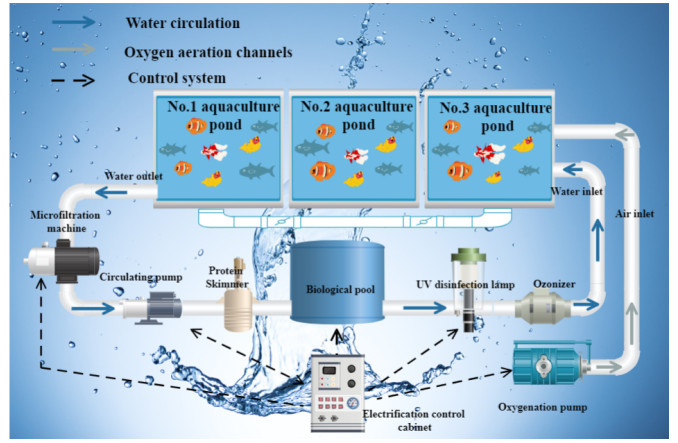
A torque control strategy based on acceleration intention recognition is proposed to address the issue of insufficient power performance in linear torque control strategies for electric racing cars, aiming to better reflect the acceleration intention of racing drivers. First, the support vector machine optimized by the sparrow search algorithm is used to recognize the acceleration intention, and the running mode of the racing car is divided into two types: Starting mode and driving mode. In driving mode, based on the recognition results of acceleration intention, fuzzy control is used for torque compensation. Based on the results of simulation and hardware in the loop testing, we can conclude that the support vector machine model optimized using the sparrow search algorithm can efficiently identify the acceleration intention of racing drivers. Furthermore, the torque control strategy can compensate for positive and negative torque based on the results of intention recognition, significantly improving the power performance of the racing car.
Citation: Anlu Yuan, Tieyi Zhang, Lingcong Xiong, Zhipeng Zhang. Torque control strategy of electric racing car based on acceleration intention recognition[J]. Mathematical Biosciences and Engineering, 2024, 21(2): 2879-2900. doi: 10.3934/mbe.2024128
[1] | Hongjie Deng, Lingxi Peng, Jiajing Zhang, Chunming Tang, Haoliang Fang, Haohuai Liu . An intelligent aerator algorithm inspired-by deep learning. Mathematical Biosciences and Engineering, 2019, 16(4): 2990-3002. doi: 10.3934/mbe.2019148 |
[2] | Huanhai Yang, Shue Liu . A prediction model of aquaculture water quality based on multiscale decomposition. Mathematical Biosciences and Engineering, 2021, 18(6): 7561-7579. doi: 10.3934/mbe.2021374 |
[3] | Delong Cui, Hong Huang, Zhiping Peng, Qirui Li, Jieguang He, Jinbo Qiu, Xinlong Luo, Jiangtao Ou, Chengyuan Fan . Next-generation 5G fusion-based intelligent health-monitoring platform for ethylene cracking furnace tube. Mathematical Biosciences and Engineering, 2022, 19(9): 9168-9199. doi: 10.3934/mbe.2022426 |
[4] | Lingmin Lin, Kailai Liu, Huan Feng, Jing Li, Hengle Chen, Tao Zhang, Boyun Xue, Jiarui Si . Glucose trajectory prediction by deep learning for personal home care of type 2 diabetes mellitus: modelling and applying. Mathematical Biosciences and Engineering, 2022, 19(10): 10096-10107. doi: 10.3934/mbe.2022472 |
[5] | Carlos Camilo-Garay, R. Israel Ortega-Gutiérrez, Hugo Cruz-Suárez . Optimal strategies for a fishery model applied to utility functions. Mathematical Biosciences and Engineering, 2021, 18(1): 518-529. doi: 10.3934/mbe.2021028 |
[6] | Jun Chen, Gangfeng Wang, Tao Xue, Tao Li . An improved polychromatic graphs-based BOM multi-view management and version control method for complex products. Mathematical Biosciences and Engineering, 2021, 18(1): 712-726. doi: 10.3934/mbe.2021038 |
[7] | Jose Guadalupe Beltran-Hernandez, Jose Ruiz-Pinales, Pedro Lopez-Rodriguez, Jose Luis Lopez-Ramirez, Juan Gabriel Avina-Cervantes . Multi-Stroke handwriting character recognition based on sEMG using convolutional-recurrent neural networks. Mathematical Biosciences and Engineering, 2020, 17(5): 5432-5448. doi: 10.3934/mbe.2020293 |
[8] | Boyi Zeng, Jun Zhao, Shantian Wen . A textual and visual features-jointly driven hybrid intelligent system for digital physical education teaching quality evaluation. Mathematical Biosciences and Engineering, 2023, 20(8): 13581-13601. doi: 10.3934/mbe.2023606 |
[9] | Mengfan Liu, Runkai Jiao, Qing Nian . Training method and system for stress management and mental health care of managers based on deep learning. Mathematical Biosciences and Engineering, 2022, 19(1): 371-393. doi: 10.3934/mbe.2022019 |
[10] | Ivan Izonin, Nataliya Shakhovska . Special issue: Informatics & data-driven medicine. Mathematical Biosciences and Engineering, 2021, 18(5): 6430-6433. doi: 10.3934/mbe.2021319 |
A torque control strategy based on acceleration intention recognition is proposed to address the issue of insufficient power performance in linear torque control strategies for electric racing cars, aiming to better reflect the acceleration intention of racing drivers. First, the support vector machine optimized by the sparrow search algorithm is used to recognize the acceleration intention, and the running mode of the racing car is divided into two types: Starting mode and driving mode. In driving mode, based on the recognition results of acceleration intention, fuzzy control is used for torque compensation. Based on the results of simulation and hardware in the loop testing, we can conclude that the support vector machine model optimized using the sparrow search algorithm can efficiently identify the acceleration intention of racing drivers. Furthermore, the torque control strategy can compensate for positive and negative torque based on the results of intention recognition, significantly improving the power performance of the racing car.
In recent years, as the scale of fishery aquaculture in China continues to expand, the contradiction between the development of related industries and environmental resources and the low productivity of labour has become increasingly prominent [1]. Such a situation has made standardised, digital and intelligent aquaculture technology a hot issue for current research [2,3]. The traditional human labour method of free-range aquaculture no longer meets the real needs of the current aquaculture market [4]. Therefore, efficient and accurate digital industrial aquaculture (DIA) is the direction of development that meets the realistic needs of aquaculture [5]. The development of DIA is an important part of building sustainable smart cities [6]. It is important to develop intelligent management solutions for DIA with the help of artificial intelligence technology [7]. The sensor network in the DIA can provide a large amount of scene monitoring data for this purpose [8]. This facilitates the implementation of intelligent management [9]. This work therefore aims to explore data-driven intelligent management solutions [10].
