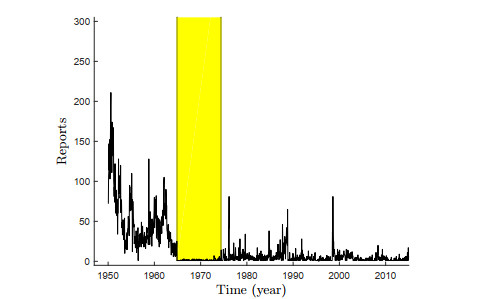
Citation: N. Akhavan Kharazian, F. M. G. Magpantay. The honeymoon period after mass vaccination[J]. Mathematical Biosciences and Engineering, 2021, 18(1): 354-372. doi: 10.3934/mbe.2021019
[1] | Ankai Liu, Felicia Maria G. Magpantay, Kenzu Abdella . A framework for long-lasting, slowly varying transient dynamics. Mathematical Biosciences and Engineering, 2023, 20(7): 12130-12153. doi: 10.3934/mbe.2023540 |
[2] | Jonathan E. Forde, Bailey Meeker . A model of varicella-zoster reactivation. Mathematical Biosciences and Engineering, 2010, 7(4): 765-777. doi: 10.3934/mbe.2010.7.765 |
[3] | Bruce Pell, Matthew D. Johnston, Patrick Nelson . A data-validated temporary immunity model of COVID-19 spread in Michigan. Mathematical Biosciences and Engineering, 2022, 19(10): 10122-10142. doi: 10.3934/mbe.2022474 |
[4] | Anthony Morciglio, R. K. P. Zia, James M. Hyman, Yi Jiang . Understanding the oscillations of an epidemic due to vaccine hesitancy. Mathematical Biosciences and Engineering, 2024, 21(8): 6829-6846. doi: 10.3934/mbe.2024299 |
[5] | Siyu Liu, Mingwang Shen, Yingjie Bi . Global asymptotic behavior for mixed vaccination strategy in a delayed epidemic model with interim-immune. Mathematical Biosciences and Engineering, 2020, 17(4): 3601-3617. doi: 10.3934/mbe.2020203 |
[6] | Kai Zhang, Xinwei Wang, Hua Liu, Yunpeng Ji, Qiuwei Pan, Yumei Wei, Ming Ma . Mathematical analysis of a human papillomavirus transmission model with vaccination and screening. Mathematical Biosciences and Engineering, 2020, 17(5): 5449-5476. doi: 10.3934/mbe.2020294 |
[7] | Islam A. Moneim, David Greenhalgh . Use Of A Periodic Vaccination Strategy To Control The Spread Of Epidemics With Seasonally Varying Contact Rate. Mathematical Biosciences and Engineering, 2005, 2(3): 591-611. doi: 10.3934/mbe.2005.2.591 |
[8] | Eunha Shim . A note on epidemic models with infective immigrants and vaccination. Mathematical Biosciences and Engineering, 2006, 3(3): 557-566. doi: 10.3934/mbe.2006.3.557 |
[9] | Glenn Ledder . Incorporating mass vaccination into compartment models for infectious diseases. Mathematical Biosciences and Engineering, 2022, 19(9): 9457-9480. doi: 10.3934/mbe.2022440 |
[10] | Roy Curtiss III . The impact of vaccines and vaccinations: Challenges and opportunities for modelers. Mathematical Biosciences and Engineering, 2011, 8(1): 77-93. doi: 10.3934/mbe.2011.8.77 |
Vaccines are biological preparations that induce a host's immunity against a disease without causing the actual illness. Their importance has been highlighted recently by the demand for the rapid development of a vaccine for COVID-19. Models help us determine how vaccination programs can change disease dynamics, depending on many factors, including the effectiveness of the vaccine and how it is distributed. Here we consider how different types of individual-level protection afforded by a vaccine can result in different population-level dynamics of a disease, with a focus on "honeymoon periods".
McLean and Anderson [2] introduced the term honeymoon period to denote the "long period of low incidence that immediately follows the initiation of a mass vaccination program". There are many papers that note the occurrence of honeymoon periods in both real data and epidemiological model trajectories. For example, Hefferman and Keeling [3] showed that a waning vaccine model with moderate waning times (between 40 to 80 years) and high vaccine coverage (greater than 70%) can lead to considerable oscillations in the infected class, with a long low incidence period preceding possibly large-scale epidemics. Mossong and Muller [4] examined the time to re-emergence of measles in different situations using age-structured models. In Figure 1 we present an example of a possible honeymoon period in pertussis data from Massachusetts.
Most research on mathematical modeling of infectious diseases focuses on the equilibrium dynamics of epidemiological systems. However, transient dynamics can also have some very unique and informative characteristics, complementing the steady state analysis of systems. The honeymoon period is a transient feature that can be an important consideration for public health agencies.
In this paper, we conduct a study of honeymoon periods arising from a simple model of an imperfect vaccine examined in [1]. This model allows for three different modes of vaccine failure: "leakiness", "all-or-nothingness" and "waning." We reviewed existing linearization methods for measuring transient dynamics in ecology, such as reactivity and amplification envelope [5], and conclude that these are not enough to determine the characteristics of honeymoon periods. We present proof of global asymptotic stability of the endemic equilibrium when the disease-free equilibrium is unstable and give a technical definition of the honeymoon period for these models. We also provide some bounds for the lengths of these periods and the size of the drop in the "effective susceptible" fraction of the population.
