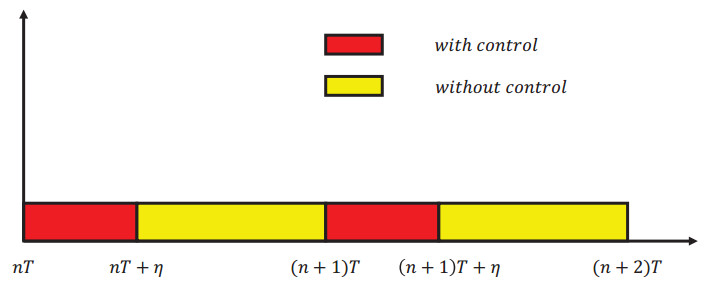
In this paper, the practical discontinuous control algorithm is used in the tracking controller design for a permanent magnet synchronous motor (PMSM). Although the theory of discontinuous control has been studied intensely, it is seldom applied to the actual systems, which encourages us to spread the discontinuous control algorithm to motor control. Due to the constraints of physical conditions, the input of the system is limited. Hence, we design the practical discontinuous control algorithm for PMSM with input saturation. To achieve the tracking control of PMSM, we define the error variables of the tracking control, and the sliding mode control method is introduced to complete the design of the discontinuous controller. Based on the Lyapunov stability theory, the error variables are guaranteed to converge to zero asymptotically, and the tracking control of the system is realized. Finally, the validity of the proposed control method is verified by a simulation example and the experimental platform.
Citation: Bin Liu, Dengxiu Yu, Xing Zeng, Dianbiao Dong, Xinyi He, Xiaodi Li. Practical discontinuous tracking control for a permanent magnet synchronous motor[J]. Mathematical Biosciences and Engineering, 2023, 20(2): 3793-3810. doi: 10.3934/mbe.2023178
[1] | Ying Shi, Keqi Mei . Adaptive nonsingular terminal sliding mode controller for PMSM drive system using modified extended state observer. Mathematical Biosciences and Engineering, 2023, 20(10): 18774-18791. doi: 10.3934/mbe.2023832 |
[2] | Jian Meng, Bin Zhang, Tengda Wei, Xinyi He, Xiaodi Li . Robust finite-time stability of nonlinear systems involving hybrid impulses with application to sliding-mode control. Mathematical Biosciences and Engineering, 2023, 20(2): 4198-4218. doi: 10.3934/mbe.2023196 |
[3] | Kangsen Huang, Zimin Wang . Research on robust fuzzy logic sliding mode control of Two-DOF intelligent underwater manipulators. Mathematical Biosciences and Engineering, 2023, 20(9): 16279-16303. doi: 10.3934/mbe.2023727 |
[4] | Yun Liu, Yuhong Huo . Predefined-time sliding mode control of chaotic systems based on disturbance observer. Mathematical Biosciences and Engineering, 2024, 21(4): 5032-5046. doi: 10.3934/mbe.2024222 |
[5] | Rui Ma, Jinjin Han, Li Ding . Finite-time trajectory tracking control of quadrotor UAV via adaptive RBF neural network with lumped uncertainties. Mathematical Biosciences and Engineering, 2023, 20(2): 1841-1855. doi: 10.3934/mbe.2023084 |
[6] | Chengxuan Wang, Jiawei Tang, Baoping Jiang, Zhengtian Wu . Sliding-mode variable structure control for complex automatic systems: a survey. Mathematical Biosciences and Engineering, 2022, 19(3): 2616-2640. doi: 10.3934/mbe.2022120 |
[7] | Xinyu Shao, Zhen Liu, Baoping Jiang . Sliding-mode controller synthesis of robotic manipulator based on a new modified reaching law. Mathematical Biosciences and Engineering, 2022, 19(6): 6362-6378. doi: 10.3934/mbe.2022298 |
[8] | Ping Li, Zhe Lin, Hong Shen, Zhaoqi Zhang, Xiaohua Mei . Optimized neural network based sliding mode control for quadrotors with disturbances. Mathematical Biosciences and Engineering, 2021, 18(2): 1774-1793. doi: 10.3934/mbe.2021092 |
[9] | Xinggui Zhao, Bo Meng, Zhen Wang . Event-triggered integral sliding mode control for uncertain networked linear control systems with quantization. Mathematical Biosciences and Engineering, 2023, 20(9): 16705-16724. doi: 10.3934/mbe.2023744 |
[10] | Xiaoqiang Dai, Hewei Xu, Hongchao Ma, Jianjun Ding, Qiang Lai . Dual closed loop AUV trajectory tracking control based on finite time and state observer. Mathematical Biosciences and Engineering, 2022, 19(11): 11086-11113. doi: 10.3934/mbe.2022517 |
In this paper, the practical discontinuous control algorithm is used in the tracking controller design for a permanent magnet synchronous motor (PMSM). Although the theory of discontinuous control has been studied intensely, it is seldom applied to the actual systems, which encourages us to spread the discontinuous control algorithm to motor control. Due to the constraints of physical conditions, the input of the system is limited. Hence, we design the practical discontinuous control algorithm for PMSM with input saturation. To achieve the tracking control of PMSM, we define the error variables of the tracking control, and the sliding mode control method is introduced to complete the design of the discontinuous controller. Based on the Lyapunov stability theory, the error variables are guaranteed to converge to zero asymptotically, and the tracking control of the system is realized. Finally, the validity of the proposed control method is verified by a simulation example and the experimental platform.
