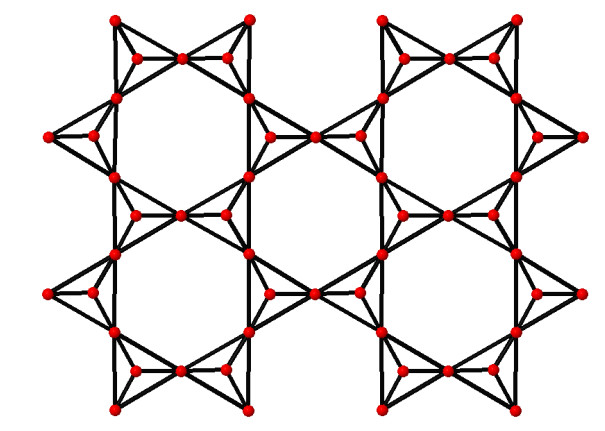
Humanity has always benefited from an intercapillary study in the quantification of natural occurrences in mathematics and other pure scientific fields. Graph theory was extremely helpful to other studies, particularly in the applied sciences. Specifically, in chemistry, graph theory made a significant contribution. For this, a transformation is required to create a graph representing a chemical network or structure, where the vertices of the graph represent the atoms in the chemical compound and the edges represent the bonds between the atoms. The quantity of edges that are incident to a vertex determines its valency (or degree) in a graph. The degree of uncertainty in a system is measured by the entropy of a probability. This idea is heavily grounded in statistical reasoning. It is primarily utilized for graphs that correspond to chemical structures. The development of some novel edge-weighted based entropies that correspond to valency-based topological indices is made possible by this research. Then these compositions are applied to clay mineral tetrahedral sheets. Since they have been in use for so long, corresponding indices are thought to be the most effective methods for quantifying chemical graphs. This article develops multiple edge degree-based entropies that correlate to the indices and determines how to modify them to assess the significance of each type.
Citation: Qingqun Huang, Muhammad Labba, Muhammad Azeem, Muhammad Kamran Jamil, Ricai Luo. Tetrahedral sheets of clay minerals and their edge valency-based entropy measures[J]. Mathematical Biosciences and Engineering, 2023, 20(5): 8068-8084. doi: 10.3934/mbe.2023350
[1] | Ricai Luo, Khadija Dawood, Muhammad Kamran Jamil, Muhammad Azeem . Some new results on the face index of certain polycyclic chemical networks. Mathematical Biosciences and Engineering, 2023, 20(5): 8031-8048. doi: 10.3934/mbe.2023348 |
[2] | Bing Wang, Fu Tan, Jia Zhu, Daijun Wei . A new structure entropy of complex networks based on nonextensive statistical mechanics and similarity of nodes. Mathematical Biosciences and Engineering, 2021, 18(4): 3718-3732. doi: 10.3934/mbe.2021187 |
[3] | Erik M. Bollt, Joseph D. Skufca, Stephen J . McGregor . Control entropy: A complexity measure for nonstationary signals. Mathematical Biosciences and Engineering, 2009, 6(1): 1-25. doi: 10.3934/mbe.2009.6.1 |
[4] | Fu Tan, Bing Wang, Daijun Wei . A new structural entropy measurement of networks based on the nonextensive statistical mechanics and hub repulsion. Mathematical Biosciences and Engineering, 2021, 18(6): 9253-9263. doi: 10.3934/mbe.2021455 |
[5] | Bei Liu, Hongzi Bai, Wei Chen, Huaquan Chen, Zhen Zhang . Automatic detection method of epileptic seizures based on IRCMDE and PSO-SVM. Mathematical Biosciences and Engineering, 2023, 20(5): 9349-9363. doi: 10.3934/mbe.2023410 |
[6] | David Cuesta-Frau, Pau Miró-Martínez, Sandra Oltra-Crespo, Antonio Molina-Picó, Pradeepa H. Dakappa, Chakrapani Mahabala, Borja Vargas, Paula González . Classification of fever patterns using a single extracted entropy feature: A feasibility study based on Sample Entropy. Mathematical Biosciences and Engineering, 2020, 17(1): 235-249. doi: 10.3934/mbe.2020013 |
[7] | Xiaoye Zhao, Yinlan Gong, Lihua Xu, Ling Xia, Jucheng Zhang, Dingchang Zheng, Zongbi Yao, Xinjie Zhang, Haicheng Wei, Jun Jiang, Haipeng Liu, Jiandong Mao . Entropy-based reliable non-invasive detection of coronary microvascular dysfunction using machine learning algorithm. Mathematical Biosciences and Engineering, 2023, 20(7): 13061-13085. doi: 10.3934/mbe.2023582 |
[8] | Enas Abdulhay, Maha Alafeef, Hikmat Hadoush, V. Venkataraman, N. Arunkumar . EMD-based analysis of complexity with dissociated EEG amplitude and frequency information: a data-driven robust tool -for Autism diagnosis- compared to multi-scale entropy approach. Mathematical Biosciences and Engineering, 2022, 19(5): 5031-5054. doi: 10.3934/mbe.2022235 |
[9] | Dawei Li, Enzhun Zhang, Ming Lei, Chunxiao Song . Zero trust in edge computing environment: a blockchain based practical scheme. Mathematical Biosciences and Engineering, 2022, 19(4): 4196-4216. doi: 10.3934/mbe.2022194 |
[10] | Ziqi Peng, Seiroh Okaneya, Hongzi Bai, Chuangxing Wu, Bei Liu, Tatsuo Shiina . Proposal of dental demineralization diagnosis with OCT echo based on multiscale entropy analysis. Mathematical Biosciences and Engineering, 2024, 21(3): 4421-4439. doi: 10.3934/mbe.2024195 |
Humanity has always benefited from an intercapillary study in the quantification of natural occurrences in mathematics and other pure scientific fields. Graph theory was extremely helpful to other studies, particularly in the applied sciences. Specifically, in chemistry, graph theory made a significant contribution. For this, a transformation is required to create a graph representing a chemical network or structure, where the vertices of the graph represent the atoms in the chemical compound and the edges represent the bonds between the atoms. The quantity of edges that are incident to a vertex determines its valency (or degree) in a graph. The degree of uncertainty in a system is measured by the entropy of a probability. This idea is heavily grounded in statistical reasoning. It is primarily utilized for graphs that correspond to chemical structures. The development of some novel edge-weighted based entropies that correspond to valency-based topological indices is made possible by this research. Then these compositions are applied to clay mineral tetrahedral sheets. Since they have been in use for so long, corresponding indices are thought to be the most effective methods for quantifying chemical graphs. This article develops multiple edge degree-based entropies that correlate to the indices and determines how to modify them to assess the significance of each type.