As artificial intelligence continues to advance, a number of scholars have begun to explore technological approaches to building assisted DIA using machine learning and deep learning in recent years [11,12,13,14]. Haq et al. [15] proposed a hybrid CNN and LSTM depth learning model to effectively predict the water quality of aquaculture. Wang et al. [16] proposed a dual neural network method including feature extraction network and full convolution semantic segmentation network to solve the problem of high cost of sample collection. Ahmed et al. [17] successfully identified the infected fish in aquaculture by using image processing and machine learning [18]. The advantages of these technologies are that they can improve the utilisation of available resources, increase productivity and free up labour while reducing costs [19]. In contrast to traditional techniques, the deep learning-based approach involves analysis and empirical learning of fish farming data and finally the development of intelligent management solutions [20,21,22]. Intelligent management encompasses many elements and has two main aspects: fish condition management and environmental condition management. The former in turn contains weight prediction, oxygen consumption prediction, feeding prediction, etc., while the latter contains water quality prediction [23]. How to use limited computing resources to achieve multiple business needs is a technical challenge for DIA [24].
To address the above issues, this paper extends the approach of single-objective prediction to a multi-objective prediction structure. Multiple objectives are predicted concurrently while maintaining the stability of the model [25,26,27,28]. To this end, this paper introduces a multi-objective neural network structure and proposes a data-driven intelligent management scheme for digital industrial aquaculture based on multi-object deep neural network (Mo-DIA). For fish condition management, a multi-objective prediction model is constructed using a double-hidden layer BP neural network [29,30]. The data on fish length, body width, temperature and feeding frequency are used to predict fish weight, fish oxygen consumption and fish feeding effectively. In terms of environmental condition management, the LSTM neural network-based multi-objective prediction model was constructed using the temporal correlation of water quality data series collection [31,32]. By training the analysis of water quality data, the time series values of eight attributes regarding Nitrite nitrogen (NO−2 - N), Nitrate nitrogen (NO−3 - N), Dissolved oxygen (DO), Temperature (T), Total nitrogen (TN), Potential of hydrogen (PH), Chemical oxygen demand (COD) and Ammonia nitrogen (NH + 4 - N) are predicted. The main contributions of this paper can be summarised as:
● This work systematically analyses the main tasks and challenges of DIA intelligent management and explores a comprehensive technology-assisted model.
● This paper introduces a multi-objective neural network structure and proposes a multi-objective deep neural network-based approach to DIA intelligent management.
● The effectiveness of this paper's multi-objective deep neural network approach is confirmed by conducting extensive experiments on datasets collected in real scenarios.
● This work provides a comprehensive evaluation of the multi-objective concurrent prediction results, and the performance of the method is positive.
The goal of intelligent aquaculture is to move from data to decision making. In order to achieve intelligent aquaculture, all aspects of the culture need to be finely controlled, including oxygen aeration, feeding and feeding rates. As shown in Figure 1, it represents the complex scene of a digital industrial aquaculture. Experience from artificial aquaculture shows that fish cannot grow without sufficient oxygen, the right amount of bait and a good water quality environment. Therefore, the intelligent management solution based on DIA proposed in this paper is to use artificial intelligence algorithm technology combined with hardware equipment to deal with the state management of the fish and the water quality state management of the aquaculture environment.
For fish condition management, the system collects images of fish behaviour and body size characteristics in real time through computer vision technology and sends them to the computer [33,34]. The computer analyses the fish length, width, temperature, feeding frequency and other data from the images. The data is then fed into the BP neural network model (Mo-BP) to predict fish weight, fish oxygen consumption and fish feeding status. The daily bait feeding rate is 2% of the fish body weight. The predicted data is used to determine whether the fish are in a normal growth process and to make decisions to ensure that the fish can grow normally. For the management of the environmental status of aquaculture is to better control the water quality environment of aquaculture. This system deploys sensors to monitor a wide range of water quality parameters in the water. These measured data are then passed into a recurrent neural network model (Mo-LSTM) to predict time series values regarding eight attributes: NO−2 - N, NO−3 - N, DO, T, TN, pH, COD and NH + 4 - N. Based on the predictions, the water quality is automatically treated using the appropriate filtration equipment to monitor the farming environment and warn of abnormalities in real time.
The DIA management approach proposed in this paper includes two major aspects: fish state management and environmental state management. As shown in Figure 2, it represents technical illustration of the two sub-modules in the proposed Mo-DIA. In terms of fish condition management, this paper proposes a Mo-BP model to implement a multi-objective prediction model. The data on fish length, body width, temperature and feeding frequency are used to predict fish weight, fish oxygen consumption and fish feeding effectively. In terms of environmental state management, this paper proposes a Mo-LSTM model to implement a multi-objective prediction model. Using the time correlation of water quality data series collection, the time series values of eight attributes regarding NO−2 - N, NO−3 - N, DO, T, TN, pH, COD and NH + 4 - N are predicted.
Back Propagation Neural Network, an extremely common artificial neural network model, is a multi-layer feed-forward neural network trained according to an error back propagation algorithm. The learning process of the Mo-BP algorithm consists of two processes: the forward propagation of the signal and the backward propagation of the error. In this experiment, the number of hidden layers is set to 2, and each hidden layer contains 4 neurons.
In the forward propagation process, the final output value and the loss between the output value and the actual value are calculated based on the input samples, combined with the given initialized weight value W and the value of the bias term b.
Step 1: Parameter initialisation. The sample input here is denoted as →a=(x1,x2). The initialised three-layer weight values and bias values are:
W(1)=[w(x1,1),w(x2,1)w(x1,2),w(x2,2)w(x1,3),w(x2,3)w(x1,4),w(x2,4)] | (3.1) |
W(2)=[w(1,5),w(2,5),w(3,5),w(4,5)w(1,6),w(2,6),w(3,6),w(3,6)w(1,7),w(2,7),w(3,7),w(4,7)w(1,8),w(2,8),w(3,8),w(4,8)] | (3.2) |
W(3)=[w(5,9),w(6,9),w(7,9),w(8,9)] | (3.3) |
where the corresponding bias values for each layer are: b(1)=[b1,b2,b3,b4], b(2)=[b5,b6,b7,b8], b(3)=[b9].
Step 2: Calculation of hidden layers. The proposed Mo-BP model has two hidden layers, where the input of the first hidden layer are z1,z2,z3,z4 according to Eq 3.4. The function g(x) is chosen as the activation function, and the outputs of this layer are g1(z1),g2(z2),g3(z3),g4(z4). The input to the second layer are z5,z6,z7,z8 and the output are g5(z5),g6(z6),g7(z7),g8(z8) according to Eq 3.5.
Z(1)=W(1)∗(→a)T+(b(1))T | (3.4) |
Z(2)=W(2)∗[z1,z2,z3,z4]T+(b(2))T | (3.5) |
Step 3: Calculation of the output layer. The output layer is set up with only one neuron, so the input to this layer can be expressed as z9 and the output as g9(z9) according to Eq 3.6.
