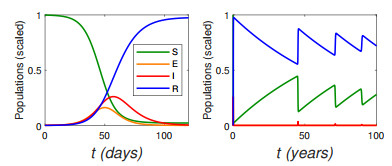
Local stability analysis is an important tool in the study of dynamical systems. When the goal is to determine the effect of parameter values on stability, it is necessary to perform the analysis without explicit parameter values. For systems with three components, the usual method of finding the characteristic polynomial as det(J−λI) and applying the Routh-Hurwitz conditions is reasonably efficient. For larger systems of four to six components, the method is impractical, as the calculations become too messy. In epidemiological models, there is often a very small parameter that appears as the ratio of a disease-based timescale to a demographic timescale; this allows efficient use of asymptotic approximation to simplify the calculations at little cost. Here, we describe the tools and a set of guidelines that are generally useful in applying the method, followed by two examples of efficient stability analysis.
Citation: Glenn Ledder. Using asymptotics for efficient stability determination in epidemiological models[J]. Mathematical Biosciences and Engineering, 2025, 22(2): 290-323. doi: 10.3934/mbe.2025012
[1] | Xue Liu, Xin You Meng . Dynamics of Bacterial white spot disease spreads in Litopenaeus Vannamei with time-varying delay. Mathematical Biosciences and Engineering, 2023, 20(12): 20748-20769. doi: 10.3934/mbe.2023918 |
[2] | Yoichi Enatsu, Yukihiko Nakata . Stability and bifurcation analysis of epidemic models with saturated incidence rates: An application to a nonmonotone incidence rate. Mathematical Biosciences and Engineering, 2014, 11(4): 785-805. doi: 10.3934/mbe.2014.11.785 |
[3] | Miled El Hajji, Amer Hassan Albargi . A mathematical investigation of an "SVEIR" epidemic model for the measles transmission. Mathematical Biosciences and Engineering, 2022, 19(3): 2853-2875. doi: 10.3934/mbe.2022131 |
[4] | Mostafa Adimy, Abdennasser Chekroun, Claudia Pio Ferreira . Global dynamics of a differential-difference system: a case of Kermack-McKendrick SIR model with age-structured protection phase. Mathematical Biosciences and Engineering, 2020, 17(2): 1329-1354. doi: 10.3934/mbe.2020067 |
[5] | Haifeng Huo, Fanhong Zhang, Hong Xiang . Spatiotemporal dynamics for impulsive eco-epidemiological model with Crowley-Martin type functional response. Mathematical Biosciences and Engineering, 2022, 19(12): 12180-12211. doi: 10.3934/mbe.2022567 |
[6] | Xing Zhang, Zhitao Li, Lixin Gao . Stability analysis of a SAIR epidemic model on scale-free community networks. Mathematical Biosciences and Engineering, 2024, 21(3): 4648-4668. doi: 10.3934/mbe.2024204 |
[7] | Wisdom S. Avusuglo, Kenzu Abdella, Wenying Feng . Stability analysis on an economic epidemiological model with vaccination. Mathematical Biosciences and Engineering, 2017, 14(4): 975-999. doi: 10.3934/mbe.2017051 |
[8] | Xiaofen Lin, Hua Liu, Xiaotao Han, Yumei Wei . Stability and Hopf bifurcation of an SIR epidemic model with density-dependent transmission and Allee effect. Mathematical Biosciences and Engineering, 2023, 20(2): 2750-2775. doi: 10.3934/mbe.2023129 |
[9] | Tailei Zhang, Hui Li, Na Xie, Wenhui Fu, Kai Wang, Xiongjie Ding . Mathematical analysis and simulation of a Hepatitis B model with time delay: A case study for Xinjiang, China. Mathematical Biosciences and Engineering, 2020, 17(2): 1757-1775. doi: 10.3934/mbe.2020092 |
[10] | Paolo Fergola, Marianna Cerasuolo, Edoardo Beretta . An allelopathic competition model with quorum sensing and delayed toxicant production. Mathematical Biosciences and Engineering, 2006, 3(1): 37-50. doi: 10.3934/mbe.2006.3.37 |
Local stability analysis is an important tool in the study of dynamical systems. When the goal is to determine the effect of parameter values on stability, it is necessary to perform the analysis without explicit parameter values. For systems with three components, the usual method of finding the characteristic polynomial as det(J−λI) and applying the Routh-Hurwitz conditions is reasonably efficient. For larger systems of four to six components, the method is impractical, as the calculations become too messy. In epidemiological models, there is often a very small parameter that appears as the ratio of a disease-based timescale to a demographic timescale; this allows efficient use of asymptotic approximation to simplify the calculations at little cost. Here, we describe the tools and a set of guidelines that are generally useful in applying the method, followed by two examples of efficient stability analysis.
Local stability analysis is an important part of the investigation of any endemic disease model. When possible, it is ideal to identify a small set of inequalities that divide the parameter space into regions that define the set of equilibria that are locally asymptotically stable. Often the stability structure is simple, with a unique stable equilibrium at each point in the parameter space and relatively simple defining inequalities as well. A typical case is for there to be two regions, one with a globally stable disease-free equilibrium (DFE) and the other with a globally stable endemic disease equilibrium (EDE), with the boundary occurring where the basic reproduction number R0 is unity. More complicated disease models can produce more complicated behavior. For example, some models exhibit backward bifurcation, in which there is a region in the parameter space with both a stable EDE and a stable DFE [1,2,3], while other models have parameter space regions where there are no stable equilibria (see, for example, [4,5]).
Local asymptotic stability for a given set of parameter values can be determined by numerical evaluation of the eigenvalues, but general results require analytical methods. The direct method is to compute the characteristic polynomial as P(λ)=det(J−λI), where J is the Jacobian matrix and I the identity matrix, followed by calculation of eigenvalues. This method requires complicated calculations; hence, it is seldom used for systems with more than two components. Systems of three components can be done without too much difficulty by finding the characteristic polynomial in the usual way and then employing the Routh-Hurwitz conditions [3,6,7]. In principle, the same method can be used for higher-order models, but this has rarely been done and no examples are given in the principal monographs of mathematical epidemiology; e.g., [1,3].
Our ability to determine stability requirements analytically depends on the efficiency of the methods we use. If the Jacobian is reducible, as is often the case for a DFE, then the eigenvalue problem decouples into a set of lower-dimensional eigenvalue problems. Otherwise, the Routh-Hurwitz conditions are more efficient than full calculation of eigenvalues. An example is in [3], page 101, where a 5th order eigenvalue problem is reduced to a 3rd order one, with the Routh-Hurwitz conditions used for the latter. There are also more efficient methods for calculating the characteristic polynomial (see Section 3). With these tools, it is frequently feasible to do analytical stability calculation for systems with four components, as in [8]. However, the additional tool of asymptotic approximation can extend the range of problems for which analytical stability analysis is possible to systems of five components, as shown below, and occasionally even six components, as in [9]. This gain comes at the minor cost of a degree of error that is insignificant when compared to error caused by uncertainty of parameter values or by neglecting minor features of disease natural history. In the development that follows, we provide detailed guidelines for the method and two illustrative examples. In so doing, we note the specific requirements for a model to be amenable to the method.
We begin in Section 2 with a general discussion of the characteristics of epidemiological models with two timescales. This is followed by a description in Section 3 of the three mathematical tools that form the basis for our method and a set of guidelines for employing the method. Section 4 consists of an example that goes through the process in some detail, culminating in a proof of stability subject to a simple, previously unknown, restriction on one of the parameter values. Section 5 consists of an example in which there is a very small region in the parameter space for which there are no stable equilibria. We conclude with a discussion of the benefits of the method.
Figure 1 shows a typical simulation for the infectious disease model,
dSdT=μ−μS−βSI,S(0)=1−I0,dEdT=−(η+μ)E+βSI,E(0)=0,dIdT=ηE−(γ+μ)I,I(0)=I0,dRdT=γI−μR,R(0)=0, | (1) |
where I0 is very small. This is the basic SEIR epidemic model with demographic terms for death rates proportional to the population and a constant birth rate just large enough to maintain the population. The initial conditions are chosen so that the state variables represent population fractions. The scenario is a typical example of an infectious disease model with disease duration far less than host lifespan and a predominantly susceptible initial population. The plots help us to understand the importance of timescales in understanding epidemiological models.
The left panel shows the epidemic phase of the simulation. We see that
1) Infected population fractions (E and I) are significant.
2) There is no indication of the endemic disease behavior that follows: we cannot tell whether there is a stable EDE.
In contrast, the plot on the right shows the endemic behavior. Here,
1) Infected population fractions are very small.
2) There is no indication of the epidemic behavior that preceded the endemic phase. Everything that happened in the first 100 days is located at the left edge of the plot.
3) Recurrences at approximately 48 and 72 years, and at shorter intervals thereafter, show rapid changes on the epidemic timescale.
The difference in model behavior on the epidemic and endemic timescales is a feature that can be utilized to simplify a given task. For investigations focused on the epidemic phase, anything that takes a long time to change the result, such as births and natural deaths, can be ignored. For investigations focused on the endemic phase, the low populations of the infected classes can be exploited through asymptotic approximation. This requires that the variables used to represent uninfected classes are scaled as population fractions, while the variables used to represent infected classes are rescaled under the assumption that they are vanishingly small in some asymptotic limit.
The parameters used for the simulation correspond to a mean lifespan of 78.3 years, a mean infectious period of 10 days, a mean incubation period of 5 days, and a basic reproduction number of 4, all typical values for infectious diseases. The ratio of disease duration to mean lifespan is 0.00035. This timescale ratio is responsible for the qualitative differences between the two panels in Figure 1. Even if the mean infectious period is as large as 30 days, this ratio is still only 0.001, which is more than small enough for the qualitative differences to be apparent.
The method we employ for equilibrium analysis is based on a combination of three mathematical tools that we describe here: an efficient scheme for calculating the characteristic polynomial, asymptotic approximation to eliminate terms with negligible impact, and the Routh array for constructing asymptotic approximations to the Routh-Hurwitz conditions.
The Characteristic Polynomial Theorem The usual way to compute the characteristic polynomial for a matrix J is to find the determinant of λI−J; 1 however, there is a more efficient method based on a theorem from matrix theory (see [10], for example):
1λI−J is preferable to the more usual J−λI because its leading order coefficient is always positive.
