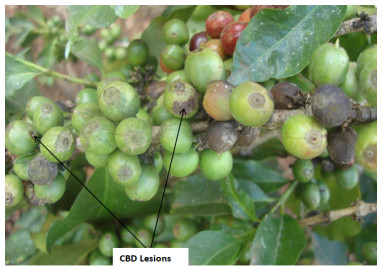
This paper focuses on a mathematical model for coffee berry disease infestation dynamics. This model considers coffee berry and vector populations with the interaction of fungal pathogens. In order to gain an insight into the global dynamics of coffee berry disease transmission and eradication on any given coffee farm, the assumption of logistic growth with a carrying capacity reflects the fact that the amount of coffee plants depends on the limited size of the coffee farm. First, we show that all solutions of the chosen model are bounded and non-negative with positive initial data in a feasible region. Subsequently, endemic and disease-free equilibrium points are calculated. The basic reproduction number with respect to the coffee berry disease-free equilibrium point is derived using a next generation matrix approach. Furthermore, the local stability of the equilibria is established based on the Jacobian matrix and Routh Hurwitz criteria. The global stability of the equilibria is also proved by using the Lyapunov function. Moreover, bifurcation analysis is proved by the center manifold theory. The sensitivity indices for the basic reproduction number with respect to the main parameters are determined. Finally, the numerical simulations show the agreement with the analytical results of the model analysis.
Citation: Abdisa Shiferaw Melese, Oluwole Daniel Makinde, Legesse Lemecha Obsu. Mathematical modelling and analysis of coffee berry disease dynamics on a coffee farm[J]. Mathematical Biosciences and Engineering, 2022, 19(7): 7349-7373. doi: 10.3934/mbe.2022347
[1] | Ana I. Muñoz, José Ignacio Tello . Mathematical analysis and numerical simulation of a model of morphogenesis. Mathematical Biosciences and Engineering, 2011, 8(4): 1035-1059. doi: 10.3934/mbe.2011.8.1035 |
[2] | Boumediene Benyahia, Tewfik Sari . Effect of a new variable integration on steady states of a two-step Anaerobic Digestion Model. Mathematical Biosciences and Engineering, 2020, 17(5): 5504-5533. doi: 10.3934/mbe.2020296 |
[3] | Noura Laksaci, Ahmed Boudaoui, Seham Mahyoub Al-Mekhlafi, Abdon Atangana . Mathematical analysis and numerical simulation for fractal-fractional cancer model. Mathematical Biosciences and Engineering, 2023, 20(10): 18083-18103. doi: 10.3934/mbe.2023803 |
[4] | Maryam Al-Yahyai, Fatma Al-Musalhi, Ibrahim Elmojtaba, Nasser Al-Salti . Mathematical analysis of a COVID-19 model with different types of quarantine and isolation. Mathematical Biosciences and Engineering, 2023, 20(1): 1344-1375. doi: 10.3934/mbe.2023061 |
[5] | Hongfan Lu, Yuting Ding, Silin Gong, Shishi Wang . Mathematical modeling and dynamic analysis of SIQR model with delay for pandemic COVID-19. Mathematical Biosciences and Engineering, 2021, 18(4): 3197-3214. doi: 10.3934/mbe.2021159 |
[6] | Darja Kalajdzievska, Michael Yi Li . Modeling the effects of carriers on transmission dynamics of infectious diseases. Mathematical Biosciences and Engineering, 2011, 8(3): 711-722. doi: 10.3934/mbe.2011.8.711 |
[7] | A. K. Misra, Jyoti Maurya, Mohammad Sajid . Modeling the effect of time delay in the increment of number of hospital beds to control an infectious disease. Mathematical Biosciences and Engineering, 2022, 19(11): 11628-11656. doi: 10.3934/mbe.2022541 |
[8] | Jinhu Xu, Yicang Zhou . Bifurcation analysis of HIV-1 infection model with cell-to-cell transmission and immune response delay. Mathematical Biosciences and Engineering, 2016, 13(2): 343-367. doi: 10.3934/mbe.2015006 |
[9] | Dwi Lestari, Noorma Yulia Megawati, Nanang Susyanto, Fajar Adi-Kusumo . Qualitative behaviour of a stochastic hepatitis C epidemic model in cellular level. Mathematical Biosciences and Engineering, 2022, 19(2): 1515-1535. doi: 10.3934/mbe.2022070 |
[10] | Bruno Buonomo, Deborah Lacitignola . On the stabilizing effect of cannibalism in stage-structured population models. Mathematical Biosciences and Engineering, 2006, 3(4): 717-731. doi: 10.3934/mbe.2006.3.717 |
This paper focuses on a mathematical model for coffee berry disease infestation dynamics. This model considers coffee berry and vector populations with the interaction of fungal pathogens. In order to gain an insight into the global dynamics of coffee berry disease transmission and eradication on any given coffee farm, the assumption of logistic growth with a carrying capacity reflects the fact that the amount of coffee plants depends on the limited size of the coffee farm. First, we show that all solutions of the chosen model are bounded and non-negative with positive initial data in a feasible region. Subsequently, endemic and disease-free equilibrium points are calculated. The basic reproduction number with respect to the coffee berry disease-free equilibrium point is derived using a next generation matrix approach. Furthermore, the local stability of the equilibria is established based on the Jacobian matrix and Routh Hurwitz criteria. The global stability of the equilibria is also proved by using the Lyapunov function. Moreover, bifurcation analysis is proved by the center manifold theory. The sensitivity indices for the basic reproduction number with respect to the main parameters are determined. Finally, the numerical simulations show the agreement with the analytical results of the model analysis.
Coffee is a plantation crop that is well adapted to different eco-physiological conditions of tropical and subtropical highlands. It is the favourite beverage and second most traded commodity next to crude oil in the world [1]. The coffee industry is estimated to be over fanxiexian_myfh100 billion worldwide with an average consumption of 500 billion cups per year. It is mostly consumed in the developed nations and produced by tropical countries, often less developed, being a big source of their economy [1,2]. However, coffee production has been challenged by diseases, weeds and pests. For instance, coffee berry disease (CBD) caused by Colletotrichum kahawae is a major challenge to Arabica coffee production in African countries [3]. It is a fungal plant pathogen [4,5]. The symptoms of CBD include black depressed wounds on coffee berries (see Figure 1) [6].
The first report of CBD (Colletotrichum kahawae) dates back to 1922 in western Kenya [7]. Soon after, the fungus quickly spread throughout most of the African countries (see Figure 2) [8]. The disease attacks flowers and fruits at all stages of growth, but it is more destructive to young berries especially during the expanding period 4–l6 weeks after flowering [9]. This disease decreases both the quality and yield of coffee berries. For instance, Ethiopia, Kenya and Cameroon annually lose up to 29.9, 75 and 60%, respectively. Losses may reach up to 100% [2,10] in high rainfall, humidity and altitude areas.
Because CBD can become very severe and there is a lack of effective control measures, there is much concern that the fungus could spread to coffee-growing areas on other continents. Currently, however, the disease is only prevalent in Africa at high altitudes with relatively high humidity [11]. There are various applications for CBD management such as chemical treatments, cultural practices, the use of resistant varieties and biological control [12,13,14,15,16,17,18]. Several chemical products (fungicides) have been evaluated and recommended for CBD control measures such as different copper fungicides, organic fungicides and mixtures of the two. For instance, fungicide spray against CBD starting six weeks after the main flowering for six rounds at a four-week interval during a crop season was recommended in Ethiopia. Cultural practices include a variety of management tactics, such as mixed cropping with shade plants, pruning infected branches, the destruction of infected material and the removal of mummified berries to create environmental conditions that limit CBD development [14]. Chemical control seems the most effective method but if incorrectly used, it causes ecological risks. On the contrary, the use of resistant varieties and cultural practices is cost-effective, biologically safe and environmentally friendly.
