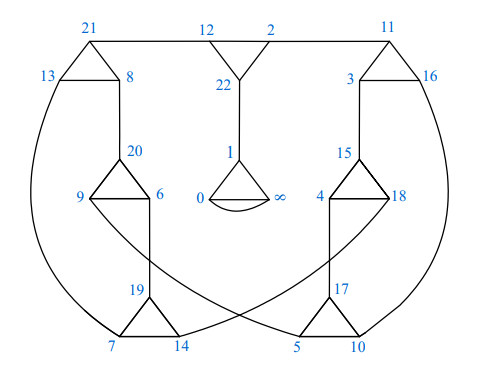
The closed-loop supply chain (CLSC) plays an important role in sustainable development and can help to increase the economic benefits of enterprises. The optimization for the CLSC network is a complicated problem, since it often has a large problem scale and involves multiple constraints. This paper proposes a general CLSC model to maximize the profits of enterprises by determining the transportation route and delivery volume. Due to the complexity of the multi-constrained and large-scale model, a genetic algorithm with two-step rank-based encoding (GA-TRE) is developed to solve the problem. Firstly, a two-step rank-based encoding is designed to handle the constraints and increase the algorithm efficiency, and the encoding scheme is also used to improve the genetic operators, including crossover and mutation. The first step of encoding is to plan the routes and predict their feasibility according to relevant constraints, and the second step is to set the delivery volume based on the feasible routes using a rank-based method to achieve greedy solutions. Besides, a new mutation operator and an adaptive population disturbance mechanism are designed to increase the diversity of the population. To validate the efficiency of the proposed algorithm, six heuristic algorithms are compared with GA-TRE by using different instances with three problem scales. The results show that GA-TRE can obtain better solutions than the competitors, especially on large-scale instances.
Citation: Bowen Ding, Zhaobin Ma, Shuoyan Ren, Yi Gu, Pengjiang Qian, Xin Zhang. A genetic algorithm with two-step rank-based encoding for closed-loop supply chain network design[J]. Mathematical Biosciences and Engineering, 2022, 19(6): 5925-5956. doi: 10.3934/mbe.2022277
[1] | Chunyan An, Wei Bai, Donglei Zhang . Meet-in-the-middle differential fault analysis on Midori. Electronic Research Archive, 2023, 31(11): 6820-6832. doi: 10.3934/era.2023344 |
[2] | Meng Xu, Xiangyang Luo, Jinwei Wang, Hao Wang . Color image steganalysis based on quaternion discrete cosine transform. Electronic Research Archive, 2023, 31(7): 4102-4118. doi: 10.3934/era.2023209 |
[3] | Xing Zhang, Xiaoyu Jiang, Zhaolin Jiang, Heejung Byun . Algorithms for solving a class of real quasi-symmetric Toeplitz linear systems and its applications. Electronic Research Archive, 2023, 31(4): 1966-1981. doi: 10.3934/era.2023101 |
[4] | Shuang Yao, Dawei Zhang . A blockchain-based privacy-preserving transaction scheme with public verification and reliable audit. Electronic Research Archive, 2023, 31(2): 729-753. doi: 10.3934/era.2023036 |
[5] | Han-Cheng Dan, Yongcheng Long, Hui Yao, Songlin Li, Yanhao Liu, Quanfeng Zhou . Investigation on the fractal characteristic of asphalt pavement texture roughness incorporating 3D reconstruction technology. Electronic Research Archive, 2023, 31(4): 2337-2357. doi: 10.3934/era.2023119 |
[6] | Xiaoyan Xu, Xiaohua Xu, Jin Chen, Shixun Lin . On forbidden subgraphs of main supergraphs of groups. Electronic Research Archive, 2024, 32(8): 4845-4857. doi: 10.3934/era.2024222 |
[7] | Wenya Shi, Xinpeng Yan, Zhan Huan . Faster free pseudoinverse greedy block Kaczmarz method for image recovery. Electronic Research Archive, 2024, 32(6): 3973-3988. doi: 10.3934/era.2024178 |
[8] | Sida Lin, Dongyao Yang, Jinlong Yuan, Changzhi Wu, Tao Zhou, An Li, Chuanye Gu, Jun Xie, Kuikui Gao . A new computational method for sparse optimal control of cyber-physical systems with varying delay. Electronic Research Archive, 2024, 32(12): 6553-6577. doi: 10.3934/era.2024306 |
[9] | Zengyu Cai, Liusen Xu, Jianwei Zhang, Yuan Feng, Liang Zhu, Fangmei Liu . ViT-DualAtt: An efficient pornographic image classification method based on Vision Transformer with dual attention. Electronic Research Archive, 2024, 32(12): 6698-6716. doi: 10.3934/era.2024313 |
[10] | Xiangquan Liu, Xiaoming Huang . Weakly supervised salient object detection via bounding-box annotation and SAM model. Electronic Research Archive, 2024, 32(3): 1624-1645. doi: 10.3934/era.2024074 |
The closed-loop supply chain (CLSC) plays an important role in sustainable development and can help to increase the economic benefits of enterprises. The optimization for the CLSC network is a complicated problem, since it often has a large problem scale and involves multiple constraints. This paper proposes a general CLSC model to maximize the profits of enterprises by determining the transportation route and delivery volume. Due to the complexity of the multi-constrained and large-scale model, a genetic algorithm with two-step rank-based encoding (GA-TRE) is developed to solve the problem. Firstly, a two-step rank-based encoding is designed to handle the constraints and increase the algorithm efficiency, and the encoding scheme is also used to improve the genetic operators, including crossover and mutation. The first step of encoding is to plan the routes and predict their feasibility according to relevant constraints, and the second step is to set the delivery volume based on the feasible routes using a rank-based method to achieve greedy solutions. Besides, a new mutation operator and an adaptive population disturbance mechanism are designed to increase the diversity of the population. To validate the efficiency of the proposed algorithm, six heuristic algorithms are compared with GA-TRE by using different instances with three problem scales. The results show that GA-TRE can obtain better solutions than the competitors, especially on large-scale instances.