In recent years, the control problem of time-varying systems has been widely considered [1,2,3,4], and most control protocols of time-varying systems are based on continuous control methods [5,6,7,8,9], which require continuous control input. However, discontinuous control [10,11] is more effective in some specific cases, and it has attracted more and more attention from researchers. Although the theoretical research on discontinuous control strategy is abundant, few researchers have applied it to practical systems, which motivates us to use this method in actual scenarios. In addition, the input of the actual system is limited, and the control input based on the traditional approach is likely to exceed the limit and affect the control performance of the controller. Thus, input saturation can-not be ignored in the process of controller design. According to the above analysis, we study the practical discontinuous control of PMSM and complete the design of an intermittent controller by the sliding mode control method.
Discontinuous control strategy includes impulsive control [12,13], intermittent control, and sampling control. According to the division of the control cycle, intermittent control is divided into periodic and non-periodic intermittent control. In [14,15], a novel control strategy consisting of an event-triggering mechanism and impulsive control showed its effectiveness in stabilizing nonlinear systems and can effectively reduce the cost of signal transmission. To solve the leader-follower synchronization problem in complex networks [16,17], a batch control scheme based on being dual event-driven was proposed. In [18], the observer-based state feedback non-periodic intermittent control for uncertain systems with structural uncertainty was studied. A class of finite-time synchronous control of reaction-diffusion nervous systems coupled with small regions with interaction diagrams on spatial boundaries was researched in [19]. In [20], the intermittent control strategy for synchronous analysis of time-varying complex dynamic networks was studied, and some numerical simulations verified the validity of the results. Although discontinuous control has received much attention from researchers, most of the above research remains at the stage of theoretical research. To improve the practical ability of the algorithm, we carry out the research of applying the discontinuous control algorithm to motor control.
For a real system, the limit of input saturation can not be ignored. Input saturation [21,22] often seriously affects the performance of the system and even leads to system instability. In [23], the global stabilization problem of discrete-time linear systems with input saturation and delay was studied, and three examples demonstrated the effectiveness of the proposed method. In [24], the researchers studied the consistency of a class of linear multi-agent systems with input saturation under a self-triggering mechanism. The adaptive control strategy of the flexible manipulator with a double pendulum structure was analyzed, and the effectiveness of the saturation controller in tracking control was verified in [25]. The tracking control problem for nonlinear stochastic systems with time-varying state constraints and input saturation was studied in [26]. In [27], adaptive fuzzy tracking control for high-order nonlinear time-delay systems with full state constraints and input saturation was studied. Considering that the input is bounded in the real system, the input saturation auxiliary system is used to compensate for the influence of input saturation on the control performance, which makes our algorithm more valuable in the application.
Currently, many control methods are applied to controller design, such as the backstepping method [28,29], model predictive control and sliding mode control [30,31]. As one of the common control methods, sliding mode control has a wide range of application scenarios [32,33]. In [34], the sliding mode control of uncertain linear systems with impulsive effects was studied, and the stability of the system was analyzed by piecewise discontinuous Lyapunov function. A robust fault-tolerant compensation control method based on the integral sliding mode method was proposed for uncertain linear systems on networks [35]. In [36], the researchers considered unknown asymmetric input saturation and control singularity and designed a fuzzy terminal sliding mode controller based on the disturbance observer. In [37], a new sliding mode switching approximation law was proposed to improve sliding mode control performance for a class of high-order self-organizing systems. The problem of event-triggered fuzzy sliding mode control for networked control systems with semi-Markov process adjustment was studied in [38]. To design a practical discontinuous controller with input saturation, sliding mode control is used in the design of the control algorithm to achieve the desired control objective.
