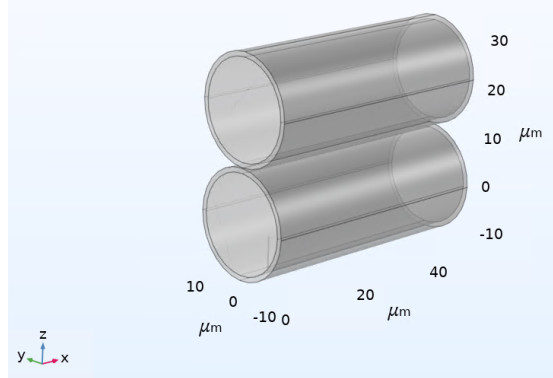
We have developed a numerical model of two osculating cylindrical elastic renal tubules to investigate the impact of neighboring tubules on the stress applied to a primary cilium. We hypothesize that the stress at the base of the primary cilium will depend on the mechanical coupling of the tubules due to local constrained motion of the tubule wall. The objective of this work was to determine the in-plane stresses of a primary cilium attached to the inner wall of one renal tubule subject to the applied pulsatile flow, with a neighboring renal tube filled with stagnant fluid in close proximity to the primary tubule. We used the commercial software COMSOLⓇ to model the fluid-structure interaction of the applied flow and tubule wall, and we applied a boundary load to the face of the primary cilium during this simulation to produces a stress at its base. We confirm our hypothesis by observing that on average the in-plane stresses are greater at the base of the cilium when there is a neighboring renal tube versus if there is no neighboring tube at all. In combination with the hypothesized function of a cilium as a biological fluid flow sensor, these results indicate that flow signaling may also depend on how the tubule wall is constrained by neighboring tubules. Our results may be limited in their interpretation due to the simplified nature of our model geometry, and further improvements to the model may potentially lead to the design of future experiments.
Citation: Nerion Zekaj, Shawn D. Ryan, Andrew Resnick. Fluid-structure interaction modelling of neighboring tubes with primary cilium analysis[J]. Mathematical Biosciences and Engineering, 2023, 20(2): 3677-3699. doi: 10.3934/mbe.2023172
[1] | Niksa Praljak, Shawn D. Ryan, Andrew Resnick . Pulsatile flow through idealized renal tubules: Fluid-structure interaction and dynamic pathologies. Mathematical Biosciences and Engineering, 2020, 17(2): 1787-1807. doi: 10.3934/mbe.2020094 |
[2] | Zhangli Peng, Andrew Resnick, Y.-N. Young . Primary cilium: a paradigm for integrating mathematical modeling with experiments and numerical simulations in mechanobiology. Mathematical Biosciences and Engineering, 2021, 18(2): 1215-1237. doi: 10.3934/mbe.2021066 |
[3] | Ling Xu, Yi Jiang . Cilium height difference between strokes is more effective in driving fluid transport in mucociliary clearance: A numerical study. Mathematical Biosciences and Engineering, 2015, 12(5): 1107-1126. doi: 10.3934/mbe.2015.12.1107 |
[4] | Huu Thuan Nguyen, Tu Anh Do, Benoît Cosson . Numerical simulation of submerged flow bridge scour under dam-break flow using multi-phase SPH method. Mathematical Biosciences and Engineering, 2019, 16(5): 5395-5418. doi: 10.3934/mbe.2019269 |
[5] | K. Maqbool, S. Shaheen, A. M. Siddiqui . Effect of nano-particles on MHD flow of tangent hyperbolic fluid in a ciliated tube: an application to fallopian tube. Mathematical Biosciences and Engineering, 2019, 16(4): 2927-2941. doi: 10.3934/mbe.2019144 |
[6] | Aftab Ahmed, Javed I. Siddique . The effect of magnetic field on flow induced-deformation in absorbing porous tissues. Mathematical Biosciences and Engineering, 2019, 16(2): 603-618. doi: 10.3934/mbe.2019029 |
[7] | Sangita Swapnasrita, Joost C de Vries, Carl M. Öberg, Aurélie MF Carlier, Karin GF Gerritsen . Computational modeling of peritoneal dialysis: An overview. Mathematical Biosciences and Engineering, 2025, 22(2): 431-476. doi: 10.3934/mbe.2025017 |
[8] | Oualid Kafi, Nader El Khatib, Jorge Tiago, Adélia Sequeira . Numerical simulations of a 3D fluid-structure interaction model for blood flow in an atherosclerotic artery. Mathematical Biosciences and Engineering, 2017, 14(1): 179-193. doi: 10.3934/mbe.2017012 |
[9] | Li Cai, Yu Hao, Pengfei Ma, Guangyu Zhu, Xiaoyu Luo, Hao Gao . Fluid-structure interaction simulation of calcified aortic valve stenosis. Mathematical Biosciences and Engineering, 2022, 19(12): 13172-13192. doi: 10.3934/mbe.2022616 |
[10] | Alex Viguerie, Sangita Swapnasrita, Alessandro Veneziani, Aurélie Carlier . A multi-domain shear-stress dependent diffusive model of cell transport-aided dialysis: analysis and simulation. Mathematical Biosciences and Engineering, 2021, 18(6): 8188-8200. doi: 10.3934/mbe.2021406 |
We have developed a numerical model of two osculating cylindrical elastic renal tubules to investigate the impact of neighboring tubules on the stress applied to a primary cilium. We hypothesize that the stress at the base of the primary cilium will depend on the mechanical coupling of the tubules due to local constrained motion of the tubule wall. The objective of this work was to determine the in-plane stresses of a primary cilium attached to the inner wall of one renal tubule subject to the applied pulsatile flow, with a neighboring renal tube filled with stagnant fluid in close proximity to the primary tubule. We used the commercial software COMSOLⓇ to model the fluid-structure interaction of the applied flow and tubule wall, and we applied a boundary load to the face of the primary cilium during this simulation to produces a stress at its base. We confirm our hypothesis by observing that on average the in-plane stresses are greater at the base of the cilium when there is a neighboring renal tube versus if there is no neighboring tube at all. In combination with the hypothesized function of a cilium as a biological fluid flow sensor, these results indicate that flow signaling may also depend on how the tubule wall is constrained by neighboring tubules. Our results may be limited in their interpretation due to the simplified nature of our model geometry, and further improvements to the model may potentially lead to the design of future experiments.
