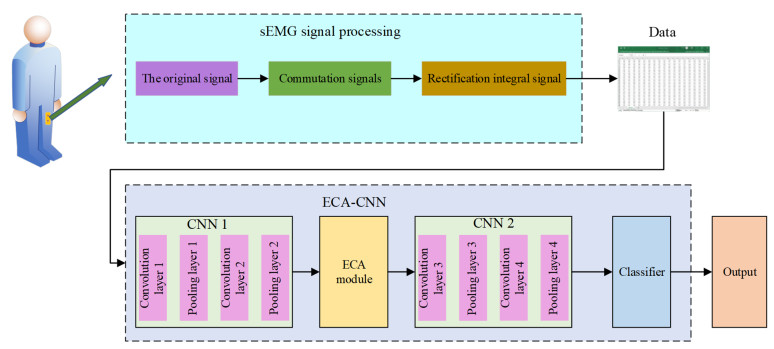
Gait recognition and classification technology is one of the essential technologies for detecting neurodegenerative dysfunction. This paper presents a gait classification model based on a convolutional neural network (CNN) with an efficient channel attention (ECA) module for gait detection applications using surface electromyographic (sEMG) signals. First, the sEMG sensor was used to collect the experimental sample data, and various gaits of different persons were collected to construct the sEMG signal data sets of different gaits. The CNN is used to extract the features of the one-dimensional input sEMG signal to obtain the feature vector, which is input into the ECA module to realize cross-channel interaction. Then, the next part of the convolutional layer is input to learn the signal features further. Finally, the model is output and tested to obtain the results. Comparative experiments show that the accuracy of the ECA-CNN network model can reach 97.75%.
Citation: Zhangjie Wu, Minming Gu. A novel attention-guided ECA-CNN architecture for sEMG-based gait classification[J]. Mathematical Biosciences and Engineering, 2023, 20(4): 7140-7153. doi: 10.3934/mbe.2023308
[1] | Ting Yao, Farong Gao, Qizhong Zhang, Yuliang Ma . Multi-feature gait recognition with DNN based on sEMG signals. Mathematical Biosciences and Engineering, 2021, 18(4): 3521-3542. doi: 10.3934/mbe.2021177 |
[2] | Xiaoguang Liu, Yubo Wu, Meng Chen, Tie Liang, Fei Han, Xiuling Liu . A double-channel multiscale depthwise separable convolutional neural network for abnormal gait recognition. Mathematical Biosciences and Engineering, 2023, 20(5): 8049-8067. doi: 10.3934/mbe.2023349 |
[3] | Yubo Sun, Yuanyuan Cheng, Yugen You, Yue Wang, Zhizhong Zhu, Yang Yu, Jianda Han, Jialing Wu, Ningbo Yu . A novel plantar pressure analysis method to signify gait dynamics in Parkinson's disease. Mathematical Biosciences and Engineering, 2023, 20(8): 13474-13490. doi: 10.3934/mbe.2023601 |
[4] | Xiao Zou, Jintao Zhai, Shengyou Qian, Ang Li, Feng Tian, Xiaofei Cao, Runmin Wang . Improved breast ultrasound tumor classification using dual-input CNN with GAP-guided attention loss. Mathematical Biosciences and Engineering, 2023, 20(8): 15244-15264. doi: 10.3934/mbe.2023682 |
[5] | Chaofan Li, Qiong Liu, Kai Ma . DCCL: Dual-channel hybrid neural network combined with self-attention for text classification. Mathematical Biosciences and Engineering, 2023, 20(2): 1981-1992. doi: 10.3934/mbe.2023091 |
[6] | Xiaoguang Liu, Mingjin Zhang, Jiawei Wang, Xiaodong Wang, Tie Liang, Jun Li, Peng Xiong, Xiuling Liu . Gesture recognition of continuous wavelet transform and deep convolution attention network. Mathematical Biosciences and Engineering, 2023, 20(6): 11139-11154. doi: 10.3934/mbe.2023493 |
[7] | Xiaoguang Liu, Meng Chen, Tie Liang, Cunguang Lou, Hongrui Wang, Xiuling Liu . A lightweight double-channel depthwise separable convolutional neural network for multimodal fusion gait recognition. Mathematical Biosciences and Engineering, 2022, 19(2): 1195-1212. doi: 10.3934/mbe.2022055 |
[8] | Wajid Aziz, Lal Hussain, Ishtiaq Rasool Khan, Jalal S. Alowibdi, Monagi H. Alkinani . Machine learning based classification of normal, slow and fast walking by extracting multimodal features from stride interval time series. Mathematical Biosciences and Engineering, 2021, 18(1): 495-517. doi: 10.3934/mbe.2021027 |
[9] | Hafiz Ghulam Murtza Qamar, Muhammad Farrukh Qureshi, Zohaib Mushtaq, Zubariah Zubariah, Muhammad Zia ur Rehman, Nagwan Abdel Samee, Noha F. Mahmoud, Yeong Hyeon Gu, Mohammed A. Al-masni . EMG gesture signal analysis towards diagnosis of upper limb using dual-pathway convolutional neural network. Mathematical Biosciences and Engineering, 2024, 21(4): 5712-5734. doi: 10.3934/mbe.2024252 |
[10] | Cong Jin, Jinjie Huang, Tianshu Wei, Yuanjian Chen . Neural architecture search based on dual attention mechanism for image classification. Mathematical Biosciences and Engineering, 2023, 20(2): 2691-2715. doi: 10.3934/mbe.2023126 |
Gait recognition and classification technology is one of the essential technologies for detecting neurodegenerative dysfunction. This paper presents a gait classification model based on a convolutional neural network (CNN) with an efficient channel attention (ECA) module for gait detection applications using surface electromyographic (sEMG) signals. First, the sEMG sensor was used to collect the experimental sample data, and various gaits of different persons were collected to construct the sEMG signal data sets of different gaits. The CNN is used to extract the features of the one-dimensional input sEMG signal to obtain the feature vector, which is input into the ECA module to realize cross-channel interaction. Then, the next part of the convolutional layer is input to learn the signal features further. Finally, the model is output and tested to obtain the results. Comparative experiments show that the accuracy of the ECA-CNN network model can reach 97.75%.
