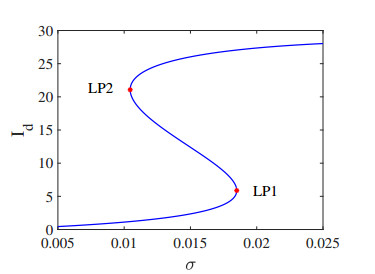
Testing-culling is a very effective measure for the prevention and control of animal diseases. In this paper, based on sheep brucellosis control policies and animal testing characteristics and considering the limitation of culling resources, a dynamic model is established to study the impact of testing-culling measure. Theoretical analysis reveals that the model may have one or three positive equilibria. The equilibrium in the middle is always unstable, and the model shows saddle-node bifurcation, generalized Hopf bifurcation and Bogdanov-Takens bifurcation. Moreover, the theoretical results are verified via numerical analysis. These results reveal that testing and culling strategies can induce complex transmission dynamics that can help us develop appropriate prevention and control measures for animal brucellosis.
Citation: Yongbing Nie, Xiangdong Sun, Hongping Hu, Qiang Hou. Bifurcation analysis of a sheep brucellosis model with testing and saturated culling rate[J]. Mathematical Biosciences and Engineering, 2023, 20(1): 1519-1537. doi: 10.3934/mbe.2023069
[1] | Linhua Zhou, Meng Fan, Qiang Hou, Zhen Jin, Xiangdong Sun . Transmission dynamics and optimal control of brucellosis in Inner Mongolia of China. Mathematical Biosciences and Engineering, 2018, 15(2): 543-567. doi: 10.3934/mbe.2018025 |
[2] | Mingtao Li, Xin Pei, Juan Zhang, Li Li . Asymptotic analysis of endemic equilibrium to a brucellosis model. Mathematical Biosciences and Engineering, 2019, 16(5): 5836-5850. doi: 10.3934/mbe.2019291 |
[3] | Mingtao Li, Guiquan Sun, Juan Zhang, Zhen Jin, Xiangdong Sun, Youming Wang, Baoxu Huang, Yaohui Zheng . Transmission dynamics and control for a brucellosis model in Hinggan League of Inner Mongolia, China. Mathematical Biosciences and Engineering, 2014, 11(5): 1115-1137. doi: 10.3934/mbe.2014.11.1115 |
[4] | Qiang Hou, Haiyan Qin . Global dynamics of a multi-stage brucellosis model with distributed delays and indirect transmission. Mathematical Biosciences and Engineering, 2019, 16(4): 3111-3129. doi: 10.3934/mbe.2019154 |
[5] | Yaoyao Qin, Xin Pei, Mingtao Li, Yuzhen Chai . Transmission dynamics of brucellosis with patch model: Shanxi and Hebei Provinces as cases. Mathematical Biosciences and Engineering, 2022, 19(6): 6396-6414. doi: 10.3934/mbe.2022300 |
[6] | Yoichi Enatsu, Yukihiko Nakata . Stability and bifurcation analysis of epidemic models with saturated incidence rates: An application to a nonmonotone incidence rate. Mathematical Biosciences and Engineering, 2014, 11(4): 785-805. doi: 10.3934/mbe.2014.11.785 |
[7] | Fang Zhang, Wenzhe Cui, Yanfei Dai, Yulin Zhao . Bifurcations of an SIRS epidemic model with a general saturated incidence rate. Mathematical Biosciences and Engineering, 2022, 19(11): 10710-10730. doi: 10.3934/mbe.2022501 |
[8] | Zongmin Yue, Yuanhua Mu, Kekui Yu . Dynamic analysis of sheep Brucellosis model with environmental infection pathways. Mathematical Biosciences and Engineering, 2023, 20(7): 11688-11712. doi: 10.3934/mbe.2023520 |
[9] | Changyong Xu, Qiang Li, Tonghua Zhang, Sanling Yuan . Stability and Hopf bifurcation for a delayed diffusive competition model with saturation effect. Mathematical Biosciences and Engineering, 2020, 17(6): 8037-8051. doi: 10.3934/mbe.2020407 |
[10] | Shilian Xu . Saturated lysing efficiency of CD8+ cells induced monostable, bistable and oscillatory HIV kinetics. Mathematical Biosciences and Engineering, 2024, 21(10): 7373-7393. doi: 10.3934/mbe.2024324 |
Testing-culling is a very effective measure for the prevention and control of animal diseases. In this paper, based on sheep brucellosis control policies and animal testing characteristics and considering the limitation of culling resources, a dynamic model is established to study the impact of testing-culling measure. Theoretical analysis reveals that the model may have one or three positive equilibria. The equilibrium in the middle is always unstable, and the model shows saddle-node bifurcation, generalized Hopf bifurcation and Bogdanov-Takens bifurcation. Moreover, the theoretical results are verified via numerical analysis. These results reveal that testing and culling strategies can induce complex transmission dynamics that can help us develop appropriate prevention and control measures for animal brucellosis.
Brucella is a gram-negative, non-motile coccobacillus that can parasitize various types of livestock and cause brucellosis [1]. Brucellosis can be transmitted to susceptible humans and animals mainly through contact with infected animals or ingestion of pathogens from the environment. Brucellosis can cause miscarriage and orchitis in animals and fever, fatigue, joint pain and other symptoms in humans [2]. Brucellosis is found all over the world, and nearly 500,000 new cases are recorded every year [3]. The brucellosis epidemic not only endangers human health but also affects animal husbandry, which has a large adverse effect on economic development and public health [4].