Primary cilla are small hair-like organelles that protrude from nearly every cell in the body [1]. Primary cilla are thought to be mechanosensory organelles, which means that when an external stimulus is applied to a primary cilium, evidence that the cell has responded includes the release of intracellular calcium from the endoplasmic reticulum into the cytosol. The initiation of this response is currently thought to be driven by an initial influx of extracellular calcium into the "cilioplasm" [2] via calcium channels located on the ciliary membrane. The complexity of primary cilium dynamics involves the mechanical and chemical properties of cilia, specifically within the context of fluid flow [3]. Our paper will primarily focus on the mechanical stress at the base of the primary cilium; our model is unique due to the setting in which we investigate these properties. We take an in-vivo perspective by modelling a single primary cilium in a linear elastic tube with a neighboring tube that deforms the primary tube. An additional benefit of this modeling framework is that it is easily adapted to account for neighboring kidney tubes rather than a single tube in isolation [4]. This further advances our understanding of kidney tubule interaction in the in-vivo setting throughout this work. This is due to the fact that kidney and nephron tubes are complex structures that are in close proximity to one another [5].
The fluid flow profile we implement is categorized as pulsatile/oscillatory, as the human body exhibits flow through the kidney in a pulsatile manner [6]. Through mathematical modelling not only can we better understand the fluid dynamics within a kidney, but we are also able to perform simulations for varying parameter values in a controlled environment. To this end, a foundation has been studied in the recent work by Praljak et al. where the authors developed a multiscale model of an idealized renal tube [4]. Also, there is experimental evidence in the field of primary cilla that suggests that fluid flow plays a significant role in the mechanical and chemical behavior [7,8]. As a result, there is significant motivation to understand and model primary cilla within the context of kidney/renal tubes. However, as previously stated, the literature explicitly connecting the tubule dynamics applied to a cilium is lacking. Typically, past models are split between only elastic tube modeling [9,10] and primary cilium modeling [11,12]. We attempt to combine both approaches in a geometric model.
The main challenge in modelling primary cilium and/or kidney tubes is in large part due to their complex geometries. The anatomy of a kidney consists of many individual nephrons, a glomerular membrane, collecting tubules, a renal pelvis and a ureter. All of these biological structures weave together in a complicated albeit efficient manner. Primary cilla, despite their tiny structure also contain many intricate properties, including the basal body on which the primary cilium protrudes, a cilium membrane, an axoneme and nine microtubules inside of the axoneme of the cilium. Recent work regarding primary cilium modelling and imaging has been done to an incredibly advanced degree [13]. As a first step, our model will assume an idealized approach to the anatomy, but the modeling framework developed here will be capable of adding relevant complexity to the geometry that may influence the mechanical responses.
The importance of studying primary cilla involves not only understanding its theoretical components, but also the links of various kidney diseases to primary cilium function [14]. The model presented in this paper can provide an essential first step in developing a complex multiscale modeling framework that can be observed and analyzed for greater understanding of the relationship between primary cilium and kidney functions. This approach will also provide a new tool which will lead to additional analysis in the future regarding primary cilium in the context of kidney fluid flow. This model can pair well with laboratory based experiments involving cilla [11,15]. The combination of experiments and modelling can unlock a plethora of information regarding primary cilla, so we expect that the model will provide testable predictions that may be the basis of future experiments.
The biological understanding underlying the model will be based on well established literature [16] and experiments [17] to help build some of the key concepts of the model. This includes the flow profile [18,19] and reference to the pulsatile nature of the proximal tubes [20], kidney tube thickness and length and thickness of primary cilla. Our model was built using the software COMSOL Multiphysics [21], a finite element analysis software with the purpose of solving systems of partial differential equations for relevant physical models with specific geometries. COMSOL® allows for the efficient numerical simulation of the model presented, as well as control for accuracy and stability. There has already been recent work using COMSOL® for primary cilium analysis [16].
We model a pair of three dimensional renal tubes that are within d of the touching distance. The tubes are presented geometrically as a pair of cylinders of length L with radius r (see Figure 1). Moreover, we model soft tubes as an isotropic linear elastic material, parameterized with the Young's modulus E, Poisson ratio u, and density ρt. Each tube is filled with an incompressible Newtonian fluid, parameterized by dynamic viscosity μ and fluid density ρf. However, only one tube will experience a fluid flow, as the other tube is filled with a stagnant fluid (with identical fluid material parameters μ and ρf). This is because we are taking the biological process that involves flow through a nephron tube that was initially not experiencing a pulsatile fluid flow. Our model captures the onset of the pulsatile inflow from a stagnant flow, and since we are attempting to model a subsection of a nephron, this implies that other subsections of the nephron may not be immediately experiencing the incoming flow yet. It is a reasonable exploration in future work to assume a consistent pulsatile flow profile along the entirety of the nephron, in which case we can design both tubes to experience flow. However, that is not the focus of this work. Since we are modeling fluid flow in renal tubules, we can take the fluid flow to be laminar and pulsatile (time-dependent flow); this is a result of biological fluid flow usually having a low Reynolds number. The resulting flow can be modeled using the time-dependent Navier-Stokes equation; furthermore we expect a fluid-structure interaction (FSI) to occur between the fluid and renal tubes as a result of fluid pressure being imposed on the renal tubule wall, which will cause the tubule to expand outward. Since the tubes are in close proximity with one another, we expect that if one tube expands outward, it will contact the neighboring tubule; thus the primary tubule exhibits constrained motion. This contact can be modelled in COMSOL® by defining a contact pair between the two tubes, with the tube experiencing FSI as the source and the neighboring tube with no FSI as the destination. The interaction between the renal tubules allows them to push against and deform each other. One significant complication of our model is that we can no longer simplify the governing equations by, for example, considering the system to be axisymmetric.
