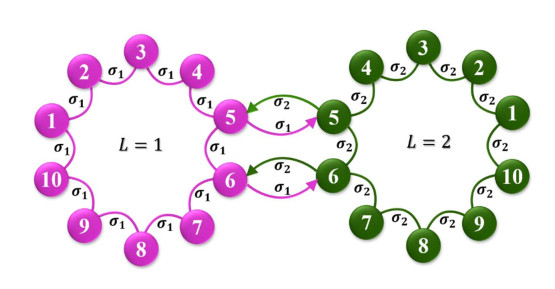
Investigating the effect of changes in neuronal connectivity on the brain's behavior is of interest in neuroscience studies. Complex network theory is one of the most capable tools to study the effects of these changes on collective brain behavior. By using complex networks, the neural structure, function, and dynamics can be analyzed. In this context, various frameworks can be used to mimic neural networks, among which multi-layer networks are a proper one. Compared to single-layer models, multi-layer networks can provide a more realistic model of the brain due to their high complexity and dimensionality. This paper examines the effect of changes in asymmetry coupling on the behaviors of a multi-layer neuronal network. To this aim, a two-layer network is considered as a minimum model of left and right cerebral hemispheres communicated with the corpus callosum. The chaotic model of Hindmarsh-Rose is taken as the dynamics of the nodes. Only two neurons of each layer connect two layers of the network. In this model, it is assumed that the layers have different coupling strengths, so the effect of each coupling change on network behavior can be analyzed. As a result, the projection of the nodes is plotted for several coupling strengths to investigate how the asymmetry coupling influences the network behaviors. It is observed that although no coexisting attractor is present in the Hindmarsh-Rose model, an asymmetry in couplings causes the emergence of different attractors. The bifurcation diagrams of one node of each layer are presented to show the variation of the dynamics due to coupling changes. For further analysis, the network synchronization is investigated by computing intra-layer and inter-layer errors. Calculating these errors shows that the network can be synchronized only for large enough symmetric coupling.
Citation: Sridevi Sriram, Hayder Natiq, Karthikeyan Rajagopal, Ondrej Krejcar, Hamidreza Namazi. Dynamics of a two-layer neuronal network with asymmetry in coupling[J]. Mathematical Biosciences and Engineering, 2023, 20(2): 2908-2919. doi: 10.3934/mbe.2023137
[1] | Guowei Wang, Yan Fu . Spatiotemporal patterns and collective dynamics of bi-layer coupled Izhikevich neural networks with multi-area channels. Mathematical Biosciences and Engineering, 2023, 20(2): 3944-3969. doi: 10.3934/mbe.2023184 |
[2] | Mahtab Mehrabbeik, Fatemeh Parastesh, Janarthanan Ramadoss, Karthikeyan Rajagopal, Hamidreza Namazi, Sajad Jafari . Synchronization and chimera states in the network of electrochemically coupled memristive Rulkov neuron maps. Mathematical Biosciences and Engineering, 2021, 18(6): 9394-9409. doi: 10.3934/mbe.2021462 |
[3] | Manuela Aguiar, Ana Dias, Miriam Manoel . Gradient and Hamiltonian coupled systems on undirected networks. Mathematical Biosciences and Engineering, 2019, 16(5): 4622-4644. doi: 10.3934/mbe.2019232 |
[4] | Hwayeon Ryu, Sue Ann Campbell . Stability, bifurcation and phase-locking of time-delayed excitatory-inhibitory neural networks. Mathematical Biosciences and Engineering, 2020, 17(6): 7931-7957. doi: 10.3934/mbe.2020403 |
[5] | Stefano Fasani, Sergio Rinaldi . Local stabilization and network synchronization: The case of stationary regimes. Mathematical Biosciences and Engineering, 2010, 7(3): 623-639. doi: 10.3934/mbe.2010.7.623 |
[6] | Hai Lin, Jingcheng Wang . Pinning control of complex networks with time-varying inner and outer coupling. Mathematical Biosciences and Engineering, 2021, 18(4): 3435-3447. doi: 10.3934/mbe.2021172 |
[7] | Anna Cattani . FitzHugh-Nagumo equations with generalized diffusive coupling. Mathematical Biosciences and Engineering, 2014, 11(2): 203-215. doi: 10.3934/mbe.2014.11.203 |
[8] | Stefano Cosenza, Paolo Crucitti, Luigi Fortuna, Mattia Frasca, Manuela La Rosa, Cecilia Stagni, Lisa Usai . From Net Topology to Synchronization in HR Neuron Grids. Mathematical Biosciences and Engineering, 2005, 2(1): 53-77. doi: 10.3934/mbe.2005.2.53 |