Through the above analysis, we consider the practical discontinuous tracking control problem of PMSM under input saturation and use the sliding mode control method to complete the design of the controller. The main contributions of this paper are described as follows:
1) To improve the applicability of the discontinuous control method, we apply the discontinuous control method to the tracking control of PMSM and carry out the experimental verification. However, most of the previous discontinuous control is at the level of theoretical research and rarely applied to the actual system.
2) Due to physical constraints, we consider the input saturation in the actual scenario. By introducing the input saturation auxiliary system, we reduce the influence of input saturation on the system and calculate the theoretical control input of the system.
3) To realize the discontinuous control of PMSM, sliding mode control is introduced into the design of the discontinuous controller, which ensures the stability and robustness of the system under the input of discontinuous control.
The remaining part of the paper is described as follows. Some essential knowledge and a necessary lemma are introduced in Section 2. We use the sliding mode control method to complete the design of the discontinuous controller and provide the stability analysis in Section 3. We resort to the simulation results to verify the availability of the controller design in Section 4. In Section 5, the experimental platform demonstrates the effectiveness of the practical discontinuous control algorithm. In Section 6, we summarize the whole paper.
This section introduces the definitions of intermittent control, systems model, and input saturation, which will be used in the rest of the work.
Intermittent control is one of the strategies of discontinuous control. The division of the control cycle can be divided into periodic intermittent control and aperiodic intermittent control. In this paper, we adopt the periodic intermittent control strategy. The main design idea is to divide the whole control cycle into equal intervals T of the control cycle. Each control period is divided into the controlled period and the uncontrolled period. The two continuous control periods, n and n+1, are described in Figure 1, and n=0,1,2,⋯.
When t∈[nT,nT+η], at nT moment, the system receives instructions from the controller, and at nT+η moment, the control instruction ends. Thus, [nT,nT+η] is the controlled period of the system. During the period of [nT+η,(n+1)T], the system does not receive any instructions from the controller. Thus, [nT+η,(n+1)T] is the uncontrolled period of the system. In addition, in periodic intermittent control, the control amplitude η=nT+η−nT>0 is a constant value.
Lemma 1. (Young's inequality) For arbitrary positive real numbers x and y, the following inequality holds.
xy≤xaa+ybb | (2.1) |
where a>0 and b>0, with (a−1)(b−1)=1. If and only if xa=yb, the equality sign holds.
Definition 1. Considering the impact of input saturation, the actual control input u(iq) is the nonlinear function of ideal control input iq. The u(iq) is defined as follows
u(iq)=sat(iq)={ iq|iq|≤iMiM⋅sgn(iq)|iq|>iM | (2.2) |
where iM is the boundary of control input saturation.
The dynamic model of a 3-phase PMSM can be described as [39]
{Jdωdt=Te−Tl−fωTe=32piq[id(Ld−Lq)+Φ] | (2.3) |
where J is the moment of inertia, ω is the angular velocity, and Te and Tl are the electromagnetic torque and load torque, respectively. f is the damping coefficient. p represents the number of pairs of the permanent magnet rotor poles. Ld and Lq are the inductance of the d-q axis, and id and iq represent the d-q axis parts of stator current. Φ is the permanent magnet flux linkage.
Normally, the space vector pulse-width modulation control method of id = 0 is used for the 3-phase PMSM with the surface-mounted permanent magnet rotor. Hence, we can the following formula [40].
Ld=Lq=Ls | (2.4) |
where Ls is the inductance of the stator.
According to Definition 1, (2.3) and (2.4), the dynamic model under input saturation can be described as the following:
{˙θ1=ω1˙ω1=32pΦJu(iq)−TlJ−fJω1 | (2.5) |
where θ1 and ω1 are angle and angular velocity, respectively.
Let g=32pΦJ, d=−Tl/J, c=−f/J, and (2.5) can be redescribed as
{˙θ1=ω1˙ω1=gu(iq)+d+cω1y1=θ1 | (2.6) |
where iq and y1 are the ideal control input and output, respectively.
The dynamics of the reference signal are described as
{˙θ2=ω2˙ω2=d+cω2y2=θ2 | (2.7) |
where θ2 and ω2 are angle and angular velocity of the reference signal.
Control objective: In this paper, the control objective is to design the discontinuous controller with the sliding mode control method under the constraint of input saturation, such that the error variables ε1 and ε2 converge to zero asymptotically.
In this section, we design the discontinuous controller through sliding mode control to realize the tracking control to reference signal.
The structure diagram of the practical discontinuous controller is shown in Figure 2. The controller is mainly composed of the discontinuous controller, input saturation auxiliary system, input saturation actuator and PMSM. The dynamic control model of PMSM can be expressed by Eq (2.6), and the constraint effect of the input saturation actuator on the control input signal can be expressed by Eq (2.2). The design of other parts will be given in detail below.