Over the last few decades, the number of organizations and individuals working on the web has increased remarkably. Because of the widespread availability of data and information in every field, which is accessible to everyone, serious issues such as unauthorized access to confidential information have cropped up. As a result of this massive quantity of work and traffic, the risks of valuable data theft have significantly increased, and preventing these situations is a challenging task. Various researchers in their respective fields have worked to secure data by employing various cryptographic, watermarking, and stenographic schemes. Cryptography is a technique that restricts access to original information to the sender and recipient only [1]. It contains algorithms to block potential unauthorized access. Cryptographic algorithms are mathematical tools that helps in protection of data. The cryptography has two main types, symmetric and asymmetric cryptography. A symmetric cryptography [2] involves the procedures requires sole key to encrypt and decrypt the related content, while the algorithm in asymmetric cryptography contains two different keys for the process of encryption and decryption [3]. The symmetric cipher is further classified into two types: stream cipher and block cipher. The stream cipher modifies the original information bit-by-bit or byte-by-byte while the block cipher does so in blocks involving several bits or bytes simultaneously [4]. Data Encryption Standard (DES), GOST, Advanced Encryption Standard (AES), BLOWFISH, etc., are the well-known block ciphers. The substitution box (S-box) is a pertinent non-linear ingredient in block cipher that plays a very decisive role in encrypting the plaintext [5]. An n×n S-box is a Boolean function f:Zn2⟶Zn2 which maps an input of n bits to an n bits output. It generates perplexity and responsible for the complex relationship between actual and encrypted contents [6]. Therefore, it is not an overstatement to state that the security level of a block cipher can be determined by analyzing the performance of S-box.
Considering the importance of the S-box in the security of cryptosystems, designing complex mathematical techniques to construct robust S-boxes has become a goal of cryptographers. The scientists working in this field are primarily interested in improving the performance of block ciphers. For this purpose, thousands of studies have been conducted and published in leading journals in recent years. A novel approach of S-box creation is introduced in [7]. The authors developed their proposed S-box using a chaotic system and fitness function. Javeed et al. [8] developed an effective framework for generating strong S-boxes relying on chaotic maps and symmetric groups. The authors designed an initial S-box with the help of chaotic dynamical system. Then the final proposed S-box is obtained by applying a permutation of S256. In [9] a specific type of graphs based the concepts of group theory were employed to develop a new S-box. Multiple performance evaluation metrics validate the resilience of the suggested S-box.
In [10] Si et al. proposes a method to create a secure S-Box for symmetric cryptography using a 2D enhanced quadratic map, and an algorithm is designed to eliminate vulnerabilities. Experimental results confirm the method's effectiveness. Lambic [11] used usual multiplication and circular shift to generate an innovative discrete-space chaotic map which further employed in the construction of S-box having good security properties. Anees and Ahmed [12] designed a potent S-box by investigating the behavior of van der pol oscillator. Firstly, the author used a numerical technique to obtain the iterative solution of chaotic map. Then the ceiling function is employed to those solution to achieve the task. Liu et al. [13] proposes a strong S-Box construction method using a non-degenerate 3D improved quadratic map. The proposed algorithm satisfies six criteria and eliminates fixed points, reverse fixed points, and short cycles. Results show effectiveness in encrypting color images and verified security. A systematic scheme to evolve a S-box with high non-linearity value is given in [14]. The chaotic map iteration yields a 16×16 matrix on which the genetic technique is applied to obtain the suggested S-box. We recommend to read [15,16,17,18,19,20] for further information on S-box generation methodologies. In [21], a secure image encryption method was introduced. It used a new framework to create chaotic signals with finite computer precision, and includes circular diffusion and local/global scrambling. In [22] the authors introduced a new encryption algorithm for color images using DNA dynamic encoding, self-adapting permutation, and a new 4D hyperchaotic system. Zhou et al. [23] proposes a secure color image cryptosystem using deep learning to train hyper-chaotic signals, which are then applied to increase the system's security. Liu et al. [24] developed a secure color image encryption algorithm using a conservative chaotic system without attractors. They employed techniques such as plane element rearrangement, dynamic selection row-column cross scrambling, and cross-plane diffusion to enhance the encryption's security and mixing. The study [25] proposed a 2D hyperchaotic map to generate S-boxes and combine them to create a secure image encryption algorithm that passed NIST and TestU01 tests and resists common attacks. In [26], a new n-dimensional conservative chaos was designed to address security issues with encryption algorithms based on dissipative chaos. A new image encryption system using true random numbers and chaotic systems has been proposed in [27]. The method is found to be more secure and resistant to classical attacks compared to existing models.
