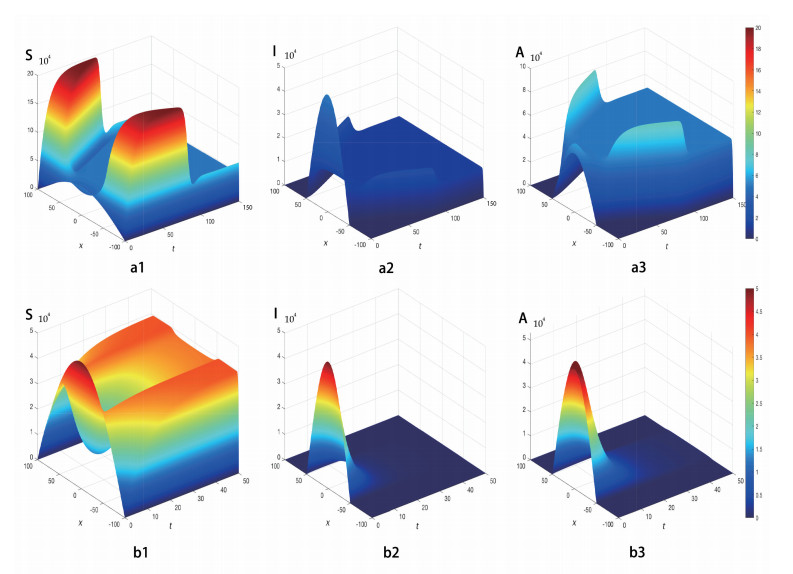
This paper presents a free-boundary epidemic model with subclinical infections and vaccination.We prove the existence and uniqueness of solutions to the model.Moreover, sufficient conditions for the disease vanishing and spreading are given.The disease will vanish if the basic reproduction number R0<1, that the corresponding ODE model defines without spatial expansion. However, the disease will spread to the whole area if RF0(t0)>1 for some t0>0 when it is introduced spatial heterogeneity. RF0(0)<R0 implies that the spillovers from hotspots to areas with no confirmed cases will reduce the outbreak threshold and increase the difficulty of prevention and control in the whole region. Under the condition RF0(0)<1<R0, if the free boundary condition of infectives h(t)<∞, t→∞, then the disease is vanishing, which indicates that RF0(0)<1 can also control the disease if the scope of hotspots expansion is limited. Furthermore, the numerical simulations illustrate that the routine vaccination would decrease the basic reproduction number and then change the disease from spreading to vanishing.
Citation: Fangyuan Chen, Siya Chen, Weizhong Yang. Vanishing and spreading conditions for a free-boundary epidemic model with subclinical infections and vaccination[J]. Mathematical Biosciences and Engineering, 2022, 19(7): 6523-6535. doi: 10.3934/mbe.2022307
[1] | Meng Zhao, Wan-Tong Li, Yang Zhang . Dynamics of an epidemic model with advection and free boundaries. Mathematical Biosciences and Engineering, 2019, 16(5): 5991-6014. doi: 10.3934/mbe.2019300 |
[2] | Min Zhu, Xiaofei Guo, Zhigui Lin . The risk index for an SIR epidemic model and spatial spreading of the infectious disease. Mathematical Biosciences and Engineering, 2017, 14(5&6): 1565-1583. doi: 10.3934/mbe.2017081 |
[3] | Qiaoling Chen, Fengquan Li, Sanyi Tang, Feng Wang . Free boundary problem for a nonlocal time-periodic diffusive competition model. Mathematical Biosciences and Engineering, 2023, 20(9): 16471-16505. doi: 10.3934/mbe.2023735 |
[4] | Chuanqing Xu, Xiaotong Huang, Zonghao Zhang, Jing'an Cui . A kinetic model considering the decline of antibody level and simulation about vaccination effect of COVID-19. Mathematical Biosciences and Engineering, 2022, 19(12): 12558-12580. doi: 10.3934/mbe.2022586 |
[5] | Qian Ding, Yunfeng Liu, Yuming Chen, Zhiming Guo . Dynamics of a reaction-diffusion SIRI model with relapse and free boundary. Mathematical Biosciences and Engineering, 2020, 17(2): 1659-1676. doi: 10.3934/mbe.2020087 |
[6] | Lusha Shi, Jianghong Hu, Zhen Jin . Dynamics analysis of strangles with asymptomatic infected horses and long-term subclinical carriers. Mathematical Biosciences and Engineering, 2023, 20(10): 18386-18412. doi: 10.3934/mbe.2023817 |
[7] | Lili Han, Mingfeng He, Xiao He, Qiuhui Pan . Synergistic effects of vaccination and virus testing on the transmission of an infectious disease. Mathematical Biosciences and Engineering, 2023, 20(9): 16114-16130. doi: 10.3934/mbe.2023719 |
[8] | Lili Liu, Xi Wang, Yazhi Li . Mathematical analysis and optimal control of an epidemic model with vaccination and different infectivity. Mathematical Biosciences and Engineering, 2023, 20(12): 20914-20938. doi: 10.3934/mbe.2023925 |
[9] | Steady Mushayabasa, Drew Posny, Jin Wang . Modeling the intrinsic dynamics of foot-and-mouth disease. Mathematical Biosciences and Engineering, 2016, 13(2): 425-442. doi: 10.3934/mbe.2015010 |
[10] | Rong Yuan, Yangjun Ma, Congcong Shen, Jinqing Zhao, Xiaofeng Luo, Maoxing Liu . Global dynamics of COVID-19 epidemic model with recessive infection and isolation. Mathematical Biosciences and Engineering, 2021, 18(2): 1833-1844. doi: 10.3934/mbe.2021095 |
This paper presents a free-boundary epidemic model with subclinical infections and vaccination.We prove the existence and uniqueness of solutions to the model.Moreover, sufficient conditions for the disease vanishing and spreading are given.The disease will vanish if the basic reproduction number R0<1, that the corresponding ODE model defines without spatial expansion. However, the disease will spread to the whole area if RF0(t0)>1 for some t0>0 when it is introduced spatial heterogeneity. RF0(0)<R0 implies that the spillovers from hotspots to areas with no confirmed cases will reduce the outbreak threshold and increase the difficulty of prevention and control in the whole region. Under the condition RF0(0)<1<R0, if the free boundary condition of infectives h(t)<∞, t→∞, then the disease is vanishing, which indicates that RF0(0)<1 can also control the disease if the scope of hotspots expansion is limited. Furthermore, the numerical simulations illustrate that the routine vaccination would decrease the basic reproduction number and then change the disease from spreading to vanishing.