[9] | Qianqian Zheng, Jianwei Shen, Lingli Zhou, Linan Guan . Turing pattern induced by the directed ER network and delay. Mathematical Biosciences and Engineering, 2022, 19(12): 11854-11867. doi: 10.3934/mbe.2022553 |
[10] | Changgui Gu, Ping Wang, Tongfeng Weng, Huijie Yang, Jos Rohling . Heterogeneity of neuronal properties determines the collective behavior of the neurons in the suprachiasmatic nucleus. Mathematical Biosciences and Engineering, 2019, 16(4): 1893-1913. doi: 10.3934/mbe.2019092 |
Investigating the effect of changes in neuronal connectivity on the brain's behavior is of interest in neuroscience studies. Complex network theory is one of the most capable tools to study the effects of these changes on collective brain behavior. By using complex networks, the neural structure, function, and dynamics can be analyzed. In this context, various frameworks can be used to mimic neural networks, among which multi-layer networks are a proper one. Compared to single-layer models, multi-layer networks can provide a more realistic model of the brain due to their high complexity and dimensionality. This paper examines the effect of changes in asymmetry coupling on the behaviors of a multi-layer neuronal network. To this aim, a two-layer network is considered as a minimum model of left and right cerebral hemispheres communicated with the corpus callosum. The chaotic model of Hindmarsh-Rose is taken as the dynamics of the nodes. Only two neurons of each layer connect two layers of the network. In this model, it is assumed that the layers have different coupling strengths, so the effect of each coupling change on network behavior can be analyzed. As a result, the projection of the nodes is plotted for several coupling strengths to investigate how the asymmetry coupling influences the network behaviors. It is observed that although no coexisting attractor is present in the Hindmarsh-Rose model, an asymmetry in couplings causes the emergence of different attractors. The bifurcation diagrams of one node of each layer are presented to show the variation of the dynamics due to coupling changes. For further analysis, the network synchronization is investigated by computing intra-layer and inter-layer errors. Calculating these errors shows that the network can be synchronized only for large enough symmetric coupling.
Brain performance analysis is one of neuroscience's most critical aspects. Understanding how the brain works and diagnosing its disorders can be improved by studying the neurons' behaviors. In the brain, the activities of neurons and their interactions produce a wide range of collective phenomena such as partial synchronization (i.e., cluster synchronization and chimera states [1,2]), complete synchronization [3], and spiral waves [4,5]. Generally, in the network of oscillations, synchronization can be affected by changes in the system, such as changes in temperature, external stimuli, or coupling strength [6,7,8]. Scientists, however, are interested in maintaining synchronization when network criteria change, for example, its dimension [9,10]. Numerous studies deal with the synchronization of neurons [11,12,13]. This phenomime has been widely studied as it plays a crucial role in brain activities, such as attention, working memory, learning, etc. [14,15]. A network science approach and graph theory can be useful in examining brain behavior. In most mental disorders, abnormalities in neural synchronization have been seen. Patients with schizophrenia are diagnosed with abnormalities in neural synchronization in the gamma range (30–50 Hz) [16], while those with Major Depression are diagnosed with disruptions in spontaneous alpha-band (8–12 Hz) synchrony [17]. Interpersonal neural synchronization is also observed among people when they cooperate. As observed in [18], such synchronization is higher among people who are solving a puzzle in a group (joint attention) rather than completing that puzzle individually (individual attention).