The study presents a novel method for constructing robust S-boxes for use in block ciphers. The following factors were considered during the creation of the S-box:
i. The generated S-box must be cryptographically robust and comply with the mandatory information security standards.
ii. The S-box must exhibit a sufficient level of confusion and complexity, while the method used to construct it remains simple and computationally efficient.
iii. The S-box should demonstrate good performance when evaluated using modern cryptographic performance assessment parameters.
iv. The S-box must meet the requirements for suitability in multimedia image encryption, as determined through a thorough evaluation of its cryptographic properties and performance under relevant metrics.
The following paragraph summarizes the main contributions and proposed scheme of this article.
By utilizing the action of the modular group on a Galois field of order 1024, GF(210)={0,κ1,κ2,κ4,…,κ1023} a coset graph is constructed. The vertices of the coset graph are utilized in a specific manner to generate a random sequence of the elements in GF(210)∗={κ1,κ5,κ9,…,κ1021}, which is presented in a 16×16 matrix. Then, a bijective mapping from the group GF(210)∗ to GF(28) yields an initial S-box that exhibits reasonable security. A new notion named "matrix transformer" which transforms a matrix into another matrix has been introduced. By applying a specific matrix transformer to the initial S-box, we obtain a proposed S-box with almost optimal features. Furthermore, a series of well-established analyses are carried out to establish the potential effectiveness of the proposed S-boxes for image encryption in the context of multimedia encryption.
The arrangement of the remaining content of this paper is as follows: The purpose of Section 2 is to discuss the newly developed matrix transformer and modular group-based coset graphs over finite fields. Using the concepts described in Section 2 as a foundation, we propose our S-box design scheme in Section 3. Assessing the algebraic robustness of the constructed S-box is the focus of Section 4. This section also includes a comparison with some recently developed S-boxes. Sections 5–7 are devoted to examining the suitability of constructed S-box for image protection. We reveal the concluding remark in Section 8.
In this section, we will discuss some fundamental concepts that are required to comprehend the proposed S-box construction scheme.
The modular group M is an infinite non-cyclic group of linear transformations. It is generated by x and y such that (s)x=−1s and (s)y=s−1s. The finite presentation of M is ⟨x,y:x2=y3=1⟩. Let p be a prime number and n∈N. Then GF(pn) denote a Galois field of order pn, it is well known that M cannot act directly on GF(pn) as (0)x=−10∉GF(pn), so the action of M is possible if ∞ is adjoined with GF(pn), that is M acts on GF(pn)∪{∞}. The graphical interpretation of the action of M is described with the help of coset graphs [28,29,30,31]. As (s)y3=s, so y has cycles of length three which are represented by triangles whose vertices are elements of GF(pn)∪{∞} are permuted counter-clockwise by y. Moreover, since (s)x2=s, therefore an undirected line connecting a pair of vertices of the triangles is drawn to represent x. The heavy dots are used to denote fixed points of x and y, if they exist. For the better understanding of readers, here we describe the action of M on GF(23)∪{∞} and draw the corresponding coset graph. We apply (s)x=−1s and (s)y=s−1s on each element of GF(23)∪{∞} and obtain permutation representations of x and y. For example, (1)x=−11≡22 and (22)x=−122≡1 mean a cycle (1,22) of x. Moreover, (2)y=12≡12, (12)y=1112≡22 and (22)y=2122≡2 give rise to a cycle (2,12,22) of y. In a similar way, all other cycles of x and y can be computed and we have the following permutation representations of x and y.
x:(0,∞)(22,1)(11,2)(15,3)(17,4)(9,5)(19,6)(13,7)(20,8)(16,10)(21,12)(18,14); |
y:(1,0,∞)(2,12,22)(11,3,16)(15,4,18)(17,5,10)(9,6,20)(19,7,14)(13,8,21). |
The permutation representation of y consists of 8 cycles. Consequently, the resulting coset graph has eight triangles. The graphical version of the cycle (1,0,∞) in y is a triangle with the vertices 1,0 and ∞ permuted counter-clockwise by y. In a similar way, we can draw and label all triangles. The permutation representation of x contains 12 transpositions which correspond to12 undirected lines joining all 24 vertices of 8 triangles. For instance, (1,22) means the vertices 1 and 22 are connected through an undirected line. Similarly, the remaining vertices can be joined with each other through x and the following coset graph is emerged (See Figure 1).