For any n∈Z+∪{0},t∈(nT,(n+1)T), the error variables are described as follows :
{ε1=θ1−θ2−∫t0ξdt+∫nT0ξdt,ε2=ω1−ω2−ξ, | (3.1) |
where ε1 and ε2 are error variables. ξ is the input saturation auxiliary system, which will be designed in subsequent processes.
We define
θ=θ1−θ2, | (3.2) |
ω=ω1−ω2, | (3.3) |
ˉε=ε2+kε1. | (3.4) |
The time derivative of (3.1) is written as
{˙ε1=ω1−ω2−ξ=ε2,˙ε2=˙ω1−˙ω2−˙ξ=˙ω−˙ξ. | (3.5) |
Case 1: For any n∈Z+∪{0},t∈[nT,nT+η), we construct the following Lyapunov function
V=12(ε21+ˉε2) | (3.6) |
The time derivative of (3.6) is written as
˙V=ε1˙ε1+ˉε˙ˉε=ε1ε2+ˉε(˙ε2+k˙ε1). | (3.7) |
Substituting (3.5) into (3.7), we have
˙V=ε1(ˉε−kε1)+ˉε(˙ω−˙ξ+k(ω1−ω2−ξ))=−kε21+ˉε(˙ω−˙ξ+k(ω−ξ)+ε1) | (3.8) |
The input saturation auxiliary system ξ and ideal control input iq are designed as follows:
˙ξ=cξ+gu(iq)−giq, ξ(nT)=0 | (3.9) |
iq=−1g[((2k+c)ε2+(k2+1)ε1)] | (3.10) |
Substituting (3.9) into (3.8), we can obtain
˙V=−kε21+ˉε(˙ω−˙ξ+k(ω−ξ)+ε1)=−kε21+ˉε(˙ω−cξ−gu(iq)+giq+k(ω−ξ)+ε1)=−kε21+ˉε((k+c)⋅(ω−ξ)+giq+ε1)=−kε21+ˉε((k+c)⋅ε2+giq+ε1) | (3.11) |
Substituting (3.10) into (3.11), we have
˙V=−k(ε21+ˉε2)=−2kV. | (3.12) |
Case 2: For t∈[nT+η,(n+1)T) and there holds that
˙V=ε1˙ε1+ˉε˙ε=ε1ε2+ˉε(˙ε2+k˙ε1)=ε1(ˉε−kε1)+ˉε(˙ω−˙ξ+k(ω1−ω2−ξ))=−kε21+ˉε(˙ω−˙ξ+k(ω−ξ)+ε1) | (3.13) |
The input saturation auxiliary system ξ and control input u are designed as follows
˙ξ=cξ+gu(iq)−giq | (3.14) |
iq=0. | (3.15) |
Substituting (3.14) and (3.15) into (3.13), we can obtain
˙V=−kε21+ˉε(˙ω−cξ−gu(iq)+giq+k(ω−ξ)+ε1)=−kε21+ˉε((k+c)⋅(ω−ξ)+ε1)=−kε21+ˉε((k+c)⋅ε2+ε1). | (3.16) |
Substituting (3.4) into (3.16), we can obtain
˙V=−kε21+(k+c)ˉεε2+ˉεε1=−kε21+(k+c)ˉε2−k(k+c)ˉεε1+ˉεε1=[1−k(k+c)]ˉεε1−kε21+(k+c)ˉε2. | (3.17) |
According to Lemma 1, we have
ˉεε1≤(|1−k(k+c)|)|ˉεε1|≤12(|1−(k+c)k|)(ε21+ˉε2). | (3.18) |
Substituting (3.18) into (3.17), we can obtain
˙V≤12(|1−(k+c)k|)(ε21+ˉε2)−kε21+(k+c)ˉε2≤12[(|1−(k+c)k|)+(k+c)](ε21+ˉε2)≤k0V | (3.19) |
where k0=|1−(k+c)k|+(k+c), 2k+c>0.
Case 1: For any n∈Z+∪{0},t∈[nT,nT+η), we have
˙V=−2kV. | (3.20) |
Then, we have
V(nT+η)=e−2kηV(nT). | (3.21) |
Proof : Multiplying both sides by e2kt, (3.20) becomes
ddt(V(t)e2kt)=0. | (3.22) |
Integrating it over [nT,nT+η], we have
V(nT+η)=e−2kηV(nT). | (3.23) |
Case 2: For any n∈Z+∪{0},t∈[nT+η,(n+1)T), we have
˙V≤k0V. | (3.24) |
Similarly, we have
V((n+1)T)≤ek0(T−η)V(nT+η). | (3.25) |
According to (3.23) and (3.25)
V((n+1)T)≤ek0(T−η)V(nT+η)≤ek0(T−η)−2kηV(nT). | (3.26) |
Therefore, when the inequality
ηT>k0k0+2k | (3.27) |
holds the function V(t) is a monotonically decreasing continuous function.