Mathematics is becoming an important tool for studying the evolution of plant pests and diseases. Some mathematical models have been formulated and analyzed to explain the dynamics of plant disease transmission and assess their controls. For example, Fotsa et al. [19] introduced the mathematical modelling and optimal control of Anthracnose disease. Anthracnose attacks a wide range of commercial crops, including coffee, mango, banana, blueberry, cherry, and strawberry. Cunniffe and Gilligan [20] investigated epidemiological models for plant pathogens. These models ensured maximum transferability across the widest range of host-pathogen systems by using the common currency of the field; they illustrated the results in a class of model that has been experimentally tested for plant disease. Fotso et al. [21] introduced and analyzed a mathematical model of coffee berry borer with optimal control strategies. Among coffee diseases, CBD, coffee wilt disease, and coffee leaf rust are the most destructive diseases threatening coffee production in developing countries like Ethiopia. However, according to the survey results, mostly CBD was more widespread than the other coffee diseases [22]. In all the previous studies, the mathematical model for CBD epidemics concerning fungal pathogen and vector population was not considered. In the present work, we developed a nonlinear deterministic mathematical model for the dynamics of CBD infestation on a coffee farm; and also we also applied their qualitative analysis.
The reproduction of the coffee tree involves flowers containing both females and males and their pollination occurs mainly by wind and to a lesser extent by insects [23]. CBD is locally spread between coffee trees and branches by wind and rain [11]. But, the common vectors of long- and medium-distance CBD dispersal are insects, birds, and coffee harvesters [6]. Hence, we assumed those common vectors that, after contacting a fungal pathogen from the environment or already CBD infected coffee berries, then contact healthy coffee berries as responsible vectors for the spread of CBD. The fungal pathogen in the environment may be transported to the coffee plant via infected vectors; once the coffee berry becomes infected, the fungus increases within the infected coffee and destroys it. Twig bark, flower cushions, and mummified berries are considered to be sources of primary inoculum [24]. Most diseased berries drop prematurely, but those that stick to the branches are the main sources of the secondary inoculum (new Colletotrichum kahawae) conidia that are dispersed to contaminate other healthy berries.
The CBD is highly dependent upon climatic factors: humidity, rainfall, and temperature [25,26,27]. These climatic conditions conducive to Colletotrichum kahawae typically occur at high elevations greater than 1600 m (i.e., the altitude at which coffee Arabica is grown); disease incidence is minimal below 1000 m [24]. The optimal temperatures for conidium germination and mycelium growth are in the range of 20 to 22℃ for CBD [28]. An increase of 0.61℃ in the global mean temperature has been recorded since the beginning of the twentieth century and the predicted warming of 2–6℃ by 2100 have direly increased the need to understand the impacts of climate change [29]. Most insects in temperate climates have optimum development at temperatures between 20 and 35℃. The total development time from egg to adult was 89.6 days at 20℃, 63.10 days at 25℃ and 55.81 days at 30℃, and no development at 35℃ [30]. Whereas at temperatures below 15℃ mating is limited, and movement such as flying becomes stalled [31]. Most conidia of Colletotrichum kahawae can be effectively dispersed by an optimum rainfall of 10 mm but heavy rainfall leads more to their leaching from the coffee tree canopy to the soil [32,33].
This study considers coffee berry and vector populations with the interaction of a fungal pathogen (B). The coffee berry population is considered into two forms: the susceptible coffee berry (Sc) and infected coffee berry (Ic). Due to the limited size of a coffee plantation, we adopted a logistic growth function for the biomass density of coffee berries. The coffee berry population grows logistically [34] at a growth rate r and an environmental carrying capacity K. Susceptible coffee berries move to the infected class following contacts with an infected vector at a per capita rate β2; its mortality rate is θ. The infected coffee berry's death rate is γ due to the disease. The coffee berry, once becoming infected, never recovers, and gives no or very low yield of coffee. The vector population is divided into two form: the susceptible vector (Sv) and infected vector (Iv). The infected vectors (insect, birds) are assumed to transport the fungal pathogen to the coffee berry. The vectors can be infected from the fungal pathogen in the environment at rate β1 or from the infected coffee berry at rate β3. The susceptible vector is recruited at rate λ. The vectors' death rate is represented by δ. The fungal pathogens in the environment may be transported to the coffee plant via the infected vectors, once the coffee berry becomes infected, the fungal increases within the infected coffee and destroy it. This invariably adds to the fungal pathogens in the environment at rate η and the infected coffee berry fungal pathogen contribution to the environment is (ηIc). The fungal pathogen's decay rate is m. The flow diagram of CBD transmission is illustrated in Figure 3. For further information, the parameters and their biological meanings are given in Table 1.
Parameter | Description | Unit | Value | Source |
β1 | Contact rate of vector with pathogen environment | day−1 | 0.000209818 | Estimated |
β2 | Contact rate of coffee berries with infected vector | day−1 | 0.000795455 | Estimated |
β3 | Contact rate of vector with infected coffee berries | day−1 | 0.000149091 | Estimated |
θ | Mortality rate of healthy coffee berries | day−1 | 0.01 | [35] |
γ | Removal rate of infected coffee berries | day−1 | 0.005 | Estimated |
r | Growth rate of new coffee berries | day−1 | 0.12 | [36] |
K | Carrying capacity | m−2 | 150 | Estimated |
η | Induced rate of infected coffee berries | ℧ | 0.009 | Estimated |
λ | Recruitment rate of vector | day−1 | 0.488364 | Estimated |
m | Decay rate of pathogen | day−1 | 0.0900982 | Estimated |
δ | Death rate of vector | day−1 | 0.009 | Estimated |
The governing mathematical model is given by
{dScdt=rSc(1−Sc+IcK)−β2ScIv−θSc,dIcdt=β2ScIv−(γ+η)Ic,dSvdt=λ−(β1B+β3Ic)Sv−δSv,dIvdt=(β1B+β3Ic)Sv−δIv,dBdt=ηIc−mB. | (2.1) |
Together with initial condition:
Sc(0)=Sc0>0,Ic(0)=Ic0≥0,Sv(0)=Sv0>0,Iv(0)=Iv0≥0,B(0)=B0≥0. | (2.2) |
Note that in the Table 1, the unit of η is to be Cells(ml−1)(Individual−1)(day−1) = ℧.
In this section, we need to prove that the solutions of system (2.1) are nonnegative for all t≥0. This will be stated as follows.
Theorem 1. Every solution of system (2.1) with initial condition (2.2) will remain positive in R5+ for all t>0.
Proof. From the system (2.1), we obtain
dScdt|[Sc=0]≥0,dIcdt|[Ic=0]=β2ScIv≥0,dSvdt|[Sv=0]=λ>0,dIvdt|[Iv=0]=(β1B+β3Ic)Sv≥0,dBdt|[B=0]=ηIc≥0. |
This implies that all the solutions with positive initial data remains nonnegative in R5+ for all t≥0. This means, the region attracts all solutions of the governing system (2.1).
Moreover, it is easy to verify that there exist an invariant region Ω where a solution set for the system (2.1) is bounded.
Theorem 2. Every solution of system (2.1) initiating in R5+ is uniformly bounded in the region Ω=Ωc×Ωv×Ωp, where
Ωc={(Sc,Ic)∈R2+:Nc≤M(1+r)h},Ωv={(Sv,Iv)∈R2+:Nv≤λδ},Ωp={B∈R+:0≤B≤M(1+r)ηmh}, |
with M=max{Sc(0),K},h=min{1,γ+η}.
Proof. Let Nc(t)=Sc(t)+Ic(t) be the total population of coffee berry. Then differentiating Nc(t) with respect to time t and adding the first two equations of system (2.1), we get
dNcdt≤rSc−(γ+η)Ic=(1+r)Sc−Sc−(γ+η)Ic. | (3.1) |
The last inequality in Eq (3.1) can be rewritten as
dNcdt+hNc≤M(1+r). | (3.2) |
Integrating Eq (3.2) by separation of variables and then as t → ∞, the feasible region of the system (2.1) for coffee population is given by
Ωc={(Sc,Ic)∈R2+:Nc≤M(1+r)h}. |
We also consider the total population of vector as Nv(t)=Sv(t)+Iv(t). Then the derivative of Nv(t) with respect to t is given by
dNvdt=λ−δNv. | (3.3) |
By solving Eq (3.3) and then as t→∞, we get the feasible region of the system (2.1) for vector population:
Ωv={(Sv,Iv)∈R2+:Nv≤λδ}. | (3.4) |
From the fifth equation of system (2.1) and by the comparison, we have
dBdt=ηIc−mB≤η(Sc+Ic)−mB≤M(1+r)ηh−mB. | (3.5) |
Solving the last inequality of Eq (3.5) and t→∞ results 0≤B(t)≤M(1+r)η/mh. Consequently, the feasible region of the system (2.1) is given by Ω=Ωc×Ωv×Ωp is positively invariant.