In the next subsection, we have introduced a new notion namely matrix transformer to generate a strong S-box from an initial S-box.
Suppose M is a square matrix of order n. Let us define the position of the elements of M as follows;
kth element = mth element of ⌈kn⌉th row
where m={n if n divides mk mod (n) otherwise, and ⌈kn⌉ means ceiling of ⌈kn⌉.
For example, in a 3×3 matrix, we have 1st element means 1st element of 1st row, 2nd element means 2nd element of 1st row, 3rd element means 3rd element of 1st row, 4th element means 1st element of 2nd row and so on.
Definition 2.1. A square matrix A of order n with entries from {1,2,3,…,n2} is called matrix transformer of square matrix M of order n if the action of A on M evolves a new matrix M' of order n in the following way; t∈{1,2,3,…,n2} is the ith element of the matrix transformer A⟺ tth element of M' is equal to ith element of M.
Example 2.1. Consider M=(fdgcaiehb) and A=(397862415). Then the action of of A on M generates M'=(hifebagcd).
In this section, we propose our S-box construction method based on the concepts describe in the previous section.
The proposed S-box generation scheme involves coset graph of the modular group M on GF(210)∪{∞}. So, in the 1st phase, we have to construct the Galois field GF(210). It is well-known that a primitive irreducible polynomial of degree 10 over Z2 is required to compute all the elements of GF(210) [32]. For that purpose, we choose p(κ)=κ10+κ7+1 and obtain GF(210)={0,κ1,κ2,κ3,…,κ1023=1}. In Table 1, we present some of the elements of GF(210) along with their binary and decimal form.
Binary form | Decimal | 𝐺𝐹 (210) | Binary form | Decimal | 𝐺𝐹(210) | Binary form | Decimal | 𝐺𝐹(210) | Binary form | Decimal | 𝐺𝐹(210) |
0000000000 | 0 | 0 | 0000000001 | 1 | 1 | 0000000010 | 2 | κ1 | 0000000100 | 4 | κ2 |
0000001000 | 8 | κ3 | 0000010000 | 16 | κ4 | 0000100000 | 32 | κ5 | 0001000000 | 64 | κ6 |
0010000000 | 128 | κ7 | 0100000000 | 256 | κ8 | 1000000000 | 512 | κ9 | 0010000001 | 129 | κ10 |
0100000010 | 258 | κ11 | 1000000100 | 516 | κ12 | 0010001001 | 137 | κ13 | 0100010010 | 274 | κ14 |
1000100100 | 548 | κ15 | 0011001001 | 201 | κ16 | 0110010010 | 402 | κ17 | 1100100100 | 804 | κ18 |
1011001001 | 713 | κ19 | 0100010011 | 275 | κ20 | 1000100110 | 550 | κ21 | 0011001101 | 205 | κ22 |
… | … | … | … | … | … | … | … | … | … | … | … |
… | … | … | … | … | … | … | … | … | … | … | … |
… | … | … | … | … | … | … | … | … | … | … | … |
1010111011 | 699 | κ311 | 0111110111 | 503 | κ312 | 1111101110 | 1006 | κ313 | 1101011101 | 861 | κ314 |
1000111011 | 571 | κ315 | 0011110111 | 247 | κ316 | 0111101110 | 494 | κ317 | 1111011100 | 988 | κ318 |
1100111001 | 825 | κ319 | 1011110011 | 755 | κ320 | 0101100111 | 359 | κ321 | 1011001110 | 718 | κ322 |
0100011101 | 285 | κ323 | 1000111010 | 570 | κ324 | 0011110101 | 245 | κ325 | 0111101010 | 490 | κ326 |
… | … | … | … | … | … | … | … | … | … | … | … |
… | … | … | … | … | … | … | … | … | … | … | … |
… | … | … | … | … | … | … | … | … | … | … | … |
0100110000 | 304 | κ1007 | 1001100000 | 608 | κ1008 | 0001000001 | 65 | κ1009 | 0010000010 | 130 | κ1010 |
0100000100 | 260 | κ1011 | 1000001000 | 520 | κ1012 | 0010010001 | 145 | κ1013 | 0100100010 | 290 | κ1014 |
1001000100 | 580 | κ1015 | 0000001001 | 9 | κ1016 | 0000010010 | 18 | κ1017 | 0000100100 | 36 | κ1018 |
0001001000 | 72 | κ1019 | 0010010000 | 144 | κ1020 | 0100100000 | 288 | κ1021 | 1001000000 | 576 | κ1022 |
To draw the coset graph of M on GF(210)∪∞, we firstly apply the generators (s)x=−1s and (s)y=s−1s of M on all elements of GF(210)∪∞toget permutation representations of x and y. For instance, (κ1)x=−1κ1=1κ1=κ1023κ1=κ1022 and (κ1022)x=−1κ1022=1κ1022=κ1023κ1022=κ1 yield a cycle (κ1,κ126) of x. Moreover, (κ1)y=κ1−1κ1=κ947κ1=κ946, (κ946)y=κ946−1κ946=κ1022κ946=κ76 and (κ76)y=κ76−1κ76=κ77κ76=κ1, generate a (κ1,κ946,κ76)of y.
