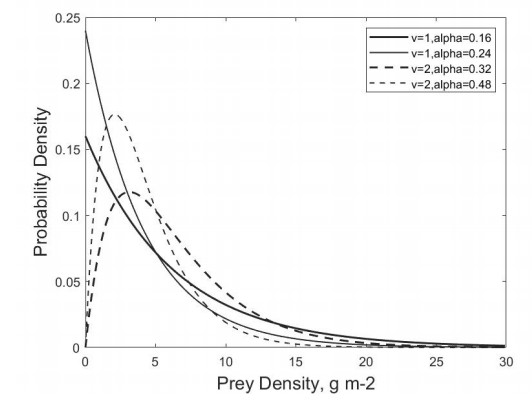
Tactile-feeding wading birds, such as wood storks and white ibises, require high densities of prey such as small fishes and crayfish to support themselves and their offspring during the breeding season. Prey availability in wetlands is often determined by seasonal hydrologic pulsing, such as in the subtropical Everglades, where spatial distributions of prey can vary through time, becoming heterogeneously clumped in patches, such as ponds or sloughs, as the wetland dries out. In this mathematical modeling study, we selected two possible foraging strategies to examine how they impact total energetic intake over a time scale of one day. In the first, wading birds sample prey patches without a priori knowledge of the patches' prey densities, moving from patch to patch, staying long enough to estimate the prey density, until they find one that meets a predetermined satisfactory threshold, and then staying there for a longer period. For this case, we solve for a wading bird's expected prey intake over the course of a day, given varying theoretical probability distributions of patch prey densities across the landscape. In the second strategy considered, it is assumed that the wading bird samples a given number of patches, and then uses memory to return to the highest quality patch. Our results show how total intake over a day is impacted by assumptions of the parameters governing the spatial distribution of prey among patches, which is a key source of parameter uncertainty in both natural and managed ecosystems. Perhaps surprisingly, the foraging strategy that uses a prey density threshold generally led to higher maximum potential prey intake than the strategy for using memory to return to the best patch sampled. These results will contribute to understanding the foraging of wading birds and to the management of wetlands.
Citation: Hyo Won Lee, Donald L. DeAngelis, Simeon Yurek, Stephen Tennenbaum. Wading bird foraging on a wetland landscape: a comparison of two strategies[J]. Mathematical Biosciences and Engineering, 2022, 19(8): 7687-7718. doi: 10.3934/mbe.2022361
[1] | Nazanin Zaker, Christina A. Cobbold, Frithjof Lutscher . The effect of landscape fragmentation on Turing-pattern formation. Mathematical Biosciences and Engineering, 2022, 19(3): 2506-2537. doi: 10.3934/mbe.2022116 |
[2] | William Wolesensky, J. David Logan . An individual, stochastic model of growth incorporating state-dependent risk and random foraging and climate. Mathematical Biosciences and Engineering, 2007, 4(1): 67-84. doi: 10.3934/mbe.2007.4.67 |
[3] | Mayee Chen, Junping Shi . Effect of rotational grazing on plant and animal production. Mathematical Biosciences and Engineering, 2018, 15(2): 393-406. doi: 10.3934/mbe.2018017 |
[4] | Yun Kang, Sourav Kumar Sasmal, Komi Messan . A two-patch prey-predator model with predator dispersal driven by the predation strength. Mathematical Biosciences and Engineering, 2017, 14(4): 843-880. doi: 10.3934/mbe.2017046 |
[5] | Christopher M. Kribs-Zaleta, Christopher Mitchell . Modeling colony collapse disorder in honeybees as a contagion. Mathematical Biosciences and Engineering, 2014, 11(6): 1275-1294. doi: 10.3934/mbe.2014.11.1275 |
[6] | Xiaorui Yang, Yumei Zhang, Xiaojiao Lv, Honghong Yang, Zengguo Sun, Xiaojun Wu . Hybrid multi-strategy chaos somersault foraging chimp optimization algorithm research. Mathematical Biosciences and Engineering, 2023, 20(7): 12263-12297. doi: 10.3934/mbe.2023546 |
[7] | Soumitra Pal, Pankaj Kumar Tiwari, Arvind Kumar Misra, Hao Wang . Fear effect in a three-species food chain model with generalist predator. Mathematical Biosciences and Engineering, 2024, 21(1): 1-33. doi: 10.3934/mbe.2024001 |
[8] | Nalin Fonseka, Jerome Goddard Ⅱ, Alketa Henderson, Dustin Nichols, Ratnasingham Shivaji . Modeling effects of matrix heterogeneity on population persistence at the patch-level. Mathematical Biosciences and Engineering, 2022, 19(12): 13675-13709. doi: 10.3934/mbe.2022638 |
[9] | Zhiqiang Wang, Jinzhu Peng, Shuai Ding . A Bio-inspired trajectory planning method for robotic manipulators based on improved bacteria foraging optimization algorithm and tau theory. Mathematical Biosciences and Engineering, 2022, 19(1): 643-662. doi: 10.3934/mbe.2022029 |
[10] | Rong Zheng, Heming Jia, Laith Abualigah, Shuang Wang, Di Wu . An improved remora optimization algorithm with autonomous foraging mechanism for global optimization problems. Mathematical Biosciences and Engineering, 2022, 19(4): 3994-4037. doi: 10.3934/mbe.2022184 |
Tactile-feeding wading birds, such as wood storks and white ibises, require high densities of prey such as small fishes and crayfish to support themselves and their offspring during the breeding season. Prey availability in wetlands is often determined by seasonal hydrologic pulsing, such as in the subtropical Everglades, where spatial distributions of prey can vary through time, becoming heterogeneously clumped in patches, such as ponds or sloughs, as the wetland dries out. In this mathematical modeling study, we selected two possible foraging strategies to examine how they impact total energetic intake over a time scale of one day. In the first, wading birds sample prey patches without a priori knowledge of the patches' prey densities, moving from patch to patch, staying long enough to estimate the prey density, until they find one that meets a predetermined satisfactory threshold, and then staying there for a longer period. For this case, we solve for a wading bird's expected prey intake over the course of a day, given varying theoretical probability distributions of patch prey densities across the landscape. In the second strategy considered, it is assumed that the wading bird samples a given number of patches, and then uses memory to return to the highest quality patch. Our results show how total intake over a day is impacted by assumptions of the parameters governing the spatial distribution of prey among patches, which is a key source of parameter uncertainty in both natural and managed ecosystems. Perhaps surprisingly, the foraging strategy that uses a prey density threshold generally led to higher maximum potential prey intake than the strategy for using memory to return to the best patch sampled. These results will contribute to understanding the foraging of wading birds and to the management of wetlands.
Stephen Gourley's contributions to theoretical ecology are many and wide-ranging. These include representations of foragers on the landscape, such as herbivore foraging (snow-shoe hare [1,2,3] and the patchy spatiotemporal distribution of a wetland bird [4]. The topic of our present paper, wading bird foraging in patchy landscapes, follows similar themes.
Unlike the large-scale distribution of patches used by migratory geese described in [1], we consider a smaller spatial scale of a local set of patches that a wading bird can access and forage within a short time period of one day. Tactile feeding wading birds forage on high concentrations of prey, such as small fishes and crayfish that form in spatially dynamic wetlands. The general process of concentration involves growth and spatial expansion of prey populations across an inundated landscape during the wet season, followed by spatial contraction of wetlands during the dry season, when prey become isolated in drying patches as the patches disconnect from the greater landscape. In this way, the spatial distributions of both hydrology and prey are seasonally pulsed. These dynamics are typical of many seasonal wetlands, such as the Everglades in Florida (USA), and the Pantanal in Brazil.
