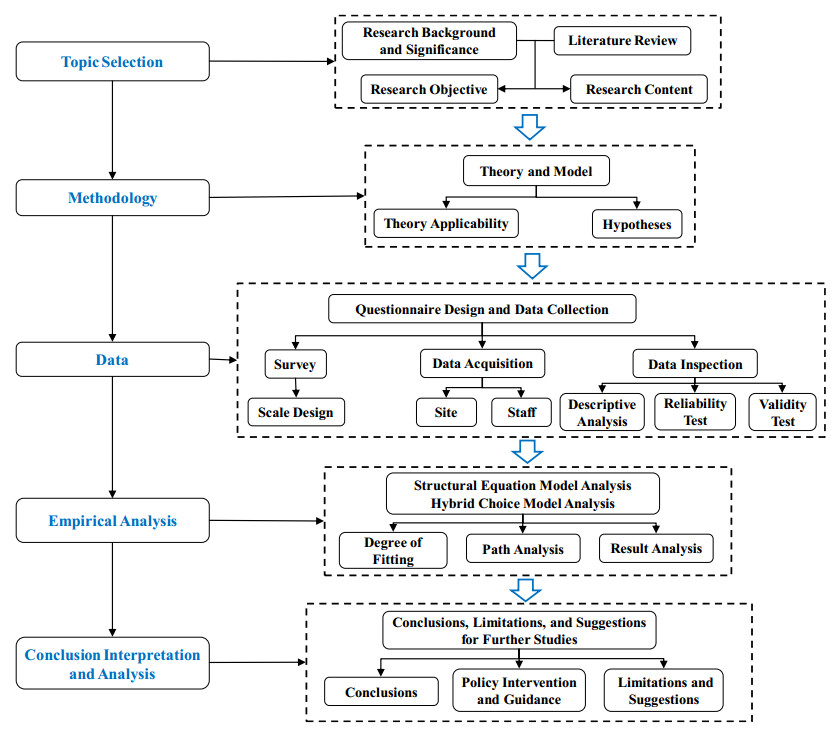
Based on the theory of planned behavior (TPB) and the protection motivation theory (PMT), this study examines the factors that influence parental choice of school travel mode during COVID-19. Structural equation modeling (SEM) and a hybrid choice model (HCM) are used to analyze this decision-making process. The results show that trust, perceived severity, perceived vulnerability, perceived built environment, attitude, subjective norm, and perceived behavioral control are significant factors. Perceived severity, perceived vulnerability, and the evaluation of pandemic risk, significantly impact the choice of public transit and private car, but not walking. Perceived built environment is the most critical factor influencing the choice of walking. The results provide a theoretical basis and reference for relevant government departments to formulate policies and measures during COVID-19.
Citation: Shuang Zhang, Peng Jing, Daibiao Yuan, Chenlu Yang. On parents' choice of the school travel mode during the COVID-19 pandemic[J]. Mathematical Biosciences and Engineering, 2022, 19(9): 9412-9436. doi: 10.3934/mbe.2022438
[1] | Ayako Suzuki, Hiroshi Nishiura . Transmission dynamics of varicella before, during and after the COVID-19 pandemic in Japan: a modelling study. Mathematical Biosciences and Engineering, 2022, 19(6): 5998-6012. doi: 10.3934/mbe.2022280 |
[2] | Adil Yousif, Awad Ali . The impact of intervention strategies and prevention measurements for controlling COVID-19 outbreak in Saudi Arabia. Mathematical Biosciences and Engineering, 2020, 17(6): 8123-8137. doi: 10.3934/mbe.2020412 |
[3] | Masaya Mori, Yuto Omae, Yohei Kakimoto, Makoto Sasaki, Jun Toyotani . Analyzing factors of daily travel distances in Japan during the COVID-19 pandemic. Mathematical Biosciences and Engineering, 2024, 21(8): 6936-6974. doi: 10.3934/mbe.2024305 |
[4] | Sarafa A. Iyaniwura, Rabiu Musa, Jude D. Kong . A generalized distributed delay model of COVID-19: An endemic model with immunity waning. Mathematical Biosciences and Engineering, 2023, 20(3): 5379-5412. doi: 10.3934/mbe.2023249 |
[5] | Fen-fen Zhang, Zhen Jin . Effect of travel restrictions, contact tracing and vaccination on control of emerging infectious diseases: transmission of COVID-19 as a case study. Mathematical Biosciences and Engineering, 2022, 19(3): 3177-3201. doi: 10.3934/mbe.2022147 |
[6] | Fang Wang, Lianying Cao, Xiaoji Song . Mathematical modeling of mutated COVID-19 transmission with quarantine, isolation and vaccination. Mathematical Biosciences and Engineering, 2022, 19(8): 8035-8056. doi: 10.3934/mbe.2022376 |
[7] | Wentao Hu, Yufeng Shi, Cuixia Chen, Ze Chen . Optimal strategic pandemic control: human mobility and travel restriction. Mathematical Biosciences and Engineering, 2021, 18(6): 9525-9562. doi: 10.3934/mbe.2021468 |
[8] | Rafiqul Chowdhury, Gary Sneddon, M. Tariqul Hasan . Analyzing the effect of duration on the daily new cases of COVID-19 infections and deaths using bivariate Poisson regression: a marginal conditional approach. Mathematical Biosciences and Engineering, 2020, 17(5): 6085-6097. doi: 10.3934/mbe.2020323 |
[9] | Qian Shen . Research of mortality risk prediction based on hospital admission data for COVID-19 patients. Mathematical Biosciences and Engineering, 2023, 20(3): 5333-5351. doi: 10.3934/mbe.2023247 |
[10] | Tao Chen, Zhiming Li, Ge Zhang . Analysis of a COVID-19 model with media coverage and limited resources. Mathematical Biosciences and Engineering, 2024, 21(4): 5283-5307. doi: 10.3934/mbe.2024233 |
Based on the theory of planned behavior (TPB) and the protection motivation theory (PMT), this study examines the factors that influence parental choice of school travel mode during COVID-19. Structural equation modeling (SEM) and a hybrid choice model (HCM) are used to analyze this decision-making process. The results show that trust, perceived severity, perceived vulnerability, perceived built environment, attitude, subjective norm, and perceived behavioral control are significant factors. Perceived severity, perceived vulnerability, and the evaluation of pandemic risk, significantly impact the choice of public transit and private car, but not walking. Perceived built environment is the most critical factor influencing the choice of walking. The results provide a theoretical basis and reference for relevant government departments to formulate policies and measures during COVID-19.
The outbreak of the COVID-19 pandemic brought an unprecedented impact on the urban transportation system. Especially in regards to school travel, parents shifted from public transit to private cars, thus exacerbating urban traffic congestion. Recently, more consideration has been given to school travel modes used by school children. Over the past few decades, the number of children in China that walk or take public transit to school has decreased, while traveling to school by car has increased [1].
In 2020, the World Health Organization declared COVID-19 a global pandemic [2]. Passengers terrified by COVID-19 began to distrust public transit because of the risk of infection from mixing with asymptomatic infected passengers [3]. Individuals with this concern tend to pay more attention to risks and are more susceptible to believing rumors and inaccurate information [4]. The panic caused by COVID-19 increased the use of private cars for transportation to and from school. The public's trust in public transit, especially from parents, quickly turned to worry and fear. Preference for private cars increased dependency, aggravated urban traffic congestion, increased air pollution and the risk of accidents [5]. These all affected children's physical and mental health.
It has become a top priority of the government and relevant departments to reduce these travel-related anxieites from parents and rebuild confidence in public transit to relieve urban traffic burdens caused by the increae in the use of private cars. This study aimed to study the influencing factors and internal mechanisms in China of parental choice of public transit, private car, and walking during COVID-19.
This study established a theoretical framework based on data collected from a survey. The framework integrated the theory of planned behavior (TPB) and the protective motivation theory (PMT). Two additional factors were also introduced: perceived built environment and trust. Structural equation model (SEM) was used to construct a model of parental choice of school travel mode during COVID-19, and a hybrid choice model (HCM) was used to analyze the internal mechanism of parental choice regarding school travel mode. The unique contributions of this study are as follows: (1) this study is the first to examine the behavioral intentions and influencing factors of parental choice regarding school travel mode during COVID-19 (2) integrated TPB with PMT to verify the applicability of an integrated model regarding choice of school travel mode during COVID-19 (3) used the original influencing factors in the theory and incorporated perceived built environment and trust in the study of school travel. Perceived severity and perceived vulnerability, which represent parental assessment of pandemic risk, were added to expand the existing theoretical model (4) combined SEM and HCM to analyze the influence of the several variables on latent variables and the influence of various factors on travel mode choice. The research results provide a valuable reference for the application and development of research in travel behavior and related fields.
The remainder of this paper is structured as follows. Sections 1.2 and 1.3 provide a literature review, and Section 2 describes the research methods. Section 3 describes the results of the analyses. Section 4 discusses the results and models. Section 5 discusses the conclusions, limitations, and potential opportunities for future studies. The research conception and technological structure are shown in Figure 1.
Most of the literature on school travel behavior has been primarily focused on the influencing factors of school travel mode choice in elementary and middle schools. Travel attributes, socioeconomic variables, and built environment are the most commonly studied factors in this field. Psychological factors have been rarely considered. Among the factors that influence school travel mode choice, integrating multiple factors is receiving more attention. For example, Berg et al. [6] incorporated psychological factors (trust, travel satisfaction), socioeconomic characterisitics (age, gender, family income), and travel attributes (distance, accompany or not) into a unified model to examine different factors on school travel. Mindell et al. [7] studied the influence of public transit in school travel, aiming to promote the use of green travel, such as public transit. These studies incorporated psychological-based latent variables with social-economic characteristics, built environment, travel attributes and other observed variables. The results found that these factors significantly impact school travel mode choice. However, most of the psychological variables in previous research were examined independently. It is impossible to determine the combined effect of these latent variables on actual travel choice. School travel choice is a complex matter, therefore it is necessary to utilize a theoretical model that is more comprehensive. This study constructed a more complex theoretical model to propose a more thorough understanding of school travel choice.
Since the beginning of 2020, COVID-19 swept the world and greatly impacted transportation systems in nearly all countries. Research on transportation during COVID-19 has begun in China and abroad. As shown in Table 1, this study summarized and analyzed the existing research literature regarding the research object, travel mode, influential factors, theoretical models and research methods.
Year of publication | Research object | Travel mode | Influential factors | Theoretical model | Research method |
Khaddar (2021) | Commuter | Public transit Private car Walk | Socioeconomic attribute variable Travel attribute variable | - | Ordered logit model |
Vickerman (2021) | Commuter | Public transit | - | - | Qualitative analysis |
Chang (2021) | Commuter | Public transit | Socioeconomic attribute variable Travel attribute variable | - | Variance analysis |
Zheng (2020) | Commuter | Public transit | Psychological latent variable | PMT | SEM |
Dong (2021) | Commuter | Public transit | Psychological latent variable | - | SEM |
Bergantino (2020) | Commuter | Public transit | Socioeconomic attribute variable Travel attribute variable psychological latent variable | - | Ordered logit model |
Parady (2020) | Commuter | Public transit | Socioeconomic attribute variable Psychological latent variable | - | Logit model |
Zenker (2021) | Commuter | Public transit | Socioeconomic attribute variable Psychological latent variable | - | SEM |
Anwari (2021) | Commuter | Public transit | Socioeconomic attribute variable Travel attribute variable | - | Logistic regression model |
Scorrano (2021) | Commuter | Public transit Private car Walk | Socioeconomic attribute variable psychological latent variable | TPB | Hybrid logit model |
Ozbilen (2021) | Commuter | Public transit Private car Shared travel | Socioeconomic attribute variable Psychological latent variable | - | Binomial logit model |
Beck (2020) | Commuter | Public transit Private car | Socioeconomic attribute variable Travel attribute variable Psychological latent variable | - | Ordered logit model |
Dai (2021) | Commuter | Public transit | - | - | Synthetic control method |
Basu (2021) | Commuter | Public transit Shared travel | - | - | Qualitative analysis |
Teixeira (2020) | Commuter | Public transit Shared travel | Travel attribute variable | - | GIS |
Regarding the research object, most existing studies have focused on commuters and explored the impact of COVID-19 on commuter choice of travel mode. Khaddar and Fatmi [8] used the ordered logit model to study the effect of commuter socioeconomic status and travel characteristics on travel. Bergantino, Intini, and Tangari [9] found that commuter perception of urban infrastructure construction and active travel were essential factors affecting the change of Italian residents from public transit to private car during the pandemic. However, existing studies rarely examined the impact of COVID-19 on school travel choice. With disease or pollution, children have greater vulnerability than other populations [10]. Although the government has taken measures to ensure public transit safety under COVID-19, parental anxiety about security will be higher than the actual level of danger.
