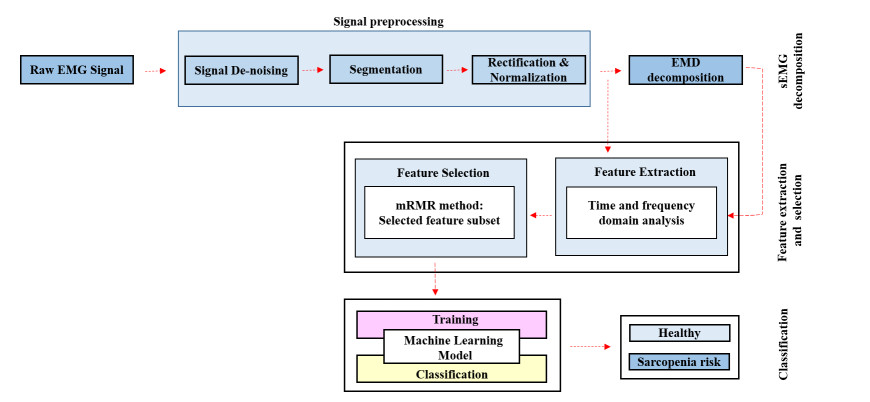
Early detection of the risk of sarcopenia at younger ages is crucial for implementing preventive strategies, fostering healthy muscle development, and minimizing the negative impact of sarcopenia on health and aging. In this study, we propose a novel sarcopenia risk detection technique that combines surface electromyography (sEMG) signals and empirical mode decomposition (EMD) with machine learning algorithms. First, we recorded and preprocessed sEMG data from both healthy and at-risk individuals during various physical activities, including normal walking, fast walking, performing a standard squat, and performing a wide squat. Next, electromyography (EMG) features were extracted from a normalized EMG and its intrinsic mode functions (IMFs) were obtained through EMD. Subsequently, a minimum redundancy maximum relevance (mRMR) feature selection method was employed to identify the most influential subset of features. Finally, the performances of state-of-the-art machine learning (ML) classifiers were evaluated using a leave-one-subject-out cross-validation technique, and the effectiveness of the classifiers for sarcopenia risk classification was assessed through various performance metrics. The proposed method shows a high accuracy, with accuracy rates of 0.88 for normal walking, 0.89 for fast walking, 0.81 for a standard squat, and 0.80 for a wide squat, providing reliable identification of sarcopenia risk during physical activities. Beyond early sarcopenia risk detection, this sEMG-EMD-ML system offers practical values for assessing muscle function, muscle health monitoring, and managing muscle quality for an improved daily life and well-being.
Citation: Konki Sravan Kumar, Daehyun Lee, Ankhzaya Jamsrandoj, Necla Nisa Soylu, Dawoon Jung, Jinwook Kim, Kyung Ryoul Mun. sEMG-based Sarcopenia risk classification using empirical mode decomposition and machine learning algorithms[J]. Mathematical Biosciences and Engineering, 2024, 21(2): 2901-2921. doi: 10.3934/mbe.2024129
[1] | Ziyang Sun, Xugang Xi, Changmin Yuan, Yong Yang, Xian Hua . Surface electromyography signal denoising via EEMD and improved wavelet thresholds. Mathematical Biosciences and Engineering, 2020, 17(6): 6945-6962. doi: 10.3934/mbe.2020359 |
[2] | Zhangjie Wu, Minming Gu . A novel attention-guided ECA-CNN architecture for sEMG-based gait classification. Mathematical Biosciences and Engineering, 2023, 20(4): 7140-7153. doi: 10.3934/mbe.2023308 |
[3] | Ting Yao, Farong Gao, Qizhong Zhang, Yuliang Ma . Multi-feature gait recognition with DNN based on sEMG signals. Mathematical Biosciences and Engineering, 2021, 18(4): 3521-3542. doi: 10.3934/mbe.2021177 |
[4] | Ravichandra Madanu, Farhan Rahman, Maysam F. Abbod, Shou-Zen Fan, Jiann-Shing Shieh . Depth of anesthesia prediction via EEG signals using convolutional neural network and ensemble empirical mode decomposition. Mathematical Biosciences and Engineering, 2021, 18(5): 5047-5068. doi: 10.3934/mbe.2021257 |
[5] | Bo Kou, Jinde Cao, Wei Huang, Tao Ma . The rutting model of semi-rigid asphalt pavement based on RIOHTRACK full-scale track. Mathematical Biosciences and Engineering, 2023, 20(5): 8124-8145. doi: 10.3934/mbe.2023353 |
[6] | Lili Jiang, Sirong Chen, Yuanhui Wu, Da Zhou, Lihua Duan . Prediction of coronary heart disease in gout patients using machine learning models. Mathematical Biosciences and Engineering, 2023, 20(3): 4574-4591. doi: 10.3934/mbe.2023212 |
[7] | Kwabena Owusu-Agyemang, Zhen Qin, Appiah Benjamin, Hu Xiong, Zhiguang Qin . Guaranteed distributed machine learning: Privacy-preserving empirical risk minimization. Mathematical Biosciences and Engineering, 2021, 18(4): 4772-4796. doi: 10.3934/mbe.2021243 |
[8] | Yufeng Qian . Exploration of machine algorithms based on deep learning model and feature extraction. Mathematical Biosciences and Engineering, 2021, 18(6): 7602-7618. doi: 10.3934/mbe.2021376 |
[9] | Hongli Niu, Yazhi Zhao . Crude oil prices and volatility prediction by a hybrid model based on kernel extreme learning machine. Mathematical Biosciences and Engineering, 2021, 18(6): 8096-8122. doi: 10.3934/mbe.2021402 |
[10] | J. Murillo-Escobar, Y. E. Jaramillo-Munera, D. A. Orrego-Metaute, E. Delgado-Trejos, D. Cuesta-Frau . Muscle fatigue analysis during dynamic contractions based on biomechanical features and Permutation Entropy. Mathematical Biosciences and Engineering, 2020, 17(3): 2592-2615. doi: 10.3934/mbe.2020142 |
Early detection of the risk of sarcopenia at younger ages is crucial for implementing preventive strategies, fostering healthy muscle development, and minimizing the negative impact of sarcopenia on health and aging. In this study, we propose a novel sarcopenia risk detection technique that combines surface electromyography (sEMG) signals and empirical mode decomposition (EMD) with machine learning algorithms. First, we recorded and preprocessed sEMG data from both healthy and at-risk individuals during various physical activities, including normal walking, fast walking, performing a standard squat, and performing a wide squat. Next, electromyography (EMG) features were extracted from a normalized EMG and its intrinsic mode functions (IMFs) were obtained through EMD. Subsequently, a minimum redundancy maximum relevance (mRMR) feature selection method was employed to identify the most influential subset of features. Finally, the performances of state-of-the-art machine learning (ML) classifiers were evaluated using a leave-one-subject-out cross-validation technique, and the effectiveness of the classifiers for sarcopenia risk classification was assessed through various performance metrics. The proposed method shows a high accuracy, with accuracy rates of 0.88 for normal walking, 0.89 for fast walking, 0.81 for a standard squat, and 0.80 for a wide squat, providing reliable identification of sarcopenia risk during physical activities. Beyond early sarcopenia risk detection, this sEMG-EMD-ML system offers practical values for assessing muscle function, muscle health monitoring, and managing muscle quality for an improved daily life and well-being.
Sarcopenia is a progressive age-related condition characterized by the loss of skeletal muscle mass, strength, and function. It is associated with an increased risk of falls, fractures, functional decline, and a reduced quality of life [1,2,3]. Sarcopenia affects approximately 50 million people worldwide, accounting for approximately 13% of individuals aged 60–70 years. Considering that the global population of individuals over 60 years is projected to double by 2050, the prevalence of sarcopenia is expected to significantly rise in the coming decades [4,5,6]. Therefore, early detection of the risk of sarcopenia at younger ages is essential for implementing preventive strategies, promoting healthy muscle development, and mitigating the adverse effects of sarcopenia on overall health and aging. It empowers individuals to take proactive steps toward maintaining muscle health and achieving better long-term outcomes [7]. A comprehensive approach to sarcopenia risk detection encompasses clinical assessments, physical performance tests, and the integration of relevant biomarkers and imaging techniques. However, this approach can be resource-intensive, time consuming, and requires specialized equipment, which may not be readily available in all health care settings. Currently, sarcopenia risk is assessed by various gold standard image modalities, such as dual-energy X-ray absorptiometry (DXA), magnetic resonance imaging (MRI), and computed tomography (CT) scans, which directly visualize and quantify muscle mass, volume, and composition. These modalities offer more precise and accurate measurements and provide detailed insights into muscle structure and distribution. Despite their widespread use, these clinical examinations appear to be underutilized due to their high cost, limited portability, radiation exposure to patients, and the need for highly skilled medical personnel [8,9,10].
