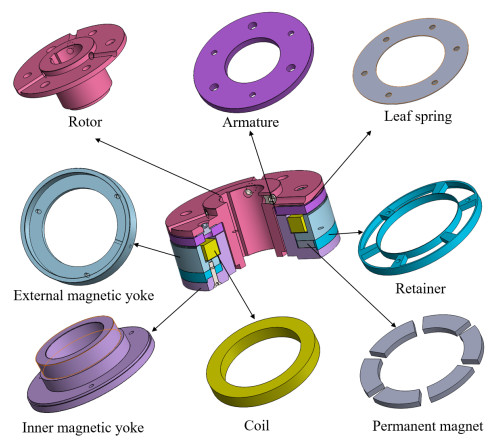
Permanent magnet brake (PMB) is a safe and effective braking mechanism used to stop and hold the load in place. Due to its complex structure and high reliability, assessing the reliability of PMB remains a challenge. The main difficulty lies in that there are several performance indicators reflecting the health state of PMB, and they are correlated with each other. In order to assess the reliability of PMB more accurately, a constant stress accelerated degradation test (ADT) is carried out to collect degradation data of two main performance indicators in PMB. An accelerated bivariate Wiener degradation model is proposed to analyse the ADT data. In the proposed model, the relationship between degradation rate and stress levels is described by Arrhenius model, and a common random effect is introduced to describe the unit-to-unit variation and correlation between the two performance indicators. The Markov Chain Monte Carlo (MCMC) algorithm is performed to obtain the point and interval estimates of the model parameters. Finally, the proposed model and method are applied to analyse the accelerated degradation data of PMB, and the results show that the reliability of PMB at the used condition can be quantified quite well.
Citation: Jihong Pang, Chaohui Zhang, Xinze Lian, Yichao Wu. Reliability assessment of permanent magnet brake based on accelerated bivariate Wiener degradation process[J]. Mathematical Biosciences and Engineering, 2023, 20(7): 12320-12340. doi: 10.3934/mbe.2023548
[1] | Fang Luo, Yuan Cui, Xu Wang, Zhiliang Zhang, Yong Liao . Adaptive rotation attention network for accurate defect detection on magnetic tile surface. Mathematical Biosciences and Engineering, 2023, 20(9): 17554-17568. doi: 10.3934/mbe.2023779 |
[2] | Hui-Yu Huang, Wei-Chang Tsai . An effcient motion deblurring based on FPSF and clustering. Mathematical Biosciences and Engineering, 2019, 16(5): 4036-4052. doi: 10.3934/mbe.2019199 |
[3] | Peihua Jiang, Longmei Shi . Statistical inference for a competing failure model based on the Wiener process and Weibull distribution. Mathematical Biosciences and Engineering, 2024, 21(2): 3146-3164. doi: 10.3934/mbe.2024140 |
[4] | Xueyan Wang . A fuzzy neural network-based automatic fault diagnosis method for permanent magnet synchronous generators. Mathematical Biosciences and Engineering, 2023, 20(5): 8933-8953. doi: 10.3934/mbe.2023392 |
[5] | K. Maqbool, S. Shaheen, A. M. Siddiqui . Effect of nano-particles on MHD flow of tangent hyperbolic fluid in a ciliated tube: an application to fallopian tube. Mathematical Biosciences and Engineering, 2019, 16(4): 2927-2941. doi: 10.3934/mbe.2019144 |
[6] | Bin Liu, Dengxiu Yu, Xing Zeng, Dianbiao Dong, Xinyi He, Xiaodi Li . Practical discontinuous tracking control for a permanent magnet synchronous motor. Mathematical Biosciences and Engineering, 2023, 20(2): 3793-3810. doi: 10.3934/mbe.2023178 |
[7] | Virginia Giorno, Amelia G. Nobile . Exact solutions and asymptotic behaviors for the reflected Wiener, Ornstein-Uhlenbeck and Feller diffusion processes. Mathematical Biosciences and Engineering, 2023, 20(8): 13602-13637. doi: 10.3934/mbe.2023607 |
[8] | M. Nagy, M. H. Abu-Moussa, Adel Fahad Alrasheedi, A. Rabie . Expected Bayesian estimation for exponential model based on simple step stress with Type-I hybrid censored data. Mathematical Biosciences and Engineering, 2022, 19(10): 9773-9791. doi: 10.3934/mbe.2022455 |
[9] | Anh Quang Tran, Tien-Anh Nguyen, Phuc Thinh Doan, Duc-Nghia Tran, Duc-Tan Tran . Parallel magnetic resonance imaging acceleration with a hybrid sensing approach. Mathematical Biosciences and Engineering, 2021, 18(3): 2288-2302. doi: 10.3934/mbe.2021116 |
[10] | José Alejandro Fernández Ramírez, Óscar Hernández-Uribe, Leonor Adriana Cárdenas-Robledo, Alfredo Chávez Luna . Universal fixation system for pad printing of plastic parts. Mathematical Biosciences and Engineering, 2023, 20(12): 21032-21048. doi: 10.3934/mbe.2023930 |
Permanent magnet brake (PMB) is a safe and effective braking mechanism used to stop and hold the load in place. Due to its complex structure and high reliability, assessing the reliability of PMB remains a challenge. The main difficulty lies in that there are several performance indicators reflecting the health state of PMB, and they are correlated with each other. In order to assess the reliability of PMB more accurately, a constant stress accelerated degradation test (ADT) is carried out to collect degradation data of two main performance indicators in PMB. An accelerated bivariate Wiener degradation model is proposed to analyse the ADT data. In the proposed model, the relationship between degradation rate and stress levels is described by Arrhenius model, and a common random effect is introduced to describe the unit-to-unit variation and correlation between the two performance indicators. The Markov Chain Monte Carlo (MCMC) algorithm is performed to obtain the point and interval estimates of the model parameters. Finally, the proposed model and method are applied to analyse the accelerated degradation data of PMB, and the results show that the reliability of PMB at the used condition can be quantified quite well.
