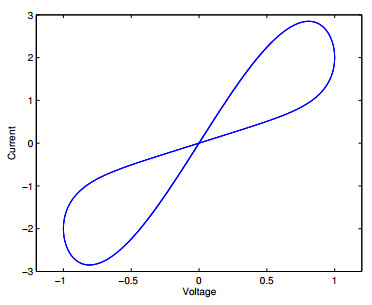
In this paper, synchronization of fractional-order memristive recurrent neural networks via aperiodically intermittent control is investigated. Considering the special properties of memristor neural network, differential inclusion theory is introduced. Similar to the aperiodically strategy of integer order, aperiodically intermittent control strategy of fractional order is proposed. Under the framework of Fillipov's solution, based on the intermittent strategy of fractional order systems and the properties Mittag-Leffler, sufficient criteria of aperiodically intermittent strategy are obtained by constructing appropriate Lyapunov functional. Some comparisons are given to demonstrate the advantages of aperiodically strategy. A simulation example is given to illustrate the derived conclusions.
Citation: Shuai Zhang, Yongqing Yang, Xin Sui, Yanna Zhang. Synchronization of fractional-order memristive recurrent neural networks via aperiodically intermittent control[J]. Mathematical Biosciences and Engineering, 2022, 19(11): 11717-11734. doi: 10.3934/mbe.2022545
[1] | Xinxin Zhang, Huaiqin Wu . Bipartite consensus for multi-agent networks of fractional diffusion PDEs via aperiodically intermittent boundary control. Mathematical Biosciences and Engineering, 2023, 20(7): 12649-12665. doi: 10.3934/mbe.2023563 |
[2] | Bingrui Zhang, Jin-E Zhang . Fixed-deviation stabilization and synchronization for delayed fractional-order complex-valued neural networks. Mathematical Biosciences and Engineering, 2023, 20(6): 10244-10263. doi: 10.3934/mbe.2023449 |
[3] | Ruoyu Wei, Jinde Cao . Prespecified-time bipartite synchronization of coupled reaction-diffusion memristive neural networks with competitive interactions. Mathematical Biosciences and Engineering, 2022, 19(12): 12814-12832. doi: 10.3934/mbe.2022598 |
[4] | Prasina Alexander, Fatemeh Parastesh, Ibrahim Ismael Hamarash, Anitha Karthikeyan, Sajad Jafari, Shaobo He . Effect of the electromagnetic induction on a modified memristive neural map model. Mathematical Biosciences and Engineering, 2023, 20(10): 17849-17865. doi: 10.3934/mbe.2023793 |
[5] | Xinyi He, Xiuping Han, Tengda Wei, Xiaodi Li . Tracking control of wheeled mobile robots via intermittent control. Mathematical Biosciences and Engineering, 2024, 21(3): 3774-3783. doi: 10.3934/mbe.2024167 |
[6] | Mahtab Mehrabbeik, Fatemeh Parastesh, Janarthanan Ramadoss, Karthikeyan Rajagopal, Hamidreza Namazi, Sajad Jafari . Synchronization and chimera states in the network of electrochemically coupled memristive Rulkov neuron maps. Mathematical Biosciences and Engineering, 2021, 18(6): 9394-9409. doi: 10.3934/mbe.2021462 |
[7] | Biwen Li, Xuan Cheng . Synchronization analysis of coupled fractional-order neural networks with time-varying delays. Mathematical Biosciences and Engineering, 2023, 20(8): 14846-14865. doi: 10.3934/mbe.2023665 |
[8] | Gayathri Vivekanandhan, Hamid Reza Abdolmohammadi, Hayder Natiq, Karthikeyan Rajagopal, Sajad Jafari, Hamidreza Namazi . Dynamic analysis of the discrete fractional-order Rulkov neuron map. Mathematical Biosciences and Engineering, 2023, 20(3): 4760-4781. doi: 10.3934/mbe.2023220 |
[9] | Qiong Wu, Zhimin Yao, Zhouping Yin, Hai Zhang . Fin-TS and Fix-TS on fractional quaternion delayed neural networks with uncertainty via establishing a new Caputo derivative inequality approach. Mathematical Biosciences and Engineering, 2022, 19(9): 9220-9243. doi: 10.3934/mbe.2022428 |
[10] | Ganesh Kumar Thakur, Sudesh Kumar Garg, Tej Singh, M. Syed Ali, Tarun Kumar Arora . Non-fragile synchronization of BAM neural networks with randomly occurring controller gain fluctuation. Mathematical Biosciences and Engineering, 2023, 20(4): 7302-7315. doi: 10.3934/mbe.2023317 |
In this paper, synchronization of fractional-order memristive recurrent neural networks via aperiodically intermittent control is investigated. Considering the special properties of memristor neural network, differential inclusion theory is introduced. Similar to the aperiodically strategy of integer order, aperiodically intermittent control strategy of fractional order is proposed. Under the framework of Fillipov's solution, based on the intermittent strategy of fractional order systems and the properties Mittag-Leffler, sufficient criteria of aperiodically intermittent strategy are obtained by constructing appropriate Lyapunov functional. Some comparisons are given to demonstrate the advantages of aperiodically strategy. A simulation example is given to illustrate the derived conclusions.