Signal processing is experiencing a vibrant revival across diverse industries. Its impact is particularly evident in industrial automation, where it empowers intelligent manufacturing systems through advanced gear health monitoring techniques [11,12,13]. Simultaneously, advancements in signal processing have revolutionized healthcare, thus paving a way for innovative solutions such as wearable devices and remote patient monitoring systems [14]. Recently, the increased popularity of smart sensors has provided a solution to overcome the limitations of the aforementioned image modalities and to enhance the accessibility of devices that can be effectively employed for the early diagnosis and monitoring of patients with sarcopenia. In addition, sEMG sensors are presently employed in numerous research studies to collect human motion data during physical activities. They have been widely used in various domains, including disease prediction, health reassessment, and medical care [15,16]. sEMG is a technique that measures the electrical activity produced by skeletal muscles. It involves the use of surface electrodes placed on the skin above the muscles. These electrodes detect and record electrical signals generated by muscle fibers during muscle contraction and relaxation [17]. The study reported in [18] investigated age-related differences in the time-frequency representation of sEMG data during a submaximal cyclic back extension exercise. The objective of this study was to examine whether EMG data analysis can serve as a potential biomarker for the early detection of sarcopenia. The results revealed age-specific differences in the time-frequency representation of the EMG data during the back extension exercise. The researchers observed distinct patterns of muscle activation between the younger and older participants. These findings suggest that analyzing EMG data with a time-frequency representation can serve as a promising biomarker for detecting early signs of sarcopenia. Another study [19] demonstrated the effectiveness of supervised classifiers in evaluating sarcopenia using an sEMG-based platform. The results showed that an sEMG-based platform combined with supervised classifiers could provide a reliable means of evaluating sarcopenia. However, the study was conducted with a limited number of older adult individuals suffering from sarcopenia and did not involve young people who were at risk of sarcopenia, thus limiting its connection to the early detection of sarcopenia. Furthermore, in the study, the performance comparison of the classification algorithms solely considered synthesized data generated from a minority oversampling technology (SMOTE) along with the edited nearest neighbor's technique. Although existing research has demonstrated the potential of sEMG and machine learning (ML) for sarcopenia assessment, there are still existing gaps in the research. These include a lack of previous studies focused on the early detection of sarcopenia before the onset of significant clinical symptoms and limited investigations using the decomposition method to enhance feature extraction in sEMG signal classification.
Our research aims to address these gaps by providing an sEMG dataset recorded during physical exercises for the early detection of sarcopenia, investigating the effectiveness of empirical mode decomposition (EMD) in pre-processing sEMG signals for improved sarcopenia risk classification, and exploring the performance of various ML algorithms in classifying young individuals with and without a risk of sarcopenia based on EMD-derived features.
This section presents the workflow of the proposed method for detecting sarcopenia risk during daily activities, as shown in Figure 1. First, wearable sEMG signal sensors were employed to record the sEMG signals from the participants during daily activities. The raw sEMG signal underwent de-noising, segmentation, normalization, and rectification through preprocessing. Subsequently, the preprocessed sEMG signal was decomposed into intrinsic mode functions (IMFs) using EMD. The time domain, frequency domain, and time-frequency domain features were extracted from each IMF. Then, a feature selection method was applied to identify the optimal subset of features. Finally, a binary classifier was employed to classify individuals into healthy and sarcopenia-risk groups based on the extracted features.
Excluding those who were illiterate, a total of 93 community-dwelling adults, aged between 20 and 60 years old, were recruited for this study from the Kyung Hee University Medical Center, South Korea. This study included 44 men (47.3%) and 49 women (52.7%). Before participation, each individual provided an informed consent form, which included approval for the removal of hair from the quadriceps and hamstring areas for the attachment of sEMG sensors. The exclusion criteria were rigorously defined to include individuals with the following: (1) chronic kidney failure or end-stage renal disease, or those undergoing hemodialysis; (2) a history of cancer within the past five years or those currently undergoing chemotherapy; (3) current hormone therapy; (4) a myocardial infarction or stroke within the past six months; (5) a pacemaker implant; (6) physical disabilities affecting the upper or lower limbs; (7) pregnant or breastfeeding women, or those planning pregnancy during the trial period; and (8) an inability to comply with the study requirements or deemed unsuitable by the principal investigator or physician. The characteristics of each group are detailed in Table 1. The study enrolled 93 subjects aged 20–59 years, categorized into a control group (n = 49) and a sarcopenia risk group (n = 44). Statistical analyses revealed that the control group exhibited significantly higher values in weight, body mass index (BMI), appendicular skeletal muscle mass index (ASMI) (p < 0.001), and knee-extension force (p < 0.05) when compared to the sarcopenia risk group. However, no significant differences were observed in terms of age and height between the two groups.
Characteristics | Total (n = 93) | Control group (n = 49) | Sarcopenia risk group (n = 44) | p-value |
Men (%) | 93 (47.3) | 26 (53.1) | 18 (40.9) | 0.300 |
Age (years) | 39.0 (29.0–49.5) | 40.0 (29.0–49.5) | 37.0 (27.5–49.8) | 0.732 |
Weight (kg) | 59.4 (53.1–66.4) | 65.0 (57.4–74.7) | 53.4 (48.6–60.9) | < 0.001 |
Height (cm) | 165.3 (160.9–174.5) | 167.2 (160.9–175.3) | 163.9 (160.9–173.2) | 0.723 |
BMI (kg/cm2) | 21.5 (19.7–23.) | 22.2–25.4 | 19.6 (18.5–20.4) | < 0.001 |
ASMI (kg/m2) | 6.6 (6.0–7.6) | 7.4 (6.3–8.3) | 6.3 (5.7–6.9) | < 0.001 |
Knee-extension force (kg) | 36.6 (29.2–51.4) | 39.6 (34.6–54.5) | 33.4 (25.9–45.6) | < 0.05 |
Knee-flexion force (kg) | 27.2 (20.2–31.9) | 28.7 (21.9–35.5) | 24.5 (19.4–29.8) | < 0.05 |
Notes: Values are presented as median (25th and 75th percentile (%)) or n (%) * P−values are calculated by the Mann-Whitney test for continuous variables and the chi-squared test or Fisher's exact test for categorical variables. ASMI, appendicular skeletal muscle mass index; BMI, body mass index. |
As shown in Figure 2, the experiment procedure included four physical exercises: normal walking, fast walking, a standard squat, and a wide squat. Each participant performed a walking task consisting of five round trips on a straight 7-meter surface at both normal and maximum walking speeds and two sets of 10 repetitions of standard squat and wide squat exercises. Four sEMG electrodes were used to record the data from the rectus femoris (RF) and biceps femoris (BF) in the thigh muscle, as well as the tibialis anterior (TA) and gastrocnemius (GA) in the calf muscle. For sEMG and inertial data acquisition (IMU), the DELSYS Trigno Wireless system was used, as shown in Figure 2. This system can simultaneously measure sEMG, acceleration, and angular velocity in various body parts. The sEMG data acquisition was performed at a sampling frequency of 1259 Hz, whereas the IMU data acquisition was conducted at a sampling frequency of 148 Hz.
sEMG data acquired during physical exercises is susceptible to several challenges, including the following: motion artifacts caused by muscle movement and electrode slippage, power line interference affecting signal quality, inter-individual variability due to anatomical differences and physiological factors, and intra-individual variability due to inconsistencies in contraction levels. Consequently, preprocessing becomes essential for improving the quality and interpretability of the sEMG data. To eliminate high-frequency noise interference and motion artifacts, a band-pass filter was applied to the sEMG signal during the filtering process. The band-pass filter used was a Butterworth filter with a passband frequency range of 20 Hz to 400 Hz.