The PMB is the core component of the braking system for manufacturing equipment. It plays an important role in ensuring the safe operation of manufacturing equipment [1]. It achieves braking by driving the armature through the magnetic force generated by the permanent magnets. Compared to other brakes, it has the advantage of small size and high torque. However, in the actual use process, the PMB is always running in a high temperature environment. This can lead to a certain degree of degradation of the components of the PMB, such as the leaf springs, coils, and permanent magnets, which can affect its performance. As the core component of the braking system, the reliability of the PMB directly affects the service life of the manufacturing equipment. Therefore, it is necessary to analyse the reliability of PMB in order to ensure the safe and stable operation of manufacturing equipment [2,3].
The evaluation of product reliability based on conventional life tests or accelerated life test (ALT) requires observations of the product's failure. Such testing methods are expensive in terms of human and financial resources and do not meet practical needs, because the PMB is a class of high-reliability and long-life product. The ADT has overcome the shortcomings of the ALT [4,5]. The degradation of PMB performance is accelerated by increasing the test stress levels to obtain sufficient degradation information and to evaluate the reliability at normal stress levels. Compared with ALT, ADT can save a large number of samples and test time, further improving test efficiency [6].
Analysing ADT data analysis is mainly based on the degradation models and acceleration function between degradation and stress levels [7,8]. The degradation model of performance characteristics describes the law of product performance degradation with time, while the acceleration function is used to characterize the relationship between the degradation rate of the performance indicators and the stress level. The commonly used degradation models include various stochastic processes, including Wiener process [9], gamma process [10], and inverse Gaussian process [11]. The gamma process and inverse Gaussian process are mainly used to describe the monotone performance degradation process. The Wiener process is a Brownian motion diffusion process, which is suitable for modeling non-monotonic performance degradation with independent Gaussian increments [12,13]. Wiener process has been widely used for reliability analysis in ADT. For instance, Hou et al. [14] proposed an improved random-effects ADT Wiener process for modeling the capacity degradation of Li-ion batteries. Zhai et al. [15] used the inverse Gaussian distribution to characterize the cellular heterogeneity of degradation pathways in the degradation modeling of the Wiener process, and the model is applied to analyse the ADT data of LED. Yan et al. [16] proposed an accelerated Wiener degradation model integrated by rate-volatility correlations and systematic error. Ye et al. [17] considered an accelerated degradation model based on Wiener process with nonlinear time scale function and random effects when interaction effects exist among stresses. Jiang et al. [18] proposed a method of constructing generalized confidence intervals of reliability function and quantile lifetime based on constant stress accelerated degradation Wiener model, and considered optimum plan problem by minimizing the mean of the upper prediction limit for the degradation characteristic at the design stress level. Jiang and Yang [19] proposed a dual-accelerated degradation test model based on Wiener process. The point estimation of model parameters is obtained by constructing a regression model. Based on point estimation of model parameters, generalized likelihood estimation of model parameters is obtained by constructing generalized prediction function. Ma et al. [20] proposes a Wiener process-based degradation model to predict the remaining useful life of equipment under imperfect maintenance. The model includes beta distribution to account for the residual degradation coefficient caused by maintenance. Random variables are used to reflect unit heterogeneity, and analytical forms of remaining useful life are obtained through the convolution operator.
The literature mentioned above involves only one degradation performance indicator. However, due to the complexity of their operating conditions and complicated structure, there usually exist multiple failure modes for PMBs. In such a case, a single performance indicator cannot accurately and comprehensively reflect their health status. Therefore, it is necessary to consider the use of multiple performance indicators to characterize the health status of PMB.
In recent years, researches on multivariate degradation modeling using copula functions have developed rapidly, mainly due to the flexibility of copula function. By substituting the marginal distributions into copula function, correlation structure among random variables can be automatically embedded [21]. Chen et al. [22] use a variety of stochastic processes to describe the mechanical seal performance degradation process, and the correlation between the performance indicators is described by different types of copula functions. Peng et al. [23] proposed a novel bivariate degradation model based on inverse Gaussian processes and copulas. Fang et al. [24] proposed a framework of bivariate stochastic process model to analyse accelerated degradation data by copula function. However, different copula functions may be suitable for different degradation data. The selection of an optimal copula function and how to interpret copula function from physical viewpoint are still limited. Recently, Song and Cui [25] proposed a bivariate gamma degradation model that naturally captures the correlation between two degradation processes through a common random effect, and expectation-maximization algorithm is used to estimate the model parameters. Fang et al. [26] proposed a novel multivariate degradation model based on inverse Gaussian process, where the correlation is incorporated by multivariate normal distributed random effects. Zhai and Ye [27] proposed an analytical multivariate Wiener process model. The model introduces a common stochastic time scale shared by all performance indicators to describe dependencies from the dynamic operating environment. Conditional on the time scale, the degradation of each performance indicator is modeled as the sum of two independent Wiener processes, one of which represents the common effect shared by all performance indicators, and the other represents the degradation caused by performance indicator itself. Yan et al. [28] used multiple correlated Wiener processes to model the degradation process of multiple performance indicators when common environmental condition is taken into account. They utilized a commonly shared environmental condition function to incorporates both the degradation correlation and random effect. The proposed model is also extended to a two-stage degradation process in order to correlate multiple performance indicators at each stage.