Existing research can be divided into three categories: studies that only included observed variables, those that only incuded psychological latent variables, and those that combined observable variables and psychological latent variables. Beck, Hensher, and Wei [11] introduced socioeconomic characteristics, travel attributes and psychological latent variables into a travel mode choice model. Scorrano and Danielis [12] integrated TPB and established a hybrid logit model to verify the feasibility of a model for travel mode during the pandemic. However, their study did not consider the influence of travel attributes on mode choice. In addition, in reference to psychological latent variables, only the traditional TPB was used and expanded, which may cause the study's results to greatly deviate from the actual situation.
In general, the following conclusions can be drawn from the relevant literature.
(1) Although many researchers have studied the influence of psychological latent variables, social economy, built environment, travel attributes, and other variables on school travel, few studies have proposed a theoretical model that integrates psychological factors.
(2) Few studies have integrated socioeconomic characteristics, travel attributes and latent psychological variables into a comprehensive theoretical framework, and few studies have expanded psychological theory to study parental choice of school travel mode during COVID-19.
The purpose of using TPB was to study whether an individual's intention to conduct a certain behavior will affect the actual behavior. TPB postulates that perceived behavior control, attitude and subjective norms impact behavioral intentions simultaneously, and also have reciprocal influences. Perceived behavior control may directly affect actual behavior under certain circumstances.
Researchers have found that in the decision-making process of travel mode choice, individual socioeconomic characteristics (e.g., gender, age, economic level, cultural background) and travel attributes (e.g., travel distance, travel costs) will impact travel mode choice. At the same time, psychological characteristics of travelers in the decision-making process also impact the results of the choice of travel mode. TPB has shown good explanatory and predictive power in travel behavior.
School travel is a specific type of travel behavior. Parents are the main decision-makers and their choice determines their children's school travel mode. Parents have different psychological considerations and concerns when choosing among different travel modes. Under existing traffic conditions, traffic congestion and chaos during the peak attendance periods have put enormous pressure on the school's surrounding areas. Perception of the environment around the school (i.e., perceived built environment) will make parents more inclined to use a private car and forego school travel by public transport [13]. Perceived severity and perceived vulnerability are additional factors that parents consider when making decisions regarding school travel. In the context of COVID-19, parental fear of the pandemic may lead them to choose transport to and from the school that feels safer.
To summarize, this study used perceived behavior control, attitude, and subjective norm in TPB. It added perceived built environment, trust, perceived severity and perceived vulnerability in PMT to expand the model. The model's framework is shown in Figure 2.
Based on existing research, this study expanded the original TPB. The assumptions of influencing factors proposed by the model are shown in Table 2.
Hypotheses | Assumption |
H1a | Under COVID-19, Subjective Norm has a negative impact on parents' Intention to choose public transit. |
H1b | Under COVID-19, Subjective Norm has a positive impact on parents' Intention to choose public transit. |
H1c | Under COVID-19, Subjective Norm has a positive impact on parents' Intention to choose walk. |
H2a | Under COVID-19, Attitude has a negative impact on parents' Intention to choose public transit. |
H2b | Under COVID-19, Attitude has a positive impact on parents' Intention to choose private car. |
H2c | Under COVID-19, Attitude has a positive impact on parents' Intention to choose walk. |
H3a | Under COVID-19, Perceived Behavioral Control has a negative impact on parents' Intention to choose public transit. |
H3b | Under COVID-19, Perceived Behavioral Control has a positive impact on parents' Intention to choose private car. |
H3c | Under COVID-19, Perceived Behavioral Control has a positive impact on parents' Intention to choose walk. |
H4a | Under COVID-19, Trust has a negative impact on parents' Subjective Norm of choosing public transit. |
H4b | Under COVID-19, Trust has a positive impact on parents' Subjective Norm of choosing private car. |
H4c | Under COVID-19, Trust has a positive impact on parents' Subjective Norm of choosing walk. |
H5a | Under COVID-19, Trust has a negative impact on parents' Attitude of choosing public transit. |
H5b | Under COVID-19, Trust has a positive impact on parents' Attitude of choosing private car. |
H5c | Under COVID-19, Trust has a positive impact on parents' Attitude of choosing walk. |
H6a | Under COVID-19, Trust has a negative impact on parents' Perceived Behavior Control of choosing public transit. |
H6b | Under COVID-19, Trust has a positive impact on parents' Perceived Behavior Control of choosing private car. |
H6c | Under COVID-19, Trust has a positive impact on parents' Perceived Behavior Control of choosing walk. |
H7a | Under COVID-19, Trust has a negative impact on parents' Intention to choose public transit. |
H7b | Under COVID-19, Trust has a positive impact on parents' Intention to choose private car. |
H7c | Under COVID-19, Trust has a positive impact on parents' Intention to choose walk. |
H8a | Under COVID-19, Perceived Built Environment has a positive impact on parents' Intention to choose public transit. |
H8b | Under COVID-19, Perceived Built Environment has a negative impact on parents' Intention to choose private car. |
H8c | Under COVID-19, Perceived Built Environment has a positive impact on parents' Intention to choose private car. |
H9a | Under COVID-19, Perceived Built Environment has a positive impact on parents' Attitude of choosing public transit. |
H9b | Under COVID-19, Perceived Built Environment has a negative impact on parents' Attitude of choosing private car. |
H9c | Under COVID-19, Perceived Built Environment has a positive impact on parents' Attitude of choosing walk. |
H10a | Under COVID-19, Perceived Severity has a negative impact on parents' Intention to choose public transit. |
H10b | Under COVID-19, Perceived Severity has a positive impact on parents' Intention to choose private car. |
H10c | Under COVID-19, Perceived Severity has a negative impact on parents' Intention to choose walk. |
H11a | Under COVID-19, Perceived Severity has a negative impact on parents' Attitude of choosing public transit. |
H11b | Under COVID-19, Perceived Severity has a positive impact on parents' Attitude of choosing private car. |
H11c | Under COVID-19, Perceived Severity has a negative impact on parents' Attitude of choosing walk. |
H12a | Under COVID-19, Perceived Vulnerability has a negative impact on parents' Intention to choose public transit. |
H12b | Under COVID-19, Perceived Vulnerability has a positive impact on parents' Intention to choose private car. |
H12c | Under COVID-19, Perceived Vulnerability has a negative impact on parents' Intention to choose walk. |
H13a | Under COVID-19, Perceived Vulnerability has a negative impact on parents' Attitude of choosing public transit. |
H13b | Under COVID-19, Perceived Vulnerability has a positive impact on parents' Attitude of choosing private car. |
H13c | Under COVID-19, Perceived Vulnerability has a negative impact on parents' Attitude of choosing walk. |
This study attempted to establish a theoretical model on the choice between three school travel modes: public transit, private car, and walking. Figure 3 shows the choice intention model for the public transit mode. Private car and walking modes are shown in Appendix A.
Structural equation model is a multivariate statistical method that establishes the relationship between variables by examing the covariance among the proposed variables [14]. SEM is a linear equation system composed of multiple unobservable and observable variables. SEM relies heavily upon a theoretical construction and is only meaningful if it has a theoretical basis. SEM analysis is an extension of multiple regression and factor analysis, which can be combined to simultaneously analyze the causal relationships between all latent and observed variables.
Individuals are affected by various factors in the decision-making process of travel mode, such as the individual socioeconomic characteristics and travel mode attributes [15]. HCM incorporates latent psychological variables into the discrete choice model (DCM). The psychological variables involved in HCM to explain travel intention and heterogeneity between individuals were measured using the latent variables in SEM.
Compared with the traditional DCM, HCM considers more comprehensive factors. HCM associates latent psychological variables with observed variables (e.g., socioeconomic characteristics) relevant to decision-makers and random error terms used to account for data noise. HCM combines the advantages of DCM and SEM to enhance the interpretative ability of the model.
The survey used a 7-point Likert scale to measure the factors that affect parental intention to choose various travel modes. This study conducted a 6-day survey, from January 11, 2021 to January 16, 2021. Based on the principle of random distribution, a questionnaire was administered in several commercial complexes, including Suning Plaza, Wanda Plaza, and Wuyue Plaza in Zhenjiang City. The passenger flow of these large-scale commercial complexes can help ensure that the sample was representative of the general population. After a brief introduction, we asked participants to complete the questionnaire if they agreed to continue. A total of 503 questionnaires were collected. After data cleaning, 460 valid questionnaire responses were retained. The socio-demographic information is listed in Table 3.
Demographic variables | Sample size | Percentage (%) | |
Gender | Men | 217 | 47.17 |
Women | 243 | 52.83 | |
Age | 18–25 | 30 | 6.52 |
26–30 | 77 | 16.74 | |
31–35 | 154 | 33.48 | |
36–40 | 88 | 19.13 | |
41–45 | 56 | 12.17 | |
46–50 | 30 | 6.52 | |
Older than 50 | 25 | 5.44 | |
Education level | Primary (elementary/middle school) | 18 | 3.91 |
Secondary (high school) | 35 | 7.61 | |
Junior college | 108 | 23.48 | |
University (undergraduate) | 260 | 56.52 | |
Postgraduate | 39 | 8.48 | |
Family monthly income (RMB) | < 5000 | 10 | 2.01 |
5001–10,000 | 117 | 25.48 | |
10,001–15,000 | 123 | 26.74 | |
15,001–20,000 | 132 | 28.65 | |
> 20,000 | 78 | 17.12 | |
Occupation | Private enterprise | 197 | 42.83 |
Joint venture /foreign-invested enterprises | 32 | 6.96 | |
State-owned enterprises | 67 | 14.56 | |
Non-profit organization/institution | 3 | 0.65 | |
Government agencies | 26 | 5.65 | |
Schools, research institutes and other academic institutions | 51 | 11.09 | |
Other | 84 | 18.26 | |
Driver's license | Yes | 355 | 77.17 |
No | 105 | 22.83 | |
Number of car ownership | 0 | 54 | 11.74 |
1 | 294 | 63.91 | |
2 | 101 | 21.96 | |
3 | 11 | 2.39 | |
Number of electric bicycles owned | 0 | 107 | 23.26 |
1 | 196 | 42.61 | |
2 | 126 | 27.39 | |
3 | 23 | 5 | |
Number of children (<18 years old) | 1 | 386 | 83.91 |
2 | 68 | 14.78 | |
3 | 5 | 1.09 | |
4 | 1 | 0.22 | |
Child Gender | Boy | 270 | 58.7 |
Girl | 190 | 41.3 | |
Child age | 6–11 | 315 | 68.48 |
12–4 | 68 | 14.78 | |
15–18 | 77 | 16.74 | |
Distance from school to home | Less than 0.5 km | 68 | 14.78 |
0.5 km–1.0 km | 105 | 22.83 | |
1.0 km–-1.5 km | 77 | 16.74 | |
1.5 km–2.0 km | 50 | 10.87 | |
2.0 km–2.5 km | 58 | 12.61 | |
2.5 km–3.0 km | 25 | 5.43 | |
More than 3.0 km | 77 | 16.74 |
This study evaluated the reliability of the psychological variables. Cronbach's alpha (α) and composite reliability (C. R.) were used to assess reliability. Cronbach's alpha is a measure of internal consistency that indicates how related items are in the measure. The formula for Cronbach's alpha is:
α=nn−1(σ2X−∑σ2i)/σ2X | (1) |
Where n is the number of items, σ2X is the total test score variance, andσ2i is the item variance.
C.R. can be thought of as being equal to the total amount of true score variance relative to the total scale score variance. The formula is:
C.R.=(∑λi)2(∑λi)2+∑var(δi) | (2) |
Whereλi is completely standardized loading for the i-th indicator, and var (δi) is the variance of the error term for the i-th indicator.
As presented in Table 4, the value of α ranged from 0.718 to 0.892, which is acceptable internal consistency. The value of C.R. ranged from 0.712 to 0.908, higher than the minimum standard, suggesting a satisfactory estimation.
Psychological variables | N of Items | α | C.R. |
Perceived Built Environment | 6 | 0.772 | 0.877 |
Perceived Severity | 3 | 0.783 | 0.872 |
Perceived Vulnerability | 3 | 0.851 | 0.896 |
Subjective Norm | 3/3/3 | 0.776/0.786/0.779 | 0.898/0.838/0.703 |
Perceived Behavior Control | 2/2/2 | 0.732/0.745/0.718 | 0.815/0.880/0.833 |
Trust | 2/2/2 | 0.857/0.764/0.892 | 0.795/0.908/0.712 |
Attitude | 2/2/2 | 0.891/0.839/0.890 | 0.892/0.7854/0.807 |
Intention | 3/3/3 | 0.824/0.854/0.823 | 0.879/0.893/0.870 |
Note: α < 0.6, unacceptable reliability; 0.6≤α < 0.7, acceptable reliability; 0.7≤α < 0.8, high reliability; 0.8≤α < 0.9, very high reliability; α≥0.9, extremely high reliability. CR > 0.6, good reliability. |
Validity refers to the degree that a scale (or survey) measures what it is supposed to measure. Higher validity suggests that the measurement tool is an accurate reflection of the construct being measured. Validity can be assessed by calculating the average variance extracted (AVE) and normalized factor loading coefficient. AVE is used to assess the relationship between the total variation of the observed variable and the potential structural variation. The average variance extracted is calculated as follows:
AVE=∑λi2∑λi2+∑var(δi) | (3) |
Where λi is the standardized loading for the i-th indicator, and var (δi) is the variance of the error term for the i-th indicator.
