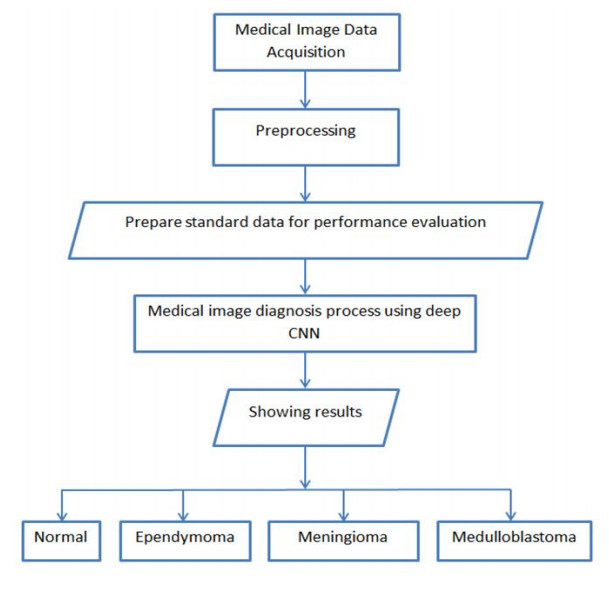
Citation: Bakhtyar Ahmed Mohammed, Muzhir Shaban Al-Ani. An efficient approach to diagnose brain tumors through deep CNN[J]. Mathematical Biosciences and Engineering, 2021, 18(1): 851-867. doi: 10.3934/mbe.2021045
[1] | Hassan Ali Khan, Wu Jue, Muhammad Mushtaq, Muhammad Umer Mushtaq . Brain tumor classification in MRI image using convolutional neural network. Mathematical Biosciences and Engineering, 2020, 17(5): 6203-6216. doi: 10.3934/mbe.2020328 |
[2] | Jiajun Zhu, Rui Zhang, Haifei Zhang . An MRI brain tumor segmentation method based on improved U-Net. Mathematical Biosciences and Engineering, 2024, 21(1): 778-791. doi: 10.3934/mbe.2024033 |
[3] | Zijian Wang, Yaqin Zhu, Haibo Shi, Yanting Zhang, Cairong Yan . A 3D multiscale view convolutional neural network with attention for mental disease diagnosis on MRI images. Mathematical Biosciences and Engineering, 2021, 18(5): 6978-6994. doi: 10.3934/mbe.2021347 |
[4] | Qian Wu, Yuyao Pei, Zihao Cheng, Xiaopeng Hu, Changqing Wang . SDS-Net: A lightweight 3D convolutional neural network with multi-branch attention for multimodal brain tumor accurate segmentation. Mathematical Biosciences and Engineering, 2023, 20(9): 17384-17406. doi: 10.3934/mbe.2023773 |
[5] | Vasileios E. Papageorgiou, Georgios Petmezas, Pantelis Dogoulis, Maxime Cordy, Nicos Maglaveras . Uncertainty CNNs: A path to enhanced medical image classification performance. Mathematical Biosciences and Engineering, 2025, 22(3): 528-553. doi: 10.3934/mbe.2025020 |
[6] | Song Yang, Huibin Wang, Hongmin Gao, Lili Zhang . Few-shot remote sensing scene classification based on multi subband deep feature fusion. Mathematical Biosciences and Engineering, 2023, 20(7): 12889-12907. doi: 10.3934/mbe.2023575 |
[7] | Hakan Özcan, Bülent Gürsel Emiroğlu, Hakan Sabuncuoğlu, Selçuk Özdoğan, Ahmet Soyer, Tahsin Saygı . A comparative study for glioma classification using deep convolutional neural networks. Mathematical Biosciences and Engineering, 2021, 18(2): 1550-1572. doi: 10.3934/mbe.2021080 |
[8] | Jun Gao, Qian Jiang, Bo Zhou, Daozheng Chen . Convolutional neural networks for computer-aided detection or diagnosis in medical image analysis: An overview. Mathematical Biosciences and Engineering, 2019, 16(6): 6536-6561. doi: 10.3934/mbe.2019326 |
[9] | Yurong Guan, Muhammad Aamir, Ziaur Rahman, Ammara Ali, Waheed Ahmed Abro, Zaheer Ahmed Dayo, Muhammad Shoaib Bhutta, Zhihua Hu . A framework for efficient brain tumor classification using MRI images. Mathematical Biosciences and Engineering, 2021, 18(5): 5790-5815. doi: 10.3934/mbe.2021292 |
[10] | Sonam Saluja, Munesh Chandra Trivedi, Ashim Saha . Deep CNNs for glioma grading on conventional MRIs: Performance analysis, challenges, and future directions. Mathematical Biosciences and Engineering, 2024, 21(4): 5250-5282. doi: 10.3934/mbe.2024232 |
Brain tumors, which are also called intracranial tumors, refer to abnormal masses of tissue where the growth of the cells is uncontrollable. It seems that the mechanisms which control normal cells fail to or do not check such abnormal tissue masses. There are over 150 different types of brain tumor, among which primary and metastatic tumors account for the major two groups [1]. In another classification, brain tumors are either malignant (cancerous) or benign (noncancerous).