Z(3)=W(3)∗[z5,z6,z7,z8]T+(b(3))T | (3.6) |
The samples are fed into the Mo-BP neural network model, ˆy denotes the result obtained by forward propagation and y denotes the true result. According to the chain rule and mathematical induction, the k layer error value ζ(k) can be calculated from the k+1 layer error value ζ(k+1) by the formula:
ζ(k)=gk′(z(k))⊙[((W)(k+1))Tζ(k+1)] | (3.7) |
where L(y,ˆy) denotes the loss function of the metric loss and ⊙ denotes the Hadamard product. The parameters are learned according to gradient descent, and the expressions for the partial derivatives of the weights W and bias b are:
∂L(y,ˆy)∂W(k)=ζ(k)(n(k−1))T | (3.8) |
∂L(y,ˆy)∂b(k)=ζ(k) | (3.9) |
The back propagation algorithm updates the weights W and bias b based on the error between the predicted and true values to minimise the error for the purpose of optimising the model.
Long Short Term Memory networks (LSTM), suitable for processing and predicting important events with relatively long intervals and delays in time series. In this paper, the environmental state management of aquaculture is to predict the water quality parameters at a future moment for the historical water quality parameters with time series characteristics. Compared with the traditional RNN, Mo-LSTM can improve the problem of gradient disappearance and gradient explosion.
The advantage of the Mo-LSTM is that it can store four states, the current and previous moment values of the output, and the current and previous moment values of the memory state vector. An LSTM cell consists of an input gate It, a forgetting gate Ft, an output gate Ot and a memory cell Ct. xt denotes the input data at moment t and the formulas are expressed as follows:
It=σ(Dixt+WiHt−1+bi) | (3.10) |
Ft=σ(Dfxt+WfHt−1+bf) | (3.11) |
Ut=tanh(Duxt+WuHt−1+bu) | (3.12) |
Ct=Ft∗Ct−1+It∗Ut | (3.13) |
Ot=σ(D0xt+W0Ht−1+b0) | (3.14) |
Ht=Ot∗tanh(Ct) | (3.15) |
where σ denotes the sigmoid activation function and tanh is also the activation function. D and W denote the weight matrices from the input layer and the hidden layer to each gate, respectively. Ht denotes the parameter state of the hidden layer. Ut denotes the intermediate temporary memory unit parameter state in the network transmission and Ct denotes the memory unit parameter state in the network transmission. bi,bf,bu and b0 denote the bias vectors of the four states of the network.
The experimental datasets in this case is from the fish farming laboratory of National Research Base of Intelligent Manufacturing Service, Chongqing Technology and Business University.
For the dataset Fish_status of fish condition management, after a 30-day video collection period, the videos of feeding periods were copied. Due to the storage period of the video recorder was 1 hour, the feeding videos were cropped using VSplayer to only retain the video segments from the beginning of feeding to the end of the fish feeding period. Each feeding segment is approximately 10 minutes long and about 200MB in size, with a total of 60 segments. The keyframe extraction algorithm was used to extract 24,000 images from the 60 feeding segments. The image resolution is 2560×1440, and the size of each image is approximately 700KB. 1000 images without any remaining feed on the water surface were selected from the original dataset as the dataset for measuring body length. 1000 images with remaining feed on the water surface and 1000 images without any remaining feed were manually selected as the training set, while 500 images were randomly selected as the test set. This dataset contains seven attributes of fish length, fish width, fish weight, water temperature, oxygen consumption rate, fish feeding frequency and feeding amount. The fish weight is predicted from the fish length and width data, the oxygen consumption rate is predicted from the fish weight and water temperature, and the feeding amount is predicted from the fish feeding frequency.
For dataset Water_quality_status of environmental condition management, a total of 4561 consecutive time-series real data were collected using sensors to detect water quality parameters. This dataset contains eight columns of water quality parameters: NO−2 - N (mg/L), NO−3 - N (mg/L), DO (mg/L), T, TN (mg/L), pH, COD (mg/L) and NH + 4 - N (mg/L). DO is an important factor in maintaining the stability of aquatic ecosystems, and can affect the respiration and metabolism of aquatic organisms. In industrial aquaculture systems, monitoring and regulating the level of DO can ensure the normal growth and reproduction of aquatic organisms. Temperature is an important factor that affects the growth and metabolism of aquatic organisms, and its changes can directly affect the physiology and behavior of aquatic organisms. In industrial aquaculture systems, controlling water temperature can promote the growth and immunity of organisms and improve the efficiency of aquaculture. PH is an indicator of the acidity and alkalinity of water, which has a certain impact on the metabolism and growth of aquatic organisms. In industrial aquaculture systems, maintaining a stable pH value of the water can promote the normal metabolism and immunity of organisms. NH + 4 - N, NO−2 - N, NO−3 - N and TN are common pollutants in water, and their content has a certain impact on the growth and health of aquatic organisms. In industrial aquaculture systems, monitoring and regulating the level of nitrogen can prevent the accumulation of pollutants in the water, ensuring the cleanliness of the water and the stability of the ecosystem. COD is an indicator of the content of organic matter in water, which can reflect the degree of water pollution. In industrial aquaculture systems, monitoring and controlling the level of chemical oxygen demand can prevent the excessive accumulation of organic matter in the water, ensuring the cleanliness of the water and the stability of the ecosystem.
Both Fish_status and Water_quality_status are raw sampled data and contain some unrealistic outliers. In order to improve the data quality, firstly, this experiment cleansed the data, removing duplicate data and dealing with missing values and outliers. Secondly, data normalisation was carried out to eliminate the effect of the difference in magnitude and range of values between different attributes on the final prediction results.
The fish state management based on multi-objective BP neural network, mainly using known data to achieve multi-objective prediction of fish weight, oxygen consumption rate and feeding amount. For the parameter settings of the Mo-BP model: the number of hidden layers is set to 2, each hidden layer contains 4 neurons, the number of training rounds is 1000, the Tanh function is selected as the activation function, and the division ratio of training set to test set is 80%. The learning rates were set to 0.01, 0.05 and 0.08 respectively to explore the best prediction of the model. In order to fully test the validity of the fish state management experiments, five commonly used evaluation metrics were used in this experiment: MAE, MSE, RMSE, R2, Adjusted_R2. Each group of experiments was performed five times and the average result was taken as the final valid value.
Based on multi-objective recurrent neural network environmental state management, mainly using Mo-LSTM model to process water quality parameters with historical time series characteristics to achieve the prediction of NO−2 - N, NO−3 - N, DO, T, TN, pH, COD and NH + 4 - N of a total of eight target water quality parameters. For the Mo-LSTM model settings: step size is set to 1, input_size is set to 7, hidden_size is set to 2, training rounds are 400 times, and the division ratio of training set to test set is 80%. The learning rate was set to 0.01, 0.05 and 0.08 for the three sets of experiments. In order to verify the validity of the environmental state management experiments, GRU and Bi-LSTM models were introduced for comparison and three typical and commonly used evaluation metrics were used: MAE, MSE and RMSE. Each group of experiments was performed five times and the average result was taken as the final valid value.