As shown in Table 5, AVE exceeded the critical value of 0.5, and the normalized factor loading coefficient was greater than 0.7, which indicates that the questionnaire used in the model has good convergent validity.
Psychological variables | Observed variables | Normalization factor loading coefficient | AVE |
Perceived Built Environment | PBE1 | 0.847 | 0.703 |
PBE2 | 0.855 | ||
PBE3 | 0.813 | ||
PBE4 | 0.863 | 0.6863 | |
PBE5 | 0.841 | ||
PBE6 | 0.779 | ||
Perceived Severity | PS1 | 0.838 | 0.6951 |
PS2 | 0.84 | ||
PS3 | 0.823 | ||
Perceived Vulnerability | PV1 | 0.891 | 0.7415 |
PV2 | 0.865 | ||
PV3 | 0.826 | ||
Subjective Norm | SN1_pt / SN1_car / SN1_walk | 0.851/0.822/0.839 | 0.7467/0.633/0.877 |
SN2_pt / SN2_car / SN2_walk | 0.886/0.754/0.839 | ||
SN3_pt / SN3_car / SN3_walk | 0.855/0.809/0.837 | ||
Perceived Behavior Control | PBC1_pt / PBC1_car / PBC1_walk | 0.843/0.886/0.907 | 0.6874/0.785/0.909 |
PBC2_pt / PBC2_car / PBC2_walk | 0.815/0.872/0.918 | ||
Trust | TRUST1_pt / TRUST1_car / TRUST1_walk | 0.813/0.901/0.871 | 0.6593/0.8319/0.832 |
TRUST2_pt / TRUST2_car / TRUST2_walk | 0.811/0.924/0.817 | ||
Attitude | ATT1_pt / ATT1_car / ATT1_walk | 0.893/0.822/0.904 | 0.8046/0.6467/0.893 |
ATT2_pt / ATT2_car / ATT2_walk | 0.901/0.786/0.893 | ||
Intention | INT1_pt / INT1_car / INT1_walk | 0.824/0.842/0.796 | 0.706/0.736/0.670 |
INT2_pt / INT2_car / INT2_walk | 0.857/0.875/0.837 | ||
INT3_pt / INT3_car / INT3_walk | 0.842/0.856/0.857 | ||
Note: AVE > 0.5, good convergent validity; normalization factor loading coefficient > 0.5, good convergent validity. |
Based on the correlation between the latent variables and their assumptions, a framework was built for SEM analysis. The box in the model is the observed variable, the ellipse is the latent variable, the circle is the error term, the solid line represents the causal relationship between the latent variables, and the dotted line represents the relationship between the latent variable and the observed variable. Since the choice of school travel mode during the pandemic involves three modes, this study established a separate SEM for each of the three travel modes. It substituted the data into the model to compare the fit. Figure 4 is a diagram of the SEM for public transit travel mode. Since the latent variables contained in the three travel modes were the same, the private car and walking models were similar and can be found in Appendix B.
Path analysis was conducted using Mplus. The data fit were obtained as shown in Tables 6 and 7. To test the fit of the structural model based on empirical research, the following six indicators were tested to verify the suitability of the model: χ2/df, root-mean-square error (RMSEA), incremental fit index (IFI), goodness of fit index (GFI), normed fit index (NFI), and comparative fit index (CFI). If the RMSEA was < 0.05, IFI, GFI, NFI, and CFI exceeds 0.90, and the χ2/df value was < 3, it indicates that the model is acceptable. The results of χ2/df, RMSEA, IFI, GFI, NFI, and CFI all met the standards and are presented in Table 6, indicating that the theoretical model obtained an excellent fit.
Index name | χ2/df | RMSEA | IFI | GFI | NFI | CFI |
Test critical value | < 3, Good | < 0.05, Excellent | > 0.90 | > 0.90 | > 0.90 | > 0.90 |
< 5, Accepted | 0.05-0.08, Good | |||||
Model parameter value (public transit) | 4.48 | 0.062 | 0.917 | 0.902 | 0.900 | 0.907 |
Model parameter value (private car) | 3.97 | 0.059 | 0.921 | 0.916 | 0.908 | 0.914 |
Model parameter value (walk) | 3.69 | 0.070 | 0.911 | 0.904 | 0.901 | 0.900 |
Null hypotheses | Path | Standardized Regression Coefficient (β) | Standard Error of Estimate (S.E.) | Significance (p) |
H1a | SN_pt→INT_pt | 0.152 | 0.060 | p < 0.05 |
H2a | ATT_pt→INT_pt | 0.471 | 0.046 | p < 0.01 |
H3a | PBC_pt→INT_pt | 0.163 | 0.051 | p < 0.01 |
H4a | TRUST_pt→SN_pt | 0.162 | 0.061 | p < 0.01 |
H5a | TRUST_pt→ATT_pt | 0.241 | 0.068 | p < 0.01 |
H6a | TRUST_pt→PBC_pt | 0.183 | 0.036 | p < 0.01 |
H7a | TRUST_pt→INT_pt | 0.083 | 0.048 | - |
H8a | PBE_pt→INT_pt | 0.174 | 0.057 | p < 0.01 |
H9a | PBE_pt→ATT_pt | 0.083 | 0.047 | p < 0.01 |
H10a | PS_pt→ATT_pt | -0.276 | 0.052 | p < 0.01 |
H11a | PS_pt→INT_pt | -0.227 | 0.039 | p < 0.01 |
H12a | PV_pt→INT_pt | -0.311 | 0.066 | p < 0.01 |
H13a | PV_pt→ATT_pt | -0.214 | 0.046 | p < 0.01 |
This study used significance (p) as the test criteria of the hypotheses. A p < 0.05 indicates that the null hypothesis could be rejected. As shown in Figure 5 and Table 7, all hypotheses are supported, except H7a. Attitude (ATT, β = 0.471, p < 0.01), perceived severity (PS, β = -0.227, p < 0.01), and perceived vulnerability (PV, β = -0.311, p < 0.01) had the most significant effect on intention of choosing public transit as a travel mode. The impact of perceived behavior control (PBE, β = 0.174, p < 0.01) on parental intention was relatively weak. This also indicated that, compared with perceived built environment, parents were more concerned about whether their children will be affected by COVID-19 during school travel. Additionally, there were many other significant effects on intention, including subjective norm (SN, β = 0.152, p < 0.05) and perceived behavior vontrol PBC, β = 0.163, p < 0.01.).
Trust had a significant impact on subjective norm (SN, β = 0.162, p < 0.01), attitude (ATT, β = 0.241, p < 0.01), and perceived behavior control (PBC, β = 0.183, p < 0.01). Parental trust in public transit affected attitudes towards choosing public transit. In addition, attitude was influenced by perceived severity (PS, β = -0.276, p < 0.01) and perceived vulnerability (PV, β = -0.214, p < 0.01) negatively, while perceived built environment (PBE, β = 0.083, p < 0.01) had a positive impact on attitude.
In addition, this study established two other models for school travel mode choice during COVID-19: private car and walking travel modes. The results of these analyses are shown in Appendix C.
People generally pursue utility maximization in the decision-making process of travel mode choice. Considering the objective based on stochastic utility maximization, DCM was used to express travel choice behavior, which is described as:
Umj=εmj+∑hjlOVjlm | (4) |
Where Umj is the utility of decision-maker m choosing travel mode j, and εmj is the error term. In the model, OVjlm is the l-th significant variable in the j-th travel mode, hjl represents the normalized factor loading coefficient of the l-th significant variable in the j-th travel mode.
The SEM was integrated into the traditional DCM, and the HCM was obtained. The utility function Umj is described as:
Umj=CONSTj+∑ajlOVjlm+∑bjkLVjkm+εmj | (5) |
Where CONSTj is the constant term in the DCM, ajl represents the normalized factor loading coefficient of the l-th significant variable in the j-th travel mode, and bjk represents the normalized factor loading coefficient of the k-th significant variable in the j-th travel mode. In the model, LVjkm is the k-th latent variable in the j-th travel mode chosen by the decision-maker m.
The SEM of the model can be described as:
Ijkmz=ζjkmzLVjkm+θjkmz | (6) |
Where Ijlm is the z-th observed variable of the k-th latent variable in the j-th travel mode selected by the decision-maker m, ζjlmz is the estimated parameter corresponding to the k-th latent variable selected by decision maker m, and θjlmz is the error term.
The influence of observed variables on latent variables is described as:
LVjkz = βjkzOVjkz+υjkz | (7) |
Where LVjlz is the k-th latent variable in the j-th travel mode, βjkz is the estimated parameter corresponding to the k-th latent variable in the j-th travel mode, OVjkz is the k-th observed variable in the j-th travel mode, and υjkz is the error term.
The HCM formula is given by:
ymj{1,Umj=Max{Uj}0, Others | (8) |
Where ymj is the selection indicator in the model, and Uj is the set of all utilities in the decision-maker.
Considering the distribution of latent variables and indicators, the following formulas were used:
fI(Ijkmz|LVjkm;ζjkmz,σθz)=1σθkzϕ(Ijkmz−ζjkmzLVjkmσθνz) | (9) |
fLV(LVjkm|OVjkz;βjkz,σνz)=1σνzϕ(LVjkz−βjkzOVjkzσνz) | (10) |
The choice probability of travel mode is described as:
Pmj=∫νPmjz(LVjkm(νjkm))fLV(νjkm)fI(LVjkm(νjkm))f(ν)dν | (11) |
This study established the HCM for parentalschool travel mode choice during COVID-19, adding psychological variables such as trust, subjective norm, perceived behavior control, attitude, perceived built environment, perceived severity, and perceived vulnerability as explanatory variables. In addition, the observed variables in HCM were further divided into four types: family socioeconomic characteristics, child attribute variables, travel attribute variables and psychometric indicators. HCM combined observed variables and latent variables as covariates to measure behavior. The structural framework is shown in Figure 6.
The HCM for school travel mode was divided into three parts: the influence of observed variables on latent variables, the relationships among the latent variables, and the impact of latent variables and observed variables on travel mode choice. The formula is expressed as follows:
Uki=hk1Gender+hk2Age+hk3Education+hk4Income+hk5Kid_age+hk6Travel_distance+hk7Kid_gender+hk8Driving_license | (12) |
k=TRUST,SN,PBC,ATT,PBE,PS,PV,INT |
There were two stages of analysis: (1) the first stage was the analysis of the relationships between observed and latent variables; (2) the second stage was the path analysis of observed and latent variables.
This study analyzed the influence of observed variables on eight psychological latent variables in three travel mode choice models. In this study, relevant results were obtained through SEM and CLOGIT commands in STATA. Table 8 shows the path coefficients of observed and latent variables for the public transit travel mode choice model. The values in parentheses refer to the path z-test results. Results for the private car and walking choices are shown in Appendix D.
Gender | Age | Edu | Income | Car_number | Child_gender | Child _age | Distance | |
Trust_pt | 0.0735 | 0.065 | 0.036 | -0.046 | -0.104* | -0.069 | 0.064 | 0.068 |
(1.44) | (0.097) | (0.061) | (-0.033) | (-2.14) | (-1.62) | (1.33) | (0.67) | |
SN_pt | 0.056 | 0.016 | 0.049 | -0.026 | 0.023 | 0.067 | 0.055 | 0.092 |
(1.76) | (0.46) | (1.43) | (-0.68) | (0.68) | (1.02) | (1.06) | (1.66) | |
PBC_pt | 0.069 | 0.015 | -0.049 | -0.036 | -0.036 | 0.073 | 0.069 | 0.089 |
(1.76) | (0.46) | (-1.23) | (-1.00) | (-0.88) | (1.33) | (0.96) | (1.44) | |
ATT_pt | 0.051 | 0.094 | -0.098* | -0.117* | -0.135** | 0.067 | 0.064 | 0.012 |
(0.96) | (0.99) | (-1.97) | (-2.31) | (-2.94) | (1.22) | (0.66) | (0.031) | |
PBE | 0.046 | -0.016 | -0.039 | 0.094 | 0.064 | -0.056* | 0.074* | -0.107** |
(0.96) | (-0.15) | (-0.66) | (0.69) | (0.99) | (-1.97) | (2.04) | (-2.57) | |
PV | 0.091 | 0.046 | 0.019 | 0.081* | 0.098 | 0.056 | 0.078 | 0.095 |
(0.51) | (0.62) | (0.91) | (2.33) | (1.55) | (0.61) | (0.99) | (1.09) | |
PS | 0.041 | 0.066 | 0.091 | 0.097* | 0.033 | 0.016 | 0.069 | 0.035 |
(0.97) | (1.01) | (0.68) | (2.55) | (1.35) | (0.96) | (0.68) | (0.68) | |
INT_pt | 0.039 | -0.011 | -0.066 | -0.069* | -0.106* | 0.015 | 0.059 | 0.038 |
(0.98) | (-0.61) | (-0.98) | (-0.89) | (-2.37) | (0.89) | (0.61) | (0.15) | |
Significance level: non-significant p > 0.05, *** p < 0.001, ** p < 0.01, and * p < 0.05. |
In the public transit school travel mode choice model, education level, family income, and number of car ownership all had a significant negative impact on attitudes about public transit to school travel. This revealed that parents with higher education levels, higher family income, and car ownership had more negative attitudes towards choosing public transit as a school travel mode during COVID-19. Child gender, child age, and travel distance had a statictically significant impact on perceived built environment. Family income had a significant positive impact on both perceived severity and perceived vulnerability. In addition, family income and number of car ownership had a statisticaly significant negative impact on parental intention to choose public transit as a school travel mode.
