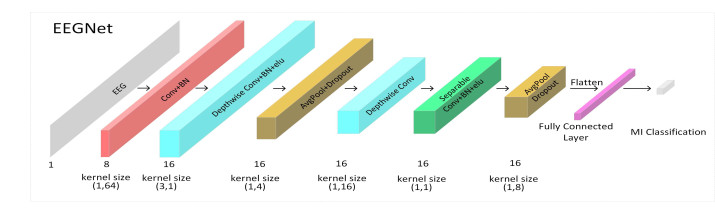
Motor Imagery EEG (MI-EEG) classification plays an important role in different Brain-Computer Interface (BCI) systems. Recently, deep learning has been widely used in the MI-EEG classification tasks, however this technology requires a large number of labeled training samples which are difficult to obtain, and insufficient labeled training samples will result in a degradation of the classification performance. To address the degradation problem, we investigate a Self-Supervised Learning (SSL) based MI-EEG classification method to reduce the dependence on a large number of labeled training samples. The proposed method includes a pretext task and a downstream classification one. In the pretext task, each MI-EEG is rearranged according to the temporal characteristic. A network is pre-trained using the original and rearranged MI-EEGs. In the downstream task, a MI-EEG classification network is firstly initialized by the network learned in the pretext task and then trained using a small number of the labeled training samples. A series of experiments are conducted on Data sets 1 and 2b of BCI competition IV and IVa of BCI competition III. In the case of one third of the labeled training samples, the proposed method can obtain an obvious improvement compared to the baseline network without using SSL. In the experiments under different percentages of the labeled training samples, the results show that the designed SSL strategy is effective and beneficial to improving the classification performance.
Citation: Yanghan Ou, Siqin Sun, Haitao Gan, Ran Zhou, Zhi Yang. An improved self-supervised learning for EEG classification[J]. Mathematical Biosciences and Engineering, 2022, 19(7): 6907-6922. doi: 10.3934/mbe.2022325
[1] | Ning Huang, Zhengtao Xi, Yingying Jiao, Yudong Zhang, Zhuqing Jiao, Xiaona Li . Multi-modal feature fusion with multi-head self-attention for epileptic EEG signals. Mathematical Biosciences and Engineering, 2024, 21(8): 6918-6935. doi: 10.3934/mbe.2024304 |
[2] | Zizhuo Wu, Qingshan She, Zhelong Hou, Zhenyu Li, Kun Tian, Yuliang Ma . Multi-source online transfer algorithm based on source domain selection for EEG classification. Mathematical Biosciences and Engineering, 2023, 20(3): 4560-4573. doi: 10.3934/mbe.2023211 |
[3] | Jin Zhang, Nan Ma, Zhixuan Wu, Cheng Wang, Yongqiang Yao . Intelligent control of self-driving vehicles based on adaptive sampling supervised actor-critic and human driving experience. Mathematical Biosciences and Engineering, 2024, 21(5): 6077-6096. doi: 10.3934/mbe.2024267 |
[4] | Ansheng Ye, Xiangbing Zhou, Kai Weng, Yu Gong, Fang Miao, Huimin Zhao . Image classification of hyperspectral remote sensing using semi-supervised learning algorithm. Mathematical Biosciences and Engineering, 2023, 20(6): 11502-11527. doi: 10.3934/mbe.2023510 |
[5] | Sakorn Mekruksavanich, Wikanda Phaphan, Anuchit Jitpattanakul . Epileptic seizure detection in EEG signals via an enhanced hybrid CNN with an integrated attention mechanism. Mathematical Biosciences and Engineering, 2025, 22(1): 73-105. doi: 10.3934/mbe.2025004 |
[6] | Xu Yin, Ming Meng, Qingshan She, Yunyuan Gao, Zhizeng Luo . Optimal channel-based sparse time-frequency blocks common spatial pattern feature extraction method for motor imagery classification. Mathematical Biosciences and Engineering, 2021, 18(4): 4247-4263. doi: 10.3934/mbe.2021213 |
[7] | Xiaotong Ding, Lei Yang, Congsheng Li . Study of MI-BCI classification method based on the Riemannian transform of personalized EEG spatiotemporal features. Mathematical Biosciences and Engineering, 2023, 20(7): 12454-12471. doi: 10.3934/mbe.2023554 |
[8] | Tianhui Sha, Yikai Zhang, Yong Peng, Wanzeng Kong . Semi-supervised regression with adaptive graph learning for EEG-based emotion recognition. Mathematical Biosciences and Engineering, 2023, 20(6): 11379-11402. doi: 10.3934/mbe.2023505 |
[9] | Jiacan Xu, Donglin Li, Peng Zhou, Chunsheng Li, Zinan Wang, Shenghao Tong . A multi-band centroid contrastive reconstruction fusion network for motor imagery electroencephalogram signal decoding. Mathematical Biosciences and Engineering, 2023, 20(12): 20624-20647. doi: 10.3934/mbe.2023912 |
[10] | Yue Zhang, Haitao Gan, Furong Wang, Xinyao Cheng, Xiaoyan Wu, Jiaxuan Yan, Zhi Yang, Ran Zhou . A self-supervised fusion network for carotid plaque ultrasound image classification. Mathematical Biosciences and Engineering, 2024, 21(2): 3110-3128. doi: 10.3934/mbe.2024138 |
Motor Imagery EEG (MI-EEG) classification plays an important role in different Brain-Computer Interface (BCI) systems. Recently, deep learning has been widely used in the MI-EEG classification tasks, however this technology requires a large number of labeled training samples which are difficult to obtain, and insufficient labeled training samples will result in a degradation of the classification performance. To address the degradation problem, we investigate a Self-Supervised Learning (SSL) based MI-EEG classification method to reduce the dependence on a large number of labeled training samples. The proposed method includes a pretext task and a downstream classification one. In the pretext task, each MI-EEG is rearranged according to the temporal characteristic. A network is pre-trained using the original and rearranged MI-EEGs. In the downstream task, a MI-EEG classification network is firstly initialized by the network learned in the pretext task and then trained using a small number of the labeled training samples. A series of experiments are conducted on Data sets 1 and 2b of BCI competition IV and IVa of BCI competition III. In the case of one third of the labeled training samples, the proposed method can obtain an obvious improvement compared to the baseline network without using SSL. In the experiments under different percentages of the labeled training samples, the results show that the designed SSL strategy is effective and beneficial to improving the classification performance.
Electroencephalography (EEG), as a non-invasive and cost-effective approach, is commonly used in various fields such as rehabilitation [1] and disease diagnosis [2]. Motor Imagery EEG (MI-EEG) classification plays an important role in different Brain-Computer Interface (BCI) systems [3]. The goal of the MI-EEG based BCI system is to control different external devices [4]. Due to non-stationary, nonlinearity, and randomness of MI-EEGs [5], how to accurately classify MI-EEGs is a crucial step in the MI-EEG based BCI system.
Up to now, different MI-EEG classification methods have been proposed by many researchers. In traditional classification methods, M¨uller-Gerking et al. [6] proposed the Common Spatial Pattern (CSP) method to classify single-channel MI-EEGs. Huang et al. [7] employed Surface Laplace Transform (SLT) and Power Spectral Density (PSD) to extract MI-EEG features. Chatterjee et al. [8] used wavelet energy, root means square error and the average power for feature extraction, and Support Vector Machine (SVM) to classify the left and right hand MI-EEGs. Recently, deep learning has developed rapidly in the computer vision and signal processing fields [9,10]. Therefore, deep learning provides an effective tool for the MI-EEG classification task [11,12]. Schirrmeister et al. [13] found that Batch Normalisation (BN) [14] and Exponential Linear Units (ELU) [15] could effectively improve the representation capability of Convolutional Neural Network (CNN). In [16], CNN and Augmented CSP (ACSP) was firstly used to extract the features and CNN was then used to classify the MI-EEGs. Besides CNN, a deep belief network was used for the MI-EEG classification task [17]. Li et al. [18] utilized the spatial location and time-frequency information to build 2D images. These images were then used as the inputs to train a network. Lawhern et al. [19] proposed EEGNet to identify the paradigm of EEGs. The above-mentioned MI-EEG classification methods belong to supervised learning, and the performance of supervised learning relies on a large number of labeled training samples.