In the past few decades, human life expectancy has significantly improved [1] and the aging population has increased rapidly [2]. At the same time, the number of patients with neurodegenerative diseases has also been increasing. Although medical technology has substantially developed, neurodegenerative diseases such as Alzheimer's disease, Parkinson's disease (PD), multiple sclerosis (MS), etc. [3,4], bring great inconvenience to people's lives. Currently, the most effective way to combat the above diseases is early detection, diagnosis, and treatment [5]. Most of these diseases significantly affect human gait and show specific stride characteristics. The detection of gait changes can effectively detect neurodegenerative diseases.
Surface electromyography (sEMG) signals reflect the degree of activation of skeletal muscles and can be obtained on the muscle surface in a non-invasive way. Therefore, sEMG signals have been widely used in biomechanical research, such as human gesture classification [6,7] and motion estimation [8,9]. They are also widely used in gait recognition. Cai et al. [10] used a support vector machine (SVM) to recognize limb movement, achieving an accuracy of 93%. Miller et al. [11] used linear discriminant analysis (LDA) and SVM classifiers to identify seven different gaits in an amputation group and a non-amputation group, and the accuracies were 97.9% and 94.7%, respectively. Naik et al. [12] proposed an sEMG classifier based on independent component analysis-entropy bound minimization to detect joint lesions. Through gait detection of healthy subjects and individuals with knee lesions, the accuracies of the test results were 96.1% and 86.2%, respectively. Narayan et al. [13] used a k-nearest neighbor (KNN) classifier to recognize limb movements, and the recognition accuracy rate was 89%. Ryu et al. [14] used an user-adaptive classifier of sEMG signals and inertial measurement unit sensor for gait detection, as well as weighted vote counting and interpolation methods to realize the user-adaptive classification and predict gait, which improved the accuracy to 97%. Wei et al. [15] used a multi-dimensional feature set composed of electroencephalogram and sEMG features to compare it with LDA, KNN, the kernel SVM (KSVM) and other methods. Piatkowska et al. [16] used a naïve Bayes model (NBM) to analyze sEMG data sets with poor accuracy compared to the KNN method. In recent years, with the wide application of deep learning, more and more scholars have applied deep learning methods in various fields. Zhang et al. [17] demonstrated, by using a backpropagation neural network (BPNN), that load variation in sEMG can significantly affect the accuracy of gait recognition; the total average accuracies of gait recognition intra-loads and inter-loads were 91.81% and 69.42%, respectively. Meng et al. [18] extracted four time-domain features from four-channel sEMG signals, established a hidden Markov model (HMM) structure corresponding to the division of the gait cycle into phases represented by states and estimated the HMM parameters by using the Baum-Welch algorithm. Zhao et al. [19] developed an adaptive gait detection method by using HMM to model human gait; they used neural networks to process the original measurements and classify the HMM while using two inertial sensors to improve the accuracy to 98.11%. Xiong et al. [20] used a deep regression neural network based on the bidirectional gated recurrent unit to extract temporal information from the synergy matrix to estimate joint angles of the lower limb; principle component analysis achieved the highest performance of 0.871 ± 0.029. The introduction of the above deep neural network method improved detection accuracy. Nevertheless, there are problems, such as a complex network structure, a large amount of data calculation and the need for dimension transformation. There are also works of literature [21,22] that have used the original one-dimensional convolutional neural network (1D-CNN) to classify limb movements, and the accuracy was high. However, for large input data sets, 1D-CNNs need to use more convolutional cores or more convolutional layers to obtain higher representation capability, which may increase the complexity of the model and reduce the training speed. Ni and Zhang [23] and Shen et al. [24] both employed attention mechanisms in their CNNs to respectively improve performance in traffic flow prediction and hyperspectral image classification. Additionally, it was demonstrated that the incorporation of attention mechanisms in these CNNs facilitated the extraction of salient features from the data.