Malignant tumors are characterized by their quick spread to other brain tissues, causing the patient's conditions to become worse [2]. Ependymoma (also called ependymal tumor) with a prevalence rate of 17.6% in adults is a common type of intradural tumor. Other common types of intradural/intramedullary tumors are meningiomas (38.8%) and nerve sheath tumors with adult prevalence rates of respectively 38.8 and 29.5% [3]. Although intradural extramedullary lesions happen outside the spinal cord, they are in close vicinity within the spinal thecal sac. These types of lesion are typically nerve sheath tumors or meningiomas. The distinction between medulloblastoma and ependymoma in children can guide the agammaessiveness which is used by the neurosurgeon to resect the tumor. However, accurate decisions about selecting an appropriate treatment protocol can be made through accurate detection of brain tumors [4].
Because of their different sizes, shapes, locations, and types in the brain, brain tumors are usually difficult and complicated to detect. In their early stages, brain tumors are even more difficult to detect partly because their size and resolution cannot be measured accurately. It is noteworthy that early detection of brain tumors while they are in formation process can increases the patient's chances for successful treatment. Therefore, it can be concluded that treatment of brain tumors is highly dependent on their early diagnosis. Tumor diagnosis is typically performed through a medical examination which is aided with magnetic imaging or computer tomography. Among the available approaches for diagnosis and evaluation of the brain, MRI imaging is a widely acceptable method which provides accurate brain images [5].
To diagnose and classify tumors from brain images, a technique was proposed which was labelled as convolutional neural networks (CNN). The convolutional neural network differs from normal neural network in that the former's channel can locally and automatically extract features from each image. The neurons with biases and weights included in this type of networks can be learned [6]. This diagnosis method was improved once a machine learning algorithm was added to it to be utilized for the purpose of feature extraction. The algorithm is a clustering algorithm which is applied to the images which are in turn applied to CNN. Since fatty masses are mistakenly regarded as tumors in some images and some tumors as fatty masses in some other images, it is necessary to extract the features of the images before they are applied to CNN. Feature extraction before applying the images to CNN can remarkably decrease medical error and increase diagnosis accuracy [7].
Different techniques have been proposed to improve the efficiency and accuracy of this method. For example, Xu et al. proposed using Image-Net for feature extraction in CNN method. It worked based on segmentation and classification of the features. Their proposed technique was found to have accuracy of 84% for segmentation and 97.5% for classification [8]. Moreover, Pan et al. used multiphase MRI images to compare the tumor grading performance of base neural networks and deep learning (DL) structures. They found out that compared to the neural networks, the network performance based on the specificity and sensitivity of CNN improved by 18% [9]. Furthermore, a deep learning-based supervised method was proposed by Samadi et al. for detection of synthetic aperture radar (SAR) image changes. Through this method, input images are used to provide a dataset of an appropriate data diversity and volume for training the deep belief network (DBN). Their proposed method proved the acceptability of deep learning-based algorithms for solving the problems with change detection [10]. The most recent fully automatic segmentation method for diagnosis of brain metastases through images include those proposed by Comelli et al and Stefano et al. In their proposed method, information obtained from previously studied fully automatic segmentation procedures for PET images is used in a system in order to detect patients with brain metastases who can respond to the treatment [11,12].
Given the significance of accurate diagnosis of brain tumors in making appropriate treatment decisions, the present study was carried out in order to develop and propose a systematic approach based on deep learning to detect brain tumors via medical image diagnosis process and according to the classes of ependymoma, meningioma, medulloblastoma, and normal brain.
The present study was carried out on the MRI data from 2015 to 2020 in order to use deep CNN to diagnose medical images which were obtained for the purpose of brain tumor diagnosis. For this purpose, a method was proposed using appropriate deep CNN architecture, and the training process was set to optimum situation properly with the dataset and necessary tools.
The necessary materials included annotated dataset to train the neural network; application platform to execute the deep learning codes, which depended on MATLAB and MATLAB deep learning toolbox; and hardware resources like graphical processing unit (GPU). All required processes were implemented on MATLAB through the version of R2019b student site. This system was applied using graphical processing unit (GPU) of NVIDIA GeForce GTX-1060 6GB with VRAM memory of 14.211 GB, processor Intel(R) Core (TM) i7-9700 kf (8 CPU) 3.6 GHz, and memory of 16.384 GB with 1200 GB memory storage.
Based on deep CNN method, the automate system was implemented according to the steps shown in Figure 1 below. As indicated in this figure, the system includes these elements: medical image data acquisition, preprocessing and data augmentation, and brain MRI image processing using deep CNN approach.
This system can be most appropriately implemented through deep CNN which is a supervised learning model in deep learning (DL) techniques. This model can perform the processes of feature extraction and classification simultaneously.
