which the disease could be eradicated while R0>1 is when σ21β>max(1,R02) as shown in Figure 1(b). Even when Rs0>1; or specifically, when the conditions of Theorem 1 are not satisfied, eradication of the disease is possible through vaccination, as we will see next.
In [28], using a deterministic model, it was shown that while R0>1, the disease could be eradicated as the uptake of the vaccine increases to the level of 100% when the peer pressure of the vaccination group is greater than the cost of the vaccine (δ>ω). However, it was also shown that if the cost of vaccination and the peer pressure of the non-vaccinating group exceed the risk of the disease (ω+δ>μμ+γ(1−1R0)), parents might skip vaccination and the disease becomes endemic; see also [27]. Moreover, both equilibria were shown to be bistable. Here, bounded rationality changes those findings in a way that noise can impede the roles played by the cost of vaccination and peer pressure in [28]. The following theorem shows how noises in the perceived utilities can change the vaccination selection, and consequently the disease dynamics.
Theorem 2. If ˉI(t)t→∞→I0, then x(t) has an almost sure logistic stable equilibrium at 0 and 1 under the following conditions:
1. If ω+κσ22−σ232>max(I0−δ,δ), then x(t)t→∞→0 logistically almost surely, and
2. If ω+κσ22−σ232<min(I0−δ,δ), then x(t)t→∞→1 logistically almost surely, and also, I(t)t→∞→0 and S(t)t→∞→0 a.s. whether Rs0 is more or less than one.
Proof. Using Itô's formula (see Lemma S2 in the Supplementary),
dlog(x(t))=κ(1−x(t))[(κσ23−δ−ω)−(κσ22+κσ23−2δ)x(t)+I(t)−κσ22+σ232(1−x(t))]dt+κ√σ22+σ23(1−x(t))dW2(t) |
and similarly
dlog(1−x(t))=−κx(t)[(κσ23−δ−ω)−(κσ22+κσ23−2δ)x(t)+I(t)+κσ22+σ232x(t)]dt−κ√σ22+σ23x(t)dW2(t). |
Therefore,
dlog(x(t)1−x(t))=κ(−κσ22−σ232−δ−ω+2δx(t)+I(t))dt+κ√σ22+σ23dW2(t). |
Since W2(t)tt→∞→0 almost surely due to the Strong Law of Large Numbers of Brownian motion, then
limt→∞log(x(t)1−x(t))t=κL(x0,I0) | (3.9) |
almost surely, where
L(x0,I0):=−κσ22−σ232−δ−ω+2δx0+I0. |
If x0=0, which is equivalent to x(t) approaches 0 almost surely, then L(x0,I0)=−κσ22−σ232−ω−δ+I0. If x0=1 and I0=0, which is equivalent to x(t) approaches 1 almost surely, then L(x0,I0)=−κσ22−σ232−ω+δ. The first and second values of L(x0,I0) could have opposite signs for the same parameter values as when I0−δ<κσ22−σ232+ω<δ. Therefore, x(t) approaches 0 logistically almost surely if −κσ22−σ232−ω−δ+I0<0 and −κσ22−σ232−ω+δ<0. Meanwhile, x(t) approaches 1 logistically almost surely if −κσ22−σ232−ω+δ>0 and −κσ22−σ232−ω−δ+I0>0.
An overlap region appears in Figure 2(a) as stated in the proof of Theorem 2 when I0−δ<κσ22−σ232+ω<δ. In that region, both equilibria could be stable, as the simulation demonstrates below. The dashed region with vertical lines in Figure 2(a) is for ω+κσ22−σ232>I0−δ in which the disease-endemic and no-vaccination equilibrium E4-type equilibrium is stable; see Figure 2(b) for a simulation of a case in that region. Meanwhile, the dashed region with diagonal lines in Figure 2(a) is for ω+κσ22−σ232<δ, in which disease-free and full-vaccination equilibrium E1 is stable; see Figure 2(c) for a simulation of a case in that region. Again, in the common region, both E1 and E4 are stable, see below.
When σ22=σ23=0, the two conditions of Theorem 2 are shown to be sufficient for the local asymptotic stability of E4 and E1, respectively, for the deterministic model in [28]. In particular, the latter equilibrium of the disease-free state was shown to be stable when δ>ω. However, the magnitudes of the white noise in the utilities of both groups, σ22 and σ23, influence the opinion and uptake of vaccination. In Figure 2(b), the disease cannot be eradicated even when δ>ω is mainly due to the level of noise in the vaccinator group. Figure 2(c) represents a case where the conditions of Theorem 1 are not satisfied, but disease eradication is still possible as implied by part 2 of Theorem 2. If Rs0>1, the disease could be eradicated by increasing the peer pressure of the vaccination group or decreasing the noise in the utility of the vaccinator's group conducing to ω+κσ22−σ232<δ. Notice also that an increase in the noise of the non-vaccinator group could also increase vaccine uptake.
Achieving the goal of disease eradication in the vaccination campaign requires minimizing variability in decision-making and promoting consistent, rational choices throughout the population. However, that aim might not be plausible due to external factors. The noise in the perceived utility of the vaccination group could increase further, exacerbating disease eradication. When the social and stochastic external factors that influence utility functions are outside of the control of the policymaker, a policy to change the cost of vaccination is warranted. A stochastic optimal control approach (see e.g., [42]) could provide a policy for preventing infectious diseases by finding the appropriate cost of vaccination. As such, let the control variable 0<u(t)≤1 be the degree of reduction in the cost of vaccination. Including that function in the stochastic replicator Eq gives rise to the following Eq
dxdt=κx(1−x)[−ω(1−u)+I+δ(2x−1)+κ(σ23−(σ22+σ23)x)+√σ22+σ23˙W2(t)], | (3.10) |
The details of the stochastic optimal control are given in S1.3. Figure 3(a) provides a simulation in which E4 is stable. Full uptake of the vaccine and disease eradication is achieved, see Figure 3(b), with an optimal control u∗(t) that reduces the cost of vaccination, see Figure 3(c). We note that the optimal reduction started to decline smoothly after being a constant over a relatively short period of time.
It would be difficult to control the disease without that discount in the cost of vaccination, especially with large noise in the vaccination group's utility function. The reason is in two scenarios: the first scenario is when ω+κσ22−σ232>I0−δ and ω+κσ22−σ232>δ, in which vaccination is completely rejected and the disease becomes endemic; see Figure 2(b). The second scenario is where ω+κσ22−σ232>I0−δ and ω+κσ22−σ232<δ, in which case both disease eradication and disease endemicity become stationary; see Figure 4. In Figure 4(a) the disease dies, while in Figure 4(b) the disease persists while all the values of the parameters and initial conditions are the same.
As shown in Figure 4, when both parts of Theorem 2 are true, namely ω+κσ22−σ232>I0−δ and ω+κσ22−σ232<δ, then the bistability of both equilibria x=0 and x=1 (impermanence) occurs. A further examination of the stability of both equilibria was performed using a simulation study. Figure 5 shows the proportion of times x(t) converges to 0 and to 1 in 200 stochastic simulations of the model at different values of σ22 and σ23 with initial values x(0)=0.1,0.2,…,0.9. The bistability region shows a Bernoulli stationary distribution whose probabilities depend on the noises in the perceived utilities σ22 and σ23, as well as the initial acceptance of vaccination x(0). The size of the bistability region could be seen to depend on x(0) as shown through the panels of Figure 5. We notice that as initial acceptance of the vaccine x(0) increases, the region in which full vaccination is stable in the σ22−σ23 plane expands. In other words, noises are more detrimental to vaccination campaigns if initial vaccine acceptance is low.