However, accurate MI-EEG labeling is time-consuming and expensive. For example, labeling EEG during sleep requires professional technicians to check the EEG for several hours and mark the 30-second window one by one [20]. In the case of a small number of the labeled training samples, the performance of supervised learning may be poor and unstable. In the past years, Self-supervised Learning (SSL) has been verified that it is a novel and effective strategy to improve the performance under a small number of labeled training samples [21]. SSL generally includes two parts: a pretext and downstream task. A network is learned by the pretext task and transferred to the downstream task. The pretext task is mainly divided into two categories: generation and contrast. The typical generation methods include Colorization, Auto-Encoders, etc. In the typical contrast methods, the training samples are generated according to some strategies and used to train a pretext network. In SSL, how to define the pretext task is the core content.
Recently, SSL has been widely used in computer vision, natural language processing and other fields. Noroozi et al. [22] utilized a puzzle approach to design the pretext task. Li et al. [23] proposed a Twin-Cycle Autoencoder (TCAE) to learn the pretext network from videos without manual annotation. Banville et al. [24] constructed a time contrastive SSL method for classifying EEGs. In the MI-EEG classification field, few SSL-based studies have been investigated. Therefore, it is meaningful to design the SSL strategy to improve the MI-EEG classification performance.
This paper aims to propose a novel SSL approach to alleviate the degradation influence in the case of a small number of the labeled training samples. In this paper, a Temporal-Rearrange based MI-EEG network (TRMINet) is designed. The pretext task based on the temporal characteristics of MI-EEGs is firstly built to learn a pretext network from the whole MI-EEGs. The pretext network is then used to initialize the downstream MI-EEG classification one. Finally, the downstream network is trained using a small number of the labeled training samples. The main contributions are outlined as follows:
● A pretext task is designed according to the temporal characteristics of MI-EEGs.
● The differences of the MI-EEG representation ability among different CNNs are investigated.
● The impact of the pretext task on the classification performance of the downstream task is analyzed.
The rest of the paper is organized as follows. Some of the networks used in this paper are described in Section 2. Section 3 describes the pretext and downstream tasks. The details of the datasets are described in Section 4. The experimental configurations and results are described in Section 5. The conclusion and future directions are given in Section 6.
This section describes the networks used in the experiments. In deep learning, there were many classic networks, such as AlexNet [25], GoogLeNet [26], ResNet [9], etc. In these classic networks, AlexNet used ReLU [27] as the activation function and was trained in parallel on two GPUs. The computational complexity of the network and the interdependence of parameters were reduced by the ReLU function. The inception architecture was proposed in GoogLeNet, which could further reduce the computational complexity of the network. Meanwhile, BN [14] was used in GoogLeNet to standardize the output mean and variance of each layer of the network. Furthermore, the BN alleviated the vanishing gradient problem. The residual network was introduced in ResNet to deal with the training of deep network models. EfficientNet [10] was proposed by the Google team in 2019 and could balance the network width, depth, and resolution to improve performance. EfficientNet could focus on more image details than other scaling methods. The MobileNet Convolution (MBConv) was used in the convolutional layers of EfficientNet, except for the first convolutional layer which used the normal convolutional structure. The MBConv included a 1×1 pointwise convolution, a depthwise convolution [28], and a Squeeze-and-Excitation (SE) module [29]. The commonly used CNNs for EEG classification included DeepConvNet, ShallowConvNet [13] and EEGNet [19]. In particular, EEGNet attempted to classify EEGs from several paradigms using a single network. EEGNet used depthwise and separable convolutions instead of the traditional ones. Additionally, ELU [15] was used as the activation function. The architecture of EEGNet is shown in Figure 1 and the parameters of each layer of EEGNet are given in Table 1.
Layer | Operator | Output Channels | Kernel Size | Stride |
1 | Conv & BN | 8 | (1, 64) | 1 |
2 | Depthwise Conv & BN & elu | 16 | (3, 1) | 1 |
3 | Avgpool & Dropout | 16 | (1, 4) | 4 |
4 | Depthwise Conv | 16 | (1, 16) | 1 |
5 | Separable Conv & BN & elu | 16 | (1, 1) | 1 |
6 | AvgPool & Dropout | 16 | (1, 8) | 8 |
Meanwhile, a glossary containing all initials and abbreviations is given in Table 2.
Abbreviations | Explanation |
MI-EEG | Motor Imagery EEG |
SSL | Self-supervised Learning |
BCI | Brain-Computer Interface |
CSP | Common Spatial Pattern |
SLT | Surface Laplace Transform |
PSD | Power Spectral Density |
SVM | Support Vector Machine |
BN | Batch Normalisation |
ELU | Exponential Linear Units |
CNN | Convolutional Neural Network |
ACSP | Augmented Common Spatial Pattern |
TCAE | Twin-Cycle Autoencoder |
TRMINet | Temporal Rearrange Motor Imagery Network |
MBConv | MobileNet Convolution |
SE | Squeeze-and-Excitation |
TR | Temporal Rearrange |
ERS | Event-Related Synchronization |
ERD | Event-Related Desynchronization |
ICA | Independent Component Analysis |
ACC | Accuracy |
AUC | Area Under the Curve |
CI | Confidence Intervals |
To address the performance degradation under a small number of the labeled training samples, we design a self-supervised network framework, as shown in Figure 2. Firstly, MI-EEGs are pre-processed by denoising and filtering. In the pretext task, the Temporal Rearrange (TR) approach generates the rearranged MI-EEGs. The network of the pretext task is then trained to learn the MI-EEG representation using the original and rearranged MI-EEGs. The network of the downstream task is initialized by the pretext one and trained using the labeled MI-EEGs.
Formally, X={(xi,yi)|i∈[1,n],xi∈RC×T,yi∈{−1,1}} is used to denote the MI-EEG dataset. xi denotes each MI-EEG and yi∈{−1,1} denotes the label of xi. C denotes the number of MI-EEG channels and T denotes the number of sampling points of MI-EEGs.
The framework of the pretext task is shown in Figure 3. To generate labeled samples from multichannel MI-EEGs, we propose a Temporal Rearrange (TR) method. A fixed-length sliding window H is used to divide each original MI-EEG into multiple time window blocks. A new MI-EEG is then obtained by rearranging these time window blocks. The label of the original MI-EEG is set to 1, and that of the rearranged MI-EEG is set to −1. The original and rearranged samples and labels are represented by xi′ and yi′, respectively, and the number of generated samples is n′. The training set of the pretext task Z={(xi′,yi′)|i∈[1,n′],xi′∈RC×T,yi′∈{−1,1}}.
In this paper, EEGNet is used as the baseline network. The prediction function is denoted as ˆY=wTFΘ(Z)+w0. The convolution function in the EEGNet is denoted as FΘ. The parameters of the convolution function are denoted as Θ. The weight and the bias of the fully connected layer are denoted as w and w0, respectively. The weight parameters are denoted as W=[Θ,w,w0]. Cross entropy is used as the loss function in the pretext task, which is calculated by Eq (1).
L(Θ,w,w0)=n′∑i=1log(1+exp(−yi′[wTFΘ(xi′)+w0])) | (1) |
The optimal network parameters W∗=[Θ∗,w∗,w0∗] can be obtained by training the pretext network.
The downstream task aims to classify MI-EEGs by training an optimal network. The network of the downstream task is the same as that of the pretext task. The convolutional function in the network is denoted as FΘd. The parameters of the convolutional function is denoted as Θd. The weight and the bias of the fully connected layer are denoted as wd and w0d, respectively. The weight parameters are denoted as Wd=[Θd,wd,w0d]. The downstream network is initialized by the pretext one, i.e., Wd=[Θ∗,w∗,w0∗]. Then, The downstream network is fine-tuned using the labeled MI-EEGs. Cross entropy is used as the loss function in the downstream task, which is calculated by Eq (2).
L(Θd,wd,w0d)=t∑i=1log(1+exp(−yi[(w∗)TFΘ∗(xi)+w0∗])) | (2) |
After fine tuning, the optimal network parameters W″ can be obtained. The MI-EEGs can be finally predicted by W″.