Hence, the solutions of the model are bounded. Therefore, the model is suitable to conduct the study, and the analysis of the system dynamics can be considered in the region Ω.
In this section, we provide the following results which guarantee that the CBD model governed by system (2.1) is epidemiologically and mathematically well posed.
Theorem 3. (Existence-uniqueness of solution). Let Θ be the domain:
|t−t0|≤a,‖x−x0‖≤b,x=(x1,x2,...,xn),x0=(x10,x20,...,xn0) | (3.6) |
and suppose that f(t,x) satisfies the Lipschitz condition:
‖f(t,x1)−f(t,x2)‖≤κ‖x1−x2‖, | (3.7) |
where (t,x1), (t,x2) ∈Θ, and κ>0. Then, there exist a constant ν>0 such that there exists a unique continuous vector solution x(t) of the system (2.1) in the interval |t−t0|≤ν. From Eq (3.7), f is satisfied by requirement that ∂fi/∂xj,i,j=1,2,...,5 be continuous and bounded in Θ.
If f(t,x) has continuous partial derivative ∂fi/∂xj on a bounded closed convex domain R, then it satisfies a Lipschitz condition in R∈(0,∞). Our concern is in the domain:
1≤ϵ≤R. | (3.8) |
Let Θ denote the region defined in Eq (3.6) such that Eqs (3.7) and (3.8) hold. Then, there exist a solution of system (2.1) which is bounded in Θ.
Proof. {Suppose that the right side of system (2.1) is re-written as:
f1=rSc(1−Sc+IcK)−β2ScIv−θSc, | (3.9) |
f2=β2ScIv−(γ+η)Ic, | (3.10) |
f3=λ−(β1B+β3Ic)Sv−δSv, | (3.11) |
f4=(β1B+β3Ic)Sv−δIv, | (3.12) |
f5=ηIc−mB. | (3.13) |
Since the functions in Eqs (3.9)–(3.13) are polynomial, then they are infinitely differentiable. Thus, for the detailed proof, we can follow the proof of classical Cauchy-Lypschitz theorem [43]. Therefore, all the partial derivatives exist and are finite, then there exists a solution for the model and hence, we can say that there exist a unique solution of system (2.1) in the domain Θ.
At DFE, there is no infections (i.e., Ic=Iv=B=0) in the populations. To find DFE, we equate the right hand side of system (2.1) to zero and solving for the noninfected state variables we get Sc=0 or Sc=K−Kθ/r,Sv=λ/δ. Thus, the equilibrium points of the system (2.1) are (0,0,λ/δ,0,0) and (K(r−θ)/r,0,λ/δ,0,0). Hence, the DFE is given by
E0=(K(r−θ)r,0,λδ,0,0). | (3.14) |
Remark 1. The disease-free equilibrium poin E0 in Eq (3.14) is biologically feasible when r>θ.
The basic reproduction number is the number of secondary infections that one infectious individual would create over the duration of the infectious period [37]. The expression of R0 for the system (2.1) can be derived using the next generation matrix method [38]. The first step to find R0 is rewriting the model equations starting with newly infective classes:
{dIcdt=β2ScIv−(γ+η)Ic,dIvdt=(β1B+β3Ic)Sv−δIv,dBdt=ηIc−mB. | (3.15) |
The right hand side of system (3.15) is written as f−g, where
f=[β2ScIv(β1B+β3Ic)Sv0],g=[(γ+η)IcδIv−ηIc+mB]. | (3.16) |
Then by linearization approach, the associated matrices of f and g at E0 are given by
F=[0K(r−θ)β2r0β3λδ0β1λδ000],G=[γ+η000δ0−η0m]. |
The basic reproduction number, R0=ρ(FG−1) is the spectral radius of the product FG−1. Thus, it is given by
R0=√Kβ2λ[mβ3+β1η](r−θ)rmδ2(γ+η). | (3.17) |
In this section, we shall investigate the local stability of disease-free equilibrium point based on the basic reproduction number, R0.
Theorem 4. If R0<1, then E0 of the system (2.1) is locally asymptotically stable in Ω.
Proof. The Jacobian matrix of the system (2.1) at E0 is given by
J(E0)=[θ−r−(r−θ)0−β2K(r−θ)r00−γ−η0β2K(r−θ)r00−β3λδ−δ0−β1λδ0β3λδ0−δβ1λδ0η00−m]. | (3.18) |
From the Jacobian matrix (3.18), we obtain characteristic polynomial:
(δ+Λ)(r−θ+Λ)(Λ3+A2Λ2+A1Λ+A0)=0. | (3.19) |
From Eq (3.19), we obtain that Λ1=−δ<0, Λ2=−(r−θ)<0 (see Remark 1, r>θ) and
Λ3+A2Λ2+A1Λ+A0=0, | (3.20) |
where
A2=γ+η+m+δ,A1=m(δ+γ+η)+δ(γ+η)ηβ1mβ3+ηβ1+δ(γ+η)mβ3mβ3+ηβ1(1−R20),A0=δ(γ+η)m(1−R20). |
Using Routh-Hurwitz criteria [38], Eq (3.20) has eigenvalues which are negative real part whenever A0>0, A1>0, A2>0, A1A2−A0>0 and A0(A1A2−A0)>0. Therefore, E0 is locally asymptotically stable for R0<1.
Theorem 5. If R0<1, then E0 of the system (2.1) is globally asymptotically stable in Ω.
Proof. To proof this theorem, we first define the linear Lyapunov function:
U=ϵ1Ic+ϵ2Iv+ϵ3B, |
where ϵ1,ϵ2 and ϵ3 are positive constants to be computed.
Then differentiating U with respect to t, we obtain
dUdt=(−ϵ1(γ+η)+ϵ2β3S0v+ϵ3η)Ic+(ϵ1β2S0c−ϵ3δ)Iv+(ϵ2β1S0v−ϵ3m)B, | (3.21) |
with S0c=K(r−θ)/r and S0v=λ/δ. The ϵ1,ϵ2,ϵ3 can be chosen such that
−ϵ1(γ+η)+ϵ2β3S0v+ϵ3η=0,ϵ1β2S0c−ϵ3δ=0,ϵ2β1S0v−ϵ3m=0. | (3.22) |
If we choose ϵ1=1, then ϵ2=β2K(r−θ)/rδ and ϵ3=β1β2K(r−θ)λ/rδ2m. Substituting ϵ1,ϵ2 and ϵ3 into Eq (3.21) leads to
dUdt=(−ϵ1(γ+η)+ϵ2β3λδ+ϵ3η)Ic+(β2K(r−θ)r−β2K(r−θ)r)Iv+(β1β2K(r−θ)λrδ2−mβ1β2K(r−θ)λrδ2m)B,=(γ+η)(R20−1)Ic. | (3.23) |
Since R0<1 and R0≥0 imply that R20<1, we have dU/dt≤0.
Moreover, the largest compact invariant set in {(Sc,Ic,Sv,Iv,B)∈Ω:dU/dt=0} is the singleton {E0}. By LaSalle [39], it then implies that E0 is globally asymptotically stable in Ω.
Endemic equilibrium point (E∗) exists when CBD persist in the populations. To obtain EEP, we set each right hand equation in the system (2.1) equal to zero. That is,
rS∗c(1−S∗c+I∗cK)−β2S∗cI∗v−θS∗c=0,β2S∗cI∗v−(γ+η)I∗c=0,λ−(β1B∗+β3I∗c)S∗v−δS∗v=0,(β1B∗+β3I∗c)S∗v−δI∗v=0,ηI∗c−mB∗=0. | (3.24) |
From the first equation of Eq (3.24) we find S∗c=0 or r(1−(S∗c+I∗c)/K)−β2I∗v−θ=0.
Case i: S∗c=0, we obtain I∗c=δm/(β1η+mβ3),S∗v=λ/2δ,I∗v=λ/2δ, B∗=ηδ/(β1η+mβ3). This endemic point is a point where the disease kills all coffee berries.