In terms of fish status management, this paper proposes a multi-objective prediction model. As shown in Table 1, the assessed target values for fish weight, oxygen consumption rate and feeding amount are indicated at learning rates of 0.01, 0.05 and 0.08 respectively. The analysis of the single target prediction shows that better prediction can be shown when the learning rate is 0.01. In an overall analysis, fish weight, oxygen consumption rate and feeding amount differed for different learning rates, but all tended to be consistently similar, which can effectively demonstrate the validity of the method.
Metrics | Fish weight | Oxygen consumption rate | Feeding amount | ||||||
lr=0.01 | lr=0.05 | lr=0.08 | lr=0.01 | lr=0.05 | lr=0.08 | lr=0.01 | lr=0.05 | lr=0.08 | |
MAE | 5.2866 | 5.6034 | 5.3497 | 0.9472 | 0.9482 | 0.9178 | 1.4090 | 1.5072 | 1.4937 |
MSE | 48.1733 | 48.8271 | 49.5273 | 1.2781 | 1.4721 | 1.2068 | 2.4721 | 2.5025 | 2.5284 |
RMSE | 6.9407 | 6.8276 | 7.0242 | 1.1305 | 1.2642 | 1.1972 | 1.5723 | 1.5798 | 1.6032 |
The prediction error analysis chart representing fish weight, oxygen consumption rate and feeding rate, as shown in Figure 3, provide a clearer analysis of the error in the model predictions. The blue curves in the chart indicate the true measured values and the orange curves indicate the results of the model predictions. As can be seen from Figure 3(a), the prediction error for fish weight is around 6.9g. The accuracy of the model prediction is relatively average. From Figure 3(b) it can be analysed that the error in the oxygen consumption rate of the fish floats at 1.1mg/L in a pond with an average fish weight of 15956.14g. That is, for every 10g of fish weight, the error in oxygen consumption rate fluctuates at 0.0007 mg/L and the model prediction is extremely accurate. From the analysis in Figure 3(c), it can be seen that 1.6g of the model fitted feeding error was introduced by the initial few feeding trials. This is evident from Table 1, whereas when fish feeding behaviour is evident, the model fitting error is not significant. Further analysis of the fitted curve in the chart shows that the curve approximates a primary function, indicating a significant positive correlation between feeding frequency and the amount that should be fed. This demonstrates that the method is a good predictor of feeding quantity to support accurate feeding decisions.
As shown in Figure 4, the R2 and Adjusted_R2 are introduced in this paper in order to better explore the interpretation and relevance of the input features to the target of the fish state. R2 is used to describe the degree to which the input variables explain the output variables, and Adjusted_R2 eliminates the effects of sample size and number of features on basis of R2, which better reflects the quality of the model assessment. Figure 4 shows the R2 for fish weight, oxygen consumption rate and feeding amount; Adjusted_R2 was applied to multiple linear regression, so Adjusted_R2 metrics for fish weight and oxygen consumption rate were tested. All data in Figure 4 are based on a learning rate of 0.01. As can be seen from the chart, the Adjusted_R2 can reach 0.96 in predicting the weight of fish, which indicates that both characteristics, fish length and fish width, are very explanatory for the weight and that the model is effective in predicting the weight of fish. In terms of fish oxygen consumption rate, the Adjusted_R2 can reach 0.97, which indicates that both fish weight and water temperature have strong explanations for fish oxygen consumption rate. In terms of feeding amount prediction analysis, it can reach an R2 of 0.9930, which indicates that the feeding frequency of the fish can influence the feeding amount by 99%.
In terms of environmental state management, an LSTM-based multi-object prediction model for water quality parameters is proposed in this paper. As shown in Table 2, this experiment tested the Co-LSTM proposed in this paper and the comparative models GRU and Bi-LSTM, yielding values for the eight prediction objects assessed at learning rates of 0.01, 0.05 and 0.08, respectively. In general, the difference in prediction error between the three models for the recycled water quality dataset is not significant, and the indicators of the model can reach a satisfactory stability at a learning rate of 0.01. As shown in Figure 5 and Figure 6, in order to observe the prediction effect of the model more comprehensively and directly, this paper visualizes the MAE and MSE indicators of water quality parameters under Co-LSTM, GRU and Bi-LSTM models. In order to meet the carbon source requirements for microbial growth and water purification, an external carbon source (glucose) is added to the biofilter in industrial aquaculture system to maintain sufficient COD concentration. Because the value of COD is adjusted manually and is very different from the other seven parameters, it is not shown in the figure. Further analysis revealed that if the model uses MAE as the main evaluation metric, Co-LSTM was found to be better than GRU and Bi-LSTM in predicting several water quality parameters, including NO−3 - N, DO, T, TN, pH, COD and NH + 4 - N, while GRU was slightly better in predicting NO−2 - N. Experience from artificial culture shows that the parameters that have a greater impact on water quality conditions are mainly DO, COD and NH + 4 - N. In the prediction of these water quality parameters, Mo-LSTM is superior to GRU and Bi-LSTM. Therefore, it is concluded that Mo-LSTM is superior to GRU and Bi-LSTM in the environmental state management prediction model.