In supervised learning methods, an imperative process is collecting and managing and annotating raw data. It is the first and best process to create a good dataset however it is challenging. Image modalities are the most important factors to get appropriate dataset. The studied depended on using magnetic resonance imaging (MRI) image modality which is the most dominant one to exhibit the brain anomalies. The dataset composed of 1258 brain MRI images were obtained from Radiopedia database of 60 patients in positions of axial, sagittal, and coronal. The images were collected according to 15 patients for each the classes of normal brain, meningioma, ependymoma, and medulloblastoma. Normal brain class folder included 286 images, meningioma class folder included 380 MRI images, ependymoma class folder included 311 MRI images, and the medulloblastoma class folder included 281 MRI images. The diagnosis had been annotated by expert radiologists. Inside the dataset, the most significant images selected into the dataset manually according to their features.
Medical image data include noise, missing values, and inhomogeneous region of interest (ROI) and lack of annotated dataset; therefore, inaccurate diagnosis is likely to occur. In this regard, preprocessing and data augmentation can be used to enhance the performance of medical image processing. After data acquisition, preprocessing was performed, which involved three processes such as; resizing, de-noising, and data augmentation the images. A resizing function was used to resize the images to an equal size because every image must have the same size to implement the method as in this study set to 512*512. Another preprocessing process was de-noising which included using statistical method to enhance image quality. For this purpose, median filter was used to remove noise and preserve edges. It was used to solve the problem of missing values. In this step, as shown in Figure 2, the image quality was preserved in its natural condition.
Before the data augmentation the dataset distributed according to 70% of the dataset for training set, 20% of the dataset for test set, and 10% of the dataset for validation set. the first 70% of each classes images assign to training set after that 20% of them to the set of testing and the last portion for validation set.
After that data augmentation implement to solve the problem of lack of sufficient amount of training, testing, and validation sets using augmented image data store which increase probability of correct predictions because the method can manipulate augmented image positions. The most important data augmentation positions are rotation, scaling, reflection, translating, and cropping, as shown in Figure 3.
It involves the ready dataset to feed into the process of deep CNN.
Deep CNN or ConvNet perceives images as volumes. In general, the deep CNN method includes two major processes: feature extraction and classification. Architecture of the deep CNN method and training process are two very important parts of the deep CNN system.
Architecture of the deep CNN is composed of image input layer, convolution 2D layer, batch normalization layer, ReLU layer, max pooling 2D Layer for feature extraction process, and fully connected layer (FCN), Softmax layer, and classification layer for diagnosis process. Organizations of the deep CNN methods vary according to the type of the tasks, datasets, optimizers, learning rate, and the number of epochs in Figure 4.
Convolution layer is performed by multiplying input image by impulse response which is known as mask or filter. The processes are performed using a 2-dimensional (2D) convolution. As shown in Eq 1, it is performs by convolving both horizontal and vertical directions in 2D spatial domain.
y[m,n]=x[m,n]∗h[m,n]=k∑i=−kk∑j=−kx[i,j].h[m−i,n−j] | (1) |
Where x [m, n] represents 2D image, m, n; are vertical and horizontal 2-D image values, h [m, n] represents the kernel which provide weights, i and j span the dimensions of the kernel, and k is range.
Rectified linear unit (ReLU) layer is an activation function to make the characteristic map of the output that has a non-linear relationship. As shown in Eq 2.
f(x)=max(0,x) | (2) |
Batch normalization is a technique for training very deep neural networks and standardizing the inputs to a layer for each mini-batch. The algorithm distributed the classes by mini batch sizes of 32 which cause decrease run-time.
Max pooling rebates feature sizes even when zero padding increases the feature more stretched inside the image. Pooling is a way to take large images and shrink them down while preserving the most important information in them which represent as features. After all these processes, the features were prepared via the pooled feature maps. This process continues till the final max pooling layer in the last epochs.
Features refer to image pieces, which are extracted through deep CNN by comparing the images piece by piece. Each feature is like a mini-image which is as small as a 2-dimensional array of values. Feature extraction in every supervised learning method relies on datasets. In this regard, DL methods are intelligent methods to learn features from the training set. The most important features in the present study were the shape of brain tumor cells and their locations inside brain. Training set feature extraction which is the most important process to recognize features in Figure 5.
FCN is the final layer where the classification and predictions happen. Fully connected layers take the high-level filtered images and translate them into votes.
SoftMax is an activation function like sigmoid, tanh, and ReLU, typically applied on the output of the very last layer.
Classification Layer is the final layer of the neural network which is responsible of determining the number of the selected classes in the proposed approach.
This study relied on multi-classification to classify four brain situations which involve three types of tumors and a type of normal brain that are arranged in four classes, including ependymoma, meningioma, medulloblastoma, and normal brain with accuracy of 96% in Figure 6.
The learning process or training was performed through back-propagation process. The method changed the weighed values to closer image feature values according to the histologically similar tissues in image diagnosis based on the number of epochs to arrive to the optimum situation, which means lowest error rate.