Case ii: r(1−(S∗c+I∗c)/K)−β2I∗v−θ=0, then we get S∗c,S∗v,I∗v and B∗:
S∗c=δ(γ+η)(m+(β1η+mβ3)I∗c)λβ2(β1η+mβ3),S∗v=λmδ(m+(β1η+β3m)I∗c),I∗v=λ(β1η+mβ3)I∗cδ(m+(β1η+mβ3)I∗c),B∗=ηI∗cm, | (3.25) |
where I∗c is the positive root of
f(I∗c)=A(I∗c)2+BI∗c+C=0, | (3.26) |
with
A=r3m2δ5(δ(γ+η)+β2λ)(γ+η)2R40K2β22λ2(r−θ)2>0,B=r2m2δ3(γ+η)Kβ2λ(r−θ)[β2λ+2δ(γ+η)+δ(β2λ−rδ)(γ+η)R20r−θ]R20,C=rδ2m2(γ+η)[r−θ−rδR20r−θ]. |
The feasibility of the endemic equilibrium is determined by the next proposition.
Proposition 1. (Feasibility of the endemic equilibrium).
Let E∗=(S∗c,I∗c,S∗v,I∗v,B∗) be as Eq (3.25).
(i) If B>0 and C<0 or B<0 and C<0, then there is a unique endemic equilibrium E∗.
(ii) If B<0 or C>0, then there exist two endemic equilibrium E∗ of the system.
Proof. The result follows Descartes' rule of signs to f(I∗c) given in Eq (3.26).
Theorem 6. If R0>1, Bi>0,i=1,2,...,5, B1B2−B3>0,B1(B2B3+B5)−B23−B21B4>0,(B2B3 −B1(B22−2B4))B5−B24(−B1B2B3+B23+B21B4)− B25>0,B5(−B4(−B1B2B3+ B23+B21B4)+(B2B3−B1(B22−2B4))B5−B25)>0, then E∗ of system (2.1) is locally asymptotically stable in Ω.
Proof. The Jacobian matrix of system (2.1) at E∗ is given by
J(E∗)=[r−r(I∗c+2S∗c)K−β2I∗v−θ−(r−θ)0−β2S∗c0β2I∗v−γ−η0β2S∗c00−β3S∗v−β1B∗−β3I∗c−δ0−β1S∗v0β3S∗vβ1B∗+β3I∗c−δβ1S∗v0η00−m] | (3.27) |
The eigenvalues of matrix (3.27) are computed from
|J(E∗)−ΛI5|=0 | (3.28) |
Let us consider the non-zero entities of matrix (3.28) as:
b11=−θ−r(I∗c+2S∗c)/K−β2I∗v+r,b12=−(r−θ),b21=β2I∗v,b22=−γ−η,b32=−β3S∗v,b42=β3S∗v,b52=η,b33=−β1B∗−β3I∗c−δ, b43=β1B∗+β3I∗c,b14=−β2S∗c,b24=β2S∗c,b44=−δ,b35=−β1S∗v,b45=β1S∗v,b55=−m.
Then, the characteristic polynomial of Eq (3.28) is given by
Λ5+B1Λ4+B2Λ3+B3Λ2+B4Λ+B5=0, | (3.29) |
where
B1=−(b11+b22+b33+b44+b55),B2=b33b44+b22(b33+b44+b55)+(b33+b44)b55+b11(b33+b44+b55)+b24b42(b11−b14)−b11((b44+b55)b33+b22+b44b55),B3=b24b33b42−b14b21b42−b24b32(b43−b24b45b52−b22b44b55−(b44+b55)b22b33+(b24b42−b44)b55+b11b24b42−(b44+b55)b11b33−b11b22−b11b44b55,B4=b14b21(b33b42−b32b43−b45b52)+b24(b33b45−b35b43)b52+(b22b33b44+b24b32b43+b14b21b42)b55+b11b24(b32b43+b45b52−b33b42)+b11b33b44b55+b11b22(b33+b44)−b11b24b42b55,B5=b14b21((b33b45−b35b43)b52+(b32b43−b42)b55+b11b24((b35(b43−b33b45)b52+(b33b42−b32b43)b55)−b11b22b33b44. |
Using the Routh Hurwitz criterion [39], the endemic equilibrium E∗ is locally asymptotically stable for R0>1 if Bi>0,i=1,2,...,5 and
B1B2−B3>0,B1(B2B3+B5)−B23−B21B4>0,(B2B3−B1(B22−2B4))B5−B24(−B1B2B3+B23+B21B4)−B25>0,B5(−B4(−B1B2B3+B23+B21B4)+(B2B3−B1(B22−2B4))B5−B25)>0. |
Hence, all eigenvalues of Eq (3.29) evaluated at E∗ have negative real parts whenever R0>1 and the equilibrium E∗ is locally asymptotically stable.
Theorem 7. If R0>1, then E∗ of the model (2.1) is globally asymptotically stable in Ω.
Proof. Let us consider a Volterra-type Lyapunov function:
V=ζ1(Sc−S∗clnSc)+ζ2(Sc−S∗clnSc)+ζ3(Sv−S∗vlnSv)+ζ4(Ic−I∗clnIc)+ζ5(B−B∗lnB), | (3.30) |
where ζ1,ζ2,ζ3,ζ4 and ζ5 are non-negative constants. Then by taking the derivative of V with respect to t, we obtain
dVdt=ζ1(1−S∗cSc)dScdt+ζ2(1−I∗cIc)dIcdt+ζ3(1−S∗vSv)dSvdt+ζ4(1−I∗vIv)dIvdt+ζ5(1−B∗B)dBdt,=ζ1Sc(1−S∗cSc)(r−rScK−rIcK−β2Iv−θ)+ζ2(1−I∗cIc)(β2ScIv−(γ+η)Ic)+ζ3(1−S∗vSv)(λ−(β1B+β3Ic+δ)Sv)+ζ4(1−I∗vIv)((β1B+β3Ic)Sv−δIv)+ζ5(1−B∗B)(ηIc−mB). | (3.31) |
Since E∗ is an equilibrium point, Eq (3.24) can be rewritten as:
r−rS∗cK−rI∗cK−β2I∗v=θ,β2S∗cI∗v=(γ+η)I∗c,λ=(β1B∗+β3I∗c+δ)S∗v,(β1B∗+β3I∗c)S∗v=δI∗v,ηI∗c=mB∗. | (3.32) |
Substituting the relations in Eq (3.32) into Eq (3.31), we obtain
dVdt=ζ1Sc(Sc−S∗cSc)(−rScK−rIcK−β2Iv+rS∗cK+rI∗cK+β2I∗v)+ζ2(1−I∗cIc)(β2ScIv−β2S∗cI∗vIcI∗c)+ζ3(1−S∗vSv)((β1B∗+β3I∗c+δ)S∗v−(β1B+β3Ic+δ)Sv)+ζ4(1−I∗vIv)((β1B+β3Ic)Sv−(β1B∗+β3I∗c)S∗vIvI∗v)+ζ5(1−B∗B)(ηIc−ηI∗cBB∗). | (3.33) |
Expanding and grouping Eq (3.33), we have
dVdt=−ζ1rK(sc−S∗c)2+[ζ1rS∗cK−ζ2β2S∗cI∗vI∗c+ζ3β3S∗v+ζ5η]Ic+[ζ1β2S∗c−ζ4(β1B∗+β3I∗c)S∗vI∗v]Iv+[ζ3β1S∗v−ζ5ηI∗cB∗]B+ζ1(rI∗cK+β2I∗v)Sc−ζ1rIcScK−ζ1rI∗cS∗cK+(−ζ1+ζ2)β2ScIv+(−ζ1+ζ2)β2S∗cI∗v−ζ2β2I∗cScIvIc+(−ζ3+ζ4)β1BSv+(−ζ3+ζ4)β3IcSv+2ζ3δS∗v−ζ3δSv−ζ3(β1B∗+β3I∗c+δ)(S∗v)2Sv+(ζ3+ζ4)(β1B∗+β3I∗c)S∗v−ζ4β1B∗−ζ5ηB∗IcB+ζ5ηI∗c. | (3.34) |
Choose ζ1,ζ2,ζ3,ζ4 and ζ5 such that the expressions in the brackets in Eq (3.34) vanish. That is