As a new public health emergency, the severe acute respiratory syndrome coronavirus 2 (SARS-CoV-2) had infected more than 400,000,000 people worldwide; and the Corona Virus Disease 2019 (COVID-19), had killed more than 5,500,000 people by the end of 2021 [1]. The epidemic was accelerated by the transport system used in China during the Chunyun season, which is a mass worker migration in the time of Spring Festival. During Chunyun, many migrant workers had to pass through the whole country regardless of whether heading to north or south. Then the SARS-CoV-2 infection was directly transmitted around China by the well-connected traffic [2,3].
In this paper, we will present a COVID-19 epidemiological model with a free boundary to describe the process of change in the region of pathogen transmission equipped with local diffusion process from epicenter to adjacent cities. The local diffusion phenomenon is reflected by introducing Laplace operator, Δf=∂2f∂x2, with the one-dimensional x∈R. In the process of local diffusion, individual movement satisfies the reverse movement rule of Fick's law. Recently, many mathematical models with free boundaries have been developed to study their vanishing and spreading conditions [4,5,6,7,8]. For example, reference [5] shows that the disease will not spread to the whole area if the basic reproduction number R0<1 or the initial infected radius h0 is sufficiently small even that R0>1. Reference [7] shows that if the spreading domain is high-risk, the disease will spread till the whole area is infected, while if the spreading domain is low-risk, the disease may vanish.
Moreover, in order to get closer to the actual transmission process of the epidemic, we will introduce subclinical infections to the epidemic model, which is an important reason for the COVID-19 transmission globally under strict travel restrictions. The clinical symptoms of coronavirus infection are not identical among the infected individuals, and they can suffer from symptoms associated with the common cold to more severe diseases, such as pneumonia [1]. Subclinical infections occur when too few lung cells are infected to cause clinical symptoms. Although subclinically infected individuals do not cause significant physical harm to patients, as observed from the results so far, they still have the ability to transmit coronavirus to others. Moreover, our study found that regardless of whether the free boundary was considered, the presence of subclinically infected persons still increased the basic reproduction number of COVID-19, which increased the difficulty of disease control.
Vaccination worldwide has been playing a crucial role in containing the COVID-19 pandemic. By the end of January 2022, the number of people who had completed the second dose was over 4 billion, which was about 51% of the global population [9]. A series of studies had reported on the effectiveness of vaccination [10]. Israel's nationwide mass vaccination campaign showed a 92% effectiveness against infection after the second dose [11]. In Chile, over the age of 16, the vaccine effectiveness was 16.13% after the first dose and 66.96% after the second dose [10]. However, vaccine-induced antibody levels would decline over time. A real-world study of more than 3.43 million people in the United States showed that fully vaccinated people were 88% effective in preventing SARS-CoV-2 infection in the first month and only 47% effective after five months [12]. As a result, some countries had proposed a third or even a fourth dose. Whether the COVID-19 vaccination should become or not a periodic schedule similar to the flu vaccination required further practical testing. However, in a mathematical modelling study, we can first propose the model with routine vaccination and predict the possible dynamic behaviour of SARS-CoV-2 transmission.