Similarly, the remaining cycles of x and y can be found and some of them are presented below;
x:(0,∞)(1)(κ1,κ1022)(κ2,κ1021)(κ3,κ1020)(κ4,κ1019)(κ5,κ1018)(κ6,κ1017)(κ7,κ1016)(κ8,κ1015) |
(κ9,κ1014)…(κ507,κ516)(κ508,κ515)(κ509,κ514)(κ510,κ513)(κ511,κ512); |
y:(κ1,κ946,κ76)(κ2,κ869,κ152)(κ3,κ1013,κ7)(κ4,κ715,κ304)(κ5,κ510,κ508)(κ6,κ1003,κ14) |
(κ8,κ407,κ608)…(κ650,κ713,κ683)(κ652,κ656,κ738)(κ654,κ695,κ697)(0,∞,1)(κ341)(κ682). |
Both permutations of x and y produced a disconnected coset graph which contains 172 number of patches. It is important to note that out of these 172 patches, 170 are of the same type, denoted by η1,η2,η3,…,η170. The other two patches are denoted by η171 and η172. We denote this coset graph by D and D=η1∪η2∪η3∪⋯∪η170∪η171∪η172 The Figures 2–4 represent η1,η171 and η172 respectively.
Step I: We first construct a square matrix of order 16 using vertices of coset graph in a specific way.
Consider a patch η1 containing κ1 as vertex from the coset graph D. The application of xyxy−1x on κ1 carries us to κ77 by following the route κ1x→κ1022y→κ947x→κ76y−1→κ946x→κ77 (see Figure 2). So, in this way, we generate a sequence κ1,κ1022,κ947,κ76,κ946,κ77 of vertices. Consider a sub-sequence {κi:i≡1 mod (4)}=κ1,κ77} of this sequence and place κ1 and κ77 at 1st and 2nd position of the first row respectively. Thereafter, we find the vertex from D−{η1} having the smallest power of κ, that is, κ2. Let us denote the copy from D−{η1} containing κ2 by η2. Note that if κ2 would been exhausted in η1 , then η2 is a copy from D−{Γ1} containing κ3 . Generate a sequence of the vertices of the type κi:i≡1 mod (4), present in η2, in a similar way as done in the case of η1. Write this sequence at the 1st row after κ77 by maintaining the order of sequence. After that, we chose a copy from d−{η1,η2} possessing a vertex κm, where m is the least positive integer. In a similar way, continue to select the copies ηi and write vertices of the type κi such that i≡1 mod (4) in the matrix until all copies ηi are used. So, a square matrix of 256 distinct entries from GF(210)∗={κ1,κ5,κ9,…,κ1021} is generated (see Table 2).
κ1 | κ77 | κ1021 | κ869 | κ1013 | κ5 | κ513 | κ1017 | κ1009 | κ9 | κ189 | κ13 | κ301 | κ709 | κ353 | κ193 |
κ209 | κ17 | κ729 | κ1005 | κ393 | κ21 | κ305 | κ1001 | κ645 | κ937 | κ25 | κ385 | κ997 | κ421 | κ817 | κ29 |
κ537 | κ457 | κ993 | κ317 | κ265 | κ637 | κ605 | κ33 | κ989 | κ401 | κ909 | κ149 | κ237 | κ273 | κ37 | κ161 |
κ985 | κ473 | κ545 | κ41 | κ849 | κ981 | κ413 | κ45 | κ977 | κ897 | κ673 | κ397 | κ49 | κ225 | κ749 | κ973 |
κ253 | κ109 | κ965 | κ181 | κ233 | κ53 | κ481 | κ969 | κ665 | κ213 | κ865 | κ57 | κ437 | κ529 | κ345 | κ737 |
κ449 | κ389 | κ61 | κ917 | κ961 | κ493 | κ65 | κ621 | κ957 | κ297 | κ945 | κ145 | κ153 | κ221 | κ69 | κ953 |
κ725 | κ261 | κ549 | κ477 | κ73 | κ949 | κ701 | κ405 | κ81 | κ177 | κ765 | κ941 | κ593 | κ785 | κ321 | κ113 |
κ197 | κ85 | κ349 | κ589 | κ489 | κ577 | κ89 | κ893 | κ933 | κ761 | κ93 | κ657 | κ929 | κ229 | κ721 | κ97 |
κ925 | κ573 | κ165 | κ417 | κ517 | κ101 | κ789 | κ133 | κ921 | κ805 | κ601 | κ525 | κ661 | κ557 | κ105 | κ837 |
κ269 | κ913 | κ597 | κ169 | κ705 | κ117 | κ461 | κ445 | κ905 | κ333 | κ553 | κ125 | κ245 | κ121 | κ357 | κ901 |
κ689 | κ257 | κ521 | κ649 | κ129 | κ561 | κ429 | κ889 | κ733 | κ329 | κ829 | κ717 | κ581 | κ137 | κ885 | κ873 |
κ141 | κ881 | κ501 | κ541 | κ877 | κ777 | κ853 | κ325 | κ157 | κ361 | κ505 | κ569 | κ613 | κ861 | κ669 | κ857 |
κ381 | κ797 | κ629 | κ173 | κ313 | κ845 | κ585 | κ841 | κ425 | κ465 | κ741 | κ185 | κ377 | κ565 | κ833 | κ609 |
κ825 | κ693 | κ201 | κ745 | κ821 | κ757 | κ205 | κ813 | κ441 | κ249 | κ809 | κ485 | κ409 | κ625 | κ217 | κ337 |
κ469 | κ801 | κ685 | κ793 | κ617 | κ773 | κ533 | κ241 | κ681 | κ781 | κ309 | κ497 | κ293 | κ769 | κ509 | κ433 |
κ633 | κ653 | κ753 | κ365 | κ277 | κ281 | κ453 | κ289 | κ285 | κ677 | κ641 | κ713 | κ373 | κ697 | κ369 | κ381 |
We can generate 3 more tables simply by replacing the type of vertices in step I, from κi:i≡1 mod (4) to κi:i≡0 mod (4), κi:i≡2 mod (4) and κi:i≡3 mod (4) .