The concept of memristor was proposed by Chua [1,2]. The characteristic of memristive systems is shown in Figure 1. Until more than 30 years later, Hewlett Packard laboratory [3,4] announced the realization of the physical devices of the memristor. The theory and application of memristor have attracted the attention of scholars again. Memristor has the advantages of smaller size, lower energy consumption, and larger information storage capacity. Memristor is Therefore, memristor is the best choice to construct the next generation neural network instead of resistor and improve the efficiency of intelligent algorithm in machine learning [5,6,7]. Therefore, memristor-based neural networks can be constructed (see Figure 2). In Figure 2, M1i1,...,M1in and M2i1,...,M2in are memristor, other parameters are same as traditional neural networks with resistor [8]. Shi and Cao et al. [9] first investigated exponential stability of complex-valued memristive neural networks. In [10], Yang and Ho analyzed the robustness of the memristive neural network. In [11], a more general situation of mixed delays is discussed. Mehrabbeik [12] et al. investigate synchronization of the m-Rulkov model. In addition, memristive neural networks with time delay [13,14], time-varying delay [15,16], mixed delays [17,18] and switching jumps mismatch [8,19] are discussed.
Fractional calculus which was proposed in 1695, appears almost at the same time as classical calculus. In later research, scholars found that some models can not be well described by using classical integral calculus. On the contrary, fractional calculus can well describe those models. In recent years, the theory of fractional calculus has been successfully applied to various fields. There are more and more works on fractional order dynamical systems. Wang and Yang [23] first discussed the quasi-synchronization problem of fractional-order systems with different structures. In [24], Bifurcations of fractional complex-valued neural networks with delays is investigated. The synchronization of fractional order systems with discontinuous activation functions is studied in [25]. In addition, the fractional order system is also analyzed from the mathematical point of view [20,21,22].
Synchronization is one of the most common collective behaviors of dynamical systems [26,27]. Synchronization usually refers to the phenomenon of phase consistency among dynamical systems. Synchronization not only exists widely in nature, but it is also very important in practical applications. There are a lot of results about synchronization. In these published results, some control strategies are used to achieve synchronization, such as predictive control [28], adaptive control [29], iterative learning control [30], fuzzy control [31], sliding-mode control [32], event-triggered control [33,34,35], sampled-date control [36], impulsive control [37,38,39,40], intermittent control [41,42,43] and so on. Among those control strategies, intermittent control strategy is the most representative one, and also the one most in line with actual needs. So, intermittent control attracts more and more attention. The intermittent strategy can be divided into periodically intermittent and aperiodically intermittent. Synchronization of integer order systems via periodically intermittent control or aperiodically intermittent control has been widely studied [41,42,43]. Synchronization of fractional order systems via periodically intermittent strategy has been investigated in [41,42]. However, as far as we know, there are no literatures on fractional order dynamical systems via aperiodically intermittent strategy.
Inspired by the idea of aperiodically intermittent strategy of integer order, aperiodically intermittent strategy is extended to fractional order systems. In the works of integer-order dynamical behavior, both periodically intermittent control and aperiodically intermittent control are widely studied. Among those works, The advantages of aperiodically intermittent control are proven. In addition, unlike periodically intermittent control with fixed width control interval and non-control interval, aperiodically control has fewer interval constraints(see Figure 3), which makes aperiodically intermittent control more flexible and more in line with application requirements. This paper investigates synchronization of fractional order memeristive neural networks via aperiodically intermittent control. Firstly, according to the characteristics of memristive neural networks, dynamical systems with differential inclusion is obtained. Then, in the sense of Filipov's solution, systems with differential inclusion are transformed into conventional forms that can be processed. Finally, based on the periodically intermittent control theory, sufficient conditions of synchronization of memeristive neural networks via aperiodically intermittent control are derived. The structure of this paper is organized as follows: In Section 2, some useful definitions, basic assumptions and lemmas are recalled. In Section 3, based on the theory of fractional order periodically control, sufficient conditions for synchronization are derived by constructing appropriate Lyapunov functional. In addition, Some comparisons with existing results are given verify advantages of the derived conditions. In Section 4, a numerical example is given to demonstrate the derived conditions. Conclusions are given in Section 5.
Notations. This paper is a conducted in the sense of Filippov's. Rm×n denote the m×n real-valued matrix set. co{x,y} represents convex hull of x and y. λmax(⋅) denotes the max eigenvalue of a matrix. T denotes the matrix transposition. Inf(⋅) and Sup(⋅) denote the upper bound and lower bound. ||⋅|| denotes the norm of a matrix vector.
The Captuto calculus is the most common of many types of calculus. The properties of some integer-order differentials can be applied directly, which is one of the reasons for the wide application of Caputo calculus. In this article, Captuto calculus is used for analysis.
Definition 1. [23] For any integrable function f(t)∈C, the fractional integral of order α of function f(t) is defined as
Iαf(t)=1Γ(α)∫tt0(t−s)α−1f(s)ds, |
where α>0, and Γ(⋅) is Gamma function.