This section describes the used data sets. Data set 1 of BCI competition IV contain 7 subjects. Each subject chooses two types from left hand, right hand, and foot for MI-EEGs. In the Data set 1, an MI-EEG trail contains 8 s of data, and a cross mark is displayed on the screen from 0 to 2 s. From 2 to 6 s, an arrow indicator appears in the center of the screen, and is superimposed on the cross mark. The subject performs the MI-EEGs according to the directions of the arrow indicator. The screen is finally blank in 6–8 s, indicating the end of this trail. The data is collected from 59 EEG channels, and the sampling frequency is set to 100 Hz. Therefore, one MI-EEG trail has 800 sampling points.
Data set IVa of BCI competition III contains 5 subjects. Only cues for right hand and foot are provided in the competition. In the Data set IVa, visual cues are displayed 3.5 s in the screen, and the presentation of target cues is intermitted by periods of random length, 1.75 to 2.25 s. The data is collected from 118 EEG channels, and the sampling frequency is set to 100Hz. In this dataset, the sample number of subjects aa, al, av, aw, and ay is 168,224, 84, 56, and 28, respectively. Since the sample number of subjects av, aw, and ay is very small, subjects aa and al are selected in the experiment. The experimental stimulus paradigms for the Data set 1 of BCI competition IV and the Data set IVa of BCI competition III are shown in Figure 4.
Data set 2b of BCI competition IV contains 9 subjects. The cue-based screening paradigm consists of two MI-EEG classes, namely the left and right hand. 120 repetitions of each MI-EEG class are available for each subject in total. More details of the dataset can be found at https://www.bbci.de/competition/iv.
Event-Related Desynchronization (ERD) and Event-Related Synchronization (ERS) are most evident in EEG channels C3, C4, and Cz [30], thus, the three channels are selected in the experiments. The positions of electrode caps [31] are generally illustrated in Figure 5.
Since electrooculogram (EOG) and noise can be collected in the MI-EEGs, the MI-EEGs is firstly preprocessed in the experiments. Generally speaking, the 8–30 Hz frequency band can reflect the characteristics of the MI-EEGs [32]. Therefore, a third-order Butterworth band-pass filter at 8–30 Hz is built to eliminate the effects of baseline drift and noise. Then, Independent Component Analysis (ICA) is used to reduce the influence of EOG.
All the used networks are implemented using Pytorch on NVIDIA GTX3090 and Intel(R) Core(TM) i9-10940X CPUs. The convolutional function in the CNN is initialized by the kaiming_normal function, and the fully connected layer is initialized by a normal distribution (N(0,0.1)). Adam is used for the optimization. In the pretext task, the epoch, batchsize, and learning rate are set to 200, 8, and 0.0001, respectively, and there is no validation or test set. The loss value is used to measure the performance of the trained pretext model. In the downstream task, the epoch, batchsize, and learning rate are set to 100, 50, and 0.001, respectively. The dataset of each subject is randomly divided by 3:1 to constitute the training and test sets. This process is repeated 10 times for each subject. The validation set is not used because the sample number for each subject is small.
Accuracy (ACC) and Area Under the Curve (AUC) are used to measure the performance of the networks. ACC can reflect the classification ability of the networks. AUC represents an aggregate measure of performance across all possible classification thresholds. In addition, Confidence Intervals (CI) at the 95% confidence level are used in the ablation study to obtain a level of accuracy confidence.
In this section, to determine the suitable network for the MI-EEG task, six popular networks are conducted on all subjects in the experiments, including ResNet [9], MobileNet [28], EfficientNet [10], DeepConvNet, ShallowConvNet [13] and EEGNet [19]. The experimental results are shown in Table 3.
Methods | ResNet | MobileNet | EfficientNet | DeepConvNet | ShallowConvNet | EEGNet | |||||||
Metrics | ACC | AUC | ACC | AUC | ACC | AUC | ACC | AUC | ACC | AUC | ACC | AUC | |
Data sets 1 (BCICIV) | a | 52.80 | 0.550 | 62.40 | 0.651 | 62.25 | 0.663 | 70.40 | 0.819 | 68.80 | 0.764 | 75.60 | 0.817 |
b | 55.20 | 0.574 | 50.40 | 0.521 | 56.00 | 0.583 | 67.00 | 0.763 | 73.20 | 0.820 | 71.60 | 0.786 | |
c | 53.60 | 0.574 | 52.80 | 0.540 | 60.80 | 0.622 | 51.40 | 0.647 | 64.40 | 0.732 | 70.60 | 0.765 | |
d | 63.60 | 0.724 | 64.80 | 0.728 | 78.00 | 0.844 | 63.40 | 0.870 | 88.80 | 0.945 | 91.80 | 0.977 | |
e | 67.34 | 0.628 | 53.06 | 0.520 | 79.59 | 0.884 | 92.20 | 0.994 | 95.40 | 0.995 | 96.40 | 0.993 | |
f | 66.50 | 0.699 | 58.50 | 0.663 | 64.40 | 0.714 | 63.00 | 0.786 | 72.60 | 0.793 | 80.20 | 0.874 | |
g | 69.50 | 0.733 | 64.80 | 0.736 | 74.00 | 0.826 | 78.80 | 0.904 | 82.60 | 0.896 | 85.20 | 0.910 | |
Data set IVa (BCICIII) | aa | 73.80 | 0.793 | 60.50 | 0.707 | 64.30 | 0.661 | 58.10 | 0.785 | 78.80 | 0.877 | 80.00 | 0.893 |
al | 91.70 | 0.966 | 82.10 | 0.944 | 83.90 | 0.952 | 75.70 | 0.964 | 95.40 | 0.986 | 95.70 | 0.987 | |
Data sets 2b (BCICIV) | 1 | 50.30 | 0.510 | 50.00 | 0.508 | 50.80 | 0.507 | 50.30 | 0.502 | 51.80 | 0.528 | 71.20 | 0.748 |
2 | 69.50 | 0.775 | 60.50 | 0.664 | 70.30 | 0.802 | 74.30 | 0.872 | 76.70 | 0.860 | 77.30 | 0.871 | |
3 | 58.00 | 0.613 | 55.70 | 0.580 | 59.30 | 0.629 | 57.50 | 0.601 | 61.80 | 0.649 | 63.00 | 0.682 | |
4 | 59.80 | 0.636 | 55.20 | 0.567 | 69.20 | 0.759 | 70.30 | 0.793 | 70.80 | 0.783 | 84.30 | 0.901 | |
5 | 95.20 | 0.993 | 75.50 | 0.837 | 96.20 | 0.994 | 93.70 | 0.957 | 99.10 | 0.999 | 98.90 | 0.999 | |
6 | 59.30 | 0.645 | 54.70 | 0.586 | 58.70 | 0.630 | 61.20 | 0.653 | 58.80 | 0.636 | 63.70 | 0.657 | |
7 | 80.00 | 0.881 | 62.00 | 0.667 | 80.20 | 0.872 | 85.00 | 0.920 | 86.00 | 0.912 | 88.20 | 0.924 | |
8 | 62.10 | 0.672 | 53.90 | 0.529 | 68.40 | 0.754 | 62.00 | 0.701 | 65.00 | 0.715 | 64.90 | 0.690 | |
9 | 63.70 | 0.705 | 51.50 | 0.549 | 66.30 | 0.701 | 70.00 | 0.762 | 76.00 | 0.824 | 73.80 | 0.793 |
It can be seen intuitively from Table 3 that EEGNet performs better than the other networks on most subjects, and ShallowConvNet can yield comparable performance to EEGNet on most subjects. It indicates that EEGNet and ShallowConvNet can learn better MI-EEG representations than the other four networks. Therefore, EEGNet and ShallowConvNet are chosen as the base classifiers.
An ablation experiment is conducted to verify the effectiveness of the proposed pretext network under a small number of labeled training samples. The base classifiers and one third of the training set are used for experiments. The MI-EEG classification method without using SSL is denoted as Base, which uses the random strategy to initialize the network weights. The proposed SSL method is denoted as TRMINet, which uses the pretext task. These pretext network is used to initialize the weights of the downstream classification network. The effectiveness of the pretext network is evaluated according to the performance of the downstream classification network. In order to visualize the accuracies of the Base and TRMINet methods, bar charts are plotted in Figures 6 and 7.
As shown in Figures 6 and 7, the accuracies of the TRMINet method are higher than the Base method on most subjects. It shows that the pretext task can learn a good representation which has a positive impact on the network training under a small number of the labeled training samples.