The following two theorems are generalizations to the disease dynamics in [28]. The following theorem identifies the boundaries of the limiting temporal means of processes when Rs0>1. It also shows the case when the disease cannot be eradicated at a low vaccination level; namely when its temporal mean of vaccine acceptance/uptake is below 1−1Rs0.
Theorem 3. If Rs0>1 and ˉx(t)→x0, then a disease-endemic equilibrium will persist in the temporal mean. That is
1. For all x0∈[0,1],
μ(1−x0)μ+β≤lim inft→∞ˉS(t)≤lim supt→∞ˉS(t)≤1−x0 | (3.11) |
and
2. If x0<1−1Rs0, then
lim inft→∞ˉI(t)≥μμ+γ[1−1Rs0−x0] | (3.12) |
and
lim supt→∞ˉS(t)≤1Rs0. | (3.13) |
Eq (3.13) holds true also when x0≥1−1Rs0 from the upper inequality in Part (1).
3. If x0<ββ−σ21(1−1Rs0) and β>σ21 then
lim supt→∞ˉI(t)≤μμ+γ[ββ−σ21(1−1Rs0)−x0]. | (3.14) |
Proof. Since
d(S+I)(t)dt=μ(1−x(t))−μ(S+I)(t)−γI(t) |
then
ˉS(t)=1−ϕ(t)−ˉx(t)−(1+γμ)ˉI(t) | (3.15) |
where ϕ(t)=1μt(S(t)+I(t)−S(0)−I(0)). Notice that limt→∞ϕ(t)=0 a.s. Since, ˉS(t)≤1−ϕ(t)−ˉx(t) for all t>0. Therefore,
lim supt→∞ˉS(t)≤1−x0. |
From Eq (2.5)
dSdt=μ(1−x)−βSI−μS−σ1SI˙W1≥μ(1−x)−(β+μ)S−σ1SI˙W1. |
Then,
S(t)−S(0)t≥μ(1−ˉx(t))−(β+μ)ˉS(t)−1tM3(t), |
where M3(t)=∫t0σ1S(u)I(u)dW1(u). Notice that M3(t) is a local continuous martingale with M3(0)=0 and E(M3(t)2)=σ21∫t0(S(u)I(u))2du≤σ21t. Since lim supt→∞E(M3(t)2)t≤σ21<∞, then by the Strong Law of Large Numbers, lim supt→∞M3(t)t=0 almost surely, see [40] and Lemma S3 in the Supplementary. But since x0<1, and S(t)−S(0)≤1 then (3.11) follows.
To prove part (2), note that
dlog(I(t))dt=βS(t)−(μ+γ+12σ21)+12σ21(1−S(t)2)+σ1S˙W1≥βS(t)−(μ+γ+12σ21)+σ1S˙W1 |
Thus
log(I(t))−log(I(0))t≥β[1−ϕ(t)−ˉx(t)−(1+γμ)ˉI(t)]−(μ+γ+12σ21)+1tM(t) |
and so
log(I(t))t≥log(I(0))t+β[1−ϕ(t)−ˉx(t)−(1+γμ)ˉI(t)]−(μ+γ+12σ21)+1tM(t). |
Thus by Lemma S4 in the Supplementary,
lim inft→∞ˉI(t)≥μμ+γ[1−1Rs0−x0] |
if x0<1−1Rs0. In which case, since
ˉS(t)=1−ϕ(t)−ˉx(t)−(1+γμ)ˉI(t). |
Then
lim supt→∞ˉS(t)≤1Rs0 |
Notice that 1Rs0>1R0 so it is more than its deterministic counterpart.
To prove part (3), similarly, if x0<ββ−σ21(1−1Rs0) and β>σ21 then
lim inft→∞ˉS(t)≥1−ββ−σ21(1−1Rs0) |
Again,
dlog(I(t))=(βS−(μ+γ)−12σ21S2)dt+σ1SdW1(t) |
and by Eq (3.15)
1tlog(I(t))−1tlog(I(0))=βˉS(t)−(μ+γ)−12σ21¯S2(t)+1tM(t) |
where M(t)=σ1∫t0S(u)dW1(u) is a local continuous martingale, with M(0)=0, see [40] and Lemma S3 in the Supplementary. Since, (ˉS(t))2≤¯S2(t), then
1tlog(I(t))≤1tlog(I(0))+β[1−ϕ(t)−ˉx(t)−(1+γμ)ˉI(t)]−(μ+γ)−12σ21[1−ϕ(t)−ˉx(t)−(1+γμ)ˉI(t)]2+1tM(t)≤Ψ(t)+β[1−ˉx(t)]−(μ+γ)−β(1+γμ)ˉI(t)−12σ21(1−ϕ(t))2+σ21(ˉx(t)+(1+γμ)ˉI(t))−12σ21(ˉx(t)+(1+γμ)ˉI(t))2≤Ξ(t)−(β−σ21)(1+γμ)ˉI(t) |
where
Ξ(t)=Ψ(t)+β[1−ˉx(t)]−(μ+γ)−12σ21(1−ϕ(t))2+σ21ˉx(t), |
and
Ψ(t)=1tlog(I(0))−βϕ(t)−σ21ϕ(t)(ˉx(t)+(1+γμ)ˉI(t))+1tM(t). |
If we show that limt→∞Ξ(t)=β(1−1Rs0)−(β−σ21)x0 a.s., then Lemma S4 in the Supplementary will imply that
lim supt→∞ˉI(t)≤μμ+γ[ββ−σ21(1−1Rs0)−x0] |
if x0<ββ−σ21(1−1Rs0). But limt→∞1tlog(I(0))=0 a.s. and limt→∞1tM(t)=0 a.s. Finally,
|−βϕ(t)−σ21ϕ(t)(ˉx(t)+(1+γμ)ˉI(t))|≤β|ϕ(t)|+σ21|ϕ(t)|(1+(1+γμ)) |
and limt→∞ϕ(t)=0 a.s. complete this part.
In [28], where the deterministic version of the model is studied, the partial vaccination and persistence of the disease equilibrium E′5≡(1R0,μμ+γ(1−1R0−x′5),x′5), where
x′5=μ(1−1R0)−(δ+ω)(μ+γ)μ−2δ(μ+γ) |
is locally asymptotically stable when
δ(1−2R0)<ω<−δ+μμ+γ(1−1R0), | (3.16) |
The left hand side of inequality (3.16) is equivalent to x′5<1−1R0, the existence condition of E′5. Part 2 of Theorem 3, exhibits a similar result in which if the temporal mean of x(t) converges to x0<1−1Rs0, then the disease persists as in the deterministic model even when x0>0. In which case, parts 2 and 3 of Theorem 3 give
0<μμ+γ[1−1Rs0−x0]≤lim inft→∞ˉI(t)≤lim supt→∞ˉI(t)≤μμ+γ[ββ−σ21(1−1Rs0)−x0], | (3.17) |
Moreover, if σ21=0 while R0>1, then
limt→∞ˉI(t)=μμ+γ[1−1R0−x0], | (3.18) |
When x0=0, the disease persists in a way similar to E4.
When Rs0>1 some non-deterministic limits of the system occur under the condition that σ21β<R02; see, e.g., [43]. Even when σ21=0 and R0>1, in view of Eq (3.18), disease prevalence still fluctuates; see Figure 6(a). The following theorem provides boundaries for those stochastic fluctuations.