To address the problems in the reliability assessment of PMB, an accelerated degradation model for PMB based on the bivariate Wiener process is proposed. The main contributions of this paper are as follows: 1) The degradation process of the two performance indicators is modelled separately based on the Wiener process, which greatly improves the accuracy of the reliability assessment. 2) A common random effect is introduced in the proposed model to represent the correlation between the two performance indicators, which describes both the common factors affecting the degradation of the two performance indicators and the unit variation. 3) The relationship between the drift coefficient and the accelerated stress level is described using the Arrhenius formula. 4) A Gibbs sampling-based MCMC algorithm is used to achieve Bayesian updating of the model parameters. Finally, the validity of the model and method is verified by the accelerated degradation data of a PMB. The rest of this paper is organized as follows. Section 3 describes the acceleration test procedure for PMB. Section 3 presents an acceleration model based on a bivariate Wiener process. Section 4 verifies the validity of the proposed model through the practical application of the constant stress ADT data of the PMB. In Section 5, the conclusions of this paper are presented.
A PMB is a device that uses permanent magnetism generated by permanent magnets to drive the rotor and clamp the magnetic yoke to achieve braking. PMB is notable for their small size, high torque, and robust resistance to interference, making them a popular choice in a range of applications. The PMB studied in this paper is manufactured by a company located in Zhejiang province, China. This type of PMB is primarily used for braking servo motors of injection molding machines, and its performance directly impacts the reliability and safety of the injection molding machine. The structure of the PMB is shown in Figure 1, and its physical diagram is depicted in Figure 2. It can be seen from the structural diagram that the PMB consists of a rotor, leaf spring, armature, coil, external magnetic yoke, permanent magnet, retainer, and inner magnetic yoke. Due to the complexity of the operating conditions inside injection molding machines, PMBs may operate in high-temperature environments, which can lead to degradation of the plate springs, coils, and permanent magnets. Inadequate braking torque and slow response time are the main failure modes of these brakes during the aging process. Brake torque, a key performance indicator, is largely influenced by the permanent magnets, which may undergo irreversible demagnetization at high temperatures due to poor thermal stability in materials like NdFeB (N35SH). The response time of the armature, a significant characteristic of PMB, relies on the cooperation of the coil, leaf spring, and permanent magnet. The coil generates an electromagnetic force when a current is produced by switching on the voltage, and this force combines with the force of the leaf spring to overcome the permanent magnetic force of the permanent magnet, releasing the armature. However, leaf springs can exhibit reduced rebound force due to stress relaxation in high-temperature environments, and the aging of coils can lead to the decreased electromagnetic force, resulting in insufficient combined force to overcome the permanent magnet force and slower armature release time, causing the brake to hold the brake. Temperature stress is identified as the primary operating environment for PMB. Obtaining sufficient lifetime data for PMB in a short time frame can be difficult because of its high reliability and long service life. However, ADT using temperature as the stress factor can simulate the aging process and provide sufficient brake reliability data.
This study conducts a constant stress ADT on the PMB using temperature as the acceleration factor in order to investigate the degradation of braking torque and armature release time. The experiment utilizes a DOS60 electric oven, as depicted in Figure 3, to apply the temperature stress to the PMB. Figure 4(a) depicts the braking torque test rig, which is utilized to measure the PMB's braking torque. It comprises a control cabinet, a Permanent Magnet Synchronous Motor, a speed and torque tester, a digital display module, and a test computer. Figure 4(b) displays the integrated test bench of a high-precision solenoid for measuring the PMB's armature release time. The temperature levels for the constant ADT were determined to be 105,120 and 140∘C. The dataset consists of 9 samples divided into 3 groups. Each group of 3 samples is tested at different temperature levels, with measurements of braking torque and armature release time taken once every 24 hours. The test lasts for 30 days (720 h). The experiment process primarily consists of the following steps. Firstly, the initial values of braking torque and armature release time of each sample are tested and recorded before the experiment. Secondly, the sample without electrical power is placed in the DOS60 electric oven, and the ambient temperature and applied stress time are set. Finally, for samples that reach the specified test time without failure, measure the braking torque and armature release time when cooled to room temperature. After all performance tests have been completed, return to the electric oven for the specified test time. After all performance indicators have been tested, they are put back into the electric oven for the specified time. This process is repeated until the end of the test period. The brake torque failure threshold is set to ω1=90, and the armature release time failure threshold is set to ω2=30. Figure 5 presents the acceleration decay data for the performance indicators at each stress level, which are used for model building and parameter estimation. Based on the ADT data, the main concern of the manufacturer is whether the reliability of the product at time 365 days has reached 0.99 at the normal used condition 80∘C.
The Wiener process is a commonly used stochastic process model, particularly for modelling the degradation process with non-monotone Gaussian increments. If a continuous-time stochastic process follows the Wiener process, then the process Xs(t) satisfies the following properties [29]:
a) Xs(0)=0 with probability 1.
b) For any moment t and t+Δt, the increment ΔXs(t)=Xs(t+Δt)−Xs(t) is normal distributed.
c) For any different time intervals [t1,t2],[t3,t4],t1<t2≤t3<t4, the increments Xs(t4)−Xs(t3) and Xs(t2)−Xs(t1) are independent of each other.
If a product has two performance indicators that exhibit the properties mentioned above, then degradation of each performance indicator can be described by Wiener process with a time scale transformation. For modeling degradation paths of two performance indicators, a bivariate Wiener process [30] can be constructed as follows:
(x1(t)x2(t))=β(μ1l1(t,γ1)μ2l2(t,γ2))+(σ100σ2)(B1(l1(t,γ1)B2(l1(t,γ2)) | (3.1) |
where μ and σ represent the degradation rate and volatility of the sth performance indicator, respectively. B(⋅) represents the standard Brownian motion. l(t,γ) is a non-decreasing time function with l(0,γ)=0. The parameter β describes random effects and unobservable common factors affecting the two performance indicators, which is assume to follow normal distribution N(1,δ2).