AUC is used to further measure the TRMINet and the Base methods. The results are shown in Table 4. As seen from Table 4, the AUC of the TRMINet method is closer, if not higher, than the Base method on most subjects. This shows that SSL under a small number of the labeled training samples can improve the classification performance of the downstream network. It also shows that the proposed pretext task can effectively learn MI-EEG representations.
Subjects | Base (EEGNet) | TRMINet (EEGNet) | Base (ShallowConvNet) | TRMINet (ShallowConvNet) | |||||||||
ACC | ACC CI | AUC | ACC | ACC CI | AUC | ACC | ACC CI | AUC | ACC | ACC CI | AUC | ||
Data sets 1 (BCICIV) | a | 53.20 | [46.10, 60.30] | 0.725 | 66.40 | [60.00, 72.80] | 0.741 | 64.20 | [57.30, 71.10] | 0.708 | 65.80 | [54.90, 76.70] | 0.739 |
b | 56.90 | [52.60, 61.20] | 0.649 | 60.40 | [54.50, 66.50] | 0.675 | 63.60 | [50.90, 76.30] | 0.712 | 65.00 | [52.00, 78.00] | 0.740 | |
c | 49.20 | [43.80, 54.50] | 0.561 | 55.20 | [50.30, 60.10] | 0.605 | 60.20 | [48.60, 71.80] | 0.651 | 61.00 | [50.30, 71.70] | 0.703 | |
d | 62.20 | [54.70, 69.70] | 0.740 | 75.80 | [64.30, 87.20] | 0.826 | 86.20 | [77.00, 95.40] | 0.938 | 84.40 | [77.20, 91.60] | 0.901 | |
e | 86.70 | [78.90, 94.40] | 0.946 | 91.20 | [84.30, 98.10] | 0.923 | 94.90 | [87.90,102.0] | 0.994 | 94.80 | [87.70,102.0] | 0.991 | |
f | 53.00 | [45.40, 60.50] | 0.648 | 68.40 | [61.30, 75.40] | 0.752 | 65.40 | [55.20, 75.60] | 0.732 | 66.00 | [58.20, 73.80] | 0.754 | |
g | 65.20 | [57.10, 73.30] | 0.814 | 75.80 | [67.10, 84.50] | 0.856 | 71.20 | [55.90, 86.50] | 0.820 | 75.00 | [59.60, 90.40] | 0.821 | |
Data set IVa (BCICIII) | aa | 61.40 | [55.20, 67.60] | 0.675 | 64.30 | [60.50, 68.10] | 0.755 | 57.90 | [46.20, 69.50] | 0.732 | 68.50 | [60.10, 76.90] | 0.785 |
al | 62.00 | [59.20, 64.70] | 0.728 | 71.10 | [65.70, 76.40] | 0.837 | 91.10 | [84.10, 98.10] | 0.975 | 91.30 | [87.90, 94.60] | 0.964 | |
Data sets 2b (BCICIV) | 1 | 54.20 | [46.50, 61.80] | 0.525 | 57.00 | [47.40, 66.60] | 0.630 | 53.30 | [48.70, 58.00] | 0.555 | 54.80 | [49.10, 60.60] | 0.560 |
2 | 61.30 | [55.70, 66.80] | 0.663 | 66.10 | [58.40, 73.70] | 0.695 | 71.70 | [63.10, 80.20] | 0.796 | 72.20 | [61.60, 82.70] | 0.813 | |
3 | 57.50 | [50.90, 64.10] | 0.629 | 58.70 | [49.00, 68.30] | 0.634 | 55.00 | [43.90, 66.10] | 0.572 | 55.70 | [47.40, 64.00] | 0.599 | |
4 | 62.30 | [55.30, 69.30] | 0.689 | 66.30 | [59.60, 73.00] | 0.739 | 58.90 | [52.50, 65.30] | 0.636 | 64.60 | [57.80, 71.50] | 0.688 | |
5 | 92.00 | [83.00,101.0] | 0.980 | 90.80 | [84.20, 97.40] | 0.977 | 93.80 | [90.00, 97.70] | 0.993 | 87.20 | [80.50, 93.80] | 0.965 | |
6 | 55.60 | [44.60, 66.60] | 0.582 | 57.90 | [47.80, 68.00] | 0.605 | 54.00 | [45.60, 62.40] | 0.542 | 56.20 | [46.80, 65.50] | 0.550 | |
7 | 63.20 | [52.00, 74.30] | 0.676 | 68.30 | [60.70, 75.90] | 0.771 | 76.20 | [66.40, 86.00] | 0.845 | 82.20 | [76.50, 87.80] | 0.898 | |
8 | 57.30 | [44.50, 70.00] | 0.583 | 55.90 | [45.20, 66.60] | 0.563 | 61.30 | [52.00, 70.60] | 0.647 | 62.30 | [51.60, 73.00] | 0.680 | |
9 | 55.60 | [44.00, 67.20] | 0.600 | 61.80 | [53.50, 70.10] | 0.661 | 71.80 | [65.90, 77.80] | 0.788 | 74.70 | [69.60, 79.70] | 0.810 |
To further verify that SSL can effectively improve the classification performance, the experiments are conducted under different percentages of the labeled training samples. The representation learning ability of the pretext task is evaluated by the classification results of the downstream classification task.
Figures 8 and 9 show the overall accuracy trends of the nine subjects as the percentage of the labeled training samples increases. The accuracies of the TRMINet method are higher and increase more obviously than the Base method as the labeled training samples increase on most subjects. This shows that the proposed pretext task can effectively learn MI-EEG representations, and SSL can effectively improve classification performance under a small number of the labeled training samples.
In this study, we propose an SSL method, called Temporal Rearrange, which exploits the temporal characteristics of MI-EEGs to learn the pretext network by identifying the original and rearranged MI-EEGs. The network learned by the pretext task is used to initialize that of the downstream task. The experimental results show that the proposed method can improve the classification performance under a small number of the labeled training samples. It is indicated that the proposed method can alleviate the performance degradation caused by insufficient labeled training samples. Therefore, it is possible to improve the classification accuracy of the MI-based BCI system and reduce the burden of labeling MI-EEGs. In the future, transfer learning and subjectwise strategy will be employed to further improve the classification performance of the network from multiple subjects.
The work was supported by the High-level Talents Fund of Hubei University of Technology under grant No. GCRC2020016, Key Laboratory of Brain Machine Collaborative Intelligence of Zhejiang Province under grant No. 2020E10010-02, Natural Science Foundation of Hubei Province under grant No. 2021CFB282, and Open Funding Project of the State Key Laboratory of Biocatalysis and Enzyme Engineering No. SKLBEE2021020 and SKLBEE2020020.
The authors declare there is no conflict of interest.