In the private car school travel mode choice model, family income had a significant positive impact on parental trust in choosing a private car as the school travel mode. Number of car ownership and child age had a significant impact on parental attitudes towards choosing private cars. Number of car ownership had a significant impact, while child age had a negative impact. The reason may be that as children grow older, alternative forms of school travel are gradually accepted and adopted. Parents may gradually reduce the frequency of using private cars to transport their children to and from school. In addition, family income, number of car ownership, and child gender had a significant impact in parental intention to choose a private car. Family income and number of car ownership had a positive impact, while child gender had a negative impact.
Travel distance had a significant impact on the walking to school travel mode choice model. This variable had a significant affect on attitude, perceived built environment and intention. The influence on attitude was the highest, indicating that travel distance was the most important influencing factor for parents to choose walking as the active travel mode. This result replicates findings of previous studies [16].
After analyzing the influence of observed variables on latent variables, the latent variables were substituted into the discrete choice model, and the model was estimated together with socioeconomic characteristics, child attribute variables, and travel attribute variables. Walking travel mode was taken as the utility benchmark for comparative analysis of hybrid choice. Table 9 shows the goodness of fit for HCM. The results show that the model fits well. Table 10 shows the results of the path analysis and model fitting of HCM.
Inspection index | Index fitting result | Explanation | |
Public transit | Private car | ||
Cox & Snell R2 | 0.571 | 0.557 | The model fits well |
Log likelihood | -3214.123 | -3172.462 | |
Hosmer-Lemeshow | > 0.05 | > 0.05 |
Attribute variable | Public transit | Private car | ||||
Parameter estimation | Z test | P test | Parameter estimation | Z test | P test | |
Constant | -2.574* | 5.12 | 0.00 | 2.146* | 3.54 | 0.01 |
Gender | 0.087 | 1.47 | 0.15 | -0.104* | -2.23 | 0.03 |
Age | 0.037 | 1.17 | 0.24 | -0.118* | 2.17 | 0.03 |
Edu | -0.066 | -1.37 | 0.27 | 0.126* | 4.33 | 0.00 |
Income | -0.038 | -1.35 | 0.31 | 0.347* | 5.21 | 0.00 |
Car_number | -0.154* | -3.21 | 0.01 | 0.139* | 4.92 | 0.00 |
Kid_gender | 0.084 | 1.17 | 0.24 | -0.105* | -6.34 | 0.00 |
Kid_age | 0.141 | 0.53 | 0.61 | -0.131* | -4.12 | 0.00 |
Distance | 0.046 | 0.17 | 0.89 | 0.119 | 0.55 | 0.42 |
Trust_pt | 0.134* | 3.11 | 0.01 | - | - | - |
SN_pt | 0.035 | 0.41 | 0.65 | - | - | - |
PBC_pt | 0.054 | 1.16 | 0.25 | |||
ATT_pt | 0.178* | 3.64 | 0.01 | - | - | - |
INT_pt | 0.314* | 3.96 | 0.01 | - | - | - |
Trust_car | - | - | - | 0.164* | 3.24 | 0.01 |
SN_car | - | - | - | 0.071* | 1.16 | 0.02 |
PBC_car | - | - | - | 0.015 | 0.65 | 0.51 |
ATT_car | - | - | - | 0.165* | 3.59 | 0.01 |
INT_car | - | - | - | 0.217* | 2.89 | 0.02 |
PV | -0.242* | -4.91 | 0.01 | 0.289* | 3.58 | 0.01 |
PS | -0.234* | -4.76 | 0.00 | 0.204* | 4.34 | 0.00 |
PBE | 0.104 | 1.56 | 0.10 | 0.143 | 1.33 | 0.19 |
Significance level: non-significant p > 0.05, *** p < 0.001, ** p < 0.01, and * p < 0.05. |
Gender had a significant impact on private car travel mode choice. Women were more inclined than men to choose a private car as the school travel mode. Age had a significant impact on parental choice of school travel mode as well. The results show that the younger the parents, the more they prefered to use a private car for school travel.
Education level and family income had a significant positive impact on parental choice of private car travel. This shows that the higher the parents' educational background, the higher the family income, and the higher the likelihood they chose a private car for school travel mode.
Child gender and child age had a significantl negative impact on parental choice of private car travel. When the child was a girl, parents were more willing to use a private car. As their children got older, parents gradually abandoned the use of private cars and switched to active modes of school travel, such as bicycles and walking. These results are consistent with the conclusions of previous research [6].
Travel distance did not have a significant impact on private car and public transit school travel modes. The reason may be that these two travel modes are both mobile modes, and families using these two modes live far from their school. Parents were not affected by travel distance.
Trust and attitude had a significant impact on parental choice of school travel mode. Generally speaking, the stronger the trust and attitude of parents towards a particular travel mode, the more inclined they were to choose this mode. This demonstrates that trust and attitude were key factors that affected parental choice of school travel mode.
Subjective norm had a positive and significant impact on parental choice of private car as a school travel mode, while perceived behavioral control had no significant impact on private car and walking school travel modes. This demonstratest hat during COVID-19, parents were more concerned about other people's suggestions on which school travel mode they chose.
Perceived severity and perceived susceptibility were variables that indicated parental assessment of pandemic risks. The results showed that perceived severity and perceived susceptibility had a significant negative impact on parental choice of public transit and a significant positive impact on parental choice of private car. This indicates that these two variables were key factors influencing parental choice of school travel mode. Even though COVID-19 in China had been well-controlled, parental risk assessment of the pandemic made them more inclined to choose a private car and other relatively safe travel modes.
Intention is a key factor affecting parental choice of travel mode. Generally speaking, the more strongly parents intended to choose a certain travel mode, the more they likely they were to choose it. However, Perceived built environment thad no significant influence on school travel choice.
First, observed variables were analyzed. The influence of observed variables were divided into two categories: those directly acting on the decision of school travel mode choice, and those affecting parents' psychological factors that indirectly influence their decision-making. Among parental choice of public transit and private car, family income and number of car ownership greatly impacted the latent variables in the two models, which ultimately directly or indirectly affected the final decision. It can be seen that family income and number of car ownership were very important influencing factors. It can also be seen from the descriptive analyses that the proportion of families with a monthly income of more than 15,000 yuan was 45.77%, and the proportion of families that owned a car was 88.26%. Therefore, understanding how to restore this group's confidence in public transit is key to increasing their use of public transit for trasnporting their children to and from school. For the walking school travel mode, travel distance was a decisive factor influencing parental choice. This is consistent with the results of Medeiros et al., [17] which showed that when families lived within 1 kilometer of the school's buffer zone, they will choose to walk.
Next, psychological latent variables were analyzed. Through the analysis of the model results, it was found that trust, subjective norm, perceived behavior control, attitude, perceived built environment, perceived severity and perceived vulnerability all had significant impacts on parental school travel mode choice intention.
(1) Trust and attitude
Attitude was the most significant factor affecting choice of public transit school travel mode. With the walking travel mode, attitude was a strong factor. This indicates that whether parents had a positive attitude towards a certain mode of travel largely determined whether they chose that travel mode or not. This is consistent with the results of Keall et al. [18].
This study added he four factors to the model: trust, perceived built environment, perceived susceptibility and perceived severity. Among them, the last three directly influenced the choice of behavioral intention. However, trust did not have a direct influence on behavioral intention. In the public transit and private car travel models, trust indirectly influenced choice intention; with the walking travel model, trust only indirectly influenced choice intention through attitude. Parents that trusted a particular mode of travel chose this way, and they had a more positive attitude towards this mode. This shows that trust was an important factor predicting parental choice of school travel mode.
(2) Perceived severity and perceived vulnerability
Perceived severity and perceived vulnerability were both significant predictors in the model. As factors in risk assessment, perceived severity and perceived vulnerability represented parental risk perception of COVID-19. These two factors significantly impacted the choice of public transit and private car, negatively and positively, respectively. The higher the level of parental perception of the risk of COVID-19, the more they preferred to use a private car instead of public transit. Eeven if the pandemic was under control, parents wanted to ensure their children's safety, and private cars seemed to be the best choice. In addition, due to the rapid development of the Internet, information reagarding COVID-19 was sometimes confusing and involved rumors, which made information-sensitive groups anxious and uneasy. If parents believed misleading information, their judgments regarding the risk of COVID-19 would be magnified, and their trust in public transit lost. This is consistent with the results of Rosen and Knäuper [19].
(3) Perceived built environment
Perceived built environment was a significant predictor of travel intentions. Perceived built environment was the most influential factor in the walking school travel mode model. With the public transit and private car travel mode models, it had a minor effect compared to risk assessment factors. Parental perception of the built environment was divided into perception of infrastructure construction, neighborhood relations, traffic safety and crime risks. This study found that walking was a relatively flexible and safe way of travel during COVID-19, but parental perception assessment of pandemic risk was less important compared to the impact of the built environment on walking.
The results of the analyses indicate that whether parents had a positive attitude towards a particular travel mode determined whether they chose that mode or not. During COVID-19, improving traveler confidence in public transit, especially among parents, has become a vital matter for the traffic management department. When a major social crisis occurs, the Internet and social media can convey relevant information in a timely manner [20,21]. However, this direct communication channel is a double-edged sword.
On the one hand, this kind of instant information can ensure that the public keeps abreast of the latest developments regarding COVID-19, new government policies, effective protection measures, and other related content, thereby reducing the anxiety of travelers. On the other hand, sensational information and rumors are often combined with valuable information, which confuses the public, especially sensitive groups such as parents who are more influenced by this information [22]. In addition, if some parents cannot obtain relevant content in time, anxiety may cause them to believe and spread rumors to other parents. Studies have shown that information from reliable media sources can effectively reduce public anxiety and suppress rumors [23]. Therefore, when restoring public transit, schools and traffic management departments should provide parents with timely and accurate information, which is also the goal of traffic information management organizations during times of crises [22], including details pertaining to effective measures taken to protect passengers and the number of people infected on public transit.
Perceived assessment (perceived severity and perceived vulnerability) of the risk of COVID-19 is a key factor that affects parental choice of school travel mode. It reflects parental subjective experiences and views on COVID-19. Public opinion plays a key role in whether it will take the recommended preventive measures [24]. The research of Dong et al. [25] showed that during a major public crisis, the frequency of social media use by the public will increase significantly. Therefore, in emergency management, the adoption of targeted and effective protective measures based on parental subjective perceptions of COVID-19 will be of greater importance. The traffic management department should deliver effective information about COVID-19 in a timely manner, promote the protection of passengers, and not cause unnecessary anxiety and panic.
Improving the operationsof public transit is vital. Public transit should protect passengers from the virus and meet their travel needs during a a health crisis. As public transit services resume, some cities have taken measures to improve satisfaction, such as making masks mandatory on public transit, installing heat-sensitive scanners in subway and bus stations, and using health QR codes that indicate the risk of infection. Studies have shown that proper physical distance between travellers and increasing the frequency of public transit services during COVID-19 can effectively reduce passenger panic about traveling [25]. Passengers can regulate their trips based on social-distancing needs and scheduling. For example, in terms of social-distancing, the waiting areas of subway stations and bus stations should be reasonably divided to avoid congestion. In terms of scheduling, recommending travel time to passengers through mobile applications and allowing passengers to book in advance is an effective strategy. The traffic management department strives to create a safe riding environment, improve comfort, punctuality and service frequency, and enhance parental confidence in public transit through these methods.