In graph modeling, nodes represent units, and links represent their connections [19]. Brain units can refer to single neural cells, groups of neurons, or cortical brain regions. Also, the connectivity can reflect physical connections between neurons, known as structural connectivity or functional connectivity, which refers to an indirect interaction between different brain regions. Different types of brain imaging can reveal these connections. For instance, the diffusion weighted imaging (DWI) technique indicates structural connectivity in the brain [20], and the functional magnetic resonance imaging (fMRI) technique shows functional connectivity [21].
Due to recent computational advances, many studies have been conducted to analyze the functional and structural characteristics of the brain [22]. In several studies, functional and anatomical asymmetry has been evident in the brain [23]. Different human criteria like gender [24] also contribute to these asymmetry patterns. As an example, anatomical asymmetry in the Sylvian fissure has been detected [25]. It has been shown that men are more likely to display this asymmetry among right-handed people than women, while vice versa is true for left-handers [26]. Besides, it has been shown that the brain exhibits asymmetries in dyslexic children [27], schizophrenic patients [28], and those with Alzheimer's disease [29]. In most cases, these asymmetrical patterns were found between the hemispheres. Other asymmetry effects in dynamical networks have also been examined [30]. Medeiros et al. [31] used asymmetry coupling to show that an ecological network becomes ordered through asymmetric coupling.
Although several studies have represented the brain as a single network [32], developing some powerful theoretical frameworks like higher-order networks [33] and multi-layer networks [34] in recent years has offered a complete representation of the brain. Multi-layer networks can represent each aspect of the brain through a different layer. For instance, in [35], each functional and anatomical connectivity was considered as one layer of a multi-layer network to investigate the brain's multiplex motifs. Hence, recently, more attention has been paid to multi-layer neuronal networks [36]. Some studies have considered the brain as a multiplex network framework in which information of the specific frequency band is encoded in one layer [37]. From another perspective, based on the cerebral cortex data, each brain hemisphere can be seen as one layer of a multi-layer network. For instance, in [38], such a model was chosen to investigate how matching a network's structural and dynamical parameters lead to the formation of chimera states in the brain.
In light of the above studies, here, a two-layer network of neuron models is considered to investigate asymmetric coupling strength effects on the network behavior and neurons' dynamics. This multi-layer model indicates that asymmetry in coupling strength results in an asymmetry in the trajectories of layers. The result is obtained by analyzing the phase space projections of nodes and plotting bifurcation diagrams of a single node of each layer by changing the coupling strength. Furthermore, computing intra-layer and inter-layer errors of the network demonstrates that it can be synchronized with large enough symmetric coupling strengths.
In this paper, the model is described in Section 2, and its obtained results are mentioned in Section 3. In this section, some trajectories of symmetric and asymmetric coupling are plotted to examine the effect of these couplings on the neurons' dynamics. To quantify the synchronizability of the multi-layer network, the error between and within layers is computed. Finally, the conclusion is drawn in Section 4.
Here, a two-layer neuronal network is considered where each layer is a ring of 10 nodes, also known as a double-chain network. This structure is used as a minimal model for the brain hemispheres connected through the corpus callosum. The model schematic is represented in Figure 1. As the node's dynamics, the Hindmarsh-Rose (HR) model is utilized. Here, the mathematical form of this model has been described in Eq (1).
{˙vL,m=uL,m+3×v2L,m−v3L,m−wL,m+I+C˙uL,m= |
1−5×v2L,m−uL,m˙wL,m=−r×wL,m+r×s×(1.6+vL,m) | (1) |
In this model, vL,m represents the axon membrane potential of the m-th neuron in the layer L, where L=1,2 and m=1,2,…,10. Also uL,m and wL,m are the spiking and bursting variables of that node, respectively. Here C=cw+cb represents two types of coupling; within layers (cw) and between layers (cb), which are defined by Eq (2) [31]. The coupling is considered as:
cw=(vL,(m−1)+vL,(m+1)−2×vL,k)×σL | (2-a) |
cb=(σL′×vL′,m′−σL×vL,m)×Amm′ | (2-b) |
In Eq (2), σ is the coupling strength or synaptic strength between neurons where subscripts L and L′ define the layer L and the opposite one, respectively. The adjacency matrix A indicates the connections between the nodes of each layer. Hence, in our model Amm′=1 for m,m′={5,6}.