Theorem 4. If Rs0>1, and σ21β<R02, then
1. lim inft→∞S(t)≤sd≤lim supt→∞S(t)≤1−x∗ a.s.
2. lim inft→∞I(t)≤(1−sd−x∗)μμ+γ a.s.
3. (1−sd−x∗)μμ+γ≤lim supt→∞I(t)≤(1−sd) a.s.
where sd:=1R021+√1−2σ21βR0≥1R0. Note that the first part implies that x∗≤1−sd.
Proof. Since,
dlog(I(t))=(−12σ21S2(t)+βS(t)−(μ+γ))dt+σ1SdW1(t). |
Let ϕ(z)=−12σ21z2+βz−(μ+γ), which has one zero sd between zero and one if and only if σ21β<R02 and Rs0>1. The function ϕ(z) can have a peak at z∗=βσ21 such that sd<z∗≤1 if and only if β≤σ21. Since ϕ(z) is increasing for z<z∗ then there exists ϵ>0 such that ϕ(z) is increasing for z<sd+ϵ. Thus, ϕ(z)<ϕ(sd−ϵ)<0 for z<sd−ϵ.
Assume that lim supt→∞S(t)<sd−ϵ on a set Ω1 with positive probability, and so 0≤S(u)<sd−ϵ<1 for all u>t0(ω) for each ω∈Ω1. Thus, ϕ(S(u))<ϕ(sd−ϵ)<0 for all u>t0(ω) for each ω∈Ω1. Then, lim supt→∞log(I(t))t≤ϕ(sd−ϵ)<0 with positive probability. That means limt→∞ˉI(t)=0 with positive probability, but that would contradict Theorem 3 part (2).
Now, assume that lim inft→∞S(t)>sd+ϵ on a set Ω2 with positive probability, and so sd+ϵ≤S(u)≤1 for all u>t0(ω) for each ω∈Ω2. Thus, ϕ(S(u))>ϕ(sd+ϵ)>0 for all u>t0(ω) for each ω∈Ω2. Then, lim inft→∞log(I(t))t≥ϕ(sd+ϵ)>0 with positive probability. But that would contradict with that log(I(t))t<0 for all t since I(t)≤1. Thus, part (1) follows.
Again, since
d(S+I)dt=μ(1−x)−μ(S+I)−γI |
then
S(t)+I(t)=e−μt(S(0)+I(0))+1−e−μt∫t0μeμsx(s)ds−γμe−μt∫t0μeμsI(s)ds, |
Therefore, by the generalized L'Hôpital rule (see Supplementary)
lim supt→∞S(t)≤1−lim inft→∞x(t)−(1+γμ)lim inft→∞I(t), |
and so
lim inft→∞I(t)≤(1−sd−x∗)μμ+γ, |
Also, by the generalized L'Hôpital rule (see Supplementary)
lim inft→∞S(t)≥1−lim supt→∞x(t)−(1+γμ)lim supt→∞I(t), |
and so
\begin{equation*} \limsup\limits_{t\to \infty} I(t)\geq (1-s_d-x^*)\frac{\mu}{\mu+\gamma}, \end{equation*} |
if x^* = \limsup\nolimits_{t\to \infty} x(t) < 1 . Since,
\begin{equation*} S(t)+I(t)\leq 1 \end{equation*} |
for all t > 0 , then
\begin{equation*} \limsup\limits_{t\to \infty} I(t)\leq 1-s_d, \end{equation*} |
Note that s_d \downarrow \frac{1}{R_0} as \sigma_1\to 0^+ . Figure 6(a) shows a simulation of one instance of the case where \sigma_1^2 = 0 and R_0^s = R_0 = 6 , in which the proportion of infected fluctuates due to the stochastic nature of vaccine uptake. In that case, S^* = S_* = \frac{1}{R_0} . Figure 6(b) illustrates the case where \sigma_1^2 > 0 and R_0^s = 4.33 with s_d = .168 demarcating S_* and S^* . In both panels of Figure 6, I^* and I_* have the boundaries given by Theorem 4 in one simulation instance. See also Figure S1.
Notice that if x^* = 1 , then x_* must also be equal to one, and in that case I\to 0 and S\to 0 a.s. If x^* = 0 , then 1-s_d is a divider of the I^* and I_* . Moreover, if x^* < 1-s_d , then \limsup\nolimits_{t\to \infty} I(t) > 0 and so \liminf\nolimits_{t\to \infty} I(t) > 0 or otherwise \lim_{t\to \infty} I(t) = 0 since 0 is an absorbing state for I . Note that s_d is always greater than or equal to \frac{1}{R_0} and are equal when \sigma_1^2 = 0 . We can infer that 1-s_d is a stochastic herd immunity threshold HIT^s . Interestingly, HIT^s is less than or equal to the deterministic herd immunity threshold 1-\frac{1}{R_0} .
Note that simulating an almost sure result requires a large number of simulation runs in which the proportion of those simulation runs revealing the result is almost one. Also, we estimated the limit supremum and infimum by long-term simulations in which the cumulative maximum and minimum values of the time-reverse of the process are found to become stable. We notice that they reach stability over a period of time but lose that stability in the last period of the simulation run, since the maximum and minimum are found over a small period of time at the end of the simulation.
The following theorem shows that the limiting temporal variability for fluctuations around levels less than or equal to the equilibria is affected by the noise in the stochastic replicator dynamics representing the parental behavior.
Theorem 5. Let (S_e, I_e, x_e) be an equilibrium of \mathcal{E}_5 type in which x_e\neq 0, 1 . If \frac{1}{2}\frac{2 \mu + \gamma}{\beta} \sigma_1^2 I_e < \mu and \delta < \frac{1}{4} k \sigma^2 , where \sigma^{2} = \sigma_{2}^{2}+\sigma_{3}^{2} , then
\begin{align*} & \limsup\limits_{t\to \infty}\frac{1}{t} \int_0^t \left[ \left( S(u)- \frac{\mu}{\mu -\frac{1}{2}\frac{2 \mu + \gamma}{\beta} \sigma_1^2 I_e} S_e \right)^2+(I(u) - I_e)^2 + (x(u)-x_e)^2 \right] du \leq \\ &\quad \frac{1}{m} \left[ \frac{\mu^2 S_e^{ 2}}{\mu -\frac{1}{2}\frac{2 \mu + \gamma}{\beta} \sigma_1^2 I_e} +\frac{1}{2} \mu \kappa \sigma^2 x_e(1-x_e)+\mu x_e +\mu S_e(1-S_e) \right], \end{align*} |
almost surely, where m = \min(\mu -\frac{1}{2}\frac{2 \mu + \gamma}{\beta} \sigma_1^2 I_e, \, \mu +\gamma, \, \mu \left(\frac{1}{2} k \sigma^2 - 2 \delta \right)) .
