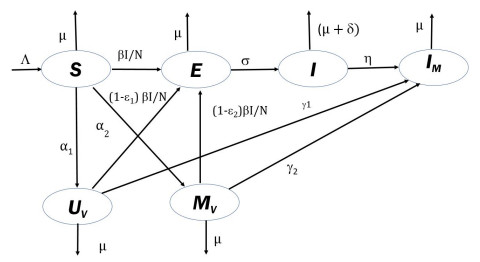
Measles is one of the highly contagious human viral diseases. Despite the availability of vaccines, measles outbreak frequently occurs in many places, including Nepal, partly due to the lack of compliance with vaccination. In this study, we develop a novel transmission dynamics model to evaluate the effects of monitored vaccination programs to control and eliminate measles. We use our model, parameterized with the data from the measles outbreak in Nepal, to calculate the vaccinated reproduction number, Rv, of measles in Nepal. We perform model analyses to establish the global asymptotic stability of the disease-free equilibrium point for Rv<1 and the uniform persistence of the disease for Rv>1. Moreover, we perform model simulations to identify monitored vaccination strategies for the successful control of measles in Nepal. Our model predicts that the monitored vaccination programs can help control the potential resurgence of the disease.
Citation: Anjana Pokharel, Khagendra Adhikari, Ramesh Gautam, Kedar Nath Uprety, Naveen K. Vaidya. Modeling transmission dynamics of measles in Nepal and its control with monitored vaccination program[J]. Mathematical Biosciences and Engineering, 2022, 19(8): 8554-8579. doi: 10.3934/mbe.2022397
[1] | N. Akhavan Kharazian, F. M. G. Magpantay . The honeymoon period after mass vaccination. Mathematical Biosciences and Engineering, 2021, 18(1): 354-372. doi: 10.3934/mbe.2021019 |
[2] | Muhammad Fakhruddin, Dani Suandi, Sumiati, Hilda Fahlena, Nuning Nuraini, Edy Soewono . Investigation of a measles transmission with vaccination: a case study in Jakarta, Indonesia. Mathematical Biosciences and Engineering, 2020, 17(4): 2998-3018. doi: 10.3934/mbe.2020170 |
[3] | Zuzana Chladná . Optimal time to intervene: The case of measles child immunization. Mathematical Biosciences and Engineering, 2018, 15(1): 323-335. doi: 10.3934/mbe.2018014 |
[4] | Deborah Lacitignola . Saturated treatments and measles resurgence episodes in South Africa: A possible linkage. Mathematical Biosciences and Engineering, 2013, 10(4): 1135-1157. doi: 10.3934/mbe.2013.10.1135 |
[5] | Eunha Shim, Beth Kochin, Alison Galvani . Insights from epidemiological game theory into gender-specific vaccination against rubella. Mathematical Biosciences and Engineering, 2009, 6(4): 839-854. doi: 10.3934/mbe.2009.6.839 |
[6] | Lili Liu, Xi Wang, Yazhi Li . Mathematical analysis and optimal control of an epidemic model with vaccination and different infectivity. Mathematical Biosciences and Engineering, 2023, 20(12): 20914-20938. doi: 10.3934/mbe.2023925 |
[7] | Miled El Hajji, Amer Hassan Albargi . A mathematical investigation of an "SVEIR" epidemic model for the measles transmission. Mathematical Biosciences and Engineering, 2022, 19(3): 2853-2875. doi: 10.3934/mbe.2022131 |
[8] | Maddalena Donà, Pieter Trapman . Possible counter-intuitive impact of local vaccine mandates for vaccine-preventable infectious diseases. Mathematical Biosciences and Engineering, 2024, 21(7): 6521-6538. doi: 10.3934/mbe.2024284 |
[9] | Siyu Liu, Yong Li, Yingjie Bi, Qingdao Huang . Mixed vaccination strategy for the control of tuberculosis: A case study in China. Mathematical Biosciences and Engineering, 2017, 14(3): 695-708. doi: 10.3934/mbe.2017039 |
[10] | Suxia Zhang, Hongbin Guo, Robert Smith? . Dynamical analysis for a hepatitis B transmission model with immigration and infection age. Mathematical Biosciences and Engineering, 2018, 15(6): 1291-1313. doi: 10.3934/mbe.2018060 |
Measles is one of the highly contagious human viral diseases. Despite the availability of vaccines, measles outbreak frequently occurs in many places, including Nepal, partly due to the lack of compliance with vaccination. In this study, we develop a novel transmission dynamics model to evaluate the effects of monitored vaccination programs to control and eliminate measles. We use our model, parameterized with the data from the measles outbreak in Nepal, to calculate the vaccinated reproduction number, Rv, of measles in Nepal. We perform model analyses to establish the global asymptotic stability of the disease-free equilibrium point for Rv<1 and the uniform persistence of the disease for Rv>1. Moreover, we perform model simulations to identify monitored vaccination strategies for the successful control of measles in Nepal. Our model predicts that the monitored vaccination programs can help control the potential resurgence of the disease.
Measles is one of the acute and highly contagious viral diseases found as early as the 7th century [1]. It is transmitted either by direct contact with infectious droplets or by airborne spread [2,3], mainly among children under five years. Before developing vaccines, measles epidemics used to occur every 2 to 5 years, resulting in an annual 20 to 30 million infections and at least 1 million deaths worldwide [4,5]. Measles incidence and related deaths decreased globally during 2000–2016 [6,7]. However, the measles cases have begun to increase again after 2017. The number of cases in 2019 reached the highest in the past 23 years. The death due to measles also increased by 22% in 2017 and climbed up to 50% in 2019 [6,7].
Measles can be successfully prevented with two doses of vaccines received at the recommended ages of 9 months (first dose) and 15 months (second dose) [8]. However, due to various reasons, such as poor health systems, lack of access to vaccination, and fear or skepticism about vaccines, the proper implementation of vaccination programs has been a huge issue. Improper and insufficient vaccines have presumably caused frequent measles outbreaks in developed and developing countries, including Nepal. In 2017, the global coverage of the vaccine's first dose was about 85%, significantly lower than the 95% recommended by the WHO to achieve herd immunity [5]. Even among developed countries, such as the United States, France, United Kingdom, Argentina, Italy, Japan, Canada, Germany, Australia, and Chile, the vaccine coverage has not met the WHO-recommended threshold for herd immunity [5]. Efforts by the anti-vaccine activists in the world [9,10] might have partially contributed to the low vaccine coverage and eventually to occasional outbreaks, such as the one in New York in 2018–2019 [11,12].
In Nepal, the monovalent vaccine, known as MCV1, against measles was first introduced in three districts in 1979. It was later expanded to the whole country in 1989 [13,14,15]. Measles was one of the major causes of childhood death before 2007, presumably because of the low coverage of MCV1. After the MCV1 coverage was increased from 81% to 88% during 2007-2014 along with Supplementary Immunization Activities (SIAs), the suspected measles incidence declined by 13% [14,15,16,17,18,19]. However, the measles cases began to increase in Nepal in 2017 (99,247, and 430 measles cases in 2017, 2018, and 2019, respectively) [20,21]. In 2019 measles outbreaks occurred in Morang, Dang, Kapilvastu, Kathmandu, and Lalitpur districts [20,21,22,23]. Notably, a frequent measles outbreak has been reported in districts with low vaccine coverage like Kapilvastu, where 95% of children were not vaccinated in 2016 [24,25,26]. Even in vaccinated people, the protection level may be reduced due to improper vaccination timing and incomplete doses. Monitored vaccination programs may help achieve success in avoiding measles epidemics. Such monitored vaccination programs promote the timely completion of the vaccination, thereby increasing the chance of complete immunity gain. Mathematical modeling is a valuable tool for identifying the ideal monitored vaccination programs in the context of Nepal.
Many SIR (susceptible, infected, recovered) based deterministic models, including age-structure and immigration-impact, have already been developed for the transmission dynamics of measles [27,28,29,30,31,32]. Moreover, some SEIR (susceptible, exposed, infected, recovered) based models [33,34,35,36] have been developed, including continuous-time linear vaccination-based control strategy, meta-populations, and immunization in pregnant women. These basic models have also been extended to include immunity, vaccination, age-dependent vaccination, time-dependent vaccine efficacy, therapy, quarantine, and treatment [2,37,38,39,40,41,42,43,44]. However, modeling has not been extensively explored to study monitored vaccination programs, especially in the context of Nepal.
Regarding measles in Nepal, some descriptive, analytic, and retrospective studies [14,45,46,47] have provided insights into the progress in measles control, the Case Fatality Rate (CFR) of measles, and the genetic type of the Asian measles virus. Many of these studies have incorporated the vaccination but lack a monitored vaccination program. In this study, we develop a novel deterministic model, incorporating monitored vaccination programs with two classes of vaccinated individuals, one under the monitored program and another without the monitored program. The model is validated using two-decade-long measles data from Nepal. Our model analysis establishes the local and global stability of disease-free equilibrium, the existence of endemic equilibrium, and the uniform persistence of the disease. Furthermore, we carry out model simulations to properly evaluate the impact of monitored vaccination programs on the short-term and long-term trend of measles transmission in Nepal.