The lifetime of the sth performance indicator can be defined as
Ts=inf{t:Xs(t)≥ωs,t>0},s=1,2, | (3.2) |
where ωs is the failure threshold of the sth performance indicators. The product life Ts first reaches obeys the transformed inverse Gaussian distribution. Given β, the probability density function (pdf) of Ts is:
fs(ts∣β)=ωs√2πσ2s(ls(ts,γs))3×exp{−(ωs−βμsls(ts,γs))22σ2sls(ts,γs)}dls(ts,γs)dts | (3.3) |
The cumulative distribution function (cdf) of Ts [31] is
FTs(ts∣β)=Φ(βμsls(ts,γs)−ωsσs√ls(ts,γs))+exp{2βμsωsσ2s}Φ(−ωs−βμsls(ts,γs)σs√ls(ts,γs)), | (3.4) |
where Φ(⋅) is the standard normal distribution function. The joint cdf of T1 and T2 can be rewritten as
F(t1,t2)=∫FT1(t1∣β)FT2(t2∣β)f(β)dβ. | (3.5) |
The analytic expression of F(t1,t2) can be found in the Appendix.
The lifetime of the system can be defined as the first time of either performance indicator exceeds the failure threshold ωs(ωs>0). Thus, the lifetime of the system T=min{T1,T2}. The reliability function of the system can be expressed as [30]
R(t)=P(T>t)=P(T1>t,T2>t)=P(T1<t,T2<t)+1−P(T1<t)−P(T2<t)=F(t,t)+1−FT1(t)−FT2(t) | (3.6) |
where the analytical form of FTs(t) is
FTs(t)=∫FTs(t∣β)f(β)dβ=Φ(μsls(t,γs)−ωs√μ2sδ2(ls(t,γs))2+σ2sls(t,γs)))+exp{2μsωsσ2s+2μ2sδ2ω2sσ4s}×Φ(−2μ2sδ2ωsls(t,γs)+σ2s(μsls(t,γs)+ωs)σ2s√μ2sδ2(ls(t,γs))2+σ2sls(t,γs)) |
The acceleration model reflects the impact of accelerated stress on the product degradation process, specifically establishes the relationship between stress level and accelerated degradation rate [32,33]. This paper incorporates an acceleration model into the bivariate Wiener process (3.1) to describe the relationship between temperature stress and degradation rate μs. In the model (3.1), μs can be expressed as a function of the acceleration stress
μps=exp(as+bskp),s=1,2,p=1,…,h, | (3.7) |
where as and bs are unknown parameters, and need to be estimated from ADT data. kp is the normalized accelerated stress, and is defined as
kp=φ(sp)−φ(s0)φ(sh)−φ(s0),p=1,…,h, |
where s0 is the minimum stress level, sh is the maximum stress level. φ(⋅) represents a given function of stress level. In this paper, we use temperature as the accelerating stress, thus the Arrhenius relation φ(sp)=1/(sp+273.13) is adopted here. Based on the models (3.1) and (3.7), accelerated bivariate Wiener degradation model can be formulated as
Xps(t)=βpμpsls(t,γs)+σsBs(ls(t,γs)),μps=exp(as+bskp),s=1,2,p=1,…,h, | (3.8) |
where βp∼N(1,δ2).
Assume that a system has two performance indicators. The stress levels in the ADT are set as s1≤⋯≤sh, and there are n systems tested at each stress level. Let xpisj be the degradation value of the sth performance indicators of the ith system at the measurement time tpj under the stress level sp, where s=1,2, p=1,2,…,h, i=1,2,…,n, j=1,2,…, mp. Let zpisj=xpisj−xpis(j−1), where xpis0=0, and τpisi(γs)=lps(tpisj,γs)−lps(tpis(j−1),γs). To simplify the notation, we use τpisj to denote τpisj(γs). The relationship between stress level and degradation rate is expressed by the Arrhenius model: μps=exp(as+bskp). Therefore, from the model (3.8), we know that conditioned on βpi, zpisj follows normal distribution, that is, zpisj∣βpi∼N(βpiexp(as+bskp)τpisj,σ2sτpisj), and βpi∼N(1,δ2). Let z={zpisj,s=1,2,i=1,…,n, j=1,2…,mp,p=1,…,h} be the observed data and Θ={δ21,σ21,σ22,γ1,γ2,a1,a2,b1,b2} be the model parameter vector. Given z, the likelihood function of Θ can be written as
L(z∣Θ)=h∏p=1n∏i=1∫2∏s=1mpis∏j=11√2πσ2sτpisj×exp{−(zpisj−βpiexp(as+bskp)τpisj)22σ2sτpisj}×(2πδ2)−12exp{−(βpi−1)22δ2}dβpi. | (3.9) |
The likelihood function (3.9) contains computation of integration, and the analytic form of the likelihood function is complex, which makes it difficult to obtain parameter estimates by traditional methods. Compared with classical maximum likelihood method, Bayesian approaches have significant advantages. The Bayesian methods can easily obtain point and interval estimates of the model parameters or functions of parameters through MCMC-Gibbs sampling algorithm, and the implementation of Bayesian approach can be carried out by the software OpenBUGS or RStan easily.
Bayesian statistics is different from classical statistical theory. It treats all unknown parameters as random variables and thus uses probability density functions to quantify them [34]. These densities are often elicited from historical information or experts' experiences, which are called prior distribution. The setting of the prior distribution is essential in Bayesian inference because it affects the inference of the posterior distribution. Prior distributions are further divided into conjugate prior distributions and uninformative prior distributions. A conjugate prior distribution means that the prior and posterior distributions belong to the same distribution family. An uninformative prior is one with little or no information about the parameters. The prior distributions of the parameters in this paper are set as follows:
σ2s∼IG(gs,ls),δ2∼IG(z,r),as∼N(0,104),bs∼N(0,104), | (3.10) |
where σ2s∼IG(gs,ps) means that σ2s obeys the inverse gamma distribution and the hyperparameters gs and ps are set to 0.001. Similarly, the prior information of δ2 is also specified as an inverse gamma distribution, and z=r=0.001. Small values of gs, ps, z and r leads to large variances of these prior distributions, which indicates absence of prior information on σ2s and δ2. Similarly, due to the lack of information on the parameters as, and bs, their priors are designated as diffuse prior distributions that have large variance. The flowchart of the method proposed herein is illustrated in Figure 6.