One important aspect is that sufficient prey is continuously available and consumed to meet energetic demands of both the adult and its clutch of offspring over long breeding periods. Prey must therefore be successfully located and delivered to chicks each day to ensure their survival. To meet these needs, wading birds often form large breeding colonies in the vicinity of foraging hotspots, which we term 'patches'. They often make use of cues from other birds and of memory to locate and retrieve prey [5,6]. Within a given day, prey availability may be relatively constant in a given patch; however, across the patches there could be considerable variation in the prey densities. On a given day, a wading bird forager will have a choice among many potential patches to acquire prey. We are interested in understanding how variations in spatial foraging strategy, i.e., how to choose how long to stay in patches of varying prey density; can impact total daily energetic consumption. In particular, can different patch selection behaviors, such as choosing to stay within or abandon a patch, result in more or less efficient consumption? We assume that during periods of high energetic needs, such as raising offspring, the forager can obtain greater intake than what is available on average across the landscape by preferentially selecting among locally concentrated prey patches. However, the specific pattern of remaining in or abandoning particular patches that result in the greatest overall yield needs to be determined.
The concepts mentioned above have been examined theoretically by ecologists over several decades, because foraging on a landscape with a heterogeneous array of patches is ubiquitous. For example, ecologists have developed theory for how a forager might maximize its intake of prey during a given time period or, alternatively, minimize the time spent foraging to acquire a desired amount of prey. For foraging in a set of patches within a time period such as a day, Charnov's (1976) [7] marginal value approach suggests that a forager should depart from its current patch and seek to add a new patch to its "itinerary", when the prey availability at the current patch falls below the average level of other patches that could be reached. That implies the concept of a 'giving up density' or GUD; that is, an available prey density at which the forager will leave a given patch to search for another. Charnov's theory considers there to be depletion of prey over the time scale of interest, such as foraging for seeds in a small patch. In our case, a patch exploited by a wading bird might be a pond whose stock of prey is large, such that the prey densities on the time scale of a single day, or at least during the time interval that an individual utilizes it, can be reasonably assumed not to change appreciably. Part of the reason is also that prey densities can actually increase as the area of inundated habitat contracts with wetland drying, despite rapid depletion by foraging. We are not accounting for these complex landscape dynamics here, and simply assume no change in prey density over a day. Thus, the time scale of estimation of prey density by the forager and the decision to remain in or abandon a patch is relatively brief, and the important scale considered in this study is across space, that is, among patches, rather than through time. However, we do acknowledge that time-dynamic prey availability is a critical aspect of wading bird ecology, which can be addressed in future studies.
We examine strategies in which the forager starts a day with no a priori knowledge about the prey densities of available patches. That is, it has no memory from previous days and does not follow cues from other wading birds. It may however have a general expectation about the possible prey densities across the patches over the whole landscape in relation to its needs. We consider two general strategies to which such expectations could lead. The first strategy is "threshold-based", in which the forager learns about prey densities upon arrival at the patch. It remains in the patch if conditions of prey on the landscape; i.e., high densities in shallow and accessible patches, would enable the forager to encounter and obtain sufficient intake to meet its target energetic threshold. Otherwise, it moves on to another patch. The second strategy is "memory-based", allowing the bird to store memory of prey densities over a short sampling time over a series of patches, and then return to the patch with the highest value. In both of the strategies, sampling is assumed to be made in swift bouts, small portions of the day. We are interested in comparing how the reward, that is, total potential daily energetic consumption, may vary with strategy and landscape conditions and the details of how the wading bird employs either strategy. The first approach follows that of Stephens and Krebs [8] in which a GUD can be assumed, which we translate to a 'giving up time', or GUT, following [9], assuming that some minimum time is required to assess patch quality. The second approach considers a cognitive ability of the wading bird to assess and remember spatial variation in prey density across the landscape over short sampling periods, and to decide which are most suitable. Although wading bird cognition is still not well understood, some studies suggest a role in foraging on spatially complex prey landscapes [10]. The difference between these two strategies is that the first case is regulated more by an expectation of the foraging bird, while the second reflects actual availability of prey determined by the landscape; however, both cases represent complex interactions between forager and landscape, determining energetic intake for the forager.
Here we develop a mathematical model for a wading bird forager, employing these two foraging strategies during the dry season, which is a critical time for reproduction and rearing of offspring. The prey densities across the landscape are represented using simple mathematical distribution functions.
The environment of the foraging wading bird is assumed to consist of potential foraging patches that are sufficiently numerous that the forager can move among patches with a large range of prey availabilities. We assume here that prey availability is equivalent to prey density, although acknowledge that availability can be impacted by a complexity of factors such as water depth. It is convenient for this theoretical study, in the absence of accurate empirical knowledge concerning prey densities across the landscape, to represent the range of potential prey conditions among foraging sites by an analytic probability density. The gamma distribution is flexible enough to describe a wide range of distributions with only two parameters, ν and α,
f(preydens)=αvΓ(v)e−α∗preydens(preydens)v−1, | (2.1) |
where preydens is the prey density, α and v are rate and shape parameters, and Γ(v) is the gamma function. For example, for v = 1 (α = 0.16, 0.24), and v = 2 (α = 0.32, 0.48) the probability density function f(preydens) has the forms shown in Figure 1. The v = 1 case is a negative exponential function such that the great majority of patches have prey biomass densities less than 10 g m-2, although some patches have at least 15 g m-2. The cases in which v = 2 are qualitatively different in that the probability density is unimodal. In both cases, parameter α affects the rate of decline of the tail of the distribution.
It is assumed here that the wading bird forages over the course of a single day using one or the other sampling strategies mentioned above (described in detail later), on a landscape of foraging patches with prey density varying among patches following the gamma distribution. The basic assumptions are as follows.
1) The wading bird has a target daily energetic requirement, preyneeded, which it attempts to fulfill through cumulative prey acquisition through feeding among patches, within a specified time. It may not meet this quota if the prey densities that it encounters are too low.
2) The wading bird has no a priori knowledge of the prey availability of the patches that it visits, such as memory from previous days. However, the effect of within-day memory will be studied as part of the second assumed strategy.
3) The wading bird feeds in every patch it visits, continuously, for as long as it is there, even though it may decide to leave the patch after deciding that prey density is too low.
4) There is an upper time limit, T minutes, for total foraging time within a day, and thus an upper limit on patches visited. The forager visits patches, given other rules below, until it reaches a desired intake of prey.
5) The time to travel from one foraging site to the next, ttransition, is included as part of total time, T. This is counted for each patch visited and is assumed the same for each patch. The wading bird does not consume food during travel, thus travel time counts against the total time spent actively feeding.
6) In strategy 1, upon arriving at a patch, the wading bird undertakes a short decision-making period, tmin, during which it samples prey through feeding, estimates the prey availability of the patch, and then decides whether to stay or leave the patch, based on a defined threshold, preythreshold. In strategy 2, the wading bird samples a pre-determined number of patches for short periods, and then returns to the patch with highest density.
7) The prey density within a given foraging site is not depleted while the forager is present in the patch.
8) There are no interactions (e.g., competition, information transfer such as using cues) with other wading birds. Also, risk of predation is not included.
This set of assumptions simplifies the complex interactions that actually exist between wading bird foraging behavior and dynamic wetland environments in the real world; however, most can easily be relaxed in the future as necessary. Given these conditions, the wading bird's general foraging strategy consists of the overall objective of at least meeting the daily requirement, preyneeded, and if met, a secondary objective of minimizing the time spent foraging, which is determined by whether or not to continue foraging after preyneeded is reached. If preyneeded is not met, the wading bird forages for the whole time period, T, obtaining what prey intake it can over that period. Strategy 1 of the wading bird is to sample a succession of foraging patches until it arrives at a patch in which the prey density exceeds a threshold value, preythreshold, (we will use that term rather than GUD here) at which point the wading bird stays until either it acquires its limit, preyneeded, or it reaches the time limit, T. In the case of strategy 2, the wading bird visits a predetermined number of patches before returning to the best patch has visited, to optimize overall energetic intake. This represents a tradeoff between opportunity for finding a high-quality patch and time spent feeding within it.
The wading bird follows the sampling approach until it finds a patch that it considers satisfactory and then stays in that patch.