limt→∞V(t)=0 | (3.28) |
According to (3.6), we have
limt→∞V(t)=12(ε21+ˉε2)=0. | (3.29) |
This indicates that
{ε1=θ1−θ2−∫t0ξdt+∫nT0ξdt=0,ε2=ω1−ω2−ξ=0. | (3.30) |
Thus, when t→∞, we have
iq=−1g[((2k+c)ε2+(k2+1)ε1)]=0. | (3.31) |
Then, at the moment 0<t1<∞, t1∈[(n−1)T,nT), iq≤iM, u(iq)=iq, the following equality holds:
˙ξ=cξ+gu(iq)−iq=cξ, ξ(nT)=0,t2∈[nT,nT+η),˙ξ=cξ,t2∈[nT+η,(n+1)T). | (3.32) |
Further, we can obtain
ξ(t2)=ec(t2−nT)ξ(nT)=0 | (3.33) |
where t2>nT.
Thus, when t→∞, we have
{ε1=θ1−θ2=0,ε2=ω1−ω2=0. | (3.34) |
According to the above analysis, when t→∞, the tracking control target is accomplished. Simultaneously, there are θ1=θ2 and ω1=ω2.
The simulation design parameters are designed as c=−2.1,d=0,k=2,g=200,η/T=0.5>0.22 and iM=5. The initial values of the system are selected as θ1(0)=0,ω1(0)=0, θ2(0)=50,ω2(0)=800 and ξ(0)=0.
Figures 3–10 show the simulation results of two kinds of control methods. Figure 3 displays the trajectories of θ1 and θ2. Figure 4 displays the trajectories of ω1 and ω2. The trajectories of error variables ε1 and ε2 are shown in Figures 5 and 6, respectively. From Figures 3–6, the simulation results indicate the proposed discontinuous control algorithm is valid. The trajectories of the control input iq are exhibited in Figure 7. Figure 8 exhibits the trajectories of saturated input u(iq). The trajectories of input saturation auxiliary system ξ are shown in Figure 9. The trajectories of signals ∫t0ξdt−∫nT0ξdt are exhibited in Figure 10. The comparison results show that the discontinuous controller designed in this paper can achieve similar control effects to the continuous controller.
The bicycle robot is a high-performance robot that integrates bicycles and intelligent control. It has both the convenience and flexibility of bicycles and the high intelligence of integrated control systems. Bicycle robots can replace human work on specific occasions, and they have broad application prospects in rescue and disaster relief, industrial production and resource exploration. The intermittent control algorithm designed in this paper has great application value in the balance control problem of the balance bicycle. Based on this, we carry out the experimental test of the intermittent control algorithm for a single balance control motor of the balance bike, which is shown in Figure 11. It can be seen that the system consists of a DC power source, a controller and a motor. The communication network is based on the controller area network (CAN). Figure 12 displays the trajectories of θ1 and θ2. Figure 13 shows the trajectories of ω1 and ω2. Figure 14 exhibits the trajectories of saturated input u(iq).
In this paper, we complete the PMSM tracking controller design by the practical discontinuous control algorithm. An input saturation auxiliary system is introduced to ensure the practicality of the controller, which overcomes the influence of input saturation on controller design and control performance. Then, the desired control goal is achieved by the sliding mode control method. Through stability analysis, the proposed controller can achieve the desired control goal. Finally, the effectiveness of the proposed algorithm is verified by simulation results and the experimental platform.
This work was funded by the National Natural Science Foundation of China under Grant 62173215, Grant 62006192, and Major Basic Research Program of the Natural Science Foundation of Shandong Province in China (ZR2021ZD04).
No potential conflict of interest was reported by the author(s).