This study found that parental choice of walking during COVID-19 was mainly affected by the built environment. The survey results showed that 37.6% of families lived a distance of less than 1 km from their school. Therefore, even during COVID-19, the government still needs to strengthen traffic control measures and infrastructure construction in the communities around the school, such as strengthening the management of motor vehicles, the construction of sidewalks around the school, and ensuring a low crime rate in nearby communities. Schools and communities can organize Walk School Bus (WSB). Under the supervision of the team leader, WSB can ensure that children arrive home safely, and can also make up for the loss of exercise caused by taking motor vehicles [6].
The specific conclusions of this research are as follows:
This study expanded previous TPB and PMT models to include psychological latent variables such as perceived severity, perceived vulnerability, perceived built environmentand, trust, as well as objective variables such as socioeconomic characteristics and travel attributes. A theoretical framework for the choice of parental school travel mode during COVID-19 was proposed. Analyses on the models were performed based on survey data, which verified the applicability of the models in explaining parental choice of school travel mode.
SEM was used to verify the main factors influencing parental choice of school travel mode during COVID-19. Modelling results demonstrated that trust, perceived severity, perceived vulnerability, perceived built environment, attitude, subjective norms and perceived behavior control greatly influenced behavioral intentions. Attitudes towards travel mode was the main factor determining whether parents chose this mode or not. Perceived severity and perceived vulnerability represented perceived pandemic risk assessment and had a significant impact on the choice of public transit and private car travel mode, but not a significant impact on choice of walking travel mode. This showed that parental choice of public transit or private car during COVID-19 was dependent upon their subjective judgment of the severity of the pandemic. However, their choice of walking was not affected by this judgement. Perceived built environment was the most important factor affecting the prediction of choosing to walk. This shows that during COVID-19, parents still paid greater attention to the built environment around the school than their perception of pandemic risk.
HCM was used to analyze the relationships between socioeconomic characteristics, travel attributes and psychological latent variables. The results showed that the observed variables had different effects on the psychological latent variables regarding public transit, private car and walking travel modes. In addition, this study analyzed the path of numerous variables using walking travel mode as the benchmark utility, and found that familyiIncome, number of car ownership, perceived severity and perceived vulnerability were the most significant variables affecting choice of travel mode.
Through the analyses and discussion of key influencing factors, this study thoroughly expored parental school travel mode choice during COVID-19, and offers several constructive suggestions to the government and public transit management departments. These suggestions could increase parental confidence in public transit and reduce the frequency of private car use, thereby alleviating urban traffic pressure.
Based on previous research, the main insights stemming from this research are as follows:
Current domestic and foreign research on travel modes during COVID-19 have usually focused on urban commuters. Research on parental choice of school travel mode has been typically conducted during non-pandemic times. Previous studies rarely explored the impact of COVID-19 on parental choice of school travel mode. Therefore, this study examined the impact of COVID-19 into the study of parental choice of school travel mode and analyzed and predicted parental choice of school travel mode during COVID-19.
In terms of theoretical models, this study combined TPB with PMT to examine travel mode choices during COVID-19. By combining parental characteristics and travel attributes, a more complete and systematic framework for understanding parental choice behavior that includes economic factors, travel attributes and psychological factors was constructed.
In terms of influencing factors, in order to study the impact of COVID-19 on parental choice, this study used several original influencing factors and retained perceived built environment in the study of school travel. Perceived severity and perceived vulnerability, which can represent parental assessment of pandemic risk, were added to expand the existing theoretical model.
Most previous studies used SEM to examine the influence of psychological factors on choice behavior, or use choice model to understand the influence of variables on choice behavior. However, few empirical studies have combined SEM and choice model.
Moreover, as the decision-making process is a collection of linear and non-linear relationships, it is far from enough to only use SEM. Therefore, this study combined HCM with SEM to analyze the influence of several obvious variables on latent variables and the influence of various factors on choice behavior.
Parents in different regions may show differences in their school travel mode choices. Since the survey data in this study only came from Zhenjiang City, it may not represent other regions in China. Future research can compare other regions in China and foreign cities.
This study introduced perceived severity and perceived vulnerability to explore parental risk assessment of COVID-19. However, it is a bit unrealistic to measure the perception of COVID-19 with only two variables. At the same time, this study considered "rational" decision-making, but only included psychological and emotional issues such as anxiety, fear, or frustration. Future research should consider several different aspects of these factors in pandemic risk assessment.
Regarding the model's fit, some indicators were "acceptable", but not excellent. Future research should add reasonable control variables to improve the model's fit.
The results of this study show that different socioeconomic characteristic may affect parental choice of school travel mode. Therefore, future research should further divide the parent population on various attributes to provide a more detailed understanding.
The authors would like to thank all the interviewees for offering their time and valuable insights. We would also gratefully acknowledge the kind support from the National Natural Science Foundation of China. In addition, we are grateful to colleagues who provided valuable comments during the paper writing process.
The authors declare there is no conflict of interest.
[1] |
P. Chen, J. F. Jiao, M. Y. Xu, X. Gao, C. Bischak, Promoting active student travel: A longitudinal study. J. Transp. Geogr., 70 (2018), 265–274. https://doi.org/10.1016/j.jtrangeo.2018.06.015 doi: 10.1016/j.jtrangeo.2018.06.015
![]() |
[2] |
E. Temkin, Extremely low prevalence of asymptomatic COVID-19 among healthcare workers caring for COVID-19 patients in Israeli hospitals: A cross-sectional study, Clin. Microbiol. Infect., 27 (2021), 1–4. https://doi.org/10.1016/j.cmi.2020.09.040 doi: 10.1016/j.cmi.2020.09.040
![]() |
[3] |
M. Wirth, L. Rauchenbach, B. Hurwitz, H. P. Schmiedebach, J. A. Herdt, The meaning of care and ethics to mitigate the harshness of triage in second-wave scenario planning during the COVID-19 pandemic, Am. J. Bioeth., 20 (2020), 17–19. https://doi.org/10.1080/15265161.2020.1777355 doi: 10.1080/15265161.2020.1777355
![]() |
[4] | A. Hubner, How did we get here? A framing and source analysis of early COVID-19 media coverage, Commun. Res. Rep., 38 (2021), 32–41. |
[5] |
R. Barff, D. Mcackay, R. W. Olshavsky, A selective review of travel-mode choice models, J. Consumer Res., 8 (1982), 370–380. https://doi.org/10.1086/208877 doi: 10.1086/208877
![]() |
[6] |
P. V. Berg, E. O. D. Waygood, I. D. Craats, A. Kemperman, Factors affecting parental safety perception, satisfaction with school travel and mood in primary school children in the Netherlands, J. Transp. Health, 16 (2020), 137–149. https://doi.org/10.1016/j.jth.2020.100837 doi: 10.1016/j.jth.2020.100837
![]() |
[7] |
J. S. Mindell, E. Christina, D. Hopkins, S. Mandic, Taking the bus? Barriers and facilitators for adolescent use of public buses to school, Travel Behav. Soc., 22 (2021), 48–58. https://doi.org/10.1016/j.tbs.2020.08.006 doi: 10.1016/j.tbs.2020.08.006
![]() |
[8] |
S. Khaddar, M. R. Fatmi, COVID-19: Are you satisfied with traveling during the pandemic? Transp. Res. Interdiscipl. Perspect., 9 (2021), 10–29. https://doi.org/10.1016/j.trip.2020.100292 doi: 10.1016/j.trip.2020.100292
![]() |
[9] |
A. S. Bergantino, M. Intini, L. Tangari, Influencing factors for potential bike-sharing users: An empirical analysis during the COVID-19 pandemic, Res.Transp. Econom., 81 (2021), 128–144. https://doi.org/10.1016/j.retrec.2020.101028 doi: 10.1016/j.retrec.2020.101028
![]() |
[10] |
F. Mikki, P. Papadopoulou, Tackling mobility environmental impacts through the promotion of student active travel, Transp. Res. Proced., 24 (2017), 321–328. https://doi.org/10.1016/j.trpro.2017.05.104 doi: 10.1016/j.trpro.2017.05.104
![]() |
[11] |
M. J. Beck, D. A. Hensher, E. Wei, Slowly coming out of COVID-19 restrictions in Australia: Implications for working from home and commuting trips by car and public transit, J. Transp. Geography, 88 (2020), 186–201. https://doi.org/10.1016/j.jtrangeo.2020.102846 doi: 10.1016/j.jtrangeo.2020.102846
![]() |
[12] |
M. Scorrano, R. Danielis, Active mobility in an Italian city: Mode choice determinants and attitudes before and during the COVID-19 emergency, Res. Transp. Econom., 83 (2021), 31–55. https://doi.org/10.1016/j.retrec.2021.101031 doi: 10.1016/j.retrec.2021.101031
![]() |
[13] |
M. Vitale, H. Mollward, J. Spinney, School siting and mode choices for school travel: Rural–urban contrasts in Halifax, Nova Scotia, Canada, Case Studies Transp. Policy, 7 (2019), 64–72. https://doi.org/10.1016/j.cstp.2018.11.008 doi: 10.1016/j.cstp.2018.11.008
![]() |
[14] |
L. Law, N. Fong, Applying partial least squares structural equation modeling (PLS-SEM) in an investigation of undergraduate students' learning transfer of academic English, J. English Acad. Purp., 46 (2020), 84–103. https://doi.org/10.1016/j.jeap.2020.100884 doi: 10.1016/j.jeap.2020.100884
![]() |
[15] | J. H. Jiang, Research on taxi service mode choice behavior based on theory of planned behavior, PhD diss., Harbin Institute of Technology, 2017. |
[16] |