Since f(preydens) is the probability of encountering a patch with a given prey density, the cumulative probability of f(preydens), Q, integrated from 0 to preythreshold, is the probability that a foraging patch chosen at random will have a prey density that is less than preythreshold. Therefore, we define the probability, P, of exceeding the threshold in a random visit as one minus the cumulative probability (Figure 2),
P=1−∫preythreshold0f(preydens)dpreydens= 1−∫preythreshold0αvΓ(v)e−α∗preydens(preydens)v−1dpreydens. |
For the gamma distribution v = 1, since Γ(1)=1, this can be shown to be
P=1−(1−e−α∗preythreshold )=e−α∗preythreshold . |
For v = 2, since Γ(2)=1, this is
P=1−1+e−α∗preythreshold+α∗preythesholde−α∗preythreshold= e−α∗preythreshold+α∗preythresholde−α∗preythreshold |
In both cases, P is equal to 1 when preythreshold=0 and decreases toward zero as preythreshold increases. This decrease is slower for smaller values of α.
Table 1 illustrates various scenarios of visits to patches in which the wading bird succeeds in the 1st, 2nd, …, or Nth patch to find prey density greater than the threshold density. The probabilities of success being achieved for any of these cases are listed in column six, where Q = 1 – P. Columns three and four are the cumulative times spent traveling to patches and the times spent in patches of lower density than preythreshold. Column five is the total time available for foraging in the patch in which preydensity>preythreshold, although the wading bird may choose to stay in that patch only as long as it takes to reach preyneeded.
Success at nth trial | Illustration | Transition time | Time spent in low quality patches | Time spent in the high quality patch | Chance to happen |
1st | ![]() |
ttransition | 0 | T−(ttransition+tmin)+tmin | P |
2nd | ![]() |
2∗ttransition | tmin | T−2(ttransition+tmin)+tmin | P*Q |
3rd | ![]() |
3∗ttransition | 2∗tmin | T−3(ttransition+tmin)+tmin | P*Q*Q |
Nth | ![]() |
N∗ttransition | (N−1)∗tmin | T−N(ttransition+tmin)+tmin=tmin | P*Q(N-1) |
Never find patch>threshold | ![]() |
N∗ttransition | N∗tmin | T−N(ttransition+tmin)=0 | QN |
The next step is to account for the time constraints on total foraging time within a day. As mentioned, foraging is partitioned into discrete bouts at separate patches, and each bout requires discrete time periods to travel to and sample each patch, ttransition and tmin, respectively. Thus, a limited number of patches, N, can be visited in a day. Based on tmin and ttransition, and the total foraging hours allowed in a day, T, we calculated this maximum number of patch visits as:
N=maximum numbers of patches to visit=Ttmin+ttransition |
We then apply this constraint to the probability of encountering a given prey density in a patch, described in Section 2.3.1, by taking the cumulative probability across n patches sampled within a day, as follows. Since daily foraging involves n-1 bouts of feeding below the threshold and a single bout of feeding above, the cumulative probability of encountering a prey density above the given acceptable threshold can be expressed as:
∑Nn=1PQn−1=1−QN. |
Thus, the expectation for failure to find an acceptable patch within a day is QN.
Based on Table 1 and Appendix 1, we can also calculate the average time spent to find a patch above threshold, given that the bird finds the patch within the daily time available for foraging.
average time to find patch above threshold assuming that the patch is found |
=∑Nn=1(time costs if the bird find the patch at nth trial )∗(chance to find at nth trial )total chance that birds find a patchabove thresold within N trials |
=∑Nn=1{N(ttransition +tmin )−tmin }PQn−11−QN |
=∑Nn=1(ttransition+tmin)NPQn−1−∑Nn=1tminPQn−11−QN |
=[(ttransition+tmin){(1−QN)P−NQN}−tmin(1−QN)](1−QN)−1 |
=(ttransition+tmin){(1−QN)P−NQN}(1−QN)−1−tmin |
=(ttransition+tmin)[1P−NQN(1−QN)]−tmin | (2.2) |
This represents average time spent to find a patch above threshold, if indeed it finds such a patch above threshold within an upper limit of N trials. In this case, the wading bird spends only the short decision-making period, tmin, in each patch, and then leaves promptly if the prey density does not meet the threshold. The decision to remain within or abandon a patch depends on whether the wading finds a foraging patch that satisfies its requirement that preydens>preythreshold.
For a general probability density function, f(preydens), of prey densities in available patches across a landscape, and a probability of reaching the cumulative probability threshold, P, the expected potential prey intake over the course of a day for a prey threshold, preythreshold, is
Mean expected daily prey intake |
=Cons∗[{(1−QN)P}tmin∗∫preythreshold0f(preydens)preydensitydpreydens+ {T(1+(QN−1)NP)+tmin(1−QN)}∗P−1∫∞preythresholdf(preydens)preydensdpreydens], | (2.3) |
See derivation in Appendix 2, Supplementary Information. The parameter Cons is the rate at which the wading bird captures and consumes prey, given a certain prey density. This value depends on particular species and circumstances. Although this is a value that has been measured for some wading birds in some circumstances, here we will choose an arbitrary value that produces reasonable prey intake rates. We also assume that it is constant, which assumes that a wading bird will continue to feed at the same rate through time; that is, it will have ceased foraging by the time it would have become satiated.
The first term on the right-hand side of Eq (2.3) represents the expected prey intake from patches visited before the wading bird finds a patch that has prey density exceeding the preythreshold, and feeds for intervals of tmin in each of those patches. The factor (1−QN)/P=1 when P = 1 and approaches N when P → 0. Because P = 1 only when preythreshold = 0, this first term of Eq (2.3) only contributes to expected prey intake when P < 1. In the limit that P → 0, which corresponds to preythreshold being high such that the probability of reaching it is small, the prey intake approaches Cons∗tmin∗N∗(Mean prey density over all available patches). That is, the prey intake is the maximum number of patches, N, that can be visited during the time T, each for time tmin, and where the expected intake in each patch is the mean of the densities over the landscape. For 0 < P < 1, the first term of Eq (2.3) represents values of prey intake corresponding to the number of patches visited and the mean prey density in the distribution below the probability threshold P. The second term is the expected prey intake from a patch with prey density > preythreshold that has been reached. The two sub-terms within the braces of the second term represent components of prey intake during the time that the wading bird samples that patch (second sub-term) and the time for the remainder of the available time for foraging in that day (first sub-term). The wading bird may not need to spend that entire time T if it consumes enough prey to meet its and its offspring's needs before the day ends. In that case the forager will likely cease foraging after it has reached preyneeded, or somewhat above that if it wants more than its minimum needs, at some time less than T. Therefore, time minimization would be the objective and the forager will simply stop foraging when its prey intake reaches that point.
Here equations for the expected prey intake are shown. The calculations of the integrals involved in computing the mean prey density encountered by the forager across foraging patches that vary in prey density, as defined by theoretical probability distributions, are given in detail in Appendix 1, where f(preydens)'s are gamma distributions with v = 1 and 2.
v = 1:
Mean expected daily prey intake |
=Cons∗{(1−QN)P}tminimum∗α[1α2−e−α∗preythreshold(preythresholdα+1α2)]+Cons∗{T(1+(QN−1)NP)+tminimum(1−QN)}∗P−1∗α[e−α∗preythreshold(preythresholdα+1α2)] | (2.4) |
v = 2:
Mean expected daily prey intake |
=Cons∗{(1−QN )P}tminimum∗α2(−e−α∗preythreshold [(preythreshold )2α+2∗preythresholdα2+2α3 ]+ [2α3 ] )+Cons∗{T(1+(QN−1 )NP )+tminimum(1−QN )}∗P−1∗α2(+e−α∗preythreshold [(preythreshold )2α+2∗preythresholdα2+2α3 ] ) | (2.5) |
These equations will be used to in calculations shown in Section 3.
In this strategy the forager uses memory within a day as part of its foraging strategy. It samples a predetermined number of patches and then returns to the one that it remembers as having the highest prey density. It then forages in that patch for the remainder of time in the day, or until it reaches, or exceeds to desired level, its goal of prey intake, preyneeded (Figure 3).
In order to model this strategy, it is first necessary to calculate the mean of the maximum prey density that a forager is likely to encounter for any number of trials. This can be done mathematically. We call the number of sampling trials n trials. Then, out of the n trials, the highest prey density is recorded. For example, if the wading bird decides to sample five patches before returning to whichever has highest prey density, then n trials = 5, and the wading bird will return to and continue to feed in the patch until it either reaches preyneeded or the time T is exceeded. The question is, what is the optimal number of patches sampled?