[1] |
D. Yu, J. Long, C. L. P. Chen, Z. Wang, Adaptive swarm control within saturated input based on nonlinear coupling degree, IEEE Trans. Syst. Man Cybern.: Syst., 52 (2022), 4900–4911. https://doi.org/10.1109/TSMC.2021.3102587 doi: 10.1109/TSMC.2021.3102587
![]() |
[2] |
H. Xu, D. Yu, S. Sui, Y. P. Zhao, C. L. P. Chen, Z. Wang, Nonsingular practical fixed-time adaptive output feedback control of mimo nonlinear systems, IEEE Trans. Neural Networks Learn. Syst., (2022), 1–13. https://doi.org/10.1109/TNNLS.2021.3139230 doi: 10.1109/TNNLS.2021.3139230
![]() |
[3] |
T. Li, X. Sun, G. Lei, Z. Yang, Y. Guo, J. Zhu, Finite-control-set model predictive control of permanent magnet synchronous motor drive systems–an overview, IEEE-CAA J. Autom. Sin., 9 (2022), 2087–2105. https://doi.org/10.1109/JAS.2022.105851 doi: 10.1109/JAS.2022.105851
![]() |
[4] |
T. Zwerger, P. Mercorelli, Using a bivariate polynomial in an ekf for state and inductance estimations in the presence of saturation effects to adaptively control a pmsm, IEEE Access, 10 (2022), 111545–111553. https://doi.org/10.1109/ACCESS.2022.3215511 doi: 10.1109/ACCESS.2022.3215511
![]() |
[5] |
Z. Li, T. Li, G. Feng, R. Zhao, Q. Shan, Neural network-based adaptive control for pure-feedback stochastic nonlinear systems with time-varying delays and dead-zone input, IEEE Trans. Syst. Man Cybern.: Syst., 50 (2020), 5317–5329. https://doi.org/10.1109/TSMC.2018.2872421 doi: 10.1109/TSMC.2018.2872421
![]() |
[6] |
Y. X. Li, G. H. Yang, Observer-based fuzzy adaptive event-triggered control codesign for a class of uncertain nonlinear systems, IEEE Trans. Fuzzy Syst., 26 (2018), 1589–1599. https://doi.org/10.1109/TFUZZ.2017.2735944 doi: 10.1109/TFUZZ.2017.2735944
![]() |
[7] |
X. Sun, T. Li, Z. Zhu, G. Lei, Y. Guo, J. Zhu, Speed sensorless model predictive current control based on finite position set for pmshm drives, IEEE Trans. Transp. Electrif., 7 (2021), 2743–2752. https://doi.org/10.1109/TTE.2021.3081436 doi: 10.1109/TTE.2021.3081436
![]() |
[8] | T. Zwerger, P. Mercorelli, Combining a pi controller with an adaptive feedforward control in pmsm, in 2020 21th International Carpathian Control Conference (ICCC), (2020), 1–5. https://doi.org/10.1109/ICCC49264.2020.9257288 |
[9] |
Y. Li, Y. Liu, S. Tong, Observer-based neuro-adaptive optimized control of strict-feedback nonlinear systems with state constraints, IEEE Trans. Neural Networks Learn. Syst., 33 (2022), 3131–3145. https://doi.org/10.1109/TNNLS.2021.3051030 doi: 10.1109/TNNLS.2021.3051030
![]() |
[10] |
X. Xie, T. Wei, X. Li, Hybrid event-triggered approach for quasi-consensus of uncertain multi-agent systems with impulsive protocols, IEEE Trans. Circuits Syst. I-Regul. Pap., 69 (2022), 872–883. https://doi.org/10.1109/TCSI.2021.3119065 doi: 10.1109/TCSI.2021.3119065
![]() |
[11] |
J. Wei, S. Zhang, A. Adaldo, J. Thunberg, X. Hu, K. H. Johansson, Finite-time attitude synchronization with distributed discontinuous protocols, IEEE Trans. Autom. Control, 63 (2018), 3608–3615. https://doi.org/10.1109/TAC.2018.2797179 doi: 10.1109/TAC.2018.2797179
![]() |
[12] |
Z. Li, L. Chen, Z. Liu, Periodic solution of a chemostat model with variable yield and impulsive state feedback control, Appl. Math. Model., 36 (2012), 1255–1266. https://doi.org/10.1016/j.apm.2011.07.069 doi: 10.1016/j.apm.2011.07.069
![]() |
[13] |
W. Zhu, D. Wang, L. Liu, G. Feng, Event-based impulsive control of continuous-time dynamic systems and its application to synchronization of memristive neural networks, IEEE Trans. Neural Networks Learn. Syst., 29 (2018), 3599–3609. https://doi.org/10.1109/TNNLS.2017.2731865 doi: 10.1109/TNNLS.2017.2731865
![]() |
[14] |
X. Li, D. Peng, J. Cao, Lyapunov stability for impulsive systems via event-triggered impulsive control, IEEE Trans. Autom. Control, 65 (2020), 4908–4913. https://doi.org/10.1109/TAC.2020.2964558 doi: 10.1109/TAC.2020.2964558