This paper is structured as follows: In Section 2 we review the imperfect vaccine model analyzed in [1] and review measures of transient dynamics defined by [5] that are based on linearization about the equilibrium. In Section 3 we demonstrate that the measures based on linearizaton are not helpful in distinguishing between models that display honeymoon periods and those that do not. We also define the effective susceptible class and present a proof of global stability of the endemic equilibrium, in order to give a technical definition of the honeymoon period. In Section 4 we prove some basic properties of the solution during the honeymoon period. Finally, in Section 5 we give a summary of the results and discuss future directions.
The imperfect vaccine model in Magpantay et al. [1] is an extension of the vaccinated-susceptible-infected-recovered (VSIR) model with unstructured population described by a system of ordinary differential equations (ODEs) similar to that presented in [6]. The three different modes of vaccine failure considered in the model are:
● Leakiness ("failure in degree")
The probability of getting infected upon exposure is decreased but not eliminated by vaccination.
● All-or-nothingness or primary vaccine failure ("failure in take")
Lifelong perfect protection is only provided to a fraction of the vaccinated population while the remaining fraction remains as susceptible as if they were never vaccinated.
● Waning ("failure in duration")
Perfect protection is provided to all vaccinees for an exponentially distributed period of time after which each vaccinee becomes as a susceptible as if they were never vaccinated.
It was shown that these different moves of protection have different epidemiological consequences [1]. Here we present a short description of the model: Let S(t), I(t) and R(t) be the fractions of susceptible, infected and recovered individuals respectively in the population at time t. Let V(t) be the proportion of individuals that has immunity from vaccination that has not waned yet. We assume a constant fraction p of newborns are vaccinated soon after birth but the vaccination fails in a fraction εA of the vaccinees. Thus (1−p)+εAp=1−(1−εA)p of newborns remain susceptible while (1−εA)p go to the vaccinated compartment. We assume that individuals in the vaccinated compartment can still become infected, although at a lower rate than susceptible individuals. This "leakiness" of the vaccine, denoted by εL, reflects the reduction in probability of infection upon exposure of individuals in the V class relative to those in the S class. Finally, we also assume that vaccine protection wanes at a rate of α. As shown in Table 1, we can re-parametrize from the waning rate α to a waning probability εW by setting εW=αα+μ. The full set of model equations is given by (2.1):
dVdt=(1−εA)pμ−εLβVI−αV−μV | (2.1a) |
dSdt=(1−(1−εA)p)μ−βSI+αV−μS | (2.1b) |
dIdt=βSI+εLβVI−γI−μI | (2.1c) |
dRdt=γI−μR | (2.1d) |
Symbol | Description | Default value in plots (if not specified) |
μ | Birth and death rate | 170 yr−1 |
γ | Recovery rate | 20 yr−1 |
β | Transmission rate | 150 yr−1 |
εL | Ratio of the probability a vaccinated individual will get infected after exposure relative to that of a susceptible individual | 0.2 |
εA | Probability of not getting protected after vaccination | 0.2 |
α | Waning rate of vaccine-derived immunity | μεW1−εW |
εW | Probability that the vaccine protection of individuals in the class V wanes within a lifetime, equal to εW=αα+μ | 0.2 |
p | Fraction of newborns vaccinated | 0.9 |
with the requirement (2.2) on the initial conditions at time t=0,
V(0)+S(0)+I(0)+R(0)=1. | (2.2) |
Here μ≥0, γ>0, β>0, p∈[0,1], and (εL,εA,εW)∈[0,1)3. The model is illustrated in Figure 2 and the parameters are described in Table 1. If (εL,εA,εW)=(0,0,0) then there is no way for a vaccinated individual to become infected so this special case is when we have a vaccine that induces perfect immunity.
To find the disease-free equilibrium, we set all the equations in (2.1) equal to zero with I=0. Solving this yields the disease-free equilibrium given by
x0=(V0,S0,I0,R0)=((1−εA)(1−εW)p,1−(1−εA)(1−εW)p,0,0) |
As in [1], we can show that the basic reproduction number with vaccination is given by
Rp=R0(1−(1−εL)(1−εA)(1−εW)p) | (2.3) |
where R0=βγ+μ is the basic reproduction number with no vaccination (p=0). The vaccine impact φ is defined to be
φ=1pR0−RpR0=(1−εL)(1−εA)(1−εW) | (2.4) |
The equation for the vaccine impact above shows that it can be thought of as the relative change in the reproduction number per unit of vaccination. This can be used to derive the critical vaccination coverage pc (the minimum fraction that needs to be vaccinated in order to drive the disease to extinction) given by
pc=1φ(1−1R0), |
if this quantity is less than one.
To obtain the endemic equilibrium of the system we again set the derivatives in (2.1) to zero but assume this time that the infected class is not zero. This yields
x∗=(V∗,S∗,I∗,R∗)=(V∗,1R0−εLV∗,μβ(R0(1−(1−εL)V∗)−1),γβ(R0(1−(1−εL)V∗)−1)). | (2.5) |
where
V∗={a−√a2−4R0εL(1−εW)φp2R0εL(1−εL)(1−εW), if εL≠0,(1−εA)(1−εW)p, if εL=0, | (2.6) |
and a=εL−(1−εW)(R0−1)+1 and R0=βγ+μ. In [1], it was shown that this equilibrium is in the positive cone and is locally asymptotically stable when Rp>1. We prove in the next section that the endemic equilibrium is globally stable when Rp>1.