Theorem 1. For an n×n matrix J, let S be the set of all nonempty subsets K of the integers 1,2,…,n. For each possible index set K, let JK be the determinant of the sub-matrix of J that contains the entries in the rows and columns indicated by K. Then the characteristic polynomial of J is
P(λ)=λn+c1λn−1+c2λn−2+⋯+cn−1λ+cn, | (2) |
where
cm=(−1)m∑|K|=mJK,cn=(−1)n|J|. | (3) |
While this formula technically requires computation of all the sub-determinants of J, this is still easier than computing the determinant of λI−J. The next tool allows us to neglect some of those sub-determinants.
Asymptotic Approximation Asymptotic approximation is simply a matter of Taylor series expansion about ϵ→0, or Γ=1/ϵ→∞ (see Chapter 1 of [11], for example). Each coefficient in the characteristic polynomial has an asymptotic expansion of the form
cm=kmΓpm+O(Γpm−1),Γ→∞; | (4) |
to determine the stability requirements to leading order, we need only to compute the km and pm. For systems that have dynamic equations on two different timescales, there are some rows in the Jacobian with a common factor of Γ and some without. The presence of factors of Γ in some rows of the Jacobian means that some of the sub-determinants in the calculations of c1 through cn−1 are dominated by others. For example, suppose row 1 in the Jacobian has a factor of Γ while rows 2,3,…,n do not. Then at least one sub-determinant of the form J1j is O(Γ), while all sub-determinants Jij with i>1 are O(1).
While one can retain terms beyond the leading order, if Γ is sufficiently large then only terms that contribute to the leading order expansions of the characteristic polynomial coefficients need be retained to get a good approximation of stability requirements. This generally means that only dominant terms need to be kept in all calculations; however, all Jacobian terms of O(1) should be kept long enough to calculate the characteristic polynomial coefficients, even if they are dominated in their differential equation by a term of O(Γ). The necessity of this precaution is seen in Section 4.
The Routh Array The Routh-Hurwitz (RH) conditions use the algebraic signs of combinations of the coefficients of the characteristic polynomial to determine if all of its roots are in the left half of the complex plane. The RH conditions for degrees 2 and 3 are well-known; for higher degrees it is convenient to construct them from the Routh array, which is a two-dimensional array with n+1 rows and ⌈n/2⌉ columns [12].2 Let Ri,j be the element in row i, column j of the array. The array is populated from a characteristic polynomial
2Other computational schemes could also be used, such as the Hurwitz matrix; however, the Routh array is particularly convenient when combined with asymptotic approximation.
P(λ)=λn+c1λn−1+c2λn−2+⋯+cn |
according to the following rules:
1) The first row consists of the leading 1 from the characteristic polynomial, followed by c2, c4, and so on.
2) The second row consists of the coefficients c1, c3, and so on, with a trailing 0 if n is even.
3) For i>2, the entries Ri,j are given by
Ri,j=Ri−1,1Ri−2,j+1−Ri−2,1Ri−1,j+1Ri−1,1, |
where formula terms requiring a column outside the dimensions of the array are taken as 0.
As an example, consider the Routh array for a fourth degree polynomial P(λ)=λ4+c1λ3+c2λ2+c3λ+c4, which takes the simple form3
3It is common to leave 0 entries as blanks.
1c2c4c1c3q1c1c4q2q1c4, | (5) |
where
q1=c1c2−c3,q2=c3q1−c21c4. | (6) |
Expressing some of the entries as rational quantities, with a previously defined quantity in the denominator and a newly defined quantity in the numerator, as has been done here, is a convenient modification to facilitate the next step.
The Routh theorem picks the RH stability conditions out of the Routh array.
Theorem 2 (Routh). The critical point with characteristic polynomial P(λ) is locally asymptotically stable if and only if all entries in column 1 of the Routh array are positive.
For the fourth degree case, the conditions are
c1>0,c4>0,q1>0,q2>0. | (7) |
Asymptotic approximation sometimes reduces the formulas that arise in the Routh array to simpler ones. For example, suppose c1=k1Γ, c2=k2Γ2, and c3=k3Γ2, all as Γ→∞ with kj=O(1). Then the product c1c2 dominates c3, and therefore q1=k1k2Γ3+O(Γ2). Since k1>0 is already required, we can replace q1>0 with the simpler k2>0. Simplifications such as this emerge naturally when the Routh array is constructed from the characteristic polynomial approximation
P(λ)∼λn+k1Γp1λn−1+⋯+knΓpn |
and only the leading order elements of the array are given.
The theorems for calculation of the characteristic polynomial and the RH conditions can be used on any problem. Obtaining asymptotic approximations to the stability conditions for an equilibrium requires that some guidelines be followed so that the method can be properly employed and so that algebraic complexity is kept to a minimum. We present a set of guidelines here; the starting point is the dimensional dynamical system model. See [6] for more detail on steps 1–4, which comprise the scaling.
1) Scale populations so that the DFE population total is 1. This can often be done during the initial model construction by choosing a birth rate coefficient to match the natural death rate.
2) Identify one principal fast timescale and one principal slow timescale.4 Use the principal slow timescale to scale the time.5
4For most infectious diseases in human populations, fast processes have timescales on the order of days or weeks, while slow processes have timescales on the order of years, or perhaps months. Usually the principal fast timescale is the amount of time spent in the principal infectious state and the principal slow timescale is the lifespan of the disease host.
5The fast timescale is preferable for simulations, but the slow timescale is preferred when the primary interest is in long-term behavior.
3) Define ϵ as the ratio of the principal slow rate to the principal fast rate. Make all other parameters dimensionless by reference to the appropriate principal scale; that is, rate parameters in fast processes, such as infection, should be referred to the principal fast rate, while rate parameters in slow processes, such as loss of immunity, should be referred to the principal slow rate.6
6Very few, if any, authors who write about scaling pay any attention to the definition of dimensionless parameters. This is unfortunate. The full set of parameters should be chosen to at least make sure there are not any parameters whose value is close to 1, such as γ/(γ+μ) and that only one parameter is "small" or "large" in the sense of being suitable for an asymptotic series.
4) Rescale "small" populations by a factor of ϵ. Small populations are generally those for which the dynamics of increase and decrease are dominated by fast processes; for example, in the SEIR model, the dynamics of the latent ("exposed") class is due to the fast processes of infection and incubation, and the dynamics of the infectious class is due primarily to the fast processes of incubation and recovery.7 A population can be seen to be small if it appears only in terms with factors of ϵ−1 relative to the other terms in the equation. These terms look large, but only because the corresponding state variable has an inherent, albeit invisible, factor of ϵ when viewed on the long timescale. The rescaling serves to make all variables O(1), so that the importance of terms is determined entirely by the visible factors of ϵ. If no populations meet this criterion, then asymptotics has limited value, if any.
7The infectious class in the SI model is not small in this sense because there is no recovery process to drain away the infectious population.
5) It is often helpful to choose an equation order for the Jacobian that groups the fast equations at the beginning of the list. These are equations for which the derivative side has a factor of ϵ compared to the terms on the right side.
6) When equilibria cannot be determined by linear equations alone, use the equilibrium relations to try to write all but one of the dependent variables in terms of a principal dependent variable z. Derive the resulting polynomial equation G(z)=0 for that dependent variable, but do not solve it. Parameter range requirements for solution existence can be determined in terms of the function G evaluated at critical values when direct comparison of the critical value with the equilibrium values is difficult.
7) Define auxiliary quantities, as needed, to simplify formulas. Examples include quantities that represent weighted averages of infectious or susceptible populations or quantities that combine together in one entry of the Jacobian matrix. Because of the scaling, there are often terms that include a variable plus 1; in these cases, a more compact notation is facilitated by the convention of using a bar over the top of any quantity to indicate that quantity plus 1. A general rule of thumb is that having more entries in the table of symbols is preferable to having more terms in an equation or factors in a term.
8) Look for opportunities to simplify formulas in the Jacobian by replacing dependent variables with versions that include an extra factor. See (21) in Section 4 below.
9) During the stability analysis, it is important to keep checking whether combinations of auxiliary quantities can be simplified or whether it would be helpful to introduce additional combinations.
10) Unless the eigenvalues are immediately apparent from the Jacobian, use the appropriate RH conditions to determine stability. In doing so, use Theorem 1 to compute the coefficients of the characteristic polynomial to leading order. For anything over three variables, use the Routh array (Theorem 2) to determine the RH conditions to leading order.
As an example of the method outlined in Section 3.1, we consider a model previously studied by Gumel [2]. The analysis in that paper identifies a complicated inequality that is both necessary and sufficient for backward bifurcation to occur, but does not attempt to find a simpler necessary condition or to show that the inequality is satisfied in a meaningful portion of the parameter space. Here we show that 1) backward bifurcation requires a mortality probability of greater than 75%, an extreme condition that calls some of the model assumptions into question, and 2) when mortality is not that high, there is always a unique EDE that is locally asymptotically stable.
The model considers a disease scenario having two risk groups of changing composition, along with standard incidence and disease-induced mortality. The schematic appears in Figure 2. We euphemistically describe the subgroups of susceptibles as "protected" and "unprotected, " to be taken in the sense that "protected" people are less susceptible than "unprotected" people, for whatever reason. Individuals are born/recruited into either of these categories, with some fixed proportion of each. In the general case, they can also move between these categories through behavior changes. By choosing parameters so that all new individuals are initially unprotected and protected individuals never switch to unprotected, we obtain the special case of a disease with a vaccine that reduces the risk of infection for individual encounters without conferring immunity. The protected are less susceptible to the disease by a factor of σ<1.
As needed during the analysis, we define new quantities to reduce the number of symbols appearing in formulas (see guidelines 7 and 8). For example, the total rate of infection can be written as βQI/N where Q=U+σP=(1−σ)U+σS. Note that this definition implies U≤Q≤S. Quantities that appear in the original form of the model are listed in Table 1, while quantities that appear in the scaled model are listed in Table 2. Additional notation defined merely for algebraic convenience is introduced in the analysis and listed in Table 3 for convenience.