Royston [11] calculated the expected value of the rth largest order statistic when the sample size is n
E(r,n)=n!(r−1)!(n−r)!∫∞0x∗f(x)∗cdf(x)n−r∗{1−cdf(x)}r−1dx |
where cdf(x) is cumulative probability distribution function of f(x).
Based on this, we set r = 1 and x = preydens to calculate expected sample maximum when the birds sampled n numbers of the ponds.
Expected sample maximum |
=n∫∞0preydens∗f(preydens)∗(∫∞0f(preydens)dpreydens)n−1dpreydens |
=n∫∞0preydens∗f(preydens)∗{cdf(preydens)}n−1dpreydens |
=[preydens∗cdf(preydens)]∞0−∫∞0{cdf(preydens)}ndpreydens |
=Emax(n) | (2.6) |
Equation (2.6) is used to calculate the Expected sample maximum for each value of n from 1 to 15 samples. In addition to the analytic calculation, we also performed simulations. For each of these values of n we performed 200 repetitions of sampling to find the average highest prey density that a forager will encounter for any value of n trials. Given that information, the expected prey intake can be calculated. First, the possible times that the forager will spend sampling are noted, and we define the sampling time as
total time spent during the sampling=n∗(tmin+ttransition), |
while the time to return to the best patch is
time cost to return to the best patch=treturn=ttransition. |
An alternative assumption besides treturn=ttransition is that the birds made a number of transitions along a linear path before returning to the best patch, in which case treturn=ttransition (n−1)2.
Based on this result, the expected prey intake from the best patch can be calculated as follows.
Expected intake from the best patch |
=(T−n∗(tmin+ttransition)−treturn+tmin)∗Cons∗Emax(n). |
Next, the average patch quality below that with Emax(n). is calculated as
Cons∗cdf(Emax(n))−1∗∫Emax(n)0preydensf(preydens)dpreydens |
Based on this, the expected prey intake from the rest of the sampled patches can be shown to be
Expected intake from the rest of the sampled patch |
=Cons∗n∗tmin∗cdf(Emax(n))−1∗∫Emax(n)0preydensP(preyden)dpreydens |
From the above equations, the total expected intake with strategy 2 is
Expected daily food intake with strategy 2 |
=Cons[Emax(n){T−n(tmin+ttransition)−treturn+tmin}+ntmincdf(Emax(n))−1∫Emax(n)0preydensP(preyden)dpreydens] | (2.7) |
The above mathematical expressions for prey intake for strategies 1 and 2 are used to calculate the prey intake as a function of both the prey threshold and the shape of the prey probability density. Parameter values used in the calculations are shown in Table 2. This is a theoretical study, and the values chosen here were only assumptions, but could be refined in later studies when empirical data are available. The combinations of parameters for the gamma prey density distributions are, (v = 1, α = 0.16, 0.24) and (v = 2, α = 0.32, 0.48), which are shown in Figure 1, are not entirely arbitrary, as prey densities of the general types represented seem plausible.
Parameter | Definition | Value |
tmin | Minimum time needed to estimate prey density | 20, 40 minutes |
ttransition | Time needed to travel between patches | 20, 40 minutes |
treturn | Time needed to return to the best patch | 20, 40 minutes |
T | Total time available for foraging in a day | 600 minutes |
Cons | Rate at which encountered prey are consumed | 0.175 g minutes-1 |
N | T/(tmin+ttransition) = Maximum numbers of patches wading birds can visit | 15 ponds |
preyneeded | Prey intake needed during day to feed self and offspring | |
preythreshold | Threshold quality during the searching | |
v, α | Parameters from gamma distribution | (1, 0.16), (1, 0.24), (2, 0.32), (2, 0.48) |
The average time spent to find a patch above threshold for a wading bird, given that it is able to do so within N trials (ν = 1, α = 0.16), is shown in Figure 4A, as calculated from Eq (2.2). Figure 4B shows the changes of probability of finding a patch above threshold within N trails as a function of threshold density. The average time to find a patch above threshold for this case does not exceed 300 minutes. However, the probability of finding a patch above threshold approaches 0 as the threshold density increases.
Prey intake was calculated for the four different gamma distributions, (v = 1, α = 0.16), (v = 1, α = 0.24), (v = 2, α = 0.32) and (v = 2, α = 0.48) as shown in Figure 1 and represented analytically by Eqs (2.4) and (2.5). The purpose of using both v = 1 and 2 was to compare the negative exponential form of v = 1 with the unimodal form of v = 2. In comparing the curves of v = 1 and 2, we doubled the value of α for v = 2 compared to v = 1, so that the expected prey intake for preythreshold=0, as well as the expected mean prey density, ν/α, arethe same for paired (v = 1, α = 0.16) and (v = 2, α = 0.32) and for paired (v = 1, α = 0.24) and (v = 2, α = 0.48) prey density distributions. The results for the four cases are shown in Figure 5. Note that these curves represent potential prey intake over a full day. Of course, the wading bird may decide to stop foraging when desired after its prey intake for the day reaches preyneeded.
The dashed horizontal line in Figure 5A represents an estimate of daily energy needed, preyneeded, by an American White Ibis to support both itself and two nestlings, roughly 598 Kcal/day, which translates into about 602 grams wet weight of prey (based on [12]). In the case represented in Figure 5, the foraging wading bird is able to surpass that minimum needed intake by different amounts, over different ranges of preythreshold, depending on the values of v and α. The effect of the parameter in the exponential terms of the gamma distributions, α, clearly has an effect on potential prey intake, with smaller values of α leading to larger prey intake, even if the mean prey density, ν/α, is the same for two cases.
It is also useful to compare the effects of differences in the times needed to sample prey density in a patch and travel between patches. In Figure 6, the results of changing tmin = 20, ttransition = 20 to tmin = 40, ttransition = 40, are compared for the above cases (ν = 1, α = 0.16) and (ν = 2, α = 0.32). Figure 6 shows that both cases have significant decreases of prey intake when the time cost of searching for new patches and for estimating prey density in the patches visited increases. This indicates that time cost of the searching can be one of the main factors that change the daily expected prey intake of wading birds.
More thorough comparisons can be made of the effects of the gamma function parameters on the maximum possible prey intake. We compared maximum expected prey intake under the distributions with the same mean quality (prey density) and different α values. The maximum expected prey intake increased as α decreased for the same mean prey density, termed 'quality' in Figure 7A. This indicates that when the mean prey density value is the same, birds will be more successful when α is smaller. It is also the case that α has a strong effect on the maximum expected prey intake than does ν (Figure 7B).
In examining foraging strategy 2, first we test how successful Eqs (2.6) and (2.7) are in predicting the best sample size and expected prey intake by comparing their predictions with simulations. Based on Eq (2.6), the expected patch sample number that maximizes prey intake is calculated. In addition, we conducted 200 simulations of each sample size to find the maximum value of the sample to calculate the average sample maximum of the simulation. Figure 8 shows that the results of the average of the replicated simulations agree with the theoretical expectation based on Eq (2.6).
Based on calculated Emax(n), Eq (2.6), mean expected daily prey intake was then estimated according to Eq (2.7). We also conducted 200 simulations that used random sampling from the given distribution then calculate the intake according to the strategy 2 assumptions. Figure 9 shows that the results of the simulations compared to the expected value from the Eq (2.7) for (v = 1, α = 0.24).
Based on the success of the comparison of simulations with the analytic curve in Figure 9 for the particular case of (v = 1, α = 0.24), Eq (2.7) appears to provide an accurate estimate of the results of strategy 2. Thus, we can further investigate the changing foraging efficiency of this strategy using the equation. Figure 10A shows that the maximum expected yield from strategy 2 varied according to the values of v and α. The ordering of the expected prey intakes followed the same ordering of values of ν and α as for strategy 1 (Figure 5), with (ν = 1, α = 0.16) leading to the largest prey intakes, even though the distribution with (ν = 2, α = 0.32) has the same mean prey density. However, the results show that the number of patches visited that produce the highest expected prey intake are somewhat closer in number between the four curves, lying between n = 4 and 5 for this case of tmin=20 and ttransition=20.