Proof. Let a = \kappa \sigma_{3}^{2} -\delta - \omega and b = \kappa \sigma^2 -2 \delta . Notice that we can write a = bx_e-I_e and I + a - bx = (I - I_e) - b(x-x_e) since I_e, x_e are zeros of the drift in the stochastic replicator Eq. Consider the following three non-negative functions:
\begin{align*} V_1 & = - x_e \log \frac{x}{x_e} - (1 - x_e) \log \frac{1-x}{1-x_e}, \\ V_2 & = \frac{1}{2} \left( S - S_e + I - I_e \right)^2, \\ V_3 & = I - I_e - I_e \log \frac{I}{I_e}. \end{align*} |
(Note: V_1 is the relative entropy.) Thus, Itô formula (see Lemma S2 in the Supplementary) implies
\begin{align*} dV_1 & = \left[ \kappa \left( x - x_e \right) \left( I + a - bx \right) \right] + \frac{1}{2} k^2 \sigma^2 (x-x_e)^2 + \frac{1}{2} k^2 \sigma^2 x_e (1-x_e)dt + k\sigma(x-x_e) dW_2 \\ & = \left[ k \left( x - x_e \right) \left( I - I_e \right) + k \left( \frac{1}{2} k \sigma^2 - b \right) \left( x - x_e \right)^2 + \frac{1}{2} k^2 \sigma^2 x_e (1-x_e) \right] dt + k \sigma \left( x - x_e \right) dW_2 \end{align*} |
Moreover,
dV_2 = (S - S_e + I - I_e) [\mu (1 - x) - \mu S - (\mu + \gamma) I] dt |
but since \mu = \mu x_e + \mu S_e + (\mu + \gamma) I_e where S_e, I_e, x_e are zeros of drift of the first Eq in (2.5), then
\begin{align*} dV_2& = \left( (S - S_e) + (I - I_e) \right) [- \mu (x - x_e) - \mu (S - S_e) - (\mu + \gamma)(I - I_e)] \\ & = -\mu (S - S_e)^2 -(\mu + \gamma) (I - I_e)^2 -\mu (S - S_e)(x - x_e) -(2\mu + \gamma) (I - I_e)(S - S_e) -\mu (x - x_e) (I - I_e). \end{align*} |
Finally,
dV_3 = \left[\beta (I - I_e) (S - S_e) + \frac{1}{2} \sigma_1^2 I_e S^2 \right] dt + \sigma_1 S (I - I_e) dW_1 |
Now, let us introduce the non-negative function
V = \frac{\mu}{k} V_1 + V_2 + \frac{2 \mu + \gamma}{\beta} V_3. |
Thus,
\begin{align*} dV & = \left[ - \mu (S - S_e)^2 -(\mu +\gamma)(I - I_e)^2 -\mu \left( \frac{1}{2} k \sigma^2 - 2 \delta \right) (x-x_e)^2 - \mu (S - S_e)(x -x_e) \right. \\ &\quad \left. +\frac{1}{2}\frac{2 \mu + \gamma}{\beta} \sigma_1^2 I_e S^2+ \frac{1}{2} \mu \kappa \sigma^2 x_e(1-x_e) \right] dt + \frac{2 \mu + \gamma}{\beta} \sigma_1 S (I - I_e) dW_1 + \mu \sigma (x - x_e) dW_2. \end{align*} |
But, since - \mu (S - S_e)(x -x_e)\leq \mu S_e x + \mu S x_e\leq \mu S_e + \mu x_e , while
- \mu (S - S_e)^2+\frac{1}{2}\frac{2 \mu + \gamma}{\beta} \sigma_1^2 I_e S^2 = -(\mu -\frac{1}{2}\frac{2 \mu + \gamma}{\beta} \sigma_1^2 I_e )\left[ S- \frac{\mu}{\mu -\frac{1}{2}\frac{2 \mu + \gamma}{\beta} \sigma_1^2 I_e} S_e \right]^2+\frac{\mu^2 S_e^{2}}{\mu -\frac{1}{2}\frac{2 \mu + \gamma}{\beta} \sigma_1^2 I_e}-\mu S_e^{2}, |
then
\begin{align*} LV &\leq -(\mu +\gamma)(I - I_e)^2 -\mu \left( \frac{1}{2} k \sigma^2 - 2 \delta \right) (x-x_e)^2 \\ &\quad -(\mu -\frac{1}{2}\frac{2 \mu + \gamma}{\beta} \sigma_1^2 I_e )\left[ S- \frac{\mu}{\mu -\frac{1}{2}\frac{2 \mu + \gamma}{\beta} \sigma_1^2 I_e} S_e \right]^2+\frac{\mu^2 S_e^{2}}{\mu -\frac{1}{2}\frac{2 \mu + \gamma}{\beta} \sigma_1^2 I_e} \\ &\quad +\frac{1}{2} \mu \kappa \sigma^2 x_e(1-x_e)+\mu x_e +\mu S_e(1-S_e) \end{align*} |
Now, if dV\leq (f(S_e, I_e, x_e)-g(S, I, x))dt+\sigma(S, I, x)dW_t for some positive functions V, f, g , then
0\leq V(S(t), I(t), x(t))\leq V(S(0), I(0), x(0))+ \int_0^t (f(S_e, I_e, x_e)-g(S, I, x))du+\int_0^t \sigma(S, I, x)dW_u |
almost surely. But then
\frac{1}{t} \int_0^t g(S, I, x) du\leq \frac{1}{t}V(S(0), I(0), x(0))+ f(S_e, I_e, x_e)+\frac{1}{t} \int_0^t \sigma(S, I, x)dW_u. |
Thus,
\limsup\limits_{t \to \infty}\frac{1}{t} \int_0^t g(S, I, x) du \leq f(S_e, I_e, x_e) |
almost surely and the result follows.
If \sigma_1^2 = 0 , as in Figure 6(a), then
\begin{eqnarray*} \limsup\limits_{t\to \infty}\frac{1}{t} \int_0^t \left[ \left( S(u)- S_e \right)^2+(I(u) - I_e)^2 + (x(u)-x_e)^2 \right] du \leq &&\\ \quad \frac{1}{\min(1 , \, \frac{1}{2} k \sigma^2 - 2 \delta )} \left[ \frac{1}{2} \kappa \sigma^2 x_e(1-x_e)+ x_e + S_e \right], && \end{eqnarray*} |
almost surely.
In this paper, we introduced a novel model of stochastic replicator dynamics (RD), from stochastic game theory, to depict human choice with noise in their perceived utility function. Including white noise in the utility function serves as a way to model irrationality and stochastic deviations from the classical rational utility. Introducing the white noise to the perceived utilities of both groups gives rise to a mutation term in the drift (deterministic) component in the stochastic RD. The mutation term depicts irrational human choices in which they explore other, possibly non-optimal, behaviors. In addition, the stochastic component of the RD reflects the unpredictable nature of human behavior and the various biases and constraints that influence decision-making. Thus, adding stochastic deviations from the classical rational utility aligns more closely with the bounded rationality framework. To our knowledge, this is the first time that parental choice of vaccination for their children has been modeled using stochastic replicator dynamics to describe their bounded rationality. See also [27,44,45] for other deterministic models of bounded rationality.
We also included noise in disease transmission to investigate its effect on vaccination decision-making. Although the latter has been investigated in the literature, this is the first time that the coupled system of stochastic differential Eqs of SIR and RD has been studied. The coupling takes place as the stochastic process of the proportion of infected children appears in the utility functions and as the stochastic process of the vaccine uptake appears in the first Eq of the stochastic SIR. The model shows new dynamics that generalize to those investigated in [28].
The presence of stochastic noise in the perceived utilities has no effect on the disease transmission if the stochastic basic reproduction number is less than one and the noise in the disease transmission is small enough. But when those conditions change, stochastic noise in perceived utility can affect the dynamical behavior of the disease due to the difference in the magnitude of that noise in the vaccinator and non-vaccinator parents in a way that can counter peer pressure. The larger the magnitude of that noise in the vaccintor's perceived utility, the more the likelihood that parents will not vaccinate their children, and vice versa for non-vaccinators. A clear and consistent view of the utility of vaccinators is required to achieve disease eradication when the disease has a large stochastic basic reproduction number. Disease eradication becomes uncertain when both magnitudes of noises approach the same value. It also depends on the initial acceptance of the vaccine.