This paper presents a novel human gait classification method, i.e., the efficient channel attention (ECA)-CNN, which employs an improved CNN architecture with ECA units to automatically classify human gait based on sEMG signals. In particular, we have constructed, trained and tested an ECA-CNN with three parts to classify and recognize the following five types: standing still, walking, a simulation of PD (SOPD), a simulation of multiple sclerosis (SOMD) and a simulation of amyotrophic lateral sclerosis (SOALS). The superiority and effectiveness of this method compared to traditional methods have been verified via experiments.
The gait detection system designed in this study is a wearable device. The sEMG sensor module collects the signal on the leg muscle, and the data transmitted to the personal computer (PC) via the serial port of the microcontroller unit. The signal processing process is shown in Figure 1.
Electromyographic signals belong to the category of bioelectrical signals. When the human body moves, a weak electrical signal produced by the muscle movement of the relevant limbs is the electromyographic signal. It is formed by the superposition of the potential generated by muscle tissue during the exercise process at a specific time, including the action potentials of multiple groups of muscle fibers, which record the activity information of muscle tissue to a certain extent [25]. The activities of these muscles can be measured by sEMG, which is measured as an electrical signal on the skin's surface and is not invasive to the human muscle.
The purpose of this study was gait analysis. According to the law of human movement, it can be known that the movement of thigh muscle groups can best reflect the change in gait [26]. Whittington et al. [27] demonstrated, through experimental studies, as well as dynamic modeling, that passively stretched biarticular muscles, particularly the rectus femoris, contribute to the net torque and force seen in normal gait. Therefore, the muscle measured in this study is the rectus femoris, which is a superficial muscle in the front and middle of the thigh that is directly related to knee extension and thigh flexion and extension. Actual data also confirm the above analysis.
As shown in Figure 2(a), the sEMG sensor was attached to the rectus femoris muscle surface through an electrode sheet, and the reference electrode was taped to the knee bone. The sEMG signal data collected by the sensor were sent to the PC for real-time recording and presented in the form of curves, which is convenient for observing the changes in muscle potential. Figure 2(b) shows the curve of muscle potential change during a sampling period.
The CNN has a classical deep neural network structure that can comprehensively observe the target information. Especially, in recent years, with the rapid development of CNNs, the number of application scenarios has been increasing; additionally, the processing methods are constantly being optimized, offering significant contributions to the research in many fields.
Particularly, 1D-CNN has the following advantages: it can accept direct input of original data to avoid excessive preprocessing, and the parameter-sharing mechanism can reduce the complexity of the network structure and improve computational efficiency [28]. Downsampling fully uses local correlation information to minimize data processing while retaining structural information [29].
In this study, using the characteristics of a 1D-CNN, the sEMG signal was filtered and directly input to the network, and the data were input to the multi-channel feature extraction. The pooling layer operation can fulfill the effect of shrinking the data and reducing the amount of calculation.
Gait analysis usually requires consideration of body movements' order and time series. For example, a move might require stepping with the left foot and then the right foot. This sequential information can be captured by a time-domain convolution, but it may decay for longer sequences. Therefore, an ECA [30] module was adopted in this study to help the CNN better deal with such long-term dependence and improve the accuracy of the model on gait analysis tasks. In addition, the ECA module can improve the efficiency of the model because it effectively reduces the number of parameters. This may be important for CNN-based gait analysis, which may require the use of larger models to capture complex sequences of movements. Using the ECA module makes the model smaller while retaining higher accuracy.
As shown in Figure 3, after global average pooling, the ECA module captures local cross-channel interactions by considering each channel and its k adjacent values. The practice has proved that this method can ensure both efficiency and effect. ECA can be applied to one-dimensional convolutional networks with convolutional kernel size k. The convolutional kernel of size k indicates that there are k neighboring values involved in the attentional prediction of a channel. Therefore, the local weighting weight ωi of the channel can be calculated by using the following method:
{ωi=σ(∑kj=1αjyji)yi=G(di),di∈P,yji∈Ωji | (1) |
where P is the set of feature vector channels that need to be weighted, di is the feature vector channel of layer i in P, G(x) is the global average pooling operation, yi is the aggregate feature of di after the global average pooling operation, Ωji is the set of k adjacent feature channels of yi, αj is the original value of the jth channel, yji is the output value of the jth channel adjacent to the ith eigenvector channel and σ is the sigmoid activation function.
The above operations can be realized by one-dimensional convolution with kernel size k. The channel attention weight ω can be calculated by using the following formula:
ω=σ(C1Dk(y)) | (2) |
where C1D represents one-dimensional convolution and y is the aggregation feature.
As mentioned above, the ECA module will calculate an adaptive convolutional kernel size k according to the result of global averaging pooling. In this way, the ECA module can dynamically adjust the convolutional kernel size according to different input data so that the network can better capture the features of input data.