This memory-based strategy shows slightly lower maximum expected prey intake compared to those of strategy 1 (threshold strategy) for identical v and α values. The results of the simulation indicate that when v becomes larger and α becomes smaller, the maximum expected prey intake increases (Figure 10B). However, this may be caused by changing the mean of the distribution. The mean of the gamma distribution is v/α. Thus, increased value of v and decreased α will cause larger mean of the distribution. When we compared maximum expected prey intake under the distributions with the same mean prey density, termed 'quality' here, and different α values, the maximum expected prey intake increased as α decreased (Figure 10D), showing the relative importance of α in affecting prey intake even for the same mean. This indicates that when the mean value is the same, birds will be more successful for prey distributions with longer tails (smaller α), as shown in Figure 10C for a number of values of α for the same value ν.
We have used modeling to study two strategies for foraging by an individual wading bird for resources in a heterogeneous wetland environment. This modeling was stimulated in part by the decline in wading bird nesting colonies in the Everglades over the past several decades. Understanding the probability of foraging success of wading birds on heterogeneously distributed patches of prey can help in guiding the regulation of water levels that contribute to foraging success.
Using modeling, we calculated the expected prey intake for a forager on hypothetical landscapes with sets of patches of varying prey densities, where the probability densities of patches were described by gamma distributions with different values of parameters v and α. We considered two mathematical representations of possible wading bird foraging strategies. The first, strategy 1, represented the situation in which the forager samples patches in order to find one that exceeds a prey density threshold preythreshold. Once it has found such a patch, it will continue to forage in that patch until it either has acquired some amount of prey intake greater than preyneeded or time T is reached. The aim of the model was to predict, for different assumptions on the gamma distribution, what choice of prey density threshold maximizes potential prey intake. In the second, strategy 2, the forager samples a fixed number of patches and then returns to whichever had the highest prey density. The aim of the model was to predict what number of predetermined patches to visit maximum potential prey intake.
A few different comparisons could be made concerning landscapes and strategies regarding maximum expected potential prey intake. The two different strategies could be compared for similar landscapes. The choices of prey threshold and predetermined patches within each strategy, and the effects of the two shapes of the gamma distributions describe the prey density distributions. In all cases unimodal peaks in potential prey intake existed. Interestingly, differences existed in the magnitude of the peaks for the two types of gamma functions, negative exponential (ν = 1) and unimodal (ν = 2), even for identical means, ν/α, with landscape of type ν = 1 producing higher peaks of potential expected prey intake amount. This may be related to the negative exponential having a longer tail, which made reaching high density prey densities more likely. Perhaps more puzzling, strategy 1 for the prey density threshold led to systematically higher prey intakes than strategy 2, in which the wading bird used memory, for the same prey density distribution (compare Figures 5A and 10A). We are not sure if this can be called a general conclusion, but it invites some further study.
A number of simplifying assumptions were made that allowed analytic solutions and facilitated interpretation, but which limit the models' applications to real systems. One assumption is that we used the models to determine the maximum possible prey intake. It is not clear that would always be the strategy of a wading bird, which may instead minimize time spent foraging. We believe that the strategies that maximize potential prey intake probably also are similar to ones that minimize time spent foraging, if the wading bird ceases foraging when it reached some value above the minimum needed prey intake, preyneeded. Our Eqs (2.2) and (2.3) would allow that calculation, but it is beyond the scope here due to space and time constraints, so that will be considered in future work. The models also made a number of other simplifying assumptions to keep the analysis within bounds. Some of those can be relaxed in the future, but some, such as ignoring interactions with other wading birds, would require further knowledge. Modifications of our models to study a discrete number of patches rather than analytic distributions will be necessary to apply the models to real systems, such as the Everglades, which will require more information about the actual patch distributions and densities. One additional thing we should note is that our analysis is for the mean expected prey intake. We are considering the visits to patches to be a stochastic process, so every actual foraging scenario would be different and produce results different from the mean. We did not try to incorporate that in our analysis. However, the standard deviation around the mean can be calculated, which we include in Appendix 3 of the Supplementary Information. We have not explored this in detail, but the figures in Appendix 3 show that the variation among scenarios is substantial. This means that wading birds need to have some resilience to fluctuations in energy intake from day to day.
We realize that the theory and modeling of foraging in a heterogeneous environment is an enormous field, which has occupied many ecologists for over fifty years; the papers [13,14,15,16,17,18,19,20] are an arbitrarily chosen assortment that represents only a tiny sampling of paper in the general area. Therefore, the topics of our work have been studied in great detail in many places. However, we have not found any previous papers that are close in enough ways to ours that we could try to make comparisons, so we will not do that here. That attests to the many variations there are on the general problem of foraging in a heterogeneous environment.
We have also not made comparisons with field data to try to test the predictions of the two strategies modeled. Despite that great amount of empirical study on wading birds in the field, we could not find sufficient data on the movements of wading birds among different foraging patches to make comparisons. Wading bird ecologists have certainly assumed that these birds use some sort of sampling strategy. For example, Kushlan [21] wrote; "…wading birds need to sample potential patches to decide where to forage. … The energy penalty for wrong choices may be rather severe, because of the relatively high costs incurred by large birds in moving from place to place. Thus, there is probably considerable pressure for wading birds to sample foraging patches efficiently." Many other observations support the hypothesis that some wading bird species, especially those that require high densities of prey, follow sampling strategies. However, due to the challenges of tracking individual wading birds in the field within a day, not to mention data on the prey densities in the patches that they visit, relevant data for testing our models are difficult to obtain. We know of no information on the pattern of movement of tactile-feeding wading birds among foraging patches such as ponds and depressions where fish and crayfish prey are concentrated. Useful experimental data do exist, however, such as Gawlik's [22] experimental pond studies that provide data on giving-up prey densities of different wading bird species. We hope that our modeling can stimulate studies in natural systems. For example, although it may be difficult to follow individual wading birds around during the course of a day, sufficient numbers of observations at particular foraging sites on how long individual wading birds bird stay can be synthesized in the sense of pattern- oriented modeling to infer such patterns.
We thank Donald Schoolmaster and two anonymous reviewers for useful comments. Any use of trade, firm, or product names is for descriptive purposes only and does not imply endorsement by the U.S. Government.
DLD and SY were supported by Greater Everglades Priority Ecosystem Science program. Any use of trade, firm, or product names is for descriptive purposes only and does not imply endorsement by the U.S. Government.
All authors declare no conflicts of interest in this paper.
Here we show examples using gamma probability distributions for the hypothetical sampling distribution of prey densities among patches.
Based on given distribution function (Eq (1)) and v and α values, we can calculate P and Q.
P=∫∞preythresholdf(preydens)dpreydens=αvΓ(v)∫∞preythresholde−α∗preydens⋅(preydens)v−1dpreydens. |
For the gamma distribution v = 1, since Γ(1)=1, this can be shown to be
P=e−α∗preythreshold |
For v = 2, since Γ(2)=1, this is
P=e−α∗preythreshold+α∗preythresholde−α∗preythreshold |
In both cases, P is equal to 1 when preythreshold=0 and decreases toward zero as preythreshold increases. This decrease is slower for smaller values of α.