ζ1rS∗cK−ζ2β2S∗cI∗vI∗c+ζ3β3S∗v+ζ5η=0,ζ1β2S∗c−ζ4(β1B∗+β3I∗c)S∗vI∗v=0,ζ3β1S∗v−ζ5ηI∗cB∗=0. | (3.35) |
Fixing ζ1=δ, then Eq (3.35) leads to
ζ2=ζ1,ζ3=ζ1ηI∗cS∗c(β1+β3m)S∗vB∗(β2I∗vI∗c−rK),ζ4=ζ1β2S∗cδ,ζ5=ζ1β1S∗cβ1+β3m(β2I∗vI∗c−rK). | (3.36) |
Replacing Eq (3.36) in Eq (3.34), we obtain
dVdt=−ζ1rK(sc−S∗c)2+ζ1(rI∗cK+β2I∗v)Sc−ζ1rIcScK−ζ1rI∗cS∗cK−ζ1β2I∗cScIvIc+ζ1(−ηI∗cS∗c(β1+β3m)S∗vB∗(β2I∗vI∗c−rK)+β2S∗cδ)(β1B+β3Ic)Sv+ζ12ηδS∗vI∗cS∗c(β1+β3m)S∗vB∗(β2I∗vI∗c−rK)−ζ1ηI∗cS∗c(β1+β3m)S∗vB∗(β2I∗vI∗c−rK)(δSv+λS∗vSv)+ζ1(ηI∗cS∗c(β1+β3m)S∗vB∗(β2I∗vI∗c−rK)+β2S∗cδ)λ−ζ1(ηI∗cS∗c(β1+β3m)S∗vB∗(β2I∗vI∗c−rK)+β2S∗cδ)δS∗v−ζ1β1β2B∗S∗cδ−ζ1β1S∗cβ1+β3m(β2I∗vI∗c−rK)ηB∗IcB+ζ1β1S∗cβ1+β3m(β2I∗vI∗c−rK)ηI∗c, | (3.37) |
or equivalently
dVdt=−ζ1rK(sc−S∗c)2−ζ1rIcScK−ζ1rI∗cS∗cK−ζ1β2I∗cScIvIc−ζ1ηI∗cS∗c(β1B+β3Ic)Sv(β1+β3m)S∗vB∗−ζ1β1rηI∗cS∗cK(β1+β3m)−ζ1ηI∗cS∗c(β1+β3m)S∗vB∗(β2I∗vI∗c−rK)(δSv+λS∗vSv)−ζ1(ηI∗cS∗c(β1+β3m)S∗vB∗(β2I∗vI∗c−rK)+β2S∗cδ)δS∗v−ζ1β1β2B∗S∗cδ−ζ1β1S∗cβ1+β3m(β2I∗vI∗c−rK)ηB∗IcB−ζ12rηδS∗vI∗cS∗cK(β1+β3m)S∗vB∗−ζ1(rηI∗cS∗cK(β1+β3m)S∗vB∗)λ+ζ1δ(rI∗c+Kβ2I∗v)Sc+Kβ2S∗c((β1B+β3Ic)Sv+λ)Kδ+ζ1K(2δ+β1B∗)I∗vS∗vS∗c+(Kβ2λI∗v+rI∗c(β1B+β3Ic)Sv)ηS∗cK(β1+β3m)S∗vB∗. | (3.38) |
From Eq (3.38), since ηI∗cS∗c(β1+β3m)S∗vB∗(β2I∗vI∗c−rK)=ζ3≥0, we have
−ζ1ηI∗cS∗c(β1+β3m)S∗vB∗(β2I∗vI∗c−rK)(δSv+λS∗vSv)≤0. |
With this in mind, and if we let
Z=−ζ1rK(sc−S∗c)2−ζ1rIcScK−ζ1rI∗cS∗cK−ζ1β2I∗cScIvIc−ζ1ηI∗cS∗c(β1B+β3Ic)Sv(β1+β3m)S∗vB∗−ζ1β1rηI∗cS∗cK(β1+β3m)−ζ1ηI∗cS∗c(β1+β3m)S∗vB∗(β2I∗vI∗c−rK)(δSv+λS∗vSv)−ζ1(ηI∗cS∗c(β1+β3m)S∗vB∗(β2I∗vI∗c−rK)+β2S∗cδ)δS∗v−ζ1β1β2B∗S∗cδ−ζ1β1S∗cβ1+β3m(β2I∗vI∗c−rK)ηB∗IcB−ζ12rηδS∗vI∗cS∗cK(β1+β3m)S∗vB∗−ζ1(rηI∗cS∗cK(β1+β3m)S∗vB∗)λ≤0,W=ζ1δ(rI∗c+Kβ2I∗v)Sc+Kβ2S∗c((β1B+β3Ic)Sv+λ)Kδ+ζ1K(2δ+β1B∗)I∗vS∗vS∗c+(Kβ2λI∗v+rI∗c(β1B+β3Ic)Sv)ηS∗cK(β1+β3m)S∗vB∗≥0 |
then (dV/dt)(Sc,Ic,Sv,Iv,B)≤0 if and only if Sc=S∗c, Ic=I∗c, Sv=S∗v, Iv=I∗v,B=B∗ and Z+(−W)≤0.
Therefore, the largest compact invariant set in {(Sc,Ic,Sv,Iv,B)∈Ω:dV/dt=0} is the singleton {E∗}. By LaSalle [39], it then implies that E∗ is globally asymptotically stable in Ω.
To investigate the possibility of existence of the bifurcation analysis of the system (2.1) at R0=1, we use the center manifold theory [40]. So using this approach, the following theorem can be established.
Theorem 8. If R0<1, then the system (2.1) exhibits forward bifurcation at R0=1.
Proof. Using center manifold theory [40], we carry out bifurcation analysis of system (2.1) at R0=1. Let us consider the change of variables Sc=x1, Ic=x2, Sv=x3, Iv=x4, B=x5. Furthermore, using the vector notation: X=(x1,x2,x3,x4,x5)T. Then the system (2.1) can be written in the form dX/dt=(g1,g2,g3,g4,g5)T as:
{dx1dt=rx1(1−x1+x2K)−β2x1x4−θx1,dx2dt=β2x1x4−(γ+η)x2,dx3dt=λ−(β1x5+β3x2)x3−δx3,dx4dt=(β1x5+β3x2)x3−δx4,dx5dt=ηx2−mx5. | (3.39) |
We consider β2 as bifurcation parameter so that R0=1 if
β2=β∗2=r(γ+η)δ2mKλ(r−θ)(mβ3+β1η). | (3.40) |
The Jacobian matrix of Eq (3.39) at disease-free equilibrium E0 is given by
J(E0)=[θ−r−(r−θ)0−K(r−θ)β∗2r00−γ−η0K(r−θ)β∗2r00−β3λδ−δ0−β1λδ0β3λδ0−δβ1λδ0η00−m]. | (3.41) |
The right eigenvector, u=(u1,u2,u3,u4,u5)T is computed from J(E0)u=0 so that
u1=−Kβ∗2r(r−θγ+η+1)u4,u2=K(r−θ)β∗2r(γ+η)u4,u3=−u4,u5=ηK(r−θ)β∗2r(γ+η)mu4, |
where u4=u4>0. Similarly, the left eigenvector, z=(z1,z2,z3,z4,z5) is computed from zJ(E0)=0 so that z1=z3=0,z2=δrK(r−θ)β∗2z4,z5=β1λδmz4;z4=z4>0. The nonzero second partial derivatives of g1,g2,g3 and g4 are given as follows:
∂2g1∂x1∂x1=∂2g1∂x1∂x1=−2rK,∂2g1∂x1∂x2=∂2g1∂x2∂x1=−rK,∂2g1∂x1∂x4=∂2g1∂x4∂x1=−β∗2,∂2g2∂x1∂x2=∂2g2∂x2∂x1=β∗2,∂2g3∂x2∂x3=∂2g3∂x3∂x2=−β3,∂2g3∂x3∂x5=∂2g3∂x5∂x3=−β1,∂2g4∂x2∂x3=∂2g4∂x3∂x2=β3,∂2g4∂x3∂x5=∂2g4∂x5∂x3=β1,∂2g1∂x4∂β∗2=∂2g1∂β∗2∂x4=−x∗1,∂2g2∂x4∂β∗2=∂2g2∂β∗2∂x4=x∗1. |
All the others second partial derivatives of gi,i=1,...,5 are zero. Based on center manifold theorem [40], the coefficients a and b are given by
a=5∑i,j,k=1zkuiuj∂2gk∂xi∂xj(E0)=−[2δ3r(γ+η)mKλ(r−θ)2(mβ3+β1η)(r−θγ+η+1)+2δβ3γ+η+2ηK(r−θ)δ2]z4u24<0,b=5∑i,k=1zkui∂2gk∂xi∂β∗2(E0)=K(r−θ)(mβ3+β1η)r(γ+η)δmz4u4>0. |
Since a is negative and b is positive, then the system (2.1) exhibits forward bifurcation at R0=1 and there exists at least one stable endemic equilibrium when R0>1 (See Figure 4).