Quantity | Reference | Dimension | Definition |
E | Figure 2 | 1 | Latent ("exposed") population |
I | Figure 2 | 1 | Infectious population |
N | Figure 2 | 1 | Total population |
P | Figure 2 | 1 | Low-risk susceptible population |
Q=U+σP | (11) | 1 | Susceptibility fraction |
R | Figure 2 | 1 | Recovered population |
S=P+U | (10) | 1 | Total susceptible population |
T | Figure 2 | T | Time |
U | Figure 2 | 1 | High-risk susceptible population |
f | Figure 2 | 1 | fraction of recruits that are high-risk |
β | Figure 2 | T−1 | Rate coefficient for infection |
γ | Figure 2 | T−1 | Rate coefficient for recovery |
δ | Figure 2 | T−1 | Mortality probability |
η | Figure 2 | T−1 | Rate coefficient for incubation |
μ | Figure 2 | T−1 | Rate coefficient for birth and death |
σ | Figure 2 | 1 | relative low-risk susceptibility |
Ψ | Figure 2 | T−1 | Rate coefficient for transition from P to U |
Ω | Figure 2 | T−1 | Rate coefficient for transition from U to P |
Quantity | Reference | Interpretation |
N, S, Q, U, σ | See Table 1. | |
t=μT | (12) | Dimensionless time, that is, time measured in lifespans |
X=ϵ−1E | (16) | Rescaled latent ("exposed") population |
Y=ϵ−1I | (16) | Rescaled infectious population |
b=β/(γ+δ+μ) | (13) | Dimensionless infection rate coefficient; maximum R0 |
m=δ/(γ+δ+μ) | (13) | Fraction of infectious individuals who die of the disease |
ϵ=μ/(γ+δ+μ) | (13) | Ratio of expected disease duration to expected host lifespan |
ρ=η/(γ+δ+μ) | (13) | Dimensionless incubation rate coefficient |
ψ=Ψ/μ | (14) | Dimensionless rate coef. for transition from low risk to high risk |
Σ=(Ψ+Ω)/μ | (14) | Dimensionless sum of risk level transition rate coefficients |
Quantities | Reference | Quantities | Reference | Quantities | Reference |
κ,h,ˉΣ,ˉρ | (18) | y,s,u,p,q | (21) | A,B,C | Prop. 3 |
R0 | (19) | z,v,r,w | (22) | k1,…,k5 | (31) |
c | (20) | z∗,ˆz | (27) | q1,q2 | (33) |
From Figure 2, the model is
dPdT=(1−f)μ−(Ψ+μ)P+ΩU−σβPIN,dUdT=fμ+ΨP−(Ω+μ)U−βUIN,dEdT=−(η+μ)E+σβPIN+βUIN,dIdT=ηE−(γ+δ+μ)I,dRdT=γI−μR, | (8) |
with
N=P+U+E+I+R | (9) |
and with T used for dimensional time in order to reserve t for dimensionless time. The constant birth rate coefficient has been assigned the same symbol (μ) as that for the natural death rate, thereby automatically scaling the model so that the disease-free population total is N=1 whenever the initial conditions sum to 1.
The total susceptible population S and the total population N are more important quantities for understanding the model than P and R; hence, we rewrite the model using S and N in place of these quantities:
dSdT=μ(1−S)−βQIN,dUdT=fμ+Ψ(S−U)−(Ω+μ)U−βUIN,dEdT=−(η+μ)I+βQIN,dIdT=ηE−(γ+δ+μ)I,dNdT=μ(1−N)−δI, | (10) |
where we have introduced the additional variable
Q=U+σP=(1−σ)U+σS,U≤Q≤S | (11) |
to simplify the notation. We can think of Q as a "susceptibility fraction" that indicates the size of an unprotected population that would have the same total susceptibility in the absence of a protected subclass as the actual overall population.
Guidelines 1–5 in Section 3.1 direct a recasting of the system to render it optimal for analysis. Our original system satisfies the first guideline. Next we identify the various rate parameters and use them to choose a timescale for nondimensionalization and an appropriate set of dimensionless parameters.
Natural demographic processes operate on a long timescale of years, giving us a natural long timescale 1/μ. Following guideline 2, we choose this as the timescale; hence, the dimensionless time variable is t=μT; this variable change is implemented as
ddT=μddt. | (12) |
The infection, incubation, recovery, and death processes operate on a short timescale of days or weeks, giving us fast rates β, η, γ, and δ, respectively. The most important fast rate is γ+δ+μ, which is the rate of departure from the infectious class. Following guideline 3, we define the small parameter ϵ and dimensionless infection, incubation, and death parameters b, ρ, and m as
ϵ=μγ+δ+μ,b=βγ+δ+μ,ρ=ηγ+δ+μ,m=δγ+δ+μ. | (13) |
Note that dimensionless versions of parameters often have a significant meaning of their own; here, m is the probability that an infected individual dies and b is the basic reproduction number for the special case where all susceptibles are high risk.8 These parameters are more significant to the model than the original parameters δ and β that they represent. While ρ does not have a special interpretation, we can see that it is the ratio of expected duration of infectiousness to expected incubation time for those individuals who do not die during the incubation period.
8The basic reproduction number if all susceptibles are low risk is σb. The actual basic reproduction number σb<R0<b must be determined in the analysis.
It is not immediately clear how to classify the status-change rate parameters Ψ and Ω, as it seems likely that the expected amount of time required to change risk class would be somewhere between the short time of infection duration and the long time of demographic change. With the benefit of hindsight (see below), we tentatively take them to be slow rates and justify this choice later; hence, we define dimensionless counterparts
ψ=Ψμ,Σ=Ω+Ψμ, | (14) |
where the combined rate constant Ω+Ψ is used rather than Ω because Ω occurs in the model (10) only as Ω+Ψ. Note that Σ can be interpreted as the expected number of times an individual changes their risk classification, while ψ is the expected fraction of lifespan required for the transition from low risk to high risk.
With all the definitions given here, the scaled model is
S′=1−S−ϵ−1bQIN,U′=f+ψS−(Σ+1)U−ϵ−1bUIN,ϵE′=−(ρ+ϵ)E+bQIN,ϵI′=ρE−I,N′=1−N−ϵ−1mI, | (15) |
where the prime symbol indicates the derivative with respect to dimensionless time t.
The asymptotic parameter ϵ appears on the left side of the differential equations for E and I as a timescale parameter, done in anticipation of these being identified as fast equations, that is, equations for variables that change rapidly in the epidemic phase, as seen in Figure 1.
Asymptotics is based on the idea that terms of O(ϵ) are less important than terms of O(1) in the limit ϵ→0. This only works if no quantities other than ϵ are arbitrarily small.9 If a problem is properly scaled, the reduced problem obtained from ϵ=0 should make sense on the long timescale. Here it does not. In the N equation, for example, there is a term −ϵ−1mI that appears to dominate the others if quantities other than ϵ are O(1). It therefore appears that the leading order approximation to this equation is I=0, which contradicts the expectation that the term ϵ−1mI is of O(ϵ−1). On closer inspection, we see that every term with I appears to be more important than it actually should be. Terms with E are similarly mis-scaled.
9See [6].
The solution to this dilemma is to realize that the full population size is not the correct scale for the infected classes E and I, as was apparent in Figure 1. Everything makes sense if we assume these quantities are O(ϵ) rather than O(1). Thus, we rescale the infected class variables using the substitutions
E=ϵX,I=ϵY. | (16) |
With these new variables, we obtain the correctly scaled model,
ϵX′=−(ρ+ϵ)X+bQYN,ϵY′=ρX−Y,S′=1−S−bQYN,U′=f+ψS−ˉΣU−bUYN,N′=1−N−mY, | (17) |
where ˉΣ=Σ+1 and the system has been reordered to put the fast equations first, as per guideline 5.
At this stage, we can see why it was best to think of the transitions between low and high risk susceptibles, ΩU and ΨP, as slow processes. Had we assumed they were fast processes and defined ˜ψ=Ψ/(γ+δ+μ) instead of the dimensionless parameter ψ that we actually used, this would have given us the term ϵ−1˜ψS instead of ψS. Since we cannot assume S=O(ϵ) without messing up the S equation, we would have had to fix the problem by redefining ψ=ϵ−1˜ψ=O(1), taking us to where we ended up with the original choice of scale for Ψ. This is not something we can expect to anticipate. Regardless of whether we make the right guess for the timescale of risk class change, we end up in the right place if we are careful to check that all terms have sizes given by the number of factors of ϵ.
Note that the total population equation, after rescaling, is
N=S+R+ϵX+ϵY. |
To leading order, this is simply R=N−S−O(ϵ). Hence, S≤N is required, but no required upper bounds for X and Y are apparent at this stage.
For algebraic convenience (guideline 7), we define
κ=σb,h=(1−σ)b,ˉw=w+1, | (18) |
where w is any generic quantity.
The DFE has X=Y=0, S=N=1, U=(ψ+f)/ˉΣ. The basic reproduction number, which would have been bS if all susceptibles were high risk, is instead10
10See Appendix A.
R0=bQ=h(ψ+f)+κˉΣˉΣ. | (19) |
It is convenient to define a parameter c to be the numerator of the fraction representing R0−1; thus,
c=h(ψ+f)−(1−κ)ˉΣ. | (20) |
Here we omit the routine demonstration via eigenvalues of the Jacobian that the DFE is stable if c<0 and unstable if c>0. We should expect a unique EDE when c>0, but this needs to be demonstrated. Similarly, there are usually no endemic disease equilibria when c<0, but this property has to be checked.
The equilibrium equations for Y′=0, X′=0, N′=0, and S′=0 yield
bQN∼1,ρX∼Y,N+mY=1,S+Y∼1, |
where the asymptotic operator ∼ indicates equality to leading order. We define new quantities
y=YN,s=SN,u=UN,p=PN,q=QN | (21) |
z=by,v=σz=κy,r=hy,w=Σ+z; | (22) |
these quantities add new symbols to the lexicon of the analysis, but their use reduces the number of symbols that appear in formulas used in the analysis. In terms of these quantities, we have
bq∼1,1N=1+my,s∼1−(1−m)y | (23) |
to leading order. From the relations q=σs+(1−σ)u and q=s+(1−σ)p, we obtain additional useful formulas
hu∼1−κ+(1−m)σz,hp∼bs−1. | (24) |
To complete the specification of the EDE, 11 we substitute the formulas for 1/N, s, and u into the equation U′=0 to get the leading order equation,
11See Appendix B.