For the prevention and control of animal brucellosis, vaccination, disinfection and testing-culling are the main measures. Many authors have studied the impact of vaccination on the spread of animal brucellosis, and quantitative results have been obtained [5,6]. However, a 100% vaccination rate of animals is difficult to achieve, and some vaccines have negative effects. For example, in Korea, vaccination of cattle with RB51 caused side-effects, including abortion, premature birth and a reduced milk yield [7]. In other words, brucellosis cannot be eradicated only by vaccination, and other measures should be taken to control animal brucellosis. Although testing and culling measures are difficult to implement in some developing countries because of the high economic cost, they can eliminate animal diseases. For example, bovine brucellosis in New Zealand caused heavy losses to the livestock industry and was eventually eliminated through testing and culling measures [8]. Testing and culling measures have been used to prevent and control brucellosis and have significantly reduced the spread of this disease in most Southeast Asian countries [9]. Therefore, testing and culling measures are necessary for brucellosis eradication.
Recent studies on the impact of testing and culling measures have contributed to the understanding of the effectiveness of brucellosis control measures [10,11,12,13,14]. However, these studies mainly focus on the impact of culling measures, and the impact of testing principles and processes on animal brucellosis is still not well researched, which is not conducive to the understanding of the impact of testing behavior and leads to neglect of this risk factor in mathematical modelling. In practice, if an infected animal is found in one place, then all animals in that place will be tested. For example, in Inner Mongolia, China, sheep are numerous, and if an infected sheep is found in one place (a township is treated as a unit), all sheep are tested. Importantly, because of the larger number of sheep, in general, a certain proportion of animals are tested per unit time. In addition, culling resources derived from government supplies may be limited because of the many positive animals. On the basis of these two risk factors and ignoring the contagion of environmental pathogens, a sheep brucellosis model is established:
{dSdt=A−βSI−μS,dIdt=βSI−(σ+μ)I−mϕIId,dIddt=mϕIId+σI−μId−cIdα+Id, | (1.1) |
where S(t) represents the susceptible population, I(t) is the infectious population and Id(t) is the infectious population with clinical features or found by serological testing. Let A be the constant recruitment rate. β and μ are the infection rate and the natural death rate, respectively. σ is the rate of infected animals with clinical features. m is the fraction of positive animals found by testing. ϕ is defined as the testing rate, the number of animals tested is ϕN per unit of infectious animals Id(t), thus the number of infected animals that have been found through testing is mϕNINId=mϕIId. c represents the maximal supply of culling resources and α is half-saturation constant, measuring the efficiency of the supply of culling resources. The parameters of the model are positive constants.
The motivation for this article is to investigate the impact of restricted culling resources on the dynamics of brucellosis transmission. The rest of this paper is arranged as follows. The basic properties of the model are given in Section 2. In Section 3, the stability of equilibria, saddle-node bifurcation, Hopf bifurcation and Bogdanov-Takens bifurcation of codimension 2 are analyzed. In Section 4, the theoretical results are verified by numerical simulation. Finally, conclusions and discussions are given in Section 5.
For model (1.1), one can find that
d(S+I+Id)dt=A−μS−μI−μId−cIdα+Id≤A−μ(S+I+Id), |
it follows that
limt→∞sup(S+I+Id)≤Aμ. |
So the set
Ω={(S,I,Id)∈R3:S,I,Id≥0,S+I+Id≤Aμ} |
is the positively invariant set of model (1.1).
It is easy to verify that model (1.1) has a disease-free equilibrium E0=(Aμ,0,0). According to the next generation matrix method [15], the basic reproduction number is given by
R0=βAμ(σ+μ). |
For the existence of the endemic equilibrium E(S∗,I∗,I∗d) of model (1.1), the following equations are given:
{A=βSI+μS,βSI=(μ+σ)I+mϕIdI,mϕIdI+σI=(μ+cα+Id)Id. | (2.1) |
According to the above equations, S∗ and I∗ can be expressed as functions of I∗d. That is,
S∗=μ+σ+mϕI∗dβ,I∗=−mϕμI∗d+μ(μ+σ)(R0−1)β(mϕI∗d+μ+σ), |
where I∗d is given by the equation
f(I∗d)=B0I∗3d+B1I∗2d+B2I∗d+B3=0, | (2.2) |
where
B0=−mμϕ(mϕ+β)<0,B1=μmϕ(μ+σ)(R0−1)−μ(αmϕ+σ)(mϕ+β)−βμ2−βcmϕ,B2=μ(μ+σ)(αmϕ+σ)(R0−1)−β(μ+σ)(αμ+c)−αmμϕσ,B3=ασμ(μ+σ)(R0−1). |
Note that I∗>0 and R0>1, then I∗d<I∗c=(μ+σ)(R0−1)mϕ.
Let
B=B21−3B0B2,C=B1B2−9B0B3,D=B22−3B1B3,Δ=C2−4BD. |
Based on the Descarte's rule of signs, the following cases can be obtained:
Case 1: B2>0 or B1<0,B2<0. There is a unique positive root of f(I∗d)=0.
Case 2: B1>0,B2<0. Similar to the method in [16], the following results can be obtained:
(a) Δ>0, there is a unique positive root of f(I∗d)=0;
(b) Δ=0, there are two positive roots of f(I∗d)=0;
(c) Δ<0, there are three positive roots of f(I∗d)=0.
To sum up the above results, the following conclusions are derived.
Theorem 1. Assumed that R0>1. If B2>0 or B1<0,B2<0 or B1>0,B2<0,Δ>0, model (1.1) has a unique endemic equilibrium E1; if B1>0,B2<0,Δ=0, model (1.1) has two endemic equilibria E1 and E∗, E∗ is an endemic equilibrium of multiplicity 2; if B1>0,B2<0,Δ<0, model (1.1) has three endemic equilibria E1,E2 and E3.
In this section, the stability of equilibria and the local bifurcation of model (1.1) are analyzed, it is of great significance for the prevention and control of brucellosis.