Step II: The outcome of Step I yields a 16×16 matrix of distinct element from GF(210)∗={κ1,κ5,κ9,…,κ1021}. To bring all the element in the range of 0 to 255, we define a mapping f:GF(210)∗⟶GF(28) by f(κn)=βn−14. Note the Galois GF(28) is generated by primitive irreducible polynomial β8+β6+β5+β3+1. Table 3 shows some of the elements of GF(28) and their binary and decimal form.
Binary form | Decimal | 𝐺𝐹 (28) | Binary form | Decimal | 𝐺𝐹 (28) | Binary form | Decimal | 𝐺𝐹 (28) | Binary form | Decimal | 𝐺𝐹 (28) |
00000000 | 0 | 0 | 00000001 | 1 | 1 | 00000010 | 2 | β1 | 00000100 | 4 | β2 |
00001000 | 8 | β3 | 00010000 | 16 | β4 | 00100000 | 32 | β5 | 01000000 | 64 | β6 |
10000000 | 128 | β7 | 01101001 | 105 | β8 | 11010010 | 210 | β9 | 11001101 | 205 | β10 |
11110011 | 243 | β11 | 10001111 | 143 | β12 | 01110111 | 119 | β13 | 11101110 | 238 | β14 |
… | … | … | … | … | … | … | … | … | … | … | … |
… | … | … | … | … | … | … | … | … | … | … | … |
… | … | … | … | … | … | … | … | … | … | … | … |
10101100 | 172 | β163 | 00110001 | 49 | β164 | 01100010 | 98 | β165 | 11000100 | 196 | β166 |
11100001 | 225 | β167 | 10101011 | 171 | β168 | 00111111 | 63 | β169 | 01111110 | 126 | β170 |
11111100 | 252 | β171 | 10010001 | 145 | β172 | 01001011 | 75 | β173 | 10010110 | 150 | β174 |
… | … | … | … | … | … | … | … | … | … | … | … |
… | … | … | … | … | … | … | … | … | … | … | … |
… | … | … | … | … | … | … | … | … | … | … | … |
11010111 | 215 | β243 | 11000111 | 199 | β244 | 11100111 | 231 | β245 | 10100111 | 167 | β246 |
00100111 | 39 | β247 | 01001110 | 78 | β248 | 10011100 | 156 | β249 | 01010001 | 81 | β250 |
10100010 | 162 | β251 | 00101101 | 45 | β252 | 01011010 | 90 | β253 | 10110100 | 180 | β254 |
In this manner, we have designed our initial S-box (See Table 4). We have examined its cryptographic strength via some well-known performance evaluation criteria and found that it provides adequate security for transmitting sensitive information. To increase its security even further, let us proceed to step III.