Object | Model | lr=0.01 | lr=0.05 | lr=0.08 | |||
MAE | MSE | MAE | MSE | MAE | MSE | ||
NO−2 - N | Mo-LSTM | 0.0058 | 0.0008 | 0.0062 | 0.0011 | 0.0081 | 0.0013 |
GRU | 0.0024 | 0.0006 | 0.0027 | 0.0007 | 0.0031 | 0.0012 | |
Bi-LSTM | 0.0061 | 0.00011 | 0.0068 | 0.0007 | 0.0089 | 0.0021 | |
NO−3 - N | Mo-LSTM | 0.4627 | 0.2349 | 0.4537 | 0.2174 | 0.4921 | 0.3845 |
GRU | 0.4694 | 0.2287 | 0.4802 | 0.2088 | 0.5692 | 0.2901 | |
Bi-LSTM | 0.4657 | 0.2248 | 0.4731 | 0.2114 | 0.5233 | 0.3646 | |
DO | Mo-LSTM | 0.258 | 0.0873 | 0.2573 | 0.0892 | 0.2689 | 0.0952 |
GRU | 0.2563 | 0.0934 | 0.2597 | 0.0945 | 0.3082 | 0.1029 | |
Bi-LSTM | 0.2572 | 0.0892 | 0.2581 | 0.0918 | 0.2894 | 0.1005 | |
T | Mo-LSTM | 0.1778 | 0.0414 | 0.1853 | 0.0492 | 0.1728 | 0.0479 |
GRU | 0.3249 | 0.11 | 0.3381 | 0.1195 | 0.3317 | 0.1204 | |
Bi-LSTM | 0.2379 | 0.0817 | 0.2181 | 0.0837 | 0.2648 | 0.0953 | |
TN | Mo-LSTM | 0.2753 | 0.0809 | 0.2742 | 0.0800 | 0.2829 | 0.0926 |
GRU | 0.4017 | 0.1673 | 0.3902 | 0.1559 | 0.4192 | 0.1857 | |
Bi-LSTM | 0.3974 | 0.1185 | 0.3147 | 0.1192 | 0.3712 | 0.1203 | |
pH | Mo-LSTM | 0.0402 | 0.0029 | 0.0481 | 0.0039 | 0.0401 | 0.0018 |
GRU | 0.0471 | 0.0038 | 0.0512 | 0.0041 | 0.0425 | 0.0034 | |
Bi-LSTM | 0.0438 | 0.0037 | 0.0494 | 0.0052 | 0.0418 | 0.0027 | |
COD | Mo-LSTM | 1.9185 | 5.3993 | 1.9022 | 5.3210 | 1.8937 | 5.2877 |
GRU | 3.3124 | 22.1170 | 3.3019 | 22.0911 | 3.3298 | 23.0374 | |
Bi-LSTM | 2.8371 | 17.2849 | 2.6290 | 16.8407 | 2.8928 | 15.3779 | |
NH + 4 - N | Mo-LSTM | 0.0152 | 0.0003 | 0.0159 | 0.0014 | 0.0184 | 0.0017 |
GRU | 0.0165 | 0.0004 | 0.0169 | 0.0017 | 0.0216 | 0.0021 | |
Bi-LSTM | 0.0171 | 0.0003 | 0.0162 | 0.0019 | 0.0207 | 0.0019 |
As shown in Figure 7, this experiment also tested the RMSE values of the eight water quality parameters at different learning rate settings, which were analysed visually to increase the validity of the model evaluation. It is evident from the chart that the Mo-LSTM model represents a curve that lies steadily below the GRU and Bi-LSTM curves. In summary, the validity of using Mo-LSTM neural networks to construct models for the prediction of environmental water quality is verified.
The intelligent digital industrial aquaculture in this paper analyses the results of the experiments in terms of fish state management and environmental state management respectively. For the former, the results in Table 1, Figure 3 and Figure 4 can well demonstrate the superiority of multi-object BP neural network-based fish state management. For the latter, the results in Table 2, Figure 5, Figure 6 and Figure 7 can well demonstrate the superiority of multi-object recurrent neural network-based environmental state management. The realisation of these results can be attributed to two possible aspects.
On the one hand, the experimental data in this paper were collected autonomously by the experimental group, and the real reliability of the data can bring a great advantage to the training of the model. On the other hand, the construction of a multi-object deep neural network-based model brings more stability to the prediction results.
This paper successfully proposes a multi-object deep neural network-based intelligent management method for digital industrial aquaculture for effectively improving multiple business requirements in aquaculture. The method is divided into two parts: fish state management and environmental state management. On the one hand, the BP neural network based on double hidden layers is used to build a multi-objective prediction model to accurately predict the weight, oxygen consumption rate and feeding amount of fish. On the other hand, the LSTM-based neural network is used to build a multi-objective prediction model to realise the prediction of water quality parameters for the aquaculture environment. Promote the intelligent management of aquaculture by combining intelligent algorithm and data drive. The experiments on real datasets show that the Mo-DIA method proposed in this paper has high performance and feasibility. In the future work, it is expected that more useful data can be collected to increase the feature magnitude and adjust the scheme model framework, so as to better improve the efficiency and accuracy of the intelligent management scheme of digital industrial aquaculture.
This work was funded by the Researchers Supporting Project number (RSPD2023R681) King Saud University, Riyadh, Saudi Arabia, also funded by the Natural Science Foundation of Chongqing Science & Technology Commission (cstc2020jcyj- msxmX0721), Science and Technology Research Program of Chongqing Municipal Education Commission (KJQN202000810), Talent Introduction Funding of Chongqing Technology and Business University (2053031), and Teaching reform project of Chongqing Technology and Business University (212027).
[1] |
W. Li, G. Zhao, Y. Zhu, X. Lin, Y. Zhang, Research on compound braking control strategy of extended-range electric vehicle based on driving intention recognition, Comput. Intell. Neurosci., 2022 (2022), 8382873. https://doi.org/10.1155/2022/8382873 doi: 10.1155/2022/8382873
![]() |
[2] | X. Zhang, B. Zu, J. Zhou, S. Liang, Research on torque control method of hybrid electric vehicle based on driving intention recognition, in 2021 5th CAA International Conference on Vehicular Control and Intelligence (CVCI), (2021), 1–6. https://doi.org/10.1109/CVCI54083.2021.9661243 |
[3] |