We develop a transmission dynamics model of measles for the population that includes all the newborns and children under 15 years. As mentioned earlier, implementing a proper vaccination program is often difficult, particularly for children whose parents have poor health knowledge and have fear or skepticism about vaccines. To improve the effectiveness of vaccination programs, we introduce a monitored vaccination program into our model. The program mainly focuses on asserting the completion of vaccines timely and accurately by children under this program. Because of extra care and regular follow-up, the children under this program are expected to have less susceptibility to infection and a higher rate of achieving immunity than those under regular (un-monitored) vaccination programs. To formulate the model, we divide the total population considered (N) into six mutually exclusive compartments: susceptible (S), un-monitored vaccinated (UV), monitored vaccinated (MV), exposed (E), infectious (I), and immune (IM).
The schematic diagram showing the flow of individuals from and to the compartments during the measles transmission dynamics is presented in Figure 1. The dynamical system equations representing the model are as follows:
dSdt=Λ−(βIN+μ+α1+α2)S, | (2.1) |
dUVdt=α1S−(γ1+μ+(1−ε1)βIN)UV, | (2.2) |
dMVdt=α2S−(γ2+μ+(1−ε2)βIN)MV, | (2.3) |
dEdt=βISN+(1−ε1)βIUVN+(1−ε2)βIMVN−(μ+σ)E, | (2.4) |
dIdt=σE−(μ+η+δ)I, | (2.5) |
dIMdt=γ1UV+γ2MV+ηI−μIM. | (2.6) |
Here, Λ represents the recruitment rate of susceptible, i.e., newly born children. The measles infection occurs with the per capita transmission rate of βI/N, transferring susceptible individuals to the exposed class. The parameters σ represents the rate of progression of individuals from the exposed class to the infectious class, and η is the recovery rate of infectious individuals from the disease. Since only the children up to the age of 15 years are considered in the study, we assumed μ is the rate at which the children become older than 15 years leaving from the dynamics. We take δ to represent the disease-induced death rate.
The parameters α1 and α2 represent the rate of un-monitored vaccination and the monitored vaccination, respectively. As discussed in Pantha et al. [49], for practical purposes, the values of α1 and α2 for un-monitored and monitored vaccination programs aiming to cover ζ1% and ζ2% of children in t1 and t2 years, respectively, can be estimated using α1=−ln(1−ζ1/100)/t1 and α2=−ln(1−ζ2/100)/t2, respectively. The individual in the monitored vaccinated group is expected to be properly monitored to ensure the timely completion of the vaccination. In contrast, individuals in the un-monitored vaccinated group have more likelihood of not completing the vaccination in time, possibly delaying the gain of complete immunity. Therefore, we assumed that the un-monitored and monitored vaccinated children become immune at different rates, γ1 and γ2, respectively. Our model assumes individuals recovered from natural infection and those completing vaccination have similar immunity. Therefore, we include both of them in the same class, namely the immune class (IM). The vaccinated children may also be infected, but at lesser infectivity rates (1−ε1)β and (1−ε2)β for un-monitored and monitored vaccinated, respectively. Since the monitoring service providers are expected to counsel individuals in the monitored vaccinated program for timely completion of vaccine and prevention practices, we also expect 0≤ε1<ε2≤1.
The publicly available data used in this work is obtained from the official site of the World Health Organization (WHO) [48]. The data includes the reported measles cases in Nepal from 2000 to 2019. Since 5% of the reported cases belong to the aged 15 and above [48], we deducted 5% of the cases from the data. The Crude Birth Rate (CBR) and Infant Mortality Rate (IMR) of Nepal are used from the "Nepal population growth rate 1950–2020" [50].
Nepal's population under fifteen years was 9,807,000 in 2000 (taken as the base year) and 8,460,000 in 2019 [48]. The actual population size in individual S,UV,MV,E,I,IM, classes is not available. It is recorded that 77% of the population was vaccinated in 2000 (the base year) [48]. For our base case simulation, among the unvaccinated 23% of the total population, we assumed that 22% were in the susceptible class (S(0)=2,157,540). We took 30% of the vaccinated population were in the un-monitored vaccinated class (UV(0)=2,206,575). Since the monitored vaccination program was not present in 2000, we took MV(0)=0. From the data, the recorded cases were 8927 (after reducing 5% over 15 years from the total recorded 9397), among which we assume E(0)=300 in the exposed class and I(0)=340 in the infectious class. The remaining population (N(0)−S(0)−UV(0)−E(0)−I(0)) is included in the immune class (IM(0)=5,442,245).
The recruitment rate (Λ=612,328) is the annual average birth rate, which is calculated by using the Crude Birth Rate (CBR) and Infant Mortality Rate (IMR) from the 2000-2019 data [50]. Since only the population below fifteen years is considered in the study, we used μ=1/15=0.0667 per year. It is given that the incubation period of measles is 10–14 days on average [4,51], and thus the disease progression rate from the exposed class to the infectious class is taken as σ=1/12×365≈30 per year. Also, since it takes about 18 days (range between 7 to 23 days) to recover from the disease [51], we used η=1/18×365≈20 per year. As per WHO guidelines, children are vaccinated with the first dose at the age of 9 months and the second dose at 15 months [8], giving a six-month interval between the two doses. Since the monitored vaccinated individuals (MV) are expected to complete them in time, we took γ2=0.5 per year. From the data [52,53], we estimated the average disease-induced death rate to be δ=0.01 per year. The remaining parameters of the model, β,α1,α2,γ1,ε1, and ε2, are estimated by fitting the model to the measles case data from Nepal.
From the model, the yearly new infections at time t can be calculated using h(t)=σE(t), which we obtained using the numerical solutions of the system (2.1–2.6). Then we estimated the parameters with the help of the nonlinear regression method [54], which minimizes the following sum of the square residuals:
n∑k=1(hk−¯hk)2, |
where hk denotes the model predicted yearly new infection, ¯hk denotes yearly new infection data, and n is the number of data points used for the model fitting. For each estimated parameter, we also computed the confidence limits using the standard errors from the sensitivity matrix (S) based on the complex-step derivative technique described in the previous study [55,56,57,58]. Our model is consistent with the yearly incidence cases observed in Nepal (Figure 2). In addition, we also show that the model prediction of the cumulative cases agrees well with the cumulative data, thereby validating our model to describe the measles epidemic in Nepal.
We note that while making all six parameters free in the data fitting process, we obtained negative lower limits of some confidence intervals. To tackle this issue, we needed to fix some of the parameters as done previously [55,56,57]. Since the parameters α2 and ε2 are the two least sensitive parameters identified from the sensitivity matrix, S, we fixed α2=0.02 per year and ε2=0.90 at their best estimate values. We also note that taking the different values of α2 and ε2 did not significantly affect estimates of other parameters, as expected, because of the least sensitivity. Then, we estimated the remaining only four parameters, β,α1,γ1,and ε1, from the further data fitting. Here the ratio of the data to the free parameters is 5:1, which lies within the recommended range of 5:1 to 10:1 for a reasonable parameter estimate [59]. Furthermore, to analyze the identifiability of the estimated four parameters, we use the sensitivity-based method [60], in which we obtained the rank of the matrix STS. For our case with four parameters estimated, the obtained full rank (Rank = 4) of the matrix STS confirms that these four parameters are practically identifiable for the model and the data used in this study. All the computations were carried out in MATLAB (The Math Works. Inc.) using its various routines, including "ode45" (ODE solver) and "fmincon" (minimizer).
Our estimates show that the transmission rate (β), the rate of un-monitored vaccination (α1), the rate of recovery from un-monitored vaccination (γ1), and the effectiveness of un-monitored vaccination (ε1) are 63.0238 (95% CI: 63.0188–63.0288), 0.31 (95% CI: 0.0902–0.5298), 0.1 (95% CI: 0.0858–0.1142), and 0.5082 (95% CI: 0.4926–0.5238), respectively (Table 1). We note that the confidence interval of the parameter α1 appears to be large compared to other parameters for this particular data set. However, we consider this parameter a control parameter and vary widely for the analysis of the vaccination program; thus, one set of confidence intervals does not significantly impact the main results of our study.
Parameters (yr)−1 | Description | Baseline Value | Confidence Interval | Sources |
α2 | Monitored | 0.02 | Fixed | Assumed |
vaccination rate | ||||
α1 | Un-monitored | 0.31 | [0.0902 0.5298] | Data Fitting |
vaccination rate | ||||
β | Transmission rate | 63.02 | [63.01 63.03] | Data Fitting |
ε1 | Effectiveness of | 0.51 (Dim.less) | [0.49 0.52] | Data Fitting |
un-monitored vacc. | ||||
ε2 | Effectiveness of | 0.9 (Dim.less) | Fixed | Assumed |
monitored vacc. | ||||
μ | Removed rate | 0.0667 | Fixed | Assumed |
γ1 | UV immunity rate | 0.1 | [0.086 0.12] | Data Fitting |
γ2 | MV immuninity rate | 0.5 | Fixed | Assumed |
σ | Disease progress rate | 30 | Fixed | [4] |
η | Recovery rate | 20 | Fixed | [4] |
δ | Disease-induced | 0.01 | Fixed | [52] |
death rate |
We first establish the biological or epidemiological validation of the model by proving the non-negativity and the boundedness of the solution of the system of equations.