In this section, we use the proposed model to analyse the ADT data of the PMB as shown in Figure 5. As can be seen in Figure 5, the degradation paths are linear, and thus we assumed that l1(t,γ1)=l2(t,γ2)=t. In order to verify whether the accelerator degradation data can be fitted by the proposed bivariate Wiener process, hypothesis testing is performed to check the normality of the degradation increments at each stress level. In this paper, the Doornik-Hansen method [35] is used to conduct multivariate normality test on the data. The results are listed in Table 1, where "E" denotes the value of test statistic, "DF" is the degree of freedom, and "MVN" represents multivariate normal distribution. From Table 1, we can see that all the p-values are significantly greater than 0.05, which indicates that the degradation increments follow bivariate normal distribution, or the proposed bivariate Wiener process is suitable for the ADT data of the PMB. As an illustration, the Q-Q plots of the multivariate normal distribution for the degradation increments are shown in Figure 7. In Figure 7, we can find that the majority of data points are in alignment with the line, which also supports the normality hypothesis.
Test Project | E | DF | p-value | MVN |
The degradation increments at 105∘C | 1.766 | 4 | 0.778 | YES |
The degradation increments at 120∘C | 1.428 | 4 | 0.839 | YES |
The degradation increments at 140∘C | 2.907 | 4 | 0.573 | YES |
After the hypothesis testing, we use the model (3.8) to fit the ADT data. The parameter estimation is done by the MCMC algorithm, which is implemented by OpenBUGS software. To assess the convergence of MCMC algorithm, we generate three Markov chains under three different initial values of the parameters, and simulate 80,000 posterior samples of the model parameter vector Θ for each chain. Then the Gelman-Rubin's diagnostic method is utilized to judge whether the MCMC algorithm has converged [36]. The results of the Gelman-Rubin's convergence diagnostic for each parameter are shown in Figure 8, where the first half posterior series are set as the burn-in samples. As can be seen in Figure 8, after 10,000 iterations, the intersample (in green) and intrasample (in blue) variabilities begin to stabilize, and their ratios (in red) tend to be 1, which indicates that the convergence of the MCMC algorithm has been achieved.
For performing posterior analysis, autocorrelation of the generated series of each parameter also needs to be checked. Figure 9 shows the autocorrelation of these series, and we can find that when the lag is 20, the autocorrelation of the posterior samples of Θ can be ignored. Thus, the thinning interval is set as 20. Then we keep 240000/20 = 12000 posterior samples for further posterior inference. Table 2 lists the posterior means, standard deviations (SD), medians, and 95 credible intervals of the parameters. Figure 10 shows the posterior densities of Θ, in which we can see that the posteriors of a1, a2, b1, b2, σ21, and σ22 are almost symmetric, and thus their posterior medians are close to their posterior means. While the posterior of δ2 is significantly skewed, we take the median as the point estimate. The main aim for ADT is to extrapolate the reliability of the product at the normal used condition. Once the Bayesian estimates of a1, a2, b1 and b2 are obtained, we can get the estimates of μ01 and μ02 (degradation rate at the normal used stress 80∘C) by the Arrhenius model (3.7). Based on the Bayesian estimates, we draw the reliability function of the product and two performance indicators under four temperature stresses according to (3.6). As shown in Figure 11, the four pairs of curves from left to right represent the reliability functions of the product under the stress levels 80,105,120, and 140∘C, respectively. Specially, under the normal used condition 80∘C, the reliability of PMB at time 365 days is 0.9965, which is beyond the manufacturer's expectation 0.99.
Parameters | Mean | SD | MC error | 2.50 | Median | 97.5 |
δ2 | 0.007 | 0.014 | 8.566×10−5 | 4.635×10−4 | 0.003 | 0.039 |
a1 | −2.275 | 0.352 | 0.002 | −3.026 | −2.255 | −1.641 |
a2 | −3.452 | 0.417 | 0.002 | -4.355 | −3.424 | −2.715 |
b1 | 1.892 | 0.397 | 0.002 | 1.160 | 1.874 | 2.718 |
b2 | 2.536 | 0.454 | 0.002 | 1.719 | 2.511 | 3.502 |
σ21 | 0.267 | 0.023 | 4.971×10−5 | 0.224 | 0.265 | 0.317 |
σ22 | 0.074 | 0.006 | 1.379×10−5 | 0.063 | 0.074 | 0.089 |
Finally, a sensitivity analysis is carried out to assess the effects of priors. As an illustration, we change the prior of a1, and keep the priors of other parameters unchanged. In addition to the normal prior N(0,104), two other priors N(0,10) and N(0,100) for a1 are also considered. Figure 12 shows the posterior densities of a1 based on different priors, in which we can see that the posterior densities are robust based on the different priors, and the posterior modes are almost the same. Figure 13 also shows the reliability functions of PMB at 80∘C based on the different priors. As can be seen in Figure 13, the effects of the priors on the results can be ignorable, which shows the robustness of the proposed method.
In this paper, we have proposed an accelerated bivariate Wiener degradation model to evaluate the reliability of PMB. Firstly, a comprehensive analysis of the structure and functioning of PMB is conducted, and the degradation of braking torque and armature release time are identified as the performance indicators of PMB. Then we design an ADT to collect the degradation data of the two performance indicators. Based on the ADT data, the influences of temperature stress on reliability are reflected by degradation rate, and thus a bivariate Wiener degradation process combined with Arrhenius model is proposed. The MCMC-Gibbs sampling algorithm is utilized to estimate the model parameters. Then the reliability of PMB at the normal used condition can also be obtained. Finally, the proposed model is applied to analyse the ADT of PMB, and we find that the reliability of PMB at time one year is 1, which has reached the manufacturer's requirement. The research data in this paper can be used as theoretical support for the future PMB reliability modeling analysis. The model proposed in this study also provides a new idea for the reliability modeling of PMB.