(1−m)σz2+[(1−κ)+(1−m)σˉΣ+(1−m)(1−σ)ψ−(1−σ)mf]z=c, | (25) |
or
G(z)=0, | (26) |
where
G(z)≡(1−m)σz2+[(1−κ)+(1−m)σˉΣ+(1−m)(1−σ)ψ−(1−σ)mf]z+(1−κ)ˉΣ−h(ψ+f). |
Gumel found a complicated formula, involving all the parameters of the original model, for the condition required for a backward bifurcation [2]. The complexity of that condition makes interpretation difficult. Here we show that backward bifurcation requires unrealistically extreme parameter values.12 Analysis of the leading order model is sufficient for this purpose, as the corresponding result for the original model would be merely an O(ϵ) correction to the leading order result.
12This is not a pejorative statement, nor is it a value judgment, but is based on the assumptions used to build the model. At the end of this subsection, we explain why the model we are using would be inappropriate with such a large disease-related death rate.
Proposition 1. In the asymptotic limit ϵ→0, endemic disease equilibria for the model (17) with R0≤1 can occur only with the combination m>κ and m>0.75.
Proof. Guideline 6 is the key to establishing Proposition 1. We first note that solutions of the equation for endemic disease equilibria depend continuously on the model parameters, with the consequence that positive solutions with c<0 (i.e., R0<1) occur if and only if the second solution for the case c=0 (the other being z=0) is positive. As the leading coefficient is positive, the non-zero solution is positive if the middle coefficient is negative; hence, we assume that the middle coefficient of G(z) is negative while c=0 and identify the implications of this assumption. Multiplying through by b and using κ=σb, this means that we require
(b−κ)mf>(1−m)κˉΣ+(1−m)(b−κ)ψ+b(1−κ) |
while
(1−κ)ˉΣ=(b−κ)(ψ+f). |
Multiplying the inequality by 1−κ and substituting from the equality yields the inequality
(b−κ)m(1−κ)f>(1−m)κ(b−κ)(ψ+f)+(1−m)(b−κ)(1−κ)ψ+b(1−κ)2, |
which we can rearrange to get
b(1−κ)2+(1−m)(b−κ)ψ<(m−κ)(b−κ)f. |
We see that this inequality requires m>κ. With the restrictions ψ≥0 and f≤1 that are part of the model assumptions, we obtain
b(1−κ)2<(m−κ)(b−κ) |
as a necessary condition for EDE existence. Rearranging yields
κ(m−κ)<b(m−κ)−b(1−κ)2=b(m−1+κ−κ2). |
The left-hand side of this inequality is already known to be positive, so the right-hand side must also be positive. Thus, we must have
m>1−κ+κ2=34+(12−κ)2≥34. |
To complete the description of existence and uniqueness properties, we consider the possibilities for solutions of (25) when R0>1.
Proposition 2. In the asymptotic limit ϵ→0, there is a unique EDE for the model (17) that satisfies all restrictions on the signs of the state variables whenever R0>1.
Proof. It is immediately clear that the equation (25) has a unique positive solution when c>0, but it still needs to be shown that the solution is always biologically meaningful. The critical requirement is p≥0, which through (24) and (23) corresponds to
z∗≤b−11−m≡ˆz, | (27) |
where z∗ is the positive root of the function G defined in (26). Given that G is a quadratic function that is negative at z=0, 0 at z=z∗, and becoming infinite as z→∞, the requirement z∗≤ˆz is equivalent to
G(ˆz)≥0. |
Replacing products (1−m)ˆz by b−1, we have
G(ˆz)=σ(b−1)ˆz+(1−σb)ˆz+σ(b−1)ˉΣ+(1−σ)(b−1)ψ−(1−σ)mfˆz+(1−σb)ˉΣ−(1−σ)bψ−(1−σ)bf, |
which simplifies to
G(ˆz)=(1−σ)ˆz+(1−σ)ˉΣ−(1−σ)ψ−(1−σ)f(b+mˆz)>(1−σ)[ˆz−f(b+mˆz]). |
Given the parameter restriction f≤1, along with (27), we have
G(ˆz)>(1−σ)[(1−m)ˆz+1−b]=0, |
as required.
The results of Propositions 1 and 2 are very important from a modeling standpoint. While backward bifurcation and nonuniqueness of equilibria are important features of the mathematical problem (8), they are not important features of the epidemiological setting from which the mathematical problem was derived. These interesting mathematical results are only possible if m>0.75. This means that more than 75% of people infected with the disease die of it; of known epidemic diseases, only the pneumonic plague might possibly have had a mortality fraction this high.13 Worse yet, the model assumptions of recruitment and encounter rates that do not depend on the total population are inappropriate for a disease with such high mortality. In reality, a population drastically reduced by disease mortality can be expected to have a much lower birth rate and a much lower average number of encounters per day than one not so reduced. Different assumptions about these processes are required for such a disease, leading to a different model than the one considered here.
13According to the World Health Organization, plague killed 30–60% of the population in affected cities in Europe. People who were not infected are included in this statistic, so the actual mortality fraction among the infected would have been a little bit higher, even possibly higher than 75% [13].
Given that we now know that there is a unique EDE if and only if R0>1, it seems likely that this EDE is always stable. To confirm this conjecture, we begin by identifying a set of three stability requirements, given as inequalities in terms of the EDE solution y and the other parameters. These follow from the use of the three tools described in Section 3 in accordance with guideline 10.
Proposition 3. An EDE for the model (17) is locally asymptotically stable in the asymptotic limit ϵ→0 if and only if A,B,C>0, where
A=(κ−m)+bhu,B=A+(κ−m)w+mhuz+hψ. | (28) |
C=ˉρA+ˉρ2(Aˉv+Aˉw−B)−ρyA2. | (29) |
Proof. The first step in the determination of these stability criteria is to obtain the characteristic polynomial, which is (see Section C for a detailed derivation)
P(λ)=λ5+k1Γλ4+k2Γλ3+k3Γ2λ2+k4Γ2λ+k5Γ2, | (30) |
to leading order, where
k1=ˉρ≡ρ+1,k2=1+k1+ˉρ(ˉv+ˉw),k3=ρyA,k5=ρyB,k4=k3+k5, | (31) |
Neglecting all lower-order terms, the corresponding Routh array takes the simplified form
1k2Γk4Γ2k1Γk3Γ2k5Γ2q1k1Γk4Γ2q2q1Γ2k5Γ2k4Γ2k5Γ2, | (32) |
where
q1=k1k2−k3,q2=k3q1−k21k4. | (33) |
The quantity k1 is already known to be positive. Given the requirements q1>0 and k4>0, the requirement q2>0 shows that k3>0 is also required. Since the formula for k3 is simpler than that for q1, we need only to check k3>0 and q2>0 to confirm q1>0, and of course we have to check k5>0. The requirement k4>0 is automatically satisfied when k3>0 and k5>0. Using the simplifying notation introduced earlier, we are left with three conditions: A>0, B>0, and C>0, where
ρyC=q2=k3(k1k2−k3)−k21k4=k1(k2−k1)k3−k21k5−k23. |
Substituting in the formulas for the k's yields the formula given in (29).
The three stability criteria given in Proposition 3 can be used to determine stability of the equilibrium solution for any specific set of parameters. However, with careful algebra we can establish a strong result (see Section D for the proof):
Proposition 4. If m≤κ or m≤0.75, any EDE for the model (17) is locally asymptotically stable in the asymptotic limit ϵ→0.
The combination of this result with Propositions 1 and 2 establishes that the result of the model analysis is straightforward in the realistic case m<0.75, with a unique stable EDE if R0>1 and a stable DFE if R0<1.
We now consider a model of variant competition, inspired by, although not specifically designed for, the possibility that the first omicron variant of COVID-19 might have been less infectious to fully susceptible individuals than the delta variant that it replaced. The model is a simplified version of one designed to illustrate cross-immunity [14].
For this simplest model of variant competition, suppose there is a resident variant I and an invading variant J. Individuals who recover from the resident variant move to the partially-recovered class P, where they remain susceptible to the invading variant. Individuals who recover from the invading variant move to the fully-recovered class F, regardless of whether they have previously been infected by the resident variant. Relative to the infectiousness of the resident variant toward the fully susceptible, the infectiousness of the invading variant is a factor 0<κ<1 toward susceptibles and a factor 0<δ≤κ toward partially-recovered individuals. Fully-recovered individuals can lose immunity to the invading variant, moving them into the partially-recovered class, but nobody can lose immunity to the resident variant. Finally, we assume that there is no mortality due to either variant14 and that the mean recovery time from both variants is the same.
14The model is inspired by COVID-19, but it is obviously not appropriate for this disease.
More features could be added to the model if we had a particular disease in mind and detailed data on a particular pair of disease variants or if we wanted to focus on the effects of differences in mortality or recovery rate. In this investigation, we want to see if the limited features we are including are sufficient to yield an outcome that favors the variant with the lower basic reproduction number. The invading variant has the advantage of being infectious toward some recovered individuals to compensate for the disadvantage of being less infectious toward fully susceptible individuals. With these assumptions, it seems plausible that different portions of the parameter space could yield a variety of outcomes, including stable equilibria that include only one of the two variants, a stable coexistence equilibrium, and possibly even an unstable coexistence equilibrium with a stable limit cycle.
The model is illustrated in the schematic diagram of Figure 3.
From Figure 3, the model is
dIdT=−(γ+μ)I+βSI, | (34) |
dJdT=−(γ+μ)J+κβSJ+δβPJ, | (35) |
dSdT=μ(1−S)−βSI−κβSJ, | (36) |
dPdT=γI−μP+θF−δβPJ, | (37) |
dFdT=γJ−(θ+μ)F, | (38) |
1=S+I+J+P+F. | (39) |
Note that the constant birth rate has been assigned the same symbol as the natural death rate. This makes the population constant; without loss of generality, we can think of the populations having been scaled so that the total population is N=1.