Inspired by the above discussions, we consider a free-boundary COVID-19 model with subclinical infections and vaccination.
{St−d1ΔS=(1−ν)Λ−β(I(r,t)+A(r,t))S(r,t)−dS(r,t), r>0,t>0,It−d2ΔI=pβ(I(r,t)+A(r,t))S(r,t)−(e0+γ1+d)I(r,t), 0<r<h(t),t>0,At−d3ΔA=(1−p)β(I(r,t)+A(r,t))S(r,t)−(γ2+d)A(r,t), 0<r<h(t),t>0,Rt−d4ΔR=γ1I(r,t)+γ2A(r,t)−dR(r,t), 0<r<h(t),t>0,Sr(0,t)=Ir(0,t)=Ar(0,t)=Rr(0,t)=0, t>0,I(r,t)=A(r,t)=R(r,t)=0, r≥h(t),t>0,h′(t)=−μ(Ir(h(t),t)+Ar(h(t),t)),h(0)=h0>0, t>0,S(r,0)=S0(r),I(r,0)=I0(r),A(r,0)=A0(r),R(r,0)=R0(r), r≥0, | (2.1) |
where Δw=wrr, r=|x| and x∈R. It is assumed that the environment is radially symmetric for simplicity.
The initial functions S0, I0, A0 and R0 are nonnegative and satisfy
{S0∈C2([0,∞))∩L∞([0,∞)),I0,A0,R0∈C2([0,h0]),I0(r)=A0(r)=R0(r)=0,r∈[h0,∞),I0(r)>0,A0(r)>0,r∈[0,h0). | (2.2) |
We divide the population into four compartments: susceptibles (S), infectives with apparent symptoms (I), subclinical infectives without recognizable clinical signs or symptoms (A) and recovered individuals (R). The notation Λ and d represent the migration rate and move-out rate, respectively. e0 is the additional mortality rate of COVID-19. The notation β represents the per capita incidence rate. γ1 and γ2 denote the recovery rate of I and A. di, i=1,2,3,4, are the diffusion coefficients for different compartments. p is the proportion of infectives with apparent symptoms, with 0<p<1. ν is the routine vaccination coverage rate. It is noteworthy that all parameters mentioned above are positive.
Theorem 3.1. For any given (S0,I0,A0,R0) satisfying (2.2) and any η∈(0,1), there is a T>0 such that model (2.1) admits a unique bounded solution
(S,I,A,R;h)∈C1+η,1+η2([0,∞)×[0,T])×[C1+η,1+η2([0,h(t)]×[0,T])]3×C1+η2([0,T]). |
Moreover,
||S||C1+η,1+η2([0,∞)×[0,T])+||I||C1+η,1+η2([0,h(t)]×[0,T])+||A||C1+η,1+η2([0,h(t)]×[0,T]) |
+||R||C1+η,1+η2([0,h(t)]×[0,T])+||h||C1+η2≤C1. |
Here C1 and T only depend on h0, η, ||S||C2([0,∞)), ||A||C2([0,h0]), ||I||C2([0,h0]) and ||R||C2([0,h0]).
Proof. At first, we straighten the free boundary as in [4]. Let ξ(s) be a function in C3[0,∞) satisfying:
{ξ(s)=1, |s−h0|<h04,ξ(s)=0, |s−h0|>h02,ξ′(s)<4h0, s∈[0,∞). |
Consider the transformation
(s,t)→(r,t) with r=s+ξ(s)(h(t)−h0), for s∈[0,∞),
which is corresponding to
(y,t)→(x,t) with x=y+ξ(|y|)(h(t)−h0y|y|), for y∈Rn.
If t is confined to
|h(t)−h0|<h08, |
then the transformation above implies that x→y is a diffeomorphism from Rn onto Rn and r→s is a diffeomorphism from [0,∞) onto [0,∞). This transformation can change the free boundary r=h(t) to the line s=h0. Then, the rest of the proof is similar to the proof of Theorem 2.1 in [5]. We omit the details. This is a common method for dealing with free boundary questions, and more details can be seen in [4,5,6,7,8].
Then we consider Theorem 3.2 to extend the local solution in Theorem 3.1 to all t≥0.
Theorem 3.2. For any given (S0,I0,A0,R0) satisfying (2.2), the solution of the model (2.1) exists and is unique for all t∈[0,∞).