It was also shown in [1] that for a fixed vaccine impact parameter φ such that Rp>1, the vaccine failure parameter set (εL,εA,εW) that leads to the highest value of the endemic steady state value of the infected class is (1−φ,0,0); that is the purely leaky model. Additionally, the endemic equilibrium of (εL,εA,εW)=(0,1−φ,0) is the same as that of (εL,εA,εW)=(0,0,1−φ). Thus, we cannot distinguish between these purely all-or-nothing and purely waning models with the same vaccine impact by just looking at the equilibrium values of the components. However, these models can be differentiated if we look at their transient dynamics, in particular what if we initialize the models at pre-vaccine era equilibrium (with p=0) then we increase the value of p (refer to Figure 3).
Suppose that we have an ordinary differential equation given by
x′=f(x) | (2.7) |
with an asymptotically stable equilibrium point at x=˜x. Let z=x−˜x be the deviation of the system from equilibrium and z0=z(0)=x(0)−˜x be the deviation at time zero. Let J=dfdx|x=˜x be the Jacobian of the system (2.7) evaluated at ˜x. The linearization of (2.7) about the equilibrium x=˜x is given by,
z′=Jz,z(0)=z0. | (2.8) |
Since all solutions of (2.1) are invariant in the positive cone with V(t)+S(t)+I(t)+R(t)=1 for t≥0 then R(t) is determined by the values of the three other states. Thus, when we look at the Jacobian of the system, we can just consider the matrix of partial derivatives of the first three equations in (2.1) with respect to the first three states. The general form of the Jacobian at ˜x is,
J(˜x)=[−(εLβ˜I+α+μ)0−εLβ˜Vα−(β˜I+μ)−β˜SεLβ˜Iβ˜Iβ˜S+εLβ˜V−(γ+μ)] | (2.9) |
To evaluate the Jacobian at the disease free equilibrium we set ˜x=x0=(V0,S0,I0,R0) and get,
J(x0)=[−(α+μ)0−εLβV0α−μ−βS000βS0+εLβV0−(γ+μ)]. |
To evaluate it at the endemic equilibrium we set ˜x=x∗=(V∗,S∗,I∗,R∗) and obtain,
J(x∗)=[−(εLβI∗+α+μ)0−εLβV∗α−(βI∗+μ)−βS∗εLβI∗βI∗0] |
We note that the element in the third row and the third column simplifies to zero after substituting in the values of V∗ and S∗ from (2.3).
Next, we review quantities that measure how sensitive a system is to deviations from equilibrium. Later on we will evaluate how these quantities depend on the parameter values of the imperfect vaccine model. We begin by discussing the reactivity and amplification envelope, two quantities defined by Neubert and Caswell [5] which have been used to measure transient dynamics when approaching a bifurcation point [7].
Definition 1 (Reactivity, from [5]).
reactivity=max|z0|≠01|z|d|z|dt|t=0 | (2.10) |
The reactivity of an asymptotically stable equilibrium is the maximum, over all perturbations, of the rate at which the trajectory departs from the equilibrium in the linearized system. It measures the maximum instantaneous amplification of perturbations of that equilibrium. Using |z|=√zTz in (2.10), we derive
d|z|dt=d√zTzdt=zT(dz/dt)+(dz/dt)Tz2|z|=zT(J+JT)z2|z| | (2.11) |
Let H(J)=(J+JT)/2. This is the Hermitian part of J and therefore its eigenvalues are all real-valued. The eigenvalues of H(J), which do not necessarily have the same sign as the eigenvalues of J, determine the instantaneous behavior of the linearized system (2.8). Let λH be the largest eigenvalue of H(J). Then we derive the following expression for reactivity in terms of J,
reactivity=max|z0|≠0zTH(J)z|z|2|t=0=max|z0|≠0z0TH(J)z0z0Tz0=λH. | (2.12) |
Definition 2 (Amplification envelope, from [5]).
amplification envelope=max|z0|≠0|z(t)||z0|. | (2.13) |
The amplification envelope is the maximum possible deviation from the equilibrium at any time following a perturbation. We note that the solutions z(t) of the linearized system (2.8) are given by z(t)=eJtz0, where eJt=∑∞n=0(Jt)nn!. Using this in (2.13) we derive,
amplification envelope=max|z0|≠0|z(t)||z0|=max|z0|≠0|eJtz0||z0|=|eJt|=σ(eJt) | (2.14) |
where σ(eJt) denotes the square root of the largest eigenvalue of (eJt)T(eJt). Note that the amplification envelope changes with time.
We used numerical methods to determine the size and extent of the honeymoon periods we obtain for the models evaluated at a range of different parameter values. MATLAB's ode45 was used for the numerical integration of the systems of differential equations.
In Figure 3, the dynamics of the infected class for the leaky, all-or-nothing (AON) and waning models with the same vaccine impact value are shown after the start of mass vaccination. We see clearly different transient dynamics between the three. All three initially start off at the pre-vaccine (p=0) endemic steady state and then vaccination coverage is set to p=0.9. The waning model displays a dip below the new equilibrium that lasts for about 50 years and then the solution displays large oscillations as it approaches its new equilibrium. In contrast, the all-or-nothing model undergoes smaller oscillations about its new equilibrium. As expected from the previous chapter, both the all-or-nothing and waning models approach the same new endemic equilibrium value despite very different transient dynamics before reaching it. The leaky model approaches a different new endemic equilibrium value which has a higher infected population at its new endemic equilibrium. It also approaches this equilibrium in a different manner, after dropping below it at first and then rebounding, taking many years of small oscillations before reaching values close to the new equilibrium value.