In this subsection, the global stability of disease-free equilibrium and the local stability of endemic equilibria are analyzed. The following results are first given:
Theorem 2. For model (1.1), if R0≤1, then E0 is globally asymptotically stable.
Proof. The Jacobian matrix of model (1.1) at E0 takes the form
JE0=(−μ−βAμ00βAμ−μ−σ00σ−μ−cα), |
then JE0 has eigenvalues λ1=−μ,λ2=−(μ+cα) and λ3=(R0−1)(σ+μ). Therefore, E0 is locally asymptotically stable if R0<1 and E0 is unstable if R0>1.
Next, when R0≤1, define the Lyapunov function L as follows:
L=S−S0−S0lnSS0+I. |
It can be concluded that
˙L=(1−S0S)dSdt+dIdt=(1−S0S)(A−βSI−μS)+βSI−(μ+σ)I−mϕIId≤μS0(2−S0S−SS0)+(R0−1)(μ+σ)I. |
Thus, ˙L≤0. Note that the equality ˙L=0 means S=S0 and I=0, it implies that E0 is the maximum invariant set of model (1.1) in the set {˙L=0}. Therefore, E0 is globally asymptotically stable by LaSalle's invariance principle.
The Jacobian matrix at any endemic equilibrium E(S∗,I∗,I∗d) is given by
JE=(ab0d0f0hl), | (3.1) |
where
a=−AS∗,b=−βS∗,d=βI∗,f=−mϕI∗,h=βS∗−μ,l=A−μS∗−μI∗−μI∗dα+I∗d−σI∗I∗d. |
The characteristic equation can be written as
λ3+α1λ2+α2λ+α3=0, | (3.2) |
where
α1=1(α+I∗d)S∗I∗d((A+μS∗)I∗2d+(μS∗2+(μI∗+σI∗−A)S∗+αA)I∗d+ασS∗I∗),α2=1(α+I∗d)S∗I∗d((S∗(β(mϕ+β)S∗−mμϕ)I∗+μA)I∗2d+((βα(mϕ+β)S∗2−mμϕαS∗+A(σ+μ))I∗+μS∗A−A2)I∗d+ασAI∗),α3=1(α+I∗d)S∗I∗d((βmϕAS∗+β2μS∗2−mμϕA)I∗2d+(−S∗2(−μI∗−σI∗−μS∗+A)β2+mϕαβAS∗−mμϕαA)I∗d+ασβ2I∗S∗2)I∗. |
From Eq (2.2), we can represent the coefficient α3 as a function on I∗d as follows:
α3(I∗d)=−β(mϕI∗d+μ+σ)I∗f′(I∗d). | (3.3) |
It follows from Eq (2.2) that f′(I∗d1)<0,f′(I∗d3)<0 and f′(I∗d2)>0 when E1,E2 and E3 exist. That is, α3(I∗d1)>0,α3(I∗d3)>0 and α3(I∗d2)<0. Thus E2 is unstable.
Let Δ(I∗d)=α1(I∗d)α2(I∗d)−α3(I∗d), the following results are derived.
Theorem 3. For model (1.1), the equilibrium E2 is unstable when it exists. If α1>0,Δ(I∗d)>0, the equilibria E1 and E3 is locally asymptotically stable when they exist.
Remark. If there is no serological test, the third equation of model (1.1) is independent of the other equations, and a general SI model can be obtained that has a disease-free equilibrium and an endemic equilibrium, both of which are globally asymptotically stable.
In this subsection, the conditions for the occurrence of saddle-node, Hopf and Bogdanov-Takens bifurcations are given, which are necessary to understand the impact of testing-culling.
Based on the Theorem 1, it is easy to verify that there are two endemic equilibria when Δ=0, which implies that the characteristic Eq (3.2) has a simple zero eigenvalue at E∗. That is, model (1.1) may undergo a saddle-node bifurcation. In the following pages, a detailed analysis is given by choosing σ as the bifurcation parameter.
Let Fσ be the derivative of F with respect to σ, where F=(F1,F2,F3)T is shown as follows:
{F1≜A−βSI−μS,F2≜βSI−(σ+μ)I−mϕIId,F3≜mϕIId+σI−μId−cIdα+Id. |
Let V and W be the eigenvectors corresponding to the eigenvalue λ=0 for JE∗ and JTE∗. Then they can be given by
V=(V1V2V3)=(−blal−h),W=(W1W2W3)=(−dlal−f), |
where a,b,d,f,h,l are given in (3.1). Furthermore, one can get
Fσ(E∗;σ∗)=(0−I∗I∗) |
and
D2F(E∗;σ∗)(V,V)=(−2βV1V22βV1V2−2mϕV2V32mϕV2V3+2cα(α+I∗d)3V23). |
It follows that
WTFσ(E∗;σ∗)=(μ+cα(α+I∗d)2)I∗≠0,WT[D2F(E∗;σ∗)(V,V)]=−2β2μS∗(βI∗+μ)2(cI∗d(α+I∗d)2−σI∗I∗d)3+2mϕ(mϕI∗d+σ)(cI∗d(α+I∗d)2−σI∗I∗d)(cI∗d(α+I∗d)2−μ−cα+I∗d)+2cmαϕI∗(mϕI∗d+σ)2(α+I∗d)3. |
Therefore, when WT[D2F(E∗;σ∗)(V,V)]≠0, model (1.1) undergoes a saddle-node bifurcation at E∗.