We noticed here the emergence of new stochastic herd immunity thresholds that are less than the deterministic one. If vaccination uptake is lower than those new thresholds, then the disease will persist.
The effect of stochasticity on vaccine acceptance and disease eradication could be controlled through a stochastic optimal control. A discount in the cost of vaccination, including but not limited to ease of access to a safe vaccination as well as lowering its price, can achieve full coverage of the vaccine. That is true even when epidemiological, social, and cognitive parameters induce disease persistence. It is shown here that an optimal discount is not required for the full period and could be gradually eased up. The optimal control policy takes into account that vaccination efforts have practical and resource-related bounds such as finite resources and supply.
Although the underlying model attempts to capture the population-level effects of shared or systematic uncertainties, it does not account for heterogeneity in the individual's perceptions of payoffs. The assumption of shared or systematic stochasticity aligns with situations in which systemic external influences, e.g., environmental, cultural, informational, and economical, dominate the individual differences, especially in tightly connected or homogeneous populations. It may be less applicable to diverse populations with significant individual variability, which emphasizes the need for models that balance collective and individual-level influences on disease spread. Future work in stochastic modeling of decision-making in behavioral epidemiology should extend itself to incorporating individual-specific (occasional) stochasticity, which would allow for the exploration of diverse behaviors and richer dynamics at both individual and population levels.
In our simulations, where time is measured in years, the proportions of the susceptible, infected, and vaccine acceptors sometimes take a long time to converge to equilibrium under certain parameter settings. This slow convergence reflects the slow mixing dynamics of the system. In future work, it is warranted to find or estimate parameter values that reflect the empirically observed behavior of these variables in real life.
Adopting stochastic game theory in epidemiological modeling assists in understanding how diseases spread and in forecasting and managing public responses to health crises. This approach provides a more nuanced view of vaccine uptake through human behavior and its impact on public health, establishing behavior management as an essential element of contemporary disease control strategies.
The authors express their sincere gratitude to the editor and the two referees for their invaluable insights and constructive suggestions, which significantly improved the quality and clarity of this manuscript.
The authors declare they have not used Artificial Intelligence (AI) tools in the creation of this article.
The authors declare no conflict of interest.
In the Supplementary, we will present some important definitions, lemmas, and propositions, some of which can be found in the literature. The rest are proven here.
Lemma S1. The generalized L'Hôpital rule [46]
\begin{equation} \liminf\limits_{t\to \infty} \dfrac{f'(t)}{g'(t)}\leq \liminf\limits_{t\to \infty} \dfrac{f(t)}{g(t)}\leq\limsup\limits_{t\to \infty} \dfrac{f(t)}{g(t)}\leq\limsup\limits_{t\to \infty} \dfrac{f'(t)}{g'(t)} \end{equation} | (S1) |
Let (\Omega, \mathcal{F}, \{\mathcal{F}_t\}_{t\geq 0}, \mathbb{P}) be a complete probability space with filtration \{\mathcal{F}_t\}_{t\geq 0} with respect to which all of the stochastic processes introduced in the following are defined.
Lemma S2 (Itô's formula). Let the one-dimensional stochastic differential Eq
dX({t}) = \mu_{t}dt+\sigma_{t}dW({t}) |
If f\left(t, x\right) is a twice-differentiable scalar function, then
df(t, X(t)) = \left(\frac{\partial f}{\partial t}+\mu_{t}\frac{\partial f}{\partial x}+\frac{\sigma_{t}^{2}}{2}\frac{\partial^{2}f}{\partial x^{2}}\right)dt+\sigma_{t}\frac{\partial f}{\partial x}dW(t) |
A d-dimensional stochastic differential Eq
dX(t) = F(t, X(t)) dt + G(t, X(t)) dW(t) |
where the function F(t, x) = \left(f_{i}(t, x)\right)_{1\leq i\leq d} is a d\times 1 vector defined on [0, \infty]\times \mathbb{R}^d and G(t, x) = \left(g_{i, j}(t, x)\right)_{1\leq i\leq d, 1\leq j\leq n} is a d\times n vector defined on [0, \infty]\times \mathbb{R}^d are both locally Lipschitz functions in x . Let W(t) be an n- dimensional Brownian motion. Let V be a function in C^{1, 2}([0, \infty]\times \mathbb{R}^d, [0, \infty)) and let the differential operator L be defined by
LV(t, x) = \dfrac{\partial V(t, x)}{\partial t}+F(t, x)^T DV(t, x) +\frac12 Trace(G(t, x)^T H(t, x) G(t, x) ) |
where DV(t, x) = \dfrac{\partial V(t, x)}{\partial x} is the gradient of V(t, x) and H(t, x) = \dfrac{\partial^2 V(t, x)}{\partial x^2} is the Hessian matrix of V(t, x) . Itô's formula for X(t) and such function V states that
dV(t, X(t)) = LV(t, X(t))dt+DV(t, X(t))^T G(t, X(t)) dW(t) |
for t\geq 0 .
The following lemma can be found in [40].
Lemma S3. Let \{M_t:t\geq 0\} be a real-valued continuous local martingale with respect to \{\mathcal{F}_t\}_{t\geq 0} such that M_0 = 0 . If \limsup\nolimits_{t \to \infty} \frac{\mathbb{E}(M_t^2)}{t} < \infty then \lim_{t \to \infty} \frac{M_t}{t} = 0 a.s.
The following lemma is an extension to the lemmas that were introduced in the Supplementary of [47]. It is new to our knowledge.
Lemma S4. Consider a function f\in C([0, \infty)\times \Omega, (0, \infty)) . Let a(t) be a sequence such that \lim_{t \to \infty} \dfrac{a(t)}{t} = a a.s. and let a and b be two positive constants such that
\log(f(t))\geq (\leq) \, a(t)-b\int_0^t f(s)ds |
for all t\geq 0 a.s. Then
\liminf\limits_{t\to \infty} (\limsup\limits_{t\to \infty})\, \frac{1}{t} \int_0^t f(s)ds\geq (\leq) \, \dfrac{a}{b} \quad a.s. |
Proof. We will prove the " \geq " part and the " \leq " follows using the same approach. Let g(t): = b\, f(t) and so the inequality becomes
\log(g(t))\geq (\leq) \, a(t)+\log(b)-\int_0^t g(s)ds. |
Let \lim_{t \to \infty} \dfrac{a(t)}{t} = a a.s. hence for arbitrary \epsilon > 0 but small enough so that there exists \Omega_\epsilon\in \mathcal{F} with \mathbb{P}(\Omega_\epsilon)\geq 1-\epsilon and 0 < a-\epsilon \leq \dfrac{a(t)}{t}(\omega)\leq a+\epsilon for all t\geq t_0(\omega) for each \omega \in \Omega_\epsilon . Let G(t) = \int_0^t g(s) ds for all t\geq 0 , then dG(t) = g(t)dt and
G(t)\geq a(t)+\log(b) -\log(g(t)) |
for all t\geq 0 . Therefore,
de^{G(t)} = e^{G(t)}g(t)dt\geq e^{a(t)+\log(b) -\log(g(t))} g(t)dt = b e^{a(t)} dt |
and e^{a(t)}\geq e^{(a-\epsilon)t} on \Omega_\epsilon . Thus, for t > t_0
G(t)\geq \log(e^{G(t_0)}+\frac{b}{(a-\epsilon)}[e^{(a-\epsilon)t}-e^{(a-\epsilon)t_0}]) |
Hence, by L'Hôpital rule (see Lemma S1)
\liminf\limits_{n\to \infty} \dfrac{G(t)}{t}\geq a-\epsilon |
and so
\liminf\limits_{t\to \infty} \frac{1}{t} \int_0^t f(s)ds\geq \dfrac{a-\epsilon}{b} \quad \text{ on }\Omega_\epsilon |
for arbitrary \epsilon > 0 , thus
\liminf\limits_{t\to \infty} \frac{1}{t} \int_0^t f(s)ds\geq \, \dfrac{a}{b} \quad a.s. |
Here, we will prove the positive invariance of the solution set, \mathcal{S} = \{(s, i, x)\in \mathbb{R}_+^3: 0\leq s+i \leq 1 \text{ and } 0\leq x \leq 1\} . It is not straightforward since the drift and volatility in the stochastic Eqs are locally Lipschitz continuous but not growing linearly. That might cause a blow-up in the solutions of the stochastic differential Eqs.