We see from Figure 3 that the initial reduction in incidence after the start of mass vaccination is often much larger than the long-term reduction in incidence (once the system reaches its new equilibrium). We also observe that the waning model exhibits a long, pronounced honeymoon period.
We derived the reactivity about both the endemic and disease-free equilibrium point of the three different vaccine models with the same vaccine impact. These were plotted in Figure 4(a)-(b) against the vaccine failure parameters. We see that there is only a small difference between the values of reactivity for the all-or-nothing and waning models despite Figure 3 showing a large difference in their transient dynamics. This indicates that reactivity is not a good measure for determining whether or not a model will display a honeymoon period. Interestingly, it also illustrates that the maximum possible instantaneous rate of amplification in the leaky model is less than the two other models.
We derived the amplification envelope of the three different vaccine models and plotted the results in Figure 4(c)-(d). Here we can observe again that there is not much of a difference between the results for the all-or-nothing and waning models, and that of the leaky model is also only slightly different from the other two. We find that the amplification envelope is also not a good indicator of the existence of significant honeymoon periods.
When observing honeymoon periods, we set the initial conditions of the models to be the pre-vaccine era equilibrium and consider how it approaches the new vaccine era equilibrium. Usually these two equilibria are significantly different from each other. This is why the reactivity and amplification envelope, which are based on linearization about equilibria and are more appropriate for measuring the behavior of small perturbations from such equilibria, may not be good indicators of which models will display substantial honeymoon periods.
It turns out that to analyze the honeymoon period, it will be important to estimate how the susceptibility of the population changes over time. Since individuals in the vaccinated compartment are still partially susceptible to the disease if εL>0, we can think of the "effective" susceptible number of individuals in a population to include both the susceptible individuals and a fraction εL of the vaccinated compartment.
Definition 3. We define the "effective susceptible class" SE(t) by
SE(t)=S(t)+εLV(t) |
This effective susceptible class is related to the effective reproduction number Reff(t) of system (2.1) via the relationship Reff(t)=R0(S(t)+εLV(t))=R0SE(t). In Figure 3, we see that the infected class does not change noticeably during the honeymoon period. This is usually the only compartment that is observed and recorded. However other important changes are occurring in the other components of the system during the honeymoon period, causing the resurgence of the disease later on. In Figure 5, we present the trajectory of the effective susceptible class that corresponds to the trajectory shown in Figure 3. We observe that the leaky and all-or-nothing models show very similar behavior whereas the waning model displays very different dynamics. We also note from the trajectories that the equilibrium value of the effective susceptible class is independent of the type of vaccine failure or vaccine coverage. This is proven in Proposition 4.
Proposition 4. Suppose that R0>1. For any p∈[0,1] such that Rp>1, the value of the effective susceptible class at the endemic equilibrium is given by
S∗E=S∗+εLV∗=1R0. | (3.1) |
Proof. If εL=0 then from (2.3), S∗E=S∗=1R0. If εL>0 then again from (2.3), S∗E=S∗+εLV∗=1R0−εLV∗+εLV∗=1R0.
Before we formally define different measures of the honeymoon period, we first prove the global stability (for all non-negative initial conditions) of the endemic equilibrium when Rp>1. Here we closely follow the work of Li et al. [8] using the LaSalle Invariance Principle.
Proposition 5 (Global asymptotic stability of endemic equilibrium). Let Rp>1. For any set of positive initial conditions such that V(0)+S(0)+I(0)+R(0)=1, the trajectory of the solution to (2.1) approaches the endemic equilibrium (V∗,S∗,I∗,R∗) given by (2.3)-(2.6).
Proof. If Rp>1 then (V∗,S∗,I∗,R∗) is in the positive cone [1]. Let
x=SS∗,y=VV∗,z=II∗. | (3.2) |
The system of Eqs (2.1) is equivalent to the system given by,
x′=x[(1−(1−εA)p)μS∗(1x−1)−βI∗(z−1)+αV∗S∗(yx−1)],y′=y[(1−εA)pμV∗(1y−1)−εLβI∗(z−1)],z′=βz[S∗(x−1)+εLV∗(y−1)]. | (3.3) |
The stability of the endemic equilibrium is maintained after transformation and is given by (x∗,y∗,z∗)=(1,1,1). Define the function L(x,y,z) by,
L(x,y,z)=a1S∗(x−1−lnx)+a2V∗(y−1−lny)+a3I∗(z−1−lnz), | (3.4) |
where the positive numbers a1,a2, and a3 will be found later. Differentiating L with respect to t along solutions of (3.3) yields,
F(x,y,z)=dLdt=a1S∗(x′−x′x)+a2V∗(y′−y′y)+a3I∗(z′−z′z),=a1S∗(x−1)[(1−(1−εA)p)μS∗(1x−1)−βI∗(z−1)+αV∗S∗(yx−1)]+a2V∗(y−1)[(1−εA)pμV∗(1y−1)−εLβI∗(z−1)]+a3I∗β(z−1)[S∗(x−1)+εLV∗(y−1)]. | (3.5) |
Following Li et al. [8], we determine the constants a1,a2, and a3 and rearrange F(x,y,z) in four steps:
Step 1. We construct Table 2 with the terms x, 1x, y, 1y, yx, z, xz and yz (which all appear in F(x,y,z)) as the column names. Then, we identify groups of terms that multiply to one and indicate each group by a row with a check mark on the relevant terms. We also write pk:=bk(nk−hk,1−hk,2−⋯−hk,nk) in the last column, where hk,i are the terms that multiply to 1 (i.e., ∏nki=1hk,i=1), ni is the number of terms and bk is a non-negative number to be found later.