Here, the effects of asymmetric coupling on network dynamics are analyzed. As the couplings are alternated, the neurons' behaviors in each layer are examined. First, one coupling strength remains constant, and the network dynamics are analyzed in phase space based on variations of the other coupling strength. Next, by choosing one coupling as a bifurcation parameter, bifurcation diagrams of one node in each layer are plotted to see the variation of the network dynamics due to asymmetric coupling. In the end, intra-layer and inter-layer synchronization errors are calculated to investigate the effects of asymmetric coupling.
Using r=0.006, s=4, and I=3.2 in Eq (1), each neuron results in chaotic oscillations when C=0, which means there is no coupling among nodes. Notice that HR does not have any coexisting attractor. Nevertheless, as the coupling asymmetry increases, different attractors emerge. To show this phenomenon, some projections of nodes are illustrated in Figure 2 for σ1=10 and variable σ2, whereas those of σ2=10 with variable σ1 are shown in Figure 3. Plotting the phase spaces of nodes allows us to analyze how asymmetry in coupling strength affects neurons' dynamics. As can be seen in both figures, the trajectories are the same under equal coupling strengths. As the couplings become more asymmetric, the trajectories of each layer become different. Increasing the asymmetries between coupling can change the dynamics of the layers and lead to periodic oscillations or even quiescent.
As variations in coupling strength result in different attractors in the layers, the bifurcation diagrams of nodes are plotted as a function of σ1 for both layers. Figure 4 illustrates the maximum potential of the first node of each layer by varying σ1 for some fixed σ2. The pink diagrams illustrate the maximum voltage of the first node of layer L=1, and the green diagrams illustrate the voltage of the first node of layer L=2. It can be seen that changing the coupling strength alters the network dynamics. As shown in Figure 4(a) (for fixed σ2=0.1), when σ1 increases and more asymmetry occurs in the coupling, the dynamics of the layer L=1 changes and finally become periodic. The periodic region emerges for σ1>1.79. Moreover, the maximum amplitude of the neurons of layer L=1 decreases. In this case, the dynamics of the second layer are not changed significantly. Figure 4(b), (c) depict results for larger σ2, σ2=1, and σ2=5, respectively. As it can be seen, for larger σ2, the variation in the dynamics of the second layer is more remarkable. Similar to Figure 4(a), for σ2=1, the maximum amplitude of the neurons of layer L=1 is decreased by increasing σ1. Although in most couplings, the behavior is chaotic, in Figure 4(c), a periodic window can be seen for 7.58<σ1<8.18 for the first neuron of both layers. Figure 4(d) shows the bifurcations for σ2=10. Here, more periodic windows can be seen for both layers. The biggest ones in L=1 and L=2 are 6.56<σ1<8.02 and 6.67<σ1<8.02, respectively. Maximum amplitude values of the first node in layer L=1 do not seem to change drastically; however, the one in layer L=2 increases at first and then remains constant.
Network behaviors can also be better understood by evaluating synchronization, which is obtained by computing intra-layer (Err1 and Err2) and inter-layer (Err) errors through Eq (3).
Err1=⟨1N−1∑Ni=2√(X1,1−X1,i)2⟩t,∀X∈{v,u,w} | (3-a) |
Err2=⟨1N−1∑Ni=2√(X2,1−X2,i)2⟩t,∀X∈{v,u,w} | (3-b) |
Err=⟨1N∑Ni=2√(X2,i−X1,i)2⟩t,∀X∈{v,u,w} | (3-c) |
As shown in Figure 5, each error is calculated in the σ1−σ2 plane and color bars indicate the error values. As a result of choosing large σ2 and small σ1 (e.g., σ1=1.1 and σ2=12), the intra-layer error of layer L=1 is significantly larger compared to the opposite choices. In the case of Err2, the result is completely opposite. Both intra-layer errors tend to be zero as asymmetry fades. The minimum error can be obtained when σ1=σ2. The result is the same for interlayer error, although in this case, any asymmetry in coupling enlarges the error.