The entropy of a probability measures the uncertainty of a system. This concept is strongly based on statistical methodology. It is mainly used for chemical structures and their corresponding graphs. It also provides information about graph structure and chemical topologies. It was used as a notion for the first time in 1955. In many scientific and technical fields, entropy has applications. Intrinsic and extrinsic entries are determined in this way. The idea of degree power is used to investigate networks as information functional. The authors put forward the idea of entropy for different topological indices. The entropy of probability distributions is the foundation as described in. This parameter imparts a lot of information about structures, graphs, and chemical topology of networks in network theory, by which a parameter is known as degree powers. It is a base for graph theory in applied mathematics to investigate networks as information functional. Authors, put forward the idea of entropy for a variety of networks, like Shannon used for the entropy of probability distribution.
In modeling and designing any chemical network can be converted into a graph and this theory plays a very important role. By using the graph theoretical transformation of a chemical network or structure, we can determine many properties like physicochemical properties, thermodynamical properties, and biological activities. Among them, by using the topological indies certain properties can be characterized. Edge-weighted base entropy is also from the family of topological indices.
A simple molecular finite graph in chemical graph theory denoted both atom and chemical bond in terms of vertices and edges, respectively. The numeric value of the topological index shows the physical, chemical, and topological properties of a graph. With the help of chemical graph theory, we can model the mathematical phenomenon of chemical networks. It has a relation to the nontrivial application of graph theory for solving molecular problems. This theory plays a vital role in the field of chemical science. Chem-informatics is the combination of chemistry, mathematics, and information science. We can predict bio-activities and physical-chemical properties of the chemical compound by using QSAR and QSPR, which are examined by chem-informative [1].
The authors of [2], computed some topological indies and corresponded to their entropies. They discussed the relationship between numerical and graphical on the basis of topological indies and their entropies. Furthermore, they also discussed the topological indies which give fruitful results for the structural properties of g−C3N4, and some related applications are also available. According to [3], the authors find the entropy value by applying different parameters like the total number of vertices, edges, and degree of any graph. Then they compared the results with different graphs. They resulted that increasing the vertices and edges of graphs, affects increasing the entropy of such graphs. Some crystal structures based on non-kekulean benzenoid sub-structures are discussed in terms of entropies by using the degree-based topological indices [4]. They also computed the relationship between degree-based topological indices and degree-based entropy. They provided some applications in different topics like chemical, biological, and physical reactivity processes. Given in the article [5], topological indices of si2c3−I and si2c3−II and based on the results entropy measures are discussed. Here in [6], the researchers contributed towards a tool for molecular graphs which is based on the weight of edges, known as entropy. They relate the entropy measure with polynomial functions. Particularly, they computed the entropy measure of magnesium iodide structure and find different entropies like Zagreb and atom bond entropies.
In [7], the authors discussed the benzenoid hydrocarbon chemical structure. Shannon applied the benzenoid entropy in the transmission rate of telephonic channels, optical communication, and wireless. The impacts of high features in a different system. In this paper, the author discusses the characteristics and effects of graph entropies in different topological indies like coronoid polycyclic aromatic hydrocarbons. They computed the entropies through topological indies on degree terminal vertices. In [8], researchers find the effective roll of metal-insulator transition superlattices (GST-Sw) with different topological properties. Particularly, they discussed the atom-bond degree-based topological indices which are applied in the heat formation of single crystal and monolayered structure of Ge-sb-te. The relation of this structure is also available. Researchers of [9], find different entropies of crystallography chemical networks. The author particularly computed hyper and augmented Zagreb, forgotten, and Balaban entropies for the crystallography structures of cup-rite cu2o and titanium difluoride tif2 by using different topological tools. First, second, modified and augmented Zagreb indies, symmetric division, harmonic, inverse sum index, and forgotten indies are computed in [10]. They computed these indices for two chemical structures crystal cubic carbon and carbon graphite networks.
In [11], the author derived some degree-based temperature descriptors. He also computed temperature entropies of molecular structure and related to degree-based temperature with the help of specific information functions. He also compared graphically with the calculated function. All the computed results from the given information provided more effective drug and covid-19 vaccines. Authors of [12], focused on the carbon nanotubes which are useful in tissue engineering and investigated the various entropies. They computed some entropies for different variants of carbon nanotubes. They also investigated and compared the results of armchair carbon nanotubes with other forms of structure. The researchers of [13], investigated the relation between the natural polymer of cellulose network and pharmacological applicantion which provided a good result in a different structure. They computed different K-Banhatti indices and their entropies. The nature polymer of cellulose networks and their entropies are effective in various topics of chemical structural theory. Structures of Polycyclic aromatic hydrocarbons are discussed in [14], and authors elaborated intriguing properties based on the graph's theoretical parameters. They discussed entropy measures and their numerical values of given structures. Different entropies of the molecular structure of HCQ, by using degrees of vertices and edges, are discussed in [15]. Particularly, they computed degree-based topological characteristics of hydroxyethyl starch conjugated structures with hydroxychloroquine. They also presented some relations of different entropies with other structures. In the end, they compared the proven results in terms of numerical and graphical form.