E. Ikeda, S. Mavoa, A. Cavadino, P. Carroll, E. Hinckson, K. Witten, et al., Keeping kids safe for active travel to school: A mixed method examination of school policies and practices and children's school travel behaviour, Travel Behav. Soc., 21 (2020), 57–68. https://doi.org/10.1016/j.tbs.2020.05.008 doi: 10.1016/j.tbs.2020.05.008
![]() |
[17] |
A. Medeiros, A. Buttazzoni, S. E. Coen, A. F. Clark, K. Wilson, J. Gilliland, Review of gender, socioeconomic status, and ethnic background considerations reported in active school travel intervention studies, J. Transp. Health, 21 (2021), 35–49. https://doi.org/10.1016/j.jth.2021.101035 doi: 10.1016/j.jth.2021.101035
![]() |
[18] |
M. Keall, D. Hopkins, K.Coppell, S. Sandretto, G. B. Enrique, J. Spence, et al., Implications of attending the closest school on adolescents' physical activity and car travel in Dunedin, New Zealand, J. Transp. Health, 18 (2020), 19–35. https://doi.org/10.1016/j.jth.2020.100900 doi: 10.1016/j.jth.2020.100900
![]() |
[19] |
N. O. Rosen, K. Bärbel, A little uncertainty goes a long way: State and trait differences in uncertainty interact to increase information seeking but also increase worry, Health Commun., 24 (2009), 228–238. https://doi.org/10.1080/10410230902804125 doi: 10.1080/10410230902804125
![]() |
[20] |
M. Chao, D. Xue, T. Liu, H. Yang, B. J. Hall, Media use and acute psychological outcomes during COVID-19 outbreak in China, J. Anxiety Disord., 74 (2020), 214–223. https://doi.org/10.1016/j.janxdis.2020.102248 doi: 10.1016/j.janxdis.2020.102248
![]() |
[21] |
W. Zhou, A. Wang, F. Xia, Y. Xiao, S. Tang, Effects of media reporting on mitigating spread of COVID-19 in the early phase of the outbreak, Math. Biosci. Eng., 17 (2020), 2693–2707. https://doi.org/10.3934/mbe.2020147 doi: 10.3934/mbe.2020147
![]() |
[22] |
A. Keselman, L. Slaughter, V. L. Pater, Toward a framework for understanding lay public's comprehension of disaster and bioterrorism information, J. Biomed. Inform., 38 (2005), 331–344. https://doi.org/10.1016/j.jbi.2005.05.001 doi: 10.1016/j.jbi.2005.05.001
![]() |
[23] |
N. Mheidly, J. Fares, Leveraging media and health communication strategies to overcome the COVID-19 infodemic, J. Public Health Policy, 41 (2020), 410–420. https://doi.org/10.1057/s41271-020-00247-w doi: 10.1057/s41271-020-00247-w
![]() |
[24] |
F. B. Pereira, F. Nunes, Media choice and the polarization of public opinion about Covid-19 in Brazil, Revista Latinoamericana de Opinión Pública, 7 (2021), 1–19. https://doi.org/10.14201/rlop.23681 doi: 10.14201/rlop.23681
![]() |
[25] |
H. Dong, S. Ma, N. Jia, J. Tian, Understanding public transport satisfaction in post COVID-19 pandemic, Transp. Policy, 101 (2021), 81–88. https://doi.org/10.1016/j.tranpol.2020.12.004 doi: 10.1016/j.tranpol.2020.12.004
![]() |
[26] |
A. S. Kong, N. Burks, C. Conklin, C. Roldan, B. Skipper, S. Scott, et al., A pilot walking school bus program to prevent obesity in hispanic elementary school children: Role of physician involvement with the school community, Clin. Pediatr., 49 (2010), 989–991. https://doi.org/10.1177/0009922810370364 doi: 10.1177/0009922810370364
![]() |
1. | Gang Cheng, Changliang He, Analysis of bus travel characteristics and predictions of elderly passenger flow based on smart card data, 2022, 30, 2688-1594, 4256, 10.3934/era.2022217 | |
2. | Yueqi Mao, Qiang Mei, Peng Jing, Xingyue Wang, Ying Xue, Ye Zha, Uncovering the behavioral determinants behind private car purchase intention during the new normal of COVID-19: An empirical investigation in China, 2023, 20, 1551-0018, 7316, 10.3934/mbe.2023318 | |
3. | Peng Jing, Weichao Wang, Chengxi Jiang, Ye Zha, Baixu Ming, Determinants of switching behavior to wear helmets when riding e-bikes, a two-step SEM-ANFIS approach, 2023, 20, 1551-0018, 9135, 10.3934/mbe.2023401 | |
4. | Sandra Mandic, Enrique García Bengoechea, Kirsten J. Coppell, Michael Keall, Melody Smith, Debbie Hopkins, Susan Sandretto, Gordon Wilson, Gavin Kidd, Charlotte Flaherty, Jennifer S. Mindell, Janet Stephenson, Kimberley King, Kaisa Kentala, Anna Rolleston, John C. Spence, Travel to school patterns and perceptions of walking to school in New Zealand adolescents before versus during the COVID-19 pandemic, 2024, 36, 22141405, 101803, 10.1016/j.jth.2024.101803 | |
5. | Peng Jing, Ye Zha, Kewen Pan, Ying Xue, Investigating Multidimensional Factors Influencing Switching Intention on School Bus among Chinese Parents—A Push–Pull–Mooring Framework, 2023, 15, 2071-1050, 7770, 10.3390/su15107770 |
Year of publication | Research object | Travel mode | Influential factors | Theoretical model | Research method |
Khaddar (2021) | Commuter | Public transit Private car Walk | Socioeconomic attribute variable Travel attribute variable | - | Ordered logit model |
Vickerman (2021) | Commuter | Public transit | - | - | Qualitative analysis |
Chang (2021) | Commuter | Public transit | Socioeconomic attribute variable Travel attribute variable | - | Variance analysis |
Zheng (2020) | Commuter | Public transit | Psychological latent variable | PMT | SEM |
Dong (2021) | Commuter | Public transit | Psychological latent variable | - | SEM |
Bergantino (2020) | Commuter | Public transit | Socioeconomic attribute variable Travel attribute variable psychological latent variable | - | Ordered logit model |
Parady (2020) | Commuter | Public transit | Socioeconomic attribute variable Psychological latent variable | - | Logit model |
Zenker (2021) | Commuter | Public transit | Socioeconomic attribute variable Psychological latent variable | - | SEM |
Anwari (2021) | Commuter | Public transit | Socioeconomic attribute variable Travel attribute variable | - | Logistic regression model |
Scorrano (2021) | Commuter | Public transit Private car Walk | Socioeconomic attribute variable psychological latent variable | TPB | Hybrid logit model |
Ozbilen (2021) | Commuter | Public transit Private car Shared travel | Socioeconomic attribute variable Psychological latent variable | - | Binomial logit model |
Beck (2020) | Commuter | Public transit Private car | Socioeconomic attribute variable Travel attribute variable Psychological latent variable | - | Ordered logit model |
Dai (2021) | Commuter | Public transit | - | - | Synthetic control method |
Basu (2021) | Commuter | Public transit Shared travel | - | - | Qualitative analysis |
Teixeira (2020) | Commuter | Public transit Shared travel | Travel attribute variable | - | GIS |
Hypotheses | Assumption |
H1a | Under COVID-19, Subjective Norm has a negative impact on parents' Intention to choose public transit. |
H1b | Under COVID-19, Subjective Norm has a positive impact on parents' Intention to choose public transit. |
H1c | Under COVID-19, Subjective Norm has a positive impact on parents' Intention to choose walk. |
H2a | Under COVID-19, Attitude has a negative impact on parents' Intention to choose public transit. |
H2b | Under COVID-19, Attitude has a positive impact on parents' Intention to choose private car. |
H2c | Under COVID-19, Attitude has a positive impact on parents' Intention to choose walk. |
H3a | Under COVID-19, Perceived Behavioral Control has a negative impact on parents' Intention to choose public transit. |
H3b | Under COVID-19, Perceived Behavioral Control has a positive impact on parents' Intention to choose private car. |
H3c | Under COVID-19, Perceived Behavioral Control has a positive impact on parents' Intention to choose walk. |
H4a | Under COVID-19, Trust has a negative impact on parents' Subjective Norm of choosing public transit. |
H4b | Under COVID-19, Trust has a positive impact on parents' Subjective Norm of choosing private car. |
H4c | Under COVID-19, Trust has a positive impact on parents' Subjective Norm of choosing walk. |
H5a | Under COVID-19, Trust has a negative impact on parents' Attitude of choosing public transit. |
H5b | Under COVID-19, Trust has a positive impact on parents' Attitude of choosing private car. |
H5c | Under COVID-19, Trust has a positive impact on parents' Attitude of choosing walk. |
H6a | Under COVID-19, Trust has a negative impact on parents' Perceived Behavior Control of choosing public transit. |
H6b | Under COVID-19, Trust has a positive impact on parents' Perceived Behavior Control of choosing private car. |
H6c | Under COVID-19, Trust has a positive impact on parents' Perceived Behavior Control of choosing walk. |
H7a | Under COVID-19, Trust has a negative impact on parents' Intention to choose public transit. |
H7b | Under COVID-19, Trust has a positive impact on parents' Intention to choose private car. |
H7c | Under COVID-19, Trust has a positive impact on parents' Intention to choose walk. |
H8a | Under COVID-19, Perceived Built Environment has a positive impact on parents' Intention to choose public transit. |
H8b | Under COVID-19, Perceived Built Environment has a negative impact on parents' Intention to choose private car. |
H8c | Under COVID-19, Perceived Built Environment has a positive impact on parents' Intention to choose private car. |
H9a | Under COVID-19, Perceived Built Environment has a positive impact on parents' Attitude of choosing public transit. |
H9b | Under COVID-19, Perceived Built Environment has a negative impact on parents' Attitude of choosing private car. |
H9c | Under COVID-19, Perceived Built Environment has a positive impact on parents' Attitude of choosing walk. |
H10a | Under COVID-19, Perceived Severity has a negative impact on parents' Intention to choose public transit. |
H10b | Under COVID-19, Perceived Severity has a positive impact on parents' Intention to choose private car. |
H10c | Under COVID-19, Perceived Severity has a negative impact on parents' Intention to choose walk. |
H11a | Under COVID-19, Perceived Severity has a negative impact on parents' Attitude of choosing public transit. |
H11b | Under COVID-19, Perceived Severity has a positive impact on parents' Attitude of choosing private car. |
H11c | Under COVID-19, Perceived Severity has a negative impact on parents' Attitude of choosing walk. |
H12a | Under COVID-19, Perceived Vulnerability has a negative impact on parents' Intention to choose public transit. |
H12b | Under COVID-19, Perceived Vulnerability has a positive impact on parents' Intention to choose private car. |
H12c | Under COVID-19, Perceived Vulnerability has a negative impact on parents' Intention to choose walk. |
H13a | Under COVID-19, Perceived Vulnerability has a negative impact on parents' Attitude of choosing public transit. |
H13b | Under COVID-19, Perceived Vulnerability has a positive impact on parents' Attitude of choosing private car. |
H13c | Under COVID-19, Perceived Vulnerability has a negative impact on parents' Attitude of choosing walk. |
Demographic variables | Sample size | Percentage (%) | |
Gender | Men | 217 | 47.17 |
Women | 243 | 52.83 | |
Age | 18–25 | 30 | 6.52 |
26–30 | 77 | 16.74 | |
31–35 | 154 | 33.48 | |
36–40 | 88 | 19.13 | |
41–45 | 56 | 12.17 | |
46–50 | 30 | 6.52 | |
Older than 50 | 25 | 5.44 | |
Education level | Primary (elementary/middle school) | 18 | 3.91 |