Y. Guo, H. Zhang, C. Wang, Q. Sun, W. Li, Driver lane change intention recognition in the connected environment, Phys. A Statist. Mechan. Appl., 575 (2021), 126057. https://doi.org/10.1016/j.physa.2021.126057 doi: 10.1016/j.physa.2021.126057
![]() |
[4] |
S. Wang, X. Zhao, Q. Yu, Vehicle stability control strategy based on recognition of driver turning intention for dual-motor drive electric vehicle, Math. Problems Eng., 2020 (2020), 3143620. https://doi.org/10.1155/2020/3143620 doi: 10.1155/2020/3143620
![]() |
[5] |
Y. Liu, P. Zhao, D. Qin, G. Li, Y. Zhang, Driving intention identification based on long short-term memory and a case study in shifting strategy optimization, IEEE Access, 7 (2019), 128593–128605. https://doi.org/10.1109/ACCESS.2019.2940114 doi: 10.1109/ACCESS.2019.2940114
![]() |
[6] |
L. Tang, H. Wang, W. Zhang, Z. Mei, L. Li, Driver lane change intention recognition of intelligent vehicle based on long short-term memory network, IEEE Access, 8 (2020), 136898–136905. https://doi.org/10.1109/ACCESS.2020.3011550 doi: 10.1109/ACCESS.2020.3011550
![]() |
[7] |
Q. Shangguan, T. Fu, J. Wang, S. Fang, L. Fu, A proactive lane-changing risk prediction framework considering driving intention recognition and different lane-changing patterns, Accident Anal. Prevent., 164 (2022), 106500. https://doi.org/10.1016/j.aap.2021.106500 doi: 10.1016/j.aap.2021.106500
![]() |
[8] |
K. Gao, P. Luo, J. Xie, B. Chen, Y. Wu, R. H. Du, Energy management of plug-in hybrid electric vehicles based on speed prediction fused driving intention and LIDAR, Energy, 284 (2023), 128535. https://doi.org/10.1016/j.energy.2023.128535 doi: 10.1016/j.energy.2023.128535
![]() |
[9] |
J. Ju, L. Bi, A. G. Feleke, Noninvasive neural signal-based detection of soft and emergency braking intentions of drivers, Biomed. Signal Process. Control, 72 (2022), 103330. https://doi.org/10.1016/j.bspc.2021.103330 doi: 10.1016/j.bspc.2021.103330
![]() |
[10] | T. Han, J. Jing, Ü. Özgüner, Driving intention recognition and lane change prediction on the highway, in 2019 IEEE Intelligent Vehicles Symposium (IV), (2019), 957–962. https://doi.org/10.1109/IVS.2019.8813987 |
[11] |
Q. Shangguan, T. Fu, J. Wang, S. Fang, L. Fu, A proactive lane-changing risk prediction framework considering driving intention recognition and different lane-changing patterns, Accident Anal. Prevent., 164 (2022), 106500. https://doi.org/10.1016/j.aap.2021.106500 doi: 10.1016/j.aap.2021.106500
![]() |
[12] |
R. Yuan, M. Abdel-Aty, X. Gu, O. Zheng, Q. Xiang, A unified modeling framework for lane change intention recognition and vehicle status prediction, Phys. A Statist. Mechan. Appl., 632 (2023), 129332. https://doi.org/10.1016/j.physa.2023.129332 doi: 10.1016/j.physa.2023.129332
![]() |
[13] | C. Zong, C. Wang, D. Yang, H. Yang, Driving intention identification and maneuvering behavior prediction of drivers on cornering, in 2009 International Conference on Mechatronics and Automation, (2009), 4055–4060. https://doi.org/10.1109/ICMA.2009.5246638 |
[14] | F. Zhao, B. Xie, Y. Tian, Multi-parameter driver intention recognition based on neural network, in 2020 4th CAA International Conference on Vehicular Control and Intelligence (CVCI), (2020), 130–135. https://doi.org/10.1109/CVCI51460.2020.9338444 |
[15] |
S. Liu, K. Zheng, L. Zhao, P. Fan, A driving intention prediction method based on hidden Markov model for autonomous driving, Computer Commun., 157 (2020), 143–149. https://doi.org/10.1016/j.comcom.2020.04.021 doi: 10.1016/j.comcom.2020.04.021
![]() |
[16] |
H. Ma, Y. Wang, R. Xiong, S. Kodagoda, L. Tang, DeepGoal: Learning to drive with driving intention from human control demonstration, Robotics Autonom. Syst., 127 (2020), 103477. https://doi.org/10.1016/j.robot.2020.103477 doi: 10.1016/j.robot.2020.103477
![]() |
[17] | Y. Li, H. Hong, D. Luigi, Y. Ye, Research on driving control strategy of an electric racing car based on pattern recognition, in Proceedings of China SAE Congress 2021: Selected Papers, 818 (2023), 578–591. https://doi.org/10.1007/978-981-19-3842-9_46 |
[18] |
J. Wang, Y. Cai, L. Chen, D. Shi, R. Wang, Z. Zhu, Review on multi-power sources dynamic coordinated control of hybrid electric vehicle during driving mode transition process, Int. J. Energy Res., 44 (2020), 6128–6148. https://doi.org/10.1002/er.5264 doi: 10.1002/er.5264
![]() |
[19] |
T. Pan, H. Zang, Anti-jerking and traction torque compensation strategy for P3+ hybrid electric vehicle during power upshift, Proceed. Instit. Mechan. Eng. Part D J. Autom. Eng., 237 (2023), 587–606. https://doi.org/10.1177/09544070221085384 doi: 10.1177/09544070221085384
![]() |
[20] |
J. Hu, C. Sun, J. Xiao, J. Li, A torque compensation strategy in two-speed automated mechanical transmission shift process for pure electric vehicles, Adv. Mechan. Eng., 7 (2015), 441–444. https://doi.org/10.1177/1687814015616915 doi: 10.1177/1687814015616915
![]() |
[21] |
S. Xu, L. Wei, X. Zhang, Z. Bai, Y. Jiao, Research on Multi-Mode Drive Optimization Control Strategy of Four-Wheel-Drive Electric Vehicles with Multiple Motors, Sustainability, 14 (2022), 7378. https://doi.org/10.3390/su14127378 doi: 10.3390/su14127378
![]() |
[22] |
S. Kitayama, M. Saikyo, Y. Nishio, K. Tsutsumi, Torque control strategy and optimization for fuel consumption and emission reduction in parallel hybrid electric vehicles, Struct. Multidisc. Optim., 52 (2015), 595–611. https://doi.org/10.1007/s00158-015-1254-8 doi: 10.1007/s00158-015-1254-8
![]() |
[23] |