x | 1x | y | 1y | yx | z | xz | yz | term |
✓ | ✓ | p1:=b1(2−x−1x) | ||||||
✓ | ✓ | ✓ | p2:=b2(3−x−1y−yx) | |||||
✓ | ✓ | p3:=b3(3−1x−y) | ||||||
✓ | p4:=b4(2−yx) | |||||||
✓ | ✓ | p5:=b5(2−y−1y) |
We now rewrite F(x,y,z) as,
F(x,y,z)=a1(2(1−(1−εA)p)μ−βS∗I∗+αV∗)+a2(2(1−εA)pμ−εLβV∗I∗)+a3β(I∗S∗+εLV∗I∗)−x(a1(1−(1−εA)p)μ−a1βS∗I∗+a1αV∗+a3βI∗S∗)−1xa1(1−(1−εA)p)μ−y(−a1αV∗+a2(1−εA)pμ−a2εLβV∗I∗+a3βεLI∗V∗)−1ya2(1−εA)pμ−yxa1αV∗+z(a1βS∗I∗+a2εLβV∗I∗−a3βI∗S∗−a3βεLV∗I∗)+xz(−a1βS∗I∗+a3βI∗S∗)+yz(−a2εLβV∗I∗+a3βεLV∗I∗) |
Step 2. Set the terms multiplying the unmarked terms in Table 2 for function F(x,y,z) to zero. This means we set the terms multiplying z, xz, yz all equal to zero:
a1βS∗I∗+a2εLβV∗I∗−a3βI∗S∗−a3βεLV∗I∗=0−a1βS∗I∗+a3βI∗S∗=0−a2εLβV∗I∗+a3βεLV∗I∗=0 |
A solution to this system is a1=a2=a3=1. Using this, the function L(x,y,z) is positive definite. Substituting these values into F(x,y,z) and using the fact that (V∗,S∗,I∗,R∗) is an endemic equilibrium (substituting them into the right-hand-side of (2.1) yields zeros) to simplify terms we derive,
F(x,y,z)=[2(1−(1−εA)p)μ+2(1−εA)pμ+αV∗]−(βS∗I∗+μS∗)x−(1−(1−εA)p)μ1x−(εLβV∗I∗+μV∗)y−(1−εA)pμ1y−αV∗yx |
Step 3. Let the coefficients for the same terms between F(x,y,z) and ∑5k=1pk be equal, which yields the following equations:
b1+b2=βS∗I∗+μS∗b1+b3=(1−(1−εA)p)μb3+b5=εLβV∗I∗+μV∗b2+b5=(1−εA)pμb2+b4=αV∗ | (3.6) |
We note that this is a linear system of five equations with five unknowns and rank of four. We can solve for the b1 through b4 in terms of b5,
b1=(1−(1−εA)p)μ−εLβV∗I∗−μV∗+b5b2=(1−εA)pμ−b5b3=εLβV∗I∗+μV∗−b5b4=αV∗−(1−εA)pμ+b5 | (3.7) |
Note that we did not use b1+b2=βS∗I∗+μS∗ in (3.6) to get (3.7). Substituting in the expressions for b1 and b2 to this equation yields,
(1−(1−εA)p)μ−εLβV∗I∗−μV∗+b5+(1−εA)pμ−b5=βS∗I∗+μS∗μ(1−V∗−S∗)−β(S∗+εLV∗)I∗=0μ(I∗+R∗)−β(1R0)I∗=0μ(I∗+R∗)−(γ+μ)I∗=0μR∗−γI∗=0 |
This equation indeed holds from endemic equilibrium, so this verifies that the system of Eqs (3.6) is consistent. To guarantee that all the bi's are nonnegative, b5 should satisfy the following inequality:
max{0,(1−εA)pμ−αV∗,εLβV∗I∗+μV∗−(1−(1−εA)p)μ}≤b5≤min{(1−εA)pμ,εLβV∗I∗+μV∗} | (3.8) |
We can further simplify this by noticing from the endemic equilibrium equations that
(1−εA)pμ=εLβV∗I∗+αV∗+μV∗, |
which implies that εLβV∗I∗+μV∗<(1−εA)pμ. Additionally, we can rearrange the equation to yield,
(1−εA)pμ−αV∗=εLβV∗I∗+μV∗, |
which implies that (1−εA)pμ−αV∗=εLβV∗I∗+μV∗≥εLβV∗I∗+μV∗−(1−(1−εA)p)μ. Thus, the inequality (3.8) simplifies to max{0,εLβV∗I∗+μV∗}≤b5≤εLβV∗I∗+μV∗ which yields,
b5=εLβV∗I∗+μV∗=(1−εA)pμ−αV∗. | (3.9) |
Step 4. From (3.7),
2b1+3b2+3b3+2b4+2b5=2[(1−(1−εA)p)μ−εLβV∗I∗−μV∗]+3[(1−εA)pμ]+3[εLβV∗I∗+μV∗]+2[αV∗−(1−εA)pμ]=2(1−(1−εA)p)μ+εLβV∗I∗+μV∗+(1−εA)pμ+2αV∗=2(1−(1−εA)p)μ+2(1−εA)pμ+αV∗ |
The last line comes from substituting in εLβV∗I∗+μV∗=(1−εA)pμ−αV∗ which comes from the equations for the endemic equilibria. This step verifies that the constant terms in F(x,y,z) and ∑5k=1pk are equal.