Authors of [16], provide the main purpose of this article to study the properties of a graph and then discuss the structure like hyaluronic acid (HA) curcumin conjugates. Moreover, the author computed the entropies by using the degree-based topological indices with the help of the information function. Indices are linked with total π-electron property and measured some entropies of trans-PD-(NH2)s lattice and metal-organic super-lattice structures, by using the different graph parameters, in [17]. Structures of dendrimers based on cyclotriphosphazene (N3P3) which are applied to the topics of balanced and computed the EPR temperature spectrum, are discussed in [18]. First of all the author computed eccentricity-based indices and their entropies. After the above indices result the author presented it numerically and graphically. Entropies of some variants of Y-shaped nano-junctions are discussed in [19]. By using topological indices, knowledge discovery and representations of molecular structures are considered in [20]. Particularly, authors computed topological indies like atom-bond connectivity (ABC), the fourth version of ABC, geometric arithmetic (GA), and the fifth version of GA. Finally, the authors computed the above indices for octa-nano sheets, equilateral triangular, tetra sheets, rectangular sheets, and rectangular tetra sheets.
Graph entropies of porous graphene chemical networks are found in [21], by using some topological indices. They also provide some application of chosen indices-based entropies, particularly for the porous graphene structure. Different topological indies-based entropies of armchair carbon nanotubes are found in [22]. They provide some applications of variants of carbon nanotubes, like capped uncapped, and semi-capped which are purely used in memory devices and tissue engineering. Authors of [23] introduced the self-powered vertex degree topological indices and computed the graph entropy measures for tessellations networks, such as the isentropic later which is known as a non-isomorphic tessellation of kekulenes. Researchers of [24], computed the entropies of the structures of fractal and Cayley tree-type dendrimers by using the different topological indies and their degree-based results. The chosen structures are types of dendrimers denoted by Fr and Cm,n.
The Mostar, Padmakar-Ivan (PI), and Szeged indies of the molecular graph of two kinds of dendrimers, in which one is Phthalocyanines and 2nd is Porphyrine are discussed in [25]. The author also computed the entropies of these structures and relates these with other topological indies and their entropies.
Three classes of reticular metal-organic frameworks, graph entropies, enumeration of circuits, walks and topological properties are computed and analyzed in [26]. They also used different properties like eccentricities, radius, diameter, vertex, and degree to find the thermochemistry for the reticular network. In the [27] article, the authors describe and explain the characterization of two-dimensional coronene fractals in terms of different topological indies. The author also computed the entropy measure and compared it with other topological indies. they used machine learning techniques for robust computation of enthalpies. Moreover, they used NMR and ESR spectroscopic patterns of coronene fractals. In [28], the authors determine the data difficulty handling by using the entropy measure. For this, they collected the data in a different field from science, for this research work they faced a problem, the data is not accurate, and for this, they used the entropy measure to get the non-redundant, non-correlated data. The authors computed a good solution by using good algorithms for research and entropy measures. There is 25 entropy measure is taken for the classification procedure and compares its result. The crystal structure of the polyphenylene network for photocatalysis is discussed in [29], and find some topological indies. By using these indices they work for the thermodynamic properties namely entropy and heat which are taken fruitful results for the crystal structure. They concluded that effective application due to the chosen topological indies and their corresponding entropies occurred.
A biochemistry network namely t-level hypertrees of corona product of hypertrees with path are produced in [30]. The author also works with different topological indies like eccentricity-based indies and their entropies for the chosen biochemical network. Crystal structure of titanium difluoride TiF2 and the crystallographic structure of CU2O are discussed in the paper given by [31]. The author also computed the different entropies of these structures and relates them to different topological indies like first, second, and third redefined Zagreb indices, fourth atom bond connectivity index, fifth geometric arithmetic, and Sanskruti index and their entropies are discussed. For further results related to the topic is found in [32,33,34]. Moreover, closely related work can be found in [35,36,37,38,39,40,41,42,43,44,45].
Let E(G) be the edge and V(G) be the vertex of a graph. The degree of a vertex v in a graph determine by the attached edges with a vertex in the graph, and it is denoted by dv. A distance d(a,b) between a and b vertices are the shortest length path of the graph. The order and size of the graph are |V(G)| and |E(G)|, respectively. Following are some formulations of the topological indices we used to develop the entropies.
● First redefined Zagreb index
RZ1(G)=∑ab∈E(G)da+dbdadb. | (2.1) |
● 2nd redefined Zagreb index
RZ2(G)=∑ab∈E(G)dadbda+db. | (2.2) |
● Third redefined Zagreb index
RZ3(G)=∑ab∈E(G)dadb(da+db). | (2.3) |
● Augmented Zagreb index
AZ(G)=∑ab∈E(G)(dadbda+db−2)3. | (2.4) |
● Albertson index
A(G)=∑ab∈E(G)|da−db|. | (2.5) |
● Irregularity measure index
IM(G)=∑ab∈E(G)(da−db)2. | (2.6) |
● Reformulated Zagreb index
RZ(G)=∑ab∈E(G)(da−db−2)2. | (2.7) |
● Forgotten index
F(G)=∑a∈V(G)d3a. | (2.8) |
● First gourava index
GO1(G)=∑ab∈E(G)[da+db+dadb]. | (2.9) |
● First hyper gourava index
HGO1(G)=∑ab∈E(G)[da+db+dadb]2. | (2.10) |
● Second gourava index
GO2(G)=∑ab∈E(G)[(da+db).(dadb)]. | (2.11) |
● Second hyper gourava index
HGO2(G)=∑ab∈E(G)[(da+db).(dadb)]2. | (2.12) |
Given above is the first part of our methodology, corresponding to Eqs (2.1)–(2.12), the given below are edge-weighted based entropies. Some of these entropies are developed already, but most of them are new and we are developing and computing for the first time. The notation of entropy is used by the letter λRα(G) for the topological index Rα for the graph G.