F. Zhang, X. Hu, R. Langari, L. Wang, Y. Cui, H. Pang, Adaptive energy management in automated hybrid electric vehicles with flexible torque request, Energy, 214 (2020), 118873. https://doi.org/10.1016/j.energy.2020.118873 doi: 10.1016/j.energy.2020.118873
![]() |
[24] |
S. Li, B. Sarlioglu, S. Jurkovic, N. R. Patel, P. Savagian, Comparative analysis of torque compensation control algorithms of interior permanent magnet machines for automotive applications considering the effects of temperature variation, IEEE Trans. Transp. Electrif., 3 (2017), 668–681. https://doi.org/10.1109/TTE.2017.2684080 doi: 10.1109/TTE.2017.2684080
![]() |
[25] |
Z. Yu, Y. Hou, B. Leng, L. Xiong, Y. Li, Disturbance compensation torque coordinated control of four in-wheel motor independent-drive electric vehicles, IEEE Access, 8 (2022), 119758–119767. https://doi.org/10.1109/ACCESS.2020.3005943. doi: 10.1109/ACCESS.2020.3005943
![]() |
[26] |
H. Wei, N. Zhang, J. Liang, Q. Ai, W. Zhao, T. Huang, et al., Deep reinforcement learning based direct torque control strategy for distributed drive electric vehicles considering active safety and energy saving performance, Energy, 238 (2022), 121725. https://doi.org/10.1016/j.energy.2021.121725 doi: 10.1016/j.energy.2021.121725
![]() |
[27] |
H. Wei, Q. A, W. Zhao, Y. Zhang, Modelling and experimental validation of an EV torque distribution strategy towards active safety and energy efficiency, Energy, 239 (2022), 121953. https://doi.org/10.1016/j.energy.2021.121953 doi: 10.1016/j.energy.2021.121953
![]() |
[28] |
X. Hu, P. Wang, Y. Hu, H. Chen, A stability-guaranteed and energy-conserving torque distribution strategy for electric vehicles under extreme conditions, Appl. Energy, 259 (2020), 114162. https://doi.org/10.1016/j.apenergy.2019.114162 doi: 10.1016/j.apenergy.2019.114162
![]() |
[29] |
J. Hu, J. Li, Z. Hu, B. Zhang, L. F. Xu, M. G. Ouyang, Energy-efficient torque-allocation strategy for a 6×6 vehicle using electric wheels, eTransportation, 10 (2021), 100136. https://doi.org/10.1016/j.etran.2021.100136 doi: 10.1016/j.etran.2021.100136
![]() |
[30] |
J. Wang, S. Gao, K. Wang, Y. Wang, Q. Wang, Wheel torque distribution optimization of four-wheel independent-drive, Control Eng. Pract., 110 (2021), https://doi.org/10.1016/j.conengprac.2021.104779 doi: 10.1016/j.conengprac.2021.104779
![]() |
[31] |
X. Zhang, D. Göhlich, J. Li, Energy-efficient toque allocation design of traction and regenerative braking for distributed drive electric vehicles, IEEE Transact. Vehic. Technol., 67 (2018), 285–295. https://doi.org/10.1109/TVT.2017.2731525 doi: 10.1109/TVT.2017.2731525
![]() |
[32] |
Q. Han, L. Zhu, J. Wang, D. Chen, Hopf-curve-based torque distribution strategy for avoiding limit cycle vibration in hybrid braking system model, Int. J. Non-Linear Mechan., 154 (2023), 104440. https://doi.org/10.1016/j.ijnonlinmec.2023.104440 doi: 10.1016/j.ijnonlinmec.2023.104440
![]() |
[33] |
Y. Jiang, H. Meng, G. Chen, C. Yang, X. Xu, L. Zhang, Differential-steering based path tracking control and energy-saving torque distribution strategy of 6WID unmanned ground vehicle, Energy, 254 (2022), 124209. https://doi.org/10.1016/j.energy.2022.124209 doi: 10.1016/j.energy.2022.124209
![]() |
[34] |
Z. Zhang, T. Zhang, J. Hong, H. Zhang, J. Yang, Entropy-based torque control strategy of Mechanical–Electric–Hydraulic Power Coupling Vehicles, Control Eng. Pract., 133 (2023), 105437. https://doi.org/10.1016/j.conengprac.2023.105437 doi: 10.1016/j.conengprac.2023.105437
![]() |
[35] |
C. Geng, B. Jin, X. Zhang, Research on torque control strategy for electric vehicle with in-wheel motor, IFAC-PapersOnLine, 51 (2018), 71–74. https://doi.org/10.1016/j.ifacol.2018.10.014 doi: 10.1016/j.ifacol.2018.10.014
![]() |
[36] |
A. I. M. Almadi, R. E. Al Mamlook, Y. Almarhabi, I. Ullah, A. Jamal, N.Bandara, A fuzzy-logic approach based on driver decision-making behavior modeling and simulation, Sustainability, 14 (2022), 8874. https://doi.org/10.3390/su14148874 doi: 10.3390/su14148874
![]() |
[37] | J. Heine, M. Sylla, I. Langer, T. Schramm, B. Abendroth, R. Bruder, Algorithm for driver intention detection with fuzzy logic and edit distance, in 2015 IEEE 18th International Conference on Intell. Trans. Syst., (2015), 1022–1027. https://doi.org/10.1109/ITSC.2015.170 |
[38] |
W. Qi, Fuzzy control strategy of pure electric vehicle based on driving intention recognition, J. Intell. Fuzzy Syst. Appl. Eng. Technol., 39 (2020), 5131–5139. https://doi.org/10.3233/JIFS-179998 doi: 10.3233/JIFS-179998
![]() |
[39] |
F. Liu, A PMSM fuzzy logic regenerative braking control strategy for electric vehicles, J. Intell. Fuzzy Syst., 41 (2021), 4873–4881.https://doi.org/10.3233/JIFS-189972 doi: 10.3233/JIFS-189972
![]() |
[40] |
S. Wang, X. Huang, J. M. López. X. Xu. P. Dong, Fuzzy adaptive-equivalent consumption minimization strategy for a parallel hybrid electric vehicle, IEEE Access, 7 (2019), 133290–133303. https://doi.org/10.1109/ACCESS.2019.2941399 doi: 10.1109/ACCESS.2019.2941399
![]() |
[41] |
K. Kakouche, T. Rekioua, S. Mezani, A. Oubelaid, D. Rekioua, V. Blazek, et al., Model predictive direct torque control and fuzzy logic energy management for multi power source electric vehicles, Sensors, 22 (2022), 5669. https://doi.org/10.3390/s22155669 doi: 10.3390/s22155669
![]() |
1. | Xiaohong Peng, Tianyu Zhou, Zhenlu Wu, Zhao Li, 2023, A Survey of Deep Learning for Intelligent Feeding in Smart Fish Farming, 9798400716485, 590, 10.1145/3653081.3653179 | |