Adam optimizer is a hyper parameter that controls how much to change the model in response to the estimated error every time the model weights are updated. Training the diagnosis process was highly dependent on Adam optimizer which is a combination of gradient descent with momentum, RMSPromp, and back-propagate loss for any image and updating the gradients. It is an adaptive learning rate method which calculates the individual learning rates to various parameters. These factors are important and effective to the training process because they enable the method to get maximum accuracy and the lowest learning rate. Figure 7 provides a representation of the accuracy according to the prepared dataset.
According to the epochs, the results showed that the feature learning accuracy of the proposed system increased remarkably with an increase in the number of epochs, such that feature learning accuracy was 47.02% with 1 epoch (under-fitting), while it became 96% when 15 epochs were involved (optimum). Moreover, overfitting (having more than 15 epochs) can lead to a decrease in feature learning accuracy. Therefore, using 15 epochs can lead to the highest level of accuracy in brain tumor diagnosis Table 1 and Figure 7.
No. of Epochs | Accuracy (%) |
Epoch 1 | 47.02 |
Epoch 2 | 62 |
Epoch 3 | 81.5 |
Epoch 4 | 87 |
Epoch 5 | 90.05 |
Epoch 6 | 92.5 |
Epoch 7 | 93.88 |
Epoch 8 | 93.02 |
Epoch 9 | 91.92 |
Epoch 10 | 92 |
Epoch 11 | 93.4 |
Epoch 12 | 94.6 |
Epoch 13 | 95.5 |
Epoch 14 | 95.8 |
Epoch 15 | 96 |
The results also showed that feature learning error of the proposed approach decreased remarkably with an increase in the number of epochs. As seen in Table 4 below, feature learning error was 47.02% with 1 epoch, while it reached 4% in the case of having 15 epochs. Therefore, using 15 epochs is the optimum method to diagnose brain tumors in Table 2 and Figure 8.
No. of Epochs | Error rate (%) |
Epoch 1 | 52.98 |
Epoch 2 | 38 |
Epoch 3 | 18.5 |
Epoch 4 | 13 |
Epoch 5 | 9.95 |
Epoch 6 | 7.5 |
Epoch 7 | 6.12 |
Epoch 8 | 6.98 |
Epoch 9 | 8.08 |
Epoch 10 | 8 |
Epoch 11 | 6.6 |
Epoch 12 | 5.4 |
Epoch 13 | 4.5 |
Epoch 14 | 4.2 |
Epoch 15 | 4 |
Moreover, as demonstrated by the results, feature learning sensitivity or recall in the proposed system had an outstanding increase with a rise in the number of epochs, such that the sensitivity was 47.02% in case of having 1 epoch, while it became 96% when there were 15 epochs. As a result, it can be stated that having 15 epochs can lead to the optimum sensitivity in brain tumor diagnosis Table 3 and Figure 9.
No. of Epochs | Sensitivity or Recall (%) |
Epoch 1 | 47.02 |
Epoch 2 | 62 |
Epoch 3 | 81.5 |
Epoch 4 | 87 |
Epoch 5 | 90.05 |
Epoch 6 | 92.5 |
Epoch 7 | 93.88 |
Epoch 8 | 93.02 |
Epoch 9 | 91.92 |
Epoch 10 | 92 |
Epoch 11 | 93.4 |
Epoch 12 | 94.6 |
Epoch 13 | 95.5 |
Epoch 14 | 95.8 |
Epoch 15 | 96 |
In order to assess the performance of the proposed approach, a confusion matrix table was employed. Confusion matrix tables were originally developed for the purpose of image analysis. As shown below, every confusion matrix is generally reliant on four situations.
a. True positive (TP): This condition involves those patients who had one of the three types of brain tumor or normal brain. It also predicted having the same situation.
b. True Negative (TN): This condition involves those patients who did not have one of the three types of brain tumor or normal brain. It also predicted that not having the same situation.
c. False positive (FP): This condition involves those patients who did not have one of the three types of brain tumor or normal brain, but the prediction showed that they had that situation.
d. False Negative (FN): This condition involves those patients who had one of the three types of brain tumor or normal brain. It also predicted not having the same situation.
According the employed confusion matrix and the automate system tested on 100 new MRI images which taken randomly different from the images dataset (25 images for each brain situation), it was seen that 96% of the images showed one of the three types of brain tumor or normal brain; therefore, the accuracy of the proposed system was TP = 96%. It was also seen that TN = 0, FP = 0, and FN = 4 in Table 4.
Predicted resultsActual results | Has one of the three types of brain tumor plus a normal brain | Does not have one of the three types of brain tumor plus a normal brain |
Has one of the three types of brain tumor plus a normal brain | 96 | 0 |
Does not have one of the three types of brain tumor plus a normal brain | 4 | 0 |
Green color: includes true positives; Blue color: includes true negatives; Orange color: includes false negatives; Violet color: includes false positives |
Error rate, accuracy, specificity, sensitivity, and perception were calculated according to the confusion matrix, as follows.