Lemma S5. If model (2.5) has initial state (S(0), I(0), x(0))\in \mathcal{S} , then the model has a unique solution (S(t), I(t), x(t))\in \mathcal{S} for all t\geq 0 with probability one.
Proof. All of the relationships in this theorem are true point-wise. By construction of the stochastic replicator Eq 0\leq x(t)\leq 1 for all t > 0 if 0\leq x(0)\leq 1 . Also, d(S+I+R) = 0 , thus if 0\leq S(0)+I(0)\leq 1 and
\begin{eqnarray*} \dfrac{d (S+I)(t)}{dt}& = &\mu(1-x(t))-\mu(S+I)(t)-\gamma I(t) \\ &\leq& \mu(1-x(t))-\mu(S+I)(t) \\ &\leq& \mu -\mu(S+I)(t) \end{eqnarray*} |
for all t > 0 . Thus, for any t > 0 ,
S(t)+I(t)\leq (S(0)+I(0))\, e^{-\mu t}+1-e^{-\mu t}\leq 1. |
On the other hand,
\begin{align*} \dfrac{d (S+I)(t)}{dt}& = \mu(1-x(t))-\mu(S+I)(t)-\gamma I(t) \\ &\geq \mu(1-x(t))-(\mu+\gamma)(S+I)(t) \\ &\geq -(\mu+\gamma)(S+I)(t) \end{align*} |
for all t > 0 . Thus, for any t > 0 ,
S(t)+I(t)\geq (S(0)+I(0))\, e^{-(\mu+\gamma) t}\geq 0. |
The processes S , I , and x are positive bounded processes. Moreover, they have bounded limits are shown in the following proposition.
Proposition S1. If the initial state of the model (2.5) is such that (S(0), I(0), x(0))\in \mathcal{S} , then the following inequality always holds true.
\begin{equation} 1-x^* - \frac{\gamma}{\mu} I^* \leq S_*+I_* \leq S^* + I^*\leq 1-x_* - \frac{\gamma}{\mu} I_* \quad a.s. \end{equation} | (S2) |
Proof. Since
\begin{equation} \frac{d (S+I)(t)}{dt} = \mu(1-x(t))-\mu(S+I)(t)-\gamma I(t) \end{equation} | (S3) |
then
\begin{equation} S(t)+I(t) = e^{-\mu t}(S(0)+I(0))+1-e^{-\mu t}\int_0^t \mu e^{\mu s} x(s) ds -\frac{\gamma}{\mu}e^{-\mu t}\int_0^t \mu e^{\mu s} I(s) ds. \end{equation} | (S4) |
Inequality (S2) follows from using Lemma S5 and the generalized L'Hôpital rule (see inequality (S1) in the Supplementary) applied to the terms e^{-\mu t}\int_{0}^{t} e^{\mu s}x(s)ds and e^{-\mu t}\int_{0}^{t} e^{\mu s}I(s)ds .
For convenience, we define the vector y(t) = [S(t), I(t), x(t)]^\top . The system can be re-written as the SDE vector form
dy(t) = f(y(t), u(t)) dt + g(y(t)) dW(t), |
with initial condition y(0) = [S(0), I(0), x(0)]^\top = y_0 where the functions f and g are vectors with the following components
\begin{equation*} \begin{aligned} f_1(y(t), u(t)) & = \mu (1-x) - \beta S I - \mu S, \\ f_2(y(t), u(t)) & = \beta S I - (\mu + \gamma) I, \\ f_3(y(t), u(t)) & = \kappa x (1-x) \left[ -\omega \, (1-u) + I + \delta (2x-1) + \kappa \left( \sigma_3^2 - (\sigma_2^2 + \sigma_3^2) x \right) \right], \end{aligned} \end{equation*} |
and
\begin{equation*} \begin{aligned} g_1(y(t)) & = -\sigma_1 S I, \\ g_2(y(t)) & = \sigma_1 S I, \\ g_3(y(t)) & = \kappa \sqrt{\sigma_2^2 + \sigma_3^2} \, x (1-x), \end{aligned} \end{equation*} |
respectively. We consider the quadratic cost functional:
J(u) = - \mathbb{E} \left[ \int_0^{T_f} \left( \alpha_1 S(t) + \alpha_2 I(t) + \frac{\alpha_3}{2} u^2(t) \right) dt \right]. |
for some constants \alpha_1, \alpha_2, \alpha_3 \geq 0 . The goal is to find an optimal control u^*(t) such that J(u) \leq J(u^*) , for all u \in U , where the set of admissible controls is U = \{u(t): u(t) \in [0, u_{\max}], \text{ for all } t \in (0, T_f] \} for some 0 \leq u_{\max} < 1 , and T_f is the termination time.