This work was supported by the National Natural Science Foundation of China (Nos. 72271167, 72071149), and the Natural Science Foundation of Zhejiang Province (No. LZ23A010003).
The authors declare there is no conflict of interest.
According to [26],
F(t1,t2)=Q1+Q2+Q3+Q4, |
where
Q1=∫Φ(βμ1l1(t1,γ1)−ω1σ1√l1(t1,γ1))Φ(βμ2l2(t2,γ2)−ω2σ2√l2(t2,γ2))f(β)dfβ=bvn(−ω1+μ1l1(t1,γ1)P1,−ω2+μ2l2(t2,γ2)P2,D5P1P2), |
Q2=∫Φ(βμ1l1(t1,γ1)−ω1σ1√l1(t1,γ1))exp{2βμ2ω2σ22}Φ(βμ2l2(t2,γ2)−ω2σ2√l2(t2,γ2))f(β)dβ=exp{2μ2ω2σ22+2μ22ω22δ2σ42}bvn(−ω1+μ1l1(t1,γ1)+D1P1−ω2−μ2l2(t2,γ2)−D4P2,−D5P1P2), |
Q3=∫exp{2βμ1ω1σ21}Φ(βμ1l1(t1,γ1)−ω1σ1√l1(t1,γ1))×Φ(βμ2l2(t2,γ2)−ω2σ2√l2(t2,γ2))f(β)dβ=exp{2μ1ω1σ21+2μ21ω21δ2σ41}bvn(−ω1−μ1l1(t1,γ1)−D3P1, −ω2+μ2l2(t2,γ2)+D2P2,−D5P1P2), |
Q4=∫exp{2βμ1ω1σ21}Φ(βμ1l1(t1,γ1)−ω1σ1√l1(t1,γ1))×Φ(βμ2l2(t2,γ2)−ω2σ2√l2(t2,γ2))f(β)dβ=exp{2μ1ω1σ21+2μ2ω2σ22+2δ2(μ1ω1σ21+μ2ω2σ22)2}×bvn(−ω1−μ1l1(t1,γ1)−D1−D3P1, −ω2−μ2l2(t2,γ2)−D2−D4P2,D5P1P2), |
where
P1=√σ21l1(t1,γ1)+μ21δ2l21(t1,γ1),P2=√σ22l2(t2,γ2)+μ22δ2l22(t2,γ2),D1=2μ1l1(t1,γ1)μ2ω2δ2/σ22,D2=2μ1ω1μ2l2(t2,γ2)δ2/σ21,D3=2μ21l1(t1,γ1)ω1δ2/σ21,D4=2μ22l2(t2,γ2)ω2δ2/σ22,D5=μ1l1(t1,γ1)μ2l2(t2,γ2)δ2, |
and
bvn(x1,x2,θ)=12π√1−θ2∫x1−∞∫x2−∞exp{−x2−2θxy+y22(1−θ2)}dxdy. |
[1] | Q. Yue, H. Qian, High torque density permanent magnet brake, in 2021 4th International Conference on Mechanical, Electrical and Material Application, 2125 (2021), 012068. https://doi.org/10.1088/1742-6596/2125/1/012068 |
[2] |
L. Zhuang, A. Xu, X. Wang, A prognostic driven predictive maintenance framework based on Bayesian deep learning, Reliab. Eng. Syst. Saf., 234 (2023), 109181. https://doi.org/10.1016/j.ress.2023.109181 doi: 10.1016/j.ress.2023.109181
![]() |
[3] |
C. Luo, L. Shen, A. Xu, Modelling and estimation of system reliability under dynamic operating environments and lifetime ordering constraints, Reliab. Eng. Syst. Saf., 218 (2022), 108136. https://doi.org/10.1016/j.ress.2021.108136 doi: 10.1016/j.ress.2021.108136
![]() |
[4] |
P. Jiang, B. Wang, X. Wang, Z. Zhou, Inverse Gaussian process based reliability analysis for constant-stress accelerated degradation data, Appl. Math. Modell., 105 (2022), 137–148. https://doi.org/10.1016/j.apm.2021.12.003 doi: 10.1016/j.apm.2021.12.003
![]() |
[5] |
S. Li, Z. Chen, Q. Liu, W. Shi, K. Li, Modeling and analysis of performance degradation data for reliability assessment: A review, IEEE Access, 8 (2020), 74648–74678. https://doi.org/10.1109/ACCESS.2020.2987332 doi: 10.1109/ACCESS.2020.2987332
![]() |
[6] |
S. Limon, O. P. Yadav, H. Liao, A literature review on planning and analysis of accelerated testing for reliability assessment, Qual. Reliab. Eng. Int., 33 (2017), 2361–2383. https://doi.org/10.1002/qre.2195 doi: 10.1002/qre.2195
![]() |
[7] |
X. Yuan, E. Higo, M. D. Pandey, Estimation of the value of an inspection and maintenance program: A Bayesian gamma process model, Reliab. Eng. Syst. Saf., 216 (2021), 107912. https://doi.org/10.1016/j.ress.2021.107912 doi: 10.1016/j.ress.2021.107912
![]() |
[8] |
F, Zhang, J. Li, H. K. T. Ng, Minimum f-divergence estimation with applications to degradation data analysis, IEEE Trans. Inf. Theory, 68 (2022), 6774–6789. https://doi.org/10.1109/TIT.2022.3169885 doi: 10.1109/TIT.2022.3169885
![]() |
[9] |
W. Yu, Y. Shao, J. Xu, C. Mechefske, An adaptive and generalized Wiener process model with a recursive filtering algorithm for remaining useful life estimation, Reliab. Eng. Syst. Saf., 217 (2022), 108099. https://doi.org/10.1016/j.ress.2021.108099 doi: 10.1016/j.ress.2021.108099
![]() |
[10] |
H. Wang, H. Liao, X. Ma, R. Bao, Remaining useful life prediction and optimal maintenance time determination for a single unit using isotonic regression and gamma process model, Reliab. Eng. Syst. Saf., 210 (2021), 107504. https://doi.org/10.1016/j.ress.2021.107504 doi: 10.1016/j.ress.2021.107504
![]() |
[11] |
Z. Ye, N. Chen, The Inverse Gaussian process as a degradation model, Technometrics, 56 (2014), 302–311. https://doi.org/10.1080/00401706.2013.830074 doi: 10.1080/00401706.2013.830074
![]() |
[12] |