● Entropy of first redefined Zagreb index
λRZ1(G)=−1RZ1(G)log[∏ab∈E(G)(da+dbdadb)da+dbdadb]+log(RZ1(G)). | (2.13) |
● Entropy the second redefined Zagreb index
λRZ2(G)=−1RZ2(G)log[∏ab∈E(G)(dadbda+db)dadbda+db]+log(RZ2(G)). | (2.14) |
● Entropy the third redefined Zagreb index
λRZ3(G)=−1RZ3(G)log∏ab∈E(G)[dadb(da+db)][dadb(da+db)]+log(RZ3(G)). | (2.15) |
● Entropy the augmented Zagreb index
λAZ(G)=−1AZI(G)log[∏ab∈E(G)[(dadbdadb−2)3](dadbdadb−2)3]+log(AZ(G)). | (2.16) |
● Entropy of Albertson index
λA(G)=−1AI(G)log[∏ab∈E(G)(|da−db|)(|da−db|)]+log(A(G)). | (2.17) |
● Entropy irregularity measure
λIM(G)=−1IM(G)log[∏ab∈E(G)[(da−db)2](da−db)2]+log(IM(G)). | (2.18) |
● Entropy reformulated Zagreb index
λRZ(G)=−1RZ(G)log[∏ab∈E(G)[(da−db−2)2](da−db−2)2]+log(RZ(G)). | (2.19) |
● Entropy of forgotten index
λF(G)=−1F(G)log[∏a∈V(G)[d3a]d3a]+log(F(G)). | (2.20) |
● Entropy of first gourava index
λGO1(G)=−1GO1(G)log[∏ab∈E(G)(da+db+dadb)(da+db+dadb)]+log(GO1(G)). | (2.21) |
● Entropy of first hyper gourava index
λHGO1(G)=−1HGO1(G)log[∏ab∈E(G)(da+db+dadb)(da+db+dadb)]+log(HGO1(G)). | (2.22) |
● Entropy of second gourava index
λGO2(G)=−1GO2(G)log[∏ab∈E(G)[(da+db)dadb][(da+db)dadb]]+log(GO2(G)). | (2.23) |
● Entropy of second hyper gourava index
λHGO2(G)=−1HGO2(G)log[∏ab∈E(G)[(da+db)dadb][(da+db)dadb]]+log(HGO2(G)). | (2.24) |
Clay mineral tetrahedral sheets are represented by the notation TSCMβ,γ. Various edge degree-based entropy measurements are applied to tetrahedral sheets in the aforementioned paper. One of the platonic graphs, the tetrahedral graph, has four vertices and six edges. It can be seen as a solid. The only planer construction of its isomorphic graphs, such as the complete graph K4 and wheel graph W4, is the tetrahedral graph. Chemical graph theory uses tetrahedral sheets made by tetrahedral graph polymerization to reflect silicone and other clay minerals. On tetrahedral sheets, interdisciplinary topics can be accommodated via combinatorial properties such as labeling, coloring, enumeration, and indexing as well as algorithmic operations such as shortest path and spanning tree. Figure 1 depicts the tetrahedral sheets of clay minerals TSCMβ,γ for β=2,γ=2. There are topological indices available in [46] for Eqs (4.1)–(4.12).
The graph TSCMβ,γ contains 10βγ+7β+γ are vertices and 24βγ+12β total count of edges. In TSCMβ,γ there are two types of vertices having degrees 3 or 6. The vertex set of TSCMβ,γ can be distributed according to their degrees.
Let
Vi={a∈V(TSCMβ,γ):da=i}. |
This indicates that the vertices of degrees i are present in the set Vi. According to their degree, the set of vertices is as follows:
V3={a∈V(TSCMβ,γ):da=3}V6={a∈V(TSCMβ,γ):da=6} |
Since |V3|=4βγ+6β+2γ and |V3|=6βγ+β−γ, we can also subdivide the edges of TSCMβ,γ into following three subsets according to the degree of its end vertices.
E3,3={ab∈TSCMβ,γ:da=3,db=3}E3,6={ab∈TSCMβ,γ:da=3,db=6}E6,6={ab∈TSCMβ,γ:da=6,db=6} |
Note that E(TSCMβ,γ)=E3,3∪E3,6∪Eβ,γ. The number of edges incident to one vertex of degree 3 and other vertices are 3,6 are 4β+2γ, 12βγ+10β+2γ respectively, so |E3,3|=4β+2γ, |E3,3|=12βγ+10β+2γ. The edges that are incident to two vertices of degree 6 are now the remaining number of edges, which are |E6,6|=12βγ−2β−4γ.
In this section, we will present our main results and some computational work in terms of topological indices and corresponding entropies.
RZ1I(G)=60βγ+20β−5γ. | (4.1) |
RZ2I(G)(G)=77128βγ+972β−1296γ. | (4.2) |
RZ3I(G)(G)=3275251242875βγ+83883843686000β−1781362531372000γ. | (4.3) |
AZ(G)=144βγ+60β−6γ. | (4.4) |
A(G)=36βγ+30β+6γ. | (4.5) |
IM(G)=108βγ+90β+18γ. | (4.6) |
RZ(G)=1392βγ+345β−270γ. | (4.7) |
F(G)=1404βγ+378β−162γ. | (4.8) |
GO1(G)=603βγ+234β−108γ. | (4.9) |
HGO1(G)=36396βγ+3582β−7308γ. | (4.10) |
GO2(G)=7128βγ+972β−1296γ. | (4.11) |
HGO2(G)=2554416βγ−9944β−688176γ. | (4.12) |
Now discuss the following properties of general topological invariant (IG) based on degree of vertices in a graphs (G).