Theorem 4.1. If S(0)>0,UV(0)≥0,MV(0)≥0,E(0)≥0,I(0)≥0, and IM(0)≥0, then the set of solution {S(t),UV(t),MV(t),E(t),I(t),IM(t)} of the system (2.1–2.6) is positive for all t≥0.
Proof. From (2.1), dSdt>−(βI/N+μ+α1+α2)S, which implies S(t)>S(0)exp(−∫t0(βI(s)/N(s)+μ+α1+α2)ds). Since S(0)>0,S(t)>0,∀t>0 confirming the positivity of S(t). Similarly from (2.2–2.6), we have dUVdt≥−(γ1+μ+(1−ε1)βI/N)UV, dMVdt≥−(μ+γ2+(1−ε2)βI/N)MV, dEdt≥−(μ+σ)E, dIdt≥−(μ+η+δ)I, and dIMdt≥−μIM. Then we get UV(t)≥UV(0)exp(−∫t0((1−ε1)βI(s)/N(s)+μ+γ1)ds)≥0, MV(t)≥MV(0)exp(−∫t0((1−ε2)βI(s)/N(s)+μ+γ2)ds)≥0, E(t)≥E(0)exp(−(μ+σ)t)≥0, I(t)≥I(0)exp(−(μ+η+δ)t)≥0, and IM(t)≥IM(0)exp(−μt)≥0,∀t>0 for UV(0)≥0,MV(0)≥0,E(0)≥0,I(0)≥0, and IM(0)≥0. Therefore, UV(t),MV(t),E(t),I(t),IM(t)≥0,∀t>0, showing the positivity of the solution set of the system (2.1–2.6).
Adding all differential equations (2.1–2.6), we get dN/dt=Λ−μN−δI≤Λ−μN, which provides N(t)≤N(0)e−μt+Λ/μ(1−e−μt) and lim supt→∞N(t)≤Λ/μ, showing that the total population is ultimately bounded by Λ/μ. Hence the solution set bounded by Λ/μ is positively invariant in the feasible region
Ω={(S(t),UV(t),MV(t),E(t),I(t),IM(t))∈R6+:N(t)≤Λ/μ}. |
In this section we discuss the disease-free equilibrium point (DFE) and the endemic equilibrium point of the system (2.1–2.6).
Setting E=0 and I=0 in the system, we obtain DFE: E0=(S0,U0V,M0V,0,0,I0M), where S0=Λα1+α2+μ, U0V=α1Λ(α1+α2+μ)(γ1+μ), M0V=α2Λ(α1+α2+μ)(γ2+μ), and I0M=Λ(α1γ1(μ+γ2)+α2γ2(μ+γ1))μ(α1+α2+μ)(γ1+μ)(γ2+μ). Note that our model implies non-zero immune class I0M at the DFE due to the immunity gained through vaccination.
The vaccinated reproduction number, denoted by Rv, is defined as the average number of secondary cases generated by a single infectious case introduced into the mixed population with susceptible and vaccinated status. Here we formulate the vaccinated reproduction number using the Next Generation method [61,62,63,64,65].
Following the Next Generation Matrix method [62], we divide the system into two groups, infected →x=(xi,i=1,2)=(E,I) and uninfected →y=(yj,j=1,2,3,4)=(S,UV,MV,IM), as follows:
˙xi=fi(→x,→y)=Fi(→x,→y)−Vi(→x,→y),i=1,2and ˙yj=gj(→x,→y),j=1,2,3,4, | (4.1) |
where Fi(→x,→y) is the rate of appearance of new infections in the compartment i, and Vi(→x,→y) is the difference between the transfer of individuals out of and into the compartment i (i=1,2). Here we have
F=((βS+(1−ε1)βUV+(1−ε2)βMV)I/N0) and V=((μ+σ)E−σE+(μ+η+δ)I). |
It is easy to verify that Fi and Vi satisfy the conditions A(1)–A(5) of [62]. As the process provided in [62], we obtained the Jacobians of F and V at the disease free equilibrium point (DF(E0)=F,DV(E0)=V):
F=(0(βS0+(1−ε1)βU0V+(1−ε2)βM0V)/N000) and V=(μ+σ0−σδ+η+μ). |
Clearly, F is non-negative and V is non singular M-matrix. The next generation matrix for our model is given by:
FV−1=(σ(βS0+(1−ε1)βU0V+(1−ε2)βM0V)N0(μ+σ)(δ+η+μ)(βS0+(1−ε1)βU0V+(1−ε2)βM0V)N0(δ+η+μ)00). |
Then the vaccinated reproduction number Rv is given by:
Rv=ρ(FV−1)=βσ(S0+(1−ε1)U0V+(1−ε2)M0V)N0(μ+σ)(δ+η+μ). |
Using the expression of DFE obtained above, we get
Rv=βμσ(α1(1−ε1)(γ2+μ)+(γ1+μ)(α2(1−ε2)+γ2+μ))(α1+α2+μ)(γ1+μ)(γ2+μ)(μ+σ)(δ+η+μ). |
Furthermore, as the system (4.1) satisfies all the conditions of [62,Theorem 2], it follows the local stability of the disease-free equilibrium for RV<1. Moreover, for the verification purpose, we also prove the local stability of DFE by showing the negative real part of eigenvalues in Subsection 4.3.1 below.
Let
λ∗=βI∗/(S∗+U∗v+M∗v+E∗+I∗+I∗M). | (4.2) |
Then solving the full system (2.1–2.6) equated to zero, we get
S∗=Λα1+α2+λ∗+μ,U∗V=α1ΛQ1(α1+α2+λ∗+μ),M∗V=α2Λ(γ2+μ)(α1+α2+μ+λ∗(1−ε2)),E∗=λ∗Λ(α1(1−ε1)(γ2+λ∗(1−ε2)+μ)+Q1(γ2+(α2+λ∗)(1−ε2)+μ))Q1(μ+σ)(α1+α2+λ∗+μ)(γ2+λ∗(1−ε2)+μ),I∗=λ∗Λσ(Q1(γ2+(α2+λ∗)(1−ε2)+μ)+α1(1−ε1)(γ2+λ∗(1−ε2)+μ))Q1(μ+σ)(α1+α2+λ∗+μ)(δ+η+μ)(γ2+λ∗(1−ε2)+μ),I∗M=Λ(P1+P2P3)Q1μ(μ+σ)(α1+α2+λ∗+μ)(δ+η+μ)(γ2+λ∗(1−ε2)+μ). | (4.3) |
where,Q1=(γ1+λ∗(1−ε1)+μ),P1=α1(γ2+(1−ε2)+μ)(η(1−ε1)λ∗σ−γ1(μ+σ)(δ+η+μ)),P2=(γ1+λ∗(1−ε1)+μ),P3=(α2(γ2(μ+σ)(δ+η+μ)+ηλ∗(1−ε2)σ)+ηλ∗σ(γ2+λ∗(1−ε2)+μ)). |
Substituting (4.3) into (4.2) and after some simplification, we obtain the following equation in terms of λ∗:
λ∗(A3λ∗3+A2λ∗2+A1λ∗+A0)=0, | (4.4) |
where,
A3=(δμ+(η+μ)(μ+σ))(1−ε1)(1−ε2),A2=(1−ε1)((1−ε2)P+α1Q+α2Q)+(1−ε2)Q(γ1+μ)+Q(γ2+μ),A1=(1−ε1)P(γ2+μ)+(γ1+μ)((1−ε2)(α1(μ+σ)(δ+η+μ)+P+α2Q)+Q(γ2+μ))+(1−ε1)(α2((γ2+μ)(μ+σ)(δ+η+μ)−β(1−ε2)μσ)+α1(Q(γ2+μ)−β(1−ε2)μσ)),A0=(1−Rv)(α1+α2+μ)(γ1+μ)(γ2+μ)(μ+σ)(δ+η+μ),P=μ((δ+η+μ)(μ+σ)−βσ),Q=δμ+(η+μ)(μ+σ). |
Note that λ∗=0 corresponds to the disease-free equilibrium point, and the endemic equilibrium points are given by the solutions of A3λ∗3+A2λ∗2+A1λ∗+A0=0. Here, A3>0 and A0<0 for Rv>1, so the equation (4.4) has at least one positive root for Rv>1. The positiveness of λ∗ implies the positiveness of I∗ and E∗. Thus, we conclude that if Rv>1, the system (2.1–2.6) has at least one endemic equilibrium point given by (4.3).
In this section, we first establish the local and global stability of the disease-free equilibrium when Rv<1 and then prove the persistence of the disease when Rv>1.