Also, Figure 6 shows errors as a function of σ1 for some fixed σ2. When σ2 is chosen as σ1≤1, errors cannot be precisely equal to zero in the symmetry case (σ1=σ2). However, the minimum Err occurs at those points. In Figure 6(a), more asymmetry reduces Err1 while a similar pattern could not be observed in Err2. Based on its bifurcation diagram in Figure 4(a), it appears that in σ1=0.1 and σ2>1.79, neurons oscillate periodically in L=1 and chaotically in L=2. Hence, there is a reduction in Err1 that reflects the ordered dynamics in the layer. Figure 6-b shows that the error trend tends to be the same, whereas in this case, when Err1 reduces, Err2 increases, and as a result, Err increases. When σ2 is set to larger values as in Figure 6(c), (d), the minimum errors are found for the symmetric coupling. Furthermore, compared to the corresponding bifurcations (Figure 4(c), (d)), for those couplings that place the neuron within the periodic window, the errors are somewhat reduced (which can be seen for σ2=5).
Asymmetry is an essential phenomenon that participates in different brain performances. In this study, we considered a multi-layer network with two layers, each one as a mini model of one brain hemisphere. This viewpoint examined the effect of asymmetry in coupling strength, which led to asymmetry in the trajectories. The HR model was used as the dynamics of each node. For each layer, a different coupling was used for connecting its nodes. Then, the dynamics of the multi-layer network were examined in the phase space. Despite the absence of coexisting attractors in the HR model, asymmetry in couplings led to various attractors in the network. For better analyses, the bifurcation diagrams of the first node of each layer were plotted as a function of one coupling strength while the other one was chosen fixed. Moreover, intra-layer and inter-layer errors of neurons were calculated to investigate the synchronization of the network. Errors were almost equal to zero for symmetric couplings that were large enough.
This work is partially funded by Centre for Nonlinear Systems, Chennai Institute of Technology, India vide funding number CIT/CNS/2022/RD/006. The work and the contribution were also supported by the SPEV project, University of Hradec Kralove, Faculty of Informatics and Management, Czech Republic (ID: 2102–2022), "Smart Solutions in Ubiquitous Computing Environments". We are also grateful for the support of student Michal Dobrovolny in consultations regarding application aspects.
The authors declare that there is no conflict of interest.
The MATLAB codes used for simulations can be found from: https://drive.google.com/file/d/1AtbEvrTsJm2CTWSNp272WnasIe85ipk5/view?usp=share_link.
[1] |
F. Parastesh, S. Jafari, H. Azarnoush, Z. Shahriari, Z. Wang, S. Boccaletti, et al., Chimeras, Phys. Rep., 898 (2021), 1–114. https://doi.org/10.1016/j.physrep.2020.10.003 doi: 10.1016/j.physrep.2020.10.003
![]() |
[2] |
S. Majhi, B. K. Bera, D. Ghosh, M. Perc, Chimera states in neuronal networks: A review, Phys. Life Rev., 28 (2019), 100–121. https://doi.org/10.1016/j.plrev.2018.09.003 doi: 10.1016/j.plrev.2018.09.003
![]() |
[3] |