Quantity | Reference | Dimension | Definition |
F | Fig 3 | 1 | Fully removed population |
I | Fig 3 | 1 | Infectious (resident variant) population |
J | Fig 3 | 1 | Infectious (invading variant) |
P | Fig 3 | 1 | Partly removed population |
S | Fig 3 | 1 | Susceptible population |
T | Fig 3 | T | Time |
β | Fig 3 | T−1 | Rate coefficient for infection |
γ | Fig 3 | T−1 | Rate coefficient for recovery |
δ | Fig 3 | T−1 | Relative susceptibility of P to J |
θ | Fig 3 | T−1 | Rate coefficient for loss of immunity |
κ | Fig 3 | 1 | Relative susceptibility of S to J |
μ | Fig 3 | T−1 | Rate coefficient for birth and death |
Quantity | Reference | Interpretation |
F, P, S, δ, κ | See Table 4. | |
t=μT | (40) | Dimensionless time, that is, time measured in lifespans |
Q=κS+δP | (45) | Effective susceptible population for Z |
X=Y+κZ | (45) | Effective infectious population for S |
Y=ϵ−1I | (43) | Rescaled infectious (resident) population |
Z=ϵ−1J | (43) | Rescaled infectious (invader) population |
b=β/(γ+μ) | (40) | Dimensionless infection rate coefficient; maximum R0 |
h=θ/μ | (40) | Dimensionless immunity loss rate coefficient |
ˉh=h+1 | (41) | |
ϵ=μ/(γ+μ) | (40) | Ratio of expected disease duration to expected host lifespan |
Quantities | Reference | Quantities | Reference | Quantities | Reference |
y,z,s,p,f | (47) | x,q,ξ | (52) | k1,…,k4 | (63) |
η,ζ,ψ | (61) | q1,q2 | (65) |
There are four dependent variables after using the algebraic equation to eliminate F from the system. A full stability analysis for systems of this size can be done using the RH criteria without asymptotics, but the algebra can be obscure and close to unmanageable. Instead, we can achieve approximate stability boundaries in a relatively simple form by making use of asymptotic approximation. In preparation for this, we need to scale the time and rescale the two infected class populations.
We consider the loss of immunity and natural demographic processes to operate on a long timescale of years, or at least months, while the infection and recovery processes operate on a short timescale of weeks. Thus, we have slow rates μ and θ and fast rates γ and β, along with rates that combine multiple processes, such as the fast rate γ+μ that represents the rate by which individuals leave either infectious class. We choose μ and γ+μ as the best rates to represent slow and fast dynamics and choose 1/μ as the timescale for the dimensionless version of the problem. In the nondimensionalization, we refer other rates to the appropriate principal rate according to whether the rate in question is fast or slow.
Our choices dictate parameter definitions and variable change
ϵ=μγ+μ,b=βγ+μ,h=θμ,t=μT. | (40) |
As in the Two Risk-Group model, the parameter ϵ is the ratio of the principal slow rate μ, which represents mean lifespan, to the principal fast rate γ+μ, which represents mean time in the infectious class. In addition, b proves to be the basic reproduction number for the resident variant and h is the ratio of lifespan to mean time for loss of immunity. We expect ϵ to be less than 0.001 (which, with a life expectancy of 80 years, would require an infections period lasting a whole month) and b>1, while h could vary greatly from one disease to another. To simplify algebraic notation, we adopt the convention of putting a bar over the top to indicate adding 1 to any other quantity; for example,
ˉh=h+1. | (41) |
At this stage, the scaled model is
ϵI′=−I+bSI,ϵJ′=−J+b(κS+δP)J,S′=1−S−ϵ−1bS(I+κJ),P′=(ϵ−1−1)I−P+hF−ϵ−1δbPJ,F′=(ϵ−1−1)J−ˉhF,1=S+P+F+I+J. | (42) |
As with the Two Risk-Group model, stability analysis of the Variant Competition model requires a rescaling of the infected populations, which we do using the substitutions
I=ϵY,J=ϵZ. | (43) |
With these new variables, we obtain the correctly scaled model,
ϵY′=−Y+bSY,ϵZ′=−Z+bQZ,S′=1−S−bSX,P′=(1−ϵ)Y−P+hF−δbPZ,F′=(1−ϵ)Z−ˉhF,1=S+P+F+ϵY+ϵZ, | (44) |
where
X=Y+κZ,Q=κS+δP | (45) |
have been introduced because it is algebraically better to have more symbols in a model than more terms in a formula.
As before, ϵ appears on the left side of the first two differential equations as a timescale parameter, where it signifies a fast variable, and on the right side of the last equations as a regular parameter, where it represents a small perturbation. In the analysis that follows, we define Γ=1/ϵ and do asymptotic approximation in the limit Γ→∞. Removing terms of O(ϵ) from (44) yields the leading order problem
ϵY′=−Y+bSY,ϵZ′=−Z+bQZ,S′=1−S−bSX,P′=Y−P+hF−δbPZ,F′=Z−ˉhF,1=S+P+F. | (46) |
The model (46) has one DFE and as many as three endemic disease equilibria, which we call EDE-Y, EDE-Z, and EDE-YZ, with the letters after the dash indicating which of the infectious populations are non-zero. In calculating these equilibria, we must be careful to check that S, P, F and Y are all non-negative, with the non-negativity of Z following from that for F.
It is convenient to define quantities
y=bY,z=bZ,s=bS,p=bP,f=bF,q=bQ,x=bX, | (47) |
as the equilibrium equations for the endemic disease equilibria are a little simpler with these modified variables.
The DFE is easily determined by setting Y=Z=0, thereby obtaining also F=P=0 and S=1.
With Z=0 and Y≠0, we obtain the solution
s=1,y=p=b−1,f=0 |
for EDE-Y. The only requirement for existence is b>1. Thus, the basic reproduction number for the resident variant is R0r=b.
With Y=0 and Z≠0, we quickly obtain results
q=1,z=ˉhf,x=κz. |
The equations κs+δp=1 and s+p+f=b then allow us to write p and f in terms of s:
δp=1−κs,δf=(δb−1)+(κ−δ)s. | (48) |
We then have
δx=κδz=ˉhκδf=ˉhκ(δb−1)+ˉhκ(κ−δ)s. |
Substituting this result into the remaining equation, sx+s=b, and multiplying by δ to clear any fractions, yields a quadratic equation for s:
G(s)=ˉhκ(κ−δ)s2+[ˉhκ(δb−1)+δ]s−δb=0. | (49) |
This equation has a unique positive solution s∗, but we still need to check that p and f are non-negative.
The requirement p≥0 is equivalent to κs∗≤1. Now note that G<0 for s<s∗ and G>0 for s>s∗; hence, the requirement κs∗≤1 is equivalent to G(1/κ)≥0. Now
κG(1κ)=ˉh(κ−δ)+[ˉhκ(δb−1)+δ]−κδb=hδ(κb−1); |
hence, p≥0 iff κb≥1.
Similarly, f≥0 is equivalent to (κ−δ)s∗≥1−δb, or
G(1−δbκ−δ)≥0. |
A similar calculation as before shows that this inequality is always satisfied when κb≥1. Hence, the existence of the EDE-Z equilibrium requires κb≥1, which confirms that the basic reproduction number for the invading variant is R0i=κb.
If neither y nor z is 0, then we must have s=1 and q=1. The equations work out to the solution
s=1,δp=1−κ,x=b−1,f=x−p,z=ˉhf,y=x−κz. | (50) |
For this equilibrium to exist, we must have both f>0 and y>0. These requirements lead to bounds on x: x>p and x>κz. The first of these comes down to δ(b−1)>1−κ, while the second is (ˉhκ−1)δx<ˉhκδp. This could be satisfied either by ˉhκ<1 or by
δ(b−1)<ˉhκ(1−κ)ˉhκ−1. |
In the latter case, we have two upper bounds on δ, the other being δ≤κ. By identifying the point where the two upper bounds match, we obtain the following:
1) If 1b≤κ≤h+bˉhb, then existence of EDE-YZ requires 1−κb−1<δ≤κ.
2) If h+bˉhb≤κ≤1, then existence of EDE-YZ requires 1−κb−1<δ<ˉhκ(1−κ)(ˉhκ−1)(b−1).
Figure 4 shows the existence regions for the endemic equilibria with b=3, h=0 (left), b=3, h=2, (center), and b=6, h=4 (right) in the κδ plane. Larger values of b move the boundary between regions 1 and 2 to the left, while larger values of h move the boundary between regions 3 and 4 to the left. Thus, shorter times for immunity loss tend to suppress the coexistence equilibrium in favor of the invader-only equilibrium, although it is not yet clear what that does to the stability of the various endemic disease equilibria. Note that the region δ>κ is not appropriate for the biological problem, as that would mean that prior infection by the resident variant increases susceptibility to the invading variant.
For stability, we can use the equation
1=S+P+F+O(ϵ) |
to eliminate F from (46), leaving state variables Y, Z, S, and P. The Jacobian for this system is
J=(−(1−s)Γ0yΓ00−(1−q)ΓκzΓδzΓ−s−κs−ˉx01−δp−h−ξ), | (51) |
where
x=y+κz,q=κs+δp,ξ=ˉh+δz. | (52) |
The Jacobian for the DFE is lower triangular, with diagonal entries (−1+b)Γ, (−1+κb)Γ, −1, and −ˉh. Given the problem requirement κ<1, the binding stability requirement is b<1; of course b is the basic reproduction number for variant 1 and κb is that for variant 2 when variant 1 is absent.
For the EDE-Y equilibrium, the Jacobian is
J=(00yΓ00(−1+q)Γ00−1−κ−b01−δ(b−1)−h−ˉh). | (53) |
The eigenvalue problem for this system decouples into three smaller problems, a degree 1 problem with eigenvalue (−1+q)Γ, a degree 1 problem with eigenvalue −ˉh and a degree 2 problem using the sub-Jacobian that omits rows 2 and 4 and columns 2 and 4,
J13=(0yΓ−1−b). | (54) |
This matrix has negative trace and positive determinant, so the corresponding eigenvalues are negative. The sole stability criterion is q<1, or
δ(b−1)+κ<1. | (55) |
This equilibrium is therefore stable in regions 1 and 2, but not in regions 3 and 4.
Note that the instability of the EDE-Y equilibrium when (55) is not satisfied means that the inequality
δ(b−1)+κ>1 | (56) |
is the criterion for successful invasion of the invading variant into a population with the resident variant. One might have expected a greater rate of immunity loss (h) to benefit the invader; it may still lead to a larger invader population, but it does not enhance the ability of the invading variant to invade.