Theorem 4.2. The disease-free equilibrium point of the system (2.1–2.6) is locally asymptotically stable if Rv<1 and unstable if Rv>1.
Proof. Jacobian of the system (2.1–2.6) at the disease free equilibrium point is J=(A3×3B3×3C3×3D3×3) where,
A3×3=(−(α1+α2+μ)00α1−(γ1+μ)0α20−(γ2+μ)),B3×3=(0−βS0/N000−βU0V(1−ε1)/N000−βM0V(1−ε2)/N00), |
C3×3=(0000000γ1γ2),D3×3=(−(μ+σ)β(S0+U0V(1−ε1)+M0V(1−ε2))/N00σ−(δ+η+μ)00η−μ). |
The eigenvalues of the matrix J are
λ1=−μ,λ2=−(α1+α2+μ),λ3=−(γ1+μ),λ4=−(γ2+μ),λ5=12(−(δ+η+2μ+σ)−√(δ+η+2μ+σ)2+4(Rv−1)(μ+σ)(δ+η+μ)),λ6=12(−(δ+η+2μ+σ)+√(δ+η+2μ+σ)2+4(Rv−1)(μ+σ)(δ+η+μ)). | (4.5) |
Since all the eigenvalues are negative if Rv<1, E0 is locally asymptotically stable if Rv<1.
In the following theorem, we prove that Rv<1 asserts the global stability of the DFE.
Theorem 4.3. If Rv<1, the disease-free equilibrium point E0 of the system (2.1–2.5) is globally asymptotically stable in R6.
Proof. The Jacobian corresponding to (2.4) and (2.5) at the disease-free equilibrium point E0 is
J0=(−μ−σβS0N0+(1−ε1)βU0VN0+(1−ε2)βM0VN0σ−(δ+η+μ)). |
The spectral bound of J0 is defined as: s(J0)=max{Re(λ):λis an eigenvalue of J0}. Using the theorem 4.2, we conclude:
1. Rv=1 if and only if s(J0)=0.
2. Rv<1 if and only if s(J0)<0.
3. Rv>1 if and only if s(J0)>0.
If Rv<1 then s(J0)<0. Also, we can obtain a sufficiently small positive number γ such that s(Jγ)<0, where
Jγ=(−μ−σβ(S0+γ)Λ/μ−γ+(1−ε1)β(U0V+γ)Λ/μ−γ+(1−ε2)β(M0V+γ)Λ/μ−γσ−(δ+η+μ)). |
From the subsections 4.1 and 4.2, N(t)→Λ/μ and S(t)→S0 as t→∞ for all γ>0. Therefore, there exists t1>0 such that ∀t≥t1, we have S≤(S0+γ),Uv≤(U0v+γ),Mv≤(M0v+γ),N≥(Λ/μ−γ). From (2.4) and (2.5), it follows that
dEdt=βSNI+(1−ε1)βUVNI+(1−ε2)βMVNI−(μ+σ)E≤β(S0+γ)Λ/μ−γI+(1−ε1)β(U0V+γ)Λ/μ−γI+(1−ε2)β(M0V+γ)Λ/μ−γI−(μ+σ)E,dIdt=σE−(μ+η+δ)I. |
dEdt=β(S0+γ)Λ/μ−γI+(1−ε1)β(U0V+γ)Λ/μ−γI+(1−ε2)β(M0V+γ)Λ/μ−γI−(μ+σ)E,dIdt=σE−(μ+η+δ)I,∀t≥t1. | (4.6) |
Clearly, the system (4.6) has the Jacobian Jγ, which is irreducible with non-negative off-diagonal elements. Then s(Jγ) is simple and associated with strongly positive eigenvector ˜v,∀t≥t1 [66]. For any solution ψ(t) of the system (4.6) with non-negative initial value ψ(0), there is a sufficiently large positive number ζ>0 such that (E(t1),I(t1))≤ζ˜v. It is easy to see that V(t)=ζes(Jγ)(t−t1)˜v,∀t≥t1 is a solution of (4.6) with V(t1)=ζ˜v. Then by the comparison principle [66,Theorem B.1], it follows that (E(t),I(t))≤ζ˜v,∀t≥t1. Since s(Jγ)<0, we get
limt→∞E(t)=0,limt→∞I(t)=0, |
which implies that (2.1) is asymptotic to the following equation:
dSdt=Λ−(μ+α1+α2)S,⇒S(t)=C1e−(μ+α1+α2)t+Λ(μ+α1+α2)(1−e−(μ+α1+α2)t),∀t≥t1. |
Hence, we get limt→∞S(t)=Λ(μ+α1+α2). Solving the system of differential equations (2.2 & 2.3) together with the help of (2.1), we get
UV=α1Λ(α1+α2+μ)(γ1+μ)+c1e−r1t+c2e−r2t,MV=α2Λ(α1+α2+μ)(γ2+μ)+c3e−k1t+c4e−k2t, |
where,
r1=k1=α1+α2+μ,r2=γ1+μ, and k2=γ2+μ. |
Thus, we get
limt→∞UV(t)=α1Λ(μ+α1+α2)(γ1+μ), | (4.7) |
limt→∞MV(t)=α2Λ(μ+α1+α2)(γ2+μ). | (4.8) |
In the limiting case, we can further verify that limt→∞IM(t)=I0M. Hence if Rv<1, the disease-free equilibrium point E0 of the system (2.1–2.5) is globally asymptotically stable.
The disease is endemic if the system is uniformly persistent. The system (2.1–2.6) is said to be uniformly persistent if there exists ζ>0 such that (S(t),UV(t),MV(t),E(t),I(t),IM(t))∈Γ satisfying
limt→∞Inf E≥ζ,limt→∞Inf I≥ζ. | (4.9) |
Here it is enough to consider the reduced system (2.1-2.5). We first define the sets
Γo={(S,UV,MV,E,I)∈R5:I≠0 or E≠0}, | (4.10) |
∂Γo={(S,UV,MV,E,I)∈R5:I=0,E=0}. | (4.11) |
We have
Γo∪∂Γo=Γ and Γo∩∂Γo=ϕ, |
which implies that ∂Γo is relatively closed in Γ=(S,UV,MV,E,I)∈R5.
To establish the disease persistence for Rv>1, we now prove the following theorem.
Theorem 4.4. If Rv>1, then the system (2.1–2.5) is uniformly persistent with respect to (Γ0,∂Γ0) in the sense that there is a positive constant ζ>0 such that every solution (S(t),UV(t),MV(t),E(t),I(t)) of (2.1–2.5) with (S(0),UV(0),MV(0),E(0),I(0))∈Γ0 satisfieslimt→∞InfE≥ζ,limt→∞InfI≥ζ.
Proof. Let ψ(t)p be the solution function of system (2.1–2.5) with initial value p. We can show the solution (ψ(t)p),t>0 is uniformly persistent with respect to (Γo,∂Γo) [67]. For any (S(0),UV(0),MV(0),E(0),I(0))∈Γ0, (2.1–2.3) provide
S(t)=exp(−∫t0a(x1)dx1)[∫t0exp(∫y0a(x1)dx1)b(y)dy+S(0)],UV(t)=exp(−∫t0a∗(x1)dx1)[∫t0exp(∫y0a∗(x1)dx1)b∗(y)dy+UV(0)],MV(t)=exp(−∫t0ao(x1)dx1)[∫t0exp(∫y0ao(x1)dx1)bo(y)dy+MV(0)], |
where a=α1+α2+μ+βI/N,b=Λ,a∗=γ1+μ+(1−ε1)βI/N, b∗=α1S,ao=γ2+μ+(1−ε2)βI/N, and bo=α2S. Here Λ>0 implies S(t)>0. This follows UV(t)>0,MV(t)>0,∀t>0. Thus the non-diseased variables S(t),UV(t),andMV(t) are positive.
Defining the two sets,
M∂={p∈∂Γo:ψ(t)p∈∂Γo}, |
ω(p)={p:ψ(t)xn→p as t→∞}, |
we claim that ω(p)={E0},∀p∈M∂. If p(t)∈M∂ then ψ(t)p∈∂Γ0 which implies I=0 and E=0. From (4.7, 4.8) and (2.1–2.5), as t→∞ we obtain S(t),UV(t), and MV(t) approaching to
Λ(μ+α1+α2),Λα1(μ+α1+α2)(γ1+μ), and Λα2(μ+α1+α2)(γ2+μ),respectively, |
which is the equilibrium point E0. Hence ω(p)={E0},∀p∈M∂.