Z. Zhang, X. Si, C. Hu, Y. Lei, Degradation data analysis and remaining useful life estimation: A review on Wiener-process-based methods, Eur. J. Oper. Res., 271 (2018), 775–796. https://doi.org/10.1016/j.ejor.2018.02.033 doi: 10.1016/j.ejor.2018.02.033
![]() |
[13] |
G. Liao, H. Yin, M. Chen, Z. Lin, Remaining useful life prediction for multi-phase deteriorating process based on Wiener process, Reliab. Eng. Syst. Saf., 207 (2021), 107361. https://doi.org/10.1016/j.ress.2020.107361 doi: 10.1016/j.ress.2020.107361
![]() |
[14] |
Y. Hou, Y. Du, Y. Peng, D. Liu, An improved random effects Wiener process accelerated degradation test model for lithium-ion battery, IEEE Trans. Instrum. Meas., 70 (2021), 1–11. https://doi.org/10.1109/TIM.2021.3091457 doi: 10.1109/TIM.2021.3091457
![]() |
[15] |
Q. Zhai, P. Chen, L. Hong, L. Shen, A random-effects Wiener degradation model based on accelerated failure time, Reliab. Eng. Syst. Saf., 180 (2018), 94–103. https://doi.org/10.1016/j.ress.2018.07.003 doi: 10.1016/j.ress.2018.07.003
![]() |
[16] |
B. Yan, X. Ma, L. Yang, H. Wang, T. Wu, A novel degradation-rate-volatility related effect Wiener process model with its extension to accelerated ageing data analysis, Reliab. Eng. Syst. Saf., 204 (2020), 107138. https://doi.org/10.1016/j.ress.2020.107138 doi: 10.1016/j.ress.2020.107138
![]() |
[17] |
X. Ye, Y. Hu, B. Zheng, C. Chen, G. Zhai, A new class of multi-stress acceleration models with interaction effects and its extension to accelerated degradation modelling, Reliab. Eng. Syst. Saf., 228 (2022), 108815. https://doi.org/10.1016/j.ress.2022.108815 doi: 10.1016/j.ress.2022.108815
![]() |
[18] |
P. Jiang, B. Wang, X. Wang, S. Qin, Optimal plan for Wiener constant-stress accelerated degradation model, Appl. Math. Modell., 84 (2020), 191–201. https://doi.org/10.1016/j.apm.2020.03.036 doi: 10.1016/j.apm.2020.03.036
![]() |
[19] |
P. Jiang, X. Yang, Reliability inference and remaining useful life prediction for the doubly accelerated degradation model based on Wiener process, AIMS Math., 8 (2023), 7560–7583. https://doi.org/10.3934/math.2023379 doi: 10.3934/math.2023379
![]() |
[20] |
J. Ma, L. Cai, G. Liao, H. Yin, X. Si, P. Zhang, A multi-phase Wiener process-based degradation model with imperfect maintenance activities, Reliab. Eng. Syst. Saf., 232 (2023), 109075. https://doi.org/10.1016/j.ress.2022.109075 doi: 10.1016/j.ress.2022.109075
![]() |
[21] |
F. Wang, H. Li, On the use of the maximum entropy method for reliability evaluation involving stochastic process modeling, Struct. Saf., 88 (2021), 102028. https://doi.org/10.1016/j.strusafe.2020.102028 doi: 10.1016/j.strusafe.2020.102028
![]() |
[22] |
R. Chen, C. Zhang, S. Wang, Y. Qian, Reliability estimation of mechanical seals based on bivariate dependence analysis and considering model uncertainty, Chin. J. Aeronaut., 34 (2021), 554–572. https://doi.org/10.1016/j.cja.2020.12.001 doi: 10.1016/j.cja.2020.12.001
![]() |
[23] |
W. Peng, Y. Li, Y. Yang, S. Zhu, H. Huang, Bivariate analysis of incomplete degradation observations based on inverse gaussian processes and copulas, IEEE Trans. Reliab., 65 (2016), 624–639. https://doi.org/10.1109/tr.2015.2513038 doi: 10.1109/tr.2015.2513038
![]() |
[24] |
G. Fang, R. Pan, Y. Hong, Copula-based reliability analysis of degrading systems with dependent failures, Reliab. Eng. Syst. Saf., 193 (2020), 106618. https://doi.org/10.1016/j.ress.2019.106618 doi: 10.1016/j.ress.2019.106618
![]() |
[25] |
K. Song, L. Cui, A common random effect induced bivariate gamma degradation process with application to remaining useful life prediction, Reliab. Eng. Syst. Saf., 219 (2022), 108200. https://doi.org/10.1016/j.ress.2021.108200 doi: 10.1016/j.ress.2021.108200
![]() |
[26] |
G. Fang, R. Pan, Y. Wang, Inverse Gaussian processes with correlated random effects for multivariate degradation modeling, Eur. J. Oper. Res., 300 (2022), 1177–1193. https://doi.org/10.1016/j.ejor.2021.10.049 doi: 10.1016/j.ejor.2021.10.049
![]() |
[27] |
Q. Zhai, Z. Ye, A multivariate stochastic degradation model for dependent performance characteristics, Technometrics, (2023), 1–13. https://doi.org/10.1080/00401706.2022.2157881 doi: 10.1080/00401706.2022.2157881