Here we present some integrals needed to calculate the mean prey density encountered by the forager over foraging sites (patches) with prey densities in various ranges within the total range of prey densities. The general equation for a conditional mean, where the pdf is f(x)
∫xhighxlowx⋅f(x)dx/∫xhighxlowf(x)dx |
We will evaluate this for the gamma distribution with v = 1 and 2. Note that the expected prey density given that a patch is above the threshold (μabove) is
μabove=P−1∫∞kpreythresholdpreydens⋅f(preydens)dpreydens |
And the expected prey density given that a patch is below the threshold (μbelow) is
μbelow=Q−1∫preythreshold0preydens⋅f(preydens)dpreydens |
v = 1
Expected prey density given that a patch is above the threshold
=P−1∫∞preythreshold f(preydens)preydensdpreydens=αPΓ(1)∫∞preythreshold preydense−α∗(preydens )dpreydens=α[−e−α∗preydens (preydensα+1α2)]∞preythreshold |
Expected prey density given that a patch is below the threshold
=Q−1∫preythreshold0 f(preydens)preydensdpreydens=αQΓ(1)∫preythreshold0 preydense−α∗(preydens )dpreydens=α2Q[−e−α∗preydens (preydensα+1α2 )]preythreshold0. |
Thus the expected prey density given that a patch is above the threshold
μabove=αP(e−α∗preythreshold(preythresholdα+1α2)) |
and the expected prey density given that a patch is below the threshold
μbelow=αQ(1α2−e−α∗preythreshold(preythresholdα+1α2)). |
v = 2
Expected prey density above threshold in the patch
=P−1∫∞preythreshold f(preydens )preydensdpreydens=α2PΓ(2)∫∞preythreshold (preydens )2e−α∗(preydens )dpreydens=α2P[−e−α∗preydens ((preydens )2α+2∗preydensα2+2α3)]∞preythreshold . |
Expected prey density below threshold in the patch
=Q−1∫preythreshold0 f(preydens )preydensdpreydens=α2QΓ(2)∫preythreshold0 (preydens )2e−α∗(preydens )dpreydens=α2Q[−e−α∗preydens ((preydens )2α+2∗preydensα2+2α3)]preythreshold0, |
which becomes the expected prey density above threshold in the patch
μabove=α2P(e−α∗preythreshold((preythreshold )2α+2∗preythresholdα2+2α3 )) |
and the expected prey density below threshold in the patch
μbelow=α2Q(2α3−e−α∗preythreshold((preythreshold )2α+2∗preythresholdα2+2α3 )). |
If we apply result from A1.1. and A1.2 to Eq (2.3) of the main text, we can compute the potential expected prey intake (consumption) by the forager across foraging patches.
Preyintake=Cons⋅[(1−QN)⋅tmin⋅μbelow+(T(1−(1−QN)NP)+tmin(1−QN))⋅μabove] |
For gamma distributions with v = 1 and 2.
v = 1
Expected daily prey intake
Prey intake=Cons⋅((1−QN)tmin⋅αP[1α2−e−α∗preythreshold(preythresholdα+1α2)]+ [T(1−(1−QN)NP)+tmin(1−QN)]⋅αP[e−α∗preythreshold(preythresholdα+1α2)]) |
v = 2
Expected daily prey intake
Preyintake=Cons((1−QN)tmin⋅α2P(2α3−e−α∗preythreshold [(preythreshold )2α+2∗preythresholdα2+2α3]). |
+.(T(1−(1−QN)NP)+tmin(1−QN))⋅α2P(e−α∗preythreshold [(preythreshold )2α+2∗preythresholdα2+2α3])) |
The goal is to calculate the mean intake of prey by a wading bird in a single day by visiting up to N prey patches, which is the maximum. The wading bird samples a patch for time tmin to decide whether or not to stay. If the prey density exceeds a threshold, preythreshold, which is assumed to happen with probability, p, it will stay. Otherwise, it will move to another patch at random, which is assumed to take time ttransition. The probabilities and times associated with the various possibilities are shown in Table 1 from the manuscript.
The chance to find a patch with prey density above the threshold is the sum of all the 'chances to happen' in Table 1;
P+PQ+PQ2+PQ3+PQ4⋯+PQ(N−1)=N∑n=1PQn−1=P(1−QN)1−Q=P(1−QN)P=1−QN. |
The chance that the bird never finds a patch with prey density above the threshold in a day is QN. Therefore, the probability of all cases together is
Chance to fail to find a good patch+Chance to find a good patch=QN+N∑n=1PQn−1=QN+(1−QN)=1 |
Figure 2 in the main text of the manuscript shows a hypothetical distribution of patch densities. The integral over the area to the right of the prey threshold, P, is the probability of a wading bird visiting a patch with prey density above the threshold.
Based on this, we can calculate average above and below threshold
Expected prey density above threshold in the patch=P−1∫∞preythreshold xf(x)dx |
Expected prey density below threshold in the patch=Q−1∫preythreshold0 xf(x)dx |
According to Table1from the manuscript, and the above expected prey densities; we can calculate the expected daily food intake wading birds. The cases refer to those shown in Table 1.
Dailyfoodintake=(Timespentinpatchesbelowthreshold)(Expected prey density of patch below threshold)+(Timespentinthepatchabovethreshold)(Expected prey density of patch above threshold) |
Dailyfoodintakeofcase1=0⋅Q−1∫k0f(x)xdx+{T−(ttransition+tmin)+tmin}⋅P−1∫∞kf(x)xdx≡C1 |
Dailyfoodintakeofcase2=tmin⋅Q−1∫k0f(x)xdx+{T−2(ttransition+tmin)+tmin}⋅P−1∫∞kf(x)xdx≡C2 |
Dailyfoodintakeofcase3=2⋅tmin⋅Q−1∫k0f(x)xdx+{T−3(ttransition+tmin)+tmin}⋅P−1∫∞kf(x)xdx≡C3 |
DailyfoodintakeofcaseN=(N−1)⋅tmin⋅Q−1∫k0f(x)xdx+{T−N(ttransition+tmin)+tmin}⋅P−1∫∞kf(x)xdx≡CN |
Dailyfoodintakeifbirdsfailstofindapatchabovethreshold=Ntmin⋅Q−1∫k0f(x)xdx+0⋅P−1∫∞kf(x)xdx≡Cnone |
Then the expected prey, or food, intake by the wading birds is the sum of the intakes of food for all of the cases, where each is multiplied by the probability of that case occurring;
Averagedailyfoodintake=Cnone(QN)+C1(P)+C2(PQ)+C3(PQ2)+C4(PQ3)⋯+CN(PQN−1)=Cnone(QN)+N∑n=1Cn(PQn−1) |
Based on above equations, we can say in general for food intake for case n ≥ 1.