In this subsection, Hopf and generalized Hopf bifurcations are considered. Let c as the bifurcation parameter, if there exists c1>0 such that α2(c1)>0 and α1(c1)α2(c1)=α3(c1), the characteristic Eq (3.2) has a pair of pure imaginary eigenvalues ±i√α2 and a real root −α1. For sufficiently small ϵ>0, when c∈(c1−ϵ,c1+ϵ), the eigenvalues can be represented as
λ1=−α1(c),λ2=ω(c)+iv(c),λ3=ω(c)−iv(c). |
Substituting λ2 into Eq (3.2), the transversality condition of Hopf bifurcation can be obtained.
sign{dω(c)dc}c=c1=sign{d(α1(c)α2(c)−α3(c))dc/(2α21(c)+α2(c))}c=c1≠0. |
Hence, model (1.1) shows a Hopf bifurcation when c=c1.
Next, we calculate the first and second Lyapunov coefficients by using normal form theory in [17]. Let x=S−S∗,y=I−I∗,z=Id−I∗d, then the following system is obtained through the Taylor series about the origin.
{dxdt=ax+by+a110xy,dydt=dx+fz+b110xy+b011yz,dzdt=hy+lz+c011yz+c002z2+c003z3+c004z4+c005z5+O(|z|6), | (3.4) |
where a,b,d,f,h,l are given in (3.1), and
a110=−β,b110=β,b011=−mϕ,c011=mϕ,c002=cα(α+I∗d)3,c003=−cα(α+I∗d)4,c004=cα(α+I∗d)5,c005=−cα(α+I∗d)6. |
Define ω=(x,y,z)T, note that system (3.4) can be written as
˙ω=Jω+F(ω), | (3.5) |
where
J=JE,F(ω)=12B(ω,ω)+13!C(ω,ω,ω)+14!D(ω,ω,ω,ω)+15!E(ω,ω,ω,ω,ω)+O(|ω|6), |
here
B(x,y)=3∑j,k=1∂2F(ω)∂ωj∂ωk|ω=0xjyk=(a110(x1y2+x2y1)b110(x1y2+x2y1)+b011(x2y3+x3y2)c011(x2y3+x3y2)+2c002x3y3),C(x,y,z)=3∑j,k,l=1∂3F(ω)∂ωj∂ωk∂ωl|ω=0xjykzl=(00−6cα(α+I∗d)4x3y3z3),D(x,y,z,v)=3∑j,k,l,r=1∂4F(ω)∂ωj∂ωk∂ωl∂ωr|ω=0xjykzlvr=(0024cα(α+I∗d)5x3y3z3v3),E(x,y,z,v,w)=3∑j,k,l,r,s=1∂5F(ω)∂ωj∂ωk∂ωl∂ωr∂ωs|ω=0xjykzlvrws=(00−120cα(α+I∗d)6x3y3z3v3w3). |
It is easy to check that
Jq=λq,JTp=¯λp,⟨p,q⟩=1, |
where
q=(bi√α2−a1hi√α2−l),p=(a2+α2)(l2+α2)α22+(a2+bd+fh+l2)α2+(fh+l2)a2+bdl2(d−i√α2−a1f−i√α2−l). |
By simple calculation
l11=−J−1B(q,¯q)=2(afh+bdl)(a2+α2)(l2+α2)(b(h(b011l2+c002fh−c011fl)a2+(a110fh+bb110l)(l2+α2)a+α2h(b011l2+c002fh−c011fl)),−a(b(ab110−a110d)l3+hb011(a2+α2)l2+(−a2c011fh+α2bb110a−α2(da110b+c011fh))l+c002fh2(a2+α2)),(a3b011hl−b(−b110l2+c002dh−c011dl−α2b110)a2+a(−da110(l2+α2)b+α2hlb011)−α2bd(c002h−c011l))h)T,l20=(2i√α2I3−J)−1B(q,q)=1−8iα322+(2al−2bd−2fh)i√α2+(db+4α2)l+afh+4α2a(2(−2i√α2l−fh−4α2)a110bi√α2−a+b(2i√α2−l)(2b110bi√α2−a+2b011hi√α2−l)+bf(2c011hi√α2−l+2c002h2(i√α2−l)2),2d(2i√α2−l)a110bi√α2−a+(2i√α2−a)(2i√α2−l)(2b110bi√α2−a+2b011hi√α2−l)+(2i√α2−a)f(2c011hi√α2−l+2c002h2(i√α2−l)2),2dha110bi√α2−a+(2i√α2−a)h(2b110bi√α2−a+2b011hi√α2−l)+(−4α2−2i√α2a−db)(2c011hi√α2−l+2c002h2(i√α2−l)2))T, |
where I3 is the 3×3 unit matrix, and the first Lyapunov coefficient l1 is given by
l1=1√α2Re(C1), |
where
C1=12⟨p,C(q,q,¯q)+B(¯q,l20)+2B(q,l11)⟩. |
If l1=0, the model (1.1) may occur generalized Hopf bifurcation, the second Lyapunov coefficient l2 can be calculated by the following form
l2=1√α2Re(C2), |
where
C2=112⟨p,E(q,q,q,¯q,¯q)+D(q,q,q,¯l20)+3D(q,¯q,¯q,l20)+6D(q,q,¯q,l11)+C(¯q,¯q,l30)+3C(q,q,¯l21)+6C(q,¯q,l21)+3C(q,¯l20,l20)+6C(q,l11,l11)+6C(¯q,l20,l11)+2B(¯q,l31)+3B(q,l22)+B(¯l20,l30)+3B(¯l21,l20)+6B(l11,l21)⟩, |
and
l20=(2i√α2I3−J)−1B(q,q),l11=−J−1B(q,¯q),l30=(3i√α2I3−J)−1[C(q,q,q)+3B(q,l20)],l21=(i√α2I3−J)−1[C(q,q,¯q)+B(¯q,l20)+2B(q,l11)−2C1q],l31=(2i√α2I3−J)−1[D(q,q,q,¯q)+3C(q,q,l11)+3C(q,¯q,l20)+3B(l20,l11)+B(¯q,l30)+3B(q,l21)−6C1l20],l22=−J−1[D(q,q,¯q,¯q)+4C(q,¯q,l11)+C(¯q,¯q,l20)+C(q,q,¯l20)+2B(l11,l11)+2B(q,¯l21)+2B(¯q,l21)+B(¯l20,l20)−4l11(C1+¯C1)], |
where I3 is the 3×3 unit matrix, and we do not present the long expressions of l30,l21,l31,l22.