Finally, by putting together Steps 1-4 we find that the function F(x,y,z) can be rewritten in the form of ∑5k=1pk=∑5k=1bk(nk−hk,1−hk,2−⋯−hk,nk) with
b1=(1−(1−εA)p)μb2=αV∗b3=0b4=0b5=εLβV∗I∗+μV∗=(1−εA)pμ−αV∗. | (3.10) |
Thus,
F(x,y,z)=(1−(1−εA)p)μ(2−x−1x)+αV∗(3−x−1y−yx)+(εLβV∗I∗+μV∗)(2−y−1y) |
The property that the arithmetic mean is greater than or equal to the geometric mean implies that F(x,y,z)≤0 for all positive values of x, y and z, and that the equality only holds only for x=y=1. This means that {(x,y,z)∈R3+:F(x,y,z)=0}={(x,y,z):x=y=1,z>0}. The maximum invariant subset of this set only contains (1,1,1). By LaSalle's Invariance Principle, this equilibrium is globally asymptotically stable for all initializations in the positive cone. It follows that the endemic equilibrium (V∗,S∗,I∗,R∗) is also globally stable for all non-negative initial conditions.
When Rp>1, the global stability of the endemic equilibrium (Proposition 5) and the fact that S∗E=1R0 independent of the value of p (Proposition 4) motivates us to introduce the following technical definition for the honeymoon period in Definition 6.
Definition 6. Let p>0 and Rp>1. Suppose that the initial conditions of the model Eqs (2.1) are the pre-vaccine era endemic equilibrium (the endemic equilibrium of the system when p is set to zero). This means,
V(0)=0,S(0)=1R0,I(0)=μβ(R0−1),R(0)=γβ(R0−1). |
Define the length of the honeymoon period of the system to be
T=min{t>0:SE(t)=1R0}, | (3.11) |
if this exists and T=∞ otherwise. The relative drop in the effective susceptible class is defined to be
d=S∗E−min{SE(t):t∈(0,T)}S∗E=1−R0min{SE(t),t∈(0,T)}, | (3.12) |
if this exists.
Under the conditions given by Definition 6, we say that the honeymoon period is the time interval (0,T). During this time, we expect that the effective number of susceptibles in the population is below its equilibrium value of 1R0 and that the infectious fraction of the population is decreasing. These statements are proven later in Proposition 7.
We plotted the length of the honeymoon period in Figure 6 for different values of transmission rate β and vaccine failure parameter (εL, εA and εW for the purely leaky, all-or-nothing and waning models respectively). The length of the honeymoon period is shown using a color bar in a log-scale. This plot shows that the waning model generally has longer honeymoon periods over the two other models.
We also plotted the relative drop in the effective susceptible class (SE in Definition 6) after the start of mass vaccination in Figure 7. We note that the waning model generally displays a larger relative drop in effective susceptibles than the other two models. The border between the colored and white area corresponds to the region where Rp=1 and a transcritical bifurcation occurs with the stability switching between the endemic and disease-free equilibria.
The results in Figures 4 show that reactivity and amplification envelope [5], two measures of transient dynamics based on linearization techniques, may not clearly distinguish which types of vaccine models will display significant honeymoon periods. This is shown when we computed these quantities for models and parameter regimes that exhibit very clearly different honeymoon periods in their numerically computed trajectories shown in Figures 3 and 5. In both the all-or-nothing and waning models we have εL=0 which sets several elements in the Jacobian (2.9) to zero. Additionally, these two models have the same values at endemic equilibrium resulting in very similar values for their reactivity and amplification envelopes. We also note that the drop in the reactivity for the leaky model in Figure 4(a) occurs near the so-called reinfection threshold where R0=1εL [10]. This is a subject we are looking into for further study.
In Figures 3 and 5 the drop in incidence that occur during honeymoon periods follow an initial drop in the effective number of susceptibles in the population. This drop in incidence eventually results in the effective number of susceptibles slowly building up again. At the end of the honeymoon period, disease outbreaks may occur due to a buildup in effectively susceptible individuals during the low incidence period. Eventually the effective susceptible population recover to its equilibrium value of 1R0 which is the same value independent of the vaccine coverage and type of vaccine failure (Proposition 4). This allowed us to give definitions for two features of the honeymoon period: its length T and the relative drop in the effective susceptible population SE (Definition 6). These quantities are measured in Figures 6, 7. From these figures we clearly see that the waning model has a propensity for longer and more pronounced honeymoon periods than the leaky and all-or-nothing models. The length of the honeymoon period depends on the model type and parameter values, as we can see in the Figure 6. The longest honeymoon periods seem to occur close to the boundary where Rp=1.
As discussed earlier, we expect that during the honeymoon period the effective number of susceptibles in the population is below its equilibrium value and that the infectious fraction of the population is decreasing. We establish these basic properties of the honeymoon period and the behavior of solutions to (2.1) during this period in Proposition 7.