F. Parastesh, M. Mehrabbeik, K. Rajagopal, S. Jafari, M. Perc, Synchronization in Hindmarsh-Rose neurons subject to higher-order interactions, Chaos, 32 (2022), 013125. https://doi.org/10.1063/5.0079834 doi: 10.1063/5.0079834
![]() |
[4] |
K. Rajagopal, S. He, A. Karthikeyan, P. Duraisamy, Size matters: Effects of the size of heterogeneity on the wave re-entry and spiral wave formation in an excitable media, Chaos, 31 (2021), 053131. https://doi.org/10.1063/5.0051010 doi: 10.1063/5.0051010
![]() |
[5] |
Z. Wang, Z. Rostami, S. Jafari, F. E. Alsaadi, M. Slavinec, M. Perc, Suppression of spiral wave turbulence by means of periodic plane waves in two-layer excitable media, Chaos Solitons Fractals, 128 (2019), 229–233. https://doi.org/10.1016/j.chaos.2019.07.045 doi: 10.1016/j.chaos.2019.07.045
![]() |
[6] |
Z. Yao, C. Wang, P. Zhou, J. Ma, Regulating synchronous patterns in neurons and networks via field coupling, Commun. Nonlinear Sci. Numer. Simul., 95 (2021), 105583. https://doi.org/10.1016/j.cnsns.2020.105583 doi: 10.1016/j.cnsns.2020.105583
![]() |
[7] |
Z. Yao, C. Wang, Control the collective behaviors in a functional neural network, Chaos Solitons Fractals, 152 (2021), 111361. https://doi.org/10.1016/j.chaos.2021.111361 doi: 10.1016/j.chaos.2021.111361
![]() |
[8] |
P. Zhou, X. Zhang, X. Hu, G. Ren, Energy balance between two thermosensitive circuits under field coupling, Nonlinear Dyn., 110 (2022), 1879–1895. https://doi.org/10.1007/s11071-022-07669-z doi: 10.1007/s11071-022-07669-z
![]() |
[9] |
N. Naseri, F. Parastesh, F. Ghassemi, S. Jafari, E. Schö ll, J. Kurths, Converting high dimensional complex networks to lower dimensional ones preserving synchronization features, Eur. Lett., 140 (2022), 21001. https://doi.org/10.1209/0295-5075/ac98de doi: 10.1209/0295-5075/ac98de
![]() |
[10] |
V. Thibeault, G. St-Onge, L. J. Dubé, P. Desrosiers, Threefold way to the dimension reduction of dynamics on networks: An application to synchronization, Phys. Rev. Res., 2 (2020), 043215. https://doi.org/10.1103/PhysRevResearch.2.043215 doi: 10.1103/PhysRevResearch.2.043215
![]() |
[11] |
Q. Xu, T. Liu, S. Ding, H. Bao, Z. Li, B. Chen, Extreme multistability and phase synchronization in a heterogeneous bi-neuron Rulkov network with memristive electromagnetic induction, Cogn. Neurodyn., 2022 (2022), 1–12. https://doi.org/10.1007/s11571-022-09866-3 doi: 10.1007/s11571-022-09866-3
![]() |
[12] |
Q. Xu, X. Tan, D. Zhu, M. Chen, J. Zhou, H. Wu, Synchronous behavior for memristive synapse-connected Chay twin-neuron network and hardware implementation, Math. Probl. Eng., 2020 (2022), 8218740. https://doi.org/10.1155/2020/8218740 doi: 10.1155/2020/8218740
![]() |
[13] |
S. Rakshit, S. Majhi, J. Kurths, D. Ghosh, Neuronal synchronization in long-range time-varying networks, Chaos, 31 (2021), 073129. https://doi.org/10.1063/5.0057276 doi: 10.1063/5.0057276
![]() |
[14] |
K. Clark, R. F. Squire, Y. Merrikhi, B. Noudoost, Visual attention: Linking prefrontal sources to neuronal and behavioral correlates, Prog. Neurobiol., 132 (2015), 59–80. https://doi.org/10.1016/j.pneurobio.2015.06.006 doi: 10.1016/j.pneurobio.2015.06.006
![]() |
[15] |