Based on the above theoretical analysis, the following results are derived.
Theorem 4. For model (1.1), if l1<0 (>0), then the bifurcating periodic solutions are asymptotically stable (unstable); if l1=0, then Hopf bifurcation is generalized.
According to Theorem 1, model (1.1) has two endemic equilibria E1 and E∗ when Δ=0. Furthermore, if α2=0, the eigenvalues of the Jacobian matrix JE∗ are λ1,2=0 and λ3=−α1, that is to say the model (1.1) may undergo a Bogdanov-Takens bifurcation. Therefore, the following results are given.
Theorem 5. Suppose that Δ=0,α2=0,M20≠0. If M11+2L20≠0, then E∗ is a cusp point of codimension 2; if M11+2L20=0, E∗ is at least a cusp point of codimension 3.
Proof. Introducing the following affine transformation
(xyz)=(−bla −bla2+ba ball−1a−h0h)(uvw), | (3.6) |
where a,b,h,l are given in (3.1). Then system (3.4) takes the form
{˙u=v+L20u2+L11uv+L02v2+w⋅O(|u,v|)+O(|u,v,w|3),˙v=M20u2+M11uv+M02v2+w⋅O(|u,v|)+O(|u,v,w|3),˙w=−α1w+K20u2+K11uv+K02v2+w⋅O(|u,v|)+O(|u,v,w|3), | (3.7) |
where
L20=−al3a110(a−l)(l+a)2+l(−b011lh−b110bl2a)(l+a)2−a(a2+al−l2)(c002h2−c011lh)h(a−l)(l+a)2,L11=a2l(a110l(−bla2+ba)+a110bla)b(a−l)(a+l)2+l(b011h+b110l(−bla2+ba)+b110lba)(a+l)2−a(a2+al−l2)c011(a−l)(a+l)2,L02=a2la110(bla2−ba)b(a−l)(a+l)2+lb110(bla2−ba)(a+l)2,M20=−a110l3a(a−l)(a+l)−a(−b011lh−b110bl2a)a+l−a2l(c002h2−c011hl)(a−l)(a+l)h,M11=−a2l(a110l(bla2−ba)−a110bla)b(a−l)(a+l)−a2lc011(a−l)(a+l)+a(−b011h+b110l(bla2−ba)−b110bla)a+l,M02=a2la110(bla2−ba)b(a−l)(a+l)−ab110(bla2−ba)a+l,K20=L20+c002h−c011l,K11=L11+c011,K02=L02. |
Applying the center manifold theorem, system (3.7) becomes
{˙u=v+L20u2+L11uv+L02v2+O(|u,v|3),˙v=M20u2+M11uv+M02v2+O(|u,v|3). | (3.8) |
Using the following transformation
u=η1+12(L11+M02)η21+L02η1η2+O(|η1,η2|3),v=η2−L20η21+M02η1η2+O(|η1,η2|3). |
It can be derived that
{˙η1=η2,˙η2=M20η21+(M11+2L20)η1η2+O(|η1,η2|3). | (3.9) |
Therefore, assumed that Δ=0,α2=0,M20≠0. If M11+2L20≠0, E∗ is a cusp point of codimension 2, and the codimension of the cusp point is at least 3 if M11+2L20=0.
In the following analysis, ϕ,c are chosen as bifurcation parameters, ϕ=ϕ0 and c=c0 satisfy Δ=0 and α2=0. The model (1.1) is perturbed with (ϕ,c) in a small neighborhood of (ϕ0,c0). Let ϕ=ϕ0+ϵ1,c=c0+ϵ2, where ϵ=(ϵ1,ϵ2) is a parameter vector in a small neighborhood of (0,0). By the translation x=S−S∗,y=I−I∗,z=Id−I∗d and using Taylor expansion, model (1.1) is transformed into
{dxdt=ax+by+a110xy,dydt=q′+dx+e′y+(f+f′)z+b110xy+(b011+b′011)yz,dzdt=c′+(h+h′)y+(l+l′)z+(c011+c′011)yz+(c002+c′002)z2+O(|z|3), | (3.10) |
where a,b,a110,d,f,b110,b011,h,l,c011,c002 are given in system (3.4) and
q′=−mϵ1I∗I∗d,e′=−mϵ1I∗d,f′=−mϵ1I∗,b′011=−mϵ1,c′=mϵ1I∗I∗d−ϵ2I∗dα+I∗d,h′=mϵ1I∗d,c′011=mϵ1,l′=1(α+I∗d)2(ϵ1I∗m(I∗d+α)2−ϵ2α),c′002=ϵ2α(α+I∗d)3. |
Applying the transformation (3.6), then system (3.10) restricted on the center manifold is
{˙u=v+a00+a10u+a01v+12a20u2+a11uv+12a02v2+O(|u,v|3),˙v=b00+b10u+b01v+12b20u2+b11uv+12b02v2+O(|u,v|3), | (3.11) |
where