We also model a single primary cilium inside the primary tubule. The primary cilium is modelled as an isotropic linear elastic material, with a slender body of length l. Geometrically, we identify the primary cilium as a slender cylinder with a hemispherical cap at the distal end of the cilium protruding into the primary tubule fluid-filled interior space. While more advanced mechanical models of a primary cilium are under development [22], the model we present here is less concerned with modeling the detailed cilium deformation due to fluid drag and more concerned with modeling the applied mechanical stress generated by the deformed tubule acting on the base of a cilium. From a three dimensional perspective however, this is a rather standard approach to modeling the primary cilia in finite-element analysis software [11,23,24]. Normally, we would additionally model the FSI between the flow and primary cilla in a manner similar to [25] and [26]; however, to reduce computational cost and complexities of modeling multiple FSI and contact pairs, we instead model the fluid-cilium FSI in terms of a force F acting along the surface of the cilium in the direction of the fluid flow. While this is a simplification, it permits calculation of the primary cilium displacement at the distal tip and calculation of the stress at the base of the cilium, which are the most significant model outputs.The basal body of the primary cilia is less developed in the mathematical modeling community, particularly from the perspective of 3-D modeling. This is because of the complicated geometry of the basal body, which encompasses many compartments. Our "basal body" is simply a portion of the primary cilia being embedded into the elastic tube. The portion inside of the tube is taken to be the basal body, which mimics the idea that the basal body is something that does not protrude from the cell body.
The model used in COMSOL® for the FSI module was a combination of laminar flow and structural mechanics; we constructed our model to mimic as close as possible to renal physiology with contact between renal tubules. The fluid flow is characterized as incompressible single phase flow with a low Reynolds number. The fluid is defined as a viscous Newtonian fluid. The imposed flow profile is pulsatile, driven by a periodic forcing of applied pressure. Fluid flow is modeled using the Navier-Stokes equation
ρf(∂u∂t+u⋅∇u)=−∇p+∇⋅(μ(∇u+(∇u)T)+F | (2.1) |
∇⋅u=0 | (2.2) |
where u describes the velocity field. We consider a full three-dimensional geometry resulting in the coordinates u=(ux,uy,uz) to describe our spatial domain. The dynamical viscosity and fluid density are denoted as μ and ρf, respectively. In previous work, the body force term F was set as zero; however, since our model has a pair of tubes in close proximity to one another, we expect some external force to be placed on the primary tube from the adjacent tube after the primary tube expands to some size. We, however, do not have control of that force as it is a result of the tube interaction; so, for now we say F≠0.
Since our model undergoes structural deformation for both the primary cilium and renal tubes, we must describe the way in which the coordinate system changes as a result of the deformation. We represent a point of a material particle in its original location as E(x,y,z), and this point E stays with the particle even under deformation; this is denoted as the material frame. We can track the material particle's position as a function of time, which we can denote as X=X(E,t), where X is denoted as the spatial frame. We adopt the fact that the spatial coordinate X is fixed spatially, and that the material frame E is fixed to the body. We can physically describe the distance travelled by the particle with the displacement vector d(E,t), so we can formulate a transformation between the material and spatial frame with the equation X=E+d(E,t). These structural formulations are important for us, as they allow us to describe physical processes that occur on the body, such as strain and stress. The process of straining can mathematically be formulated with the deformation gradient δ:
δ=∂X∂E=I+∂d∂E | (2.3) |
δ is the Jacobian matrix that describes the transformation between the material and spatial frame and I is the identity tensor. So, if we take the determinant of the deformation gradient, we obtain det(δ)=J, where J describes the volume deformation changes, which will prove to be very important when COMSOL® computes the deformation of the renal tubes and primary cilium. We can also express the determinant of the deformation gradient as J=dVdV0, which can tell us explicitly how the volume changes under deformation.
Naturally, before material deformation occurs the following equation can be introduced for any such volume V0
ρ0∂2d∂t2=fv+∇E⋅PT | (2.4) |
This is the differential momentum balance equation for a solid. P is the first Piola-Kirchhoff stress tensor and fv represents the volumetric body forces. We introduce the following expression for the Piola-Kirchhoff stress tensor P=(I+Δd)S which relates the Cauchy and Kirchhoff stress tensors by the equation P=Jσδ−T=τδ−T, where σ and τ are the Cauchy and Kirchhoff stress tensors, respectively. Setting S=S(Λ), and substituting our known relations we obtain the following equation:
ρ0∂2d∂t2=fv+∇E⋅(I+Δd)S(Λ) | (2.5) |
This equation allows us to describe the differential momentum balance equation for a linear elastic material through the relation of S(Λ) where Λ is the Green-Lagrange strain tensor.
COMSOL® uses an arbitrary Lagrange-Eulerian (ALE) formulation when modeling FSI in the respective software [4,27]. In the ALE formulation, both the Eulerian and Lagrangian frames are used to describe the physical behavior of the system. Solid mechanics are described using the Lagrangian framework and fluid dynamics are described using the Eulerian framework. The specific dynamics are from the fluid interacting with the tube walls via pressure and viscous forces on the fluid solid interface of the renal tubes, which cause the tube walls to deform (usually expanding outwards from the pressure). We also expect a change in the flow pattern as a result of the renal tubes changing their shapes. The following equations play a key role in describing this interaction:
u=∂v∂t | (2.6) |
(σ⋅n)fluid=(σ⋅n)wall | (2.7) |
The above equation tells us that the fluid velocity at the solid boundary wall is equal to the solid stress at the boundary of the renal tube forming a type of continuity condition. We also will mention that the tubes are surrounded by a vacuum (which means that there is no physical material outside of the tubes); future work can include submerging the renal tubes in a fluid that generates a visco-elastic response, coupling the two tubes even when not in physical contact. Also, choosing a vacuum surrounding reduces computational cost and allows us to isolate wall-wall interplay between the renal tubes.
We also consider the force placed on the primary cilium, and we take a parameterization approach by gently ramping up the force placed on the face of the cilium as time goes forward
c∗t=FA | (2.8) |
This equation is the specific load we define for our analysis. The variable c is the initial strength of the force placed on the cilium surface, and t is the time parameter used in COMSOL®. We take FA to be the force per area, and we place the force along the face of the cilium in the same direction as the fluid flow is expected to enter (the x-direction).
Instead of a point load approach, we take an area approach to closely mimic the force that would be placed on the primary cilium if it was purely fluid flow instead. The reason why we take the approach of artificially placing a force on the cilium rather than utilizing a FSI module, is two fold: first it is better from a computational cost/time perspective, and second we are able to easily adjust the force placed on the cilium by simply adjusting the constant c, so we can perform numerical experiments in a cost effective manner and yield useful results (Figure 2).