Definition 2. [23] For any function f(t), Caputo's derivative of order α is defined by
Dαf(t)=1Γ(n−α)∫tt0(t−s)n−α−1fn(s)ds, |
where n is a positive number and n−1<α<n. Particularly, when n=1,
Dαf(t)=1Γ(1−α)∫tt0f(s)(t−s)αds. |
Fractional-order memristive neural networks of Caputo's derivative are defined by
Dαx(t)=−Cx(t)+A(x(t))f(x(t))+I(t), | (2.1) |
where α is the order of Captuto's derivative, x(t)=(x1(t),x2(t),⋅⋅⋅,xn(t))T∈Cn, xi(t) is the complex-valued state variable. C=diag{ci}n×n∈Rn×n is the ith self-feedback connection weight with ci>0(i=1,2,...,n). A(x(t))=aij(xij(t))n×n are connection weights f(x(t))=(f1(x1(t)),f2(x2(t)),...,fn(xn(t)))T are activation function. I(t)=(I1(t),I2(t),...,In(t))T∈Cn are external input.
Similarly, the slave-systems are denoted as follows:
Dαy(t)=−Cy(t)+A(y(t))f(y(t))+I(t)+u(t), | (2.2) |
where u(t)=(u1(t),u2(t),...,un(t))T are controller.
For the convenience of discussion, Figure 4 is used for discussion. Figure 4 is a simplified model of Figure 1. In this case, the connection weight aij(xj(t)) of (2.1) are defined as follows [15]
aij(vj(s))={a′,|vj(s)|≤T,a″,|vj(s)|>T, |
where T is the switching threshold value with master-systems (2.1) and slave-systems (2.2). a′ and a″ denote two states of aij(vj(t)).
Lemma 1. [42] If x(⋅):[t0,∞)→R is a continuous differential function and
Dαx(t)≤−ax(t),t≥t0, |
where a and b are positive constants, then
x(t)≤(x(t0)−ba)Eα(−a(t−t0)α),t≥t0≥0, |
where Eα(−a(t−tα0)) is one-parameter Mittag-Leffler function which is defined by
Eα(z)=Eα,1(z)=∞∑k=0zkΓ(α+1). |
Lemma 2. [41] For x(⋅) and y(⋅) are two continuous differential functions, if α,β>0, then
DαD−βx(t)=Dα−βx(t), |
Dα(x(t)±y(t))=Dαx(t)±Dαy(t). |
Lemma 3. [25] Let f(t)=(f1(t),f2(t),...,fn(t))T is a n-dimensional vector differentiable function, for any positive definite symmetric matrix Q∈Rn×n. The following inequalities can be derived
Dα(fT(s)Qf(s))≤2fT(s)QDαf(s), |
where α∈[0,1],0≤s.
Assumption 1. [24] For the aperiodically intermittent strategy, the control interval and non-control interval satisfy the following equations
{Infk(sk−tk)=h,Supk(tk+1−tk)=H, |
where h and H are two positive scalars and satisfy 0<h<H<+∞.
Based on the Assumption 1, (2.1) can be rewritten as the following differential inclusion:
Dαx(t)∈−Cx(t)+co[A(x(t))]f(x(t))+I(t),Dαy(t)∈−Cy(t)+co[A(y(t))]f(y(t))+I(t). | (2.3) |
Under the Assumption 1, define ej(t)=yj(t)−xj(t) for j=1,2,...,n. So, error systems can be obtained:
Dαe(t)∈−C(y(t)−x(t))+co[A(y(t))]f(y(t))−co[A(x(t))]f(x(t))+u(t), | (2.4) |
u(t)={−K(y(t)−x(t)),tk≤t<sk,0,sk≤t<tk+1, | (2.5) |
where K are gain coefficients.
Lemma 4. [42] For any activation functions f(±T)=0, then one can derive that
co[aij(y(t))]fj(yj(t))−co[aij(xj(t))]fj(xj(t))≤a∗ijlj|yj(t)−xj(t)|, |
where lj is the Lipschitz condition and a∗ij is the maximum of a′ and a″.
In this section, the synchronization problems of fractional order memristor-based neural netwroks via aperiodically intermittent control strategy would be investigated. Sufficient conditions will be derived.
Theorem 1. Under the Lemmas 1–4, and Assumption 1, fractional-order master-system (2.1) and slave-systems (2.2) can achieve synchronization under controller (2.5), if
−||C||+||A||||L||<||K||, |
Eα(−γ1hα)Eα(γ2(H−h)α)<1, |
where, γ1=−||C||+||A||||L||−||K|| and γ2=−||C||+||A||||L|| are two positive scalers.