Z. Bahmani, K. Clark, Y. Merrikhi, A. Mueller, W. Pettine, M. I. Vanegas, et al., Prefrontal contributions to attention and working memory, Curr. Top Behav. Neurosci., 41 (2019), 129–153. https://doi.org/10.1007/7854_2018_74 doi: 10.1007/7854_2018_74
![]() |
[16] |
J. S. Kwon, B. F. O'Donnell, G. V. Wallenstein, R. W. Greene, Y. Hirayasu, P. G. Nestor, et al., Gamma frequency-range abnormalities to auditory stimulation in schizophrenia, Arch. Gen. Psychiatry, 56 (1999), 1001–1005. https://doi.org/10.1001/archpsyc.56.11.1001 doi: 10.1001/archpsyc.56.11.1001
![]() |
[17] |
G. G. Knyazev, J. Y. Slobodskoj-Plusnin, A. V. Bocharov, L. V. Pylkova, The default mode network and EEG alpha oscillations: An independent component analysis, Brain Res., 1402 (2011), 67–79. https://doi.org/10.1016/j.brainres.2011.05.052 doi: 10.1016/j.brainres.2011.05.052
![]() |
[18] |
F. A. Fishburn, V. P. Murty, C. O. Hlutkowsky, C. E. MacGillivray, L. M. Bemis, M. E. Murphy, et al., Putting our heads together: interpersonal neural synchronization as a biological mechanism for shared intentionality, Soc. Cogn. Affect. Neurosci., 13 (2018), 841–849. https://doi.org/10.1093/scan/nsy060 doi: 10.1093/scan/nsy060
![]() |
[19] |
E. Bullmore, O. Sporns, Complex brain networks: Graph theoretical analysis of structural and functional systems, Nat. Rev. Neurosci., 10 (2009), 186–198. https://doi.org/10.1038/nrn2575 doi: 10.1038/nrn2575
![]() |
[20] |
P. Hagmann, L. Cammoun, X. Gigandet, R. Meuli, C. J. Honey, V. J. Wedeen, et al., Mapping the structural core of human cerebral cortex, PLoS Biol., 6 (2008), e159. https://doi.org/10.1371/journal.pbio.0060159 doi: 10.1371/journal.pbio.0060159
![]() |
[21] |
B. P. Rogers, V. L. Morgan, A. T. Newton, J. C. Gore, Assessing functional connectivity in the human brain by fMRI, Magn. Reson. Imaging, 25 (2007), 1347–1357. https://doi.org/10.1016/j.mri.2007.03.007 doi: 10.1016/j.mri.2007.03.007
![]() |
[22] |
M. Gosak, M. Milojević, M. Duh, K. Skok, M. Perc, Networks behind the morphology and structural design of living systems, Phys. Life Rev., 41 (2022), 1–21. https://doi.org/10.1016/j.plrev.2022.03.001 doi: 10.1016/j.plrev.2022.03.001
![]() |
[23] |
S. D. Glick, D. A. Ross, L. B. Hough, Lateral asymmetry of neurotransmitters in human brain, Brain Res., 234 (1982), 53–63. https://doi.org/10.1016/0006-8993(82)90472-3 doi: 10.1016/0006-8993(82)90472-3
![]() |
[24] |
L. Tian, J. Wang, C. Yan, Y. He, Hemisphere-and gender-related differences in small-world brain networks: a resting-state functional MRI study, Neuroimage, 54 (2011), 191–202. https://doi.org/10.1016/j.neuroimage.2010.07.066 doi: 10.1016/j.neuroimage.2010.07.066
![]() |
[25] |
S. F. Witelson, D. L. Kigar, Sylvian fissure morphology and asymmetry in men and women: bilateral differences in relation to handedness in men, J. Comp. Neurol., 323 (1992), 326–340. https://doi.org/10.1002/cne.903230303 doi: 10.1002/cne.903230303
![]() |
[26] | L. Jäncke, G. Schlaug, Y. Huang, H. Steinmetz, Asymmetry of the planum parietale, Neuroreport, 5 (1994), 1161–1163. https://psycnet.apa.org/doi/10.1097/00001756-199405000-00035 |
[27] |
G. W. Hynd, M. Semrud-Clikeman, A. R. Lorys, E. S. Novey, D. Eliopulos, Brain morphology in developmental dyslexia and attention deficit disorder/hyperactivity, Arch. Neurol., 47 (1990), 919–926. https://doi.org/10.1001/archneur.1990.00530080107018 doi: 10.1001/archneur.1990.00530080107018