Using the theorem 4.3 (Condition 3, Rv>1 for s(J0)>0), we can get a sufficiently small ρ>0 such that the perturbation Jρ on J0 satisfies s(Jρ)>0, (see [67], [68,Section II.5.8]) for Rv>1, where
Jρ=(−μ−σβ(S0−ρ)Λ/μ+ρ+(1−ε1)β(U0V−ρ)Λ/μ+ρ+(1−ε2)β(M0V−ρ)Λ/μ+ρσ−δ−η−μ). |
Now we claim that disease-free equilibrium point E0 is uniform weak repeller with any solution ψ(t). For this we need to show
limt→∞ Sup ‖ψ(t)p−E0‖≥ρ,∀p∈Γ0. |
On contrary, suppose there exists a p0∈Γ0 such that limt→∞ Sup ‖ψ(t)p0−E0‖<ρ. From the subsections 4.1 and 4.2, N(t)→Λ/μ and S(t)→S0 as t→∞ implying that for all ρ>0 there exists t2>0 such that ∀t≥t2, S≥(S0−ρ),Uv≥(U0v−ρ),Mv≥(M0v−ρ),N≤(Λ/μ+ρ). Then (2.4) and (2.5) follows that,
dEdt=βSNI+(1−ε1)βUVNI+(1−ε2)βMVNI−(μ+σ)E≥β(S0−ρ)Λ/μ+ρI+(1−ε1)β(U0v−ρ)Λ/μ+ρI+(1−ε2)β(M0v−ρ)Λ/μ+ρI−(μ+σ)E,dIdt=σE−(μ+η+δ)I. |
We consider the following auxiliary equations:
dEdt=β(S0−ρ)Λ/μ+ρI+(1−ε1)β(U0v−ρ)Λ/μ+ρI+(1−ε2)β(M0v−ρ)Λ/μ+ρI−(μ+σ)E,dIdt=σE−(μ+η+δ)I. | (4.12) |
Here, Jρ is the Jacobian of the system (4.12), is irreducible with non-negative off-diagonal elements, then s(Jρ) is simple and associated with strongly positive eigenvector ˜v,∀t≥t2, (E(t2),I(t2))>0. Thus there is a positive number ξ>0 such that (E(t2),I(t2))≥ξ˜v holds. It is easy to see that V=ξes(Jρ)(t−t2)˜v,∀t≥t2 is a solution of (4.12) with V(t2)=ξ˜v. Hence by comparison principle [66,Theorem B.1], we get
(E(t),I(t))≥ξes(Jρ)(t−t2)˜v,∀t≥t2. |
Also, for Rv>1, we have s(Jρ)>0, implying limt→∞E(t)=∞,limt→∞I(t)=∞, which is a contradiction. This proves that the solution repels from E0. It follows that forward orbit of any solutions in M∂ converges to E0 is isolated in R5.
Now, we define a stable set of E0:
Ws(E0)={p∈Γ:d(ψ(t)p,E0)→0 as t→∞}. |
Clearly, Ws(E0)∩Γ0=∅. It follows that there is no cycle in M∂ from E0 to E0. Applying [69,Theorem 1.3.1], we conclude that the system (2.1–2.5) is uniformly persistent, i.e., there exists ζ>0 satisfying (4.9).
We first present the properties of basic dynamics of measles in Nepal, particularly vaccinated reproduction numbers and long-term dynamics.
Using the estimated parameters (Table 1), we obtain the reproduction number of measles in the presence of vaccination in Nepal to be Rv=1.0098. While Rv>1 is consistent with the currently ongoing endemic of measles, the actual magnitude we observed is significantly lower than the previously estimated reproduction number between 5 and 18 in other places [70]. The low value of Rv is expected as its estimate is based on the parameters influenced by the vaccination program. We now compute the time-dependent effective reproduction number, Rt, which describes the time-varying average number of secondary cases. The value of Rt allows us to track whether the epidemic at time t is in an increasing (Rt>1) or decreasing (Rt<1) trend. For our model, the effective reproduction number is given by
Rt=βσ(S(t)+UV(t)(1−ε1)+MV(t)(1−ε2))N(t)(μ+σ)(δ+η+μ). |
Using the estimated parameters (Table 1), we obtained the pattern of Rt as shown in Figure 3. As predicted by our model, the value of the effective reproduction number remains less than unity, indicating the epidemic is in decreasing trend until 2030, after which Rt increases and remains greater than unity until 2095, showing the increasing trend of the disease in the period 2030–2095 (Figure 3).
In this section, we present our model prediction for the long-term dynamics of measles transmission in Nepal. If the current trend continues, there will still be 178 cases in 2023 (Figure 4), indicating an obstacle to the measles elimination program set by the government of Nepal. Our model predicts that the cases remain at a significantly low level until 2033 but persist at a low level without being eradicated. After 2033, the resurgence of the outbreak will begin and reach the peak value (7670) in 2097. The dynamic is relatively slow, reaching a steady-state only after about 2100. The resurgence of measles in Nepal predicted by our model supports the worldwide trend of the epidemic, which shows an eventual resurgence in many places such as the UK, the US (31 states including New York), and the Philippines. We also predict the contribution of susceptible (unvaccinated), un-monitored vaccinated, and monitored vaccinated to the resurgence of the measles cases. As per our model prediction, the major contribution to the resurgence is from the susceptible and the un-monitored vaccinated groups. Note that the un-monitored vaccinated group can be significantly high in Nepal, as shown by the data that while 92% are vaccinated with MCV1, only about 76% are vaccinated with MCV2 in 2019 [48]. Therefore, proper implementation of the monitored vaccination program may be needed to avoid the resurgence of the disease.
We first observe the local sensitivity of Rv to each of the parameters. For this, we obtain the sensitivity index Sx, given by
Sx=(xRv)(∂Rv∂x), |
where x is the parameter of which the sensitivity is to be obtained. Based on Sx, we found that Rv is highly sensitive to β. The parameter γ1 also affects Rv more than the other parameters, and the ε2 affects less while the effect of σ and α2 are negligible (Figure 5).
We also extend the analysis to the global sensitivity by using Latin Hypercube Sampling (LHS) [71], taking 1000 sample points from the global parameter space. We compute the partial rank correlation coefficients to identify the most influential parameters. We observed that in the global parameter space, the parameters ε1,α1, and β are the most strongly effective to Rv, followed by α2,ε2,γ1, and σ, while γ2 is less effective (Figure 5).
In this section, we use Latin Hypercube Sampling [71] from the global parameter space to identify the sensitivity level of the peak value of the infected class and that of the time at the peak of the epidemic. The computed partial rank correlation coefficient corresponding to each parameter is presented in Figure 6. Our analysis shows that the peak value of the infected class is highly correlated to β (positive correlation). The peak value is moderately affected by α1,α2, γ1, and σ and is weakly correlated to the parameters γ2, ε2, and ε1 (Figure 6). Similarly, the peak time of the epidemic is mostly affected by the parameters β and ε1, while it is less influenced by the parameters α1 and γ1. The parameters σ,γ2,α2, and ε2 have the least effect on the peak time.
We use five parameters, the un-monitored vaccination rate (α1), the monitored vaccination rate (α2), the immunization rate of un-monitored vaccination (γ1), the effectiveness of un-monitored vaccination (ε1), and the effectiveness of monitored vaccination (ε2) to evaluate the effects of the vaccination programs.
Note that Rv<1 can be associated with a condition required to eradicate the disease (Section 4.3). We now use our Rv formulation to identify vaccine-related parameters that can reduce Rv below one (Figure 7). We found that at the current level of α1, either the monitored vaccination rate α2 needs to rise by 250% (α2≥.07) or the effectiveness of un-monitored vaccination ε1 needs to rise by 4% (ε1≥0.52) to make Rv<1. On the other hand, with the current effectiveness of monitored vaccination (ε2), the effectiveness of un-monitored vaccination (ε1) needs to be greater than 0.52 to make Rv<1 (Figure 7).
If the un-monitored vaccination rate (α1) is increased by 20% (α1≈0.42per year), the activity of the monitored vaccination program can be somewhat relaxed at the current level of α2≈0.02per year for Rv<1. On the other hand, if α1 is decreased by 26% (α1≈0.23 per year), the activity of the monitored vaccination program needs to be raised, making the level of α2 exceed more than 0.1 per year to achieve Rv<1.
In the absence of monitored vaccination, the model predicts that the cases will rise and reach the peak value of 85,750 in 2038 for α2=0. With the reduction of un-monitored vaccination by 50% or α1=0.15, our model predicts that the cases will rise and reach the peak value of 647,300 in 2028 (Figure 8). If the immunity rate of un-monitored vaccination is decreased by 50%, i.e., γ1=0.05, the cases rise, leading to the peak value of about 452,800 in 2030. Similarly, if the effectiveness of un-monitored vaccination is decreased by 20% (ε1=0.4), the cases may rise and reach the peak value of 268,200 in 2027.
We explore how the different levels of vaccination-related parameters, α1,α2,γ1,γ2,ε1, and ε2, (Figure 9) affect the level of steady-state of new infections (Figure 9). As expected, an increase in the vaccination rate decreases the steady-state level of new infections. Based on our model prediction, the current level of vaccination results in 7,312 new cases at a steady state. To sufficiently reduce the steady-state level of new infections, the rate of un-monitored vaccination should be increased by 6.5% (α1=0.33), or the rate of monitored vaccination should be increased by 50% (α2=0.03). Similarly, the immunizing rate of un-monitored vaccine needs to be increased by 100% (γ1=0.2), or its effectiveness needs to be increased by 20% (ε1=0.6) to bring the steady-state level of new infections to a sufficiently low level.