![]() |
[15] |
X. Li, X. Yang, J. Cao, Event-triggered impulsive control for nonlinear delay systems, Automatica, 117 (2020), 108981. https://doi.org/10.1016/j.automatica.2020.108981 doi: 10.1016/j.automatica.2020.108981
![]() |
[16] |
X. Tan, J. Cao, Intermittent control with double event-driven for leader-following synchronization in complex networks, Appl. Math. Model., 64 (2018), 372–385. https://doi.org/10.1016/j.apm.2018.07.040 doi: 10.1016/j.apm.2018.07.040
![]() |
[17] | Z. Wang, C. Mu, S. Hu, C. Chu, X. Li, Modelling the dynamics of regret minimization in large agent populations: a master equation approach, in Proceedings of the Thirty-First International Joint Conference on Artificial Intelligence (IJCAI-22), 23 (2022), 534–540. https://doi.org/10.24963/ijcai.2022/76 |
[18] |
Y. Yang, Y. He, Non-fragile observer-based robust control for uncertain systems via aperiodically intermittent control, Inf. Sci., 573 (2021), 239–261. https://doi.org/10.1016/j.ins.2021.05.046 doi: 10.1016/j.ins.2021.05.046
![]() |
[19] |
S. Chen, G. Song, B. C. Zheng, T. Li, Finite-time synchronization of coupled reaction–diffusion neural systems via intermittent control, Automatica, 109 (2019), 108564. https://doi.org/10.1016/j.automatica.2019.108564 doi: 10.1016/j.automatica.2019.108564
![]() |
[20] |
Y. Wu, H. Li, W. Li, Intermittent control strategy for synchronization analysis of time-varying complex dynamical networks, IEEE Trans. Syst. Man Cybern.: Syst., 51 (2021), 3251–3262. https://doi.org/10.1109/TSMC.2019.2920451 doi: 10.1109/TSMC.2019.2920451
![]() |
[21] |
B. Wang, W. Chen, B. Zhang, Semi-global robust tracking consensus for multi-agent uncertain systems with input saturation via metamorphic low-gain feedback, Automatica, 103 (2019), 363–373. https://doi.org/10.1016/j.automatica.2019.02.002 doi: 10.1016/j.automatica.2019.02.002
![]() |
[22] |
V. T. Do, S. G. Lee, Neural integral backstepping hierarchical sliding mode control for a ridable ballbot under uncertainties and input saturation, IEEE Trans. Syst. Man Cybern.: Syst., 51 (2021), 7214–7227. https://doi.org/10.1109/TSMC.2020.2967433 doi: 10.1109/TSMC.2020.2967433
![]() |
[23] |
X. Yang, B. Zhou, F. Mazenc, J. Lam, Global stabilization of discrete-time linear systems subject to input saturation and time delay, IEEE Trans. Autom. Control, 66 (2021), 1345–1352. https://doi.org/10.1109/TAC.2020.2989791 doi: 10.1109/TAC.2020.2989791
![]() |
[24] |
Y. Su, Q. Wang, C. Sun, Self-triggered consensus control for linear multi-agent systems with input saturation, IEEE-CAA J. Autom. Sin., 7 (2020), 150–157. https://doi.org/10.1109/JAS.2019.1911837 doi: 10.1109/JAS.2019.1911837
![]() |
[25] |
C. Behn, K. Siedler, Adaptive pid-tracking control of muscle-like actuated compliant robotic systems with input constraints, Appl. Math. Model., 67 (2019), 9–21. https://doi.org/10.1016/j.apm.2018.10.012 doi: 10.1016/j.apm.2018.10.012
![]() |
[26] |
Q. Zhu, Y. Liu, G. Wen, Adaptive neural network control for time-varying state constrained nonlinear stochastic systems with input saturation, Inf. Sci., 527 (2020), 191–209. https://doi.org/10.1016/j.ins.2020.03.055 doi: 10.1016/j.ins.2020.03.055
![]() |
[27] |
Y. Wu, X. J. Xie, Adaptive fuzzy control for high-order nonlinear time-delay systems with full-state constraints and input saturation, IEEE Trans. Fuzzy Syst., 28 (2020), 1652–1663. https://doi.org/10.1109/TFUZZ.2019.2920808 doi: 10.1109/TFUZZ.2019.2920808
![]() |
[28] |
D. Yu, J. Long, C. L. P. Chen, Z. Wang, Bionic tracking-containment control based on smooth transition in communication, Inf. Sci., 587 (2022), 393–407. https://doi.org/10.1016/j.ins.2021.12.060 doi: 10.1016/j.ins.2021.12.060
![]() |
[29] |