![]() |
[28] |
B. Yan, H. Wang, X. Ma, Correlation‐driven multivariate degradation modeling and RUL prediction based on Wiener process model, Qual. Reliab. Eng. Int., (2022). https://doi.org/10.1002/qre.3105 doi: 10.1002/qre.3105
![]() |
[29] |
S. Zhou, Y. Tang, A. Xu, A generalized Wiener process with dependent degradation rate and volatility and time-varying mean-to-variance ratio, Reliab. Eng. Syst. Saf., 216 (2021), 107895. https://doi.org/10.1016/j.ress.2021.107895 doi: 10.1016/j.ress.2021.107895
![]() |
[30] |
A. Xu, L. Shen, B. Wang, Y. Tang, On modeling bivariate Wiener degradation process, IEEE Trans. Reliab., 67 (2018), 897–906. https://doi.org/10.1109/TR.2018.2791616 doi: 10.1109/TR.2018.2791616
![]() |
[31] |
Z. Ye, Y. Wang, K. Tsui, M. Pecht, Degradation data analysis using Wiener processes with measurement errors, IEEE Trans. Reliab., 62 (2013), 772–780. https://doi.org/10.1109/TR.2013.2284733 doi: 10.1109/TR.2013.2284733
![]() |
[32] |
W. Yan, X. Xu, D. Bigaud, W. Cao, Optimal design of step-stress accelerated degradation tests based on the Tweedie exponential dispersion process, Reliab. Eng. Syst. Saf., 230 (2023), 108917. https://doi.org/10.1016/j.ress.2022.108917 doi: 10.1016/j.ress.2022.108917
![]() |
[33] |
X. Zhao, B. Liu, Y. Liu, Reliability modeling and analysis of load-sharing systems with continuously degrading components, IEEE Trans. Reliab., 67 (2018), 1096–1110. https://doi.org/10.1109/TR.2018.2846649 doi: 10.1109/TR.2018.2846649
![]() |
[34] |
W. Peng, S. Zhu, L. Shen, The transformed inverse gaussian process as an age-and state-dependent degradation model, Appl. Math. Modell., 75 (2019), 837–852. https://doi.org/10.1016/j.apm.2019.07.004 doi: 10.1016/j.apm.2019.07.004
![]() |
[35] |
D. W. Joenssen, J. Vogel, A power study of goodness-of-fit tests for multivariate normality implemented in R, J. Stat. Comput. Simul., 84 (2014), 1055–1078. https://doi.org/10.1080/00949655.2012.739620 doi: 10.1080/00949655.2012.739620
![]() |
[36] |
S. Brooks, A. Gelman, General methods for monitoring convergence of iterative simulations, J. Comput. Graph. Stat., 7 (1998), 434–455. https://doi.org/10.1080/10618600.1998.10474787 doi: 10.1080/10618600.1998.10474787
![]() |
1. | Peihua Jiang, Longmei Shi, Statistical inference for a competing failure model based on the Wiener process and Weibull distribution, 2024, 21, 1551-0018, 3146, 10.3934/mbe.2024140 | |
2. | Haijie Mao, Zhi Liu, Xiaorui Zhang, 2023, Remaining Life Prediction Research of Stage Boom Synchronization System Considering Time-Varying Operation Conditions Under Composite Degradation Quantity, 979-8-3503-0375-9, 2364, 10.1109/CAC59555.2023.10451114 |
Test Project | E | DF | p-value | MVN |
The degradation increments at 105∘C | 1.766 | 4 | 0.778 | YES |
The degradation increments at 120∘C | 1.428 | 4 | 0.839 | YES |
The degradation increments at 140∘C | 2.907 | 4 | 0.573 | YES |
Parameters | Mean | SD | MC error | 2.50 | Median | 97.5 |
δ2 | 0.007 | 0.014 | 8.566×10−5 | 4.635×10−4 | 0.003 | 0.039 |
a1 | −2.275 | 0.352 | 0.002 | −3.026 | −2.255 | −1.641 |
a2 | −3.452 | 0.417 | 0.002 | -4.355 | −3.424 | −2.715 |
b1 | 1.892 | 0.397 | 0.002 | 1.160 | 1.874 | 2.718 |
b2 | 2.536 | 0.454 | 0.002 | 1.719 | 2.511 | 3.502 |
σ21 | 0.267 | 0.023 | 4.971×10−5 | 0.224 | 0.265 | 0.317 |
σ22 | 0.074 | 0.006 | 1.379×10−5 | 0.063 | 0.074 | 0.089 |
Test Project | E | DF | p-value | MVN |
The degradation increments at 105∘C | 1.766 | 4 | 0.778 | YES |
The degradation increments at 120∘C | 1.428 | 4 | 0.839 | YES |
The degradation increments at 140∘C | 2.907 | 4 | 0.573 | YES |
Parameters | Mean | SD | MC error | 2.50 | Median | 97.5 |
δ2 | 0.007 | 0.014 | 8.566×10−5 | 4.635×10−4 | 0.003 | 0.039 |
a1 | −2.275 | 0.352 | 0.002 | −3.026 | −2.255 | −1.641 |
a2 | −3.452 | 0.417 | 0.002 | -4.355 | −3.424 | −2.715 |
b1 | 1.892 | 0.397 | 0.002 | 1.160 | 1.874 | 2.718 |
b2 | 2.536 | 0.454 | 0.002 | 1.719 | 2.511 | 3.502 |
σ21 | 0.267 | 0.023 | 4.971×10−5 | 0.224 | 0.265 | 0.317 |
σ22 | 0.074 | 0.006 | 1.379×10−5 | 0.063 | 0.074 | 0.089 |