The last important set of equations we have to describe are the contact pair equations. We take the default, i.e., penalty factor, approach that calculates the contact using a stiff approximation. We believe that using the penalty factor is sufficient for the model, since we are modeling contact between two different renal tubes. If there was self-contact between tubes, we would consider the augmented Lagrangian approach. We also make the assumption that there is no friction or adhesion at the contact point, which is consistent with the biology of the model. The main governing equation to describe the contact pair using the penalty method is
Tn={−pndg+p0ifdg<p0pn0ifdg≥p0pn | (2.9) |
where dg is the gap distance between the two surfaces, pn is the penalty factor parameter, which we can think of as the spring stiffness between the contact points, and p0 is the pressure at the zero gap (the point of contact). We can think of the penalty method as having a spring between the contact points, where there is a "pushback" between the points of contact. Also, the penalty factor parameter can be manually defined or preset depending on the physics of the model. COMSOL® gives a preset choice of stability or speed, and since the tubes are not starting off in contact, we utilize the stability preset. However, if they had started off in contact, it would be conventional to prefer the speed preset [28]. Equation (2.9) particularly defines the contact pressure (Tn) between the renal tubes.
Since we are modelling tube flow, the first two boundary conditions we must consider are the inlet and outlet conditions. At the inlet, a pulsatile pressure is applied, and for the outlet, we have the flow described using the atmospheric fluid pressure at the outlet. We take the flow profile (and notation) from a previous paper by Praljak et al. [4], which we review briefly here
P(t)=P0+Pθ={P0ψ(t)t=[0−0.1]P0(1+αsin(ωt))t≥0.1 | (2.10) |
where P0 is the time-independent, pressure profile and Pθ is the time-dependent pressure profile. From a modelling perspective, the flow is initially introduced by first "filling" the tube with fluid until it reaches P0, at which time we introduce the time varying component of the flow Pθ, so as to introduce a fully developed pulsatile flow. Furthermore, utilizing a ramping function makes the initial conditions at t = 0 seconds, with zero for the fluid profile and no physical deformation initially. The stability of the solution is therefore improved upon, due to the problem being well-conditioned. The other important parameters are stated as follows: ψ(t) is the ramping step function, which is used to introduced the initial ramping/filling of the flow in the tube, α is the flow amplitude, and ω is the flow angular frequency. By convention, we set the angular frequency to be ω=2π[Hz], since the renal tubes are modeled for humans (this frequency can be adjusted depending on physical context such as animal species). The interior walls of the tubes maintain the conventional no-slip boundary conditions; however, future studies may require more sophisticated boundary conditions to consider due to the complexities of the renal tubes such as wall permeability [29].
We also describe the boundary conditions for the contact pair, with the primary tube (the tube containing the cilium) being the source and the adjacent tube (tube adjacent to primary tube) as the destination. We apply the contact pair boundary condition only on the section of the tubes guaranteed to touch, and for the adjacent tube, we apply a fixed constraint condition on the opposite face to prevent the adjacent tube from being pushed upward entirely as a structure (see Figure 3). We can, therefore, think of this boundary condition as an anchor, which prevents unrealistic movement. Lastly, as stated previously, we place a load across the face of the primary cilia, applied in the same direction as the fluid flow.
A brief discussion regarding the attachment point of the primary cilium and inner tube wall is required. We constructed the geometry in such a way that the cilium is anchored to the inner tube wall. This can loosely be compared to the basal body of the primary cilium, with the main body of the cilium extending out into the tube lumen. We do not apply any specific boundary condition to the distal attachment point of the cilium and inner tube, so as to allow the cilium to be free to deform at that attachment via the the renal deformation due to the fluid flow. This way, the physics of the attachment point is free to behave, via the influence of the FSI by the inner tube and flow pressure, and the boundary load is on the face of the cilium. Our goal for this work was to explore the stress at the place of attachment between the cilium and renal tube, which we will refer to as the base of the primary cilium moving forward (Figure 4).
Parameter values used for the model were found through various sources; the parameter values with references are presented in Table 1. We can adjust certain parameters to emphasize a particular physical behavior. Adjustable parameters include the fluid pressure, force placed on the primary cilium and depending on the fluid pressure, we also adjust the fluid oscillation. This is to keep within the time scale defined. The parameters we keep constant for the numerical experiment and analysis include the Young's modulus (both the tube and primary cilium), fluid density, density of the primary cilium and tube, fluid viscosity, diameter of the inner and outer renal tubes, length of the primary cilium and length of the renal tubes. We also have our time as t∈[02] with a time step of tn=0.1 seconds.
Parameters (symbol) | Numerical Values | Ref. |
Distance between tubes (d) | 0.1 μm | |
Length of tubes (L) | 50 μm | [18] |
Radius of inner tubes (r) | 10 μm | [20] |
Tube thickness | 1 μm | [20] |
Young's modulus (E) | 1×105 Pa | [18] |
Poisson ratio (u) | 0.3 | |
Tube density (ρt) | 1100 kgm3 | [30] |
Dynamic viscosity (μ) | 5×10−3 Pas | [31] |
Fluid density (ρf) | 1010 kgm3 | [32] |
Time averaged pressure (P0) | 0.1 kPa | [19] |
Length of primary cilia (l) | 6 μm | |
Bendinging rigidity (EI) | 5×10−23 Pa m4 | [33] |
Given the context of this research, it is appropriate to introduce the following dimensionless numbers: Reynolds number and Womersley number. The Reynolds number is defined as follows:
Re=ρfuLμ | (2.11) |
where Re is the Reynolds number, ρf is the fluid density, u is the speed of the fluid, μ is the dynamic viscosity and L is the characteristic length, which, in this case, would be the length of the tubule. Since we are in the context of bioflow, the Reynolds number is taken to be very low, which is common in these kinds of problems. The velocity of our model varies; however, for illustrative purposes, we present a calculation. We take the maximum fluid speed to be at t=1 seconds, and since our flow is modelled as a Poiseuille flow, the max flow speed is taken to be at the center of the elastic tube (radius being zero). COMSOL allows us to extract this data directly from the velocity profile generated from the solution. Furthermore, at t=1 seconds, the pulsatile flow is near a maximum for the pressure distribution, which is our justification for the time chosen. Thus, at t=1 seconds, we calculate that Re≈0.11 for a fine mesh (this data point is taken from Figure 4) and a pressure of 100 [Pa]. As a result, the Reynolds number will not play a significant role in this study. The Womersley number is defined as follows:
Wo=L(ωρfμ)12 | (2.12) |
The parameters are similar to the Reynolds number; however, in addition, we take ω to be the angular frequency of the fluid oscillation. This number is relevant in our paper since it relates pulsatile flow, using a pressure driven profile, to viscous effects of fluids. Considering the parameters taken, Wo≈.056.