a00=lq′(a+l)2−a(a2+al−l2)c′h(a−l)(l+a)2,a10=l(−dbla+e′l−fh)(a+l)2−a(a2+al−l2)((h+h′)l−(l+l′)h)h(a−l)(a+l)2,a01=−e′l(a+l)2+a(a2+al−l2)h′h(a−l)(a+l)2,a20=2L20−2b′011l2h(a+l)2−2a(a2+al−l2)(c′002h2−c′011lh)h(a−l)(a+l)2,a11=L11+l(blha+b′011h)(a+l)2−a(a2+al−l2)c′011(a−l)(a+l)2,a02=2L02,b00=−aq′a+l−a2lc′(a−l)h(a+l),b10=−a(−dbla+e′l−fh)a+l−a2l((h+h′)l−(l+l′)h)(a−l)(a+l)h,b01=−al2(a+l)(a−l)+a2e′−bda+bdla(a+l)+a2l(h+h′)(a+l)(a−l)h,b20=2M20+2a(b′011lh)a+l−2a2l(−c′011lh+c′002h2)(a−l)(a+l)h,b11=M11+a(−blha−b′011h)a+l−a2lc′011(a+l)(a−l),b02=2M02. |
Define a nonlinear transformation
x=u,y=v+a00+a10u+a01v+12a20u2+a11uv+12a02v2+O(|u,v|3). |
It can be derived from system (3.11) that
{˙x=y,˙y=g00+g10x+g01y+12g20x2+g11xy+12g02y2+O(|x,y|3), | (3.12) |
where
g00=b00+O(ϵ2),g10=b10+a11b00−b11a00+O(ϵ2),g01=b01+a10+a02b00−(a11+b02)a00+O(ϵ2),g20=b20+O(ϵ),g11=a20+b11+O(ϵ),g02=b02+2a11+O(ϵ). |
Introducing the transformation
x=v1−g01g11, y=v2. |
One can obtain
{˙v1=v2,˙v2=h00+h10v1+12h20v21+h11v1v2+12h02v22+O(|v1,v2|3), | (3.13) |
where
h00=g00−g01g11g10+O(ϵ2),h20=g20+O(ϵ),h10=g10−g01g11g20+O(ϵ2),h11=g11+O(ϵ),h02=g02+O(ϵ). |
Introducing a new time variable τ by dt=(1−h022v1)dτ and rewriting τ as t. Then
{˙v1=v2−h022v1v2,˙v2=h00+(h10−h022h00)v1+12(h20−h02h10)v21+h11v1v2+12h02v22+O(|v1,v2|3). | (3.14) |
Using the transformation
ξ1=v1,ξ2=v2−h022v1v2, |
system (3.14) becomes
{˙ξ1=ξ2,˙ξ2=μ1+μ2ξ1+μ3ξ21+μ4ξ1ξ2+O(|ξ1,ξ2|3), | (3.15) |
where
μ1=h00,μ2=h10−12h00h02,μ3=12(h20−h10h02),μ4=h11. |
Introducing the change of variables and rescaling of time
η1=μ24μ3ξ1,η2=sign(μ3μ4)μ34μ23ξ2,t=|μ3μ4|τ. |
Then system (3.15) takes the required form
{˙η1=η2,˙η2=β1+β2η1+η21+sη1η2+O(|η1,η2|3), | (3.16) |
where
β1=μ44μ33μ1,β2=μ24μ23μ2,s=sign(μ4μ3). |
Next, a numerical example is proposed to show Bogdanov-Takens bifurcation. Taking ϕ=0.05558,c=3.69082, other parameters are given in (4.1). By simple calculation, it can be obtained that
β1=(1.6710ϵ2−14.134ϵ1)(−0.0011511+0.0030950ϵ1−9.6342×10−7ϵ2)7/(−0.00010783ϵ31+0.000022199ϵ41+1.7360×10−8ϵ2−7.3281×10−7ϵ1ϵ2+0.000042818ϵ21+2.6542×10−6ϵ21ϵ2−2.5058×10−6ϵ31ϵ2+1.4214×10−11ϵ22−5.8151×10−10ϵ1ϵ22+1.4172×10−9ϵ21ϵ22−1.0591×10−6ϵ1−1.1977×10−9)3,β2=(−0.0011511+0.0030950ϵ1−9.6342×10−7ϵ2)4(0.000075528ϵ31−2.9602×10−5ϵ2−0.0045697ϵ21+0.000074794ϵ1ϵ2−3.1634×10−6ϵ21ϵ2−2.4213×10−8ϵ22+3.0469×10−8ϵ1ϵ22+0.0018344ϵ1−2.3246×10−8)/(−1.0783×10−4ϵ31+0.000022199ϵ41+1.7360×10−8ϵ2−7.3281×10−7ϵ1ϵ2+4.2818×10−5ϵ21+2.6542×10−6ϵ21ϵ2−2.5058×10−6ϵ31ϵ2+1.4214×10−11ϵ22−5.8151×10−10ϵ1ϵ22+1.4172×10−9ϵ21ϵ22−1.0591×10−6ϵ1−1.1977×10−9)2×(−0.0011511+0.0030950ϵ1−9.6342×10−7ϵ2), |
then
|∂(β1(ϵ),β2(ϵ))∂(ϵ1,ϵ2)|ϵ=0=4.4863×1012. |
Therefore, model (1.1) undergoes a Bogdanov-Takens bifurcation of codimension 2 in a small neighborhood of (ϕ0,c0). According to Lemma 8.7 in Kuznetsov [17], the following Theorem can be obtained.
Theorem 6. For model (1.1), we take ϕ and c as bifurcation parameters, the bifurcation cures are given by the following:
1) Saddle-node bifurcation occurs from the bifurcation curve:
SN={(ϵ1,ϵ2):4β1=β22}; |
2) Hopf bifurcation occurs from the bifurcation curve:
H={(ϵ1,ϵ2):β1=0,β2<0}; |
3) Homoclinic orbit occurs from the bifurcation curve:
HL={(ϵ1,ϵ2):25β1+6β22≈0,β2<0}. |
In this section, the theoretical conclusions of model (1.1) are validated by the numerical simulation. The following data are used for numerical analysis in the full text.