Errorrate(ERR)=FalsePositive+FalseNegativeTruePositive+TrueNegative+FalsePositive+FalseNegative | (3) |
Accuracy(ACC)=TruePositive+TrueNegativeTruePositive+TrueNegative+FalsePositive+FalseNegative | (4) |
Sensitivity(SN)(Recallortruepositiverate)=TruePositiveTruePositive+FalseNegative | (5) |
Specificity(SP)=TrueNegativeTrueNegative+FalsePositive | (6) |
Precision(PREC)(PositivePredictiveValue)=TruePositiveTruePositive+FalsePositive | (7) |
According to the above equations, ERR = 0.04, ACC = 0.96, SN = 0.96, SP = 0, and PREC = 1. It seems that ERR is reverse of ACC.
Diagnosis the MRI images revealed that the correct predictions of normal brain situation was true positive (TP) in 25 new images, ependymoma was true positive in 23 images and false negative (FN) in 2 images, medulloblastoma was true positive in 23 images and false negative (FN) in 2 images, and meningioma was true positive in 25 images Table 5.
Brain situation | Tested images | TP | TN | FP | FN |
Normal | 25 | 25 | 0 | 0 | 0 |
Eependymoma | 25 | 23 | 0 | 0 | 2 |
Medulloblastoma | 25 | 23 | 0 | 0 | 2 |
Meningioma | 25 | 25 | 0 | 0 | 0 |
With its broad continuum of practical uses, image classification is a process in which an input image is assigned a label which is chosen from a fixed set of categories. Although this process seems quite simple, it is one of the major issues in computer vision [13]. One of the most significant image classification techniques in convolutional neural networks (CNN) which is widely utilized in applications designed for object recognition. The efficiency of CNN can be attributed to its architecture which is created through different layers, including the input, convolution, pooling, fully connected, and output layers. For different purposes, different layouts of these layers are used to come up with different models [14].
Based on the confusion matrix, the proposed approach had an accuracy level of 96%. This level of diagnosis accuracy in deep CNN has been related to the fact that it integrates feature extraction process with feature classification process, which in turn leads to improvement in the prediction accuracy based on MRI images [15]. Compared to other methods and techniques, an automatic system that is prepared based on deep CNN method can bring about a remarkable rise in the accuracy of detection and classification of brain. It has also been indicated that despite requiring small time intervals (epochs) and employing a limited number of training samples, its performance is quite acceptable. Moreover, it allows a remarkable decrease in the process time [16].
One of the most efficient types of machine learning that has recently been introduced in deep learning in which learning occurs through deep-seated architectures. DNN architectures were developed from the same old nerve networks. The accuracy and remarkable performance of convolutional neural networks in different areas can be attributed to the fact that they are based on the input data and automatic feature engineering which enables them to perform without operators' interference. Automatic feature learning from the input data happens though deep learning of a set of nerve-based techniques [17]. Siar and Teshnehlab studied brain tumor diagnosis through deep neural network and machine learning algorithm and reported that CNN has an accuracy of 98.677% in appropriate classification of images [17]. In the present study, the process of image classification was assisted by fully connected softmax layers, which can be a justification for the obtained high accuracy of the proposed approach. In line with the results of the present study, Siar and Teshnehlab concluded that softmax classifier gives the best image classification accuracy to CNN approach [17].
In the present study, it was observed that the feature learning accuracy of the proposed system increased remarkably with an increase in the number of epochs, such that feature learning accuracy rose from 47.02 to 96% when the number of epochs increased from 1 to 15. Therefore, the optimum number of epochs in the current study was found to be 15. However, different studies have reported different numbers of epochs to be optimum. For example, Hossain et al observed that maximum accuracy for both training and validation was obtained using CNN with 9 epochs [18].
Decreasing the number of epochs leads to under-fitting, and increasing them results in overfitting, causing the level of feature learning accuracy of the proposed approach to decrease remarkably. In case of having more epochs than the necessary number to train a neural network model, a large number of patterns specific to sample data are learned by the model, limiting the model's capacity to perform well on new input data. In this case, although the model has a high level of accuracy on the training set (sample data), its accuracy on the test set decreases. In other words, overfitting causes the model to lose its generalization capacity over the training data [19].
The results of the present study also revealed that feature learning error of the proposed approach decreased remarkably from 50 to 4% with an increase in the number of epochs from 1 to 15. To mitigate overfitting and to increase the generalization capacity of the neural network and decrease its feature learning error, the model should be trained for an optimal number of epochs. In order to assess the performance of the model following each epoch of training, the model is validated by dedicating a part of training data to it. The number of epochs after which the model starts overfitting is controlled and looked over by monitoring the accuracy and loss on the training and validation sets [20,21].
The results of the present study demonstrated that feature learning sensitivity or recall of the proposed system would be at the most appropriate condition in case of 15 epochs, such that any decrease or increase at the number of epochs would cause a decrease in its sensitivity or recall. Similarly, John et al reported a remarkable increase in the sensitivity of feature learning sensitivity in their proposed system as a result of adequate number of epochs, which obviously increases a longer time for the model to perform the process [22]. It has also stated that employing an adequate number of epochs can lead to an outstanding decrease in the model's bias a remarkable increase in its sensitivity, performance, and specificity [23]. Similar to the present study, Gupta et al utilized deep CNN method for feature classification based on brain tumor MRI images. The results of their study showed that their utilized method had accuracy, sensitivity, and specificity rates of respectively 80, 84 and 92%. As a result, they recommend their proposed method for clinical uses [24].