● Entropy of first redefined Zagreb index
λRZ1(G)=−1RZ1(G)log[∏ab∈E(G)da+dbdadbda+dbdadb]+log(RZ1(G)). |
Computing the First Redefined Zagreb index by using Eq (2.1), we will get the result given in Eq (4.1). Now using Eq (4.1) in the formula of entropy of First Redefined Zagreb index, which is given in the Eq (2.13). After simplification, we will get the result given in the Eq (4.13).putting the values
λRZ1(G)=−110βγ+7β+γlog[[(23)23]4β+2γ×[(12)12]12βγ+10β+2γ×[(13)13]12βγ−2β−4γ]. |
λRZ1(G)=−110βγ+7β+γlog[(0.7631)(0.7071)(0.6934)]+log[10βγ+7β+γ]. | (4.13) |
● Entropy the second redefined Zagreb index
λRZ2(G)=−1RZ2(G)log[∏ab∈E(G)[dadbda+db][dadbda+db]]+log(RZ2(G)). |
Computing the Second Redefined Zagreb index by using Eq (2.1), we will get the result given in Eq (4.1). Now using Eq (4.1) in the formula of entropy of Second Redefined Zagreb index, which is given in the Eq (2.13). After simplification, we will get the result given in the Eq (4.14). Putting the value
λRZ2(G)=−160βγ+20β−5γlog[[2323]4β+2γ×[12]12βγ+10β+2γ×[13][13]12βγ−2β−4γ]+log[60βγ+20β−5γ]. |
λRZ2(G)=−160βγ+20β−5γlog[1.8371427]+log[60βγ+20β−5γ]. | (4.14) |
● Entropy the third redefined Zagreb index
λRZ3(G)=−1RZ3(G)log[∏ab∈E(G)[dadb(da+db)]dadb(da+db)]+log(RZ3(G)). |
Computing the Third Redefined Zagreb index by using Eq (2.2), we will get the result given in Eq (4.2). Now using Eq (4.2) in the formula of entropy of Third Redefined Zagreb index, which is given in the Eq (2.14). After simplification, we will get the result given in the Eq (4.15). Putting the values
λRZ3(G)=−17128βγ+972β−1296γlog[[3232]4β+2γ×[(2)2]12βγ+10β+2γ×[(3)3]12βγ−2β−4γ]+log[7128βγ+972β−1296γ]. |
λRZ3(G)=−17128βγ+972β−1296γlog[[(54)(54)(162)(162)](432)(432)]+log[7128βγ+972β−1296γ]. | (4.15) |
● Entropy the augmented Zagreb index {AZ(G)}
λAZ(G)=−1AZ(G)log[∏ab∈E(G)[(dadbdadb−2)3](dadbdadb−2)3]+log(AZ(G)),=−13275251242875βγ+83883843686000β−1781362531372000γ. |
Computing the augmented Zagreb AZ(G) index by using Eq (2.3), we will get the result given in Eq (4.3). Now using Eq (4.3) in the formula of entropy of augmented Zagreb AZ(G) index, which is given in the Eq (2.15). After simplification, we will get the result given in the Eq (4.16).
λAZ(G)=−13275251242875βγ+83883843686000β−1781362531372000γlog[[(54)54][4β+2γ]×[(162)(162)][12βγ+10β+2γ]×[(432)(432)][12βγ−2β−4γ]]+log[13275251242875βγ+83883843686000β−1781362531372000γ]. |
log[(64)64(187)187(3610)3610]+log[3275251242875βγ+83883843686000β−1781362531372000γ]. | (4.16) |
● Entropy for variation of Randić index R′(G)
λR′(G)=−1R′(G)log[∏ab∈E(G)[1max(dadb)][1max(dadb)]]+log(R′(G)) |
Computing the Variation of Randić index R′(G) by using Eq (2.4), we will get the result given in Eq (4.4). Now using Eq (4.4) in the formula of entropy of Variation of Randić index R′(G), which is given in the Eq (2.16). After simplification, we will get the result given in the Eq (4.17). Putting the values
λR′(G)=−1144βγ+60β−6γlog[((729343)729343)4β+2γ×((729512)729512)12βγ+10β+2γ×((58324913)58324913)12βγ−2β−4γ]+1144βγ+60β−6γ. |
λR′(G)=−1144βγ+60β−6γlog[1max31max61max6]+[144βγ+60β−6γ]. | (4.17) |
● Entropy Albertson index
λA(G)=−1A(G)log[∏ab∈E(G)(|da−db|)(|da−db|)]+log(A(G)). |
Computing the Albertson index by using Eq (2.5), we will get the result given in Eq (4.5). Now using Eq (4.5) in the formula of entropy of Albertson index, which is given in the Eq (2.17). After simplification, we will get the result given in the Eq (4.18). Putting the values
λA(G)=−136βγ+30β+6γlog[(3)12βγ+10β+2γ]+log[36βγ+30β+6γ]. | (4.18) |
● Entropy irregularity measure
λIM(G)=−1IM(G)log[∏ab∈E(G)[(da−db)2](da−db)2]+log(IM(G)). |
Computing the Irregularity measure by using Eq (2.6), we will get the result given in Eq (4.6). Now using Eq (4.6) in the formula of entropy of Irregularity measure, which is given in the Eq (2.18). After simplification, we will get the result given in the Eq (4.18). Putting the values
λIM(G)=−1108βγ+90β+18γlog[((9)9)12βγ+10β+2γ]+log[108βγ+90β+18γ]. | (4.19) |
● Enrtopy Reformulated Zagreb index
λRZ(G)=−1RZ(G)log[∏ab∈E(G)[(da−db−2)2](da−db−2)2]+log(RZ(G)). |
Computing the reformulated Zagreb index by using Eq (2.7), we will get the result given in Eq (4.7). Now using Eq (4.7) in the formula of entropy of Reformulated Zagreb index, which is given in the Eq (2.19). After simplification, we will get the result given in the Eq (4.20). Putting the values
λRZ(G)=−11392βγ+345β−270γlog[[(4)4]4β+2γ×[(25)25]12βγ+10β+2β×[(4)4]12βγ−2β−4γ]+log[1392βγ+345β−270γ]. | (4.20) |
● Entropy of forgotten index
λF(G)=−1F(G)log[∏a∈V(G)[d3a]d3a]+log(F(G)). |
Computing the forgotten Zagreb index by using Eq (2.8), we will get the result given in Eq (4.8). Now using Eq (4.8) in the formula of entropy of Forgotten Zagreb index, which is given in the Eq (2.20). After simplification, we will get the result given in the Eq (4.21). Puttting the values
λF(G)=−11404βγ+378β−162γlog[[((3)3)27]4βγ+6β+2γ×[((6)3)216]6βγ+β−γ]+log[1404βγ+378β−162γ]. | (4.21) |
● Entropy of first gourava index
λGO1(G)=−1GO1(G)log[∏ab∈E(G)(da+db+dadb)(da+db+dadb)]+log(GOI1(G)). |
Computing the first gourava index by using Eq (2.9), we will get the result given in Eq (4.9). Now using Eq (4.9) in the formula of entropy of First Gourava index, which is given in the Eq (2.21). After simplification, we will get the result given in the Eq (4.22). Putting the values.