As mentioned previously, we used finite element analysis software (COMSOL®) to obtain stable solutions. The benefit of using COMSOL® is the ability to construct the geometry of the tubes and cilium, in addition to having control of the solution method. As COMSOL® is advanced software, we are able to control for how we want to solve the problem numerically, in addition to the number of mesh elements we would like to use to construct our solution. Moreover, the model requires solving the Navier-Stokes equations and equations of solid mechanics, all of which are coupled. COMSOL® is capable of handling such large and often complicated computations. The user of the software still needs to consider the type of solution method, tolerance factor, desired accuracy of the model, meshing and geometry complications. Lastly, we can stabilize or simplify the model without losing physical meaning and still obtain accurate, stable solutions.
We must first consider how we interpret flow in the model. Previous work has considered ramping up the fluid profile steadily until it reaches a steady state, and then applying a oscillatory flow. We also adopt that approach by having the pressure of the fluid increase from a pressure gradient of zero at t=0 to P0 at t=0.1. After it reaches this state, oscillation is induced in the pressure profile to mimic the biological flow expected in renal tubes from t=0.1 to t=tend. This approach allows for the initial conditions to be zero for fluid flow, solid mechanic deformation, and meshing. Praljak et al. showed that the accuracy of solutions relative to the fineness of the mesh must be considered [4], as a result, we chose a user-controlled meshing. From their specific model, they primarily utilized a normal mesh; however, since we have contact between two renal tubes, we chose to make the mesh fine, as it is suggested that due to contact analysis [34], a finer mesh may be considered. Since we have a three-dimensional model, our mesh elements will be composed of tetrahedrons.
In the COMSOL® model, we implement a fluid loading in solid structure coupling, which is applicable since we expect a single directional coupling due to the fluid flow pressure applied to the renal tube boundaries. The way we have set up the solution method on our model is through a parameterization method. We solve from t=0 to t=tend with a time step of tstep=0.1, but for each time step, we solve for the steady-state solution of the solid mechanics for each time-parameterized time step, as well as for the time-dependent solution for the flow profile. First, the flow profile is solved for, and at each time step, the physical process for each time step induced by the flow is assigned to the solid mechanic. So, each time step represents a "steady state" solution profile, but the flow profile is time-dependent. This parameterization method is a standard procedure for highly nonlinear models, and it improved computational cost compared to the standard time-dependent solution method. The time-dependent method solves the problem through a nonlinear approach, utilizing the constant Newton method. With a damping factor of 0.9, our termination is done by using a tolerance method. We take the number of iterations in our tolerance method to be nine, with a tolerance factor of 0.5. The stationary solver, which is done through our parameterization, uses the double dogleg nonlinear method, with an initial damping of 1. Similar to the time-dependent approach, it uses a tolerance method for termination, with the maximum number of iterations taken to be 25 and a tolerance factor of 1.
To ensure grid independence it is crucial for us to partake in different mesh configurations; in COMSOL, this can be either physically controlled or manually controlled. We utilized a physics-controlled function and explored three different types of meshes: coarse, normal, and fine. Since our model is three-dimensional tetrahedrons are generated to construct the model geometry. Praljak et al. saw that for an idealized tube model, no divergence or mesh dependency was found [4]. They observed that the mesh generated after normal converged similarly to finer meshes; however, extremely coarse mesh saw some numerical output differences. This implied that more mesh elements contributed to more accurate solutions. This is why for our grid independence, we chose only coarse, normal and fine. Since the model depends on the shear forces and normal forces that expand the tubes as a result of fluid pressure, we focus on the fluid velocity profile along the radius of the tube. We begin near the center of the tube with pulsatile flow (primary tube), and we move out near the walls of the tubule.
We see in Figure 5 that there is almost no difference in model output. This shows that our model does not have a dependency on meshing, which verifies that our numerical model is indeed stable. To ensure the utmost accuracy in the analysis, we constructed the geometry as outlined in Section 2, and we saw no divergence or error. We present the relevant statistics for each case. For a coarse mesh, we have 64,982 domain elements, 8396 boundary elements and 715 edge elements. The numbers of tetrahedra and triangles were 55,610 and 8260, respectively, with a simulation time of 3 minutes and 16 seconds. For a normal mesh, we have 116,895 domain elements, 12,534 boundary elements and 898 edge elements. The numbers of tetrahedra and triangles were 102,751 and 12,370, respectively, with a simulation time of 5 minutes and 23 seconds. For a fine mesh, we have 336,358 domain elements, 23,484 boundary elements and 1296 edge elements. The numbers of tetrahedra and triangles were 306,398 and 23,256, respectively, with a simulation time of 15 minutes and 36 seconds. Boundary layers are generated along the inner walls of the tubules, which is the case for every mesh generated. The differences of computation time for the simulation is to be expected, since a finer mesh would equate to more elements that need to be accounted for during the simulation time. So, we expect that the finer the mesh, the longer the simulation time. Finer meshes for our model is possible; however, this would increase computational time drastically and geometric properties may have to be readjusted depending on the meshes generated. Regardless, our grid independence study tells us that we do not need to venture further into meshing analysis and generation, since our model converges to a solution in a predictable manner and with reasonable time.
The model we have developed can be used to study multiple mechanistic behaviors of a renal and primary cilium system. For the purpose of this paper however, we will be studying the attachment point of the primary cilium and the renal tube, as there is some mathematical and physical interest regarding this area. The study of the attachment point of the cilium and renal tube, may have strong correlation with the mechanistic behavior of the basal body, an important section of the primary cilium. The basal body is a biological structure located at the base of the primary cilium, where the cilium protrudes. Mathematically, the behavior of the basal body is important in understanding the boundary conditions for a classical Euler-Bernoulli beam equation, which is a 4th-order partial differential equation, often used in the literature to model a cilium as an elastic rod. Biologically, there could be important relations with basal body behavior and various known kidney diseases. Understanding the behavior at the base of the cilium may subsequently unlock information of the cilium itself, which in turn, can allow us to potentially make inferences for some kidney diseases (Figure 6).