[1] | P. Sandheep, S. Vineeth, M. Poulose, D. P. Subha, Performance analysis of deep learning CNN in classification of depression EEG signals, in TENCON 2019 - 2019 IEEE Region 10 Conference (TENCON), IEEE, (2019), 1339–1344. https://doi.org/10.1109/TENCON.2019.8929254 |
[2] |
S. M. Usman, S. Khalid, R. Akhtar, Z. Bortolotto, Z. Bashir, H. Qiu, Using scalp EEG and intracranial EEG signals for predicting epileptic seizures: Review of available methodologies, Seizure, 71 (2019), 258–269. https://doi.org/10.1016/j.seizure.2019.08.006 doi: 10.1016/j.seizure.2019.08.006
![]() |
[3] |
F. Lotte, L. Bougrain, A. Cichocki, M. Clerc, M. Congedo, A. Rakotomamonjy, et al., A review of classification algorithms for EEG-based brain-computer interfaces: a 10 year update, J. Neural Eng., 15 (2018), 031005. https://doi.org/10.1088/1741-2552/aab2f2 doi: 10.1088/1741-2552/aab2f2
![]() |
[4] | B. J. Edelman, J. Meng, D. Suma, C. A. Zurn, E. Nagarajan, B. Baxter, et al., Noninvasive neuroimaging enhances continuous neural tracking for robotic device control, Sci. Rob., 4 (2019). https://doi.org/10.1126/scirobotics.aaw6844 |
[5] |
Q. He, S. Du, Y. Zhang, G. Jiang, P. Xie, Classification of motor imagery based on single-channel frame and multi-channel frame, Yi Qi Yi Biao Xue Bao/Chin. J. Sci. Instrum., 39 (2018), 20–29. https://doi.org/10.19650/j.cnki.cjsi.J1803816 doi: 10.19650/j.cnki.cjsi.J1803816
![]() |
[6] |
J. M¨uller-Gerking, G. Pfurtscheller, H. Flyvbjerg, Designing optimal spatial filters for single-trial EEG classification in a movement task, Clin. Neurophysiol., 110 (1999), 787–798. https://doi.org/10.1016/S1388-2457(98)00038-8 doi: 10.1016/S1388-2457(98)00038-8
![]() |
[7] | D. Huang, P. Lin, D. Fei, X. Chen, O. Bai, Decoding human motor activity from EEG single trials for a discrete two-dimensional cursor control, J. Neural Eng., 6 (2009). https://doi.org/10.1088/1741-2560/6/4/046005 |
[8] | R. Chatterjee and T. Bandyopadhyay, EEG based motor imagery classification using SVM and MLP, in 2016 2nd International Conference on Computational Intelligence and Networks (CINE), (2016), 84–89. https://doi.org/10.1109/CINE.2016.22 |
[9] | K. He, X. Zhang, S. Ren, J. Sun, Deep residual learning for image recognition, in 2016 IEEE Conference on Computer Vision and Pattern 2016 IEEE Conference on Computer Vision and Pattern Recognition (CVPR), IEEE, (2016), 770–778. https://doi.org/10.1109/CVPR.2016.90 |
[10] | M. Tan and Q. V. Le, Efficientnet: Rethinking model scaling for convolutional neural networks, in Proceedings of the 36th International Conference on Machine Learning ICML (eds. K. Chaudhuri and R. Salakhutdinov), 97 (2019), 6105–6114. Available from: http://proceedings.mlr.press/v97/tan19a/tan19a.pdf. |
[11] | X. Liu, Y. Shen, J. Liu, J. Yang, P. Xiong, F. Lin, Parallel spatial-temporal self-attention cnn-based motor imagery classification for bci, Front. Neurosci., 14 (2020). https://doi.org/10.3389/fnins.2020.587520 |
[12] | P. Autthasan, R. Chaisaen, T. Sudhawiyangkul, S. Kiatthaveephong, P. Rangpong, N. Dilokthanakul, et al., MIN2net: End-to-end multi-task learning for subject-independent motor imagery EEG classification, IEEE Trans. Biomed. Eng., 2021. https://doi.org/10.1109/TBME.2021.3137184 |
[13] |
R. T. Schirrmeister, J. T. Springenberg, L. D. J. Fiederer, M. Glasstetter, K. Eggensperger, M. Tangermann, et al., Deep learning with convolutional neural networks for EEG decoding and visualization, Hum. Brain Mapp., 38 (2017), 5391–5420. https://doi.org/10.1002/hbm.23730 doi: 10.1002/hbm.23730
![]() |
[14] | S. Ioffe and C. Szegedy, Batch normalization: Accelerating deep network training by reducing internal covariate shift, in Proceedings of the 32nd International Conference on Machine Learning ICML (eds. F. R. Bach and D. M. Blei), 37 (2015), 448–456. https://doi.org/10.48550/arXiv.1502.03167 |
[15] | D. Clevert, T. Unterthiner, S. Hochreiter, Fast and accurate deep network learning by exponential linear units (elus), preprint, arXiv: 1511.07289. |
[16] | H. Yang, S. Sakhavi, K. K. Ang, C. Guan, On the use of convolutional neural networks and augmented CSP features for multi-class motor imagery of EEG signals classification, in 2015 37th Annual International Conference of the IEEE Engineering in Medicine and Biology Society (EMBC), IEEE, (2015), 2620–2623. https://doi.org/10.1109/EMBC.2015.7318929 |
[17] | X. An, D. Kuang, X. Guo, Y. Zhao, L. He, A deep learning method for classification of EEG data based on motor imagery, in Intelligent Computing in Bioinformatics (eds. D. S. Huang, K. Han, M. Gromiha), ICIC 2014, Lecture Notes in Computer Science, Springer, 8590 (2014), 203–210. https://doi.org/10.1007/978-3-319-09330-7_25 |
[18] |
M. Li, J. Han, J. Yang, Automatic feature extraction and fusion recognition of motor imagery EEG using multilevel multiscale CNN, Med. Biol. Eng. Comput., 59 (2021), 2037–2050. https://doi.org/10.1007/s11517-021-02396-w doi: 10.1007/s11517-021-02396-w
![]() |
[19] |
V. J. Lawhern, A. J. Solon, N. R. Waytowich, S. M. Gordon, C. P. Hung, B. J. Lance, EEGNet: A compact convolutional neural network for EEG-based brain–computer interfaces, J. Neural Eng., 15 (2018), 056013. https://doi.org/10.1088/1741-2552/aace8c doi: 10.1088/1741-2552/aace8c
![]() |
[20] | R. K. Malhotra and A. Y. Avidan, Sleep stages and scoring technique, in Atlas of Sleep Medicine, (2013), 77–99. https://doi.org/10.1016/B978-1-4557-1267-0.00003-5 |
[21] | D. Hendrycks, M. Mazeika, S. Kadavath, D. Song, Using self-supervised learning can improve model robustness and uncertainty, in 33rd Conference on Neural Information Processing Systems (NeurIPS 2019), Vancouver, Canada, (2019), 15637–15648. Available from: https://papers.nips.cc/paper/2019/file/a2b15837edac15df90721968986f7f8e-Paper.pdf. |