The study indicates that the brain tumor diagnosis is the hardest diagnosis situation in Radiology so more than all of the other processes need auto-diagnose system. The results reveal that some factors have the most influences on decision making, these factors have effective role to get correct predictions. The factors consist of the dataset which represents as the feature bank, deep CNN method which involves feature extraction and classification processes together, training options which include the optimizer and number of epochs. The performance of Deep CNN in terms of its feature learning accuracy and sensitivity is highly dependent on the number of utilized epochs, which set to 15 in the present study. The whole automate system runtime did not subtend more than five minutes, representing real-time diagnosis.
Accurate diagnosis of brain tumor MRI images is highly useful in clinical diagnosis, in turn rising the patient's lifetime. In this regard, deep CNN is one of the most significant and effective models that can be employed in automated tumor diagnosis, with the classification capacity of hundreds of images per second. Therefore, radiologists are highly recommended to have working knowledge of deep CNN in order to be able to utilize these tools for clinical purposes.
The authors have no competing interests to declare.
[1] |
S. S. Khalsa, T. C. Hollon, R. A. Arja, Automated histologic diagnosis of CNS tumors with machine learning, CNS Oncol., 9 (2020), 56. doi: 10.2217/cns-2020-0003
![]() |
[2] | M. Karuna, A. Joshi, Automatic detection and severity analysis of brain tumors using gui in matlab, Int. J. Res. Eng. Technol., 10 (2013), 586-594. |
[3] |
Q. T. Ostrom, G. Cioffi, H. Gittleman, CBTRUS statistical report: Primary brain and other central nervous system tumors diagnosed in the United States in 2012-2016, Neurol. Oncol., 21 (2019), 1-100. doi: 10.1093/neuonc/noy189
![]() |
[4] |
E. M. Thompson, T. Hielscher, E. Bouffet, Prognostic value of medulloblastoma extent of resection after accounting for molecular subgroup: A retrospective integrated clinical and molecular analysis, Lancet Oncol., 17 (2016), 484-495. doi: 10.1016/S1470-2045(15)00581-1
![]() |
[5] | J. P. Poonam, Review of image processing techniques for automatic detection of tumor in human brain, Int. J. Comput. Sci. Mobile Comput., 2 (2013), 117-122. |
[6] | M. M. Ghazani, A. H. Phan, Graph convolutional neural networks for analysis of EEG signals, preprint, arXiv: 2006.14540. |
[7] | R. Bayot, T. Gonalves, A survey on object classification using convolutional neural networks, Artif. Int. Rev., 53 (2015), 5455-5516. |
[8] | X. Yan, Z. Jia, Y. Ai, F. Zhang, Deep convolutional activation features for large scale brain tumor histopathology image classification and segmentation, 2015 IEEE Int. Conf. Acoust. Speech Signal Process., (2015), 947-951. |
[9] | Y. Pan, Brain tumor grading based on neural networks and convolutional neural networks, 2015 37th Ann. Int. Conf. IEEE Eng. Med. Biol. Soc., (2015), 699-702. |
[10] |
F. Samadi, G. Akbarizadeh, H. Kaabi, Change detection in SAR Images using deep belief network: A new training approach based on morphological images, IET Image Process., 13 (2019), 2255-2264. doi: 10.1049/iet-ipr.2018.6248
![]() |
[11] | A. Comelli, A. Stefano, S. Bignardi, C. Coronnello, G. Russo, M. Sabini, et al., Tissue classification to support local active delineation of brain tumors, Ann. Conf. Med. Image Understanding Anal., (2020), 3-14. |
[12] | A. Stefano, A. Comelli, V. Bravatà, S. Barone, I. Daskalovski, G. Savoca, G., et al., A preliminary PET radiomics study of brain metastases using a fully automatic segmentation method, BMC Bioinformat., 21 (2019), 1-14. |
[13] | I. Shahzadi, T. B. Tang, F. Meriadeau, A. Quyyum. CNNLSTM: Cascaded framework for brain tumour classification, IEEE-EMBS Conf. Biomed. Eng. Sci., (2018), 633-637. |