Proof. Constructing the following Lyapunov functional
V(t)=12eT(t)e(t). |
For, tk≤t<sk, deriving derivative of V(t) along along with (2.3), one can get
DαV(t)≤eT(t)Dαe(t). |
Combining the error systems (2.4) and Lemma 3,
DαV(t)≤eT(t)(−Ce(t)+A(y(t))f(y(t))−A(x(t))f(x(t))+u(t))≤eT(t)(−Ce(t)+(A(y(t))f(y(t))−A(x(t))f(x(t)))−Ke(t)))≤−C||e(t)||2+||eT(t)||||(A(y(t))f(y(t))−A(x(t))f(x(t)))||−||K||||e(t)||2≤−C||e(t)||2+||A||||L||||e(t)||2−||K||||e(t)||2=(−||C||+||A||||L||−||K||)||e(t)||2. |
According to the Theorem conditions, −γ1=−||C||+||A||||L||−||K||<0, so
Dα≤−γ1V(t). |
Based on the Lemma 4, the following equalities can be derived
V(t)≤V(tk)Eα(−γ1(t−ttk)α). |
Similarly, For, sk≤t<tk+1 Deriving derivative of V(t) along along with (2.4), one can get
DαV(t)≤eT(t)Dαe(t). |
Combining the error system (2.4) and Lemma 3,
DαV(t)≤eT(t)(−Ce(t)+A(y(t))f(y(t))−A(x(t))f(x(t)))≤eT(t)(−Ce(t)+(A(y(t))f(y(t))−A(x(t))f(x(t)))))≤−C||e(t)||2+||eT(t)||||(A(y(t))f(y(t))−A(x(t))f(x(t)))||≤−C||e(t)||2+||A||||L||||e(t)||2=(−||C||+||A||||L||)||e(t)||2. |
According to the Theorem conditions, γ2=−C+Al>0, so
Dα≤γ2V(t). |
Based on the Lemma 4, the following equalities can be derived
V(t)≤V(sk)Eα(γ2(t−sk)α). |
According to the principle of mathematical induction, combining the control period sk−tk and non-control period tk+1−sk, when k=0, one can get that:
For 0=t0≤t<s0, based on the Lemma 2, one can get
V(t)≤V(t0)Eα(−γ1(t−t0)α)=V(0)Eα(−γ1tα), | (3.1) |
so, one can derive
V(s0)≤V(t0)Eα(−γ1(s0−t0)α). | (3.2) |
For s0≤t<t1,
V(t)≤V(s0)Eα(γ2(t−s0)α), | (3.3) |
so, one can derive
V(t1)≤V(t0)Eα(−γ1(s0−t0)α)Eα(γ2(t1−s0)α). | (3.4) |
For t1≤t<s1,
V(t)≤V(t1)Eα(−γ1(t−t1)α), | (3.5) |
so, one can derive
V(s1)≤V(t0)Eα(−γ1(s0−t0)α)Eα(γ2(t1−s0)α)Eα(−γ1(s1−t1)α). | (3.6) |
For s1≤t<t2,
V(t)≤V(s1)Eα(γs(t−s1)α), | (3.7) |
so, one can derive
V(t2)≤V(t0)Eα(−γ1(s0−t0)α)Eα(γ2(t1−s0)α)Eα(−γ1(s1−t1)α)Eα(γs(t2−s1)α). | (3.8) |
For t2≤t<s2
V(t)≤V(t2)Eα(−γ1(t−t2)α), | (3.9) |
so, one can derive
V(s2)≤V(t0)Eα(−γ1(s0−t0)α)Eα(γ2(t1−s0)α)Eα(−γ1(s1−t1)α)Eα(γ1(t2−s1)α)Eα(−γ1(s2−t2)α). | (3.10) |
For s2≤t<t3,
V(t)≤V(s2)Eα(−γ1(t−s2)α), | (3.11) |
so, one can derive that
V(t3)≤V(t0)Eα(−γ1(s0−t0)α)Eα(γ2(t1−s0)α)Eα(−γ1(s1−t1)α)Eα(γs(t2−s1)α)Eα(−γ1(s2−t2)α)Eα(−γ1(t−s2)α). | (3.12) |
According to the principle of mathematical analogy, when k=m, one can assume
For tm≤t<sm,
V(t)≤V(t0)m−1∏k=1(Eα(−γ1(sk−tk)α)Eα(tk+1−sk))Eα(−γ1(t−tm)α), | (3.13) |
so, one can derive that
V(sm)≤V(t0)m−1∏k=1(Eα(−γ1(sk−tk)α)Eα(γ2(tk+1−sk)α))Eα(−γ1(sm−tm)α). | (3.14) |
For, sm≤t<tm+1,
V(t)≤V(s0)m−1∏k=1(Eα(−γ1(sk−tk)α)Eα(γ2(tk+1−sk)α))Eα(−γ1(sm−tm)α)Eα(γ2(t−sm)), | (3.15) |
so, one can get that
V(tm+1)≤V(s0)m−1∏k=1(Eα(−γ1(sk−tk)α)Eα(γ2(tk+1−sk)α))Eα(−γ1(sm−tm)α)Eα(γ2(tm+1−sm))=V(t0)m∏k=1(Eα(−γ1(sk−tk)α)Eα(γ2(tk+1−sk)α)). | (3.16) |
Suppose the Eqs (3.13)–(3.16) are hold. According to the principle of mathematical recursive reasoning, when tm+1≤t<sm+1, one can get
V(t)≤V(tm+1)(−γ1Eα(t−tm+1)α). | (3.17) |
One can derive
V(sm+1)≤V(t0)m∏k=1(Eα(−γ1(sk−tk)α)Eα(γ2(tk+1−sk)α))(−γ1Eα(sm+1−tm+1)α). | (3.18) |
For sm+1≤t<tm+2,
V(t)≤V(t0)m∏k=1(Eα(−γ1(sk−tk)α)Eα(γ2(tk+1−sk)α))Eα(−γ1(sm+1−tm+1)α)Eα(γ2(t−sm+1)α). | (3.19) |
Based on the Assumption 1, one can have that the lower bound of sk−tk is h and the upper bound of tk+1−tk is H. The upper bound of tk−sk is H−h. Therefore, one can obtain that:
For tm+1≤t<sm+1,
V(t)≤V(t0)m∏k=1(Eα(−γ1(h)α)Eα(γ2(H−h)α))Eα(−γ1(t−tm+1)α). | (3.20) |
For sm+1≤t<tm+2,
V(t)≤V(t0)(Eα(−γ1(h)α)mEα(γ2(H−h)α))mEα(−γ1(h)α)Eα(γ2(t−sm+1)α). | (3.21) |
From the condition (Eα(−γ1hα)mEα(γ2(H−h)α))m<1 and 0≤t−h−tm<H−h, we can derive that (Eα(−γ1hα)mEα(γ2(t−h−tk)α))m is bounded. So, one can obtain
limm→∞(Eα(−γ1(h)α)mEα(γ2(H−h)α))m=0. |
Then, limt→∞V(t)=0, the proof of theorem is over.