A=5,β=0.003,μ=0.01,σ=0.01,m=0.02,α=0.5. | (4.1) |
In Figure 1, it is easy to see that the model (1.1) may exist one or three positive equilibria as the change of σ. In addition, there are two limit points for model (1.1), that is, the model (1.1) undergoes saddle-node bifurcation.
The bifurcation curve of co-dimension 1 of model (1.1) is given in Figure 2(a). Taking c as the bifurcation parameter, there are two Hopf points and two limit points with the change of c. When c=3.81680, E1(λ1=−0.6,λ2,3=−0.0003±0.08i) and E3(λ1,2=−0.01±0.1i,λ3=−0.01) are stable foci, E2 is a saddle point and an unstable limit cycle (l1=2.1206×10−5>0) emerges from the Hopf point (H1) near the equilibrium E1, which is illustrated in Figure 3(a), (b). If c=3.92610, E1(λ1=−0.6,λ2,3=−0.07±0.07i) and E3(λ1,2=−0.01±0.1i,λ3=−0.01) are also stable foci, E2 is a saddle point and an unstable limit cycle (l1=3.1916×10−5>0) arises from the Hopf point (H2) near the equilibrium E3, it is shown in Figure 3(c), (d).
The bifurcation curves of co-dimension 2 are generated from Figure 2(b), one of them shows a generalized Hopf bifurcation (GH) and the other is a Bogdanov-Takens bifurcation (BT). In Figure 3(e), E3(λ1,2=−0.01±0.1i,λ3=−0.01) is locally stable and E2 is a saddle point, the Bautin (generalized Hopf) bifurcation (l1=0,l2=−6.9961×10−6<0) occurs at (ϕ,c)=(0.13458,3.84246). There are two limit cycles that appear near equilibrium E1, and the large limit cycle is stable and the small one is unstable, the phase portrait is illustrated in Figure 3(f). In Figure 4(a), (b), E1(λ1=−0.03,λ2=−0.7,λ3=−0.4) is a stable node and E∗ is a BT point of order 2. As shown in Figure 4(c), (d), (e), (f), with the values of ϕ and c increase, E1 is still a stable node and E3 is a stable focus, the model first appears an unstable limit cycle around E3, then the limit cycle disappears, model (1.1) has a homoclinic orbit to the saddle point E2 and it eventually disappears.
Testing-culling is a major prevention and control measure for brucellosis used by the animal disease regulatory authorities of some countries [8,18]. Therefore, the impact of testing and culling measures is an interesting research topic. In this paper, on the basis of brucellosis control policies and the characteristics of animal testing and considering the limitation of culling resources, a mathematical model is established to demonstrate the impact of testing and culling measures on the dynamics of brucellosis transmission. The model is found to have a disease-free equilibrium that is globally asymptotically stable if R0≤1, and there may be one or three positive equilibria if R0>1, which leads to saddle-node bifurcation, (generalized) Hopf bifurcation and Bogdanov-Takens bifurcation in the model. Biologically speaking, saddle node bifurcation means that the model appears multi-stable; that is, the initial state of each subpopulation may affect the level of brucellosis transmission. The Hopf bifurcation and Bogdanov-Takens bifurcation imply that the model undergoes periodic oscillations. In other words, if the epidemic is at the lowest point of the cycle, it prompts people to think that the disease may disappear, affecting the implementation of prevention and control measures. In addition, the endemic equilibrium of the model is globally asymptotically stable if no testing and culling are conducted, which implies that testing and culling measures can lead to complex dynamics of brucellosis transmission.
Our study has some limitations. Some features of animal culling are still not considered in the model. For example, the disease may spread because of the delayed culling of infected animals. Further, in practice, because of the sensitivity of the test reagents, the test results need to be reconfirmed, and this test process is also not expressed in our model. We need to further consider these aspects.
This research is partially supported by the Fundamental Research Program of Shanxi Province (20210302123031, 20210302123019), the National Youth Science Foundation of China (11501528).
The authors declare there is no conflict of interest.