Proof. By the uniqueness of solutions in Theorem 3.1, there is a fixed T such that the solution (S,I,A,R,h) is confined to [0,T). If there exist Mi, i=1,2,3, independent of T such that
0≤S(r,t)≤M1, (r,t)∈[0,∞)×[0,T),0≤I(r,t), A(r,t), R(r,t)≤M2, (r,t)∈[0,h(t)]×[0,T),0<h′(t)≤M3, t∈[0,T).. | (3.1) |
Then using the standard parabolic regularity, we can find M0 depending on δ∈(0,T), T and Mi, i=1,2,3 such that
||S(⋅,t)||C2([0,∞)),||I(⋅,t)||C2([0,h(t)]),||A(⋅,t)||C2([0,h(t)]),||R(⋅,t)||C2([0,h(t)])≤M0 for t∈[δ,T]
To repeat the process again by the Theorem 3.1, we can always find a τ>0 depending on Mi, i=1,2,3 such that the solution of (2.1) with initial time T−τ2 can be extended uniquely to the time T+τ2, then to the time T+3τ2, ..., then to the infinite time +∞ as long as the solutions remain bounded.
At last, we focus on the existence of Mi, i=1,2,3, in the proof of Theorem 3.2.
Lemma 3.3. Let (S,I,A,R,h) be a solution to model (2.1) defined for t∈[0,T) for some T∈(0,∞). Then, there exists constant Mi, i=1,2,3, independent of T satisfying (3.1).
Proof. Since S(r,t) satisfies
{St−d1ΔS≤(1−ν)Λ−dS(r,t),r>0,0<t<T,S(r,0)=S0(r),r≥0, | (3.2) |
we can obtain
S≤max{||S0||L∞([0,∞)),(1−ν)Λd}:=M1 |
by the strong maximum principle. Similarly, let N=S+I+A+R, then it is obvious that
N≤max{||S0||L∞([0,∞))+||I0||C([0,h0])+||A0||C([0,h0])+||R0||C([0,h0]),(1−ν)Λd}:=M2. |
Moreover, it is evident to see that S≥0, I≥0, A≥0 and R≥0 in [0,∞)×[0,T) by the strong maximum principle as long as the solution exists. Therefore, S,I,A,R≤N and we can get I,A,R≤M2.
Using the Hopf lemma to the equations of I and A implies that
Ir(h(t),t)<0andAr(h(t),t)<0for0<t<T. |
Therefore, h′(t)>0 for 0<t<T by the free boundary conditions.
Then we construct an auxiliary function w(r,t):=M2[2C(h(t)−r)−C2(h(t)−r)2] in order to obtain w(r,t)≥I(r,t)+A(r,t) in Ω by choosing appropriate C, where Ω={(r,t):h(t)−C−1<r<h(t),0<t<T}.
Direct calculations show that,
wt=2CM2h′(t)(1−C(h(t)−r))≥0, |
and
−Δw=2C2M2. |
If we choose
C:=max{√βM1min{d2,d3},4(||I0||C1([0,h0])+||A0||C1([0,h0]))3M2,12h0}, |
then we can get
wt−DΔw≥2DM2C2≥2βM1M2≥βS(I+A)−γ(I+A) |
in Ω, with D=min{d2,d3}, γ=max{e0+γ1+d,γ2+d},
wr(r,0)≤−CM2≤I′0(r)+A′0(r),r∈[h0−(2C)−1,h0] |
and then
w(r,0)≥I0(r)+A0(r),r∈[h0−(2C)−1,h0] |
due to w(h0,0)=I0(h0)+A0(h0)=0.
By the maximum principle, we can obtain
h′(t)=−μ(Ir(h(t),t)+Ar(h(t),t))≤−μwr(h(t),t)=2μCM2:=M3. |
At first, we define the basic reproduction number R0 without spatial heterogeneity as the corresponding ODE model (4.1) by the method in [13].
{dS(t)dt=(1−ν)Λ−β(I(t)+A(t))S(t)−dS(t),t>0,dI(t)dt=pβ(I(t)+A(t))S(t)−(e0+γ1+d)I(t),t>0,dA(t)dt=(1−p)β(I(t)+A(t))S(t)−(γ2+d)A(t),t>0,dR(t)dt=γ1I(t)+γ2A(t)−dR(t),t>0. | (4.1) |
The Jacobian matrix of (I,A) is
J=( pβS−(e0+γ1+d)pβS(1−p)βS(1−p)βS−(γ2+d)). |
Let J=F−V, F be the rate of appearance of new infections in compartment I, V be the rate of transfer of individuals out of compartment I. Then, we get
F=(pβSpβS(1−p)βS(1−p)βS),V= (e0+γ1+d00γ2+d). |
We call FV−1 be the next generation matrix for the model (4.1) and set Rs=ρ(FV−1|E0), where E0=((1−ν)Λd,0,0,0) is the disease-free equilibrium of model (4.1) and ρ(A) denotes the spectral radius of a matrix A.