Secondary (high school) | 35 | 7.61 | |
Junior college | 108 | 23.48 | |
University (undergraduate) | 260 | 56.52 | |
Postgraduate | 39 | 8.48 | |
Family monthly income (RMB) | < 5000 | 10 | 2.01 |
5001–10,000 | 117 | 25.48 | |
10,001–15,000 | 123 | 26.74 | |
15,001–20,000 | 132 | 28.65 | |
> 20,000 | 78 | 17.12 | |
Occupation | Private enterprise | 197 | 42.83 |
Joint venture /foreign-invested enterprises | 32 | 6.96 | |
State-owned enterprises | 67 | 14.56 | |
Non-profit organization/institution | 3 | 0.65 | |
Government agencies | 26 | 5.65 | |
Schools, research institutes and other academic institutions | 51 | 11.09 | |
Other | 84 | 18.26 | |
Driver's license | Yes | 355 | 77.17 |
No | 105 | 22.83 | |
Number of car ownership | 0 | 54 | 11.74 |
1 | 294 | 63.91 | |
2 | 101 | 21.96 | |
3 | 11 | 2.39 | |
Number of electric bicycles owned | 0 | 107 | 23.26 |
1 | 196 | 42.61 | |
2 | 126 | 27.39 | |
3 | 23 | 5 | |
Number of children (<18 years old) | 1 | 386 | 83.91 |
2 | 68 | 14.78 | |
3 | 5 | 1.09 | |
4 | 1 | 0.22 | |
Child Gender | Boy | 270 | 58.7 |
Girl | 190 | 41.3 | |
Child age | 6–11 | 315 | 68.48 |
12–4 | 68 | 14.78 | |
15–18 | 77 | 16.74 | |
Distance from school to home | Less than 0.5 km | 68 | 14.78 |
0.5 km–1.0 km | 105 | 22.83 | |
1.0 km–-1.5 km | 77 | 16.74 | |
1.5 km–2.0 km | 50 | 10.87 | |
2.0 km–2.5 km | 58 | 12.61 | |
2.5 km–3.0 km | 25 | 5.43 | |
More than 3.0 km | 77 | 16.74 |
Psychological variables | N of Items | α | C.R. |
Perceived Built Environment | 6 | 0.772 | 0.877 |
Perceived Severity | 3 | 0.783 | 0.872 |
Perceived Vulnerability | 3 | 0.851 | 0.896 |
Subjective Norm | 3/3/3 | 0.776/0.786/0.779 | 0.898/0.838/0.703 |
Perceived Behavior Control | 2/2/2 | 0.732/0.745/0.718 | 0.815/0.880/0.833 |
Trust | 2/2/2 | 0.857/0.764/0.892 | 0.795/0.908/0.712 |
Attitude | 2/2/2 | 0.891/0.839/0.890 | 0.892/0.7854/0.807 |
Intention | 3/3/3 | 0.824/0.854/0.823 | 0.879/0.893/0.870 |
Note: α < 0.6, unacceptable reliability; 0.6≤α < 0.7, acceptable reliability; 0.7≤α < 0.8, high reliability; 0.8≤α < 0.9, very high reliability; α≥0.9, extremely high reliability. CR > 0.6, good reliability. |
Psychological variables | Observed variables | Normalization factor loading coefficient | AVE |
Perceived Built Environment | PBE1 | 0.847 | 0.703 |
PBE2 | 0.855 | ||
PBE3 | 0.813 | ||
PBE4 | 0.863 | 0.6863 | |
PBE5 | 0.841 | ||
PBE6 | 0.779 | ||
Perceived Severity | PS1 | 0.838 | 0.6951 |
PS2 | 0.84 | ||
PS3 | 0.823 | ||
Perceived Vulnerability | PV1 | 0.891 | 0.7415 |
PV2 | 0.865 | ||
PV3 | 0.826 | ||
Subjective Norm | SN1_pt / SN1_car / SN1_walk | 0.851/0.822/0.839 | 0.7467/0.633/0.877 |
SN2_pt / SN2_car / SN2_walk | 0.886/0.754/0.839 | ||
SN3_pt / SN3_car / SN3_walk | 0.855/0.809/0.837 | ||
Perceived Behavior Control | PBC1_pt / PBC1_car / PBC1_walk | 0.843/0.886/0.907 | 0.6874/0.785/0.909 |
PBC2_pt / PBC2_car / PBC2_walk | 0.815/0.872/0.918 | ||
Trust | TRUST1_pt / TRUST1_car / TRUST1_walk | 0.813/0.901/0.871 | 0.6593/0.8319/0.832 |
TRUST2_pt / TRUST2_car / TRUST2_walk | 0.811/0.924/0.817 | ||
Attitude | ATT1_pt / ATT1_car / ATT1_walk | 0.893/0.822/0.904 | 0.8046/0.6467/0.893 |
ATT2_pt / ATT2_car / ATT2_walk | 0.901/0.786/0.893 | ||
Intention | INT1_pt / INT1_car / INT1_walk | 0.824/0.842/0.796 | 0.706/0.736/0.670 |
INT2_pt / INT2_car / INT2_walk | 0.857/0.875/0.837 | ||
INT3_pt / INT3_car / INT3_walk | 0.842/0.856/0.857 | ||
Note: AVE > 0.5, good convergent validity; normalization factor loading coefficient > 0.5, good convergent validity. |
Index name | χ2/df | RMSEA | IFI | GFI | NFI | CFI |
Test critical value | < 3, Good | < 0.05, Excellent | > 0.90 | > 0.90 | > 0.90 | > 0.90 |
< 5, Accepted | 0.05-0.08, Good | |||||
Model parameter value (public transit) | 4.48 | 0.062 | 0.917 | 0.902 | 0.900 | 0.907 |
Model parameter value (private car) | 3.97 | 0.059 | 0.921 | 0.916 | 0.908 | 0.914 |
Model parameter value (walk) | 3.69 | 0.070 | 0.911 | 0.904 | 0.901 | 0.900 |
Null hypotheses | Path | Standardized Regression Coefficient (β) | Standard Error of Estimate (S.E.) | Significance (p) |
H1a | SN_pt→INT_pt | 0.152 | 0.060 | p < 0.05 |
H2a | ATT_pt→INT_pt | 0.471 | 0.046 | p < 0.01 |
H3a | PBC_pt→INT_pt | 0.163 | 0.051 | p < 0.01 |
H4a | TRUST_pt→SN_pt | 0.162 | 0.061 | p < 0.01 |
H5a | TRUST_pt→ATT_pt | 0.241 | 0.068 | p < 0.01 |
H6a | TRUST_pt→PBC_pt | 0.183 | 0.036 | p < 0.01 |
H7a | TRUST_pt→INT_pt | 0.083 | 0.048 | - |
H8a | PBE_pt→INT_pt | 0.174 | 0.057 | p < 0.01 |
H9a | PBE_pt→ATT_pt | 0.083 | 0.047 | p < 0.01 |
H10a | PS_pt→ATT_pt | -0.276 | 0.052 | p < 0.01 |
H11a | PS_pt→INT_pt | -0.227 | 0.039 | p < 0.01 |
H12a | PV_pt→INT_pt | -0.311 | 0.066 | p < 0.01 |
H13a | PV_pt→ATT_pt | -0.214 | 0.046 | p < 0.01 |
Gender | Age | Edu | Income | Car_number | Child_gender | Child _age | Distance | |
Trust_pt | 0.0735 | 0.065 | 0.036 | -0.046 | -0.104* | -0.069 | 0.064 | 0.068 |
(1.44) | (0.097) | (0.061) | (-0.033) | (-2.14) | (-1.62) | (1.33) | (0.67) | |
SN_pt | 0.056 | 0.016 | 0.049 | -0.026 | 0.023 | 0.067 | 0.055 | 0.092 |
(1.76) | (0.46) | (1.43) | (-0.68) | (0.68) | (1.02) | (1.06) | (1.66) | |
PBC_pt | 0.069 | 0.015 | -0.049 | -0.036 | -0.036 | 0.073 | 0.069 | 0.089 |
(1.76) | (0.46) | (-1.23) | (-1.00) | (-0.88) | (1.33) | (0.96) | (1.44) | |
ATT_pt | 0.051 | 0.094 | -0.098* | -0.117* | -0.135** | 0.067 | 0.064 | 0.012 |
(0.96) | (0.99) | (-1.97) | (-2.31) | (-2.94) | (1.22) | (0.66) | (0.031) | |
PBE | 0.046 | -0.016 | -0.039 | 0.094 | 0.064 | -0.056* | 0.074* | -0.107** |
(0.96) | (-0.15) | (-0.66) | (0.69) | (0.99) | (-1.97) | (2.04) | (-2.57) | |
PV | 0.091 | 0.046 | 0.019 | 0.081* | 0.098 | 0.056 | 0.078 | 0.095 |
(0.51) | (0.62) | (0.91) | (2.33) | (1.55) | (0.61) | (0.99) | (1.09) | |
PS | 0.041 | 0.066 | 0.091 | 0.097* | 0.033 | 0.016 | 0.069 | 0.035 |
(0.97) | (1.01) | (0.68) | (2.55) | (1.35) | (0.96) | (0.68) | (0.68) | |
INT_pt | 0.039 | -0.011 | -0.066 | -0.069* | -0.106* | 0.015 | 0.059 | 0.038 |
(0.98) | (-0.61) | (-0.98) | (-0.89) | (-2.37) | (0.89) | (0.61) | (0.15) | |
Significance level: non-significant p > 0.05, *** p < 0.001, ** p < 0.01, and * p < 0.05. |
Inspection index | Index fitting result | Explanation | |
Public transit | Private car | ||
Cox & Snell R2 | 0.571 | 0.557 | The model fits well |
Log likelihood | -3214.123 | -3172.462 | |
Hosmer-Lemeshow | > 0.05 | > 0.05 |
Attribute variable | Public transit | Private car | ||||
Parameter estimation | Z test | P test | Parameter estimation | Z test | P test | |
Constant | -2.574* | 5.12 | 0.00 | 2.146* | 3.54 | 0.01 |
Gender | 0.087 | 1.47 | 0.15 | -0.104* | -2.23 | 0.03 |
Age | 0.037 | 1.17 | 0.24 | -0.118* | 2.17 | 0.03 |
Edu | -0.066 | -1.37 | 0.27 | 0.126* | 4.33 | 0.00 |
Income | -0.038 | -1.35 | 0.31 | 0.347* | 5.21 | 0.00 |
Car_number | -0.154* | -3.21 | 0.01 | 0.139* | 4.92 | 0.00 |
Kid_gender | 0.084 | 1.17 | 0.24 | -0.105* | -6.34 | 0.00 |
Kid_age | 0.141 | 0.53 | 0.61 | -0.131* | -4.12 | 0.00 |
Distance | 0.046 | 0.17 | 0.89 | 0.119 | 0.55 | 0.42 |
Trust_pt | 0.134* | 3.11 | 0.01 | - | - | - |
SN_pt | 0.035 | 0.41 | 0.65 | - | - | - |
PBC_pt | 0.054 | 1.16 | 0.25 | |||
ATT_pt | 0.178* | 3.64 | 0.01 | - | - | - |
INT_pt | 0.314* | 3.96 | 0.01 | - | - | - |
Trust_car | - | - | - | 0.164* | 3.24 | 0.01 |
SN_car | - | - | - | 0.071* | 1.16 | 0.02 |
PBC_car | - | - | - | 0.015 | 0.65 | 0.51 |
ATT_car | - | - | - | 0.165* | 3.59 | 0.01 |
INT_car | - | - | - | 0.217* | 2.89 | 0.02 |
PV | -0.242* | -4.91 | 0.01 | 0.289* | 3.58 | 0.01 |
PS | -0.234* | -4.76 | 0.00 | 0.204* | 4.34 | 0.00 |
PBE | 0.104 | 1.56 | 0.10 | 0.143 | 1.33 | 0.19 |
Significance level: non-significant p > 0.05, *** p < 0.001, ** p < 0.01, and * p < 0.05. |
Year of publication | Research object | Travel mode | Influential factors | Theoretical model | Research method |
Khaddar (2021) | Commuter | Public transit Private car Walk | Socioeconomic attribute variable Travel attribute variable | - | Ordered logit model |
Vickerman (2021) | Commuter | Public transit | - | - | Qualitative analysis |
Chang (2021) | Commuter | Public transit | Socioeconomic attribute variable Travel attribute variable | - | Variance analysis |
Zheng (2020) | Commuter | Public transit | Psychological latent variable | PMT | SEM |
Dong (2021) | Commuter | Public transit | Psychological latent variable | - | SEM |
Bergantino (2020) | Commuter | Public transit | Socioeconomic attribute variable Travel attribute variable psychological latent variable | - | Ordered logit model |
Parady (2020) | Commuter | Public transit | Socioeconomic attribute variable Psychological latent variable | - | Logit model |
Zenker (2021) | Commuter | Public transit | Socioeconomic attribute variable Psychological latent variable | - | SEM |
Anwari (2021) | Commuter | Public transit | Socioeconomic attribute variable Travel attribute variable | - | Logistic regression model |
Scorrano (2021) | Commuter | Public transit Private car Walk | Socioeconomic attribute variable psychological latent variable | TPB | Hybrid logit model |
Ozbilen (2021) | Commuter | Public transit Private car Shared travel | Socioeconomic attribute variable Psychological latent variable | - | Binomial logit model |
Beck (2020) | Commuter | Public transit Private car | Socioeconomic attribute variable Travel attribute variable Psychological latent variable | - | Ordered logit model |
Dai (2021) | Commuter | Public transit | - | - | Synthetic control method |
Basu (2021) | Commuter | Public transit Shared travel | - | - | Qualitative analysis |
Teixeira (2020) | Commuter | Public transit Shared travel | Travel attribute variable | - | GIS |
Hypotheses | Assumption |
H1a | Under COVID-19, Subjective Norm has a negative impact on parents' Intention to choose public transit. |
H1b | Under COVID-19, Subjective Norm has a positive impact on parents' Intention to choose public transit. |
H1c | Under COVID-19, Subjective Norm has a positive impact on parents' Intention to choose walk. |
H2a | Under COVID-19, Attitude has a negative impact on parents' Intention to choose public transit. |
H2b | Under COVID-19, Attitude has a positive impact on parents' Intention to choose private car. |
H2c | Under COVID-19, Attitude has a positive impact on parents' Intention to choose walk. |
H3a | Under COVID-19, Perceived Behavioral Control has a negative impact on parents' Intention to choose public transit. |
H3b | Under COVID-19, Perceived Behavioral Control has a positive impact on parents' Intention to choose private car. |
H3c | Under COVID-19, Perceived Behavioral Control has a positive impact on parents' Intention to choose walk. |
H4a | Under COVID-19, Trust has a negative impact on parents' Subjective Norm of choosing public transit. |
H4b | Under COVID-19, Trust has a positive impact on parents' Subjective Norm of choosing private car. |
H4c | Under COVID-19, Trust has a positive impact on parents' Subjective Norm of choosing walk. |