We explore the attachment point, which we will refer to as the base of the primary cilium moving forward, by analyzing the stress that the base of the cilium undergoes when it is 1) the primary cilium is displaced by some force, and 2) the renal tube to which the cilium is attached is deformed as a result of fluid pressure (the deformation is an outward expansion). We are also going to have to be aware of the two specific placements of the primary cilium we must analyze as well; the first place is if the cilium is adjacent to a neighboring tube and the second place is if the cilium is away from the neighboring tube and is therefore unaffected by it. We hypothesize that interplay of the neighboring renal tubes will play a role in the stress analysis at the base of the primary cilium. The numerical experiment will be that a single tube, called the primary tube (since the primary cilium will be located in it), will have an inflow of fluid with an assigned pressure distribution, and the adjacent tube will have the same geometric and parametric structure of the primary tube; however, there is no flow present and it only acts as a barrier that interacts with the primary tube. This interaction occurs when the primary tube expands and, upon expansion, makes contact with the adjacent tube (see Figure 3). The primary cilium will be located on the tube directly adjacent to the adjacent tube, so the base of the cilium will be near the adjacent tube; however, no direct contact is made.
We carry out the analysis by following this procedure: We first construct a combination of varying displacements of the primary cilium by inducing a load on the face of the cilium in the same direction of the fluid flow and the magnitude in pressure of the incoming flow. The boundary load on the primary cilium will be 1, 5, 25, 50, 75 or 100 t (all in units of Pascals), and the magnitude of pressure will be 100,500, 1000, 1500, 2000 or 2500 (all in units of Pascals).
From our data, we observe that, indeed, the neighboring tube does play an important role when analyzing the stress at the attachment point of the primary cilium. We show this by taking the difference of the stress at the attachment point of the primary cilium with a neighboring renal tube near that attachment point, versus if it was away.
Sd=Sn−Sa, | (4.1) |
where Sd is the stress difference, Sn is the stress of the attachment point when the cilium is near the neighboring tube, and Sa is the stress of the attachment point when the cilium is away from the neighboring tube. We observed that by obtaining consistent values of Sd>0, it would determine the stress of the primary cilium attachment point near the neighboring tube was greater than if it was away; this gives us a simple equation, but with meaningful physical results. We observed that throughout all possible experiments carried out, with the varying parameter, consistently the stress of the attachment point of the cilium was greater when the neighboring renal tube was adjacent to the attachment point, as compared to if the attachment point was away from the neighboring renal tube. In addition to the consistent difference, we observed that the discrepancy of the differences between the stress of the primary cilium near the neighboring tube, versus away from the neighboring tube, would increase depending on the pressure of the incoming fluid. We also observed that we obtained negative values for some of the stresses, which implies that compression was occurring at the attachment point. We saw that this happened mainly as a result of the displacement due to the boundary load playing a more significant role compared to that of the expansion of the primary renal tube as a result of the fluid pressure.
In Figures 7–12, we show the stress for when the primary cilium is near the adjacent tube, so we can observe the dynamics with wall interference. From Figures 10–12, we see some overlap of the differences; however, this is likely a result of the oscillation pattern of the flow profile in relation to the magnitude of the pressure; further numerical studies would be required to find a definitive answer for the overlap. It may also be because of the primary cilium displacement as a result of the boundary load. Thus, it is possible that after a certain amount of load is placed on the primary cilium, the tube expansion becomes obsolete or saturates to a max value, since, for Figures 9–11, we stop seeing obvious oscillatory stress differences that we would expect to coincide with the flow pattern. The current results, however, tell us that primary cilium positioning relative to the neighboring tube does play a role in stress at the attachment point. We also see that fluid pressure is an important factor. For example, throughout Figures 7–12, we see that if the maximum pressure is only at 100 [Pa], there is almost no difference at all; this is because the fluid pressure is not strong enough to cause a tube expansion large enough to interact with the neighboring tube in any meaningful, physical manner. However, the moment we begin to increase the pressure, we see that the differences begin to become more profound from our numerical experiments.
Figures 13–18 show the stress for when the primary cilium is away from the neighboring tube; that way, we can observe the dynamics without potential interference. We see from figure 13 that, initially, there is no compression occurring at the base of the primary cilium; this is because the displacement of the primary cilium is minuscule in relation to the tube expansion. However, as we move from Figures 13–18, we see that compression occurs at the base of the primary cilium. However, when the tube force of the expansion supersedes the displacement of the primary cilium, eventually, the tensile stress usurps the compression stress. What is curious is that from Figures 16–18, we see for a small fluid pressure (100 [Pa]), that the stress exhibits a type of upward parabolic shape, and this may explain the shape of the stress difference toward the end of the simulation time.
In this paper, we presented a novel COMSOL® model exploring the effects of neighboring renal tubes with a stress analysis of the attachment of a primary cilium. We did this by utilizing an FSI built-in module in COMSOL®, numerical modeling software that solves coupled partial differential equations by methods of discretization. Through our numerical experiments, we were able to successfully show that neighboring renal tubes play an important role in the stress placed on the primary cilium. As a result, we believe that future experimental work may place a greater emphasis on the neighboring environment of the primary cilium within a flow chamber or some type of elastic tube. Novel understanding was gained in this work by performing analysis of a primary cilium with a neighboring renal tube present and comparing results when there is or is not an adjacent tube within the vicinity of the primary tube. The results presented may also open up the possibility to explore compression analysis for primary cilla, since mainly compression occurs near the base of the cilium. It could be that not only tensile stress but also compression may influence the chemical and mechanical properties of the base of the cilium and, subsequently, the basal body.
The following are suggestions to advance the model, that will be explored in future works.
● The model uses an artificial boundary load; we believe that this concept can be coupled with lab-based experiments to obtain data for both the numerical and experimental settings. This could then be used for direct comparisons. This can illuminate the model through experimental justification, and it can help build future experimental protocols.
● While the artificial boundary load has its advantages, the reality is that the primary cilium is displaced with fluid flow, and as a result, it would greatly improve the realism of the model if we could incorporate a dual fluid structure interaction procedure, with both tube expansion and primary cilium displacement.
● The geometry of both the tube and primary cilium are idealized; the reality is that both the tube and primary cilium are complicated geometric structures, and so it would be beneficial to potentially add advanced CAD software to improve upon the geometry of the model.