[22] | M. Noroozi and P. Favaro, Unsupervised learning of visual representations by solving jigsaw puzzles, in Computer Vision - ECCV 2016 - 14th European Conference, Lecture Notes in Computer Science, Springer, (2016), 69–84. https://doi.org/10.1007/978-3-319-46466-4_5 |
[23] | Y. Li, J. Zeng, S. Shan, X. Chen, Self-supervised representation learning from videos for facial action unit detection, in 2019 IEEE/CVF Conference on Computer Vision and Pattern Recognition (CVPR), IEEE, (2019), 10924–10933. https://doi.org/10.1109/CVPR.2019.01118 |
[24] | H. J. Banville, G. Moffat, I. Albuquerque, D. Engemann, A. Hyvärinen, A. Gramfort, Self-supervised representation learning from electroencephalography signals, in 2019 IEEE 29th International Workshop on Machine Learning for Signal Processing (MLSP), IEEE, (2019), 1–6. https://doi.org/10.1109/MLSP.2019.8918693 |
[25] | A. Krizhevsky, I. Sutskever, G. E. Hinton, Imagenet classification with deep convolutional neural networks, in Advances in Neural Information Processing Systems 25: 26th Annual Conference on Neural Information Processing Systems 2012 (eds. P. L. Bartlett, F. C. N. Pereira, C. J. C. Burges, L. Bottou, K. Q. Weinberger), (2012), 1106–1114. https://doi.org/10.1145/3065386 |
[26] | C. Szegedy, W. Liu, Y. Jia, P. Sermanet, S. E. Reed, D. Anguelov, et al., Going deeper with convolutions, in 2015 IEEE Conference on Computer Vision and Pattern Recognition (CVPR), IEEE, (2015), 1–9. https://doi.org/10.1109/CVPR.2015.7298594 |
[27] | V. Nair, G. E. Hinton, Rectified linear units improve restricted boltzmann machines, in Proceedings of the 27th International Conference on Machine Learning (eds. J. Fürnkranz and T. Joachims), (2010), 807–814. Available from: https://icml.cc/Conferences/2010/papers/432.pdf. |
[28] | A. G. Howard, M. Zhu, B. Chen, D. Kalenichenko, W. Wang, T. Weyand, et al., Mobilenets: Efficient convolutional neural networks for mobile vision applications, preprint, arXiv: 1704.04861. |
[29] | J. Hu, L. Shen, G. Sun, Squeeze-and-excitation networks, in 2018 IEEE Conference on Computer Vision and Pattern Recognition CVPR, (2018), 7132–7141. Available from: https://openaccess.thecvf.com/content_cvpr_2018/papers/Hu_Squeeze-and-Excitation_Networks_CVPR_2018_paper.pdf. |
[30] |
G. Pfurtscheller and F. H. Lopes da Silva, Event-related EEG/MEG synchronization and desynchronization: basic principles, Clin. Neurophysiol., 110 (1999), 1842–1857. https://doi.org/10.1016/s1388-2457(99)00141-8 doi: 10.1016/s1388-2457(99)00141-8
![]() |
[31] | E. Dong, G. Zhu, C. Chen, Classification of four categories of EEG signals based on relevance vector machine, in 2017 IEEE International Conference on Mechatronics and Automation (ICMA), (2017), 1024–1029. https://doi.org/10.1109/ICMA.2017.8015957 |
[32] |
Y. Meirovitch, H. Harris, E. Dayan, A. Arieli, T. Flash, Alpha and beta band event-related desynchronization reflects kinematic regularities, J. Neurosci., 35 (2015), 1627–1637. https://doi.org/10.1523/jneurosci.5371-13.2015 doi: 10.1523/jneurosci.5371-13.2015
![]() |
1. | Yixuan Qiu, Feng Lin, Weitong Chen, Miao Xu, Pre-training in Medical Data: A Survey, 2023, 2731-538X, 10.1007/s11633-022-1382-8 | |
2. | Shuo Zhang, Yonghao Ren, Jing Wang, Bo Song, Runzhi Li, Yuming Xu, GSTCNet: Gated spatio-temporal correlation network for stroke mortality prediction, 2022, 19, 1551-0018, 9966, 10.3934/mbe.2022465 | |
3. | Hu Cunlin, Ye Ye, Xie Nenggang, Self-supervised motor imagery EEG recognition model based on 1-D MTCNN-LSTM network, 2024, 21, 1741-2560, 036014, 10.1088/1741-2552/ad48bc | |
4. | In-Nea Wang, Choel-Hui Lee, Hakseung Kim, Dong-Joo Kim, Negative-Sample-Free Contrastive Self-Supervised Learning for Electroencephalogram-Based Motor Imagery Classification, 2024, 12, 2169-3536, 132714, 10.1109/ACCESS.2024.3459866 | |
5. | Aurora Saibene, Hafez Ghaemi, Eda Dagdevir, Deep learning in motor imagery EEG signal decoding: A Systematic Review, 2024, 610, 09252312, 128577, 10.1016/j.neucom.2024.128577 | |
6. | Tianhui Sha, Yikai Zhang, Yong Peng, Wanzeng Kong, Semi-supervised regression with adaptive graph learning for EEG-based emotion recognition, 2023, 20, 1551-0018, 11379, 10.3934/mbe.2023505 | |
7. | Federico Del Pup, Manfredo Atzori, Applications of Self-Supervised Learning to Biomedical Signals: A Survey, 2023, 11, 2169-3536, 144180, 10.1109/ACCESS.2023.3344531 | |
8. | Sergio Kazatzidis, Siamak Mehrkanoon, 2024, A novel dual-stream time-frequency contrastive pretext tasks framework for sleep stage classification, 979-8-3503-5931-2, 1, 10.1109/IJCNN60899.2024.10650151 | |
9. | Pierre Guetschel, Sara Ahmadi, Michael Tangermann, Review of deep representation learning techniques for brain–computer interfaces, 2024, 21, 1741-2560, 061002, 10.1088/1741-2552/ad8962 | |
10. | Huanyu Wu, Siyang Li, Dongrui Wu, Motor Imagery Classification for Asynchronous EEG-Based Brain–Computer Interfaces, 2024, 32, 1534-4320, 527, 10.1109/TNSRE.2024.3356916 | |
11. | Ilya Mikheev, Helen Steiner, Olga Martynova, Detecting cognitive traits and occupational proficiency using EEG and statistical inference, 2024, 14, 2045-2322, 10.1038/s41598-024-55163-w | |
12. | Wonjun Ko, Seungwoo Jeong, Sa-Kwang Song, Heung-Il Suk, EEG-Oriented Self-Supervised Learning With Triple Information Pathways Network, 2024, 54, 2168-2267, 6495, 10.1109/TCYB.2024.3410844 | |
13. | Zara Cook, Chengzong Zhao, Livia Murray, Jivan Kesan, Nabil Belacel, Sam M. Doesburg, George Medvedev, Vasily A. Vakorin, Pengcheng Xi, 2024, Decoding Brain Age: A Self-Supervised Graph Neural Network Framework for EEG Analysis, 979-8-3503-6351-7, 1, 10.1109/SENSORS60989.2024.10784646 | |
14. | Heng Li, Zhongwei Lu, Yun Mo, Bao Feng, Tianyou Yu, A two-stage feature redundancy minimization methodology framework for motor imagery EEG classification, 2025, 1573-7721, 10.1007/s11042-025-20605-8 | |
15. | Wonjun Ko, Jeongwon Choe, Jonggu Kang, SLEEP-SAFE: Self-Supervised Learning for Estimating Electroencephalogram Patterns With Structural Analysis of Fatigue Evidence, 2025, 13, 2169-3536, 35805, 10.1109/ACCESS.2025.3545094 |