H5a | Under COVID-19, Trust has a negative impact on parents' Attitude of choosing public transit. |
H5b | Under COVID-19, Trust has a positive impact on parents' Attitude of choosing private car. |
H5c | Under COVID-19, Trust has a positive impact on parents' Attitude of choosing walk. |
H6a | Under COVID-19, Trust has a negative impact on parents' Perceived Behavior Control of choosing public transit. |
H6b | Under COVID-19, Trust has a positive impact on parents' Perceived Behavior Control of choosing private car. |
H6c | Under COVID-19, Trust has a positive impact on parents' Perceived Behavior Control of choosing walk. |
H7a | Under COVID-19, Trust has a negative impact on parents' Intention to choose public transit. |
H7b | Under COVID-19, Trust has a positive impact on parents' Intention to choose private car. |
H7c | Under COVID-19, Trust has a positive impact on parents' Intention to choose walk. |
H8a | Under COVID-19, Perceived Built Environment has a positive impact on parents' Intention to choose public transit. |
H8b | Under COVID-19, Perceived Built Environment has a negative impact on parents' Intention to choose private car. |
H8c | Under COVID-19, Perceived Built Environment has a positive impact on parents' Intention to choose private car. |
H9a | Under COVID-19, Perceived Built Environment has a positive impact on parents' Attitude of choosing public transit. |
H9b | Under COVID-19, Perceived Built Environment has a negative impact on parents' Attitude of choosing private car. |
H9c | Under COVID-19, Perceived Built Environment has a positive impact on parents' Attitude of choosing walk. |
H10a | Under COVID-19, Perceived Severity has a negative impact on parents' Intention to choose public transit. |
H10b | Under COVID-19, Perceived Severity has a positive impact on parents' Intention to choose private car. |
H10c | Under COVID-19, Perceived Severity has a negative impact on parents' Intention to choose walk. |
H11a | Under COVID-19, Perceived Severity has a negative impact on parents' Attitude of choosing public transit. |
H11b | Under COVID-19, Perceived Severity has a positive impact on parents' Attitude of choosing private car. |
H11c | Under COVID-19, Perceived Severity has a negative impact on parents' Attitude of choosing walk. |
H12a | Under COVID-19, Perceived Vulnerability has a negative impact on parents' Intention to choose public transit. |
H12b | Under COVID-19, Perceived Vulnerability has a positive impact on parents' Intention to choose private car. |
H12c | Under COVID-19, Perceived Vulnerability has a negative impact on parents' Intention to choose walk. |
H13a | Under COVID-19, Perceived Vulnerability has a negative impact on parents' Attitude of choosing public transit. |
H13b | Under COVID-19, Perceived Vulnerability has a positive impact on parents' Attitude of choosing private car. |
H13c | Under COVID-19, Perceived Vulnerability has a negative impact on parents' Attitude of choosing walk. |
Demographic variables | Sample size | Percentage (%) | |
Gender | Men | 217 | 47.17 |
Women | 243 | 52.83 | |
Age | 18–25 | 30 | 6.52 |
26–30 | 77 | 16.74 | |
31–35 | 154 | 33.48 | |
36–40 | 88 | 19.13 | |
41–45 | 56 | 12.17 | |
46–50 | 30 | 6.52 | |
Older than 50 | 25 | 5.44 | |
Education level | Primary (elementary/middle school) | 18 | 3.91 |
Secondary (high school) | 35 | 7.61 | |
Junior college | 108 | 23.48 | |
University (undergraduate) | 260 | 56.52 | |
Postgraduate | 39 | 8.48 | |
Family monthly income (RMB) | < 5000 | 10 | 2.01 |
5001–10,000 | 117 | 25.48 | |
10,001–15,000 | 123 | 26.74 | |
15,001–20,000 | 132 | 28.65 | |
> 20,000 | 78 | 17.12 | |
Occupation | Private enterprise | 197 | 42.83 |
Joint venture /foreign-invested enterprises | 32 | 6.96 | |
State-owned enterprises | 67 | 14.56 | |
Non-profit organization/institution | 3 | 0.65 | |
Government agencies | 26 | 5.65 | |
Schools, research institutes and other academic institutions | 51 | 11.09 | |
Other | 84 | 18.26 | |
Driver's license | Yes | 355 | 77.17 |
No | 105 | 22.83 | |
Number of car ownership | 0 | 54 | 11.74 |
1 | 294 | 63.91 | |
2 | 101 | 21.96 | |
3 | 11 | 2.39 | |
Number of electric bicycles owned | 0 | 107 | 23.26 |
1 | 196 | 42.61 | |
2 | 126 | 27.39 | |
3 | 23 | 5 | |
Number of children (<18 years old) | 1 | 386 | 83.91 |
2 | 68 | 14.78 | |
3 | 5 | 1.09 | |
4 | 1 | 0.22 | |
Child Gender | Boy | 270 | 58.7 |
Girl | 190 | 41.3 | |
Child age | 6–11 | 315 | 68.48 |
12–4 | 68 | 14.78 | |
15–18 | 77 | 16.74 | |
Distance from school to home | Less than 0.5 km | 68 | 14.78 |
0.5 km–1.0 km | 105 | 22.83 | |
1.0 km–-1.5 km | 77 | 16.74 | |
1.5 km–2.0 km | 50 | 10.87 | |
2.0 km–2.5 km | 58 | 12.61 | |
2.5 km–3.0 km | 25 | 5.43 | |
More than 3.0 km | 77 | 16.74 |
Psychological variables | N of Items | α | C.R. |
Perceived Built Environment | 6 | 0.772 | 0.877 |
Perceived Severity | 3 | 0.783 | 0.872 |
Perceived Vulnerability | 3 | 0.851 | 0.896 |
Subjective Norm | 3/3/3 | 0.776/0.786/0.779 | 0.898/0.838/0.703 |
Perceived Behavior Control | 2/2/2 | 0.732/0.745/0.718 | 0.815/0.880/0.833 |
Trust | 2/2/2 | 0.857/0.764/0.892 | 0.795/0.908/0.712 |
Attitude | 2/2/2 | 0.891/0.839/0.890 | 0.892/0.7854/0.807 |
Intention | 3/3/3 | 0.824/0.854/0.823 | 0.879/0.893/0.870 |
Note: α < 0.6, unacceptable reliability; 0.6≤α < 0.7, acceptable reliability; 0.7≤α < 0.8, high reliability; 0.8≤α < 0.9, very high reliability; α≥0.9, extremely high reliability. CR > 0.6, good reliability. |
Psychological variables | Observed variables | Normalization factor loading coefficient | AVE |
Perceived Built Environment | PBE1 | 0.847 | 0.703 |
PBE2 | 0.855 | ||
PBE3 | 0.813 | ||
PBE4 | 0.863 | 0.6863 | |
PBE5 | 0.841 | ||
PBE6 | 0.779 | ||
Perceived Severity | PS1 | 0.838 | 0.6951 |
PS2 | 0.84 | ||
PS3 | 0.823 | ||
Perceived Vulnerability | PV1 | 0.891 | 0.7415 |
PV2 | 0.865 | ||
PV3 | 0.826 | ||
Subjective Norm | SN1_pt / SN1_car / SN1_walk | 0.851/0.822/0.839 | 0.7467/0.633/0.877 |
SN2_pt / SN2_car / SN2_walk | 0.886/0.754/0.839 | ||
SN3_pt / SN3_car / SN3_walk | 0.855/0.809/0.837 | ||
Perceived Behavior Control | PBC1_pt / PBC1_car / PBC1_walk | 0.843/0.886/0.907 | 0.6874/0.785/0.909 |
PBC2_pt / PBC2_car / PBC2_walk | 0.815/0.872/0.918 | ||
Trust | TRUST1_pt / TRUST1_car / TRUST1_walk | 0.813/0.901/0.871 | 0.6593/0.8319/0.832 |
TRUST2_pt / TRUST2_car / TRUST2_walk | 0.811/0.924/0.817 | ||
Attitude | ATT1_pt / ATT1_car / ATT1_walk | 0.893/0.822/0.904 | 0.8046/0.6467/0.893 |
ATT2_pt / ATT2_car / ATT2_walk | 0.901/0.786/0.893 | ||
Intention | INT1_pt / INT1_car / INT1_walk | 0.824/0.842/0.796 | 0.706/0.736/0.670 |
INT2_pt / INT2_car / INT2_walk | 0.857/0.875/0.837 | ||
INT3_pt / INT3_car / INT3_walk | 0.842/0.856/0.857 | ||
Note: AVE > 0.5, good convergent validity; normalization factor loading coefficient > 0.5, good convergent validity. |
Index name | χ2/df | RMSEA | IFI | GFI | NFI | CFI |
Test critical value | < 3, Good | < 0.05, Excellent | > 0.90 | > 0.90 | > 0.90 | > 0.90 |
< 5, Accepted | 0.05-0.08, Good | |||||
Model parameter value (public transit) | 4.48 | 0.062 | 0.917 | 0.902 | 0.900 | 0.907 |
Model parameter value (private car) | 3.97 | 0.059 | 0.921 | 0.916 | 0.908 | 0.914 |
Model parameter value (walk) | 3.69 | 0.070 | 0.911 | 0.904 | 0.901 | 0.900 |
Null hypotheses | Path | Standardized Regression Coefficient (β) | Standard Error of Estimate (S.E.) | Significance (p) |
H1a | SN_pt→INT_pt | 0.152 | 0.060 | p < 0.05 |
H2a | ATT_pt→INT_pt | 0.471 | 0.046 | p < 0.01 |
H3a | PBC_pt→INT_pt | 0.163 | 0.051 | p < 0.01 |
H4a | TRUST_pt→SN_pt | 0.162 | 0.061 | p < 0.01 |
H5a | TRUST_pt→ATT_pt | 0.241 | 0.068 | p < 0.01 |
H6a | TRUST_pt→PBC_pt | 0.183 | 0.036 | p < 0.01 |
H7a | TRUST_pt→INT_pt | 0.083 | 0.048 | - |
H8a | PBE_pt→INT_pt | 0.174 | 0.057 | p < 0.01 |
H9a | PBE_pt→ATT_pt | 0.083 | 0.047 | p < 0.01 |
H10a | PS_pt→ATT_pt | -0.276 | 0.052 | p < 0.01 |
H11a | PS_pt→INT_pt | -0.227 | 0.039 | p < 0.01 |
H12a | PV_pt→INT_pt | -0.311 | 0.066 | p < 0.01 |
H13a | PV_pt→ATT_pt | -0.214 | 0.046 | p < 0.01 |
Gender | Age | Edu | Income | Car_number | Child_gender | Child _age | Distance | |
Trust_pt | 0.0735 | 0.065 | 0.036 | -0.046 | -0.104* | -0.069 | 0.064 | 0.068 |
(1.44) | (0.097) | (0.061) | (-0.033) | (-2.14) | (-1.62) | (1.33) | (0.67) | |
SN_pt | 0.056 | 0.016 | 0.049 | -0.026 | 0.023 | 0.067 | 0.055 | 0.092 |
(1.76) | (0.46) | (1.43) | (-0.68) | (0.68) | (1.02) | (1.06) | (1.66) | |
PBC_pt | 0.069 | 0.015 | -0.049 | -0.036 | -0.036 | 0.073 | 0.069 | 0.089 |
(1.76) | (0.46) | (-1.23) | (-1.00) | (-0.88) | (1.33) | (0.96) | (1.44) | |
ATT_pt | 0.051 | 0.094 | -0.098* | -0.117* | -0.135** | 0.067 | 0.064 | 0.012 |
(0.96) | (0.99) | (-1.97) | (-2.31) | (-2.94) | (1.22) | (0.66) | (0.031) | |
PBE | 0.046 | -0.016 | -0.039 | 0.094 | 0.064 | -0.056* | 0.074* | -0.107** |
(0.96) | (-0.15) | (-0.66) | (0.69) | (0.99) | (-1.97) | (2.04) | (-2.57) | |
PV | 0.091 | 0.046 | 0.019 | 0.081* | 0.098 | 0.056 | 0.078 | 0.095 |
(0.51) | (0.62) | (0.91) | (2.33) | (1.55) | (0.61) | (0.99) | (1.09) | |
PS | 0.041 | 0.066 | 0.091 | 0.097* | 0.033 | 0.016 | 0.069 | 0.035 |
(0.97) | (1.01) | (0.68) | (2.55) | (1.35) | (0.96) | (0.68) | (0.68) | |
INT_pt | 0.039 | -0.011 | -0.066 | -0.069* | -0.106* | 0.015 | 0.059 | 0.038 |
(0.98) | (-0.61) | (-0.98) | (-0.89) | (-2.37) | (0.89) | (0.61) | (0.15) | |
Significance level: non-significant p > 0.05, *** p < 0.001, ** p < 0.01, and * p < 0.05. |
Inspection index | Index fitting result | Explanation | |
Public transit | Private car | ||
Cox & Snell R2 | 0.571 | 0.557 | The model fits well |
Log likelihood | -3214.123 | -3172.462 | |
Hosmer-Lemeshow | > 0.05 | > 0.05 |
Attribute variable | Public transit | Private car | ||||
Parameter estimation | Z test | P test | Parameter estimation | Z test | P test | |
Constant | -2.574* | 5.12 | 0.00 | 2.146* | 3.54 | 0.01 |
Gender | 0.087 | 1.47 | 0.15 | -0.104* | -2.23 | 0.03 |
Age | 0.037 | 1.17 | 0.24 | -0.118* | 2.17 | 0.03 |
Edu | -0.066 | -1.37 | 0.27 | 0.126* | 4.33 | 0.00 |
Income | -0.038 | -1.35 | 0.31 | 0.347* | 5.21 | 0.00 |
Car_number | -0.154* | -3.21 | 0.01 | 0.139* | 4.92 | 0.00 |
Kid_gender | 0.084 | 1.17 | 0.24 | -0.105* | -6.34 | 0.00 |
Kid_age | 0.141 | 0.53 | 0.61 | -0.131* | -4.12 | 0.00 |
Distance | 0.046 | 0.17 | 0.89 | 0.119 | 0.55 | 0.42 |
Trust_pt | 0.134* | 3.11 | 0.01 | - | - | - |
SN_pt | 0.035 | 0.41 | 0.65 | - | - | - |
PBC_pt | 0.054 | 1.16 | 0.25 | |||
ATT_pt | 0.178* | 3.64 | 0.01 | - | - | - |
INT_pt | 0.314* | 3.96 | 0.01 | - | - | - |
Trust_car | - | - | - | 0.164* | 3.24 | 0.01 |
SN_car | - | - | - | 0.071* | 1.16 | 0.02 |
PBC_car | - | - | - | 0.015 | 0.65 | 0.51 |
ATT_car | - | - | - | 0.165* | 3.59 | 0.01 |
INT_car | - | - | - | 0.217* | 2.89 | 0.02 |
PV | -0.242* | -4.91 | 0.01 | 0.289* | 3.58 | 0.01 |
PS | -0.234* | -4.76 | 0.00 | 0.204* | 4.34 | 0.00 |
PBE | 0.104 | 1.56 | 0.10 | 0.143 | 1.33 | 0.19 |
Significance level: non-significant p > 0.05, *** p < 0.001, ** p < 0.01, and * p < 0.05. |