Then we get
R0=(1−ν)pβΛd(e0+γ1+d)+(1−ν)(1−p)βΛd(γ2+d). |
As indicates above, the first term (1−ν)pβΛd(e0+γ1+d) is the contribution to the basic reproduction number by the clinical infections; the second term (1−ν)(1−p)βΛd(γ2+d) is the contribution by the subclinical infections.
We prove in Theorem 4.1 that R0<1 is the sufficient condition for disease vanishing. However, R0>1 is not the sufficient condition for disease spreading. Reference [5] shows that the disease will also not spread to the whole area if the initial infected radius h0 is sufficiently small even that R0>1.
If the free boundary condition is transformed into a fixed boundary h0, then the system (2.1) is transformed to (4.2).
{St−d1ΔS=(1−ν)Λ−β(I(r,t)+A(r,t))S(r,t)−dS(r,t), r>0,t>0,It−d2ΔI=pβ(I(r,t)+A(r,t))S(r,t)−(e0+γ1+d)I(r,t), 0<r<h0,t>0,At−d3ΔA=(1−p)β(I(r,t)+A(r,t))S(r,t)−(γ2+d)A(r,t), 0<r<h0,t>0,Rt−d4ΔR=γ1I(r,t)+γ2A(r,t)−dR(r,t), 0<r<h0,t>0,I(h0,t)=A(h0,t)=R(h0,t)=0,S(r,0)=S0(r), I(r,0)=I0(r), A(r,0)=A0(r), R(r,0)=R0(r), r≥0. | (4.2) |
We consider the eigenvalue problems of I and A in system (4.2) at disease-free equilibirum E0, respectively. For I, let λ1 be the first eigenvalue of the following eigenvalue problem
{d2ψ″1+(pβΛ(1−ν)d−e0−γ1−d)ψ1+λψ1=0, 0<r<h0,ψ1(h0)=0, |
and the eigenfunction ψ1 with respect to λ1 be positive on (0,h0), then λ1 can be represented by the following variational form
λ1=d2(πh0)2−(pβΛ(1−ν)d−e0−γ1−d)=[d2(πh0)2+e0+γ1+d](1−(1−ν)pβΛd(d2(πh0)2+e0+γ1+d)). |
For A, let λ2 be the first eigenvalue of the following eigenvalue problem
{d3ψ″2+((1−p)βΛ(1−ν)d−γ2−d)ψ2+λψ2=0, 0<r<h0,ψ2(h0)=0, |
and the eigenfunction ψ2 with respect to λ2 be positive on (0,h0), then λ2 can be represented by the following variational form
λ2=d3(πh0)2−((1−p)βΛ(1−ν)d−γ2−d)=[d3(πh0)2+γ2+d](1−(1−ν)(1−p)βΛd(d3(πh0)2+γ2+d)). |
Therefore, the basic reproduction number of system (4.2) can be defined as
RD0=max{(1−ν)pβΛd(d2(πh0)2+e0+γ1+d), (1−ν)(1−p)βΛd(d3(πh0)2+γ2+d)}. |
Furthermore, we define another basic reproduction number RF0(t) of model (2.1) with free boundary condition h(t) as in [7].
RF0(t)=max{(1−ν)pβΛd(d2(πh(t))2+e0+γ1+d), (1−ν)(1−p)βΛd(d3(πh(t))2+γ2+d)}. |
In view of Lemma 3.3, we can observe that h(t) is monotone increasing. Therefore, RF0(t) is also monotone increasing with t. It is clear to see that R0≥RF0(t). Moreover, it follows that R0 and RF0(0) would decrease with routine vaccination by their definitions.
Theorem 4.1. If R0<1, then limt→∞||I(⋅,t)||C([0,h(t)])=0, limt→∞||A(⋅,t)||C([0,h(t)])=0, h∞<∞, which implies that the disease is vanishing; If RF0(0)>1, then limt→∞inf||I(⋅,t)||C([0,h(t)])>0, limt→∞inf||A(⋅,t)||C([0,h(t)])>0, h∞=∞, which implies that the disease is spreading.
Proof. According to the comparison principle, we can obtain S(r,t)≤u(t) for r≥0 and t∈(0,∞), where
u(t):=(1−ν)Λd+(||S0||∞−(1−ν)Λd)e−dt, |
which is the solution of
{dudt=(1−ν)Λ−du,t>0,u(0)=||S0||∞. |
Since limt→∞u(t)=(1−ν)Λd, we deduce that limt→∞supS(r,t)≤(1−ν)Λd uniformly for r∈[0,∞).