This completes the proof of Theorem.
Remark 1. In Theorem 1, by using aperiodically intermittent controller, synchronization problem is investigated. Based on the property of Mittag-Leffler function, through strict mathematical reasoning, we can derive V(t)→0 when t→∞. Therefore, we can get that the theorem holds.
Remark 2. Intermittent synchronization strategy has been studied [41] and [42]. In [41], synchronization via aperiodically intermittent strategy is first given in the form of a theory. Compared with [41] and [42], aperiodically intermittent strategy is more general. In Theorem 1, if the control interval tk−sk≡h and tk−sk≡H, aperiodically intermittent strategy is degraded to periodically intermittent control. That is to say, periodically intermittent control is a special form of aperiodically intermittent strategy. Aperiodically intermittent strategy owns more practical characteristics. In this case, a corollary (see Corollary 1) is given.
Remark 3. Synchronization of integer order dynamical systems via aperiodically intermittent control has been investigated. As far as we know, there are no works on fractional order. As is known to all, integral order derivative is a special case of fractional order derivative. When the fractional order derivative a = 1, the derived conclusion can be directly applied to the integral order works. That is to say, the works of integer order on aperiodically intermittent are a special case of Theorem 1.
Corollary 1. When tk−sk≡h and tk−tk+1≡J, the controller (2.5) is degenerated into
u(t)={−K(y(t)−x(t)),tk≤t<sk+h,0,sk+h≤t<tk+1. | (3.22) |
Controller (3.22) is rewritten as
u(t)={−K(y(t)−x(t)),kH≤t<kH+h,0,kH+h≤t<(k+1)H. | (3.23) |
Under the Lemmas 1–4, and Assumption 1, fractional-order master-system (2.1) and slave-systems (2.2) can achieve synchronization under controller (3.22) or (3.23), if
−||C||+||A||||L||<||K||, |
Eα(−γ1hα)Eα(γ2(H−h)α)<1, |
where, γ1=−||C||+||A||||L||−||K|| and γ2=−||C||+||A||||L|| are two positive scalers. All the parameters are the same as Theorem 1.
In this section, an example is given to verify the validity of the sufficient conditions derived by the Theorem 1.
Example 1. Considering three-neuron fractional-order MRNNs, the master-systems are as follows:
Dαy(t)=−Cx(t)+A(x(t))f(x(t))+I(t), | (4.1) |
where α=0.95, Ij(t)=(0,0,0),j=1,2,3
C=(300030003), |
fj(xj(t))=tanh(xj(t))(j=1,2,3), |
and
a11(x1(t))={2,|x1(t)|≤1,1.85,|x1(t)|>1,,a12(x2(t))={1.6,|x2(t)|≤1,1.5,|x2(t)|>1,,a13(x3(t))={−9,|x3(t)|≤1,−8.8,|x3(t)|>1,, |
a21(x1(t))={−9,|x1(t)|≤1,−8.8,|x1(t)|>1,,a22(x2(t))={2,|x2(t)|≤1,1.85,|x2(t)|>1,,a23(x3(t))={1.6,|x3(t)|≤1,1.5,|x3(t)|>1,, |
a31(x1(t))={1.6,|x1(t)|≤1,1.5,|x1(t)|>1,,a32(x2(t))={−9,|x2(t)|≤1,−8.8,|x2(t)|>1,,a33(x3(t))={2,|x3(t)|≤1,1.85,|x3(t)|>1. |
The slave-systems of MRNNs are considered as follows:
Dαy(t)=−Cy(t)+A(y(t))f(y(t))+I(t)+u(t), | (4.2) |
some parameters are given as follows: H=2,h=1.4, K=18, l1=l2=l3=1, one can get γ1=−||C||+||L||||A||−K<0, γ2=7.5357>0 Eα(−γ1hα)Eα(γ2(H−h)α)=0.5730<1. The state trajectories of x1(t),x2(t),x3(t) are depicted on Figure 5 with initial condition x(0)=[1,−2,3]T. Under the initial condition of y(0)=[−1,2,−3]T, Figure 6 depicts the errors of x(t) and y(t) without control. Figures 7–9 depict synchronization state trajectories of (x1(t),y1(t))), (x2(t),y2(t)) and (x3(t),y3(t)) with control. Each dimensional synchronization error is described in Figure 10. From synchronization states Figures 7–9, one can derive that master-slave systems can get synchronization with designed controller. Synchronization error Figure 10 also verifies the derived theory.