24 | 41 | 1 | 180 | 2 | 140 | 113 | 52 | 32 | 4 | 190 | 42 | 125 | 102 | 90 | 60 |
8 | 128 | 205 | 45 | 253 | 16 | 250 | 86 | 162 | 109 | 17 | 115 | 244 | 81 | 217 | 64 |
238 | 48 | 101 | 57 | 156 | 59 | 148 | 78 | 35 | 105 | 122 | 98 | 100 | 39 | 210 | 182 |
104 | 167 | 50 | 239 | 46 | 231 | 38 | 13 | 243 | 221 | 213 | 97 | 72 | 132 | 199 | 143 |
248 | 164 | 83 | 215 | 119 | 55 | 214 | 223 | 219 | 181 | 177 | 200 | 3 | 135 | 224 | 235 |
111 | 216 | 6 | 54 | 80 | 99 | 108 | 241 | 12 | 73 | 30 | 94 | 27 | 76 | 149 | 185 |
96 | 232 | 87 | 129 | 192 | 195 | 67 | 116 | 240 | 166 | 233 | 188 | 254 | 23 | 69 | 58 |
7 | 187 | 133 | 51 | 203 | 63 | 212 | 29 | 68 | 31 | 153 | 186 | 62 | 74 | 93 | 79 |
124 | 157 | 28 | 173 | 154 | 141 | 77 | 14 | 92 | 146 | 171 | 91 | 229 | 137 | 144 | 85 |
5 | 155 | 193 | 10 | 82 | 36 | 20 | 168 | 174 | 230 | 18 | 121 | 21 | 40 | 71 | 249 |
227 | 130 | 196 | 9 | 252 | 61 | 176 | 134 | 201 | 160 | 178 | 43 | 88 | 117 | 107 | 44 |
22 | 208 | 11 | 150 | 33 | 19 | 66 | 236 | 114 | 246 | 112 | 202 | 118 | 152 | 34 | 194 |
138 | 251 | 197 | 169 | 237 | 26 | 145 | 158 | 56 | 179 | 95 | 15 | 255 | 161 | 159 | 106 |
234 | 120 | 220 | 198 | 110 | 222 | 147 | 183 | 142 | 218 | 123 | 191 | 228 | 131 | 139 | 151 |
245 | 165 | 89 | 163 | 209 | 189 | 206 | 65 | 47 | 225 | 175 | 103 | 53 | 247 | 207 | 75 |
211 | 136 | 226 | 25 | 170 | 242 | 70 | 184 | 126 | 84 | 172 | 49 | 37 | 127 | 204 | 0 |
Step III: Since an S-box is a square matrix of order 16. Therefore, the newly defined notion "matrix transformer" (see Section 2.2) can be used on initial S-box to enhance the security level. For this purpose, we tried several matrix transformers on our initial S-box by using MATLAB program and found that the matrix transformer displayed in Table 5 is the most suitable. The application of this matrix transformer on our initial S-box gives rise an S-box (See Table 6) with very high NL value 111. We call it our proposed S-box. An algorithm illustrating the process of using matrix transformers on the initial S-box is presented in Figure 5, while a flowchart can be found in Figure 6 to facilitate comprehension.
102 | 62 | 108 | 235 | 184 | 163 | 44 | 240 | 53 | 89 | 70 | 150 | 160 | 155 | 220 | 164 |
191 | 172 | 135 | 79 | 174 | 109 | 12 | 201 | 144 | 251 | 133 | 186 | 134 | 71 | 228 | 147 |
96 | 14 | 50 | 114 | 65 | 32 | 106 | 120 | 255 | 218 | 94 | 177 | 136 | 233 | 115 | 219 |
226 | 250 | 211 | 176 | 68 | 230 | 6 | 199 | 156 | 61 | 9 | 165 | 26 | 196 | 139 | 41 |
1 | 22 | 209 | 125 | 215 | 180 | 63 | 113 | 193 | 192 | 241 | 43 | 17 | 127 | 20 | 67 |
169 | 208 | 256 | 198 | 33 | 28 | 243 | 54 | 234 | 45 | 247 | 101 | 73 | 202 | 252 | 248 |
246 | 154 | 207 | 78 | 19 | 3 | 232 | 236 | 224 | 131 | 59 | 31 | 171 | 39 | 238 | 34 |
40 | 24 | 142 | 72 | 83 | 217 | 103 | 82 | 187 | 52 | 210 | 23 | 7 | 205 | 124 | 123 |
64 | 110 | 170 | 153 | 57 | 112 | 253 | 189 | 56 | 229 | 188 | 60 | 86 | 42 | 36 | 121 |
30 | 140 | 76 | 168 | 122 | 141 | 97 | 152 | 146 | 137 | 27 | 16 | 162 | 195 | 145 | 25 |
221 | 105 | 111 | 69 | 81 | 13 | 194 | 15 | 107 | 48 | 249 | 119 | 8 | 74 | 254 | 35 |
117 | 128 | 173 | 2 | 18 | 242 | 90 | 84 | 167 | 116 | 143 | 132 | 11 | 26 | 99 | 46 |
138 | 88 | 190 | 77 | 104 | 231 | 200 | 204 | 151 | 197 | 178 | 158 | 183 | 213 | 87 | 222 |
55 | 58 | 92 | 161 | 37 | 95 | 100 | 166 | 157 | 85 | 148 | 245 | 91 | 179 | 181 | 4 |
75 | 214 | 38 | 98 | 212 | 149 | 5 | 130 | 244 | 175 | 21 | 203 | 51 | 227 | 182 | 66 |
185 | 225 | 47 | 10 | 129 | 206 | 118 | 49 | 80 | 223 | 216 | 237 | 239 | 126 | 93 | 159 |
248 | 150 | 195 | 151 | 206 | 38 | 62 | 88 | 213 | 25 | 118 | 250 | 61 | 48 | 134 | 121 |