In this section, we compute the sensitivity index of basic reproduction number, R0, with respect to the main parameters [41]. This help us to check and identify parameters which highly affect R0 on the eradication or spread of CBD. For example, sensitivity index of R0 with respect to K is given by
ΔR0K=∂R0∂K×KR0=0.5. |
In the same way, the sensitivity index of R0 are also computed and the corresponding sensitivity indeces are given in Table 2.
Parameter | Sensitivity indices of R0 | Parameter | Sensitivity indices of R0 |
K | +0.5 | θ | –0.0454545 |
β1 | +0.0616258 | λ | +0.5 |
β2 | +0.5 | δ | –1 |
β3 | +0.438374 | γ | –0.178571 |
η | –0.259803 | m | –0.0616258 |
r | +0.0454545 |
The parameters: K,β1,β2,β3,r and λ have positive sensitivity indices show that the more impact on expanding the disease in the population if their values are increasing since R0 increases. Besides, the parameters: θ,η,δ,γ and m have negative sensitivity indices show that less impact on expanding the disease as their values increase. The bar diagram of the sensitivity indices of R0 in Table 2 is depicted in Figure 5. Hence, the parameters: K,β2 and λ are the most in influential in expanding the disease.
Furthermore, in Figure 6, we have seen that the contour plot of basic reproduction number R0 with respect to the parameters K, β2 and K, λ, respectively. It can be observed from Figure 6(a) that for decreasing value of K and β2, R0 decreases. For increasing K and λ the basic reproduction number R0 increases, which has been shown in Figure 6(b).
In order to illustrate the analytical results, the numerical simulations of the system (2.1) were performed. Some parameter values were assumed and some of them were obtained from literature. In addition to parameter values in Table 1, we assumed the initial data: Sc(0)=100,Ic(0)=50,Sv(0)=50,Iv(0)=10,B(0)=2.
Figures 7(a) and 8(a) depict the global asymptotic behaviour of the infected coffee and infected vector at the disease-free equilibrium E0 when R0<1 where the disease dies out regardless of large initial values of the infectious population. On the other hand, Figures 7(b) and 8(b) show the global asymptotic behaviour of the infected coffee and infected vector at the endemic equilibrium E∗ when R0>1 where the disease persists regardless of the initial sizes of the infectious population.
From the Figure 9(a) susceptible coffee berries decreases while the infected coffee berries increases with R0>1 due to there is very favorable condition for breeding of pathogen and vector that transmit the disease. The susceptible vector population in the Figure 9(c) decreases while infected vector population increases. The CBD pathogen population in the Figure 9(b) increases to a maximum point and then proceed decrease with minimum at unfavorable climate.
In the Figure 10(a), (b), the effects of the modification factor for asymptomatic coefficient at different values: K=150 and β2=0.000795455 are displayed. The projection shows that the cumulative number of individuals becoming infected coffee berries is high when K and β2 become high and becoming decreasing with low values of K and β2, which minimize also the values of basic reproduction number less one. As shown in Figure 10(c), (d), when the rates: K and β2 increase, the number of individuals becoming infected vector is high and decreasing the value of decreases the number of individuals becoming infected. As shown in Figure 11(a), (b), when the rates: K and β2 increase, the concentration of the pathogen becomes high, and decreasing the value of decreases the pathogen.
In this paper, we formulated and analyzed a nonlinear deterministic mathematical model for CBD transmission dynamics in a coffee farm. A compartmental mathematical model by including coffee plants and vectors of disease with the interaction of fungal pathogen was investigated. We obtained the feasible region where the model is epidemiologically and mathematically meaningful. The positivity, existence and uniqueness of model solutions are also demonstrated. The equilibria (disease-free and endemic equilibrium) of the model were computed. We calculated the basic reproduction number at the disease-free equilibrium point by using the next-generation matrix method. The local stability of disease-free and endemic equilibria is shown using the Routh-Hurwitz criteria. Besides, the global stability of equilibria is proved by using the Lyapunov function. Moreover, bifurcation analysis is proved by the Center Manifold theory. The sensitivity indices of basic reproduction number with respect to the main parameters are computed. Further, sensitivity indices are graphically shown and the most influential parameters in expanding the disease are identified. Despite coffee playing a dominant role in the social, cultural, and national economy, the country's coffee industry is potentially at risk due to climate changes. We discussed the impact of Colletotrichum kahawae fungus and vectors of disease in relation to climatic variables. More vectors and CBD pathogens are produced at the favourable climate which indirectly increases the rate of infected coffee berries. Thus, the frequency and severity of climate extremes are increasing and making adaptation an absolute imperative necessity through using current information on climate variability to develop long-term plans for managing CBD. Since CBD is highly dependent upon climatic factors, we are doing for the future with a paper applying the climatic variability in this model. Numerical simulations of the model suggested we apply effective control interventions on some parameters to control the disease. This will be done using chemical, cultural, and biological control strategies. We conclude that according to our mathematical model we have an endemic equilibrium point and the coffee disease remains endemic provided that the basic reproduction number is greater than one. Thus, we are developing the model with optimal control for future work to eradicate the disease.
The authors declare that there is no conflict of interests.
The authors wish to express their special gratitude to the editor and the reviewers for the helpful comments given for this paper. This work was supported by Adama Science and Technology University (grant number: ASTU/SP-R/001/19). We would like to express our appreciation for their support.