● The material specified for both the tubes and the primary cilium models are linear elastic; however, for biological models, it would be more realistic to consider nonlinear material responses. Doing so would greatly increase computational cost; thus where adding the complexity may be useful, figuring out a cost effective manner to do so will also be of great benefit to the field.
We believe that the crucial findings of the paper suggests that a thorough examination of wall interplay and primary cilium dynamics will be important in understanding the mechanistic properties of the primary cilium.
The authors gratefully acknowledge the support and funding from the National Institutes of Health (1R15GM132829) and the National Science Foundation (1951568)
The authors declare that there is no conflict of interest.
[1] | Robert A. Bloodgood, Chapter 1–from central to rudimentary to primary: The history of an underappreciated organelle whose time has come.the primary cilium, in Methods in Cell Biology, Academic Press. https://doi.org/10.1016/S0091-679X(08)94001-2 |
[2] |
X. Jin, A. M. Mohieldin, B. S. Muntean, J. A. Green, J. V. Shah, K. Mykytyn, et al., Cilioplasm is a cellular compartment for calcium signaling in response to mechanical and chemical stimuli, Cell. Mol. Life Sci., 71 (2014), 2165–2178. https://doi.org/10.1007/s00018-013-1483-1 doi: 10.1007/s00018-013-1483-1
![]() |
[3] |
S. Nag, A. Resnick, Biophysics and biofluid dynamics of primary cilia: Evidence for and against the flow-sensing function, Am. J. Physiol. Renal Physiol., 313 (2017), F706–F720. https://doi.org/10.1152/ajprenal.00172.2017 doi: 10.1152/ajprenal.00172.2017
![]() |
[4] |
N. Praljak, S. D. Ryan, A. Resnick, Pulsatile flow through idealized renal tubules: Fluid-structure interaction and dynamic pathologies, Math. Biosci. Eng., 17 (2019), 1787–1807. https://doi.org/10.3934/mbe.2020094 doi: 10.3934/mbe.2020094
![]() |
[5] | C. Rouiller, 2-General anatomy and histology of the kidney, in The Kidney, Academic Press. https://doi.org/10.1016/B978-1-4832-2825-9.50008-5 |
[6] |
A.K O'Connor, E. B. Malarkey, N. F. Berbari, M. J. Croyle, C. J. Haycraft, P. D. Bell, et al., An inducible CiliaGFP mouse model for in vivo visualization and analysis of cilia in live tissue, Cilia, 2013 (2013), 8. https://doi.org/10.1186/2046-2530-2-8 doi: 10.1186/2046-2530-2-8
![]() |
[7] |
L. M. Satlin, S. Sheng, C. B. Woda, T. R. Kleyman, Epithelial Na+ channels are regulated by flow, Am. J. Physiol. Renal Physiol., 280 (2001), 1010–1018. https://doi.org/10.1152/ajprenal.2001.280.6.F1010 doi: 10.1152/ajprenal.2001.280.6.F1010
![]() |
[8] | H. A. Praetorius, K. R. Spring, The renal cell primary cilium functions as a flow sensor, Curr. Opin. Nephrol. Hypertens., 12 (2003), 517–520. |
[9] |
I. Mnassri, A. El Baroudi, Vibrational frequency analysis of finite elastic tube filled with compressible viscous fluid, Acta Mech. Solida Sin., 30 (2017), 435–444. https://doi.org/10.1016/j.camss.2017.07.010 doi: 10.1016/j.camss.2017.07.010
![]() |
[10] |
O. San, A. E. Staples, Dynamics of pulsatile flows through elastic microtubes, Int. J. Appl. Mech., 4 (2012), 1250006. https://doi.org/10.1142/S175882511200135X doi: 10.1142/S175882511200135X
![]() |
[11] |
M. E. Downs, A. M. Nguyen, F. A. Herzog, D. A. Hoey, C. R. Jacobs, An experimental and computational analysis of primary cilia deflection under fluid flow, Comput. Methods Biomech. Biomed. Eng., 17 (2014), 2–10. https://doi.org/10.1080/10255842.2011.653784 doi: 10.1080/10255842.2011.653784
![]() |
[12] |
H. Khayyeri, S. Barreto, D. Lacroix, Primary cilia mechanics affects cell mechanosensation: A computational study, J. Theor. J., 379 (2015), 38–46. https://doi.org/10.1016/j.jtbi.2015.04.034 doi: 10.1016/j.jtbi.2015.04.034
![]() |
[13] |
S. Sun, R. L. Fisher, S. S. Bowser, B. T. Pentecost, H. Sui, Three-dimensional architecture of epithelial primary cilia, Proc. Natl. Acad. Sci., 116 (2019), 9370–9379. https://doi.org/10.1073/pnas.1821064116 doi: 10.1073/pnas.1821064116
![]() |
[14] |
B. K. Yoder, Role of primary cilia in the pathogenesis of polycystic kidney disease, J. Am. Soc. Nephrol., 18 (2007), 1381–1388. https://doi.org/10.1681/ASN.2006111215 doi: 10.1681/ASN.2006111215
![]() |
[15] |
S. Wang, Z. Dong, Primary cilia and kidney injury: current research status and future perspectives, Am. J. Physiol. Renal Physiol., 305 (2013), F1085–F1098. https://doi.org/10.1152/ajprenal.00399.2013 doi: 10.1152/ajprenal.00399.2013
![]() |
[16] |
Z. Peng, A. Resnick, Y. N. Young, Primary cilium: a paradigm for integrating mathematical modeling with experiments and numerical simulations in mechanobiology, Math. Biosci. Eng., 18 (2021), 1215–1237. https://doi.org/10.3934/mbe.2021066 doi: 10.3934/mbe.2021066
![]() |
[17] |
L. C. Espinha, D. A. Hoey, P. R. Fernandes, H. C. Rodrigues, C. R. Jacobs, Oscillatory fluid flow influences primary cilia and microtubule mechanics, Cytoskeleton, 71 (2014), 435–445. https://doi.org/10.1002/cm.21183 doi: 10.1002/cm.21183