EDE-Z has Jacobian
J=(−(1−s)Γ00000κzΓδzΓ−s−κs−ˉx01−δp−h−ξ), | (57) |
where
δp=1−κs,δz=ˉh[(δb−1)+(κ−δ)s],x=1+κz,ξ=ˉh+δz, |
and s comes from the quadratic equation G(s)=0 (49). As with EDE-Y, the system is decoupled, this time with eigenvalue (−1+s)Γ and three eigenvalues coming from
J234=(0κzΓδzΓ−κs−ˉx0−δp−h−ξ). | (58) |
Given the characteristic polynomial formula
P(λ)=λ3+c1λ2+c2λ+c3, |
we have
c1=ˉx+ξ,c2=(κ2s+δ2p)Γz+ˉxξ,c3=(κ2sξ+δ2pˉx−hδκs)Γz. |
The requirements c1>0, c2>0, and c1c2>c3 are immediate, and c3>0 follows from κξ>δh This leaves the criterion s<1, which is equivalent to G(1)>0, where
G(1)=(ˉhκ−1)δ(b−1)−ˉhκ(1−κ). |
This comes down to
δ(b−1)(ˉhκ−1)>ˉhκ(1−κ). | (59) |
Thus, EDE-Z is stable in region 4 and unstable in regions 2 and 3. Note that region 4 disappears if h=0; hence, with no loss of immunity to the invading variant, the resident strain is always persistent.
The Jacobian for EDE-YZ is
J=(00yΓ000κzΓδzΓ−1−κ−b01−δp−h−ξ), | (60) |
where p, z, and y are given in (50). Instead of computing the characteristic polynomial for J as the determinant of λI−J, we use the characteristic polynomial theorem [6] along with asymptotic approximation to find just the leading order characteristic polynomial coefficients.
There are only a small number of non-zero terms in the matrix, and some combine together in the same way for more than one coefficient. One such example is the product of the 4, 2 and 2, 4 elements, which appear in J24, J234, and det(J). It is helpful to look for such combinations and give them separate symbols; here we define
η=y+κ2z,ζ=δ2pz,ψ=hκδz. | (61) |
The coefficient c1 is the negative of the trace:
c1=b+ξ. |
For c2, we have four non-zero 2×2 sub-determinants, yielding
c2=(y+κ2z+δ2pz)Γ+bξ=(η+ζ)Γ+O(1). |
Continuing, we obtain
c3=(ξη+bζ−ψ)Γ,c4=δyzΓ2. |
With leading order terms for each coefficient, the characteristic polynomial is
P(λ)=λ4+k1λ3+k2Γλ2+k3Γλ+k4Γ2, | (62) |
where
k1=b+ξ,k2=η+ζ,k3=ξη+bζ−ψ,k4=δyz, | (63) |
The RH conditions are
k1>0,k4>0,q1>0,q2>0, | (64) |
where
q1=k1k2−k3,q2=k3q1−k4k21. | (65) |
The first two are clearly satisfied, and
q1=(b+ξ)(η+ζ)−(ξη+bζ−ψ)=bη+ξζ+ψ>0. | (66) |
This leaves only the condition q2>0, or
(ξη+bζ−ψ)(bη+ξζ+ψ)>δyz(b+ξ)2. | (67) |
We now know that there is a unique stable equilibrium (EDE-Y) in regions 1 and 2 of Figure 4, a unique stable equilibrium (EDE-Z) in region 4, and a possible stable equilibrium in region 3 (EDE-YZ). For the EDE-YZ equilibrium, there remains one inequality that needs to be checked. The form of the inequality as written is simple; in practice, we have to use numerical methods since the criterion is nonlinear. The parameter spaces obtained by asymptotic approximation for b=5, h=0 and b=5, h=1 are shown in the left panels of Figure 5. Each has a small region of instability (red with black border), bounded in part by the line δ=κ and lying toward the midpoint of the region where EDE-YZ exists. Sample points in the unstable region that are used for illustrative simulations are marked by black dots. In the h=1 case, the region of instability is so small that it is entirely covered by the black dot.
The center and right panels of Figure 5 examine the solution at the points of instability marked on the region plots. The initial conditions were chosen to hit the point in the limit cycle where the resident population (Y) is largest. The fully susceptible population is low at that point, relative to its average, while the partly recovered population is high. These levels cause a decrease in the resident population and an increase in the invader population. At roughly 7 years, the resident population reaches its low point while the invader population is still decreasing; however, the reduced competition from the decreasing invader is enough to reverse the decline of the resident. The resident population continues to increase while the invader population reaches its low point and begins to recover. This instability only happens for a narrow range of κ and δ very close to κ. Otherwise, the EDE-YZ solution is stable in its region of existence.
Note that loss of immunity for fully-recovered individuals (h>0) has no effect on persistence of the invader, serving instead to increase the equilibrium size of the group infected by the invader and help the invader drive the resident to extinction. As h increases beyond 1 (which corresponds to a very slow loss of immunity), the large region where both variants persist quickly diminishes in favor of a large region where the invader alone persists. By h=10, corresponding to loss of immunity with mean time of 8 years, the invader becomes dominant in most of the region where it is persistent.
To get a picture of just how changes in b and h affect the region of instability, we plot instability regions in the bκ plane with various values of h and with δ=κ; these appear in Figure 6. The top group is two pairs of curves, a blue pair that shows the instability region for h=0 with asymptotics and a black pair that shows the slightly smaller instability region for h=0 with ϵ=0.001. As h increases, the region of instability quickly shrinks.
We have now seen two examples of local stability analysis facilitated by asymptotic approximation in conjunction with the simplest formula for calculating the characteristic polynomial of a matrix and a mechanism for constructing RH conditions for polynomials of any degree. Using the characteristic polynomial coefficient theorem and the Routh array come at no cost in accuracy, while the use of asymptotic approximation comes with the cost of using an approximate result in place of an exact result. We now discuss in more detail the advantages and disadvantages of approximation.
The main advantage of asymptotic approximation is that it can simplify complex formulas to the point where intractable ones become tractable, as in the stability demonstration for the Two Risk-Group model. While this demonstration required some messy algebra, it is instructive to compare it to the alternative computation performed without benefit of asymptotics. Using the generic form for the characteristic polynomial that includes the c coefficients rather than products of the k's with factors of Γ, the entry in row 5, column 1 of the Routh array is given by q4>0, where q4 is calculated from the successive formulas
q1=c1c2−c3,q2=c3q1−c1q3,q3=c1c4−c5,q4=q2q3−c5q21. | (68) |
If these are combined into a single formula with all parentheses removed, the result is
q4=c1[c1c2c3c4+c2c3c5+2c1c4c5−c23c4−c21c24−c1c22c5−c25]. | (69) |
This is sufficiently complicated to deter anyone from attempting analytical stability analysis for systems with five components.
Now suppose we apply asymptotics from this starting point. When we replace the c's with factors of k's and Γ, we discover that the first, fourth, and fifth terms in q4 are O(Γ6), while the other four are O(Γ5) or smaller. Removing the lower order terms and simplifying reduces the formula to q4=c1c4q2. Other RH conditions have already required c1 and q2 to be positive, so the condition reduces further to the single condition c4>0, corresponding to the condition k4>0 that appeared in the same position of the Routh array with asymptotics. In our development, we showed that this condition is never binding because k4=k3+k5, a result that would have been impossible to obtain from (69).
It would have been far more difficult to obtain results in the general case similar to what we obtained using asymptotics. There remains the question of whether results that require an asymptotic limit have general value. In problems such as the Two Risk-Group model, where stability is guaranteed for any reasonable parameter values (m≤0.75), asymptotic stability as ϵ→0 must at least hold for ϵ values below some finite threshold. In epidemiological models where the slow and fast timescales are as different as the duration of a typical human disease and the typical human life span, actual values of ϵ are quite small, so there is a very high likelihood that the results hold for any realistic values of ϵ.
There is also the question of whether retaining generality in parameter values is sufficiently important to justify the complicated calculations required for a general analysis. In the Variant Competition model, the analysis found a very small portion of the parameter space where there are no stable EDEs. Had we instead looked for such a region by viewing example simulations, not knowing what parameter ranges to examine, we would have had to view quite a lot of examples in order to be lucky enough to find one with instability.
Even in the case of quantitative results, such as in the Variant Competition model of Section 5, the error caused by the approximation ϵ→0 is insignificant compared to the natural error in results caused by inexact knowledge of parameter values and neglect of minor epidemiological features that were left out of the model. This is seen in Figure 6, where the black region corresponding to ϵ=0.001 is almost visually indistinguishable from the corresponding blue region obtained by asymptotics. The value ϵ=0.001 is not unreasonably small; with an 80-year human lifespan, this corresponds to an infectious period of duration 0.08 years, corresponding to almost a month. Most diseases have a shorter infectious period, which would yield a smaller value of ϵ.
It is also worth noting that the most convenient method for obtaining the boundary for ϵ>0 relies on prior knowledge of the asymptotic stability result. Points on the boundary curves in Figure 6 are found by converting the defining inequality into a function q=f(b,h,κ,δ) whose value is positive for stability and negative for instability and then numerically solving the equation for κ using the fixed values of b and h and δ=κ. The asymptotic work identified which of the RH conditions fails at instability, allowing the function f to be obtained from a single entry of the Routh array, calculated numerically. Absent knowledge of which specific condition fails, the RH conditions could not have been used to create the function f and stability would instead have had to be determined by numerical computation of the dominant eigenvalue.
Two minor observations on the guidelines are warranted. First, one could avoid the rescaling by assuming a regular perturbation structure and accepting the non-standard possibility of a leading order term of 0, that is, using I=I0+ϵI1 rather than I=ϵY. This only works if we begin the analysis by determining the result I0=0 and then retaining the "correction" term I1 while not using a regular perturbation expansion for S, U, and N. The result is identical to what we have done here, except with the symbol I1 in place of the symbol Y. The two choices are thus mathematically equivalent, but the rescaling choice has the advantage of being conceptually more clear. Second, the introduction of so much additional notation adds a degree of inconvenience, as one must keep a list of symbol definitions handy. However, the advantage of guidelines 7 and 8 becomes clear if the reader replaces the extra symbols in the formula for the Jacobian with their definitions and then tries to obtain the characteristic polynomial coefficients. It is far better to have more symbols in the table of notation than to have more symbols in the formulas needed for analysis.
There is no reason why the method presented here should be restricted to epidemiological models. Any model that has processes with timescales that are significantly different could be approached with the same method. In a food web model, for example, there could be organisms with a very short life span, such as microorganisms, along with organisms with much longer life spans. It is common for predator species to have a longer lifespan than their prey, but we should be wary of using the asymptotic approach if the ratio of the lifespans is on the order of 10 rather than 1000, as we generally have in epidemiology models. But even in these less clear cases, the asymptotic approach can give useful approximations, as long as they are not taken to be quantitatively accurate.