If R0<1, there exists T0 such S(r,t)≤(1−ν)(1+R0)Λ2dR0 in [0,∞)×[T0,∞). Then I(r,t) satisfies
{It−d2ΔI≤[ (1−ν)(1+R0)pβΛ2dR0−(e0+γ1+d)]I(r,t), 0<r<h(t),t>T0,I(h(t),t)=0, t>0,I(r,T0)>0, 0≤r≤h(T0). | (4.3) |
Therefore limt→∞||I(⋅,t)||C([0,h(t)])||=0 due to (1−ν)(1+R0)pβΛ2dR0(e0+γ1+d)<R0<1. Similarly, we can obtain limt→∞||A(⋅,t)||C([0,h(t)])=0.
Next, we show that h∞<∞ if R0<1. Direct calculation shows that
ddt∫h(t)0rn−1I(r,t)dr=∫h(t)0rn−1It(r,t)dr+h′(t)hn−1(t)I(h(t),t) |
=−d2μhn−1h′(t)+∫h(t)0rn−1I(r,t)(pβS(r,t)−e0−γ1−d)dr. |
Then integrating it from T0 to t, t>T0, we can obtain
∫h(t)0rn−1I(r,t)dr≤∫h(T0)0rn−1I(r,T0)dr+d2nμhn(T0)−d2nμhn(t), |
which implies that h∞<∞. Moreover, it follows from the first equation of (2.1) that limt→∞S(r,t)=(1−ν)Λd uniformly in any bounded subset of [0,∞)
We now use proof by contradiction to show the results when RF0(0)>1. Suppose that h∞<∞. For some δ>0, there exists a T1>0 such that S(r,t)≥(1−ν)Λd−δ when t>T1,r∈[0,∞), and then I(r,t) satisfies that
{It−d2ΔI≥[ pβ((1−ν)Λd−δ)−(e0+γ1+d)]I(r,t), 0<r<h(t),t>T1,I(h(t),t)=0, t>T1,I(r,T1)>0, 0≤r≤h0. | (4.4) |
Then it is obvious that the I(r,t) has a lower solution I_(r,t), which satisfies that
{I_t−d2ΔI=[ pβ((1−ν)Λd−δ)−(e0+γ1+d)]I_(r,t), 0<r<h(t),t>T1,I_(h(t),t)=0, t>T1,I_(r,T1)=I(r,T1), 0≤r≤h0. | (4.5) |
Since RF0(0)>1, there exists sufficiently small δ such that
pβ((1−ν)Λd−δ)−(e0+γ1+d)>d2(πh0)2. |
Without loss of generality, we suppose that (1−ν)pβΛd(d2(πh0)2+e0+γ1+d)>(1−ν)(1−p)βΛd(d3(πh0)2+γ2+d). Therefore, I_(r,t) is unbounded in [0,h(t))×[T1,∞). Then we can obtain limt→∞||I(⋅,t)||C([0,h(t)])=∞.
However, if limt→∞||I(⋅,t)||C([0,h(t)])=∞, then there exists a sequence (rk,tk) in [0,h(t))×(0,∞) such that I(rk,tk)≥δ2 for all k∈N, and tk→∞ as k→∞. Since that 0≤rk<h(t)<h∞<∞, we then have a subsequence of {rkn} converges to r0∈[0,h∞). Assume rn:=rkn, then we obtain a new sequence {rn}, rn→r0 as n→∞ such that I(rn,tn)≥δ2.
Define Sn(r,t)=S(r,tn+t), In(r,t)=I(r,tn+t), An(r,t)=A(r,tn+t) and Rn(r,t)=R(r,tn+t) for (r,t)∈(0,h(tn+t))×(tn,∞). It follows from the parabolic regularity that {(Sn,In,An,Rn)} has a subsequence {(Sni,Ini,Ani,Rni)} converges to (˜S,˜I,˜A,˜R) as i→∞, which satisfies
{˜St−d1Δ˜S=(1−ν)Λ−β(˜I(r,t)+˜A(r,t))˜S(r,t)−d˜S(r,t),0<r<h∞,t>0,˜It−d2Δ˜I=pβ(˜I(r,t)+˜A(r,t))˜S(r,t)−(e0+γ1+d)˜I(r,t),0<r<h∞,t>0,˜At−d3Δ˜A=(1−p)β(˜I(r,t)+˜A(r,t))˜S(r,t)−(γ2+d)˜A(r,t),0<r<h∞,t>0,˜Rt−d4Δ˜R=γ1˜I(r,t)+γ2˜A(r,t)−d˜R(r,t),0<r<h∞,t>0. | (4.6) |
Since ˜I(r0,0)≥δ2, we have ˜I>0 in [0,h∞)×(0,∞). Using the Hopf lemma, there exists σ>0 such that ˜Ir(h∞,0)≤−σ. Moreover, h(t) is increasing and bounded by Theorem 3.1. By the Lp estimates and the Sobolev's embedding theorem, there exists a constant C0, which depends on η, h0, h∞ and ||I0||C([0,h0]), for any 0<η<1, such that
||I||C1+η,1+η2([0,h∞)×[0,∞))+||h||C1+η2([0,∞))≤C0. |
Therefore, h′(t)→0 as t→∞. According to the definition of h′(t), we can obtain Ir(h(tn),tn)→0, which implies ˜Ir(h∞,0)=0. It leads to a contradiction to the fact ˜Ir(h∞,0)≤−σ depending on h∞<∞.