[14] |
A. Çinar, M. Yildirim, Detection of tumors on brain MRI images using the hybrid convolutional neural network architecture, Med. Hypotheses, 139 (2020), 109684. doi: 10.1016/j.mehy.2020.109684
![]() |
[15] |
M. Malathi, P. Sinthia, Brain tumour segmentation using convolutional neural network with tensor flow, Asian.Pac. J. Cancer Prev., 20 (2019), 2095-2101. doi: 10.31557/APJCP.2019.20.7.2095
![]() |
[16] | R. Chelghoum, A. Ikhlef, A. Hameurlaine, Transfer learning using convolutional neural network architectures for brain tumor classification from MRI images, Artific. Int. Appl. Innovat., 583 (2020), 189-200. |
[17] | M. Siar, M. Teshnehlab, Brain tumor detection using deep neural network and machine learning algorithm, 9th Int. Conf. Comput. Knowl. Eng., 2019. |
[18] | T. Hossain, F. S. Shishir, M. Ashraf, Brain tumor detection using convolutional neural network. international conference on advances in science, Eng. Robot. Technol., 2019. |
[19] |
T. C. Hollon, B. Pandian, A. R. Adapa, Near real-time intraoperative brain tumor diagnosis using stimulated raman histology and deep neural networks, Nat. Med., 26 (2020), 52-58. doi: 10.1038/s41591-019-0715-9
![]() |
[20] |
B. N. Mostefa, S. Rachida, A. Mohamed, K. Rostom, Fully automatic brain tumor segmentation using end-to-end incremental deep neural networks in MRI images, Comput. Methods. Programs. Biomed., 166 (2018), 39-49. doi: 10.1016/j.cmpb.2018.09.007
![]() |
[21] |
S. S. Begum, D. R. Lakshmi. Combining optimal wavelet statistical texture and recurrent neural network for tumour detection and classification over MRI, Multimed. Tools. Appl, 79 (2020), 14009-14030. doi: 10.1007/s11042-020-08643-w
![]() |
[22] | D. John, H. Elad, S. Yoram, Adaptive subgradient methods for online learning and stochastic optimization, J. Mach. Learn. Res., 12 (2011), 2121-2159. |
[23] | L. Sun, S. Zhang, H. Chen, L. Luo, Brain tumor segmentation and survival prediction using multimodal MRI scans with deep learning, Front. Neurosci., 16 (2019). |
[24] | T. Gupta, T. K. Gandhi, R. K. Gupta, B. K. Panigrahi, Classification of patients with tumor using MR FLAIR images, Pattern Recogn Lett., 139 (2017), 112-117. |
1. | Yuting Xie, Fulvio Zaccagna, Leonardo Rundo, Claudia Testa, Raffaele Agati, Raffaele Lodi, David Neil Manners, Caterina Tonon, Convolutional Neural Network Techniques for Brain Tumor Classification (from 2015 to 2022): Review, Challenges, and Future Perspectives, 2022, 12, 2075-4418, 1850, 10.3390/diagnostics12081850 | |
2. | Mahsa Arabahmadi, Reza Farahbakhsh, Javad Rezazadeh, Deep Learning for Smart Healthcare—A Survey on Brain Tumor Detection from Medical Imaging, 2022, 22, 1424-8220, 1960, 10.3390/s22051960 | |
3. | Yi Lin, Yangfan Lan, Shunbo Wang, A method for evaluating the learning concentration in head-mounted virtual reality interaction, 2022, 1359-4338, 10.1007/s10055-022-00689-5 | |
4. | Xuelin Gu, Banghua Yang, Shouwei Gao, Lin Feng Yan, Ding Xu, Wen Wang, Application of bi-modal signal in the classification and recognition of drug addiction degree based on machine learning, 2021, 18, 1551-0018, 6926, 10.3934/mbe.2021344 | |
5. | Dillip Ranjan Nayak, Neelamadhab Padhy, Pradeep Kumar Mallick, Dilip Kumar Bagal, 2022, Chapter 37, 978-981-16-8738-9, 403, 10.1007/978-981-16-8739-6_37 | |
6. | Carla Pitarch, Gulnur Ungan, Margarida Julià-Sapé, Alfredo Vellido, Advances in the Use of Deep Learning for the Analysis of Magnetic Resonance Image in Neuro-Oncology, 2024, 16, 2072-6694, 300, 10.3390/cancers16020300 | |
7. | Surajit Das, Rajat Subhra Goswami, Review, Limitations, and future prospects of neural network approaches for brain tumor classification, 2023, 83, 1573-7721, 45799, 10.1007/s11042-023-17215-7 | |
8. | N. Veni, J. Manjula, 2023, Chapter 26, 978-981-19-8337-5, 319, 10.1007/978-981-19-8338-2_26 | |