To use the stochastic maximum principle, we define Hamiltonian H(y, u, p, q) by
H(y, u, p, q) = -l(y, u) + \langle f(y, u), p \rangle + \langle g(y), q \rangle |
where \langle \mathbf{u}, \mathbf{v} \rangle = \sum_{\mathbf{i} = \mathbf{1}}^\mathbf{n} \mathbf{u}_\mathbf{i} \mathbf{v}_\mathbf{i} is the Euclidean inner product and l(y, u) = \alpha_1 S + \alpha_2 I + \frac{\alpha_3}{2} u^2 , while p = [p_1, p_2, p_3]^\top is the adjoint vector of costates and q = [q_1, q_2, q_3]^\top are costates volatilities. Hence, the associated Hamiltonian is given by
\begin{equation} \begin{aligned} H & = -\alpha_1 S - \alpha_2 I - \frac{\alpha_3}{2} u^2 \\ &\quad + p_1 \left( \mu (1-x) - \beta S I - \mu S \right) + p_2 \left( \beta S I - (\mu + \gamma) I \right) \\ &\quad + p_3 \left( \kappa x (1-x) \left[ -\omega \, (1-u) + I + \delta (2x-1) + \kappa \left( \sigma_3^2 - (\sigma_2^2 + \sigma_3^2) x \right) \right] \right) \\ &\quad + q_1 \left( -\sigma_1 S I \right) + q_2 \left( \sigma_1 S I \right)+ q_3 \left(\kappa \sqrt{\sigma_2^2 + \sigma_3^2}\, x (1-x)\right). \end{aligned} \end{equation} | (S5) |
According to the stochastic maximum principle,
H(y^*, u^*, p, q) = \max\limits_{u\in U} H(y^*, u, p, q) |
where the optimal states satisfy
dy^*(t) = \frac{\partial H(y^*, u^*, p, q)}{\partial p} dt + g(y^*(t)) dW(t), |
with the backward stochastic differential Eqs of the costates
dp(t) = -\frac{\partial H(y^*, u^*, p, q)}{\partial y} dt + q(t) dW(t), |
where u^* solves
\frac{\partial H(y^*, u, p, q)}{\partial u} = 0, |
and exists since H is concave in u . Thus, the optimal control is given by
\begin{equation} u^* = \max \left\{ 0, \min \left\{ \frac{\kappa \omega}{\alpha_3}p_3 x^* (1-x^*) , u_{\max} \right\} \right\}. \end{equation} | (S6) |
The function p_3(t) is found by solving the system of backward stochastic differential Eqs (BSDEs) given by
\begin{align} dp_1(t) & = \left[ \alpha_1 + p_1 \left( \beta I + \mu - {2}\sigma_1^2 S I^2 \right) - p_2 \left(\beta I+{2} \sigma_1^2 S I^2\right) \right] dt -\sigma_1 p_1 S I dW_1(t), \end{align} | (S7) |
\begin{align} dp_2(t) & = \left[ \alpha_2 + p_1 (\beta S-{2} \sigma_1^2 S^2 I) - p_2 \left( \beta S - (\mu + \gamma)+{2} \sigma_1^2 S^2 I \right) -\kappa p_3 x (1-x) \right] dt + \sigma_1 p_2 S I dW_1(t), \end{align} | (S8) |
\begin{align} dp_3(t) & = \left[ \mu p_1 - \kappa p_3 \left( \left( 1-2x \right) \left( -\omega \, (1-u) + I + \delta (2x-1) + \kappa \left( \sigma_3^2 - (\sigma_2^2 + \sigma_3^2) x \right) \right) \right.\right. \\ &\quad \left. \left. + \left( 2 \delta - \kappa (\sigma_2^2 + \sigma_3^2) \right) x (1-x) + {2} \kappa (\sigma_2^2 + \sigma_3^2) x (1-x) \left( 1-2x \right) \right)\right] dt \\ & \quad + \kappa \sqrt{\sigma_2^2 + \sigma_3^2} p_3 x (1-x) dW_2(t), \end{align} | (S9) |
such that p_i(T_f) = 0 for i = 1, 2, 3 . While x^*(t) solves Eq (3.10) at u^*(t) given by (S6). Including the reduction of susceptible in the objective function results in a longer reduction in the vaccination cost than when excluded from the objective.
[1] |
A. Bish, S. Michie, Demographic and attitudinal determinants of protective behaviours during a pandemic: A review, Br. J. Health Psychol., 15 (2010), 797–824. https://doi.org/10.1348/135910710X485826 doi: 10.1348/135910710X485826
![]() |
[2] |
A. Tversky, D. Kahneman, Judgment under uncertainty: Heuristics and biases, Science, 185 (1974), 1124–1131. https://doi.org/10.1126/science.185.4157.1124 doi: 10.1126/science.185.4157.1124
![]() |
[3] |
W. O. Kermack, A. G. McKendrick, A contribution to the mathematical theory of epidemics, Proc. R. Soc. A., 115 (1927), 700–721. https://doi.org/10.1098/rspa.1927.0118 doi: 10.1098/rspa.1927.0118
![]() |
[4] | N. T. J. Bailey, The Mathematical Theory of Infectious Diseases and Its Applications, 2nd edition, Charles Griffin & Company, London, 1975. |
[5] |
L. J. Allen, Some discrete–time SI, SIR, and SIS epidemic models, Math. Biosci., 124 (1994), 83–114. https://doi.org/10.1016/0025-5564(94)90025-6 doi: 10.1016/0025-5564(94)90025-6
![]() |
[6] |
A. Gray, D. Greenhalgh, L. Hu, X. Mao, J. Pan, A stochastic differential Eq SIS epidemic model, SIAM J. Appl. Math., 71 (2011), 876–902. https://doi.org/10.1137/10081856X doi: 10.1137/10081856X
![]() |
[7] |
E. Tornatore, S. M. Buccellato, P. Vetro, Stability of a stochastic SIR system, Physica A, 354 (2005), 111–126. https://doi.org/10.1016/j.physa.2005.02.057 doi: 10.1016/j.physa.2005.02.057
![]() |
[8] |
N. Gao, Y. Song, X. Wang, J. Liu, Dynamics of a stochastic SIS epidemic model with nonlinear incidence rates, Adv. Differ. Equ., 41 (2019). https://doi.org/10.1186/s13662-019-1980-0 doi: 10.1186/s13662-019-1980-0
![]() |
[9] |
B. Cao, M. Shan, Q. Zhang, W. Wang, A stochastic SIS epidemic model with vaccination, Physica A, 486 (2017), 127–143. https://doi.org/10.1016/j.physa.2017.05.083 doi: 10.1016/j.physa.2017.05.083
![]() |
[10] |
Y. Liu, Stationary distribution and probability density function of a stochastic waterborne pathogen model with saturated direct and indirect transmissions, Math. Meth. Appl. Sci., 46 (2023), 13830–13854. https://doi.org/10.1002/mma.9293 doi: 10.1002/mma.9293
![]() |
[11] | L. J. Allen, An introduction to stochastic epidemic models, In: Brauer, F., van den Driessche, P., Wu, J. (eds) Mathematical Epidemiology. Lecture Notes in Mathematics, vol 1945. Springer, Berlin, Heidelberg, 2008. https://doi.org/10.1007/978-3-540-78911-6_3 |
[12] |
L. Jian, X. Bai, S. Ma, Stochastic dynamical analysis for the complex infectious disease model driven by multisource noises, PLOS One, 19 (2024), e0296183. https://doi.org/10.1371/journal.pone.0296183 doi: 10.1371/journal.pone.0296183
![]() |
[13] |
A. Babaei, H. Jafari, S. Banihashemi, M. Ahmadi, Mathematical analysis of a stochastic model for spread of coronavirus, Chaos Soliton Fract., 145 (2021). https://doi.org/10.1016/j.chaos.2021.110788 doi: 10.1016/j.chaos.2021.110788
![]() |
[14] |
W. A. Iddrisu, I. Iddrisu, A. K. Iddrisu, Modeling cholera epidemiology using stochastic differential Eqs, J. Appl. Math., 2023 (2023), 7232395. https://doi.org/10.1155/2023/7232395 doi: 10.1155/2023/7232395
![]() |
[15] | P. C. Fishburn, The Foundations of Expected Utility, D. Reidel Publishing Company, 1982. https://doi.org/10.1007/978-94-017-3329-8 |
[16] | L. J. Savage, The Foundations of Statistics, John Wiley and Sons, 1954. |