Proposition 7. Let p>0 and Rp>1.
1. SE(t)∈(0,1R0) for t∈(0,T).
2. dI(t)dt<0 for t∈(0,T).
Proof. To prove Part 1 we first solve for dSEdt=dSdt+εLdVdt and group some terms together to get,
dSEdt=[1−(1−εL)(1−εA)p]μ−μSE−β(S+ε2LV)I+α(1−εL)V. |
We find that this derivative must be negative at t=0 by using the values of the prevaccine era endemic equilibrium as initial conditions (Definition 6).
dSEdt|t=0=−(1−εL)(1−εA)pμ<0. |
Thus there exists δ>0 such that dSEdt<0 for all t∈[0,δ), implying that T>0 and the honeymoon period interval is nonempty. Since we initialize the system with SE(0)=1R0, by the definition of T we must have SE(t)<1R0 for t∈(0,T). The invariance of the system (2.1) in the positive cone implies that SE(t)∈(0,1R0) for t∈(0,T).
We note also here that from Proposition 4 the endemic equilibrium with or without vaccination is S∗E=1R0. Thus SE(t)→1R0 by the global stability of the endemic equilibrium (Proposition 5). We note that in many numerically generated trajectories, we have found that SE(t) approaches 1R0 in an oscillatory manner, implying that T is finite. Looking at the properties of how the vaccine era endemic equilibrium is approached is a topic for future study.
To prove Part 2, we rewrite the equation for the derivative of I in (2.1) as,
dIdt=(βSE(t)−(γ+μ))I=(SE(t)−1R0)βI. |
Then dIdt<0 for t∈(0,T) follows from Part 1.
Part 2 of Proposition 7 imply that if the disease is going to rebound, this can only occur after the honeymoon period. The I class is continuously decreasing during the entire honeymoon period.
It is difficult to model childhood diseases and vaccination [9]. There is a lot of interest in modeling these correctly because vaccination is one of the most powerful and cost-effective interventions for diseases. While most studies focus on the equilibrium behavior of these systems, measuring and understanding the transient behavior is also important as they can last for very long periods of time. Transient behaviors, such as honeymoon periods can also provide clues into the type of imperfect vaccine that is being used, and that can be very important for the design and cost-benefit evaluation of the rollout of vaccination for some diseases.
In this manuscript we reviewed the VSIR model analyzed in [1] which allows for three modes of vaccine failure: leaky, all-or-nothing, and waning. It has previously been observed that, given the same value of vaccine impact, the all-or-nothing and waning models have the same endemic equilibrium, so it is not possible to distinguish between them at equilibrium. However, by studying their transient dynamics numerically, especially the dynamics after the start of mass vaccination, we often observe very clear differences in how these models approach the vaccine era endemic equilibrium. For some parameter values, the waning model may display a very pronounced honeymoon period while the all-or-nothing model with the same vaccine impact displays a less eventful drop in incidence to its new equilibrium value.
We attempted to use linearization methods in ecology to characterize which models can display honeymoon periods. We reviewed the reactivity and amplification envelope [5], however the results show that these cannot distinguish which of the all-or-nothing and waning models will exhibit significant honeymoon periods. So far, we can only confirm if a model displays honeymoon periods when we numerically solve for the trajectory of the models.
As a basis for future study on honeymoon periods, we presented a technical definition of some features of this transient period in Definition 6 and proves some properties of the solutions during this time interval in Proposition 7 (using the global stability of the endemic equilibrium in Proposition 5). These features were (1) the length of the honeymoon period (plotted in Figure 6 for different values of transmission rate and vaccine failure parameter) and (2) the relative drop in the susceptible class (plotted in Figure 7). From this we saw that the waning model usually has the largest relative drop in the effective susceptible class after the start of mass vaccination, and the longest honeymoon period. Interestingly, the trajectory of the effective number of susceptibles for the leaky model is similar to that of the all-or-nothing model.
There is still a lot to learn about honeymoon periods. The results of this study indicate that they may not be detected by the reactivity and amplification envelope, measures of transient dynamics that are based on linearization techniques. Further study of the honeymoon periods will probably require nonlinear techniques.
We would like to thank Queen's University, the University of Manitoba and NSERC for funding this research. We would also like to thank J. Arino, S. Portet, P. Taylor, T. Day, P. Rohani and A.A. King for their helpful comments and suggestions that have helped us with the development of ideas in this manuscript.
We have no conflicts of interest to disclose.