![]() |
[18] |
T. Sakai, D. A. Craig, A. S. Wexler, D. J. Marsh. Fluid waves in renal tubules, Biophys. J., 50 (1986), 805–813. https://doi.org/10.1016/S0006-3495(86)83521-4 doi: 10.1016/S0006-3495(86)83521-4
![]() |
[19] |
N. H. Holstein-Rathlou, D. J. Marsh, Oscillations of tubular pressure, flow, and distal chloride concentration in rats, Am. J. Physiol. Renal Physiol., 256 (1989), F1007–F1014. https://doi.org/10.1152/ajprenal.1989.256.6.F1007 doi: 10.1152/ajprenal.1989.256.6.F1007
![]() |
[20] |
S. Cortell, F. J. Gennari, M. Davidman, W. H. Bossert, W. B. Schwartz, A definition of proximal and distal tubular compliance. practical and theoretical implications, J. Clin. Invest., 52 (1973), 2330–2339. https://doi.org/10.1172/JCI107422 doi: 10.1172/JCI107422
![]() |
[21] | COMSOL Multiphysics. Available from: www.comsol.com. |
[22] |
J. Flaherty, Z. Feng, Z. Peng, Y. N. Young, A. Resnick, Primary cilia have a length-dependent persistence length, Biomech. Model. Mechanobiol., 19 (2020), 445–460. https://doi.org/10.1007/s10237-019-01220-7 doi: 10.1007/s10237-019-01220-7
![]() |
[23] |
S. Rydholm, G. Zwartz, J. M. Kowalewski, P. K. Zare, T. Frisk, H. Brismar, Mechanical properties of primary cilia regulate the response to fluid flow, Am. J. Physiol. Renal Physiol., 298 (2009), F1096–F1102. https://doi.org/10.1152/ajprenal.00657.2009 doi: 10.1152/ajprenal.00657.2009
![]() |
[24] |
P. S. Mathieu, J. C. Bodle, E. G. Loboa, Primary cilium mechanotransduction of tensile strain in 3d culture: Finite element analyses of strain amplification caused by tensile strain applied to a primary cilium embedded in a collagen matrix, J. Biomech., 47 (2014), 2211–2217. https://doi.org/10.1016/j.jbiomech.2014.04.004 doi: 10.1016/j.jbiomech.2014.04.004
![]() |
[25] |
J. Cui, T. Wu, Y. Liu, B. M. Fu, Y. Jin, Z. Zhu, A three-dimensional simulation of the dynamics of primary cilia in an oscillating flow, Appl. Math. Modell., 108 (2022), 825–839. https://doi.org/10.1016/j.apm.2022.04.024 doi: 10.1016/j.apm.2022.04.024
![]() |
[26] |
J. O'Connor, A. Revell, P. Mandal, P. Day, Application of a lattice boltzmann-immersed boundary method for fluid-filament dynamics and flow sensing, J. Biomech., 49 (2016), 2143–2151. https://doi.org/10.1016/j.jbiomech.2015.11.057 doi: 10.1016/j.jbiomech.2015.11.057
![]() |
[27] | COMSOL multiphysics, Fluid structure interaction. Available from: https://www.comsol.com/model/fluid-structure-interaction-361. |
[28] | COMSOL multiphysics, Contact Analysis. Available from: https://doc.comsol.com/5.5/doc/com.comsol.help.sme/sme_ug_theory.06.65.html. |
[29] |
H. P. Peters, C. M. Laarakkers, P. Pickkers, R. Masereeuw, O. C. Boerman, A. Eek, et al., Tubular reabsorption and local production of urine hepcidin-25, BMC Nephrol., 14 (2013), 70. https://doi.org/10.1186/1471-2369-14-70 doi: 10.1186/1471-2369-14-70
![]() |
[30] | J. Howard, R. L. Clark, Mechanics of motor proteins and the cytoskeleton, Appl. Mech. Rev., 55 (2002), B39. |
[31] |
B. Vahidi, N. Fatouraee, A. Imanparast, A. N. Moghadam, A mathematical simulation of the ureter: Effects of the model parameters on ureteral pressure/flow relations, J. Biomech. Eng., 133 (2011), 031004. https://doi.org/10.1115/1.4003316 doi: 10.1115/1.4003316
![]() |
[32] |
M. Pradella, R. M. Dorizzi, F. Rigolin, B. E. Statland, Relative density of urine: methods and clinical significance, Crit. Rev. Clin. Lab. Sci., 26 (1988), 195–242. https://doi.org/10.3109/10408368809105890 doi: 10.3109/10408368809105890
![]() |
[33] | C. Battle, Mechanics & dynamics of the primary cilium, Ph. D thesis, Georg-August-University G''ottingen, 2013. http://dx.doi.org/10.53846/goediss-4037 |
[34] | COMSOL multiphysics, Meshing for Contact Analysis. Available from: https://doc.comsol.com/5.5/doc/com.comsol.help.sme/sme_ug_modeling.05.102.html. |
Parameters (symbol) | Numerical Values | Ref. |
Distance between tubes (d) | 0.1 μm | |
Length of tubes (L) | 50 μm | [18] |
Radius of inner tubes (r) | 10 μm | [20] |
Tube thickness | 1 μm | [20] |
Young's modulus (E) | 1×105 Pa | [18] |
Poisson ratio (u) | 0.3 | |
Tube density (ρt) | 1100 kgm3 | [30] |
Dynamic viscosity (μ) | 5×10−3 Pas | [31] |
Fluid density (ρf) | 1010 kgm3 | [32] |
Time averaged pressure (P0) | 0.1 kPa | [19] |
Length of primary cilia (l) | 6 μm | |
Bendinging rigidity (EI) | 5×10−23 Pa m4 | [33] |
Parameters (symbol) | Numerical Values | Ref. |
Distance between tubes (d) | 0.1 μm | |
Length of tubes (L) | 50 μm | [18] |
Radius of inner tubes (r) | 10 μm | [20] |
Tube thickness | 1 μm | [20] |
Young's modulus (E) | 1×105 Pa | [18] |
Poisson ratio (u) | 0.3 | |
Tube density (ρt) | 1100 kgm3 | [30] |
Dynamic viscosity (μ) | 5×10−3 Pas | [31] |
Fluid density (ρf) | 1010 kgm3 | [32] |
Time averaged pressure (P0) | 0.1 kPa | [19] |
Length of primary cilia (l) | 6 μm | |
Bendinging rigidity (EI) | 5×10−23 Pa m4 | [33] |