Cn=(n−1)⋅tmin⋅Q−1∫k0f(x)xdx+{T−n(ttransition+tmin)+tmin}⋅P−1∫∞kf(x)xdx |
Note that it is assumed that the wading bird spends the time tmin that it samples the patch that is above threshold, the remaining time at its disposal during the day, which is T−n(ttransition+tminimum). This can be rewritten as
Cn=(n−1)⋅tmin⋅Q−1∫k0f(x)xdx+{T−n(ttransition+tmin)}⋅P−1∫∞kf(x)xdx+tmin⋅P−1∫∞kf(x)xdx |
Therefore, it is possible to write the food intake that would occur for the nth case as
Cn(PQn−1)=(n−1)⋅P⋅Qn−1⋅tmin⋅Q−1∫k0f(x)xdx+{T−n(ttransition+tmin)}⋅P⋅Q(n−1)⋅P−1∫∞kf(x)xdx+tminPQ(n−1)∗P−1∫∞kf(x)xdx. |
Summing over all N cases, we obtain
∑Nn=1Cn(PQn−1)=∑Nn=1(n−1)P⋅Qn−1tmin⋅Q−1∫k0f(x)xdx+∑Nn=1{T−n(ttransition+tmin)}P⋅Q(n−1)⋅P−1∫∞kf(x)xdx+∑Nn=1tmin⋅P⋅Q(n−1)⋅P−1∫∞kf(x)xdx | (A1) |
The first term on the right-hand side of Eq (A1) can be manipulated as follows,
N∑n=1(n−1)⋅P⋅Qn−1tmin⋅Q−1∫k0f(x)xdx={N∑n=1n⋅P⋅Q(n−1)−N∑n=1PQ(n−1)}tmin⋅Q−1∫k0f(x)xdx. |
We next employ the following identities,
N∑n=1n⋅P⋅Q(n−1)=(1−QN)P−NQN |
N∑n=1PQ(n−1)=(1−QN). |
Therefore, the first term can be rewritten as
N∑n=1(n−1)PQ(n−1)tmin⋅Q−1∫k0f(x)xdx={N∑n=1n⋅PQ(n−1)−N∑n=1PQ(n−1)}tmin⋅Q−1∫k0f(x)xdx={(1−QN)P−NQN−(1−QN)}tmin⋅Q−1∫k0f(x)xdx={(1−QN)(1−P)P−NQN}tmin⋅Q−1∫k0f(x)xdx={Q(1−QN)P−NQN}tmin⋅Q−1∫k0f(x)xdx. |
Using similar operations, the second term of Eq (A1) can be written as,
N∑n=1{T−n(ttransition+tmin)}pq(n−1)∗P−1∫∞kf(x)xdx={TN∑n=1PQ(n−1)−(ttransition+tmin)N∑n=1nPQ(n−1)}⋅P−1∫∞kf(x)xdx={T(1−QN)−(ttransition+tmin)((1−QN)P−NQN)}⋅P−1∫∞kf(x)xdx={T(1−QN)−TN((1−QN)P−NQN)}⋅P−1∫∞kf(x)xdx={T(1−QN)−T((1−QN)NP−QN)}⋅P−1∫∞kf(x)xdx={T−T((1−QN)NP)}⋅P−1∫∞kf(x)xdx=T(1+(QN−1)NP)⋅P−1∫∞kf(x)xdx. |
There is one final term in Equation (1). It can be rewritten as follows;
N∑n=1tminimumPQ(n−1)⋅P−1∫∞kf(x)xdx=tminN∑n=1PQ(n−1)⋅P−1∫∞kf(x)xdx=tmin(1−QN)⋅P−1∫∞kf(x)xdx |
Putting these three terms together, we obtain
N∑n=1Cn(PQ(n−1))=N∑n=1(n−1)PQ(n−1)tmin⋅Q−1∫k0f(x)xdx+N∑n=1{T−n(ttransition+tmin)}PQ(n−1)⋅P−1∫∞kf(x)xdx+N∑n=1tminPQ(n−1)⋅P−1∫∞kf(x)xdx={Q(1−QN)P−NQN}tmin⋅Q−1∫k0f(x)xdx+T(1+(qN−1)Np)⋅P−1∫∞kf(x)xdx+tmin(1−QN)⋅P−1∫∞kf(x)xdx={Q(1−QN)P−NQN}tmin⋅Q−1∫k0f(x)xdx+{T(1−(1−QN)NP)+tmin(1−QN)}⋅P−1∫∞kf(x)xdx |
Therefore, the daily food intake can be written
Averagedailyfoodintake=Cnone(QN)+N∑n=1Cn(PQ(n−1))=QNNtmin⋅Q−1∫k0f(x)xdx+{Q(1−QN)P−NQN}tmin⋅Q−1∫k0f(x)xdx+{T(1+(QN−1)NP)+tmin(1−QN)}⋅P−1∫∞kf(x)xdx={Q(1−QN)P−NQN+NQN}tmin⋅Q−1∫k0f(x)xdx+{T(1+(qN−1)NP)+tmin(1−QN)}⋅P−1∫∞kf(x)xdx={Q(1−QN)P}tmin⋅Q−1∫k0f(x)xdx+{T(1+(QN−1)NP)+tmin(1−QN)}⋅P−1∫∞kf(x)xdx |
Then we multiply this value with Cons, which is parameter of conversion of prey density in a patch to the uptake of prey by the wading bird.
To conclude, we can find an expression for the mean expected daily food intake.
Dailyfoodintake=cons[{(1−QN)P}tmin⋅∫k0f(x)xdx+{T(1+(QN−1)NP)+tmin(1−QN)}⋅P−1∫∞kf(x)xdx] |
The stochastic simulations give reassurance that the analytical solutions for the expected values are valid. But more than that, it points out that, though there is clearly an optimal threshold for switching foraging behavior suggested by the expected prey consumption, the range of prey consumption on any given day can vary widely leading to possibly a great uncertainty in foraging success. In fact, if we look at the coefficient of variation there is a local minimum at a threshold slightly lower than the optimal determined by the mean intake alone, 12.3 g/m2versus 14.4 g/m2 respectively. This raises the question of whether a slightly lower threshold that results in a bit more certainty in the results of foraging efforts is a preferable objective function than just average rate of food consumption. In order to address this question we examine the probability that a bird will meet its minimum daily nutritional requirements.
Following are two sets of figures corresponding to two of the hypothetical patch distributions modeled by Gamma probability density functions with shape parameter ν and rate parameter α.
The top graph in each set is the resulting potential food intake over the course of a day as a function of the threshold value that bird uses to decide when to stop searching and remain in a patch where the food density exceeds the threshold. This graph consists of 3 lines. The middle line is the expected value of the potential food intake for that day. The top and bottom (dotted lines) are the expected values plus or minus one standard deviation. Each of these lines are actually a series of points generated by 10,000 realizations of a stochastic simulation of the search process, incrementing the threshold values each time by 0.3 g/m2. In other words, we start at a threshold near 0 g/m2 run 10,000 realizations of the process, increment the threshold to 0.3 g/m2 run another 10,000 trials then increase the threshold to 0.6 g/m2 and so on. Just adding or subtracting the standard deviation is not entirely accurate since negative food intake values are nonsensical, but we use a Normal distribution approximation to illustrate the overall variation possible in the output of the search procedure.
The second, lower graph in each set is the probability (again based on an assumption of Normality) of the consumer successfully meeting its minimum necessary food intake level for each value of the threshold corresponding to those given on the first graph. We illustrate 7 different minimum necessary food intake level in each set. Higher minimums result in lower probabilities of success and the peak success is closely tied to the optimal threshold values. However, at very low minimum necessary food intake levels the consumer can not only be successful at the optimal threshold but also by setting the threshold arbitrarily high so that it can meet its nutritional requirements by browsing the landscape without consideration of any search criteria.