H. Xu, D. Yu, S. Sui, C. L. P. Chen, An event-triggered predefined time decentralized output feedback fuzzy adaptive control method for interconnected systems, IEEE Trans. Fuzzy Syst., (2022), 1–14. https://doi.org/10.1109/TFUZZ.2022.3184834 doi: 10.1109/TFUZZ.2022.3184834
![]() |
[30] | T. Zwerger, P. Mercorelli, Combining smc and mtpa using an ekf to estimate parameters and states of an interior pmsm, in 2019 20th International Carpathian Control Conference (ICCC), (2019), 1–6. https://doi.org/10.1109/CarpathianCC.2019.8766063 |
[31] |
D. Yu, C. L. P. Chen, H. Xu, Fuzzy swarm control based on sliding-mode strategy with self-organized omnidirectional mobile robots system, IEEE Trans. Syst. Man Cybern.: Syst., 52 (2022), 2262–2274. https://doi.org/10.1109/TSMC.2020.3048733 doi: 10.1109/TSMC.2020.3048733
![]() |
[32] |
D. Shang, X. Li, M. Yin, F. Li, Dynamic modeling and fuzzy compensation sliding mode control for flexible manipulator servo system, Appl. Math. Model., 107 (2022), 530–556. https://doi.org/10.1016/j.apm.2022.02.035 doi: 10.1016/j.apm.2022.02.035
![]() |
[33] |
N. Zhang, W. Qi, G. Pang, J. Cheng, K. Shi, Observer-based sliding mode control for fuzzy stochastic switching systems with deception attacks, Appl. Math. Comput., 427 (2022), 127153. https://doi.org/10.1016/j.amc.2022.127153 doi: 10.1016/j.amc.2022.127153
![]() |
[34] |
W. H. Chen, X. Deng, W. X. Zheng, Sliding-mode control for linear uncertain systems with impulse effects via switching gains, IEEE Trans. Autom. Control, 67 (2022), 2044–2051. https://doi.org/10.1109/TAC.2021.3073099 doi: 10.1109/TAC.2021.3073099
![]() |
[35] |
L. Y. Hao, J. H. Park, D. Ye, Integral sliding mode fault-tolerant control for uncertain linear systems over networks with signals quantization, IEEE Trans. Neural Networks Learn. Syst., 28 (2017), 2088–2100. https://doi.org/10.1109/TNNLS.2016.2574905 doi: 10.1109/TNNLS.2016.2574905
![]() |
[36] |
A. Vahidi-Moghaddam, A. Rajaei, M. Ayati, Disturbance-observer-based fuzzy terminal sliding mode control for mimo uncertain nonlinear systems, Appl. Math. Model., 70 (2019), 109–127. https://doi.org/10.1016/j.apm.2019.01.010 doi: 10.1016/j.apm.2019.01.010
![]() |
[37] |
H. Xu, S. Li, D. Yu, C. Chen, T. Li., Adaptive swarm control for high-order self-organized system with unknown heterogeneous nonlinear dynamics and unmeasured states, Neurocomputing, 440 (2021), 24–35. https://doi.org/10.1016/j.neucom.2021.01.069 doi: 10.1016/j.neucom.2021.01.069
![]() |
[38] |
B. Jiang, H. R. Karimi, Y. Kao, C. Gao, Takagi–sugeno model based event-triggered fuzzy sliding-mode control of networked control systems with semi-markovian switchings, IEEE Trans. Fuzzy Syst., 28 (2020), 673–683. https://doi.org/10.1109/TFUZZ.2019.2914005 doi: 10.1109/TFUZZ.2019.2914005
![]() |
[39] |
G. Wang, J. Kuang, N. Zhao, G. Zhang, D. Xu, Rotor position estimation of pmsm in low-speed region and standstill using zero-voltage vector injection, IEEE Trans. Power Electron., 33 (2018), 7948–7958. https://doi.org/10.1109/TPEL.2017.2767294 doi: 10.1109/TPEL.2017.2767294
![]() |
[40] |
A. Kolli, O. Béthoux, A. D. Bernardinis, E. Labouré, G. Coquery, Space-vector pwm control synthesis for an h-bridge drive in electric vehicles, IEEE Trans. Veh. Technol., 62 (2013), 2441–2452. https://doi.org/10.1109/TVT.2013.2246202 doi: 10.1109/TVT.2013.2246202
![]() |
1. | Jia Tan, ShiLong Chen, ZhengQiang Li, Robust tracking control of a flexible manipulator with limited control input based on backstepping and the Nussbaum function, 2023, 20, 1551-0018, 20486, 10.3934/mbe.2023906 | |
2. | Xiao Wang, Xusheng Wu, Xi Xiao, Yulong Xie, A Polynomial Model and Multi-Objective Optimization Based Travel Curve Planning Method for Direct Motor Drive Actuators, 2023, 11, 2169-3536, 69773, 10.1109/ACCESS.2023.3289395 |