[1] |
F. M. G. Magpantay, M. A. Riolo, M. D. De Celles, A. A. King, P. Rohani, Epidemiological consequences of imperfect vaccines for immunizing infections, SIAM J. Appl. Math., 74 (2014), 1810-1830. doi: 10.1137/140956695
![]() |
[2] |
A. McLean, R. Anderson, Measles in developing countries. part ii. the predicted impact of mass vaccination, Epidemiol. Infect., 100 (1988), 419-442. doi: 10.1017/S0950268800067170
![]() |
[3] | J. Heffernan, M. Keeling, Implications of vaccination and waning immunity, P. Roy. Soc. B-Biol. Sci., 276 (2009), 2071-2080. |
[4] |
J. Mossong, C. P. Muller, Modelling measles re-emergence as a result of waning of immunity in vaccinated populations, Vaccine, 21 (2003), 4597-4603. doi: 10.1016/S0264-410X(03)00449-3
![]() |
[5] |
M. G. Neubert, H. Caswell, Alternatives to resilience for measuring the responses of ecological systems to perturbations, Ecology, 78 (1997), 653-665. doi: 10.1890/0012-9658(1997)078[0653:ATRFMT]2.0.CO;2
![]() |
[6] |
A. R. McLean, S. M. Blower, Imperfect vaccines and herd immunity to HIV, P. Roy. Soc. B-Biol. Sci., 253 (1993), 9-13. doi: 10.1098/rspb.1993.0075
![]() |
[7] |
S. M. O'Regan, E. B. O'Dea, P. Rohani, J. M. Drake, Transient indicators of tipping points in infectious diseases, J. R. Soc. Interface, 17 (2020), 20200094. doi: 10.1098/rsif.2020.0094
![]() |
[8] |
J. Li, Y. Yang, Y. Zhou, Global stability of an epidemic model with latent stage and vaccination, Nonlinear Anal.- Real, 12 (2011), 2163-2173. doi: 10.1016/j.nonrwa.2010.12.030
![]() |
[9] | C. Allotey, A comparison of existing measles models, Master's thesis, University of Manitoba, 2017. |
[10] | M. Gomes, L. White, G. Medley, Infection, reinfection, an vaccination under suboptimal immune protection: epidemiological perspectives, J. Theor. Biol., 228 (2004), 539-549. |
[11] | W. van Panhuis, J. Grefenstette, S. Jung, N. Chok, A. Cross, H. Eng, et al., Contagious diseases in the United States from 1888 to the present, New Engl. J. Med., 369 (2013), 2152-2158. |
1. | Maryam Shafaati, Kowsar Bagherzadeh, Majid Lotfinia, Hesam Karimi, Ali Teimoori, Mehdi Razazian, Sepideh Meidaninikjeh, Hamed Hosseini, Hamid Reza Jamshidi, Hasan Jalili, Asghar Abdoli, The protection quest is a primary key to sharing the neutralizing antibody response to cover against all emerging VOCs based on BIV1-CovIran studies, 2023, 9, 24058440, e14108, 10.1016/j.heliyon.2023.e14108 | |
2. | Ugo Avila-Ponce de León, Eric Avila-Vales, Kuan-lin Huang, Modeling the Transmission of the SARS-CoV-2 Delta Variant in a Partially Vaccinated Population, 2022, 14, 1999-4915, 158, 10.3390/v14010158 | |
3. | Fernando Javier Aguilar-Canto, Ugo Avila-Ponce de León, Eric Avila-Vales, Sensitivity theorems of a model of multiple imperfect vaccines for COVID-19, 2022, 156, 09600779, 111844, 10.1016/j.chaos.2022.111844 | |
4. | Bradford Greening, Martin I. Meltzer, 2023, Chapter 60-1, 978-1-4939-9544-8, 1, 10.1007/978-1-4939-9544-8_60-1 | |
5. | Ankai Liu, Felicia Maria G. Magpantay, Kenzu Abdella, A framework for long-lasting, slowly varying transient dynamics, 2023, 20, 1551-0018, 12130, 10.3934/mbe.2023540 | |
6. | F.M.G. Magpantay, J. Mao, S. Ren, S. Zhao, T. Meadows, The reinfection threshold, revisited, 2023, 363, 00255564, 109045, 10.1016/j.mbs.2023.109045 |
Symbol | Description | Default value in plots (if not specified) |
μ | Birth and death rate | 170 yr−1 |
γ | Recovery rate | 20 yr−1 |
β | Transmission rate | 150 yr−1 |
εL | Ratio of the probability a vaccinated individual will get infected after exposure relative to that of a susceptible individual | 0.2 |
εA | Probability of not getting protected after vaccination | 0.2 |
α | Waning rate of vaccine-derived immunity | μεW1−εW |
εW | Probability that the vaccine protection of individuals in the class V wanes within a lifetime, equal to εW=αα+μ | 0.2 |
p | Fraction of newborns vaccinated | 0.9 |
x | 1x | y | 1y | yx | z | xz | yz | term |
✓ | ✓ | p1:=b1(2−x−1x) | ||||||
✓ | ✓ | ✓ | p2:=b2(3−x−1y−yx) | |||||
✓ | ✓ | p3:=b3(3−1x−y) | ||||||
✓ | p4:=b4(2−yx) | |||||||
✓ | ✓ | p5:=b5(2−y−1y) |
Symbol | Description | Default value in plots (if not specified) |
μ | Birth and death rate | 170 yr−1 |
γ | Recovery rate | 20 yr−1 |
β | Transmission rate | 150 yr−1 |
εL | Ratio of the probability a vaccinated individual will get infected after exposure relative to that of a susceptible individual | 0.2 |
εA | Probability of not getting protected after vaccination | 0.2 |
α | Waning rate of vaccine-derived immunity | μεW1−εW |
εW | Probability that the vaccine protection of individuals in the class V wanes within a lifetime, equal to εW=αα+μ | 0.2 |
p | Fraction of newborns vaccinated | 0.9 |
x | 1x | y | 1y | yx | z | xz | yz | term |
✓ | ✓ | p1:=b1(2−x−1x) | ||||||
✓ | ✓ | ✓ | p2:=b2(3−x−1y−yx) | |||||
✓ | ✓ | p3:=b3(3−1x−y) | ||||||
✓ | p4:=b4(2−yx) | |||||||
✓ | ✓ | p5:=b5(2−y−1y) |