To mitigate the computational challenges posed by temporal variations in sEMG signals, segmentation was employed. This technique divides the signal into smaller time intervals, thereby facilitating the extraction of dynamic patterns and changes in muscle activation by ML algorithms, thus enhancing the model's ability to discern diverse muscle activities. In this study, the IMU sensor data was employed to segment the filtered sEMG signal. Therefore, we applied time synchronization in both the sEMG and IMU sensor data. For sEMG data segmentation during the walking action, the angular velocity signal for the flexion/extension of the shank and the sEMG signal of the TA muscle was used, as shown in Figure 3. By identifying the maximum peak point of the angular velocity, the minimum left peak was determined as the point where the foot falls off the ground (Toe-off, TO), as marked with a green circle; moreover, the minimum right peak corresponded to the foot's contact with the ground (Heel Strike, HS), as marked with a red circle. The corresponding sEMG data was segmented by calculating the duration (one step time) between successive HS events detected from the angular velocity [20]. For sEMG data segmentation during the half and wide squat actions, the angular velocity signal for the flexion/extension of the shank and the sEMG signal of the RF muscle were used, as shown in Figure 4. The sign change point where the sign of the angular velocity changed from positive to negative was detected, and the sign change point to the next change point was defined as one cycle of the squat. The corresponding sEMG data was segmented by calculating the duration of one squat cycle and then used for analysis.
Addressing both inter- and intra-individual variability in sEMG data acquired during specific actions necessitated signal normalization. This was achieved by dividing segmented sEMG signals by the reference maximum voluntary contraction (MVC) values obtained from the corresponding muscle during the wide squat action. Additionally, the sEMG signal was rectified using full rectification to ensure comparability and suitability for further processing of the normalized sEMG signals. [21].
Analyzing EMG signals is crucial to assess muscle weakness and fall risks in patients with sarcopenia. However, raw EMG signals are complex and often contain various frequency components originating from muscle action potentials (MAPs), motor unit action potentials, and noise. The presence of these various components can make it difficult to directly analyze the features of MAPs that are most relevant to the risk of sarcopenia. Signal decomposition techniques help separate the different components of the EMG signal, thus allowing for a focused analysis of muscle activity relevant to sarcopenia. EMD is a signal processing technique used to decompose a non-linear and non-stationary signal into a set of IMFs. Each IMF represents a component with a well-defined instantaneous frequency [22]. Because of their non-linear and non-stationary characteristics, sEMG signals can be effectively analyzed using the EMD method. Moreover, sarcopenia risk detection relies on identifying specific features or patterns within the sEMG signals that correspond to muscle dysfunction. By decomposing the EMG signal into IMFs, EMD allows for a more detailed examination of the underlying motor unit activity, thus providing insights into changes associated with a reduced muscle mass. A decrease in muscle mass results in fewer motor units and muscle fibers, thus leading to heightened effort and strain on the remaining muscle tissue during physical activities, ultimately causing a faster onset of muscle fatigue. Muscle fatigue is associated with changes in the frequency and amplitude characteristics of EMG signals. EMD decomposition enables the identification and analysis of specific frequency bands that are indicative of fatigue-related alterations. In this study, IMFs were extracted from the preprocessed EMG signal. Extracting time and frequency domain features from IMF signals can lead to more reliable and sensitive markers of muscle weakness. The EMD algorithm can be summarized as follows. First, identify the local maxima and minima (extrema points) in the preprocessed sEMG signal x(t), as shown in Figure 5. The mean envelope mi(t) is calculated by averaging the upper xmax(t) and lower envelopes xmin(t) as follows:
mi(t)=(xmax(t)+xmin(t))2 | (1) |
In order to obtain the first intrinsic mode function di(t), subtract the mean envelope mi(t) from the preprocessed sEMG signal x(t) as follows:
di(t) = x(t) -mi(t) | (2) |
It is necessary to examine whether di(t) satisfies the criterion to be classified as an IMF or not, as written below:
1) The number of zero-crossings must be either equal to or differ by at most one from the number of extrema;
2) The mean value of the envelope defined by the local maxima and the envelope defined by the local minima must be either zero or very close to zero across the entire data segment. If the signal di(t) does not satisfy the criterion, it is considered as a new signal and the decomposition process is repeated on this new signal to obtain the next IMF. If the signal di(t) satisfies the above conditions, it is treated as an IMF and then subtracted from x(t) to obtain the residual as follows:
rk(t)=x(t)−∑ki = 1ck(t) | (3) |
The process concludes with the extraction of all IMF1(t), IMF2(t)..., IMFi(t) in a descending order of frequency and residual component. The number of IMFs generated by the EMD can vary for each EMG signal. The number of IMFs depends on factors such as the characteristics of the EMG signal, its length, complexity, and the presence of noise. The first few IMFs typically capture the dominant frequency components of the signal. These components are crucial for understanding the primary oscillatory patterns present in the EMG signal. In this study, we used the first five IMFs to extract EMG features and the decomposed IMFs of sEMG during the normal walk action, as shown in Figure 6.
sEMG signals are large and complex, encompassing a considerable amount of redundant information. Not all segments of the sEMG signal equally contribute to understanding muscle activity. Feature extraction helps to identify and extract the most relevant and informative features from the signal, thereby significantly reducing its dimensionality and focuses on extracting specific characteristics, such as amplitude, frequency, and time-domain parameters, that are directly related to muscle activation patterns and muscle strength [23,24,25]. This study explored 36 prominent EMG features, which were subsequently employed for training and evaluating different ML classifiers. A comprehensive list of these features is available in Table 2. We conducted feature extraction in two different cases. Case 1 involved extracting several features from the normalized sEMG signal without using the EMD decomposition technique (36 features * 4 muscles = 144 features).
1 | Mean absolute value (MAV) | 19 | Modified mean absolute value (MMAV) |
2 | RMS value (RMS) | 20 | Modified mean absolute value (MMAV2) |
3 | Variance (VAR) | 21 | Integrated EMG (IEMG) |
4 | Standard deviation (STD_DEV) | 22 | Average energy (ME) |
5 | Kurtosis (KURT) | 23 | Interquartile range (IQR) |
6 | Skewness (SKEW) | 24 | Difference variance value (DVARV) |
7 | Willison amplitude (WAMP) | 25 | Cardinality (CARD) |
8 | Slope sign change (SSC) | 26 | Hurst exponent (H) |
9 | Waveform length (WL) | 27 | Sample entropy (SE) |
10 | Mean absolute deviation (MEAN_AD) | 28 | Poincare plot index (SD1) |
11 | Median absolute deviation (MED_AD) | 29 | Poincare plot index (SD2) |
12 | Simple square integral (SSI) | 30 | Zero crossing (ZC) |
13 | Average amplitude change (AAC) | 31 | Mean frequency (MNF) |
14 | Difference absolute standard deviation value (DASDV) | 32 | Median frequency (MDF) |
15 | Myopulse percentage rate (MYOP) | 33 | Total power (TTP) |
16 | Max fractal length (MFL) | 34 | Mean power (MNP) |
17 | Difference absolute mean value (DAMV) | 35 | Peak frequency (PKF) |
18 | Log detector (LD) | 36 | Maximum EMG power (MAXP) |
Case 2 entailed extracting features from the normalized sEMG signal and the IMFs generated through the EMD decomposition technique (144 features from normalized EMG, 720 from IMFs, and 4 demographic variables). Therefore, the feature set consisted of 868 features, including the following four variables as demographic information: age, sex, height, and weight of the subject. To enhance the ML model performance and reduce the signal processing complexity, the minimum redundancy maximum relevance (mRMR) feature selection technique was implemented to identify the most influential subset of EMG features [26]. The best subset of features from sEMG during the four activities (i.e., normal walking, fast walking, standard squat, and wide squat) is presented in Table 3.