Furthermore, by the definition of I and A, we can obtain the result about A, which is similar to the proof above.
Assume that Λ=2 day−1, p=20%, d2=d3=4 day−1, S0(x)=5cosπx4h0, x∈R, I0(x)=A0(x)=5cosπx2h0, x∈[−h0,h0], h0=50 km. a1, a2 and a3 in Figure 1 show the long time behaviors of S, I and A when RF0(0)=3.29>1 with ν=0. And Figure 1(b1)–(b3) show the long time behaviors of S, I and A when R0=0.82<1 with ν=80%. The numerical simulations show that routine vaccination would decrease the basic reproduction number, and then change the disease from spreading to vanishing. Moreover, if RF0(0)>1, then the number of infections would expand from hotspots area x∈[−50,50] to free areas without confirmed cases, x∈[−100,−50)∪(50,100], and the local diffusion would make such expansion continuous as shown in Figuer 1(a2), (a3). If R0<1, then the diseases would vanish in hotspots area as shown in Figure 1(b2), (b3).
The consideration of subclinical infection increases the difficulty of disease control since we have got a more complicated expression of the basic reproduction numbers R0 and RF0(0). The parameter sensitivity analysis is shown in Figure 2, which is consistent with the theoretical analysis results that RF0(0)<RF0(∞)<R0. We further obtain that the difficulty of disease control is lower than that of infinite boundary diffusion for a limited area. With the free boundary h(t) increase, the basic reproduction number would also increase. This conclusion theoretically verifies the significance of epicenter being in lockdown for COVID-19 control in China at the beginning of 2020. Limiting spatial population migration could theoretically reduce the threshold conditions for disease outbreaks. Furthermore, there were significant differences in the same amount of vaccination effects. As shown in the Figure 2, vaccine demand of RF0(0)<1 was significantly smaller than that of R0<1. These analyses were crucial for the real-time adjustments of disease control and prevention responses.
This paper presented a free-boundary epidemic model with subclinical infections and vaccination. Then, we gave sufficient conditions for the disease vanishing or spreading. Although R0<1 is the sufficient condition for disease vanishing, R0>1 is not the sufficient condition for disease spreading. Therefore, we used another basic reproduction number RF0(t) to discuss the sufficient condition for disease spreading. Under the condition RF0(0)<1<R0, if h∞<∞, then the disease is vanishing; if h∞=∞, then the disease is spreading. The disease will spread to the whole area if RF0(0)>1. In order to emphasize the effect of basic reproduction number on the disease vanishing and spreading, we omitted the confine by the initially infected radius h0. The participation of subclinical infections increase the numerical value of R0 and RF0(0), and then magnify the difficulty to control the disease. A higher routine vaccination rate might be needed to reduce to R0<1.
The global pandemic has provided more considerations on spatial heterogeneity for studying the COVID-19 dynamics model. In the classical infectious disease compartment model, it is generally assumed that the individuals in the population are uniformly mixed and homogeneous. However, the population structure shows heterogeneity characteristics of non-uniform mixing in the real world. The uneven flow of people between regions affects the transmission dynamics of COVID-19. In this paper, we described the local spread of COVID-19 from the hot spot city of Wuhan to neighboring cities in the early stages of the outbreak in China. The local diffusion phenomenon was reflected by introducing the Laplace operator. Since it was difficult to observe the numerical simulation in two-dimensional space, this study simulated the local spatial diffusion of disease through one-dimensional and time-varying scenarios. Our hot spot area was a 100 km straight line, evenly distributed among 200,000 people, with a birth rate of 2 people per day. The diffusion coefficient was 4 people per day. Then the infected population would unconsciously expand from hot spots into a larger space of 200 km at the beginning of the epidemic. Figure 2 showed that the specific results of the numerical simulation. Through these simulation results and mathematical theory, we obtained the influence of the participation of subclinical infection on the spread of disease and analyzed the suppression effect of vaccination.