2. | Sherine Ragab, Seyed Hossein Hoseinifar, Hien Van Doan, Waldemar Rossi, Simon Davies, Mohamed Ashour, Ehab El-Haroun, Overview of aquaculture Artificial Intelligence (AAI) applications: enhance sustainability and productivity, reduce labor costs, and increase the quality of aquatic products, 2024, 2300-8733, 10.2478/aoas-2024-0075 | |
3. | Juqiang Feng, Feng Cai, Long Wu, Xing Zhang, Kaifeng Huang, State of charge estimation for lithium‐ion battery pack based on real vehicle data and optimized backpropagation method by adaptive cross mutation sparrow search algorithm, 2024, 12, 2050-0505, 896, 10.1002/ese3.1656 | |
4. | Zheng Zhang, Xiang Lu, Shouqi Cao, An efficient detection model based on improved YOLOv5s for abnormal surface features of fish, 2024, 21, 1551-0018, 1765, 10.3934/mbe.2024076 | |
5. | Thales Francisco Gonçalves , Johanna Marcela Concha Obando, Luiz Cláudio Chiavani Júnior, Ana Paula Andrade-Santos, Esthefany Caroline França Silva, Thalisia Cunha dos Santos, Roberto Kazuyoshi Naoe, Érico Tadao Teramoto, Guilherme Wolff Bueno, Piscicultura inteligente: a integração das Tecnologia 4.0 e “Business Intelligence” para gestão ágil na aquicultura, 2025, 16, 2178-9010, e4524, 10.7769/gesec.v16i1.4524 |
Metrics | Fish weight | Oxygen consumption rate | Feeding amount | ||||||
lr=0.01 | lr=0.05 | lr=0.08 | lr=0.01 | lr=0.05 | lr=0.08 | lr=0.01 | lr=0.05 | lr=0.08 | |
MAE | 5.2866 | 5.6034 | 5.3497 | 0.9472 | 0.9482 | 0.9178 | 1.4090 | 1.5072 | 1.4937 |
MSE | 48.1733 | 48.8271 | 49.5273 | 1.2781 | 1.4721 | 1.2068 | 2.4721 | 2.5025 | 2.5284 |
RMSE | 6.9407 | 6.8276 | 7.0242 | 1.1305 | 1.2642 | 1.1972 | 1.5723 | 1.5798 | 1.6032 |
Object | Model | lr=0.01 | lr=0.05 | lr=0.08 | |||
MAE | MSE | MAE | MSE | MAE | MSE | ||
NO−2 - N | Mo-LSTM | 0.0058 | 0.0008 | 0.0062 | 0.0011 | 0.0081 | 0.0013 |
GRU | 0.0024 | 0.0006 | 0.0027 | 0.0007 | 0.0031 | 0.0012 | |
Bi-LSTM | 0.0061 | 0.00011 | 0.0068 | 0.0007 | 0.0089 | 0.0021 | |
NO−3 - N | Mo-LSTM | 0.4627 | 0.2349 | 0.4537 | 0.2174 | 0.4921 | 0.3845 |
GRU | 0.4694 | 0.2287 | 0.4802 | 0.2088 | 0.5692 | 0.2901 | |
Bi-LSTM | 0.4657 | 0.2248 | 0.4731 | 0.2114 | 0.5233 | 0.3646 | |
DO | Mo-LSTM | 0.258 | 0.0873 | 0.2573 | 0.0892 | 0.2689 | 0.0952 |
GRU | 0.2563 | 0.0934 | 0.2597 | 0.0945 | 0.3082 | 0.1029 | |
Bi-LSTM | 0.2572 | 0.0892 | 0.2581 | 0.0918 | 0.2894 | 0.1005 | |
T | Mo-LSTM | 0.1778 | 0.0414 | 0.1853 | 0.0492 | 0.1728 | 0.0479 |
GRU | 0.3249 | 0.11 | 0.3381 | 0.1195 | 0.3317 | 0.1204 | |
Bi-LSTM | 0.2379 | 0.0817 | 0.2181 | 0.0837 | 0.2648 | 0.0953 | |
TN | Mo-LSTM | 0.2753 | 0.0809 | 0.2742 | 0.0800 | 0.2829 | 0.0926 |
GRU | 0.4017 | 0.1673 | 0.3902 | 0.1559 | 0.4192 | 0.1857 | |
Bi-LSTM | 0.3974 | 0.1185 | 0.3147 | 0.1192 | 0.3712 | 0.1203 | |
pH | Mo-LSTM | 0.0402 | 0.0029 | 0.0481 | 0.0039 | 0.0401 | 0.0018 |
GRU | 0.0471 | 0.0038 | 0.0512 | 0.0041 | 0.0425 | 0.0034 | |
Bi-LSTM | 0.0438 | 0.0037 | 0.0494 | 0.0052 | 0.0418 | 0.0027 | |
COD | Mo-LSTM | 1.9185 | 5.3993 | 1.9022 | 5.3210 | 1.8937 | 5.2877 |
GRU | 3.3124 | 22.1170 | 3.3019 | 22.0911 | 3.3298 | 23.0374 | |
Bi-LSTM | 2.8371 | 17.2849 | 2.6290 | 16.8407 | 2.8928 | 15.3779 | |
NH + 4 - N | Mo-LSTM | 0.0152 | 0.0003 | 0.0159 | 0.0014 | 0.0184 | 0.0017 |
GRU | 0.0165 | 0.0004 | 0.0169 | 0.0017 | 0.0216 | 0.0021 | |
Bi-LSTM | 0.0171 | 0.0003 | 0.0162 | 0.0019 | 0.0207 | 0.0019 |
Metrics | Fish weight | Oxygen consumption rate | Feeding amount | ||||||
lr=0.01 | lr=0.05 | lr=0.08 | lr=0.01 | lr=0.05 | lr=0.08 | lr=0.01 | lr=0.05 | lr=0.08 | |
MAE | 5.2866 | 5.6034 | 5.3497 | 0.9472 | 0.9482 | 0.9178 | 1.4090 | 1.5072 | 1.4937 |
MSE | 48.1733 | 48.8271 | 49.5273 | 1.2781 | 1.4721 | 1.2068 | 2.4721 | 2.5025 | 2.5284 |
RMSE | 6.9407 | 6.8276 | 7.0242 | 1.1305 | 1.2642 | 1.1972 | 1.5723 | 1.5798 | 1.6032 |
Object | Model | lr=0.01 | lr=0.05 | lr=0.08 | |||
MAE | MSE | MAE | MSE | MAE | MSE | ||
NO−2 - N | Mo-LSTM | 0.0058 | 0.0008 | 0.0062 | 0.0011 | 0.0081 | 0.0013 |
GRU | 0.0024 | 0.0006 | 0.0027 | 0.0007 | 0.0031 | 0.0012 | |
Bi-LSTM | 0.0061 | 0.00011 | 0.0068 | 0.0007 | 0.0089 | 0.0021 | |
NO−3 - N | Mo-LSTM | 0.4627 | 0.2349 | 0.4537 | 0.2174 | 0.4921 | 0.3845 |
GRU | 0.4694 | 0.2287 | 0.4802 | 0.2088 | 0.5692 | 0.2901 | |
Bi-LSTM | 0.4657 | 0.2248 | 0.4731 | 0.2114 | 0.5233 | 0.3646 | |
DO | Mo-LSTM | 0.258 | 0.0873 | 0.2573 | 0.0892 | 0.2689 | 0.0952 |
GRU | 0.2563 | 0.0934 | 0.2597 | 0.0945 | 0.3082 | 0.1029 | |
Bi-LSTM | 0.2572 | 0.0892 | 0.2581 | 0.0918 | 0.2894 | 0.1005 | |
T | Mo-LSTM | 0.1778 | 0.0414 | 0.1853 | 0.0492 | 0.1728 | 0.0479 |
GRU | 0.3249 | 0.11 | 0.3381 | 0.1195 | 0.3317 | 0.1204 | |
Bi-LSTM | 0.2379 | 0.0817 | 0.2181 | 0.0837 | 0.2648 | 0.0953 | |
TN | Mo-LSTM | 0.2753 | 0.0809 | 0.2742 | 0.0800 | 0.2829 | 0.0926 |
GRU | 0.4017 | 0.1673 | 0.3902 | 0.1559 | 0.4192 | 0.1857 | |
Bi-LSTM | 0.3974 | 0.1185 | 0.3147 | 0.1192 | 0.3712 | 0.1203 | |
pH | Mo-LSTM | 0.0402 | 0.0029 | 0.0481 | 0.0039 | 0.0401 | 0.0018 |
GRU | 0.0471 | 0.0038 | 0.0512 | 0.0041 | 0.0425 | 0.0034 | |
Bi-LSTM | 0.0438 | 0.0037 | 0.0494 | 0.0052 | 0.0418 | 0.0027 | |
COD | Mo-LSTM | 1.9185 | 5.3993 | 1.9022 | 5.3210 | 1.8937 | 5.2877 |
GRU | 3.3124 | 22.1170 | 3.3019 | 22.0911 | 3.3298 | 23.0374 | |
Bi-LSTM | 2.8371 | 17.2849 | 2.6290 | 16.8407 | 2.8928 | 15.3779 | |
NH + 4 - N | Mo-LSTM | 0.0152 | 0.0003 | 0.0159 | 0.0014 | 0.0184 | 0.0017 |
GRU | 0.0165 | 0.0004 | 0.0169 | 0.0017 | 0.0216 | 0.0021 | |
Bi-LSTM | 0.0171 | 0.0003 | 0.0162 | 0.0019 | 0.0207 | 0.0019 |