The authors declare they have not used Artificial Intelligence (AI) tools in the creation of this article.
Glenn Ledder is a guest editor for Mathematical Biosciences and Engineering and was not involved in the editorial review or the decision to publish this article. The author declares there is no conflict of interest.
The most straightforward way to calculate the basic reproduction number is to use its definition as the expected number of secondary infections per primary infective in an otherwise fully-susceptible population. In the model (17), new infections are created at rate ϵ−1bQY/N, or ϵ−1bQ/N per infectious individual. The expected duration of time in the infectious compartment is ϵ−1; hence, the expected number of secondary infections per infective is bQ/N, leading to the result
R0=bQN, |
where the state variables Q and N are evaluated at the DFE. We have N=1 at the DFE, along with
Q=(1−σ)U+σS=(1−σ)(ψ+f)+σˉΣˉΣ. |
Multiplying by b and using (18) yields (19).
Beginning with the U′=0 equation, we divide by N and substitute from (21) and (22) to get
(ˉΣ+z)u=ψs+fN. | (70) |
Multiplying by h=(1−σ)b then yields
(ˉΣ+z)hu=(1−σ)ψbs+(1−σ)fbN. | (71) |
From (23), we have
bN=b+mby=b+mz,bs=b−(1−m)by=b−(1−m)z. |
Substituting these formulas and (24) into (71) yields
(ˉΣ+z)[(1−κ)+(1−m)σz]=(1−σ)ψ[b−(1−m)z]+(1−σ)f(b+mz), |
after which gathering like terms yields (26).
The Jacobian, given in terms of the additional symbol definitions of (18), (21), and (22), takes the compact form
J=(−(ρΓ+1)ΓvΓrΓ−yΓρΓ−Γ0000−1−ˉv−ry0−buψ−ˉwuz0−m00−1), | (72) |
where Γ=1/ϵ. The leading order characteristic polynomial is obtained from this matrix by a combination of the characteristic polynomial theorem (Theorem 1) and asymptotic approximation.
The coefficient c1 is the negative of the trace. Some terms are O(Γ), while others are O(1). To leading order, we need only to consider the former; hence,
c1=ρΓ+Γ+O(1)=ˉρΓ+O(1). | (73) |
At first glance, c12 appears to be dominated by an O(Γ2) term coming from J12; however, the terms of that order cancel, giving J12=Γ. This means that the other O(Γ) terms, coming from sub-determinants with indices 1 or 2 paired with 3, 4, or 5, also contribute to the leading order approximation. The result is
c2=[1+ˉρ(1+ˉv+ˉw)]Γ+O(1). | (74) |
Note that we need the second term in the 11 entry even though it is subdominant to the first term. That second term is not needed for any other characteristic polynomial coefficients, but it was necessary to retain it in the Jacobian.
The coefficient c3 is dominated by terms of O(Γ2) that come from the three sub-determinants of form J12j. These conform to a simple pattern:
c3∼−(J123+J124+J125)=ρΓ2[g(κy,1,ˉv)+g(hy,bu,ˉw)+g(−y,m,1)], |
where
g(D,E,F)=−|−11D1−100−E−F|=DE. |
Thus,
c3=ρyAΓ2+O(Γ), | (75) |
where A was defined in (28).
The full determinant calculation yields
c5=−|J|=ρyBΓ2, | (76) |
with B given in (28).
The coefficient c4 is the sum of the 4×4 sub-determinants of J. The three (of the total of five) that include both rows 1 and 2 contribute terms of O(Γ2). Each of the three required sub-determinants is described by the template
J12ij=(−ρΓΓˆAyΓˆByΓρΓ−Γ000−ˆC−ˆEˆG0−ˆDˆH−ˆF). |
Cofactor expansion on the first column yields
J12ij=−ρΓ(−Γ00−ˆC−ˆEˆG−ˆDˆH−ˆF)−ρΓ(ΓˆAyΓˆByΓ−ˆC−ˆEˆG−ˆDˆH−ˆF)=−ρyΓ2(0ˆAˆB−ˆC−ˆEˆG−ˆDˆH−ˆF)=ρyΓ2(ˆDˆAˆG+ˆDˆBˆE+ˆCˆAˆF+ˆCˆBˆH). |
Careful accounting of the terms in this formula from each of the three relevant sub-matrices yields the convenient result
c4=c3+c5. | (77) |
To summarize, we have found the leading order characteristic polynomial
P(λ)=λ5+k1Γλ4+k2Γλ3+k3Γ2λ2+k4Γ2λ+k5Γ2, | (78) |
where
k1=ˉρ,k2=1+k1+ˉρ(ˉv+ˉw),k3=ρyA,k5=ρyB,k4=k3+k5, | (79) |
Note that asymptotic simplification only applies to terms within each individual coefficient. We cannot compare the magnitudes of the different terms because there are eigenvalues of different orders. As an example, suppose we assume λ=O(1). The leading order characteristic polynomial is then quadratic and has two roots. Hence, only two of the eigenvalues are O(1). If instead we assume λ=O(Γ), then the dominant terms are the first and second, leading to one eigenvalue, −k1Γ. The remaining two eigenvalues are of an intermediate order. We need not be concerned with details, as we do not need to find the eigenvalues to determine stability.
The first step in establishing Proposition 4 is to obtain a tight lower bound for C in terms of a formula with form similar to that of A and B, that is, C>(κ−m)Ca+Cb, where Ca and Cb are non-negative. We begin by multiplying (70) by b, rewriting in terms of w, and using (24) and p+u=s to get
buˉw−ψ=b(ψs+fN)−ψ=ψhp+bfN=h(ψs−ψu)+bfN. |
Rewriting (70) as
(ˉz+ω)u=ψs−ψu+fN |
allows us to complete the calculation as
buˉw−ψ=hu(ˉz+ω)+bσfN, |
leading to a useful computational result:
buˉw−ψ≥huˉz. | (80) |
From
Aˉv=(κ−m)ˉv+κhuz+bhuAˉw=A+(κ−m)w+bhuwB=A+(κ−m)w+mhuz+hψ, |
we obtain
Aˉv+Aˉw−B=(κ−m)(ˉv+huz)+h(buˉw−ψ). | (81) |
Applying (80) gives the result
Aˉv+Aˉw−B≥(κ−m)(ˉv+huz)+h2uˉz | (82) |
Next, we write
yA2=(κ−m)2y+2(κ−m)bhuy+b2h2u2y=(κ−m)(κy−my+2huz)+bh2u2z. |
Then u≤q and bq=1 combine to give bu≤1, so
yA2≤(κ−m)(v+2huz−my)+h2uz, | (83) |
Finally, we substitute (82) and (83) into (29) to obtain the desired lower bound
C≥(κ−m)[ˉρ2+(ˉρ2−2ρ)huz+(ˉρ2−ρ)v+ρmy]+(ˉρ2−ρ)h2uz+ˉρ2h2u+ˉρA. | (84) |
From (28) and (84), we see immediately that stability is guaranteed if m≤κ. It remains to be shown that m≤0.75 is sufficient to guarantee stability when m>κ. The first step in this demonstration is to rewrite (84) as
C≥A+ˉρ2C1+(ˉρ2−ρ)zC2+ρC3, |
where
C1=h2u−(m−κ),C2=h2u−(m−κ)(hu+σ), | (85) |
C3=bhu−(m−κ)(1+my−huz). | (86) |
Given h<b, hu+σ=(1−σ)bu+σ≤(1−σ)+σ=1, and m>κ, we have A≥C1 and C2≥C1, leaving C1>0, C3>0, and B>0 to be confirmed.
From the formula for C1 and hu∼1−κ+(1−m)σz≥1−κ, we have
C1≥(b−κ)(1−κ)+κ−m=b(1−κ)+κ2−m>14−κ+κ2=(12−κ)2≥0. |
For C3, we first note that s≥0 requires y≤1/(1−m)≤4, and we also have bhu>hu≥1−κ. If m>bhu, then
1+y(m−bhu)<1+4(34−1+κ)=4κ |
and
C3>(1−κ)−(34−κ)(4κ)=1−4κ−κ2=(1−2κ)2≥0. |
If m≤bhu, then 1+y(m−bhu)≤1 and
C3>bhu−(m−κ)=1−m>0. |
To show B≥0, we first note the result
mhu−m+κ≥m(1−κ)−m+κ=(1−m)κ≥0. |
This allows us to eliminate the z terms from (28), leaving
B≥b(1−κ)+hψ−(m−κ)ˉΣ, |
Multiplying by 1−κ and using b>h, this becomes
(1−κ)B>h(1−κ)2+(1−κ)hψ−(m−κ)(1−κ)ˉΣ. |
With m≤0.75, there is no backward bifurcation; hence, solutions occur only if c>0; along with the modeling restriction f≤1, we then have
(1−κ)ˉΣ≤hψ+h. |
Substituting into the previous inequality yields
(1−κ)B>h(1−κ)2+(1−κ)hψ−(m−κ)hψ−(m−κ)h≥h[(1−κ)2−(34−κ)]=h(12−κ)2≥0. |
This completes the proof of Proposition 4.