The kernel size k of the one-dimensional convolution is proportional to the size of the channel dimension C. That is, there is a mapping Φ between k and C:
C=Φ(k) | (3) |
Obviously, the simplest way to map is to use a linear function, namely, φ(k)=γ∗k−b, but the linearity is very limited. Moreover, the channel dimension C (the number of convolutional kernels) is usually set to the n power of 2. Therefore, the linear function φ(k)=γ∗k−b can be extended to a nonlinear function, namely,
C=Φ(k)=2(γ∗k−b) | (4) |
According to the given channel dimension C, the size of the one-dimensional convolutional kernel k can be adaptively determined as follows:
k=ψ(C)=|log2(C)γ+bγ|odd | (5) |
where |t|odd denotes the nearest odd number from t. Respectively, we set γ and b to fixed values of 2 and 1 in all experiments.
The framework of the ECA-CNN is mainly divided into three parts: a CNN, the ECA module and a CNN.
The two parts of the CNN each have two layers of structure, which is a total of four layers. In the first part, the first layer of the CNN contains the convolutional layer comprising 1 × 64 convolutional kernel with 16 channels and a maximum pooling layer of size 1 × 2. The moving step of each convolutional kernel was set to 16, and the padding was set to be the same. The convolutional result at the boundary is retained so that the output shape is the same as the input shape. After activation by the ReLu activation function, Convolutional layer 1 is obtained. After the operation of the maximum pooling layer with the size of 1 × 2 in Convolutional layer 1, Pooling layer 1 is obtained. After pooling, the number of feature vectors is reduced to half, and the dimension remains unchanged. The second, third and fourth layers of the CNN only change the filters and kernel size relative to the first layer, while the other operations are the same.
Pooling layer 2 is input into the ECA module. First, the number of channels in Pooling layer 2 is extracted, and then the adaptive convolutional kernel size k is calculated according to Eq (5). Then, the global average pooling of Pooling layer 2 is carried out to obtain x1. After transposing x1, a convolution with a kernel size of k is carried out to obtain x2. Then, x2 is activated by the sigmoid function and transposed to obtain x3. Finally, x3 and Pooling layer 2 are subjected to matrix multiplication to get the output result R.
After R is operated by the third and fourth CNN layers, Pooling layer 4 is obtained.
Finally, the n mapping feature vector values output by Pooling layer 4 are connected end to end into a one-dimensional vector, which is used as the final extracted feature vector layer V. The ReLu activation function is applied to the feature vector V to get the activation result, and the dropout operation is applied to the activation result to get the dropout result. Finally, the output is obtained through the Softmax layer.
The ECA-CNN needs to select the structural parameters according to the actual situation. The structure of the ECA-CNN used in this study is shown in Figure 4.
In this experiment, five gaits were collected: standing still, walking, SOPD, SOMS and SOALS. Due to epidemic control and environmental constraints, it was impossible to find experimental samples with the above-mentioned diseases. Therefore, for this experiment, the subjects imitated the corresponding movements according to the symptoms of the disease to achieve the effect of simulating gait [31].
The gait characteristics of PD, MS and amyotrophic lateral sclerosis (ALS) can be obtained by analyzing their symptoms [32]. PD patients are characterized by a decrease in walking speed, an increase in rhythm, a decrease in step length, a decrease in wavering time and an increase in double support time. For MS patients, the speed decreases, step length decreases and double support time increases. ALS patients have a decreased speed, an increased change in step length and an increased step duration. It can be seen that the gait characteristics of patients with these diseases have these features, so the gait can be simulated by adjusting several basic parameters of gait [33,34]. The basic parameters of the five gaits are shown in Figure 5 and Table 1.
Gait | Gait feature |
Standing still | The speed, step length and step frequency are all 0 and the two feet are constantly touching the ground. |
Walking | The speed is six steps every 6 seconds, the step length is 0.8 m and the step frequency is one step per second. |
PD (Simulation) | The speed is four steps every 6 seconds, the step length is 0.5 m, the step frequency is faster than one step per second and the contact time of the two feet is 1 second. |
ALS (Simulation) | The speed is four steps every 6 seconds, the step length is 0.5 m, the first two steps each have a stride time of 1 second and the last two steps each have a stride time of 0.2 seconds. |
MS (Simulation) | The speed is four steps every 6 seconds, the step length is 0.5 m, the step frequency is slower than one step per second and the contact time of the two feet is 1 second. |
The experiment stipulates that each movement is recorded every 6 seconds as a group. There were six volunteers who participated in the measurement of experimental data, each of whom were tested for 50 groups of each action, providing a total of 1500 groups of data.
In order to facilitate deep neural network training, the original data was preprocessed. Since the measurement range of the sEMG in this experiment was 0~800 mV, the low level was Vmin=0 and the high level was Vmax=800m, but the originally recorded data were mixed with data greater than Vmax, which is a false signal that needed to be filtered out. The method is as follows:
Val={input∈[Vmin,Vmax]0 | (6) |
An 8-fold moving average further filtered the data filtered by using Eq (6). The first eight data points are averaged:
x0=∑8i=0xi08 | (7) |
The sliding input of data can be realized after the eighth data point, and the value of the filter output is
xi=7xi−1+xi+78 | (8) |
Each data point can be filtered by using Eq (8), and real-time signals can be calculated after filtering. The data length of the signal was 3000. To facilitate the training and detection of the deep neural network, the length of each data group was unified into 3000 valid data points, starting from the initial value of each data group. If there was a vacancy, it was filled as 0, which did not affect the experimental results.