In this paper, by using aperiodically intermittent controller, the synchronization problem of fractional-order memristor-based recurrent neural networks is investigated. Although there have been some results about aperiodically intermittent control, those results are studied in integer domain. In the case of fractional calculus, as far as we know, there are only some works on periodically intermittent control. With reasonable assumptions and suitable lemmas, by means of Lyapunov method, synchronization is obtained via aperiodically intermittent control. A simulation example is presented to illustrate the validity of derived sufficient conditions which. Delays are widespread in dynamical systems. Different kinds of delay will have different effects on the dynamical system. It is of great significance to study the influence of delay on dynamical system. In this paper, system are considered without delay. Therefore, in the future, we consider the situation with delays. Furthermore, In addition, the selection of parameters usually depends on experience, resulting in high control costs. Therefore, in the future, optimization algorithm is considered to find the optimal parameter.
This work was jointly supported by the Natural Science Foundation of Jiangsu Province of China under Grant No. BK20170171, Postgraduate Research & Practice Innovation Program of Jiangsu Province under Grant No. KYCX18_1857, No. KYCX18_1858.
The authors declare there is no conflict of interest.
[1] |
L. Chua, Memrisor-the missing circuit element, IEEE Trans. Circuit Theory, 18 (1971), 507–519. https://doi.org/10.1109/TCT.1971.1083337 doi: 10.1109/TCT.1971.1083337
![]() |
[2] |
L. Chua, S. Kang, Memristive devices and systems. Proc. IEEE, 64 (1976), 209–223. https://doi.org/10.1109/PROC.1976.10092 doi: 10.1109/PROC.1976.10092
![]() |
[3] |
D. Strukov, G. Snider, D. Stewart, R. Williams, The missing memristor found, Nature, 453 (2008), 80–83. https://doi.org/10.1038/nature06932 doi: 10.1038/nature06932
![]() |
[4] |
J. Tour, T. He, Electronics: The fourth element, Nature, 453 (2008), 42–43. https://doi.org/10.1038/453042a doi: 10.1038/453042a
![]() |
[5] |
W. Mao, Y. Liu, L. Ding, A. Safian, X. Liang, A new structured domain adversarial neural network for transfer fault diagnosis of rolling bearings under different working conditions, IEEE Trans. Instrum. Meas., 70 (2021), 1–13. https://doi.org/10.1109/TIM.2020.3038596 doi: 10.1109/TIM.2020.3038596
![]() |
[6] |
S. Wang, Z. Dou, D. Chen, H. Yu, Y. Li, P. Pan, Multimodal multiclass boosting and its application to cross-modal retrieval, Neurocomputing, 357 (2019), 11–23. https://doi.org/10.1016/j.neucom.2019.05.040 doi: 10.1016/j.neucom.2019.05.040
![]() |
[7] |
W. Mao, J. Wang, Z. Xue, An ELM-based model with sparse-weighting strategy for sequential data imbalance problem, Int. J. Mach. Learn. Cybern., 8 (2017), 1333–1345. https://doi.org/10.1007/s13042-016-0509-z doi: 10.1007/s13042-016-0509-z
![]() |
[8] |
S. Zhang, Y. Yang, L. Li, D. Wu, Quasi-synchronization of fractional-order complex-valued memristive recurrent neural networks with switching jumps mismatch, Neural Process Lett., 53 (2021), 865–-891. https://doi.org/10.1007/s11063-020-10342-4 doi: 10.1007/s11063-020-10342-4
![]() |
[9] |
Y. Shi, J. Cao, G. Chen, Exponential stability of complex-valued memristor-based neural networks with time-varying delays, Appl. Math. Comput., 313 (2017), 222–234. https://doi.org/10.1016/j.amc.2017.05.078 doi: 10.1016/j.amc.2017.05.078
![]() |
[10] |
X. Yang, D. Ho, Synchronization of delayed memristive neural networks: robust analysis approach, IEEE Trans. Cybern., 46 (2016), 3377–3387. https://doi.org/10.1109/TCYB.2015.2505903 doi: 10.1109/TCYB.2015.2505903
![]() |
[11] |
G. Zhang, Z. Zeng, Exponential stability for a class of memristive neural networks with mixed time-varying delays, Appl. Math. Comput., 321 (2018), 544–554. https://doi.org/10.1016/j.amc.2017.11.022 doi: 10.1016/j.amc.2017.11.022
![]() |
[12] |
M. Mehrabbeik, F. Parastesh, J. Ramadoss, K. Rajagopal, H. Namazi, S. Jafari, Synchronization and chimera states in the network of electrochemically coupled memristive Rulkov neuron maps, Math. Biosci. Eng., 18 (2021), 9394–9409. https://doi.org/10.3934/mbe.2021462 doi: 10.3934/mbe.2021462
![]() |
[13] |