[1] |
O. Sujaritpong, S. Yoo-Kong, P. Bhadola, Analysis and dynamics of the international coffee trade network, J. Phys. Conf. Ser., 1719 (2021), 012106. https://doi.org/10.1088/1742-6596/1719/1/012106 doi: 10.1088/1742-6596/1719/1/012106
![]() |
[2] |
W. Garedew, F. Lemessa, F. Pinard, Assessment of berry drop due to coffee berry disease and non-CBD factors in arabica coffee under farmer's fields of Southwestern Ethiopia, Crop Prot., 98 (2017), 276–282. https://doi.org/10.1016/j.cropro.2017.04.012 doi: 10.1016/j.cropro.2017.04.012
![]() |
[3] |
A. Vieira, D. N. Silva, V. Varzea, O. S. Paulo, D. Batista, Novel insights on colonization routes and evolutionary potential of Colletotrichum kahawae, a severe pathogen of Coffea arabica, Mol. Plant Pathol., 19 (2018), 2488–2501. https://doi.org/10.1111/mpp.12726 doi: 10.1111/mpp.12726
![]() |
[4] |
D. Batista, D. N. Silva, A. Vieira, A. Cabral, A. S. Pires, A. Loureiro, et al., Legitimacy and implications of reducing Colletotrichum kahawae to subspecies in plant pathology, Front. Plant Sci., 7 (2017), 2051. https://doi.org/10.3389/fpls.2016.02051 doi: 10.3389/fpls.2016.02051
![]() |
[5] |
J. A. Mouen Bedimo, D. Bieysse, S. Nyasse, J. L. Notteghem, C. Cilas, Role of rainfall in the development of coffee berry disease in Coffea arabica caused by Colletotrichum kahawae, in Cameroon, Plant Pathol., 59 (2010), 324–329. https://doi.org/10.1111/j.1365-3059.2009.02214.x doi: 10.1111/j.1365-3059.2009.02214.x
![]() |
[6] | A. L. Gaitan, M. A. Cristancho, B. L. Castro Caicedo, C. A. Rivillas, PART I: Infectious diseases, compendium of coffee diseases and pests, Am. Phytopathol. Soc., 2016 (2016), 3–44. |
[7] |
J. McDonald, A preliminary account of a disease of green Coffee berries in Kenya Colony, Trans. Br. Mycol. Soc., 11 (1926), 145–154. https://doi:10.1016/s0007-1536(26)80033-6 doi: 10.1016/s0007-1536(26)80033-6
![]() |
[8] | H. Hindorf, Colletotrichum occurring on Coffea arabica; a review, J. Coffee Res., 5 (1975), 43–56. |
[9] |
M. C. Silva, M. Nicole, L. Rijo, J. P. Geiger, Jr. C. J. Rodrigues, Cytochemical aspects of the plant–rust fungus interface during the compatible interaction Coffea arabica (cv. Caturra)–Hemileia vastatrix (race Ⅲ), Int. J. Plant Sci., 160 (1999), 79–91. https://doi.org/10.1086/314113 doi: 10.1086/314113
![]() |
[10] | K. Alemu, G. Adugna, F. Lemessa, D. Muleta, Current status of coffee berry disease (Colletotrichum kahawae Waller and Bridge) in Ethiopia, Arch. Phytopathol. Plant Prot., 49 (2016), 421–433. |
[11] |
E. Griffiths, J. N. Gibbs, J. M. Waller, Control of coffee berry disease, Ann. Appl. Biol., 67 (1991), 45–74. https://doi:10.1111/j.1744-7348.1971.tb02907.x doi: 10.1111/j.1744-7348.1971.tb02907.x
![]() |
[12] | G. Adugna, C. Jefuka, Resistance levels of Arabica coffee cultivars to coffee berry disease, coffee wilt and leaf rust diseases in Ethiopia, in Proceedings of the 12th Crop Science Society of Ethiopia (CSSE), 2006 (2006), 22–24. |
[13] | M. Ejeta, A review of coffee diseases and their control in Ethiopia, in Proceedings of the First Ethiopian Crop Protection Symposium, Addis Ababa (Ethiopia), 1985 (1985), 4–7. |
[14] |
J. A. M. Bedimo, D. Bieysse, I. Njiayouom, J. P. Deumeni, C. Cilas, J. L. Notteghem, Effect of cultural practices on the development of arabica coffee berry disease, caused by Colletotrichum kahawae, Eur. J. Plant Pathol., 119 (2007), 391–400. https://doi:10.1007/s10658-007-9169-x doi: 10.1007/s10658-007-9169-x
![]() |
[15] |
J. M. Bedimo, I. Njiayouom, D. Bieysse, M. N. Nkeng, C. Cilas, J. L. Notteghem, Effect of shade on Arabica coffee berry disease development: Toward an agroforestry system to reduce disease impact, Phytopathology, 98 (2008), 1320–1325. https://doi.org/10.1094/PHYTO-98-12-1320 doi: 10.1094/PHYTO-98-12-1320
![]() |
[16] |
D. N. Silva, P. Talhinhas, L. Cai, L. Manuel, E. K. Gichuru, A. Loureiro, et al., Host-jump drives rapid and recent ecological speciation of the emergent fungal pathogen Colletotrichum kahawae, Mol. Ecol., 21 (2012), 2655–2670. https://doi.org/10.1111/j.1365-294X.2012.05557.x doi: 10.1111/j.1365-294X.2012.05557.x
![]() |
[17] |
M. D. C. Silva, V. Varzea, L. Guerra-Guimaraes, H. G. Azinheira, D. Fernandez, A. S. Petitot, et al., Coffee resistance to the main diseases: leaf rust and coffee berry disease, Braz. J. Plant Physiol., 18 (2006), 119–147. https://doi:10.1590/s1677-04202006000100010 doi: 10.1590/s1677-04202006000100010
![]() |
[18] | W. Workafes, K. Kassu, Coffee production systems in Ethiopia, in Proceedings of the workshop on control of coffee berry disease in Ethiopia, 2000. |
[19] | D. Fotsa, E. Houpa, D. Bekolle, C. Thron, M. Ndoumbe, Mathematical modelling and optimal control of anthracnose, preprint, arXiv: 1307.1754. |
[20] |
N. J. Cunniffe, C. A. Gilligan, Invasion, persistence and control in epidemic models for plant pathogens: the effect of host demography, J. R. Soc., Interface, 7 (2010), 439–451. https://doi.org/10.1098/rsif.2009.0226 doi: 10.1098/rsif.2009.0226
![]() |
[21] |
Y. Fotso, S. Touzeau, B. Tsanou, S. Bowong, F. Grognard, Modelling and optimal strategy to control coffee berry borer, Math. Methods Appl. Sci., 44 (2021), 14569–14592. https://doi.org/10.1002/mma.7726 doi: 10.1002/mma.7726
![]() |
[22] |
A. Mohammed, A. Jambo, Importance and characterization of coffee berry disease (Colletotrichum kahawae) in Borena and Guji zones, Southern Ethiopia, J. Plant Pathol. Microb., 6 (2015), 302. https://doi.org/10.4172/2157-7471.1000302 doi: 10.4172/2157-7471.1000302
![]() |
[23] | A. M. Klein, I. Steffan-Dewenter, T. Tscharntke, Fruit set of highland coffee increases with the diversity of pollinating bees, in Proceedings of the Royal Society of London. Series B: Biological Sciences, 270 (2003), 955–961. https://doi.org/10.1098/rspb.2002.2306 |
[24] |
J. A. Mouen Bedimo, D. Bieysse, C. Cilas, J. L. Nottˊeghem, Spatio-temporal dynamics of arabica coffee berry disease caused by Colletotrichum kahawae on a plot scale, Plant Dis., 91 (2007), 1229–1236. https://doi.org/10.1094/PDIS-91-10-1229\newpage doi: 10.1094/PDIS-91-10-1229
![]() |
[25] |
H. Kagezi, K. Patrick, K. Judith, O. D. Nicholas, N. Lilian, W. Wagoire, Predicting the response of insect pests and diseases of Arabica coffee to climate change along an altitudinal gradient in Mt. Elgon region, Uganda, J. Agric. Environ. Sci., 7 (2018), 134–140. https://doi.org/10.15640/jaes.v7n1a14 doi: 10.15640/jaes.v7n1a14
![]() |
[26] |
J. S. Bale, G. J. Masters, I. D. Hodkinson, C. Awmack, T. M. Bezemer, V. K. Brown, et al., Herbivory in global climate change research: direct effects of rising temperature on insect herbivores, Global Change Biol., 8 (2002), 1–16. https://doi.org/10.1046/j.1365-2486.2002.00451.x doi: 10.1046/j.1365-2486.2002.00451.x
![]() |
[27] | J. M. Bedimo, C. Cilas, J. L. Nottˊeghem, D. Bieysse, Effect of temperatures and rainfall variations on the development of coffee berry disease caused by Colletotrichum kahawae, Crop Prot., 31 (2012), 125–131. |
[28] | M. Jirata, S. Asefa, The status of coffee berry disease in Oromia, in Processing of the workshop on control coffee berry disease in Ethiopia 13–15 August 1999, 2000. |
[29] | N. N. Nicholls, Observed climate variability and change, Cambridge University Press Cambridge, 1996 (1996), 135–192. https://hal.archives-ouvertes.fr/hal-03344530. |