The PC used in the experiment was configured with a Windows 10 64-bit operating system, an Intel i5 6-core CPU, 16 GB RAM, an NVIDIA RTX3050 graphics card, a Python 3.9 environment, and a Keras 2.9.0 (version number) deep learning framework. The experimental process is shown in Figure 6.
First, the sEMG sensor system's measurement parameters were set up, the data of various movements were collected and the training data set was then established. Finally, the collected data were input into the ECA-CNN for training. The size of a single set of data input by the CNN was 1 × 3000. The number of training epochs was 30, and the batch size of each iteration was 10. A complete training session took 20 seconds.
After the model training, a real-time test was used to demonstrate the model's efficacy. Thirty percent of the data was randomly selected as the test set, and the model's gait recognition accuracy was set to be presented by a heat map (confusion matrix), as shown in Figure 7(a). As seen in Figure 7(b), with the increase in training time, the accuracy of model identification increased and tended to become stable.
In order to make an objective and fair evaluation of the gait detection system designed in this study, the proposed method was compared with the traditional methods of the decision tree (DT), SVM, KNN, NBM and BPNN to analyze the recognition accuracy of each gait.
First, the proposed and traditional methods were respectively used to train and test the data. The test results are shown in Table 2.
![]() |
Standing still | Walking | SOPD | SOALS | SOMS |
DT | 91% | 80% | 64% | 84% | 75% |
SVM | 100% | 69% | 62% | 86% | 79% |
KNN | 100% | 59% | 70% | 78% | 54% |
NBM | 82% | 73% | 67% | 13% | 73% |
BPNN | 100% | 52% | 46% | 70% | 60% |
1D-CNN | 100% | 95% | 94% | 97% | 95% |
ECA-CNN | 100% | 96% | 100% | 97% | 96% |
It can be seen that, as compared with the traditional classifiers, the deep neural network has a significant effect on the classification of different gaits. The traditional classifiers could not easily identify complex gaits, while the recognition accuracy of deep learning neural networks was much higher than that of traditional classifiers. In addition, the CNN also demonstrated superior efficiency in terms of runtime. While the traditional classifiers required 300–600 seconds for training, the CNN structure used in this study completed training in just 20 seconds given the same number of training iterations. Additionally, the deep neural network with an ECA mechanism was able to further improve the recognition of gait. The accuracy of the CNN without the attention mechanism was 96.13%, and the running time was 19.39 seconds. The recognition accuracy of the ECA-CNN with the attention mechanism was 97.75%, and the running time was 19.96 seconds. It can be ascertained from the results that, when the running time difference between the two was tiny, the network was more focused on the features of the data after the ECA mechanism was added; and, the accuracy increased by 1.62%. By exploring the network structure, we found that the reason why the training and testing speed of the network slowed down after adding the attention mechanism is that the ECA structure increases the complexity of the network. However, this had little impact on the current experiment.
Through comparison and analysis of the above experimental results, the following conclusions have been drawn:
Compared with traditional classifiers, CNNs have better performance in terms of running time and classification accuracy. After adding an ECA module, the accuracy and stability of model recognition were significantly improved. The combination of the ECA module and 1D-CNN can effectively simplify the network structure, reduce the amount of data computation, enhance the accuracy of recognition and adapt the size of the convolutional kernel.
This paper presented a gait detection method based on sEMG. The approach has several notable benefits: it requires minimal environmental setup, is highly convenient and efficient, focuses on real-time signal acquisition from targeted areas, minimizes interference and respects the privacy of individuals. To analyze the collected sEMG data, we designed an ECA-CNN model that combines CNNs with an ECA module. We created data sets of five different gait types and conducted training and testing. Our results showed that the ECA-CNN model outperformed a comparable CNN model without the ECA module, demonstrating the effectiveness of the ECA module in improving the model's ability to identify key features in the sEMG signals. These findings suggest that our proposed gait detection algorithm based on the ECA-CNN can significantly improve detection accuracy and provide a promising approach for the early detection of neurodegenerative diseases. However, due to constraints related to the ongoing pandemic and other factors, our study only used simulated data to represent the gait patterns of these diseases. Future work will involve developing a portable sEMG data transfer device and recruiting volunteers with these diseases for more comprehensive testing and analysis.
The authors gratefully acknowledge the research funding support from the National Students' Innovation and Entrepreneurship Training Program (No. 202210338031).
The authors declare that there is no conflict of interest.