[17] | J. Von Neumann, O. Morgenstern, Theory of Games and Economic Behavior, Princeton University Press, 1944. |
[18] | H. A. Simon, Models of Bounded Rationality, Volume 3: Empirically Grounded Economic Reason, MIT Press, 1982. https://doi.org/10.7551/mitpress/4711.001.0001 |
[19] | H. A. Simon, Models of Bounded Rationality: Behavioral Economics and Business Organization, MIT Press, 1984. 10.1604/9780262192064 |
[20] | C. F. Camerer, Behavioral Game Theory: Experiments in Strategic Interaction, Princeton University Press, 2011. |
[21] |
C. T. Bauch, Imitation dynamics predict vaccinating behaviour, Proc. R. Soc. B., 272 (2005), 1669–1675. https://doi.org/10.1098/rspb.2005.3153 doi: 10.1098/rspb.2005.3153
![]() |
[22] |
A. D'Onofrio, P. Manfredi, P. Poletti, The impact of vaccine side effects on the natural history of immunization programmes: an imitation-game approach, J. Theor. Biol., 273 (2011), 63–71. https://doi.org/10.1016/j.jtbi.2010.12.029 doi: 10.1016/j.jtbi.2010.12.029
![]() |
[23] | P. Manfredi, A. D'Onofrio, Modeling the interplay between human behavior and the spread of infectious diseases, Springer, 2013. https://doi.org/10.1007/978-1-4614-5474-8 |
[24] |
T. C. Reluga, C. T. Bauch, A. P. Galvani, Evolving public perceptions and stability in vaccine uptake, Math. Biosci., 204 (2006), 185–198. https://doi.org/10.1016/j.mbs.2006.08.015 doi: 10.1016/j.mbs.2006.08.015
![]() |
[25] |
Z. Wang, C. T. Bauch, S. Bhattacharyya, A. d'Onofrio, P. Manfredi, M. Perc, et al., Statistical physics of vaccination, Phys. Rep., 664 (2016), 1–113. https://doi.org/10.1016/j.physrep.2016.10.006 doi: 10.1016/j.physrep.2016.10.006
![]() |
[26] |
C. T. Bauch, S. Bhattacharyya, Evolutionary game theory and social learning can determine how vaccine scares unfold, PLoS Comput. Biol., 8 (2012), e1002452. https://doi.org/10.1371/journal.pcbi.1002452 doi: 10.1371/journal.pcbi.1002452
![]() |
[27] |
T. Oraby, C. T. Bauch, Bounded rationality alters the dynamics of paediatric immunization acceptance, Sci Rep., 5 (2015), 10724. https://doi.org/10.1038/srep10724 doi: 10.1038/srep10724
![]() |
[28] |
T. Oraby, V. Thampi, C. T. Bauch, The influence of social norms on the dynamics of vaccinating behaviour for paediatric infectious diseases, Proc. R. Soc. B., 281 (2014), 20133172. https://doi.org/10.1098/rspb.2013.3172 doi: 10.1098/rspb.2013.3172
![]() |
[29] |
Y. Ibuka, M. Li, J. Vietri, G. B. Chapman, A. P. Galvani, Free–riding behavior in vaccination decisions: an experimental study, PLoS One, 9 (2014), e87164. https://doi.org/10.1371/journal.pone.0087164 doi: 10.1371/journal.pone.0087164
![]() |
[30] |
K. T. Eames, Networks of influence and infection: parental choices and childhood disease, J. R. Soc. Interface, 6 (2009), 811–814. https://doi.org/10.1098/rsif.2009.0085 doi: 10.1098/rsif.2009.0085
![]() |
[31] |
M. L. Ndeffo Mbah, J. Z. Liu, C. T. Bauch, Y. I. Tekel, J. Medlock, L. A. Meyers, et al., The impact of imitation on vaccination behavior in social contact networks, PLoS Comput. Biol., 8 (2012), e1002469. https://doi.org/10.1371/journal.pcbi.1002469 doi: 10.1371/journal.pcbi.1002469
![]() |
[32] |
C. E. Wagner, J. A. Prentice, C. M. Saad-Roy, L. J. Yang, B. T. Grenfell, S. A. Levin, et al., Economic and behavioral influencers of vaccination and antimicrobial use, Front. Public Health, 8 (2020), 614113. https://doi.org/10.3389/fpubh.2020.614113 doi: 10.3389/fpubh.2020.614113
![]() |
[33] | D. Fudenberg, D. K. Levine, Theory of Learning in Games, MIT Press, 1998. |
[34] |
C. T. Bauch, D. J. Earn, Vaccination and the theory of games, Proc. Natl. Acad. Sci. U.S.A., 101 (2004), 13391–13394. https://doi.org/10.1073/pnas.0403823101 doi: 10.1073/pnas.0403823101
![]() |
[35] |
C. Betsch, R. Böhm, L. Korn, Inviting free–riders or appealing to prosocial behavior? game–theoretical reflections on communicating herd immunity in vaccine advocacy, Health Psychol., 32 (2013), 978. https://doi.org/10.1037/a0031590 doi: 10.1037/a0031590
![]() |
[36] |
M. Benaïm, J. Hofbauer, W. H. Sandholm, Robust permanence and impermanence for stochastic replicator dynamics, J. Biol. Dyn., 2 (2008), 180–195. https://doi.org/10.1080/17513750801915269 doi: 10.1080/17513750801915269
![]() |
[37] |
D. Fudenberg, C. Harris, Evolutionary dynamics with aggregate shocks, J. Econ. Theory, 57 (1992), 420–441. https://doi.org/10.1016/0022-0531(92)90044-I doi: 10.1016/0022-0531(92)90044-I
![]() |
[38] | J. Hofbauer, K. Sigmund, Evolutionary Games and Population Dynamics, Cambridge University Press, 1998. https://doi.org/10.1017/CBO9781139173179 |
[39] | P. E. Kloeden, E. Platen, Numerical Solution of Stochastic Differential Eqs, Springer, Berlin, Heidelberg, 1992. https://doi.org/10.1037/a0031590 |
[40] | X. Mao, Stochastic Differential Eqs and Applications, Woodhead Publishing, 2007. https://doi.org/10.1533/9780857099402 |
[41] |
Q. Han, D. Jiang, S. Lin, C. Yuan, The threshold of stochastic SIS epidemic model with saturated incidence rate, Adv. Differ. Equ., 2015 (2015), 1–10. https://doi.org/10.1186/s13662-015-0355-4 doi: 10.1186/s13662-015-0355-4
![]() |
[42] | J. Yong, X. Y. Zhou, Stochastic Controls: Hamiltonian Systems and HJB Eqs, Springer Science & Business Media, 43 (2012). https://doi.org/10.1007/978-1-4612-1466-3 |
[43] |
A. Lahrouz, A. Settati, Necessary and sufficient condition for extinction and persistence of SIRS system with random perturbation, Appl. Math. Comput., 233 (2014), 10–19. https://doi.org/10.1016/j.amc.2014.01.158 doi: 10.1016/j.amc.2014.01.158
![]() |
[44] |
T. Oraby, A. Balogh, Modeling the effect of observational social learning on parental decision-making for childhood vaccination and diseases spread over household networks, Front. Epidemiol., 3 (2024), 1177752. https://doi.org/10.3389/fepid.2023.1177752 doi: 10.3389/fepid.2023.1177752
![]() |
[45] | W. Yin, M. Ndeffo-Mbah, T. Oraby, Vaccination games of boundedly rational parents toward new childhood immunization, Preprint at Research Square, (2024). https://doi.org/10.21203/rs.3.rs-4572949/v1 |
[46] |
W. H. Young, On indeterminate forms, Proc. Lond. Math. Soc., s2-8 (1910), 40–76. https://doi.org/10.1112/plms/s2-8.1.40 doi: 10.1112/plms/s2-8.1.40
![]() |
[47] |
C. Ji, D. Jiang, Threshold behaviour of a stochastic SIR model, Appl. Math. Model., 38 (2014), 5067–5079. https://doi.org/10.1016/j.apm.2014.03.037 doi: 10.1016/j.apm.2014.03.037
![]() |