Furthermore, the reduction of 6.5% of the un-monitored vaccination rate (α1) increases the steady-state new cases by 206% (22.35 thousand), showing the high impact of the vaccination rate. Also, lowering the monitored vaccination rate (α2) by 50% increases the steady-state level of new cases by approximately 250% (25.56 thousand). Similarly, decreasing the immunity rate of un-monitored vaccination (γ1) by 10% increases the steady-state new cases by about 300% (29.1 thousand) while reducing the effectiveness of monitored vaccination (ε2) by 18% increases the steady-state new cases by 30% (9.528 thousand).
In recent years, the frequent outbreaks of measles in developing and developed countries have become a significant obstacle to achieving the goal of elimination. Mainly, unvaccinated children are the victims of the disease [6,7,26]. Despite the Supplementary Immunization Activities (SIAs), most of the population is not vaccinated in some districts of Nepal, including Rautahat, Kapilvastu, Morang, and Bajura. Furthermore, many of those initiating the vaccination does not complete the vaccine doses correctly, making them not completely protected. Because of the resurgence of measles in Nepal since 2017 [20,21], WHO's deadline for the elimination goal has been extended to 2023 [53]. We developed a novel deterministic model validated by the data from Nepal to evaluate the monitored vaccination programs.
We thoroughly analyzed our model to formulate the vaccinated reproduction number (Rv>1), the stability analysis, and the disease persistence theory. Using the model and the available data from the official websites of WHO, we estimated key parameters related to the un-monitored vaccination. Moreover, we performed a global sensitivity analysis using Latin Hypercube Sampling from the wider parameter space. Our model predicts that the measles elimination goal can be achieved if the monitored vaccination rate is increased by 50% (α2=0.03) or the un-monitored vaccination rate is increased by 6.5% (α1=0.33). The elimination goal can also be achieved by the high effectiveness of the un-monitored vaccine (i.e., with ε1≥0.53). However, if the current trend continues, our model predicts that the measles will persist causing an obstacle to the measles elimination goal of Nepal. Furthermore, our model predicts that without any additional interventions, measles transmission will continue with a rise in the epidemic after 2033 (Figure 4). Our model also predicts that the major contributor to the measles resurgence in Nepal is susceptible (unvaccinated) and unmonitored vaccinated groups, emphasizing the need for the expedition of monitored-vaccination programs. The epidemic dynamics in Nepal is quite slow, reaching the peak only in about 2097 (Figure 4). The observed slow dynamics is consistent with the previous study [72].
We acknowledge some limitations of our study. The parameter estimations are based on the limited data of yearly incidence cases (2000–2019). Moreover, measles is a short-term disease recovered within a month, so the daily or weekly more detailed and accurate data can help improve the prediction of our model. We could obtain the closed form of the unique endemic equilibrium but are unable to perform a detailed analysis of the endemic equilibrium. Instead, we established the disease persistence criteria. The homogeneous mixing assumed among the children of Nepal can be improved by the model with an appropriate network of contact among children.
In summary, we develop a model of measles transmission in the context of Nepal, where monitoring is critical for the successful implementation of the vaccination program. Our thorough analysis and the detailed numerical simulations of the model can provide helpful information for policymakers to design ideal monitored-vaccination programs to achieve the elimination goal of measles from Nepal.
This research was supported by the GRAID (Graduate Research Assistantships in Developing Countries) awards from the International Mathematical Union (IMU). AP acknowledges the Nepal Mathematical Society (NMS) for the NMS Ph.D. Fellowship Award 2020. KA acknowledges the Nepal Academy of Science and Technology (NAST) for Ph.D. Fellowship and the Nepal Mathematical Society (NMS) for the NMS Ph.D. Fellowship award 2020. RG acknowledges the University Grants Commission (UGC) for Ph.D. Fellowship and Nepal Mathematical Society (NMS) for the NMS Ph.D. Fellowship Award 2020. The work of NKV was supported by NSF grants DMS-1951793 and DEB-2030479 from the National Science Foundation of the USA and the UGP award from San Diego State University.
The authors declare there is no conflict of interest.
[1] | J. Hamborsky, A. Kroger, S. Wolfe, Epidemiology and prevention of vaccine-preventable diseases, 13th ed. Washington D.C. Public Health Foundation, 2015. |
[2] |
E. Stephen, K. Raymond, K. Gabriel, F. Nestory, M. Godfrey, M. Arbogast, A mathematical model for control and elimination of the transmission dynamics of measles, Appl. Comput. Math., 4 (2015), 396–408. https://doi.org/10.11648/j.acm.20150406.12 doi: 10.11648/j.acm.20150406.12
![]() |
[3] | Center for Disease Control and Prevention (CDC), Measles epidemic: Overcoming vaccine prejudice (History of measles outbreaks), A Train Education, available from: https://www.atrainceu.com/content/2-history-and-pathology-measles (accessed on 8 February 2021). |
[4] | World Health Organization, Measles, available from: https://www.who.int/news-room/fact-sheets/detail/measles, (accessed on 10 March 2021). |
[5] | UNICEF, UNICEF for every child, Over 20 million children worldwide missed out on measles vaccine annually in past 8 years, creating a pathway to current global outbreaks, available from: https://www.unicef.org/nepal/press-releases/over-20-million-children-worldwide-missed-out-measles-vaccine-annually-\past-8-years (accessed on 28 March 2020). |
[6] | United Nations: UN News, Measles cases hit 23-year high last year, killing 200,000 as vaccination stalls, WHO says, available from: https://news.un.org/en/story/2020/11/1077482 (accessed on 10 March 2020). |
[7] |
M. K. Patel, J. L. Goodson, J. P. Alexander Jr, K. Kretsinger, S. V. Sodha, C. Steulet, et al., Progress toward regional measles elimination– worldwide, 2000–2019, Morb. Mortal. Wkly. Rep., 69 (2020), 1700. https://doi.org/10.15585/mmwr.mm6848a1 doi: 10.15585/mmwr.mm6848a1
![]() |
[8] | Government of Nepal, Ministry of Health and Population, National immunization programme, available from: https://www.mohp.gov.np/eng/program/child-health-services/nip (accessed on 28 March 2021). |
[9] | L. H. Sun, B. Guarino, Anti-vaxxers target communities battling measles, available from: https://www.washingtonpost.com/national/health-science/antivaxxers-go-viral-in-communities-battling-measles/2019/05/20/a476417c-78d7-11e9-bd25-c989555e7766_story.html(accessed: 20 April 2020). |
[10] | B. Zadrozny, E. Edwards, Anti-vaccine groups take dangerous online harassment into the real world, available from: https://www.nbcnews.com/health/kids-health/anti-vaccine-groups-take-dangerous-harassment-offline-real-world-n1096461 (accessed on 20 April 2020). |
[11] | New York City (NYC) Health, Measles, available from: https://www1.nyc.gov/site/doh/health/health-topics/measles.page (accessed on 10 August 2020). |
[12] | B. Guarino, L. H. Sun, New York anti-vaccine event attracts pro-vaccine protests amid measles outbreak, available from: https://www.washingtonpost.com/health/2019/06/05/brooklyn-anti-vaccine-event-attracts-pro-vaccine-protests-amid-measles\-outbreak/, (accessed on 20 April 2020). |
[13] | Public Health Update, National immunization schedule, Nepal (Updated), available from: https://publichealthupdate.com/national-immunization-schedule-nepal/ (accessed on 10 March 2020). |
[14] |