T. Dong, X. Gong, T. Huang, Zero-Hopf bifurcation of a memristive synaptic Hopfield neural network with time delay, Neural Networks, 149 (2022), 146–156. https://doi.org/10.1016/j.neunet.2022.02.009 doi: 10.1016/j.neunet.2022.02.009
![]() |
[14] |
X. Yang, J. Cao, J. Liang, Exponential synchronization of memristive neural networks with delays: interval matrix method, IEEE Trans. Neural Networks Learn. Syst., 28 (2017), 1878–1888. https://doi.org/10.1109/TNNLS.2016.2561298 doi: 10.1109/TNNLS.2016.2561298
![]() |
[15] |
Y. Shi, J. Cao, G. Chen, Exponential stability of complex-valued memristor-based neural networks with time-varying delays, Appl. Math. Comput., 313 (2017), 222–234. https://doi.org/10.1016/j.amc.2017.05.078 doi: 10.1016/j.amc.2017.05.078
![]() |
[16] |
G. Zhang, Z. Zeng, J. Hu, New results on global exponential dissipativity analysis of memristive inertial neural networks with distributed time-varying delays, Neural Networks, 97 (2018), 183–191. https://doi.org/10.1016/j.neunet.2017.10.003 doi: 10.1016/j.neunet.2017.10.003
![]() |
[17] |
A. Wu, Y. Chen, Z. Zeng, Multi-mode function synchronization of memristive neural networks with mixed delays and parameters mismatch via event-triggered control, Inf. Sci., 572 (2021), 147–166. https://doi.org/10.1016/j.ins.2021.04.101 doi: 10.1016/j.ins.2021.04.101
![]() |
[18] |
X. Yang, J. Cao, J. Qiu, pth moment exponential stochastic synchronization of coupled memristor-based neural networks with mixed delays via delayed impulsive control, Neural Networks, 65 (2015), 80–91. https://doi.org/10.1016/j.neunet.2015.01.008 doi: 10.1016/j.neunet.2015.01.008
![]() |
[19] |
L. Zhang, Y. Yang, F. Wang, Projective synchronization of fractional-order memristive neural networks with switching jumps mismatch, Phys. A, 471 (2017), 402–415. https://doi.org/10.1016/j.physa.2016.12.030 doi: 10.1016/j.physa.2016.12.030
![]() |
[20] |
J. Zhang, Z. Lou, Y. Jia, W. Shao, Ground state of Kirchhoff type fractional Schrödinger equations with critical growth, J. Math. Anal. Appl., 462 (2018), 57–83. https://doi.org/10.1016/j.jmaa.2018.01.060 doi: 10.1016/j.jmaa.2018.01.060
![]() |
[21] |
B. Łupińska, E. Schmeidel, Analysis of some Katugampola fractional differential equations with fractional boundary conditions, Math. Biosci. Eng., 18 (2021), 1–19. https://doi.org/10.3934/mbe.2021359 doi: 10.3934/mbe.2021359
![]() |
[22] |
J. Zhang, J. Wang, Numerical analysis for Navier–Stokes equations with time fractional derivatives, Appl. Math. Comput., 30 (2022), 2747–2758. https://doi.org/10.1016/j.amc.2018.04.036 doi: 10.1016/j.amc.2018.04.036
![]() |
[23] |
F. Wang, Y. Yang, Quasi-synchronization for fractional-order delayed dynamical networks with heterogeneous nodes, Appl. Math. Comput., 339 (2018), 1–14. https://doi.org/10.1016/j.amc.2018.07.041 doi: 10.1016/j.amc.2018.07.041
![]() |
[24] | C. Huang, J. Cao, M. Xiao, A. Alsaedi, T. Hayat, Bifurcations in a delayed fractional complex-valued neural network, Appl. Math. Comput., 292 (2017), 210–227. https://doi.org/0.1016/j.amc.2018.07.041 |
[25] |
L. Zhang, Y. Yang, F. Wang, Synchronization analysis of fractional-order neural networks with time-varying delays via discontinuous neuron activations, Neurocomputing, 275 (2018), 40–49. https://doi.org/10.1016/j.neucom.2017.04.056 doi: 10.1016/j.neucom.2017.04.056
![]() |
[26] |
C. Hu, H. Jiang, Special functions-based fixed-time estimation and stabilization for dynamic systems, IEEE Trans. Syst. Man Cybern., 5 (2022), 3251–3262. https://doi.org/10.1109/TSMC.2021.3062206 doi: 10.1109/TSMC.2021.3062206
![]() |
[27] |
S. Yang, C. Hu, J. Yu, H. Jiang, Finite-time cluster synchronization in complex-variable networks with fractional-order and nonlinear coupling, Neural Networks, 135 (2021), 212–224. https://doi.org/10.1016/j.neunet.2020.12.015 doi: 10.1016/j.neunet.2020.12.015
![]() |
[28] |
J. Fei, L. Liu, Real-time nonlinear model predictive control of active power filter using self-feedback recurrent fuzzy neural network estimator, IEEE Trans. Ind. Electron., 69 (2022), 8366–8376. https://doi.org/10.1109/TIE.2021.3106007 doi: 10.1109/TIE.2021.3106007
![]() |
[29] |
W. Sun, L. Peng, Observer-based robust adaptive control for uncertain stochastic Hamiltonian systems with state and input delays, Nonlinear Anal. Modell. Control, 19 (2014), 626–645. https://doi.org/10.15388/NA.2014.4.8 doi: 10.15388/NA.2014.4.8
![]() |
[30] |