[1] |
A. Gulland, Global life expectancy has risen, reports WHO, BMJ, 348 (2014). https://doi.org/10.1136/bmj.g3369 doi: 10.1136/bmj.g3369
![]() |
[2] |
W. C. Sanderson, S. Scherbov, The Characteristics approach to the measurement of population aging, Popul. Dev. Rev., 39 (2013), 673–685. https://doi.org/10.1111/j.1728-4457.2013.00633.x doi: 10.1111/j.1728-4457.2013.00633.x
![]() |
[3] |
A. Snijders, N. Giladi, B. Bloem, B. van de Warrenburg, Neurological gait disorders in elderly people: clinical approach and classification, Lancet Neurol., 6 (2007), 63–74. https://doi.org/10.1016/S1474-4422(06)70678-0 doi: 10.1016/S1474-4422(06)70678-0
![]() |
[4] |
C. Artusi, M. Mishra, P. Latimer, J. Vizcarra, L. Lopiano, W. Maetzler, et al., Integration of technology-based outcome measures in clinical trials of Parkinson and other neurodegenerative diseases, Parkinsonism Relat. Disord., 46 (2018), 53–56. https://doi.org/10.1016/j.parkreldis.2017.07.022 doi: 10.1016/j.parkreldis.2017.07.022
![]() |
[5] |
J. Li, The experiences of early detection, early diagnosis and early treatment of cancer in rural areas of China, J. Global Oncol., 4 (2018). https://doi.org/10.1200/jgo.18.60300 doi: 10.1200/jgo.18.60300
![]() |
[6] |
G. Emayavaramban, S. Divyapriya, V. M. Mansoor, A. Amudha, M. Ramkumar, P. Nagaveni, et al., SEMG based classification of hand gestures using artificial neural network, Mater. Today Proc., 37 (2021), 2591–2598. https://doi.org/10.1016/j.matpr.2020.08.504 doi: 10.1016/j.matpr.2020.08.504
![]() |
[7] |
N. Karnam, A. Turlapaty, S. Dubey, B. Gokaraju, Classification of sEMG signals of hand gestures based on energy features, Biomed. Signal Process. Control, 70 (2021). https://doi.org/10.1016/J.BSPC.2021.102948 doi: 10.1016/J.BSPC.2021.102948
![]() |
[8] |
G. I. Papagiannis, A. I. Triantafyllou, I. M. Roumpelakis, F. Zampeli, P. Eleni, P. Koulouvaris, et al., Methodology of surface electromyographyin gait analysis: review of the literature, J. Med. Eng. Technol., 43 (2019), 59–65. https://doi.org/10.1080/03091902.2019.1609610 doi: 10.1080/03091902.2019.1609610
![]() |
[9] |
C. Frigo, P. Crenna, Multichannel SEMG in clinical gait analysis: A review and state-of-the-art, Clin. Biomech., 24 (2008), 236–245. https://doi.org/10.1016/j.clinbiomech.2008.07.012 doi: 10.1016/j.clinbiomech.2008.07.012
![]() |
[10] |
S. Cai, Y. Chen, S. Huang, Y. Wu, H. Zheng, X. Li, et al., SVM-Based classification of sEMG signals for upper-limb self-rehabilitation training, Front. Neurorob., 13 (2019). https://doi.org/10.3389/fnbot.2019.00031 doi: 10.3389/fnbot.2019.00031
![]() |
[11] |
J. Miller, M. Beazer, M. Hahn, Myoelectric walking mode classification for transtibial amputees, IEEE Trans. Biomed. Eng., 60 (2013), 2745–2750 https://doi.org/10.1109/TBME.2013.2264466 doi: 10.1109/TBME.2013.2264466
![]() |
[12] |
G. R. Naik, S. Selvan, S. Arjunan, A. Acharyya, D. Kumar, A. Ramanujam, et al., An ICA-EBM-based sEMG classifier for recognizing lower limb movements in individuals with and without knee pathology, IEEE Trans. Neural Syst. Rehabil. Eng., 26 (2018), 675–686. https://doi.org/10.1109/TNSRE.2018.2796070 doi: 10.1109/TNSRE.2018.2796070
![]() |
[13] |
Y. Narayan, SEMG signal classification using KNN classifier with FD and TFD features, Mater. Today Proc., 37 (2021), 3219–3225. https://doi.org/10.1016/j.matpr.2020.09.089 doi: 10.1016/j.matpr.2020.09.089
![]() |
[14] |
R. Jaehwan, B. Lee, J. Maeng, D. Kim, sEMG-signal and IMU sensor-based gait sub-phase detection and prediction using a user-adaptive classifier, Med. Eng. Phys., 69 (2019), 50–57. https://doi.org/10.1016/j.medengphy.2019.05.006 doi: 10.1016/j.medengphy.2019.05.006
![]() |
[15] |
P. Wei, J. Zhang, F. Tian, J. Hong, A comparison of neural networks algorithms for EEG and sEMG features based gait phases recognition, Biomed. Signal Process. Control, 68 (2021). https://doi.org/10.1016/j.bspc.2021.102587 doi: 10.1016/j.bspc.2021.102587
![]() |
[16] |
W. Piatkowska, F. Spolaor, M. Romanato, R. Polli, A. Huang, A. Murgia, et al., A supervised classification of children with fragile X syndrome and controls based on kinematic and sEMG parameters, Appl. Sci., 12 (2022), 1612. https://doi.org/10.3390/app12031612 doi: 10.3390/app12031612