Exercise | Normalized EMG | IMF1 | IMF2 | IMF3 | IMF4 | IMF5 |
Normal walk | PKF_TA, WAMP_BF, SE_TA, WAMP_GA, MFL_BF, PKF_RF, MNF_RF | DVARV_RF, SE_TA, SKEW_BF, ZC_BF, MFL_BF, VAR_TA, PKF_GA, SD2_TA, KURT_BF | VAR_TA, PKF_GA, SE_TA, SD2_TA, PKF_RF, MFL_BF | PKF_GA, MNF_RF | SKEW_RF, KURT_RF, SKEW_GA, SE_TA, MFL_BF | MFL_BF |
Fast walk | ZC_BF, MFL_BF, PKF_GA, SE_TA, PKF_BF, CARD_BF, SSC_TA, MNF_GA | SE_TA, SKEW_BF, MFL_BF, PKF_GA, MDF_BF, ZC_BF, MDF_RF | PKF_GA, SE_TA, MAXP_TA, MFL_BF, TTP_TA, ZC_TA | TTP_TA, SE_TA | H_RF, SKEW_RF, PKF_GA, H_TA, MFL_BF | MFL_BF, SE_TA |
Standard squat | CARD_RF, SKEW_RF, WAMP_BF, SE_BF | PKF_BF, WAMP_GA, MDF_GA, ZC_BF, SE_BF, MYOP_BF, MFL_RF | SKEW_RF, MYOP_GA, SE_TA, MAXP_BF, PKF_BF, H_TA, PKF_TA, SSI_BF, MDF_GA, MYOP_BF | TTP_RF, PKF_BF, MDF_GA, PKF_GA, SSI_RF, | PKF_BF, H_TA | H_TA, PKF_BF |
Wide squat | WAMP_GA, PKF_RF, ZC_GA, SE_BF, CARD_BF, MDF_GA | MNF_GA, SE_TA, VAR_GA, MYOP_BF, PKF_BF, ZC_BF, MFL_TA | PKF_BF, TTP_BF, MNF_GA, MDF_TA, MYOP_BF, MDF_GA | PKF_BF, IEMG_RF, MNF_GA | SD2_RF, PKF_TA, PKF_BF, IEMG_RF | KURT_GA, |
In this study, the sEMG data was categorized into two classes: the healthy group and the sarcopenia risk group. Leave-One-Subject-Out Cross-Validation (LOSO-CV) was used to evaluate the classification performance. LOSO-CV uses all data points of subjects for both training and validation. In each iteration, the model excludes samples of one subject for validation and trains the model on the remaining subject's samples. The process is repeated for all subjects in the dataset, with each subject being excluded once. The performance of the model was assessed by aggregating the results obtained from each iteration. This approach is valuable for providing unbiased performance estimates and insights into its ability to handle various data patterns and potential overfitting issues. To effectively classify sarcopenia risk, we employed a diverse array of ML algorithms, each with unique strengths and approaches.
The K-nearest neighbors (KNN) algorithm is a widely used non-parametric classification method known for its ease of implementation and high accuracy. KNN makes predictions by identifying the majority class among the k-nearest data points to a given query instance in the feature space. The variable k is the number of nearest neighbors to consider for classification. Choosing the right k value is crucial for an optimal performance [27].
The naive Bayes (NB) classifier is a probabilistic ML algorithm that is widely employed for classification tasks. It is based on the Bayes theorem and operates on the assumption of feature independence, thereby simplifying computations by treating each feature as conditionally independent given the class label [28].
The random forest (RF) classifier is a powerful ensemble learning algorithm that is widely employed for both classification and regression tasks. Built upon the idea of constructing multiple decision trees and combining their outputs, the RF classifier excels in improving the predictive accuracy and mitigating overfitting. Each tree in the forest is trained on a random subset of the dataset, and the final prediction is determined by aggregating the individual predictions through voting [29].
The extreme gradient boosting (XGB) classifier is a state-of-the-art machine learning algorithm that belongs to the ensemble learning family, specifically gradient boosting frameworks. Renowned for its exceptional predictive performance, XGB builds a strong predictive model by combining the outputs of multiple weak learners, typically decision trees. It employs a gradient boosting approach, thereby sequentially adding trees that correct the errors of the previous ones [30].
The multi-layer perceptron (MLP) classifier is a type of artificial neural network that is known for its capability to handle complex and non-linear relationships within data. As a feedforward neural network, the MLP consists of multiple layers, including an input layer, one or more hidden layers, and an output layer. Each layer contains interconnected nodes (neurons), and the network uses a supervised learning approach to adjust its weights and biases during training, thus optimizing for accurate predictions [31].
This section presents the performance of the proposed ML classifiers for predicting sarcopenia risk using features extracted both with and without EMD decomposition. To evaluate the classifier performance, experiments were conducted on 93 subjects belonging to two classes: healthy and sarcopenia risk. The classifiers performance was assessed by comparing the feature extraction with and without EMD decomposition. Each ML model was trained using the optimal feature set determined through the mRMR feature selection technique. Subsequently, the hyper-parameters of each classifier were optimized using the GridSearchCV technique. To analyze the classifier performance, several metrics were calculated: accuracy, precision, recall, F1-score, and confusion matrices. Confusion matrices allow for visualization of the model performance in classifying instances from each class. Performance metrics for the different classifiers were recorded using features extracted without EMD decomposition for normal walking, fast walking, and standard and wide squat actions, as shown in Tables 4 and 5.
Model | Normal walking action | Fast walking action | ||||||
Accuracy | Precision | Recall | F-1 score | Accuracy | Precision | Recall | F-1 score | |
KNN | 0.68 | 0.72 | 0.72 | 0.72 | 0.70 | 0.77 | 0.77 | 0.77 |
NB | 0.46 | 0.36 | 0.46 | 0.40 | 0.68 | 0.68 | 0.68 | 0.68 |
RF | 0.76 | 0.78 | 0.78 | 0.78 | 0.78 | 0.77 | 0.77 | 0.77 |
XGB | 0.81 | 0.81 | 0.81 | 0.81 | 0.78 | 0.75 | 0.75 | 0.75 |
MLP | 0.83 | 0.86 | 0.86 | 0.86 | 0.85 | 0.89 | 0.89 | 0.89 |
Model | Standard squat action | Wide squat action | ||||||
Accuracy | Precision | Recall | F-1 score | Accuracy | Precision | Recall | F-1 score | |
KNN | 0.64 | 0.71 | 0.71 | 0.71 | 0.65 | 0.68 | 0.65 | 0.66 |
NB | 0.61 | 0.72 | 0.66 | 0.69 | 0.68 | 0.72 | 0.70 | 0.71 |
RF | 0.75 | 0.77 | 0.76 | 0.76 | 0.72 | 0.74 | 0.74 | 0.74 |
XGB | 0.81 | 0.82 | 0.82 | 0.82 | 0.75 | 0.76 | 0.76 | 0.76 |
MLP | 0.71 | 0.76 | 0.76 | 0.76 | 0.79 | 0.79 | 0.79 | 0.79 |
As shown in Table 4, among the classifiers evaluated for normal walking action, NB exhibited the lowest accuracy at 0.46, while MLP achieved the highest with 0.83. This trend persists for fast walking actions, with NB again demonstrating the lowest accuracy at 0.68 and MLP attaining the peak at 0.85. Overall, XGB and MLP surpassed other models in performance for both normal and fast walking. Furthermore, Table 5 reveals the classification performance on squat actions. NB displayed the lowest accuracy at 0.61, while XGB achieved the highest accuracy at 0.81 for the standard squat. In the case of the wide squat action, KNN demonstrated the lowest accuracy at 0.68, and MLP showed the highest at 0.79. The consistent high performance of XGB and MLP across all four actions highlights their effectiveness in extracting relevant EMG features for sarcopenia risk classification tasks. Classification accuracy alone may not adequately represent the true effectiveness of a model and does not separately consider the individual performance of each class. To address this limitation, the confusion matrices of the subject-wise average accuracies were evaluated for the best performing classifier in all four actions, as depicted in Figure 7.
Table 6 and Table 7 comprehensively illustrate the performance metrics of various classifiers for four actions, thereby employing features extracted via EMD decomposition. The tabulated results indicate that the MLP model achieved the highest classification accuracy of 0.88 for normal walking, 0.89 for fast walking, 0.81 for the standard squat, and 0.80 for wide squat actions. KNN and NB demonstrated the lowest accuracy across all actions. Overall, XGB and MLP emerge as consistent top performers across all action categories. Additionally, Figure 8 illustrates the confusion matrices of the subject-wise average accuracies for the best performing classifier in all four actions, further highlighting the superiority of the MLP model in terms of classification performance across all actions.