![]() |
[28] |
R. M. Bilder, H. Wu, B. Bogerts, M. Ashtari, D. Robinson, M. Woerner, et al., Cerebral volume asymmetries in schizophrenia and mood disorders: A quantitative magnetic resonance imaging study, Int. J. Psychophysiol., 34 (1999), 197–205. https://doi.org/10.1016/S0167-8760(99)00077-X doi: 10.1016/S0167-8760(99)00077-X
![]() |
[29] |
P. M. Thompson, J. Moussai, S. Zohoori, A. Goldkorn, A. A. Khan, M. S. Mega, et al., Cortical variability and asymmetry in normal aging and Alzheimer's disease, Cereb. Cortex, 8 (1998), 492–509. https://doi.org/10.1093/cercor/8.6.492 doi: 10.1093/cercor/8.6.492
![]() |
[30] |
S. Rakshit, F. Parastesh, S. N. Chowdhury, S. Jafari, J. Kurths, D. Ghosh, Relay interlayer synchronisation: invariance and stability conditions, Nonlinearity, 35 (2021), 681. https://doi.org/10.1088/1361-6544/ac3c2f doi: 10.1088/1361-6544/ac3c2f
![]() |
[31] |
E. S. Medeiros, U. Feudel, A. Zakharova, Asymmetry-induced order in multilayer networks, Phys. Rev. E, 104 (2021), 024302. https://doi.org/10.1103/PhysRevE.104.024302 doi: 10.1103/PhysRevE.104.024302
![]() |
[32] |
B. M. Tijms, A. M. Wink, W. de Haan, W. M. van der Flier, C. J. Stam, P. Scheltens, et al., Alzheimer's disease: Connecting findings from graph theoretical studies of brain networks, Neurobiol. Aging, 34 (2013), 2023–2036. https://doi.org/10.1016/j.neurobiolaging.2013.02.020 doi: 10.1016/j.neurobiolaging.2013.02.020
![]() |
[33] |
S. Majhi, M. Perc, D. Ghosh, Dynamics on higher-order networks: A review, J. R. Soc. Interface, 19 (2022), 20220043. https://doi.org/10.1098/rsif.2022.0043 doi: 10.1098/rsif.2022.0043
![]() |
[34] |
M. De Domenico, A. Solé-Ribalta, E. Cozzo, M. Kivelä, Y. Moreno, M. A. Porter, et al., Mathematical formulation of multilayer networks, Phys. Rev. X, 3 (2013), 041022. https://doi.org/10.1103/PhysRevX.3.041022 doi: 10.1103/PhysRevX.3.041022
![]() |
[35] |
F. Battiston, V. Nicosia, M. Chavez, V. Latora, Multilayer motif analysis of brain networks, Chaos, 27 (2017), 047404. https://doi.org/10.1063/1.4979282 doi: 10.1063/1.4979282
![]() |
[36] |
A. Karthikeyan, I. Moroz, K. Rajagopal, P. Duraisamy, Effect of temperature sensitive ion channels on the single and multilayer network behavior of an excitable media with electromagnetic induction, Chaos Solitons Fractals, 150 (2021), 111144. https://doi.org/10.1016/j.chaos.2021.111144 doi: 10.1016/j.chaos.2021.111144
![]() |
[37] |
M. Yu, M. M. A. Engels, A. Hillebrand, E. C. W. Van Straaten, A. A. Gouw, C. Teunissen, et al., Selective impairment of hippocampus and posterior hub areas in Alzheimer's disease: An MEG-based multiplex network study, Brain, 140 (2017), 1466–1485. https://doi.org/10.1093/brain/awx050 doi: 10.1093/brain/awx050
![]() |
[38] |
L. Kang, C. Tian, S. Huo, Z. Liu, A two-layered brain network model and its chimera state, Sci. Rep., 9 (2019), 14389. https://doi.org/10.1038/s41598-019-50969-5 doi: 10.1038/s41598-019-50969-5
![]() |
1. | Sebastian Ocklenburg, Zengcai V. Guo, Cross-hemispheric communication: Insights on lateralized brain functions, 2024, 112, 08966273, 1222, 10.1016/j.neuron.2024.02.010 |