![]() |
[17] |
X. Zhang, S. Sun, C. Li, Z. Tang, Impact of load variation on the accuracy of gait recognition from surface EMG signals, Appl. Sci., 8 (2018), 1462. https://doi.org/10.3390/app8091462 doi: 10.3390/app8091462
![]() |
[18] | M. Meng, Q. She, Y. Gao, Z. Luo, EMG signals based gait phases recognition using hidden Markov models, in The 2010 IEEE International Conference on Information and Automation, (2010), 852–856. https://doi.org/10.1109/ICINFA.2010.5512456. |
[19] |
H. Zhao, Z. Wang, S. Qiu, J. Wang, F. Xu, Z. Wang, et al., Adaptive gait detection based on foot-mounted inertial sensors and multi-sensor fusion, Inf. Fusion, 52 (2019), 157–166. https://doi.org/10.1016/j.inffus.2019.03.002 doi: 10.1016/j.inffus.2019.03.002
![]() |
[20] |
D. Xiong, D. Zhang, X. Zhao, Y. Chu, Y. Zhao, Synergy-based neural interface for human gait tracking with deep learning, IEEE Trans. Neural Syst. Rehabil. Eng., 29 (2021), 2271–2280. https://doi.org/10.1109/TNSRE.2021.3123630. doi: 10.1109/TNSRE.2021.3123630
![]() |
[21] |
A. Vijayvargiya, Khimraj, R. Kumar, N. Dey, Voting-based 1D CNN model for human lower limb activity recognition using sEMG signal, Phys. Eng. Sci. Med., 44 (2021), 1297–1309. https://doi.org/10.1007/s13246-021-01071-6 doi: 10.1007/s13246-021-01071-6
![]() |
[22] |
M. Coskun, O. Yildirim, Y. Demir, U. Acharya, Efficient deep neural network model for classification of grasp types using sEMG signals, J. Ambient Intell. Hum. Comput., 13 (2022), 4437–4450. https://doi.org/10.1007/s12652-021-03284-9 doi: 10.1007/s12652-021-03284-9
![]() |
[23] |
Q. Ni, M. Zhang, STGMN: A gated multi-graph convolutional network framework for traffic flow prediction, Appl. Intell., 52 (2022), 15026–15039. https://doi.org/10.1007/s10489-022-03224-w doi: 10.1007/s10489-022-03224-w
![]() |
[24] |
J. Shen, Z. Zheng, Y. Sun, M. Zhao, Y. Chang, Y. Shao, et al., HAMNet: hyperspectral image classification based on hybrid neural network with attention mechanism and multi-scale feature fusion, Int. J. Remote Sens., 43 (2022), 4233–4258. https://doi.org/10.1080/01431161.2022.2109222 doi: 10.1080/01431161.2022.2109222
![]() |
[25] |
A. Vijayvargiya, B. Singh, R. Kumar, J. Tavares, Human lower limb activity recognition techniques, databases, challenges and its applications using sEMG signal: an overview, Biomed. Eng. Lett., 12 (2022), 343–358. https://doi.org/10.1007/s13534-022-00236-w doi: 10.1007/s13534-022-00236-w
![]() |
[26] |
D. Yungher, M. Wininger, J. Barr, W. Craelius, A. Threlkeld, Surface muscle pressure as a measure of active and passive behavior of muscles during gait, Med. Eng. Phys., 33 (2011), 464–471. https://doi.org/10.1016/j.medengphy.2010.11.012 doi: 10.1016/j.medengphy.2010.11.012
![]() |
[27] |
H. Sun, L. Wang, R. Lin, Z. Zhang, B. Zhang, Mapping plastic greenhouses with two-temporal sentinel-2 images and 1D-CNN deep learning, Remote Sens., 13 (2021). https://doi.org/10.3390/rs13142820 doi: 10.3390/rs13142820
![]() |
[28] |
B. Whittington, A. Silder, B. Heiderscheit, D. G. Thelen, The contribution of passive-elastic mechanisms to lower extremity joint kinetics during human walking, Gait Posture, 27 (2008), 628–634. https://doi.org/10.1016/j.gaitpost.2007.08.005 doi: 10.1016/j.gaitpost.2007.08.005
![]() |
[29] |
S. Liu, S. You, C. Zeng, H. Yin, Z. Lin, Y. Dong, et al., Data source authentication of synchrophasor measurement devices based on 1D-CNN and GRU, Electr. Power Syst. Res., 196 (2021). https://doi.org/10.1016/j.epsr.2021.107207 doi: 10.1016/j.epsr.2021.107207
![]() |
[30] | Q. Wang, B. Wu, P. Zhu, P. Li, W. Zuo, Q. Hu, ECA-Net: Efficient channel attention for deep convolutional neural networks, in 2020 IEEE/CVF Conference on Computer Vision and Pattern Recognition (CVPR), (2020), 11531–11539. https://doi.org/10.1109/CVPR42600.2020.01155 |
[31] |
T. Arakawa, T. Otani, Y. Kobayashi, M. Tanaka, 2-D forward dynamics simulation of gait adaptation to muscle weakness in elderly gait, Gait Posture, 85 (2021), 71–77. https://doi.org/10.1016/j.gaitpost.2021.01.011 doi: 10.1016/j.gaitpost.2021.01.011
![]() |
[32] |