Model | Normal walking action | Fast walking action | ||||||
Accuracy | Precision | Recall | F-1 score | Accuracy | Precision | Recall | F-1 score | |
KNN | 0.66 | 0.70 | 0.70 | 0.70 | 0.66 | 0.72 | 0.72 | 0.72 |
NB | 0.60 | 0.65 | 0.63 | 0.64 | 0.64 | 0.65 | 0.65 | 0.65 |
RF | 0.82 | 0.83 | 0.82 | 0.82 | 0.76 | 0.75 | 0.75 | 0.75 |
XGB | 0.77 | 0.76 | 0.76 | 0.76 | 0.78 | 0.79 | 0.79 | 0.79 |
MLP | 0.88 | 0.89 | 0.89 | 0.89 | 0.89 | 0.92 | 0.92 | 0.92 |
Model | Standard squat action | Wide squat action | ||||||
Accuracy | Precision | Recall | F-1 score | Accuracy | Precision | Recall | F-1 score | |
KNN | 0.63 | 0.73 | 0.73 | 0.73 | 0.70 | 0.73 | 0.71 | 0.72 |
NB | 0.64 | 0.67 | 0.66 | 0.66 | 0.66 | 0.76 | 0.71 | 0.73 |
RF | 0.79 | 0.80 | 0.79 | 0.79 | 0.78 | 0.78 | 0.78 | 0.78 |
XGB | 0.80 | 0.81 | 0.81 | 0.81 | 0.78 | 0.78 | 0.78 | 0.78 |
MLP | 0.81 | 0.82 | 0.82 | 0.82 | 0.80 | 0.80 | 0.80 | 0.80 |
This paper presents a novel technique that leverages sEMG signals, EMD decomposition, and ML to identify sarcopenia risk during physical activities. We evaluated the ML model classification performance on sEMG data collected from 93 subjects, including both control and sarcopenia risk groups. We used sEMG signals to understand muscle function and potential dysfunction related to sarcopenia. sEMG signals contain diverse information about muscle activity, including their strength, contraction patterns, and even specific frequency components. This rich data makes sEMG valuable for detecting and potentially predicting sarcopenia. However, sEMG signals can be complex and challenging to directly interpret. EMD decomposition offers a powerful tool for further investigation by analyzing the frequency components associated with sarcopenia-related changes in muscle activity. Therefore, we employed the EMD method to decompose the sEMG signal into multiple IMFs. Each IMF represents a specific frequency range, thus providing insights into the various muscle activities contributing to the overall signal. Then, EMG features were extracted from both the normalized EMG signal and each IMF. Notably, the mRMR feature selection technique was employed to identify the most relevant features for each ML model. In this study, five ML models were trained using the best feature set obtained through a feature selection technique.
The performance of the model was evaluated by comparing the feature extraction with and without EMD decomposition. Based on the above results and utilizing feature extraction with EMD decomposition, it is evident that the MLP classifier demonstrated the best performance across all four actions: the classification accuracy for normal walking, fast walking, and standard and wide squat actions were 0.88, 0.89, 0.81, and 0.80, respectively. This demonstrates a notable enhancement in the model performance due to feature extraction from IMFs, with improvements ranging from 4-5% across all actions. The integration of feature extraction from normalized EMG and IMFs generated through the EMD technique offers distinct advantages. It enables the capture of multi-dimensional information while effective handling of the non-stationary nature of the sEMG signals. Furthermore, features selected from the first three IMFs, which typically capture the dominant frequency components, can potentially enhance the accuracy of sarcopenia risk prediction, as evidenced in Table 3. Figure 8 (a) and (b) presented the confusion matrices for healthy and sarcopenia risk individuals during four actions: normal walking, fast walking, standard squat, and wide squat. Each matrix visualizes the classifier performance by showing how many subjects were correctly and incorrectly classified for each group. Based on the confusion matrices for normal walking and fast walking, it is evident that the MLP classifier exhibits an accuracy of over 0.90 for each individual class. The MLP algorithm outperforms other ML models in classifying sarcopenia risk due to its multiple hidden layers. These layers act as intermediate stages, thus allowing the model to learn intricate relationships between complex data such as EMG features and the corresponding subject groups. Additionally, a multi-layer structure may give it an edge over simpler models that might struggle with such complexities.
In the classification accuracy for all actions, fast walking demonstrated a higher accuracy compared to normal walking, the standard squat, and the wide squat. Conversely, the wide squat exhibited a lower classification accuracy compared to all other actions. The EMG signal captured during fast walking typically exhibited greater strength and amplitude compared to the EMG signal recorded during normal walking. The greater amplitude of signals in the EMG data during fast walking may provide additional discriminative information that can potentially facilitate the classification process using ML algorithms. The results presented above provide evidence that the proposed classification technique in this study effectively achieves a high classification performance for identifying sarcopenia risk during daily activities. However, the proposed models exhibited a misclassification rate of 15% to 20% due to the criteria used to group the healthy individuals and those with sarcopenia, which involved a combination of BMI and self-reported physical activity questionnaires. To achieve a significantly higher classification accuracy, our work will be extended to incorporate sequence models, transformers, and multi-head attention-based networks. Additionally, we plan to extract more non-linear features to enhance the model's performance. Furthermore, it is essential to test the model with a broader range of daily actions to ensure its robustness and generalizability. To ensure more accurate ground truth labels, we will utilize grouping criteria based on quantitative analyses, such as muscle mass and handgrip strength.
In conclusion, our paper presents a novel sarcopenia risk detection method that combines sEMG signals and empirical mode decomposition with ML algorithms. To evaluate the classification performance of the model, sEMG data was recorded from healthy individuals and those at risk for sarcopenia during four physical activities. EMD enabled the decomposition of the sEMG signals into IMFs for an enhanced analysis. Then, the extracted EMG features from the IMFs were fed into the ML algorithms for the classification of sarcopenia risk. Our results demonstrate that the proposed system achieves a promising performance in sarcopenia risk classification. By leveraging the power of ML algorithms, we successfully classified individuals into healthy and sarcopenia risk groups based on the sEMG signals. The proposed system displayed a high accuracy, with accuracy rates of 0.88 for normal walking, 0.89 for fast walking, 0.81 for the standard squat, and 0.80 for the wide squat, thus providing reliable identification of sarcopenia risk during daily activities.
Moreover, the integration of EMG signals and the EMD technique proves to be advantageous, as it captures multi-dimensional information and effectively handles the non-stationary nature of the signals. This enables a more comprehensive analysis of muscle dysfunction and aids in the early detection of sarcopenia. In future work, we will focus on expanding the dataset to include individuals diagnosed with sarcopenia, incorporating more diverse daily activities, and exploring advanced ML techniques such as recurrent neural networks and attention-based models. Overall, our research highlights the potential of combining sEMG and EMD with ML algorithms in designing accurate and efficient sarcopenia risk detection techniques for improved healthcare and quality of life.
This research was supported by the Korea Medical Device Development Fund grant funded by the Korea government (the Ministry of Science and ICT, the Ministry of Trade, Industry and Energy, the Ministry of Health & Welfare, the Ministry of Food and Drug Safety) (Project Number: RS-2022-00164554 and KMDF_PR_20200901_0101).
The authors declare they have not used Artificial Intelligence (AI) tools in the creation of this article.
The authors declare there is no conflict of interest.