λGO1(G)=−1603βγ+234β−108γlog[[(15)15]4β+2γ×[(27)27]12βγ+10β+2γ×[(48)48]12βγ−2β−4γ]+log[603βγ+234β−108γ]. | (4.22) |
● Entropy of first hyper gourava index
λHGO1(G)=−1HGO1(G)log[∏ab∈E(G)(da+db+dadb)(da+db+dadb)]+log(HGO1(G)). |
Computing the first hyper gourava index by using Eq (2.10), we will get the result given in Eq (4.10). Now using Eq (4.10) in the formula of entropy of First Hyper Gourava index, which is given in the Eq (2.22). After simplification, we will get the result given in the Eq (4.23). Putting the values
λHGO1(G)=−136396βγ+3582β−7308γlog[[(15)15]4β+2γ×[(27)27]12βγ+10β+2γ×[(48)48]12βγ−2β−4γ]+log[36396βγ+3582β−7308γ]. | (4.23) |
● Entropy of second gourava index
λGO2(G)=−1GO2(G)log[∏ab∈E(G)[(da+db)dadb][(da+db)dadb]]+log(GO2(G)). |
Computing the second gourava index by using Eq (2.11), we will get the result given in Eq (4.11). Now using Eq (4.11) in the formula of entropy of Second Gourava index, which is given in the Eq (2.23). After simplification, we will get the result given in the Eq (4.24). Putting the values.
λGO2(G)=−17128βγ+972β−1296γlog[[(54)54]4β+2γ×[(162)162]12βγ+10β+2γ×[(432)432]12βγ−2β−4γ]+log[7128βγ+972β−1296γ]. | (4.24) |
● Entropy of second hyper gourava index
λHGO2(G)=−1HGO2(G)log[∏ab∈E(G)[(da+db)dadb][(da+db)dadb]]+log(HGO2(G)). |
Computing the scond Hyper Gourava index by using Eq (2.12), we will get the result given in Eq (4.12). Now using Eq (4.12) in the formula of entropy of Second Hyper Gourava index, which is given in the Eq (2.24). After simplification, we will get the result given in the Eq (4.25). Putting the values
λHGOI2(G)=−12554416βγ−99144β−68817γlog[((54)54)4β+4γ×((162)162)12βγ+10β+2γ]+log((432)432)12βγ−2β−4γ+log[2554416βγ−99144β−688176γ]. | (4.25) |
A network or structure's edge weight-based entropy offers structural details and in-depth content in the form of mathematical equations. This study contained the edge valency-based entropies of tetrahedral sheets of clay minerals. It highlights the molecular attributes in the form of a logarithmic function and offers the structural information of chemical networks or their related build-up graphs. These entropies are newly developed in this work and can be considered as an application or computational work for other networks or structures as a future direction for the researchers.
This work was supported by the National Science Foundation of China (11961021 and 11561019), Guangxi Natural Science Foundation (2020GXNSFAA159084), and Hechi University Research Fund for Advanced Talents (2019GCC005).
The author declare that they have no conflict of interest.
[1] |
R. Luo, A. Khalil, A. Ahmad, M. Azeem, I. Gafurjan, M. F. Nadeem, Computing The partition dimension of certain families of toeplitz graph, Front. Comput. Neurosci., 2022 (2022), 1–7. https://doi.org/10.3389/fncom.2022.959105 doi: 10.3389/fncom.2022.959105
![]() |
[2] | Z. Chu, M. K. Siddiqui, S. Manzoor, S. A. K. Kirmani, M. F. Hanif, M. H. Muhammad, On rational curve fitting between topological indices and entropy measures for graphite carbon nitride, Polycyclic Aromat. Compd., 2022 (2022), 1–18. |
[3] | I. Tugal, D. Murat, Çizgelerde yapısal karmaşıklığın olçülmesinde farklı parametrelerin kullanımı, Muş Alparslan Üniv. Mühendislik Mimarlık Fak. Derg., 2022 (2022), 22–29. |
[4] | M. A. Alam, M. U. Ghani, M. Kamran, Degree-based entropy for a non-kekulean benzenoid graph, J. Math., 2022 (2022). |
[5] | X. Wang, M. K. Siddiqui, S. A. K. Kirmani, S. Manzoor, S. Ahmad, M. Dhlamini, On topological analysis of entropy measures for silicon carbides networks, Complexity, 2021 (2021). https://doi.org/10.1155/2021/4178503 |
[6] | M. S. Alatawi, A. Ahmad, A. N. A. Koam, Edge weight-based entropy of nagnesium iodide graph, J. Math., 2021 (2021), 1–7. |
[7] | M. Rashid, S. Ahmad, M. Siddiqui, M. Kaabar, On computation and analysis of topological index-based invariants for complex coronoid systems, Complexity, 2021 (2021). |
[8] |
R. Huang, M. H. Muhammad, M. K. Siddiqui, S. Khalid, S. Manzoor, E. Bashier, Analysis of topological aspects for Metal-Insulator transition superlattice network, Complexity, 2022 (2022), 8344699. https://doi.org/10.1155/2022/8344699 doi: 10.1155/2022/8344699
![]() |
[9] |
M. K. Siddiqui, S. Manzoor, S. Ahmad, M. K. A. Kaabar, On computation and analysis of entropy measures for crystal structures, Math. Probl. Eng., 2021 (2021), 9936949. https://doi.org/10.1155/2021/9936949 doi: 10.1155/2021/9936949
![]() |
[10] |
Y. Chu, M. Imran, A. Q. Baig, S. Akhter, M. K. Siddiqui, On M-polynomial-based topological descriptors of chemical crystal structures and their applications, Eur. Phys. J. Plus, 135 (2020), 874. https://doi.org/10.1140/epjp/s13360-020-00893-9 doi: 10.1140/epjp/s13360-020-00893-9