S. Khanal, T. R. Sedai, G. R. Choudary, J. N. Giri, R. Bohara, R. Pant, et al., Progress toward measles elimination — Nepal, 2007–2014, Morb. Mortal. Wkly. Rep., 65 (2016), 206–210. https://doi.org/10.15585/mmwr.mm6508a3 doi: 10.15585/mmwr.mm6508a3
![]() |
[15] | B. K. Suvedi, Twenty-five years of immunization program in Nepal, Kathmandu Univ. Med. J., 3 (2005), 4. |
[16] | Government of Nepal, Ministry of Health and Population Child Health Division, Department of Health Services (DoHS), National immunization programme, available from: http://www.chd.gov.np (accessed on 10 March 2020). |
[17] | Child Health Division, DoHS, MoHP, National immunization program, reaching every child, comprehensive multi-year plan 2068–2072 (2011–2016), MoHP, Nepal (2011). |
[18] | A. Poudel, Routin immunization: How to Measles Transmission, (2014), available from: http://arjunmaske.blogspot.com/2014/09/how-to-measlestransmission.html (accessed on 12 August 2020). |
[19] | A. Poudel, Measles outbreak in Morang, Dang and Kapilvastu raises concern, available from: https://kathmandupost.com/national/2019/04/30/measles-outbreak-in-morang-dang-and-kapilvastu-raises-concern (accessed on 12 September 2020). |
[20] | A. Poudel, Low vaccine coverage rate, floating population leading to repeat measles outbreaks, available from: https://reliefweb.int/report/nepal/low-vaccine-coverage-rate-floating-population-leading-repeat-measles-outbreaks (accessed on 12 September 2020). |
[21] | New Spotlight Online, Number Of Measles Cases In Nepal Increased by more than two times in 2018, available from: https://www.spotlightnepal.com/2019/04/25/number-measles-cases-nepal-increased/ (accessed on 25 March 2020). |
[22] | A. Poudel, Measles outbreaks reported in five districts including in Kathmandu and Lalitpur in last one month, available from: https://kathmandupost.com/national/2020/04/29/measles-outbreaks-reported-in-five-districts-including-in-kathmandu-and-lalitpur\-in-last-one-month, (accessed on 5 March 2021). |
[23] | S. Uprety, Meanwhile, a measles outbreak in Nepal, available from: https://www.nepalitimes.com/here-now/meanwhile-a-measles-outbreak-in-nepal/ (accessed on 28 March 2021). |
[24] | K. B. Karki, M. Dhimal, A. R. Pandey, B. Bista, A. Pandey, B. R. Giri, et al., Measles outbreak in Kapilvastu, Nepal: an outbreak investigation, Nepal Health Research Council, Ramshah Path, Kathmandu, Nepal, 2016. |
[25] | M. Poudel, Unidentified disease claims lives of six kids, available from: https://kathmandupost.com/national/2016/01/20/unidentified-disease-claims-lives-of-six-kids (accessed on 10 September 2020). |
[26] |
S. Sitaula, G. R. Awasthi, J. B. Thapa, K. P Joshi, A. Ramaiya, Measles outbreak among unvaccinated children in Bajura, JNMA J. Nepal Med. Assoc., 50 (2010), 273–276. https://doi.org/10.31729/jnma.48 doi: 10.31729/jnma.48
![]() |
[27] | H. Trottier, P. Philippe, Deterministic modeling of infectious diseases: measles cycles and the role of births and vaccination, Internet J. Infect. Dis., 2 (2002), 8 page. |
[28] | H. Trottier, P. Philippe, Deterministic modeling of infectious diseases: theory and methods, Internet J. Infect. Dis., 1 (2000), 3. |
[29] |
S. N. Mitku, Mathematical modeling and simulation study for the control and transmission dynamics of measles, Am. J. Appl. Math., 5 (2017), 99–107. https://doi.org/10.11648/j.ajam.20170504.11 doi: 10.11648/j.ajam.20170504.11
![]() |
[30] |
M. G. Robert, M. I. Tobias, Predicting and preventing measles epidemics in New Zealand, Epidemiol. Infect., 124 (2000), 279–287. https://doi.org/10.11648/j.ajam.20170504.11 doi: 10.11648/j.ajam.20170504.11
![]() |
[31] |
A. Franceschetti, A. Pugliese, Threshold behaviour of a SIR epidemic model with age structure and immigration, J. Math. Biol., 57 (2008), 1–27. https://doi.org/10.1007/s00285-007-0143-1 doi: 10.1007/s00285-007-0143-1
![]() |
[32] | J. F. Christopher, S. Katriona, C. Spencer, C. M. Jose, G. Christopher, G. James, et al., Measles outbreak response decision-making under uncertainty: a retrospective analysis, J. R. Soc. Interface, 15 (2017), 20170575. http://doi.org/10.1098/rsif.2017.0575R |
[33] |
A. A. Momoh, M. O. Ibrahim, I. J. Uwanta, S. B. Manga, Mathematical model for control of measles epidemiology, Inter. J. Pure Appl. Math., 67 (2013), 707–718. https://doi.org/10.12732/ijpam.v87i5.4 doi: 10.12732/ijpam.v87i5.4
![]() |
[34] | M. D. la Sens, A. Quesadas, A. Ibeas, R. Nistal, An observed-based vaccination law for a SEIR epidemic model, Int. J. Comput. Theory Eng., 4 (2012), 1–32. http://doi.org/10.7763/IJCTE.2012.V4.488 |
[35] |
E. F. Doungmo Goufo, S. C. Oukouomi Noutchie, S. Mugisha, A fractional SEIR epidemic model for spatial and temporal spread of measles in metapopulations, Abstr. Appl. Anal., 2014 (2014), 6. https://doi.org/10.1155/2014/781028 doi: 10.1155/2014/781028
![]() |
[36] |
J. Ochoche, R. Gweryina, A mathematical model of measles with vaccination and two phases of infectiousness, J. Math., 10 (2019), 95–105. https://doi.org/10.9790/5728-101495105 doi: 10.9790/5728-101495105
![]() |
[37] |
E. M, Musyoki, R. Ndung'u, S. Osman, A mathematical model for the transmission of measles with passive immunity, Int. J. Res. Math. Stat. Sci., 6 (2019), 1–8. https://doi.org/10.26438/ijsrmss/v6i2.18 doi: 10.26438/ijsrmss/v6i2.18
![]() |
[38] |
M. Fred, J. Sigey, J. A. Okello, J. Okwoyo, G. J. Kang'ethe, Mathematical modeling on the control of measles by vaccination: case study of KISⅡ County Kenya, SIJ Trans. Comput. Sci. Eng. Appl. (CSEA), 2 (2015), 38–46. https://doi.org/10.9756/SIJCSEA/V2I4/0203150101 doi: 10.9756/SIJCSEA/V2I4/0203150101
![]() |
[39] | O. Christopher, A. I. Ibrahim, A. S. Timothy, Mathematical model of the dynamics of measles under the combined effect of vaccination and measles therapy, Int. J. Sci. Technol., 6 (2017), 118–173. |
[40] | M. J. Wanjau, R. Titus, C. Isaac, Mathematical modeling of the transmission dynamics of measles under the effect of vaccination, IOSR J. Math., 15 (2019), 10–19. |
[41] | R. Y. M'pika Massoukou, S. C. Oukouomi Noutchie, R. Guiem, Global dynamics of an SVEIR model with age-dependent vaccination, infection, and latency, Abstr. Appl. Anal., 2018 (2018), 1–21. https://doi.org/10.1155/2018/8479638 |
[42] | T. Bakhtiar, Control policy mix in measles transmission dynamics using vaccination, therapy, and treatment, Int. J. Math. Math. Sci., 2020 (2020), 1–20. |
[43] |
Z. Memon, S. Qureshi, B. R. Memon, Mathematical analysis for a new nonlinear measles epidemiological system using real incidence data from Pakistan, Eur. Phys. J. Plus, 135 (2020), 1–21. https://doi.org/10.1140/epjp/s13360-020-00392-x doi: 10.1140/epjp/s13360-020-00392-x
![]() |
[44] |
D. Aldila, D. Asrianti, A deterministic model of measles with imperfect vaccination and quarantine intervention, Int. Conf. Mathematics: Pure, Appl. Computat., 1218 (2019), 012044–012054. https://doi.org/10.1088/1742-6596/1218/1/012044 doi: 10.1088/1742-6596/1218/1/012044
![]() |
[45] | Centers for Disease Control and Prevention (CDC), Progress in measles control–Nepal, 2000-2006, MMWR Morb. Mortal Wkly Rep., 56 (2007), 1028–1031. |
[46] |
A. T. Truong, M. N. Mulders, D. C. Gautam, W. Ammerlaan, R. L. de Swart, C. C. King, et al., Genetic analysis of Asian measles virus strains–new endemic genotype in Nepal, Virus Res., 76 (2001), 71–78. https://doi.org/10.1016/S0168-1702(01)00255-6 doi: 10.1016/S0168-1702(01)00255-6
![]() |
[47] |
A. B. Joshi, Measles deaths in Nepal: estimating the national cases in fatality ratio, Bull World Health Organ, 87 (2009), 405–484. https://doi.org/10.2471/BLT.07.050427 doi: 10.2471/BLT.07.050427
![]() |
[48] | World Health Organization, WHO vaccine-preventable diseases: monitoring system. 2020 global summary, 2019, available from: https://apps.who.int/immunization_monitoring/globalsummary (accessed on 28 march 2020). |
[49] |
B. Pantha, S. Giri, H. R. Joshi, N. K.Vaidya, Modeling transmission dynamics of rabies in Nepal, Infect. Dis. Model., 6 (2021), 284–301. https://doi.org/10.1016/j.idm.2020.12.009 doi: 10.1016/j.idm.2020.12.009