Layer | Operator | Output Channels | Kernel Size | Stride |
1 | Conv & BN | 8 | (1, 64) | 1 |
2 | Depthwise Conv & BN & elu | 16 | (3, 1) | 1 |
3 | Avgpool & Dropout | 16 | (1, 4) | 4 |
4 | Depthwise Conv | 16 | (1, 16) | 1 |
5 | Separable Conv & BN & elu | 16 | (1, 1) | 1 |
6 | AvgPool & Dropout | 16 | (1, 8) | 8 |
Abbreviations | Explanation |
MI-EEG | Motor Imagery EEG |
SSL | Self-supervised Learning |
BCI | Brain-Computer Interface |
CSP | Common Spatial Pattern |
SLT | Surface Laplace Transform |
PSD | Power Spectral Density |
SVM | Support Vector Machine |
BN | Batch Normalisation |
ELU | Exponential Linear Units |
CNN | Convolutional Neural Network |
ACSP | Augmented Common Spatial Pattern |
TCAE | Twin-Cycle Autoencoder |
TRMINet | Temporal Rearrange Motor Imagery Network |
MBConv | MobileNet Convolution |
SE | Squeeze-and-Excitation |
TR | Temporal Rearrange |
ERS | Event-Related Synchronization |
ERD | Event-Related Desynchronization |
ICA | Independent Component Analysis |
ACC | Accuracy |
AUC | Area Under the Curve |
CI | Confidence Intervals |
Methods | ResNet | MobileNet | EfficientNet | DeepConvNet | ShallowConvNet | EEGNet | |||||||
Metrics | ACC | AUC | ACC | AUC | ACC | AUC | ACC | AUC | ACC | AUC | ACC | AUC | |
Data sets 1 (BCICIV) | a | 52.80 | 0.550 | 62.40 | 0.651 | 62.25 | 0.663 | 70.40 | 0.819 | 68.80 | 0.764 | 75.60 | 0.817 |
b | 55.20 | 0.574 | 50.40 | 0.521 | 56.00 | 0.583 | 67.00 | 0.763 | 73.20 | 0.820 | 71.60 | 0.786 | |
c | 53.60 | 0.574 | 52.80 | 0.540 | 60.80 | 0.622 | 51.40 | 0.647 | 64.40 | 0.732 | 70.60 | 0.765 | |
d | 63.60 | 0.724 | 64.80 | 0.728 | 78.00 | 0.844 | 63.40 | 0.870 | 88.80 | 0.945 | 91.80 | 0.977 | |
e | 67.34 | 0.628 | 53.06 | 0.520 | 79.59 | 0.884 | 92.20 | 0.994 | 95.40 | 0.995 | 96.40 | 0.993 | |
f | 66.50 | 0.699 | 58.50 | 0.663 | 64.40 | 0.714 | 63.00 | 0.786 | 72.60 | 0.793 | 80.20 | 0.874 | |
g | 69.50 | 0.733 | 64.80 | 0.736 | 74.00 | 0.826 | 78.80 | 0.904 | 82.60 | 0.896 | 85.20 | 0.910 | |
Data set IVa (BCICIII) | aa | 73.80 | 0.793 | 60.50 | 0.707 | 64.30 | 0.661 | 58.10 | 0.785 | 78.80 | 0.877 | 80.00 | 0.893 |
al | 91.70 | 0.966 | 82.10 | 0.944 | 83.90 | 0.952 | 75.70 | 0.964 | 95.40 | 0.986 | 95.70 | 0.987 | |
Data sets 2b (BCICIV) | 1 | 50.30 | 0.510 | 50.00 | 0.508 | 50.80 | 0.507 | 50.30 | 0.502 | 51.80 | 0.528 | 71.20 | 0.748 |
2 | 69.50 | 0.775 | 60.50 | 0.664 | 70.30 | 0.802 | 74.30 | 0.872 | 76.70 | 0.860 | 77.30 | 0.871 | |
3 | 58.00 | 0.613 | 55.70 | 0.580 | 59.30 | 0.629 | 57.50 | 0.601 | 61.80 | 0.649 | 63.00 | 0.682 | |
4 | 59.80 | 0.636 | 55.20 | 0.567 | 69.20 | 0.759 | 70.30 | 0.793 | 70.80 | 0.783 | 84.30 | 0.901 | |
5 | 95.20 | 0.993 | 75.50 | 0.837 | 96.20 | 0.994 | 93.70 | 0.957 | 99.10 | 0.999 | 98.90 | 0.999 | |
6 | 59.30 | 0.645 | 54.70 | 0.586 | 58.70 | 0.630 | 61.20 | 0.653 | 58.80 | 0.636 | 63.70 | 0.657 | |
7 | 80.00 | 0.881 | 62.00 | 0.667 | 80.20 | 0.872 | 85.00 | 0.920 | 86.00 | 0.912 | 88.20 | 0.924 | |
8 | 62.10 | 0.672 | 53.90 | 0.529 | 68.40 | 0.754 | 62.00 | 0.701 | 65.00 | 0.715 | 64.90 | 0.690 | |
9 | 63.70 | 0.705 | 51.50 | 0.549 | 66.30 | 0.701 | 70.00 | 0.762 | 76.00 | 0.824 | 73.80 | 0.793 |
Subjects | Base (EEGNet) | TRMINet (EEGNet) | Base (ShallowConvNet) | TRMINet (ShallowConvNet) | |||||||||
ACC | ACC CI | AUC | ACC | ACC CI | AUC | ACC | ACC CI | AUC | ACC | ACC CI | AUC | ||
Data sets 1 (BCICIV) | a | 53.20 | [46.10, 60.30] | 0.725 | 66.40 | [60.00, 72.80] | 0.741 | 64.20 | [57.30, 71.10] | 0.708 | 65.80 | [54.90, 76.70] | 0.739 |
b | 56.90 | [52.60, 61.20] | 0.649 | 60.40 | [54.50, 66.50] | 0.675 | 63.60 | [50.90, 76.30] | 0.712 | 65.00 | [52.00, 78.00] | 0.740 | |
c | 49.20 | [43.80, 54.50] | 0.561 | 55.20 | [50.30, 60.10] | 0.605 | 60.20 | [48.60, 71.80] | 0.651 | 61.00 | [50.30, 71.70] | 0.703 | |
d | 62.20 | [54.70, 69.70] | 0.740 | 75.80 | [64.30, 87.20] | 0.826 | 86.20 | [77.00, 95.40] | 0.938 | 84.40 | [77.20, 91.60] | 0.901 | |
e | 86.70 | [78.90, 94.40] | 0.946 | 91.20 | [84.30, 98.10] | 0.923 | 94.90 | [87.90,102.0] | 0.994 | 94.80 | [87.70,102.0] | 0.991 | |
f | 53.00 | [45.40, 60.50] | 0.648 | 68.40 | [61.30, 75.40] | 0.752 | 65.40 | [55.20, 75.60] | 0.732 | 66.00 | [58.20, 73.80] | 0.754 | |
g | 65.20 | [57.10, 73.30] | 0.814 | 75.80 | [67.10, 84.50] | 0.856 | 71.20 | [55.90, 86.50] | 0.820 | 75.00 | [59.60, 90.40] | 0.821 | |
Data set IVa (BCICIII) | aa | 61.40 | [55.20, 67.60] | 0.675 | 64.30 | [60.50, 68.10] | 0.755 | 57.90 | [46.20, 69.50] | 0.732 | 68.50 | [60.10, 76.90] | 0.785 |
al | 62.00 | [59.20, 64.70] | 0.728 | 71.10 | [65.70, 76.40] | 0.837 | 91.10 | [84.10, 98.10] | 0.975 | 91.30 | [87.90, 94.60] | 0.964 | |
Data sets 2b (BCICIV) | 1 | 54.20 | [46.50, 61.80] | 0.525 | 57.00 | [47.40, 66.60] | 0.630 | 53.30 | [48.70, 58.00] | 0.555 | 54.80 | [49.10, 60.60] | 0.560 |
2 | 61.30 | [55.70, 66.80] | 0.663 | 66.10 | [58.40, 73.70] | 0.695 | 71.70 | [63.10, 80.20] | 0.796 | 72.20 | [61.60, 82.70] | 0.813 | |
3 | 57.50 | [50.90, 64.10] | 0.629 | 58.70 | [49.00, 68.30] | 0.634 | 55.00 | [43.90, 66.10] | 0.572 | 55.70 | [47.40, 64.00] | 0.599 | |
4 | 62.30 | [55.30, 69.30] | 0.689 | 66.30 | [59.60, 73.00] | 0.739 | 58.90 | [52.50, 65.30] | 0.636 | 64.60 | [57.80, 71.50] | 0.688 | |
5 | 92.00 | [83.00,101.0] | 0.980 | 90.80 | [84.20, 97.40] | 0.977 | 93.80 | [90.00, 97.70] | 0.993 | 87.20 | [80.50, 93.80] | 0.965 | |
6 | 55.60 | [44.60, 66.60] | 0.582 | 57.90 | [47.80, 68.00] | 0.605 | 54.00 | [45.60, 62.40] | 0.542 | 56.20 | [46.80, 65.50] | 0.550 | |
7 | 63.20 | [52.00, 74.30] | 0.676 | 68.30 | [60.70, 75.90] | 0.771 | 76.20 | [66.40, 86.00] | 0.845 | 82.20 | [76.50, 87.80] | 0.898 | |
8 | 57.30 | [44.50, 70.00] | 0.583 | 55.90 | [45.20, 66.60] | 0.563 | 61.30 | [52.00, 70.60] | 0.647 | 62.30 | [51.60, 73.00] | 0.680 | |
9 | 55.60 | [44.00, 67.20] | 0.600 | 61.80 | [53.50, 70.10] | 0.661 | 71.80 | [65.90, 77.80] | 0.788 | 74.70 | [69.60, 79.70] | 0.810 |