[1] |
A. Cruz-Jentoft, G. Bahat, J. Bauer, Y. Boirie, O. Bruyère, T. Cederholm, et al., Sarcopenia: Revised European consensus on definition and diagnosis, Age Ag., 48 (2019), 16–31. https://doi.org/10.1093/ageing/afy169 doi: 10.1093/ageing/afy169
![]() |
[2] |
R. A. Fielding, B. Vellas, W. J. Evans, S. Bhasin, J. E. Morley, A. B. Newman, et al., Sarcopenia: An undiagnosed condition in older adults. Current consensus Definition: Prevalence, etiology, and consequences. International Working Group on Sarcopenia, J. Am. Med. Dir. Assoc., 12 (2011), 249–256. https://doi.org/10.1016/j.jamda.2011.01.003 doi: 10.1016/j.jamda.2011.01.003
![]() |
[3] |
I. Janssen, Evolution of sarcopenia research, Appl. Physiol. Nutr. Metab., 35 (2010), 707–712. https://doi.org/10.1139/h10-067 doi: 10.1139/h10-067
![]() |
[4] |
A. Dawson, E. Dennison, Measuring the musculoskeletal aging phenotype, Maturitas, 93 (2016), 13–17. https://doi.org/10.1016/j.maturitas.2016.04.014 doi: 10.1016/j.maturitas.2016.04.014
![]() |
[5] |
C. Beaudart, R. Rizzoli, O. Bruyère, J. Y. Reginster, E. Biver, Sarcopenia: Burden and challenges for public health, Arch. Public Health, 72 (2014). https://doi.org/10.1186/2049-3258-72-45 doi: 10.1186/2049-3258-72-45
![]() |
[6] |
C. Beaudart, E. McCloskey, O. Bruyère, M. Cesari, Y. Rolland, R. Rizzoli, et al., Sarcopenia in daily practice: Assessment and management, BMC Geriatr., 16 (2016). https://doi.org/10.1186/s12877-016-0349-4 doi: 10.1186/s12877-016-0349-4
![]() |
[7] |
M. Cho, S. Lee, S. Song, A review of Sarcopenia Pathophysiology, diagnosis, treatment and future direction, J. Korean Med. Sci, 37 (2022). https://doi.org/10.3346/jkms.2022.37.e146 doi: 10.3346/jkms.2022.37.e146
![]() |
[8] |
D. Albano, C. Messina, J. Vitale, L. M. Sconfienza, Imaging of sarcopenia: Old evidence and new insights, Eur. Radiol., 30 (2020), 2199–2208. https://doi.org/10.1007/s00330-019-06573-2 doi: 10.1007/s00330-019-06573-2
![]() |
[9] |
G. Guglielmi, F. Ponti, M. Agostini, M. Amadori, G. Battista, A. Bazzocchi, The role of DXA in sarcopenia, Ag. Clin. Exp. Res., 28 (2016), 1047–1060. https://doi.org/10.1007/s40520-016-0589-3 doi: 10.1007/s40520-016-0589-3
![]() |
[10] |
P. Tandon, M. Mourtzakis, G. Low, L. Zenith, M. Ney, M. Carbonneau, et al., Comparing the variability between measurements for sarcopenia using magnetic resonance imaging and computed tomography imaging, Am. J. Transplant., 16 (2016), 2766–2767. https://doi.org/10.1111/ajt.13832 doi: 10.1111/ajt.13832
![]() |
[11] |
K. Feng, J. Ji, Q. Ni, A novel gear fatigue monitoring indicator and its application to remaining useful life prediction for spur gear in intelligent manufacturing systems, Int. J. Fatigue, 168 (2023), 107459. https://doi.org/10.1016/j.ijfatigue.2022.107459 doi: 10.1016/j.ijfatigue.2022.107459
![]() |
[12] |
K. Feng, J. Ji, K. Wang, D. Wei, C Zhou, Q Ni, A novel order spectrum-based Vold-Kalman filter bandwidth selection scheme for fault diagnosis of gearbox in offshore wind turbines, Ocean Eng., 266 (2022), 112920. https://doi.org/10.1016/j.oceaneng.2022.112920 doi: 10.1016/j.oceaneng.2022.112920
![]() |
[13] |
K. Feng, J. Ji, Q. Ni, Y Li, W Mao, L Liu, A novel vibration-based prognostic scheme for gear health management in surface wear progression of the intelligent manufacturing system, Wear, 522 (2023), 204697. https://doi.org/10.1016/j.wear.2023.204697 doi: 10.1016/j.wear.2023.204697
![]() |
[14] |
S. Zhao, J. Liu, Z. Gong, Y. S. Lei, X. OuYang, C. C. Chan, et al., Wearable physiological monitoring system based on electrocardiography and electromyography for upper limb rehabilitation training, Sensors, 20 (2020), 4861. https://doi.org/10.3390/s20174861 doi: 10.3390/s20174861
![]() |
[15] |
S. Prabu, K. Srinivas, B. K. Rani, R. Sujat, B. D. Parameshachari, Prediction of muscular paralysis disease based on hybrid feature extraction with machine learning technique for COVID-19 and post-COVID-19 patients, Pers. Ubiquit. Comput., 27 (2023), 831–844. https://doi.org/10.1007/s00779-021-01531-6 doi: 10.1007/s00779-021-01531-6
![]() |
[16] |
I. Campanini, C. Disselhorst-Klug, W. Z. Rymer, R. Merletti, Surface EMG in clinical assessment and neurorehabilitation: Barriers limiting its use, Front. Neurol., 11 (2020), 934. https://doi.org/10.3389/fneur.2020.00934 doi: 10.3389/fneur.2020.00934
![]() |
[17] |
M. Al-Ayyad, H. A. Owida, R. De Fazio, B. Al-Naami, P. Visconti, Electromyography monitoring systems in rehabilitation: A review of clinical applications, wearable devices and signal acquisition methodologies, Electronics, 12 (2023), 1520. https://doi.org/10.3390/electronics12071520 doi: 10.3390/electronics12071520
![]() |
[18] |
R. Habenicht, G. Ebenbichler, P. Bonato, S. Ziegelbecker, L. Unterlerchner, P. Mair, et al., Age-specific differences in the time-frequency representation of surface electromyographic data recorded during a submaximal cyclic back extension exercise: a promising biomarker to detect early signs of sarcopenia, J. NeuroEng. Rehabil., 8 (2020). https://doi.org/10.1186/s12984-020-0645-2 doi: 10.1186/s12984-020-0645-2
![]() |
[19] |
A. Leone, G. Rescio, A. Manni, P. Siciliano, A. Caroppo, Comparative analysis of supervised classifiers for the evaluation of sarcopenia using a sEMG-based platform, Sensors, 22 (2022), 2721. https://doi.org/10.3390/s22072721 doi: 10.3390/s22072721
![]() |
[20] |
J. M. Jasiewicz, J. H. Allum, J. W. Middleton, A. Barriskill, P. Condie, B. Purcell, et al., Gait event detection using linear accelerometers or angular velocity transducers in able-bodied and spinal-cord injured individuals, Gait Posture, 24 (2006), 502–509. https://doi.org/10.1016/j.gaitpost.2005.12.017 doi: 10.1016/j.gaitpost.2005.12.017
![]() |
[21] | M. Halaki, G. Karen, Normalization of EMG signals: To normalize or not to normalize and what to normalize to?, in computational intelligence in electromyography analysis–a perspective on current applications and future challenges (ed Ganesh R. Naik), InTech, (2012). https://doi.org/10.5772/49957 |
[22] | E. H. Norden, S. Zheng, L. R. Steven, M. C. Wu, H. H. Shih, Q. N. Zheng, et al., The empirical mode decomposition and the hilbert spectrum for nonlinear and non-stationary time series analysis, in Proceedings: Mathematical, Physical and Engineering Sciences, 454 (1998), 903–995. https://doi.org/10.1098/rspa.1998.0193 |