![]() |
[50] | Macrotrends, Nepal Population 1950–2020, available from: https://www.macrotrends.net/countries/NPL/nepal/population (accessed on 2 October 2020). |
[51] | World Health Organization, Nepal - Deaths due to measles among children aged < 5 years, available from: https://www.who.int/immunization/monitoring_surveillance/burden/vpd/WHO_SurveillanceVaccinePreventable_11_Measles_R1.pdf?ua=1 (accessed on 2 October 2020). |
[52] | World Data Atlas, Nepal-Deaths due to measles among children aged < 5 years, available from: https://knoema.com/atlas/Nepal/topics/Health/Deaths-among-children-under-5-by-cause/Deaths-due-to-measles (accessed on 3 October 2020). |
[53] | H. S. Rathore, Measles outbreak kills two children and infects over 150 in Chepang settlements of Dhading district, available from: https://kathmandupost.com/province-no-3/2020/04/06/measles-outbreak-kills-two-children-and-infects-over-150-in-chepang-\settlements-of-dhading-district (accessed on 3 October 2020). |
[54] | H. Motulasky, A. Christopoulos, Fitting Model to Biological Data Using Linear and Non Linear Regression. A Practical Guide to Curve Fitting, Graph Pad Software Inc.San Diego, CA, (2003), www.graphpad.com https://doi.org/10.5206/mase/10847. |
[55] | M. Rahman, K. Bekele-Maxwell, L. L. Cates, H. T. Banks, N. K. Vaidya, Modeling zika virus transmission dynamics: parameter estimates, disease characteristics, and prevention, Sci. Rep., 9 (2019), 10575. https://doi.org/10.1038/s41598-019-46218-4 |
[56] | H. T. Banks, S. Hu, W. C. Tompson, Modeling and inverse problems in the presence of uncertainty, CRC Press, Taylor and Francis Group, A Chapman and Hall Book, 2014. |
[57] |
H. T. Banks, M. L. Joyner, Information content in data sets: a review of methods for interrogation and model comparison, J. Inverse Ill-Posed Probl., 26 (2018), 423–452. https://doi.org/10.1515/jiip-2017-0096 doi: 10.1515/jiip-2017-0096
![]() |
[58] |
K. Adhikari, R. Gautam, A. Pokharel, K. N. Uprety, N. K. Vaidya, Transmission dynamics of COVID-19 in Nepal: Mathematical model uncovering effective control, J. Theor. Biol., 521 (2021), 110680. https://doi.org/10.1016/j.jtbi.2021.110680 doi: 10.1016/j.jtbi.2021.110680
![]() |
[59] | C. D. Schunn, D. P. Wallach, Evaluating goodness-of-fit in comparison of models to data, Psychologie der Kognition: Reden and Vorträge anlässlich der Emeritierung von Werner Tack, (2005), 115–135. |
[60] |
H. Miao, X. Xia, A. S. Perelson, H. Wu, On identifiability of nonlinear ODE models and applications in viral dynamics, SIAM Rev., 53 (2011), 3–39. https://doi.org/10.1137/090757009 doi: 10.1137/090757009
![]() |
[61] | O. Diekmann, J. A. P. Heesterbeek, Mathematical Epidemiology of Infectious Diseases: Model Building, Analysis and Interpretation, Wiley, New York, 1999. |
[62] |
P. van den Driessche, J. Watmough, Reproduction numbers and sub-threshold endemic equilibria for compartmental models of disease transmission, Math. Biosci., 180 (2002), 29–48. https://doi.org/10.1016/S0025-5564(02)00108-6 doi: 10.1016/S0025-5564(02)00108-6
![]() |
[63] | G. Chowell, J. M. Hyman, Mathematical and statistical modeling for emerging and re-emerging infectious diseases, Springer International Publishing Switzerland, 2016. |
[64] |
O. Diekmann, J. A. P. Heesterbeek, M. G. Roberts, The construction of next-generation matrices for compartmental epidemic models, J. R. Soc. Interface, 7 (2010), 873–885. https://doi.org/10.1098/rsif.2009.0386 doi: 10.1098/rsif.2009.0386
![]() |
[65] |
M. A. Kuddus, M. Mohiuddin, A. Rahman, Mathematical analysis of a measles transmission dynamics model in Bangladesh with double dose vaccination, Sci. Rep., 11 (2021), 1–16. https://doi.org/10.1038/s41598-021-95913-8 doi: 10.1038/s41598-021-95913-8
![]() |
[66] | H. L. Smith, P. Waltman, The theory of the chemostat, Cambridge University Press, 1995. |
[67] |
J. M. Mutua, F. Bin Wang, N. K. Vaidya, Modeling malaria and typhoid fever co-infection dynamics, Math. Biosci., 264 (2015), 128–144. https://doi.org/10.1016/j.mbs.2015.03.014 doi: 10.1016/j.mbs.2015.03.014
![]() |
[68] | T. Kato, Perturbation theory for linear operators, Springer-Verlag, Berlin Heidelberg, 1976. |
[69] | X. Q. Zhao, Dynamical systems in population biology, Springer International Publishing, 2017. |
[70] | Centers for Disease Control and Prevention, Measles symptoms and complications, available from: https://www.cdc.gov/measles/symptoms/index.html (accessed on 10 September 2020). |
[71] |
S. Marino, I. B. Hogue, C. J. Ray, D. E. Kirschner, A methodology for performing global uncertainty and sensitivity analysis in systems biology, J. Theor. Biol., 254 (2008), 178–196. https://doi.org/10.1016/j.jtbi.2008.04.011 doi: 10.1016/j.jtbi.2008.04.011
![]() |
[72] |
J. Horrocks, C. T. Bauc, Algorithmic discovery of dynamic models from infectious disease data, Sci. Rep., 10 (2020), 7061. https://doi.org/10.1038/s41598-020-63877-w doi: 10.1038/s41598-020-63877-w
![]() |
1. | Haileyesus Tessema Alemneh, Asnakew Mesele Belay, Fahad Al Basir, Modelling, Analysis, and Simulation of Measles Disease Transmission Dynamics, 2023, 2023, 1607-887X, 1, 10.1155/2023/9353540 | |
2. | Olumuyiwa James Peter, Sania Qureshi, Mayowa M. Ojo, Ratchada Viriyapong, Amanullah Soomro, Mathematical dynamics of measles transmission with real data from Pakistan, 2022, 2363-6203, 10.1007/s40808-022-01564-7 | |
3. | Anjana Pokharel, Khagendra Adhikari, Ramesh Gautam, Kedar Nath Uprety, Naveen K. Vaidya, Modeling measles transmission in adults and children: Implications to vaccination for eradication, 2024, 9, 24680427, 1007, 10.1016/j.idm.2024.04.012 | |
4. | W. Ahmad, A. I. K. Butt, M. Rafiq, Z. Asif, T. Ismaeel, N. Ahmad, Modeling, analyzing and simulating the Measles transmission dynamics through efficient computational optimal control technique, 2024, 139, 2190-5444, 10.1140/epjp/s13360-024-05368-9 | |
5. | Muhammad Bilal Riaz, Nauman Raza, Jan Martinovic, Abu Bakar, Harun Kurkcu, Osman Tunç, Fractional dynamics and sensitivity analysis of measles epidemic model through vaccination, 2024, 31, 2576-5299, 265, 10.1080/25765299.2024.2345424 | |
6. | Ramesh Gautam, Khagendra Adhikari, Anjana Pokharel, Kedar Nath Uprety, Naveen K. Vaidya, Role of cross-border mobility on the backward bifurcation of malaria transmission model: Implications for malaria control in Nepal, 2025, 81, 14681218, 104173, 10.1016/j.nonrwa.2024.104173 |
Parameters (yr)−1 | Description | Baseline Value | Confidence Interval | Sources |
α2 | Monitored | 0.02 | Fixed | Assumed |
vaccination rate | ||||
α1 | Un-monitored | 0.31 | [0.0902 0.5298] | Data Fitting |
vaccination rate | ||||
β | Transmission rate | 63.02 | [63.01 63.03] | Data Fitting |
ε1 | Effectiveness of | 0.51 (Dim.less) | [0.49 0.52] | Data Fitting |
un-monitored vacc. | ||||
ε2 | Effectiveness of | 0.9 (Dim.less) | Fixed | Assumed |
monitored vacc. | ||||
μ | Removed rate | 0.0667 | Fixed | Assumed |
γ1 | UV immunity rate | 0.1 | [0.086 0.12] | Data Fitting |
γ2 | MV immuninity rate | 0.5 | Fixed | Assumed |
σ | Disease progress rate | 30 | Fixed | [4] |
η | Recovery rate | 20 | Fixed | [4] |
δ | Disease-induced | 0.01 | Fixed | [52] |
death rate |
Parameters (yr)−1 | Description | Baseline Value | Confidence Interval | Sources |
α2 | Monitored | 0.02 | Fixed | Assumed |
vaccination rate | ||||
α1 | Un-monitored | 0.31 | [0.0902 0.5298] | Data Fitting |
vaccination rate | ||||
β | Transmission rate | 63.02 | [63.01 63.03] | Data Fitting |
ε1 | Effectiveness of | 0.51 (Dim.less) | [0.49 0.52] | Data Fitting |
un-monitored vacc. | ||||
ε2 | Effectiveness of | 0.9 (Dim.less) | Fixed | Assumed |
monitored vacc. | ||||
μ | Removed rate | 0.0667 | Fixed | Assumed |
γ1 | UV immunity rate | 0.1 | [0.086 0.12] | Data Fitting |
γ2 | MV immuninity rate | 0.5 | Fixed | Assumed |
σ | Disease progress rate | 30 | Fixed | [4] |
η | Recovery rate | 20 | Fixed | [4] |
δ | Disease-induced | 0.01 | Fixed | [52] |
death rate |