9. | Huan Zhou, Pei-Ying Zhang, Xiao Zou, Jia Liu, Wen-Jie Wang, Chronic disease diagnosis model based on convolutional neural network and ensemble learning method, 2023, 9, 2055-2076, 10.1177/20552076231198643 | |
10. | Jayneet Jain, Mihika Kubadia, Monika Mangla, Prachi Tawde, 2024, Comparison of Transfer Learning Techniques to Classify Brain Tumours Using MRI Images, 144, 10.3390/engproc2023059144 | |
11. | Prachiti Pimple, Manoj Ashok Wakchaure, 2024, Implementing Deep Learning and Machine Learning Technologies In Brain Disease Diagnosis, 979-8-3503-7024-9, 1, 10.1109/ICCCNT61001.2024.10725229 | |
12. | Hiba A. Alahmed, Ghaida A. Al-Suhail, 2024, Exploring Transfer Learning Techniques for Brain Tumor Diagnosis in MRI Data, 979-8-3315-3355-7, 1, 10.1109/ICETI63946.2024.10777184 |
No. of Epochs | Accuracy (%) |
Epoch 1 | 47.02 |
Epoch 2 | 62 |
Epoch 3 | 81.5 |
Epoch 4 | 87 |
Epoch 5 | 90.05 |
Epoch 6 | 92.5 |
Epoch 7 | 93.88 |
Epoch 8 | 93.02 |
Epoch 9 | 91.92 |
Epoch 10 | 92 |
Epoch 11 | 93.4 |
Epoch 12 | 94.6 |
Epoch 13 | 95.5 |
Epoch 14 | 95.8 |
Epoch 15 | 96 |
No. of Epochs | Error rate (%) |
Epoch 1 | 52.98 |
Epoch 2 | 38 |
Epoch 3 | 18.5 |
Epoch 4 | 13 |
Epoch 5 | 9.95 |
Epoch 6 | 7.5 |
Epoch 7 | 6.12 |
Epoch 8 | 6.98 |
Epoch 9 | 8.08 |
Epoch 10 | 8 |
Epoch 11 | 6.6 |
Epoch 12 | 5.4 |
Epoch 13 | 4.5 |
Epoch 14 | 4.2 |
Epoch 15 | 4 |
No. of Epochs | Sensitivity or Recall (%) |
Epoch 1 | 47.02 |
Epoch 2 | 62 |
Epoch 3 | 81.5 |
Epoch 4 | 87 |
Epoch 5 | 90.05 |
Epoch 6 | 92.5 |
Epoch 7 | 93.88 |
Epoch 8 | 93.02 |
Epoch 9 | 91.92 |
Epoch 10 | 92 |
Epoch 11 | 93.4 |
Epoch 12 | 94.6 |
Epoch 13 | 95.5 |
Epoch 14 | 95.8 |
Epoch 15 | 96 |
Predicted resultsActual results | Has one of the three types of brain tumor plus a normal brain | Does not have one of the three types of brain tumor plus a normal brain |
Has one of the three types of brain tumor plus a normal brain | 96 | 0 |
Does not have one of the three types of brain tumor plus a normal brain | 4 | 0 |
Green color: includes true positives; Blue color: includes true negatives; Orange color: includes false negatives; Violet color: includes false positives |
Brain situation | Tested images | TP | TN | FP | FN |
Normal | 25 | 25 | 0 | 0 | 0 |
Eependymoma | 25 | 23 | 0 | 0 | 2 |
Medulloblastoma | 25 | 23 | 0 | 0 | 2 |
Meningioma | 25 | 25 | 0 | 0 | 0 |
No. of Epochs | Accuracy (%) |
Epoch 1 | 47.02 |
Epoch 2 | 62 |
Epoch 3 | 81.5 |
Epoch 4 | 87 |
Epoch 5 | 90.05 |
Epoch 6 | 92.5 |
Epoch 7 | 93.88 |
Epoch 8 | 93.02 |
Epoch 9 | 91.92 |
Epoch 10 | 92 |
Epoch 11 | 93.4 |
Epoch 12 | 94.6 |
Epoch 13 | 95.5 |
Epoch 14 | 95.8 |
Epoch 15 | 96 |
No. of Epochs | Error rate (%) |
Epoch 1 | 52.98 |
Epoch 2 | 38 |
Epoch 3 | 18.5 |
Epoch 4 | 13 |
Epoch 5 | 9.95 |
Epoch 6 | 7.5 |
Epoch 7 | 6.12 |
Epoch 8 | 6.98 |
Epoch 9 | 8.08 |
Epoch 10 | 8 |
Epoch 11 | 6.6 |
Epoch 12 | 5.4 |
Epoch 13 | 4.5 |
Epoch 14 | 4.2 |
Epoch 15 | 4 |
No. of Epochs | Sensitivity or Recall (%) |
Epoch 1 | 47.02 |
Epoch 2 | 62 |
Epoch 3 | 81.5 |
Epoch 4 | 87 |
Epoch 5 | 90.05 |
Epoch 6 | 92.5 |
Epoch 7 | 93.88 |
Epoch 8 | 93.02 |
Epoch 9 | 91.92 |
Epoch 10 | 92 |
Epoch 11 | 93.4 |
Epoch 12 | 94.6 |
Epoch 13 | 95.5 |
Epoch 14 | 95.8 |
Epoch 15 | 96 |
Predicted resultsActual results | Has one of the three types of brain tumor plus a normal brain | Does not have one of the three types of brain tumor plus a normal brain |
Has one of the three types of brain tumor plus a normal brain | 96 | 0 |
Does not have one of the three types of brain tumor plus a normal brain | 4 | 0 |
Green color: includes true positives; Blue color: includes true negatives; Orange color: includes false negatives; Violet color: includes false positives |
Brain situation | Tested images | TP | TN | FP | FN |
Normal | 25 | 25 | 0 | 0 | 0 |
Eependymoma | 25 | 23 | 0 | 0 | 2 |
Medulloblastoma | 25 | 23 | 0 | 0 | 2 |
Meningioma | 25 | 25 | 0 | 0 | 0 |