[23] |
J. Too, A. R. Abdullah, N. M. Saad, Classification of Hand Movements based on Discrete Wavelet Transform and Enhanced Feature Extraction, Int. J. Adv. Comput. Sci. Appl., 10 (2019). https://doi.org/10.14569/ijacsa.2019.0100612 doi: 10.14569/ijacsa.2019.0100612
![]() |
[24] |
S. A. Christopher, I. MdRasedul, A comprehensive study on EMG feature extraction and classifiers, J. Biomed. Eng. Biosci., 1 (2018). https://doi.org/10.32474/oajbeb.2018.01.000104 doi: 10.32474/oajbeb.2018.01.000104
![]() |
[25] |
P. Qin, X. Shi, Evaluation of feature extraction and classification for lower limb motion based on SEMG signal, Entropy, 22 (2020), 852. https://doi.org/10.3390/e22080852 doi: 10.3390/e22080852
![]() |
[26] |
C. Ding, H. Peng, Minimum redundancy feature selection from microarray gene expression data, J. Bioinform. Comput. Biol., 3 (2015), 185–205. https://doi.org/10.1142/s0219720005001004 doi: 10.1142/s0219720005001004
![]() |
[27] |
T. M. Cover, P. D. Hart, Nearest neighbor pattern classification, IEEE Trans. Inf. Theory, 13 (1967), 21–27. https://doi.org/10.1109/tit.1967.1053964 doi: 10.1109/tit.1967.1053964
![]() |
[28] |
M. Hall, A decision Tree-Based attribute weighting filter for naive bayes, In Springer eBooks, 2007, 59–70. https://doi.org/10.1007/978-1-84628-663-6_5 doi: 10.1007/978-1-84628-663-6_5
![]() |
[29] |
T. K. Ho, Random decision forests, Proceedings of 3rd International Conference on Document Analysis and Recognition, 1 (1995), 278-282. doi: 10.1109/ICDAR.1995.598994 doi: 10.1109/ICDAR.1995.598994
![]() |
[30] | T. Chen, C. Guestrin, XGBoost: A scalable tree boosting system, in Proceedings of the 22nd ACM SIGKDD International Conference on Knowledge Discovery and Data Mining, 2016,785–794. https://doi.org/10.1145/2939672.2939785 |
[31] |
F. Murtagh, Multilayer perceptrons for classification and regression, Neurocomputing, 2 (1991), 183–197. https://doi.org/10.1016/0925-2312(91)90023-5 doi: 10.1016/0925-2312(91)90023-5
![]() |
1. | Jihoon Shin, Kwangsub Song, Sung-Woo Kim, Sangui Choi, Hooman Lee, Il-Soo Kim, Sun Im, Min Seok Baek, A wearable approach for Sarcopenia diagnosis using stimulated muscle contraction signal, 2025, 2093-9868, 10.1007/s13534-025-00461-z |
Characteristics | Total (n = 93) | Control group (n = 49) | Sarcopenia risk group (n = 44) | p-value |
Men (%) | 93 (47.3) | 26 (53.1) | 18 (40.9) | 0.300 |
Age (years) | 39.0 (29.0–49.5) | 40.0 (29.0–49.5) | 37.0 (27.5–49.8) | 0.732 |
Weight (kg) | 59.4 (53.1–66.4) | 65.0 (57.4–74.7) | 53.4 (48.6–60.9) | < 0.001 |
Height (cm) | 165.3 (160.9–174.5) | 167.2 (160.9–175.3) | 163.9 (160.9–173.2) | 0.723 |
BMI (kg/cm2) | 21.5 (19.7–23.) | 22.2–25.4 | 19.6 (18.5–20.4) | < 0.001 |
ASMI (kg/m2) | 6.6 (6.0–7.6) | 7.4 (6.3–8.3) | 6.3 (5.7–6.9) | < 0.001 |
Knee-extension force (kg) | 36.6 (29.2–51.4) | 39.6 (34.6–54.5) | 33.4 (25.9–45.6) | < 0.05 |
Knee-flexion force (kg) | 27.2 (20.2–31.9) | 28.7 (21.9–35.5) | 24.5 (19.4–29.8) | < 0.05 |
Notes: Values are presented as median (25th and 75th percentile (%)) or n (%) * P−values are calculated by the Mann-Whitney test for continuous variables and the chi-squared test or Fisher's exact test for categorical variables. ASMI, appendicular skeletal muscle mass index; BMI, body mass index. |
1 | Mean absolute value (MAV) | 19 | Modified mean absolute value (MMAV) |
2 | RMS value (RMS) | 20 | Modified mean absolute value (MMAV2) |
3 | Variance (VAR) | 21 | Integrated EMG (IEMG) |
4 | Standard deviation (STD_DEV) | 22 | Average energy (ME) |
5 | Kurtosis (KURT) | 23 | Interquartile range (IQR) |
6 | Skewness (SKEW) | 24 | Difference variance value (DVARV) |
7 | Willison amplitude (WAMP) | 25 | Cardinality (CARD) |
8 | Slope sign change (SSC) | 26 | Hurst exponent (H) |
9 | Waveform length (WL) | 27 | Sample entropy (SE) |
10 | Mean absolute deviation (MEAN_AD) | 28 | Poincare plot index (SD1) |
11 | Median absolute deviation (MED_AD) | 29 | Poincare plot index (SD2) |
12 | Simple square integral (SSI) | 30 | Zero crossing (ZC) |
13 | Average amplitude change (AAC) | 31 | Mean frequency (MNF) |
14 | Difference absolute standard deviation value (DASDV) | 32 | Median frequency (MDF) |
15 | Myopulse percentage rate (MYOP) | 33 | Total power (TTP) |
16 | Max fractal length (MFL) | 34 | Mean power (MNP) |
17 | Difference absolute mean value (DAMV) | 35 | Peak frequency (PKF) |
18 | Log detector (LD) | 36 | Maximum EMG power (MAXP) |
Exercise | Normalized EMG | IMF1 | IMF2 | IMF3 | IMF4 | IMF5 |
Normal walk | PKF_TA, WAMP_BF, SE_TA, WAMP_GA, MFL_BF, PKF_RF, MNF_RF | DVARV_RF, SE_TA, SKEW_BF, ZC_BF, MFL_BF, VAR_TA, PKF_GA, SD2_TA, KURT_BF | VAR_TA, PKF_GA, SE_TA, SD2_TA, PKF_RF, MFL_BF | PKF_GA, MNF_RF | SKEW_RF, KURT_RF, SKEW_GA, SE_TA, MFL_BF | MFL_BF |
Fast walk | ZC_BF, MFL_BF, PKF_GA, SE_TA, PKF_BF, CARD_BF, SSC_TA, MNF_GA | SE_TA, SKEW_BF, MFL_BF, PKF_GA, MDF_BF, ZC_BF, MDF_RF | PKF_GA, SE_TA, MAXP_TA, MFL_BF, TTP_TA, ZC_TA | TTP_TA, SE_TA | H_RF, SKEW_RF, PKF_GA, H_TA, MFL_BF | MFL_BF, SE_TA |
Standard squat | CARD_RF, SKEW_RF, WAMP_BF, SE_BF | PKF_BF, WAMP_GA, MDF_GA, ZC_BF, SE_BF, MYOP_BF, MFL_RF | SKEW_RF, MYOP_GA, SE_TA, MAXP_BF, PKF_BF, H_TA, PKF_TA, SSI_BF, MDF_GA, MYOP_BF | TTP_RF, PKF_BF, MDF_GA, PKF_GA, SSI_RF, | PKF_BF, H_TA | H_TA, PKF_BF |
Wide squat | WAMP_GA, PKF_RF, ZC_GA, SE_BF, CARD_BF, MDF_GA | MNF_GA, SE_TA, VAR_GA, MYOP_BF, PKF_BF, ZC_BF, MFL_TA | PKF_BF, TTP_BF, MNF_GA, MDF_TA, MYOP_BF, MDF_GA | PKF_BF, IEMG_RF, MNF_GA | SD2_RF, PKF_TA, PKF_BF, IEMG_RF | KURT_GA, |
Model | Normal walking action | Fast walking action | ||||||
Accuracy | Precision | Recall | F-1 score | Accuracy | Precision | Recall | F-1 score | |
KNN | 0.68 | 0.72 | 0.72 | 0.72 | 0.70 | 0.77 | 0.77 | 0.77 |
NB | 0.46 | 0.36 | 0.46 | 0.40 | 0.68 | 0.68 | 0.68 | 0.68 |
RF | 0.76 | 0.78 | 0.78 | 0.78 | 0.78 | 0.77 | 0.77 | 0.77 |
XGB | 0.81 | 0.81 | 0.81 | 0.81 | 0.78 | 0.75 | 0.75 | 0.75 |
MLP | 0.83 | 0.86 | 0.86 | 0.86 | 0.85 | 0.89 | 0.89 | 0.89 |
Model | Standard squat action | Wide squat action | ||||||
Accuracy | Precision | Recall | F-1 score | Accuracy | Precision | Recall | F-1 score | |
KNN | 0.64 | 0.71 | 0.71 | 0.71 | 0.65 | 0.68 | 0.65 | 0.66 |