S. Liu, J. Wang, Y. Zhou, M. Feckan, Iterative learning control with pulse compensation for fractional differential systems, Math. Slovaca, 68 (2018), 563–574. https://doi.org/10.1515/ms-2017-0125 doi: 10.1515/ms-2017-0125
![]() |
[31] |
M. Sabzalian, A. Mohammadzadeh, S. Lin, W. Zhang, Robust fuzzy control for fractional-order systems with estimated fraction-order, Nonlinear Dyn., 98 (2019), 2375–2385. https://doi.org/10.1007/s11071-019-05217-w doi: 10.1007/s11071-019-05217-w
![]() |
[32] |
Z. Wang, J. Fei, Fractional-order terminal sliding-mode control using self-evolving recurrent chebyshev fuzzy neural network for mems gyroscope, IEEE Trans. Fuzzy Syst., 30 (2022), 2747– 2758. https://doi.org/10.1109/TFUZZ.2021.3094717 doi: 10.1109/TFUZZ.2021.3094717
![]() |
[33] |
Y. Cao, S. Wang, Z. Guo, T. Huang, S. Wen, Synchronization of memristive neural networks with leakage delay and parameters mismatch via event-triggered control, Neural Networks, 119 (2019), 178–189. https://doi.org/10.1016/j.neunet.2019.08.011 doi: 10.1016/j.neunet.2019.08.011
![]() |
[34] |
X. Li, X. Yang, J. Cao, Event-triggered impulsive control for nonlinear delay systems, Automatica, 117 (2020), 108981. https://doi.org/10.1016/j.automatica.2020.108981 doi: 10.1016/j.automatica.2020.108981
![]() |
[35] |
X. Li, D. Peng, J. Cao, Lyapunov stability for impulsive systems via event-triggered impulsive control, IEEE Trans. Autom. Control, 65 (2020), 4908–4913. https://doi.org/10.1109/TAC.2020.2964558 doi: 10.1109/TAC.2020.2964558
![]() |
[36] |
H. Li, X. Gao, R. Li, Exponential stability and sampled-data synchronization of delayed complex-valued memristive neural networks, Neural Process Lett., 51 (2020), 193–209. https://doi.org/10.1007/s11063-019-10082-0 doi: 10.1007/s11063-019-10082-0
![]() |
[37] |
H. Fan, K. Shi, Y. Zhao, Global μ-synchronization for nonlinear complex networks with unbounded multiple time delays and uncertainties via impulsive control, Phys. A, 599 (2022), 127484. https://doi.org/10.1016/j.physa.2022.127484 doi: 10.1016/j.physa.2022.127484
![]() |
[38] |
X. Li, D. Ho, J. Cao, Finite-time stability and settling-time estimation of nonlinear impulsive systems, Automatica, 99 (2019), 361–368. https://doi.org/10.1016/j.automatica.2018.10.024 doi: 10.1016/j.automatica.2018.10.024
![]() |
[39] |
S. Yang, C. Hu, J. Yu, H. Jiang, Exponential stability of fractional-order impulsive control systems with applications in synchronization, IEEE Trans. Cybern., 50 (2020), 3157–3168. https://doi.org/10.1109/TCYB.2019.2906497 doi: 10.1109/TCYB.2019.2906497
![]() |
[40] |
H. Fan, K. Shi, Y. Zhao, Pinning impulsive cluster synchronization of uncertain complex dynamical networks with multiple time-varying delays and impulsive effects, Phys. A, 587 (2022), 126534. https://doi.org/10.1016/j.physa.2021.126534 doi: 10.1016/j.physa.2021.126534
![]() |
[41] |
F. Wang, Y. Yang, Intermittent synchronization of fractional order coupled nonlinear systems based on a new differential inequality, Phys. A, 512 (2018), 142–152. https://doi.org/10.1016/j.physa.2018.08.023 doi: 10.1016/j.physa.2018.08.023
![]() |
[42] |
L. Zhang, Y. Yang, F. Wang, Lag synchronization for fractional-order memristive neural networks via period intermittent control, Nonlinear Dyn., 89 (2017), 367–381. https://doi.org/10.1007/s11071-017-3459-4 doi: 10.1007/s11071-017-3459-4
![]() |
[43] |
C. Hu, H. He, H. Jiang, Synchronization of complex-valued dynamic networks with intermittently adaptive coupling: A direct error method, Automatica, 112 (2020), 108675. https://doi.org/10.1016/j.automatica.2019.108675 doi: 10.1016/j.automatica.2019.108675
![]() |
1. | Taiyan Jing, Tongyang He, Synchronization of Fractional-order Neural Networks via Intermittent Quantized Control: Optimal Algorithm, 2024, 63, 1572-9575, 10.1007/s10773-024-05701-z | |
2. | Jianbing Hu, Shuguang Li, Zhe Jin, Xiaochao Chao, Studying the transient process of an intermittent control system from its response property, 2024, 139, 10075704, 108309, 10.1016/j.cnsns.2024.108309 | |
3. | Pan Wang, Xuechen Li, Qianqian Zheng, Synchronization of inertial complex-valued memristor-based neural networks with time-varying delays, 2024, 21, 1551-0018, 3319, 10.3934/mbe.2024147 | |
4. | Saravanan Shanmugam, R. Vadivel, S. Sabarathinam, P. Hammachukiattikul, Nallappan Gunasekaran, Enhancing synchronization criteria for fractional-order chaotic neural networks via intermittent control: an extended dissipativity approach, 2025, 5, 2767-8946, 31, 10.3934/mmc.2025003 |