[1] |
R. Liu, S. A. Gourley, D. L. DeAngelis, J. P. Bryant, Modeling the dynamics of woody plant–herbivore interactions with age-dependent toxicity, J. Math. Biol, 65 (2012), 521–552. https://doi.org/10.1007/s00285-011-0470-0 doi: 10.1007/s00285-011-0470-0
![]() |
[2] |
R. Liu, S. A. Gourley, D. L. DeAngelis, J. P. Bryant, A mathematical model of woody plant chemical defenses and snowshoe hare feeding behavior in boreal forests: the effect of age-dependent toxicity of twig segments, SIAM J. Appl. Math., 73 (2013), 281–304. https://doi.org/10.1137/110848219 doi: 10.1137/110848219
![]() |
[3] |
D. L. DeAngelis, J. P. Bryant, R. Liu, S. A. Gourley, C. J. Krebs, P. B Reichardt, A plant toxin mediated mechanism for the lag in snowshoe hare population recovery following cyclic declines, Oikos, 124 (2015), 796–805. https://doi.org/10.1111/oik.01671 doi: 10.1111/oik.01671
![]() |
[4] |
S. A. Gourley, R. Liu, J. Wu, Spatiotemporal distributions of migratory birds: Patchy models with delay, SIAM J. Appl. Dyn. Syst., 9 (2010), 589–610. https://doi.org/10.1137/090767261 doi: 10.1137/090767261
![]() |
[5] | G. T. Bancroft, A. M. Strong, R. J. Sawicki, W. Hoffman, S. D. Jewell, Relationship among wading bird foraging patterns, colony locations, and hydrology in the Everglades, St. Lucie Press, (1994), 615–665. Available from: https://www.taylorfrancis.com/chapters/edit/10.1201/9781466571754-34. |
[6] | J. C. Ogden, A comparison of wading bird nesting colony dynamics (1931–1946 and 1974–1989) as an indication of ecosystem conditions in the Southern Everglades, St. Lucie Press, (1994), 533–570. Available from: https://www.taylorfrancis.com/chapters/edit/10.1201/9781466571754-34. |
[7] |
E. L. Charnov, Optimal foraging: attack strategy of a mantid, Am. Nat., 110 (1976), 141–151. https://doi.org/10.1086/283054 doi: 10.1086/283054
![]() |
[8] | D. W. Stephens, J. R. Krebs, Foraging theory, Princeton University Press, 2019. https://doi.org/10.1515/9780691206790 |
[9] |
D. L. DeAngelis, S. Yurek, S. Tennenbaum, H. W. Lee, Hierarchical functional response of a forager on a wetland landscape, Front. Ecol. Evol., (2021), 655. https://doi.org/10.3389/fevo.2021.729236 doi: 10.3389/fevo.2021.729236
![]() |
[10] |
E. A. Adams, P. C. Frederick, Effects of methylmercury and spatial complexity on foraging behavior and foraging efficiency in juvenile white ibises (Eudocimus albus), Environ. Toxicol. Chem., 27 (2009), 1708–1712. https://doi.org/10.1897/07-466.1 doi: 10.1897/07-466.1
![]() |
[11] |
J. P. Royston, Algorithm AS 177: Expected normal order statistics (exact and approximate), J. R. Stat. Soc., Ser. C (Appl. Stat.), 31 (1982), 161–165. https://doi.org/10.2307/2347982 doi: 10.2307/2347982
![]() |
[12] |
J. A. Kushlan, Population energetics of the American white ibis, The Auk, 94 (1977), 114–122. https://doi.org/10.1093/auk/94.1.114 doi: 10.1093/auk/94.1.114
![]() |
[13] |
G. Marion, D. L. Swain, M. R. Hutchings, Understanding foraging behaviour in spatially heterogeneous environments, J. Theor. Biol., 232 (2007), 127–142. https://doi.org/10.1016/j.jtbi.2004.08.005 doi: 10.1016/j.jtbi.2004.08.005
![]() |
[14] |
P. Skórka, M. Lenda, R. Martyka, S. Tworek, The use of metapopulation and optimal foraging theories to predict movement and foraging decisions of mobile animals in heterogeneous landscapes, Landscape Ecol., 24 (2009), 599–609. https://doi.org/10.1007/s10980-009-9333-0 doi: 10.1007/s10980-009-9333-0
![]() |
[15] |
S. Focardi, P. Marcellini, P. Montanaro, Do ungulates exhibit a food density threshold? A field study of optimal foraging and movement patterns, J. Anim. Ecol., 65 (1996), 606–620. https://doi.org/10.2307/5740 doi: 10.2307/5740
![]() |
[16] |
H. M. Hagy, R. M. Kaminski, Determination of foraging thresholds and effects of application on energetic carrying capacity for waterfowl, PLoS One, 10 (2015), 0118349. https://doi.org/10.1371/journal.pone.0118349 doi: 10.1371/journal.pone.0118349
![]() |
[17] |
R. Arditi, B. Dacorogna, Optimal foraging on arbitrary food distributions and the definition of habitat patches, Am. Nat., 131 (1988), 837–846. https://doi.org/10.1086/284825 doi: 10.1086/284825
![]() |
[18] |
J. R. Lovvorn, S. E. W. De La Cruz, J. Y. Takekawa, L. E. Shaskey, S. E. Richman, Niche overlap, threshold food densities, and limits to prey depletion for a diving duck assemblage in an estuarine bay, Mar. Ecol.: Prog. Ser., 476 (2013), 251–268. https://doi.org/10.3354/meps10104 doi: 10.3354/meps10104
![]() |
[19] |
D. Boyer, P. D. Walsh, Modelling the mobility of living organisms in heterogeneous landscapes: does memory improve foraging success? Philos. Trans. R. Soc., A, 368 (2010), 5645–5659. https://doi.org/10.1098/rsta.2010.0275 doi: 10.1098/rsta.2010.0275
![]() |
[20] |
A. Kacelnik, C. Bernstein, Optimal foraging and arbitrary food distributions: patch models gain a lease of life, Trends Ecol. Evol., 3 (1988), 252–253. https://doi.org/10.1016/0169-5347(88)90057-2 doi: 10.1016/0169-5347(88)90057-2
![]() |
[21] | J. A. Kushlan, Resource use strategies of wading birds, Wilson Bull., 93 (1981), 145–163. https://sora.unm.edu/node/129818 |
[22] |
D. E. Gawlik, The effects of prey availability on the numerical response of wading birds, Ecol. Monogr., 72 (2002), 329–346. https://doi.org/10.1890/0012-9615(2002)072[0329:TEOPAO]2.0.CO;2 doi: 10.1890/0012-9615(2002)072[0329:TEOPAO]2.0.CO;2
![]() |
1. | Simeon Yurek, Donald L. DeAngelis, Hyo Won Lee, Stephen Tennenbaum, Visualizing wading bird optimal foraging decisions with aggregation behaviors using individual-based modeling, 2024, 493, 03043800, 110702, 10.1016/j.ecolmodel.2024.110702 | |
2. | Gabriela S. P. Arnoso, Henrique C. Delfino, Caio J. Carlos, Foraging Repertoire of American Oystercatcher (Haematopus palliatus) in Two Different Habitats in an Important South American Wetland, 2024, 46, 1524-4695, 10.1675/063.046.0416 | |
3. | Henrique C. Delfino, Caio J. Carlos, Foraging plasticity in Chilean flamingos: influence of water depth, food abundance and intra-flock distances, 2024, 36, 0394-9370, 412, 10.1080/03949370.2024.2307025 |
Success at nth trial | Illustration | Transition time | Time spent in low quality patches | Time spent in the high quality patch | Chance to happen |
1st | ![]() |
ttransition | 0 | T−(ttransition+tmin)+tmin | P |
2nd | ![]() |
2∗ttransition | tmin | T−2(ttransition+tmin)+tmin | P*Q |
3rd | ![]() |
3∗ttransition | 2∗tmin | T−3(ttransition+tmin)+tmin | P*Q*Q |
Nth | ![]() |
N∗ttransition | (N−1)∗tmin | T−N(ttransition+tmin)+tmin=tmin | P*Q(N-1) |
Never find patch>threshold | ![]() |
N∗ttransition | N∗tmin | T−N(ttransition+tmin)=0 | QN |
Parameter | Definition | Value |
tmin | Minimum time needed to estimate prey density | 20, 40 minutes |
ttransition | Time needed to travel between patches | 20, 40 minutes |
treturn | Time needed to return to the best patch | 20, 40 minutes |
T | Total time available for foraging in a day | 600 minutes |
Cons | Rate at which encountered prey are consumed | 0.175 g minutes-1 |
N | T/(tmin+ttransition) = Maximum numbers of patches wading birds can visit | 15 ponds |
preyneeded | Prey intake needed during day to feed self and offspring | |
preythreshold | Threshold quality during the searching | |
v, α | Parameters from gamma distribution | (1, 0.16), (1, 0.24), (2, 0.32), (2, 0.48) |
Success at nth trial | Illustration | Transition time | Time spent in low quality patches | Time spent in the high quality patch | Chance to happen |
1st | ![]() |
ttransition | 0 | T−(ttransition+tmin)+tmin | P |
2nd | ![]() |
2∗ttransition | tmin | T−2(ttransition+tmin)+tmin | P*Q |
3rd | ![]() |
3∗ttransition | 2∗tmin | T−3(ttransition+tmin)+tmin | P*Q*Q |
Nth | ![]() |
N∗ttransition | (N−1)∗tmin | T−N(ttransition+tmin)+tmin=tmin | P*Q(N-1) |
Never find patch>threshold | ![]() |
N∗ttransition | N∗tmin | T−N(ttransition+tmin)=0 | QN |
Parameter | Definition | Value |
tmin | Minimum time needed to estimate prey density | 20, 40 minutes |
ttransition | Time needed to travel between patches | 20, 40 minutes |
treturn | Time needed to return to the best patch | 20, 40 minutes |
T | Total time available for foraging in a day | 600 minutes |
Cons | Rate at which encountered prey are consumed | 0.175 g minutes-1 |
N | T/(tmin+ttransition) = Maximum numbers of patches wading birds can visit | 15 ponds |
preyneeded | Prey intake needed during day to feed self and offspring | |
preythreshold | Threshold quality during the searching | |
v, α | Parameters from gamma distribution | (1, 0.16), (1, 0.24), (2, 0.32), (2, 0.48) |