NB | 0.61 | 0.72 | 0.66 | 0.69 | 0.68 | 0.72 | 0.70 | 0.71 |
RF | 0.75 | 0.77 | 0.76 | 0.76 | 0.72 | 0.74 | 0.74 | 0.74 |
XGB | 0.81 | 0.82 | 0.82 | 0.82 | 0.75 | 0.76 | 0.76 | 0.76 |
MLP | 0.71 | 0.76 | 0.76 | 0.76 | 0.79 | 0.79 | 0.79 | 0.79 |
Model | Normal walking action | Fast walking action | ||||||
Accuracy | Precision | Recall | F-1 score | Accuracy | Precision | Recall | F-1 score | |
KNN | 0.66 | 0.70 | 0.70 | 0.70 | 0.66 | 0.72 | 0.72 | 0.72 |
NB | 0.60 | 0.65 | 0.63 | 0.64 | 0.64 | 0.65 | 0.65 | 0.65 |
RF | 0.82 | 0.83 | 0.82 | 0.82 | 0.76 | 0.75 | 0.75 | 0.75 |
XGB | 0.77 | 0.76 | 0.76 | 0.76 | 0.78 | 0.79 | 0.79 | 0.79 |
MLP | 0.88 | 0.89 | 0.89 | 0.89 | 0.89 | 0.92 | 0.92 | 0.92 |
Model | Standard squat action | Wide squat action | ||||||
Accuracy | Precision | Recall | F-1 score | Accuracy | Precision | Recall | F-1 score | |
KNN | 0.63 | 0.73 | 0.73 | 0.73 | 0.70 | 0.73 | 0.71 | 0.72 |
NB | 0.64 | 0.67 | 0.66 | 0.66 | 0.66 | 0.76 | 0.71 | 0.73 |
RF | 0.79 | 0.80 | 0.79 | 0.79 | 0.78 | 0.78 | 0.78 | 0.78 |
XGB | 0.80 | 0.81 | 0.81 | 0.81 | 0.78 | 0.78 | 0.78 | 0.78 |
MLP | 0.81 | 0.82 | 0.82 | 0.82 | 0.80 | 0.80 | 0.80 | 0.80 |
Characteristics | Total (n = 93) | Control group (n = 49) | Sarcopenia risk group (n = 44) | p-value |
Men (%) | 93 (47.3) | 26 (53.1) | 18 (40.9) | 0.300 |
Age (years) | 39.0 (29.0–49.5) | 40.0 (29.0–49.5) | 37.0 (27.5–49.8) | 0.732 |
Weight (kg) | 59.4 (53.1–66.4) | 65.0 (57.4–74.7) | 53.4 (48.6–60.9) | < 0.001 |
Height (cm) | 165.3 (160.9–174.5) | 167.2 (160.9–175.3) | 163.9 (160.9–173.2) | 0.723 |
BMI (kg/cm2) | 21.5 (19.7–23.) | 22.2–25.4 | 19.6 (18.5–20.4) | < 0.001 |
ASMI (kg/m2) | 6.6 (6.0–7.6) | 7.4 (6.3–8.3) | 6.3 (5.7–6.9) | < 0.001 |
Knee-extension force (kg) | 36.6 (29.2–51.4) | 39.6 (34.6–54.5) | 33.4 (25.9–45.6) | < 0.05 |
Knee-flexion force (kg) | 27.2 (20.2–31.9) | 28.7 (21.9–35.5) | 24.5 (19.4–29.8) | < 0.05 |
Notes: Values are presented as median (25th and 75th percentile (%)) or n (%) * P−values are calculated by the Mann-Whitney test for continuous variables and the chi-squared test or Fisher's exact test for categorical variables. ASMI, appendicular skeletal muscle mass index; BMI, body mass index. |
1 | Mean absolute value (MAV) | 19 | Modified mean absolute value (MMAV) |
2 | RMS value (RMS) | 20 | Modified mean absolute value (MMAV2) |
3 | Variance (VAR) | 21 | Integrated EMG (IEMG) |
4 | Standard deviation (STD_DEV) | 22 | Average energy (ME) |
5 | Kurtosis (KURT) | 23 | Interquartile range (IQR) |
6 | Skewness (SKEW) | 24 | Difference variance value (DVARV) |
7 | Willison amplitude (WAMP) | 25 | Cardinality (CARD) |
8 | Slope sign change (SSC) | 26 | Hurst exponent (H) |
9 | Waveform length (WL) | 27 | Sample entropy (SE) |
10 | Mean absolute deviation (MEAN_AD) | 28 | Poincare plot index (SD1) |
11 | Median absolute deviation (MED_AD) | 29 | Poincare plot index (SD2) |
12 | Simple square integral (SSI) | 30 | Zero crossing (ZC) |
13 | Average amplitude change (AAC) | 31 | Mean frequency (MNF) |
14 | Difference absolute standard deviation value (DASDV) | 32 | Median frequency (MDF) |
15 | Myopulse percentage rate (MYOP) | 33 | Total power (TTP) |
16 | Max fractal length (MFL) | 34 | Mean power (MNP) |
17 | Difference absolute mean value (DAMV) | 35 | Peak frequency (PKF) |
18 | Log detector (LD) | 36 | Maximum EMG power (MAXP) |
Exercise | Normalized EMG | IMF1 | IMF2 | IMF3 | IMF4 | IMF5 |
Normal walk | PKF_TA, WAMP_BF, SE_TA, WAMP_GA, MFL_BF, PKF_RF, MNF_RF | DVARV_RF, SE_TA, SKEW_BF, ZC_BF, MFL_BF, VAR_TA, PKF_GA, SD2_TA, KURT_BF | VAR_TA, PKF_GA, SE_TA, SD2_TA, PKF_RF, MFL_BF | PKF_GA, MNF_RF | SKEW_RF, KURT_RF, SKEW_GA, SE_TA, MFL_BF | MFL_BF |
Fast walk | ZC_BF, MFL_BF, PKF_GA, SE_TA, PKF_BF, CARD_BF, SSC_TA, MNF_GA | SE_TA, SKEW_BF, MFL_BF, PKF_GA, MDF_BF, ZC_BF, MDF_RF | PKF_GA, SE_TA, MAXP_TA, MFL_BF, TTP_TA, ZC_TA | TTP_TA, SE_TA | H_RF, SKEW_RF, PKF_GA, H_TA, MFL_BF | MFL_BF, SE_TA |
Standard squat | CARD_RF, SKEW_RF, WAMP_BF, SE_BF | PKF_BF, WAMP_GA, MDF_GA, ZC_BF, SE_BF, MYOP_BF, MFL_RF | SKEW_RF, MYOP_GA, SE_TA, MAXP_BF, PKF_BF, H_TA, PKF_TA, SSI_BF, MDF_GA, MYOP_BF | TTP_RF, PKF_BF, MDF_GA, PKF_GA, SSI_RF, | PKF_BF, H_TA | H_TA, PKF_BF |
Wide squat | WAMP_GA, PKF_RF, ZC_GA, SE_BF, CARD_BF, MDF_GA | MNF_GA, SE_TA, VAR_GA, MYOP_BF, PKF_BF, ZC_BF, MFL_TA | PKF_BF, TTP_BF, MNF_GA, MDF_TA, MYOP_BF, MDF_GA | PKF_BF, IEMG_RF, MNF_GA | SD2_RF, PKF_TA, PKF_BF, IEMG_RF | KURT_GA, |
Model | Normal walking action | Fast walking action | ||||||
Accuracy | Precision | Recall | F-1 score | Accuracy | Precision | Recall | F-1 score | |
KNN | 0.68 | 0.72 | 0.72 | 0.72 | 0.70 | 0.77 | 0.77 | 0.77 |
NB | 0.46 | 0.36 | 0.46 | 0.40 | 0.68 | 0.68 | 0.68 | 0.68 |
RF | 0.76 | 0.78 | 0.78 | 0.78 | 0.78 | 0.77 | 0.77 | 0.77 |
XGB | 0.81 | 0.81 | 0.81 | 0.81 | 0.78 | 0.75 | 0.75 | 0.75 |
MLP | 0.83 | 0.86 | 0.86 | 0.86 | 0.85 | 0.89 | 0.89 | 0.89 |
Model | Standard squat action | Wide squat action | ||||||
Accuracy | Precision | Recall | F-1 score | Accuracy | Precision | Recall | F-1 score | |
KNN | 0.64 | 0.71 | 0.71 | 0.71 | 0.65 | 0.68 | 0.65 | 0.66 |
NB | 0.61 | 0.72 | 0.66 | 0.69 | 0.68 | 0.72 | 0.70 | 0.71 |
RF | 0.75 | 0.77 | 0.76 | 0.76 | 0.72 | 0.74 | 0.74 | 0.74 |
XGB | 0.81 | 0.82 | 0.82 | 0.82 | 0.75 | 0.76 | 0.76 | 0.76 |
MLP | 0.71 | 0.76 | 0.76 | 0.76 | 0.79 | 0.79 | 0.79 | 0.79 |
Model | Normal walking action | Fast walking action | ||||||
Accuracy | Precision | Recall | F-1 score | Accuracy | Precision | Recall | F-1 score | |
KNN | 0.66 | 0.70 | 0.70 | 0.70 | 0.66 | 0.72 | 0.72 | 0.72 |
NB | 0.60 | 0.65 | 0.63 | 0.64 | 0.64 | 0.65 | 0.65 | 0.65 |
RF | 0.82 | 0.83 | 0.82 | 0.82 | 0.76 | 0.75 | 0.75 | 0.75 |
XGB | 0.77 | 0.76 | 0.76 | 0.76 | 0.78 | 0.79 | 0.79 | 0.79 |
MLP | 0.88 | 0.89 | 0.89 | 0.89 | 0.89 | 0.92 | 0.92 | 0.92 |
Model | Standard squat action | Wide squat action | ||||||
Accuracy | Precision | Recall | F-1 score | Accuracy | Precision | Recall | F-1 score | |
KNN | 0.63 | 0.73 | 0.73 | 0.73 | 0.70 | 0.73 | 0.71 | 0.72 |
NB | 0.64 | 0.67 | 0.66 | 0.66 | 0.66 | 0.76 | 0.71 | 0.73 |
RF | 0.79 | 0.80 | 0.79 | 0.79 | 0.78 | 0.78 | 0.78 | 0.78 |
XGB | 0.80 | 0.81 | 0.81 | 0.81 | 0.78 | 0.78 | 0.78 | 0.78 |
MLP | 0.81 | 0.82 | 0.82 | 0.82 | 0.80 | 0.80 | 0.80 | 0.80 |