![]() |
[11] |
C. Feng, M. H. Muhammad, M. K. Siddiqui, S. A. K. Kirmani, S. Manzoor, M. F. Hanif, On entropy measures for molecular structure of remdesivir system and their applications, Int. J. Quantum Chem., 122 (2022), e26957. https://doi.org/10.1002/qua.26957 doi: 10.1002/qua.26957
![]() |
[12] |
M. Imran, A. Ahmad, Y. Ahmad, M. Azeem, Edge weight based entropy measure of different shapes of carbon nanotubes, IEEE Access, 9 (2021), 139712–139724. https://doi.org/10.1109/ACCESS.2021.3119032 doi: 10.1109/ACCESS.2021.3119032
![]() |
[13] |
R. Huang, M. K. Siddiqui, S. Manzoor, S. Khalid, S. Almotairi, On physical analysis of topological indices via curve fitting for natural polymer of cellulose network, Eur. Phys. J. Plus, 137 (2022), 410. https://doi.org/10.1140/epjp/s13360-022-02629-3 doi: 10.1140/epjp/s13360-022-02629-3
![]() |
[14] |
K. Julietraja, P. Venugopal, S. Prabhu, A. K. Arulmozhi, M. K. Siddiqui, Structural analysis of three types of PAHs using entropy measures, Polycyclic Aromat. Compd., 42 (2022), 1–31. https://doi.org/10.1080/10406638.2021.1884101 doi: 10.1080/10406638.2021.1884101
![]() |
[15] | S. Manzoor, M. K. Siddiqui, S. Ahmad, On entropy measures of polycyclic hydroxychloroquine used for novel coronavirus (COVID-19) treatment, Polycyclic Aromat. Compd., 2020 (2020), 1–26. |
[16] | S. Manzoor, M. K. Siddiqui, S. Ahmad, Degree-based entropy of molecular structure of hyaluronic acid–curcumin conjugates, Eur. Phys. J. Plus, 136 (2021), 1–21. |
[17] | S. Manzoor, M. K. Siddiqui, S. Ahmad, On physical analysis of degree-based entropy measures for metal–organic superlattices, Eur. Phys. J. Plus, 136 (2021), 1–22. |
[18] | R. Huang, M. K. Siddiqui, S. Manzoor, S. Ahmad, M. Cancan, On eccentricity-based entropy measures for dendrimers, Heliyon, 7 (2021), e07762. |
[19] |
A. N. A. Koam, M. Azeem, M. K. Jamil, A. Ahmad, K. H. Hakami, Entropy measures of Y-junction based nanostructures, Shams Eng. J., 14 (2023), 101913. https://doi.org/10.1016/j.asej.2022.101913 doi: 10.1016/j.asej.2022.101913
![]() |
[20] |
F. E. Alsaadi, S. A. H. Bokhary, A. Shah, U. Ali, J. Cao, M. O. Alassafi, et al., On knowledge discovery and representations of molecular structures using topological indices, J. Artif. Intell. Soft Comput. Res., 11 (2021), 21–32. https://doi.org/10.2478/jaiscr-2021-0002 doi: 10.2478/jaiscr-2021-0002
![]() |
[21] |
M. C. Shanmukha, A. Usha, N. S. Basavarajappa, K. C. Shilpa, Graph entropies of porous graphene using topological indices, Comput. Theor. Chem., 1197 (2021), 113142. https://doi.org/10.1016/j.comptc.2021.113142 doi: 10.1016/j.comptc.2021.113142
![]() |
[22] |
X. Zuo, M. F. Nadeem, M. K. Siddiqui, M. Azeem, Edge weight based entropy of different topologies of carbon nanotubes, IEEE Access, 9 (2021), 102019–102029. https://doi.org/10.1109/ACCESS.2021.3097905 doi: 10.1109/ACCESS.2021.3097905
![]() |
[23] |
S. R. J. Kavitha, J. Abraham, M. Arockiaraj, J. Jency, K. Balasubramanian, Topological characterization and graph entropies of tessellations of kekulene structures: existence of isentropic structures and applications to thermochemistry, nuclear magnetic resonance, and electron spin resonance, J. Phys. Chem., 125 (2021), 8140–8158. https://doi.org/10.1021/acs.jpca.1c06264 doi: 10.1021/acs.jpca.1c06264
![]() |
[24] |
M. Imran, S. Manzoor, M. K. Siddiqui, S. Ahmad, M. H. Muhammad, On physical analysis of synthesis strategies and entropy measures of dendrimers, Arab. J. Chem., 15 (2022), 103574. https://doi.org/10.1016/j.arabjc.2021.103574 doi: 10.1016/j.arabjc.2021.103574
![]() |
[25] | S. Manzoor, M. K. Siddiqui, S. Ahmad, Computation of entropy measures for phthalocyanines and porphyrins dendrimers, Int. J. Quant. Chem., 122 (2022), e26854. |
[26] |
J. Abraham, M. Arockiaraj, J. Jency, S. R. J. Kavitha, K. Balasubramanian, Graph entropies, enumeration of circuits, walks and topological properties of three classes of isoreticular metal organic frameworks, J. Math. Chem., 60 (2022), 695–732. https://doi.org/10.1007/s10910-021-01321-8 doi: 10.1007/s10910-021-01321-8
![]() |
[27] |
M. Arockiaraj, J. Jency, J. Abraham, S. R. J. Kavitha, K. Balasubramanian, Two-dimensional coronene fractal structures: topological entropy measures, energetics, NMR and ESR spectroscopic patterns and existence of isentropic structures, Mol. Phys., 120 (2022), e2079568. https://doi.org/10.1080/00268976.2022.2079568 doi: 10.1080/00268976.2022.2079568
![]() |
[28] |
P. Juszczuk, J. Kozak, G. Dziczkowski, S. Głowania, T. Jach, B. Probier, Real-world data difficulty estimation with the use of entropy, Entropy, 23 (2021), 1621. https://doi.org/10.3390/e23121621 doi: 10.3390/e23121621
![]() |
[29] |