[1] | F. Brauer, C. Castillo-Chavez, Z. Feng, Mathematical Models in Epidemiology, Springer, 2019. https://doi.org/10.1007/978-1-4939-9828-9 |
[2] |
A. Gumel, Causes of backward bifurcations in some epidemiological models, J. Math. Anal. Appl., 395 (2012), 355–365. https://doi.org/10.1016/j.jmaa.2012.04.077 doi: 10.1016/j.jmaa.2012.04.077
![]() |
[3] | M. Martcheva, An Introduction to Mathematical Epidemiology, Springer, 2015. https://doi.org/10.1007/978-1-4899-7612-3 |
[4] |
C. Bauch, Imitation dynamics predict vaccinating behaviour, Proc. Royal Soc. B: Biol. Sci., 272 (2005), 1669–1675. https://doi.org/10.1098/rspb.2005.3153 doi: 10.1098/rspb.2005.3153
![]() |
[5] |
Y. Jiang, K. M. Kurianski, J. H. Lee, Y. Ma, D. Cicala, G. Ledder, Incorporating changeable attitudes toward vaccination into compartment models for infectious diseases, Math. Biosci. Eng., 2 (2025), 260–289. https://doi.org/10.3934/mbe.2025011 doi: 10.3934/mbe.2025011
![]() |
[6] |
G. Ledder, Scaling for dynamical systems in biology, Bull. Math. Biol., 79 (2017), 2747–2772. https://doi.org/10.1007/s11538-017-0339-5 doi: 10.1007/s11538-017-0339-5
![]() |
[7] | G. Ledder, Mathematical Modeling for Epidemiology and Ecology, Springer, New York, 2023. |
[8] |
G. Ledder, Incorporating mass vaccination into compartment models for infectious diseases, Math. Biosci. Eng., 19 (2022), 9457–9480. https://doi.org/10.3934/mbe.2022440 doi: 10.3934/mbe.2022440
![]() |
[9] | Y. Jiang, K. M. Kurianski, J. H. Lee, Y. Ma, D. Cicala, G. Ledder, Incorporating changeable attitudes toward vaccination into an SIR infectious disease model, preprint, arXiv: 2405.03931. |
[10] | C. D. Meyer, Matrix Analysis and Applied Linear Algebra, 2nd edition, SIAM, 2023. |
[11] | M. Holmes, Introduction to Perturbation Methods, Springer, 2013. |
[12] | E. Routh, A treatise on the stability of a given state of motion, in Stability of Motion (ed. A. Fuller), Taylor and Francis, London, 1975. |
[13] | World Health Organization, Plague, 2022, Accessed: 2024/12/07. Available from: https://www.who.int/news-room/fact-sheets/detail/plague. |
[14] |
C. Castillo-Chavez, H. Hethcote, V. Andreasen, S. Levin, W. Liu, Epidemiological models with age structure, proportionate mixing, and cross-immunity, J. Math. Biol., 27 (1989), 233–258. https://doi.org/10.1007/BF00275810 doi: 10.1007/BF00275810
![]() |
Quantity | Reference | Dimension | Definition |
E | Figure 2 | 1 | Latent ("exposed") population |
I | Figure 2 | 1 | Infectious population |
N | Figure 2 | 1 | Total population |
P | Figure 2 | 1 | Low-risk susceptible population |
Q=U+σP | (11) | 1 | Susceptibility fraction |
R | Figure 2 | 1 | Recovered population |
S=P+U | (10) | 1 | Total susceptible population |
T | Figure 2 | T | Time |
U | Figure 2 | 1 | High-risk susceptible population |
f | Figure 2 | 1 | fraction of recruits that are high-risk |
β | Figure 2 | T−1 | Rate coefficient for infection |
γ | Figure 2 | T−1 | Rate coefficient for recovery |
δ | Figure 2 | T−1 | Mortality probability |
η | Figure 2 | T−1 | Rate coefficient for incubation |
μ | Figure 2 | T−1 | Rate coefficient for birth and death |
σ | Figure 2 | 1 | relative low-risk susceptibility |
Ψ | Figure 2 | T−1 | Rate coefficient for transition from P to U |
Ω | Figure 2 | T−1 | Rate coefficient for transition from U to P |
Quantity | Reference | Interpretation |
N, S, Q, U, σ | See Table 1. | |
t=μT | (12) | Dimensionless time, that is, time measured in lifespans |
X=ϵ−1E | (16) | Rescaled latent ("exposed") population |
Y=ϵ−1I | (16) | Rescaled infectious population |
b=β/(γ+δ+μ) | (13) | Dimensionless infection rate coefficient; maximum R0 |
m=δ/(γ+δ+μ) | (13) | Fraction of infectious individuals who die of the disease |
ϵ=μ/(γ+δ+μ) | (13) | Ratio of expected disease duration to expected host lifespan |
ρ=η/(γ+δ+μ) | (13) | Dimensionless incubation rate coefficient |
ψ=Ψ/μ | (14) | Dimensionless rate coef. for transition from low risk to high risk |
Σ=(Ψ+Ω)/μ | (14) | Dimensionless sum of risk level transition rate coefficients |
Quantities | Reference | Quantities | Reference | Quantities | Reference |
κ,h,ˉΣ,ˉρ | (18) | y,s,u,p,q | (21) | A,B,C | Prop. 3 |
R0 | (19) | z,v,r,w | (22) | k1,…,k5 | (31) |
c | (20) | z∗,ˆz | (27) | q1,q2 | (33) |
Quantity | Reference | Dimension | Definition |
F | Fig 3 | 1 | Fully removed population |
I | Fig 3 | 1 | Infectious (resident variant) population |
J | Fig 3 | 1 | Infectious (invading variant) |
P | Fig 3 | 1 | Partly removed population |
S | Fig 3 | 1 | Susceptible population |
T | Fig 3 | T | Time |
β | Fig 3 | T−1 | Rate coefficient for infection |
γ | Fig 3 | T−1 | Rate coefficient for recovery |
δ | Fig 3 | T−1 | Relative susceptibility of P to J |
θ | Fig 3 | T−1 | Rate coefficient for loss of immunity |
κ | Fig 3 | 1 | Relative susceptibility of S to J |
μ | Fig 3 | T−1 | Rate coefficient for birth and death |
Quantity | Reference | Interpretation |
F, P, S, δ, κ | See Table 4. | |
t=μT | (40) | Dimensionless time, that is, time measured in lifespans |
Q=κS+δP | (45) | Effective susceptible population for Z |
X=Y+κZ | (45) | Effective infectious population for S |
Y=ϵ−1I | (43) | Rescaled infectious (resident) population |
Z=ϵ−1J | (43) | Rescaled infectious (invader) population |
b=β/(γ+μ) | (40) | Dimensionless infection rate coefficient; maximum R0 |
h=θ/μ | (40) | Dimensionless immunity loss rate coefficient |
ˉh=h+1 | (41) | |
ϵ=μ/(γ+μ) | (40) | Ratio of expected disease duration to expected host lifespan |
Quantities | Reference | Quantities | Reference | Quantities | Reference |
y,z,s,p,f | (47) | x,q,ξ | (52) | k1,…,k4 | (63) |
η,ζ,ψ | (61) | q1,q2 | (65) |
Quantity | Reference | Dimension | Definition |
E | Figure 2 | 1 | Latent ("exposed") population |
I | Figure 2 | 1 | Infectious population |
N | Figure 2 | 1 | Total population |
P | Figure 2 | 1 | Low-risk susceptible population |
Q=U+σP | (11) | 1 | Susceptibility fraction |
R | Figure 2 | 1 | Recovered population |
S=P+U | (10) | 1 | Total susceptible population |
T | Figure 2 | T | Time |
U | Figure 2 | 1 | High-risk susceptible population |
f | Figure 2 | 1 | fraction of recruits that are high-risk |
β | Figure 2 | T−1 | Rate coefficient for infection |
γ | Figure 2 | T−1 | Rate coefficient for recovery |
δ | Figure 2 | T−1 | Mortality probability |
η | Figure 2 | T−1 | Rate coefficient for incubation |
μ | Figure 2 | T−1 | Rate coefficient for birth and death |
σ | Figure 2 | 1 | relative low-risk susceptibility |
Ψ | Figure 2 | T−1 | Rate coefficient for transition from P to U |
Ω | Figure 2 | T−1 | Rate coefficient for transition from U to P |
Quantity | Reference | Interpretation |
N, S, Q, U, σ | See Table 1. | |
t=μT | (12) | Dimensionless time, that is, time measured in lifespans |
X=ϵ−1E | (16) | Rescaled latent ("exposed") population |
Y=ϵ−1I | (16) | Rescaled infectious population |
b=β/(γ+δ+μ) | (13) | Dimensionless infection rate coefficient; maximum R0 |
m=δ/(γ+δ+μ) | (13) | Fraction of infectious individuals who die of the disease |
ϵ=μ/(γ+δ+μ) | (13) | Ratio of expected disease duration to expected host lifespan |
ρ=η/(γ+δ+μ) | (13) | Dimensionless incubation rate coefficient |
ψ=Ψ/μ | (14) | Dimensionless rate coef. for transition from low risk to high risk |
Σ=(Ψ+Ω)/μ | (14) | Dimensionless sum of risk level transition rate coefficients |
Quantities | Reference | Quantities | Reference | Quantities | Reference |
κ,h,ˉΣ,ˉρ | (18) | y,s,u,p,q | (21) | A,B,C | Prop. 3 |
R0 | (19) | z,v,r,w | (22) | k1,…,k5 | (31) |
c | (20) | z∗,ˆz | (27) | q1,q2 | (33) |
Quantity | Reference | Dimension | Definition |
F | Fig 3 | 1 | Fully removed population |
I | Fig 3 | 1 | Infectious (resident variant) population |
J | Fig 3 | 1 | Infectious (invading variant) |
P | Fig 3 | 1 | Partly removed population |
S | Fig 3 | 1 | Susceptible population |
T | Fig 3 | T | Time |
β | Fig 3 | T−1 | Rate coefficient for infection |
γ | Fig 3 | T−1 | Rate coefficient for recovery |
δ | Fig 3 | T−1 | Relative susceptibility of P to J |
θ | Fig 3 | T−1 | Rate coefficient for loss of immunity |
κ | Fig 3 | 1 | Relative susceptibility of S to J |
μ | Fig 3 | T−1 | Rate coefficient for birth and death |
Quantity | Reference | Interpretation |
F, P, S, δ, κ | See Table 4. | |
t=μT | (40) | Dimensionless time, that is, time measured in lifespans |
Q=κS+δP | (45) | Effective susceptible population for Z |
X=Y+κZ | (45) | Effective infectious population for S |
Y=ϵ−1I | (43) | Rescaled infectious (resident) population |
Z=ϵ−1J | (43) | Rescaled infectious (invader) population |
b=β/(γ+μ) | (40) | Dimensionless infection rate coefficient; maximum R0 |
h=θ/μ | (40) | Dimensionless immunity loss rate coefficient |
ˉh=h+1 | (41) | |
ϵ=μ/(γ+μ) | (40) | Ratio of expected disease duration to expected host lifespan |
Quantities | Reference | Quantities | Reference | Quantities | Reference |
y,z,s,p,f | (47) | x,q,ξ | (52) | k1,…,k4 | (63) |
η,ζ,ψ | (61) | q1,q2 | (65) |