G. Cicirelli, D. Impedovo, V. Dentamaro, R. Marani, G. Pirlo, T. R. D'Orazio, Human gait analysis in neurodegenerative diseases: a review, IEEE J. Biomed. Health Inf., 26 (2022), 229–242. https://doi.org/10.1109/JBHI.2021.3092875 doi: 10.1109/JBHI.2021.3092875
![]() |
[33] |
J. A. Martin, M. W. Kindig, C. J. Stender, W. R. Ledoux, D. G. Thelen, Calibration of the shear wave speed-stress relationship in in situ Achilles tendons using cadaveric simulations of gait and isometric contraction, J. Biomech., 106 (2020), 109799. https://doi.org/10.1016/j.jbiomech.2020.109799 doi: 10.1016/j.jbiomech.2020.109799
![]() |
[34] |
M. Woiczinski, C. Lehner, T. Esser, M. Kistler, M. Azqueta, J. Leukert, et al., Influence of treadmill design on gait: Does treadmill size affect muscle activation amplitude? A musculoskeletal calculation with individualized input parameters of gait analysis, Front. Neurol., 13 (2022), 830762–830762. https://doi.org/10.3389/fneur.2022.830762 doi: 10.3389/fneur.2022.830762
![]() |
1. | Yuxin Qin, Baojiang Li, Wenlong Wang, Xingbin Shi, Haiyan Wang, Xichao Wang, ETCNet: An EEG-based motor imagery classification model combining efficient channel attention and temporal convolutional network, 2024, 1823, 00068993, 148673, 10.1016/j.brainres.2023.148673 | |
2. | Yongyue Yang, Wenjie Chen, Yali Zhi, Yuanyuan Liu, Xiantao Sun, 2024, A Multi-stream Convolutional Model Using Attention Module for sEMG Gesture Recognition, 9798400709937, 360, 10.1145/3687488.3687552 | |
3. | Xianhua Liang, Bo Wei, Yu Wei, Lingrui Xu, Jie Cheng, Kegen Yu, Mahmoud Reza Delavar, 2025, A dual attention mechanism improved Swin transformer for extracting eucalyptus from GF-1 imagery, 9781510688216, 27, 10.1117/12.3059044 | |
4. | Yuxin Qin, Baojiang Li, Wenlong Wang, Xingbin Shi, Cheng Peng, Xichao Wang, Haiyan Wang, ECA-FusionNet: a hybrid EEG-fNIRS signals network for MI classification, 2025, 22, 1741-2560, 016030, 10.1088/1741-2552/adaf58 |
Gait | Gait feature |
Standing still | The speed, step length and step frequency are all 0 and the two feet are constantly touching the ground. |
Walking | The speed is six steps every 6 seconds, the step length is 0.8 m and the step frequency is one step per second. |
PD (Simulation) | The speed is four steps every 6 seconds, the step length is 0.5 m, the step frequency is faster than one step per second and the contact time of the two feet is 1 second. |
ALS (Simulation) | The speed is four steps every 6 seconds, the step length is 0.5 m, the first two steps each have a stride time of 1 second and the last two steps each have a stride time of 0.2 seconds. |
MS (Simulation) | The speed is four steps every 6 seconds, the step length is 0.5 m, the step frequency is slower than one step per second and the contact time of the two feet is 1 second. |
![]() |
Standing still | Walking | SOPD | SOALS | SOMS |
DT | 91% | 80% | 64% | 84% | 75% |
SVM | 100% | 69% | 62% | 86% | 79% |
KNN | 100% | 59% | 70% | 78% | 54% |
NBM | 82% | 73% | 67% | 13% | 73% |
BPNN | 100% | 52% | 46% | 70% | 60% |
1D-CNN | 100% | 95% | 94% | 97% | 95% |
ECA-CNN | 100% | 96% | 100% | 97% | 96% |
Gait | Gait feature |
Standing still | The speed, step length and step frequency are all 0 and the two feet are constantly touching the ground. |
Walking | The speed is six steps every 6 seconds, the step length is 0.8 m and the step frequency is one step per second. |
PD (Simulation) | The speed is four steps every 6 seconds, the step length is 0.5 m, the step frequency is faster than one step per second and the contact time of the two feet is 1 second. |
ALS (Simulation) | The speed is four steps every 6 seconds, the step length is 0.5 m, the first two steps each have a stride time of 1 second and the last two steps each have a stride time of 0.2 seconds. |
MS (Simulation) | The speed is four steps every 6 seconds, the step length is 0.5 m, the step frequency is slower than one step per second and the contact time of the two feet is 1 second. |
![]() |
Standing still | Walking | SOPD | SOALS | SOMS |
DT | 91% | 80% | 64% | 84% | 75% |
SVM | 100% | 69% | 62% | 86% | 79% |
KNN | 100% | 59% | 70% | 78% | 54% |
NBM | 82% | 73% | 67% | 13% | 73% |
BPNN | 100% | 52% | 46% | 70% | 60% |
1D-CNN | 100% | 95% | 94% | 97% | 95% |
ECA-CNN | 100% | 96% | 100% | 97% | 96% |