However, the local free boundary conditions are not accurate enough to describe the global prevalence of COVID-19 for long-distance population movements by airplanes or high-speed trains, which is the part of this article that we have not considered. In addition, because there are many parameters in the COVID-19 model, we cannot know the specific parameter estimates, and even if we did get them, they would not be very accurate. More extensive epidemiological investigation and more accurate parameter range are conducive to further studying mathematical models of infectious diseases such as COVID-19.
This study was supported by funding from Chinese Academy of Medical Sciences (CAMS) Innovation Fund for Medical Sciences (2020-I2M-1-001).
The authors declare there is no conflict of interest.
[1] | World Health Organization, Coronavirus. Available from: https://www.who.int/healthtopics/coronavirus. |
[2] |
H. Huang, Y. Wei, Intra-metropolitan location of foreign direct investment in wuhan, china: Institution, urban structure, and accessibility, Appl. Geogr., 47 (2014), 78–88. https://10.1016/j.apgeog.2013.11.012 doi: 10.1016/j.apgeog.2013.11.012
![]() |
[3] |
A. Phelan, R. Katz, L. Gostin, The novel coronavirus originating in wuhan, china: Challenges for global health governance, JAMA, 323 (2020), 709–710. https://10.1001/jama.2020.1097 doi: 10.1001/jama.2020.1097
![]() |
[4] |
X. Chen, A. Friedman, A free boundary problem arising in a model of wound healing, SIAM J. Math. Anal., 32 (2000), 778–800. https://10.1137/s0036141099351693 doi: 10.1137/s0036141099351693
![]() |
[5] |
K. Kim, Z. Lin, Q. Zhang, An sir epidemic model with free boundary, Nonlinear Anal-Real, 14 (2013), 1992–2001. https://10.1016/j.nonrwa.2013.02.003 doi: 10.1016/j.nonrwa.2013.02.003
![]() |
[6] |
Y. Du, Z. Lin, The diffusive competition model with a free boundary: Invasion of a superior or inferior competitor, Discrete Contin. Dyn. Syst. Ser. B, 19 (2014), 3105–3132. https://10.3934/dcdsb.2014.19.3105 doi: 10.3934/dcdsb.2014.19.3105
![]() |
[7] |
J. Ge, K. Kim, Z. Lin, H. Zhu, A sis reaction-diffusion-advection model in a low-risk and high-risk domain, J. Differ. Equations, 259 (2015), 5486–5509. https://10.1016/j.jde.2015.06.035 doi: 10.1016/j.jde.2015.06.035
![]() |
[8] |
C. Tian, S. Ruan, On an advection–reaction–diffusion competition system with double free boundaries modeling invasion and competition of Aedes Albopictus and Aedes Aegypti mosquitoes, J. Differ. Equations, 265 (2018), 4016–4051. https://10.1016/j.jde.2018.05.027 doi: 10.1016/j.jde.2018.05.027
![]() |
[9] | World Health Organization, Coronavirus disease (covid-19). Available from: https://www.who.int/emergencies/diseases/novel-coronavirus-2019. |
[10] |
C. Zheng, W. Shao, X. Chen, B. Zhang, G.Wang, W. Zhang, Real-world effectiveness of COVID-19 vaccines: a literature review and meta-analysis, Int. J. Infect. Dis., 114 (2022), 252–260. https://10.1016/j.ijid.2021.11.009 doi: 10.1016/j.ijid.2021.11.009
![]() |
[11] |
N. Dagan, N. Barda, E. Kepten, O. Miron, S. Perchik, M. Katz, et al., BNT162b2 mRNA Covid-19 Vaccine in a Nationwide Mass Vaccination Setting, N. Engl. J. Med., 384 (2021), 1412–1423. https://10.1056/NEJMoa2101765 doi: 10.1056/NEJMoa2101765
![]() |
[12] |
S. Tartof, J. Slezak, H. Fischer, V. Hong, B. Ackerson, O. Ranasinghe, et al., Effectiveness of mRNA BNT162b2 COVID-19 vaccine up to 6 months in a large integrated health system in the USA: a retrospective cohort study, Lancet, 398 (2021), 1407–1416. https://10.1016/S0140-6736(21)02183-8 doi: 10.1016/S0140-6736(21)02183-8
![]() |
[13] |
P. Dreessche, J. Watmough, Reproduction numbers and sub-threshold endemic equilibria for compartmental models of disease transmission, SIAM J. Math. Anal., 180 (2002), 29–48. https://10.1016/S0025-5564(02)00108-6 doi: 10.1016/S0025-5564(02)00108-6
![]() |
[14] |
C. You, Y. Deng, W. Hu, J. Sun, Q. Lin, F. Zhou, et al., Estimation of the time-varying reproduction number of covid-19 outbreak in china, Int. J. Hyg. Environ. Health, 288 (2020), 113555. https://10.2139/ssrn.3539694 doi: 10.2139/ssrn.3539694
![]() |