[1] |
T. Barbier, F. Collard, A. Zúñiga-Ripa, I. Moriyón, T. Godard, J. Becker, et al., Erythritol feeds the pentose phosphate pathway via three new isomerases leading to D-erythrose-4-phosphate in Brucella, Proc. Natl. Acad. Sci. U. S. A., 111 (2014), 17815–17820. https://doi.org/10.1073/pnas.1414622111 doi: 10.1073/pnas.1414622111
![]() |
[2] |
P. Andriopoulos, M. Tsironi, S. Deftereos, A. Aessopos, G. Assimakopoulos, Acute brucellosis: Presentation, diagnosis, and treatment of 144 cases, Int. J. Infect. Dis., 11 (2007), 52–57. https://doi.org/10.1016/j.ijid.2005.10.011 doi: 10.1016/j.ijid.2005.10.011
![]() |
[3] |
G. Pappas, P. Papadimitriou, N. Akritidis, L. Christou, E. V. Tsianos, The new global map of human brucellosis, Lancet Infect. Dis., 6 (2006), 91–99. https://doi.org/10.1016/S1473-3099(06)70382-6 doi: 10.1016/S1473-3099(06)70382-6
![]() |
[4] |
H. Zeng, Y. M. Wang, X. D. Sun, P. Liu, Q. G. Xu, D. Huang, et al., Status and influencing factors of farmers' private investment in the prevention and control of sheep brucellosis in China: A cross-sectional study, PLoS Neglected Trop. Dis., 13 (2019), e0007285. https://doi.org/10.1371/journal.pntd.0007285 doi: 10.1371/journal.pntd.0007285
![]() |
[5] |
A. J. S. Alves, F. Rocha, M. Amaku, F. Ferreira, E. O. Telles, J. H. H. Grisi Filho, et al., Economic analysis of vaccination to control bovine brucellosis in the States of Sao Paulo and Mato Grosso, Brazil, Prev. Vet. Med., 118 (2015), 351–358. https://doi.org/10.1016/j.prevetmed.2014.12.010 doi: 10.1016/j.prevetmed.2014.12.010
![]() |
[6] |
Q. Hou, X. D. Sun, J. Zhang, Y. J. Liu, Y. M. Wang, Z. Jin, Modeling the transmission dynamics of sheep brucellosis in Inner Mongolia Autonomous Region, China, Math. Biosci., 242 (2013), 51–58. https://doi.org/10.1016/j.mbs.2012.11.012 doi: 10.1016/j.mbs.2012.11.012
![]() |
[7] |
H. Yoon, O. K. Moon, S. H. Lee, W. C. Lee, M. Her, W. Jeong, et al., Epidemiology of brucellosis among cattle in Korea from 2001 to 2011, J. Vet. Sci., 15 (2014), 537–543. https://doi.org/10.4142/jvs.2014.15.4.537 doi: 10.4142/jvs.2014.15.4.537
![]() |
[8] |
R. M. Davidson, Control and eradication of animal diseases in New Zealand, N. Z. Vet. J., 50 (2002), 6–12. https://doi.org/10.1080/00480169.2002.36259 doi: 10.1080/00480169.2002.36259
![]() |
[9] |
M. Zamri-Saad, M. I. Kamarudin, Control of animal brucellosis: The Malaysian experience, Asian Pac. J. Trop. Med., 9 (2016), 1136–1140. https://doi.org/10.1016/j.apjtm.2016.11.007 doi: 10.1016/j.apjtm.2016.11.007
![]() |
[10] |
N. Zhang, D. S. Huang, W. Wu, J. Liu, F. Liang, B. S. Zhou, et al., Animal brucellosis control or eradication programs worldwide: A systematic review of experiences and lessons learned, Prev. Vet. Med., 160 (2018), 105–115. https://doi.org/10.1016/j.prevetmed.2018.10.002 doi: 10.1016/j.prevetmed.2018.10.002
![]() |
[11] |
P. O. Lolika, C. Modnak, S. Mushayabasa, On the dynamics of brucellosis infection in bison population with vertical transmission and culling, Math. Biosci., 305 (2018), 42–54. https://doi.org/10.1016/j.mbs.2018.08.009 doi: 10.1016/j.mbs.2018.08.009
![]() |
[12] |
L. Bolzoni, R. D. Marca, M. Groppi, A. Gragnani, Dynamics of a metapopulation epidemic model with localized culling, Discrete Cont. Dyn.-B, 25 (2020), 2307–2330. https://doi.org/10.3934/dcdsb.2020036 doi: 10.3934/dcdsb.2020036
![]() |
[13] |
J. Zinsstag, F. Roth, D. Orkhon, G. Chimed-Ochir, M. Nansalmaa, J. Kolar, et al., A model of animal-human brucellosis transmission in Mongolia, Prev. Vet. Med., 69 (2005), 77–95. https://doi.org/10.1016/j.prevetmed.2005.01.017 doi: 10.1016/j.prevetmed.2005.01.017
![]() |
[14] |
M. F. Abakar, H. Yahyaoui Azami, P. Justus Bless, L. Crump, P. Lohmann, M. Laager, et al., Transmission dynamics and elimination potential of zoonotic tuberculosis in morocco, PLoS Neglected Trop. Dis., 11 (2017), e0005214. https://doi.org/10.1371/journal.pntd.0005214 doi: 10.1371/journal.pntd.0005214
![]() |
[15] |
P. van den Driessche, J. Watmough, Reproduction numbers and sub-threshold endemic equilibria for compartmental models of disease transmission, Math. Biosci., 180 (2002), 29–48. https://doi.org/10.1016/S0025-5564(02)00108-6 doi: 10.1016/S0025-5564(02)00108-6
![]() |
[16] |
G. H. Li, Y. X. Zhang, Dynamic behaviors of a modified SIR model in epidemic diseases using nonlinear incidence and recovery rates, PLoS One, 12 (2017), e0175789. https://doi.org/10.1371/journal.pone.0175789 doi: 10.1371/journal.pone.0175789
![]() |
[17] | Y. A. Kuznetsov, Elements of Applied Bifurcation Theory, 3rd edition, Springer-Verlag, New York, 2004. https://doi.org/10.1007/978-1-4757-3978-7 |
[18] |
D. A. Abernethy, J. Moscard-Costello, E. Dickson, R. Harwood, K. Burns, E. McKillop, et al., Epidemiology and management of a bovine brucellosis cluster in Northern Ireland, Prev. Vet. Med., 98 (2011), 223–229. https://doi.org/10.1016/j.prevetmed.2010.11.002 doi: 10.1016/j.prevetmed.2010.11.002
![]() |
1. | Zhiguo Liu, Miao Wang, Yingqi Wang, Min Yuan, Zhenjun Li, Retrospective Analysis of the Epidemiological Evolution of Brucellosis in Animals — China, 1951–1989 and 1996–2021, 2024, 6, 2097-3101, 1159, 10.46234/ccdcw2024.235 | |
2. | Shuangjie Bai, Boqiang Cao, Ting Kang, Qingyun Wang, A two-stage sheep-environment coupled brucellosis transmission dynamic model: Stability analysis and optimal control, 2024, 0, 1531-3492, 0, 10.3934/dcdsb.2024126 |