Layer | Operator | Output Channels | Kernel Size | Stride |
1 | Conv & BN | 8 | (1, 64) | 1 |
2 | Depthwise Conv & BN & elu | 16 | (3, 1) | 1 |
3 | Avgpool & Dropout | 16 | (1, 4) | 4 |
4 | Depthwise Conv | 16 | (1, 16) | 1 |
5 | Separable Conv & BN & elu | 16 | (1, 1) | 1 |
6 | AvgPool & Dropout | 16 | (1, 8) | 8 |
Abbreviations | Explanation |
MI-EEG | Motor Imagery EEG |
SSL | Self-supervised Learning |
BCI | Brain-Computer Interface |
CSP | Common Spatial Pattern |
SLT | Surface Laplace Transform |
PSD | Power Spectral Density |
SVM | Support Vector Machine |
BN | Batch Normalisation |
ELU | Exponential Linear Units |
CNN | Convolutional Neural Network |
ACSP | Augmented Common Spatial Pattern |
TCAE | Twin-Cycle Autoencoder |
TRMINet | Temporal Rearrange Motor Imagery Network |
MBConv | MobileNet Convolution |
SE | Squeeze-and-Excitation |
TR | Temporal Rearrange |
ERS | Event-Related Synchronization |
ERD | Event-Related Desynchronization |
ICA | Independent Component Analysis |
ACC | Accuracy |
AUC | Area Under the Curve |
CI | Confidence Intervals |
Methods | ResNet | MobileNet | EfficientNet | DeepConvNet | ShallowConvNet | EEGNet | |||||||
Metrics | ACC | AUC | ACC | AUC | ACC | AUC | ACC | AUC | ACC | AUC | ACC | AUC | |
Data sets 1 (BCICIV) | a | 52.80 | 0.550 | 62.40 | 0.651 | 62.25 | 0.663 | 70.40 | 0.819 | 68.80 | 0.764 | 75.60 | 0.817 |
b | 55.20 | 0.574 | 50.40 | 0.521 | 56.00 | 0.583 | 67.00 | 0.763 | 73.20 | 0.820 | 71.60 | 0.786 | |
c | 53.60 | 0.574 | 52.80 | 0.540 | 60.80 | 0.622 | 51.40 | 0.647 | 64.40 | 0.732 | 70.60 | 0.765 | |
d | 63.60 | 0.724 | 64.80 | 0.728 | 78.00 | 0.844 | 63.40 | 0.870 | 88.80 | 0.945 | 91.80 | 0.977 | |
e | 67.34 | 0.628 | 53.06 | 0.520 | 79.59 | 0.884 | 92.20 | 0.994 | 95.40 | 0.995 | 96.40 | 0.993 | |
f | 66.50 | 0.699 | 58.50 | 0.663 | 64.40 | 0.714 | 63.00 | 0.786 | 72.60 | 0.793 | 80.20 | 0.874 | |
g | 69.50 | 0.733 | 64.80 | 0.736 | 74.00 | 0.826 | 78.80 | 0.904 | 82.60 | 0.896 | 85.20 | 0.910 | |
Data set IVa (BCICIII) | aa | 73.80 | 0.793 | 60.50 | 0.707 | 64.30 | 0.661 | 58.10 | 0.785 | 78.80 | 0.877 | 80.00 | 0.893 |
al | 91.70 | 0.966 | 82.10 | 0.944 | 83.90 | 0.952 | 75.70 | 0.964 | 95.40 | 0.986 | 95.70 | 0.987 | |
Data sets 2b (BCICIV) | 1 | 50.30 | 0.510 | 50.00 | 0.508 | 50.80 | 0.507 | 50.30 | 0.502 | 51.80 | 0.528 | 71.20 | 0.748 |
2 | 69.50 | 0.775 | 60.50 | 0.664 | 70.30 | 0.802 | 74.30 | 0.872 | 76.70 | 0.860 | 77.30 | 0.871 | |
3 | 58.00 | 0.613 | 55.70 | 0.580 | 59.30 | 0.629 | 57.50 | 0.601 | 61.80 | 0.649 | 63.00 | 0.682 | |
4 | 59.80 | 0.636 | 55.20 | 0.567 | 69.20 | 0.759 | 70.30 | 0.793 | 70.80 | 0.783 | 84.30 | 0.901 | |
5 | 95.20 | 0.993 | 75.50 | 0.837 | 96.20 | 0.994 | 93.70 | 0.957 | 99.10 | 0.999 | 98.90 | 0.999 | |
6 | 59.30 | 0.645 | 54.70 | 0.586 | 58.70 | 0.630 | 61.20 | 0.653 | 58.80 | 0.636 | 63.70 | 0.657 | |
7 | 80.00 | 0.881 | 62.00 | 0.667 | 80.20 | 0.872 | 85.00 | 0.920 | 86.00 | 0.912 | 88.20 | 0.924 | |
8 | 62.10 | 0.672 | 53.90 | 0.529 | 68.40 | 0.754 | 62.00 | 0.701 | 65.00 | 0.715 | 64.90 | 0.690 | |
9 | 63.70 | 0.705 | 51.50 | 0.549 | 66.30 | 0.701 | 70.00 | 0.762 | 76.00 | 0.824 | 73.80 | 0.793 |
Subjects | Base (EEGNet) | TRMINet (EEGNet) | Base (ShallowConvNet) | TRMINet (ShallowConvNet) | |||||||||
ACC | ACC CI | AUC | ACC | ACC CI | AUC | ACC | ACC CI | AUC | ACC | ACC CI | AUC | ||
Data sets 1 (BCICIV) | a | 53.20 | [46.10, 60.30] | 0.725 | 66.40 | [60.00, 72.80] | 0.741 | 64.20 | [57.30, 71.10] | 0.708 | 65.80 | [54.90, 76.70] | 0.739 |
b | 56.90 | [52.60, 61.20] | 0.649 | 60.40 | [54.50, 66.50] | 0.675 | 63.60 | [50.90, 76.30] | 0.712 | 65.00 | [52.00, 78.00] | 0.740 | |
c | 49.20 | [43.80, 54.50] | 0.561 | 55.20 | [50.30, 60.10] | 0.605 | 60.20 | [48.60, 71.80] | 0.651 | 61.00 | [50.30, 71.70] | 0.703 | |
d | 62.20 | [54.70, 69.70] | 0.740 | 75.80 | [64.30, 87.20] | 0.826 | 86.20 | [77.00, 95.40] | 0.938 | 84.40 | [77.20, 91.60] | 0.901 | |
e | 86.70 | [78.90, 94.40] | 0.946 | 91.20 | [84.30, 98.10] | 0.923 | 94.90 | [87.90,102.0] | 0.994 | 94.80 | [87.70,102.0] | 0.991 | |
f | 53.00 | [45.40, 60.50] | 0.648 | 68.40 | [61.30, 75.40] | 0.752 | 65.40 | [55.20, 75.60] | 0.732 | 66.00 | [58.20, 73.80] | 0.754 | |
g | 65.20 | [57.10, 73.30] | 0.814 | 75.80 | [67.10, 84.50] | 0.856 | 71.20 | [55.90, 86.50] | 0.820 | 75.00 | [59.60, 90.40] | 0.821 | |
Data set IVa (BCICIII) | aa | 61.40 | [55.20, 67.60] | 0.675 | 64.30 | [60.50, 68.10] | 0.755 | 57.90 | [46.20, 69.50] | 0.732 | 68.50 | [60.10, 76.90] | 0.785 |
al | 62.00 | [59.20, 64.70] | 0.728 | 71.10 | [65.70, 76.40] | 0.837 | 91.10 | [84.10, 98.10] | 0.975 | 91.30 | [87.90, 94.60] | 0.964 | |
Data sets 2b (BCICIV) | 1 | 54.20 | [46.50, 61.80] | 0.525 | 57.00 | [47.40, 66.60] | 0.630 | 53.30 | [48.70, 58.00] | 0.555 | 54.80 | [49.10, 60.60] | 0.560 |
2 | 61.30 | [55.70, 66.80] | 0.663 | 66.10 | [58.40, 73.70] | 0.695 | 71.70 | [63.10, 80.20] | 0.796 | 72.20 | [61.60, 82.70] | 0.813 | |
3 | 57.50 | [50.90, 64.10] | 0.629 | 58.70 | [49.00, 68.30] | 0.634 | 55.00 | [43.90, 66.10] | 0.572 | 55.70 | [47.40, 64.00] | 0.599 | |
4 | 62.30 | [55.30, 69.30] | 0.689 | 66.30 | [59.60, 73.00] | 0.739 | 58.90 | [52.50, 65.30] | 0.636 | 64.60 | [57.80, 71.50] | 0.688 | |
5 | 92.00 | [83.00,101.0] | 0.980 | 90.80 | [84.20, 97.40] | 0.977 | 93.80 | [90.00, 97.70] | 0.993 | 87.20 | [80.50, 93.80] | 0.965 | |
6 | 55.60 | [44.60, 66.60] | 0.582 | 57.90 | [47.80, 68.00] | 0.605 | 54.00 | [45.60, 62.40] | 0.542 | 56.20 | [46.80, 65.50] | 0.550 | |
7 | 63.20 | [52.00, 74.30] | 0.676 | 68.30 | [60.70, 75.90] | 0.771 | 76.20 | [66.40, 86.00] | 0.845 | 82.20 | [76.50, 87.80] | 0.898 | |
8 | 57.30 | [44.50, 70.00] | 0.583 | 55.90 | [45.20, 66.60] | 0.563 | 61.30 | [52.00, 70.60] | 0.647 | 62.30 | [51.60, 73.00] | 0.680 | |
9 | 55.60 | [44.00, 67.20] | 0.600 | 61.80 | [53.50, 70.10] | 0.661 | 71.80 | [65.90, 77.80] | 0.788 | 74.70 | [69.60, 79.70] | 0.810 |