J. Liu, M. H. Muhammad, S. A. K. Kirmani, M. K. Siddiqui, S. Manzoor, On Analysis of Topological Aspects of Entropy Measures for Polyphenylene Structure, Polycyclic Aromat. Compd., 2022 (2022), 1–21. https://doi.org/10.1080/10406638.2022.2043914 doi: 10.1080/10406638.2022.2043914
![]() |
[30] |
M. Rashid, S. Ahmad, M. K. Siddiqui, S. Manzoor, M. Dhlamini, An analysis of eccentricity-based invariants for biochemical hypernetworks, Complexity, 2021 (2021), 1974642. https://doi.org/10.1155/2021/1974642 doi: 10.1155/2021/1974642
![]() |
[31] |
M. K. Siddiqui, S. Manzoor, S. Ahmad, M. K. A. Kaabar, On computation and analysis of entropy measures for crystal structures, Math. Probl. Eng., 2021 (2021), 9936949. https://doi.org/10.1155/2021/9936949 doi: 10.1155/2021/9936949
![]() |
[32] |
Y. Shang, Sombor index and degree-related properties of simplicial networks, Appl. Math. Comput., 419 (2022), 126881. https://doi.org/10.1016/j.amc.2021.126881 doi: 10.1016/j.amc.2021.126881
![]() |
[33] |
Y. Shang, Lower bounds for Gaussian Estrada index of graphs, Symmetry, 10 (2018), 325. https://doi.org/10.3390/sym10080325 doi: 10.3390/sym10080325
![]() |
[34] |
S. Khan, S. Pirzada, Y. Shang, On the sum and spread of reciprocal distance laplacian eigenvalues of graphs in terms of Harary index, Symmetry, 14 (2022), 1937. https://doi.org/10.3390/sym14091937 doi: 10.3390/sym14091937
![]() |
[35] |
M. Azeem, M. F. Nadeem, Metric-based resolvability of polycyclic aromatic hydrocarbons, Eur. Phys. J. Plus, 136 (2021), 1–14. https://doi.org/10.1140/epjp/s13360-021-01399-8 doi: 10.1140/epjp/s13360-021-01399-8
![]() |
[36] |
A. Ahmad, A. N. A. Koam, M. H. F. Siddiqui, M. Azeem, Resolvability of the starphene structure and applications in electronics, Shams Eng. J., 2021 (2021), forthcoming. https://doi.org/10.1016/j.asej.2021.09.014 doi: 10.1016/j.asej.2021.09.014
![]() |
[37] |
M. F. Nadeem, M. Hassan, M. Azeem, S. U. D. Khan, M. R. Shaik, M. A. F. Sharaf, et al., Application of resolvability technique to investigate the different polyphenyl structures for polymer industry, J. Chem., 2021 (2021), 1–8. https://doi.org/10.1155/2021/6633227 doi: 10.1155/2021/6633227
![]() |
[38] |
M. Azeem, M. K. Jamil, A. Javed, A. Ahmad, Verification of some topological indices of Y-junction based nanostructures by M-polynomials, J. Math., 2022 (2022), forthcoming. https://doi.org/10.1155/2022/8238651 doi: 10.1155/2022/8238651
![]() |
[39] |
M. Azeem, M. Imran, and M. F. Nadeem, Sharp bounds on partition dimension of hexagonal Möbius ladder, J. King Saud Univ. Sci., 2021 (2021), forthcoming. https://doi.org/10.1016/j.jksus.2021.101779 doi: 10.1016/j.jksus.2021.101779
![]() |
[40] |
H. Raza, J. B. Liu, M. Azeem, M. F. Nadeem, Partition dimension of generalized petersen graph, Complexity, 2021 (2021), 1–14. https://doi.org/10.1155/2021/5592476 doi: 10.1155/2021/5592476
![]() |
[41] |
A. N. A. Koam, A. Ahmad, M. Ibrahim, M. Azeem, Edge metric and fault-tolerant edge metric dimension of hollow coronoid, Mathematics, 9 (2021), 1405. https://doi.org/10.3390/math9121405 doi: 10.3390/math9121405
![]() |
[42] |
H. Wang, M. Azeem, M. F. Nadeem, A. U, Rehman, A. Aslam, On fault-tolerant resolving sets of some families of ladder networks, Complexity, 2021 (2021), 9939559. https://doi.org/10.1155/2021/9939559 doi: 10.1155/2021/9939559
![]() |
[43] |
A. Shabbir, M. Azeem, On the partition dimension of tri-hexagonal alpha-boron nanotube, IEEE Access, 9 (2021), 55644–55653. https://doi.org/10.1109/ACCESS.2021.3071716 doi: 10.1109/ACCESS.2021.3071716
![]() |
[44] |
M. F. Nadeem, M. Azeem, A. Khalil, The locating number of hexagonal Möbius ladder network, J. Appl. Math. Comput., 66 (2021), 149–165. https://doi.org/10.1007/s12190-020-01430-8 doi: 10.1007/s12190-020-01430-8
![]() |
[45] |
H. M. A. Siddiqui, M. A. Arshad, M. F. Nadeem, M. Azeem, A. Haider, M. A. Malik, Topological properties of supramolecular chain of different complexes of N-salicylidene-L-Valine, Polycyclic Aromat. Compd., 42 (2022), 6185–6198. https://doi.org/10.1080/10406638.2021.1980060 doi: 10.1080/10406638.2021.1980060
![]() |
[46] |
H. Raza, M. F. Nadeem, A. Ahmad, M. A. Asim, M. Azeem, Comparative study of valency-based topological indices for tetrahedral sheets of clay minerals, Curr. Org. Synth., 18 (2021), 711–718. https://doi.org/10.2174/1570179418666210709094729 doi: 10.2174/1570179418666210709094729
![]() |
1. | Amal S. Alali, Shahbaz Ali, Muhammad Adnan, Delfim F. M. Torres, On Sharp Bounds of Local Fractional Metric Dimension for Certain Symmetrical Algebraic Structure Graphs, 2023, 15, 2073-8994, 1911, 10.3390/sym15101911 | |
2. | Muhammad Farhan Hanif, Hasan Mahmood, Shahbaz Ahmad, On degree-based entropy measure for zero-divisor graphs, 2024, 16, 1793-8309, 10.1142/S1793830923501045 |