[30] |
A. G. Ahmed, L. K. Murungi, R. Babin, Developmental biology and demographic parameters of antestia bug Antestiopsis thunbergii (Hemiptera: Pentatomidae), on Coffea arabica (Rubiaceae) at different constant temperatures, Int. J. Trop. Insect. Sci., 36, (2016), 119–127. https://doi.org/10.1017/S1742758416000072 doi: 10.1017/S1742758416000072
![]() |
[31] |
J. S. Bale, G. J. Masters, I. D. Hodkinson, C. Awmack, T. M. Bezemer, V. K. Brown, et al., Herbivory in global climate change research: direct effects of rising temperature on insect herbivores, Global Change Biol., 8 (2002), 1–16. https://doi.org/10.1046/j.1365-2486.2002.00451.x doi: 10.1046/j.1365-2486.2002.00451.x
![]() |
[32] | G. Adunga, The influence of Climate condition on resistance level of released coffee types to coffee berry disease (CBD), in proceeding of the 2nd Annual conference of Crop Protection Society of Ethiopia (CPSE), 1994 (1994), 26–27. |
[33] |
J. M. Waller, Water-borne spore dispersal in coffee berry disease and its relation to control, Ann. Appl. Biol., 71 (1972), 1–18. https://doi:10.1111/j.1744-7348.1972.tb04712.x doi: 10.1111/j.1744-7348.1972.tb04712.x
![]() |
[34] |
T. Abraha, F. Al Basir, L. L. Obsu, D. F. Torres, Pest control using farming awareness: impact of time delays and optimal use of biopesticides, Chaos, Solitons, Fractals, 146 (2021), 110869. https://doi.org/10.1016/j.chaos.2021.110869 doi: 10.1016/j.chaos.2021.110869
![]() |
[35] | Y. Fotso, S. Touzeau, B. Tsanou, F. Grognard, S. Bowong, Mathematical modelling of a pest in an age-structured crop model: the coffee berry borer case, preprint, arXiv: hal-03505679v2. |
[36] | M. M Campanha, R. H. S. Santos, G. B. D. Freitas, H. E. P. Martinez, C. Jaramillo-Botero, S. L. Garcia, Comparative analysis of litter and soil characteristics under coffee (Coffea arabica L.) crop in agroforestry and monoculture systems, Revista Árvore, 31 (2007), 805–812. |
[37] | M. A. Khan, A. Wahid, S. Islam, I. Khan, S. Shafie, T. Gul, Stability analysis of an SEIR epidemic model with non-linear saturated incidence and temporary immunity, Int. J. Adv. Appl. Math. Mech., 2 (2015), 1–14. |
[38] |
A. Mojeeb, E. Osman, AK. Isaac, Simple mathematical model for malaria transmission, J. Adv. Math. Comp. Sci., 25 (2017), 1–24. https://doi.org/10.9734/jamcs/2017/37843 doi: 10.9734/jamcs/2017/37843
![]() |
[39] | J. P. LaSalle, The stability of dynamical systems, society for industrial and applied mathematics, in Proceedings of the Conference Series in Applied Mathematics, 1976. |
[40] |
C. Castillo-Chavez, B. Song, Dynamical models of tuberculosis and their applications, Math. Biosci. Eng., 1 (2004), 361. http://doi.org/10.3934/mbe.2004.1.361 doi: 10.3934/mbe.2004.1.361
![]() |
[41] |
N. Chitnis, J. M. Hyman, J. M. Cushing, Determining important parameters in the spread of malaria through the sensitivity analysis of a mathematical model, Bull. Math. Biol., 70 (2008), 1272. https://doi.org/10.1007/s11538-008-9299-0 doi: 10.1007/s11538-008-9299-0
![]() |
[42] |
P. Van den Driessche, J. Watmough, Reproduction numbers and sub-threshold endemic equilibria for compartmental models of disease transmission, Math. Biosci., 180 (2002), 29–48. https://doi.org/10.1016/s0025-5564(02)00108-6 doi: 10.1016/s0025-5564(02)00108-6
![]() |
[43] |
G. Peyser, On the Cauchy-Lipschitz Theorem, Am. Math. Mon., 65 (1958), 760–762. https://doi.org/10.2307/2310680 doi: 10.2307/2310680
![]() |
1. | Mohammedsultan Abaraya Abawari, Legesse Lemecha Obsu, Abdisa Shiferaw Melese, Optimal control analysis of coffee berry borer infestation in the presence of farmer's awareness, 2023, 31, 2769-0911, 10.1080/27690911.2023.2169684 | |
2. | Kumlachew Alemu, Girma Adugna, Fikre Lemessa, Diriba Muleta, Biocontrol potentials of native bacterial strains for the management of coffee berry disease (Colletotrichum kahawae) in Ethiopia, 2023, 33, 0958-3157, 98, 10.1080/09583157.2022.2163981 | |
3. | Yong Zhou, Yiming Ding, Minrui Guo, Path analysis method in an epidemic model and stability analysis, 2023, 11, 2296-424X, 10.3389/fphy.2023.1158814 | |
4. | Abdisa Shiferaw Melese, Mathematical model and optimal control analysis of coffee berry borer with temperature and rainfall variability, 2024, 17, 1793-5245, 10.1142/S179352452350047X | |
5. | Carlos Andrés Trujillo-Salazar, Gerard Olivar-Tost, Deissy Milena Sotelo-Castelblanco, Bifurcation Analysis in a Coffee Berry-Borer-and-Ants Prey–Predator Model, 2024, 12, 2227-7390, 1670, 10.3390/math12111670 | |
6. | Tariq Q. S. Abdullah, Gang Huang, Wadhah Al-Sadi, Yasser Aboelmagd, Wael Mobarak, Fractional Dynamics of Cassava Mosaic Disease Model with Recovery Rate Using New Proposed Numerical Scheme, 2024, 12, 2227-7390, 2386, 10.3390/math12152386 | |
7. | Raveena Selvanarayanan, Surendran Rajendran, 2023, Roaming the Coffee Plantations Using Grey Wolves Optimisation and the Restricted Boltzmann Machine to Predict Coffee Berry Disease, 979-8-3503-0085-7, 681, 10.1109/ICSSAS57918.2023.10331629 | |
8. | Abayneh Kebede Fantaye, Fractional order for the transmission dynamics of coffee berry diseases (CBD), 2024, 0956-7925, 1, 10.1017/S0956792524000780 |
Parameter | Description | Unit | Value | Source |
β1 | Contact rate of vector with pathogen environment | day−1 | 0.000209818 | Estimated |
β2 | Contact rate of coffee berries with infected vector | day−1 | 0.000795455 | Estimated |
β3 | Contact rate of vector with infected coffee berries | day−1 | 0.000149091 | Estimated |
θ | Mortality rate of healthy coffee berries | day−1 | 0.01 | [35] |
γ | Removal rate of infected coffee berries | day−1 | 0.005 | Estimated |
r | Growth rate of new coffee berries | day−1 | 0.12 | [36] |
K | Carrying capacity | m−2 | 150 | Estimated |
η | Induced rate of infected coffee berries | ℧ | 0.009 | Estimated |
λ | Recruitment rate of vector | day−1 | 0.488364 | Estimated |
m | Decay rate of pathogen | day−1 | 0.0900982 | Estimated |
δ | Death rate of vector | day−1 | 0.009 | Estimated |
Parameter | Sensitivity indices of R0 | Parameter | Sensitivity indices of R0 |
K | +0.5 | θ | –0.0454545 |
β1 | +0.0616258 | λ | +0.5 |
β2 | +0.5 | δ | –1 |
β3 | +0.438374 | γ | –0.178571 |
η | –0.259803 | m | –0.0616258 |
r | +0.0454545 |
Parameter | Description | Unit | Value | Source |
β1 | Contact rate of vector with pathogen environment | day−1 | 0.000209818 | Estimated |
β2 | Contact rate of coffee berries with infected vector | day−1 | 0.000795455 | Estimated |
β3 | Contact rate of vector with infected coffee berries | day−1 | 0.000149091 | Estimated |
θ | Mortality rate of healthy coffee berries | day−1 | 0.01 | [35] |
γ | Removal rate of infected coffee berries | day−1 | 0.005 | Estimated |
r | Growth rate of new coffee berries | day−1 | 0.12 | [36] |
K | Carrying capacity | m−2 | 150 | Estimated |
η | Induced rate of infected coffee berries | ℧ | 0.009 | Estimated |
λ | Recruitment rate of vector | day−1 | 0.488364 | Estimated |
m | Decay rate of pathogen | day−1 | 0.0900982 | Estimated |
δ | Death rate of vector | day−1 | 0.009 | Estimated |
Parameter | Sensitivity indices of R0 | Parameter | Sensitivity indices of R0 |
K | +0.5 | θ | –0.0454545 |
β1 | +0.0616258 | λ | +0.5 |
β2 | +0.5 | δ | –1 |
β3 | +0.438374 | γ | –0.178571 |
η | –0.259803 | m | –0.0616258 |
r | +0.0454545 |