3 | 33 | 192 | 224 | 175 | 164 | 186 | 187 | 249 | 152 | 18 | 99 | 72 | 5 | 188 | 59 |
80 | 58 | 44 | 144 | 110 | 89 | 23 | 7 | 143 | 137 | 200 | 113 | 73 | 194 | 226 | 160 |
184 | 101 | 53 | 31 | 32 | 241 | 234 | 92 | 154 | 120 | 233 | 91 | 221 | 41 | 214 | 124 |
156 | 75 | 235 | 46 | 9 | 190 | 81 | 51 | 27 | 117 | 245 | 193 | 169 | 129 | 45 | 126 |
252 | 29 | 203 | 236 | 218 | 229 | 159 | 251 | 4 | 66 | 228 | 220 | 204 | 122 | 222 | 238 |
20 | 163 | 34 | 147 | 94 | 24 | 212 | 237 | 130 | 148 | 201 | 1 | 16 | 157 | 196 | 141 |
223 | 57 | 210 | 246 | 22 | 70 | 43 | 78 | 85 | 82 | 79 | 93 | 215 | 127 | 135 | 208 |
170 | 65 | 166 | 202 | 17 | 244 | 205 | 100 | 230 | 138 | 199 | 155 | 36 | 133 | 112 | 162 |
71 | 174 | 64 | 123 | 189 | 42 | 56 | 168 | 173 | 232 | 102 | 243 | 142 | 15 | 0 | 125 |
198 | 21 | 140 | 60 | 97 | 183 | 114 | 10 | 111 | 28 | 254 | 128 | 11 | 253 | 225 | 239 |
98 | 95 | 131 | 55 | 139 | 207 | 255 | 2 | 211 | 115 | 68 | 171 | 14 | 197 | 8 | 181 |
219 | 176 | 40 | 132 | 179 | 54 | 13 | 145 | 86 | 76 | 103 | 158 | 74 | 242 | 87 | 216 |
83 | 153 | 50 | 209 | 161 | 165 | 119 | 172 | 63 | 105 | 182 | 90 | 227 | 106 | 84 | 240 |
136 | 104 | 247 | 217 | 146 | 231 | 26 | 67 | 39 | 12 | 180 | 116 | 49 | 69 | 37 | 52 |
177 | 19 | 108 | 47 | 191 | 96 | 30 | 185 | 178 | 167 | 109 | 149 | 77 | 107 | 35 | 6 |
This section contains performance evaluation of the suggested S-box through different state of the art metrics such as the nonlinearity test, differential uniformity, bit independence criterion, strict avalanche criterion and linear approximation probability. We see that the outcome scores of our S-box obtained via these analyses are nearly equals to the ideal ones, demonstrating the effectiveness and capability of the proposed scheme. The analyses applied on our S-box are detailed below.
Nonlinearity is a key factor to determine the robustness of a substitution box. If an S-box maps input to output linearly, its resistance is very low [33]. A powerful S-box nonlinearly maps input to output. Any S-box with a higher nonlinearity value guarantees more security against cryptanalytic attacks. In the case of Boolean function of the form θ:Fn2⟶F2, The nonlinearity is calculated as
Nθ=2n−1−12[(h∈GF(2)nmax)|Sθ(h)| | (4.1) |
Note that, Sθ(h)=∑g∈GF(2)n(−1)θ(g)⊕g.h represents the Walsh spectrum of θ(g). Table 7 indicates the nonlinearity values of all Boolean functions of the proposed S-box. The average Non-linearity of our S-box is 110.75.
Boolean mapping | θ0 | θ1 | θ2 | θ3 | θ4 | θ5 | θ6 | θ7 | Mean |
NL score | 110 | 112 | 110 | 112 | 110 | 110 | 110 | 112 | 110 |
SAC is another effective tool to judge the security of an S-box. It was proposed by Webster and Tavares [34]. To meet this requirement, the input bit of any cryptosystem must change along with a 50% change in the output bits. The SAC performance of the S-box is determined by the dependency matrix. The perfect SAC score for the best cryptographic confusion is 0.5. Table 8 shows the dependency matrix of SAC values obtain by proposed S-box. The mean SAC value of proposed S-box is 0.5051, which differs slightly from the optimal value. Therefore, the suggested S-box fulfills the SAC criterion.
0.4844 | 0.5469 | 0.4688 | 0.5625 | 0.5312 | 0.5312 | 0.5156 | 0.4844 |
0.4531 | 0.4844 | 0.5469 | 0.5 | 0.5 | 0.4844 | 0.4844 | 0.5625 |
0.5312 | 0.4688 | 0.5312 | 0.5312 | 0.4375 | 0.4688 | 0.5156 | 0.5 |
0.4375 | 0.5 | 0.5469 | 0.5 | 0.5469 | 0.5312 | 0.4844 | 0.5156 |
0.4531 | 0.5625 | 0.5625 | 0.4688 | 0.4688 | 0.5156 | 0.4375 | 0.5312 |
0.5781 | 0.4844 | 0.5312 | 0.5469 | 0.5156 | 0.5 | 0.5156 | 0.5 |
0.5 | 0.4531 | 0.4531 | 0.4219 | 0.5156 | 0.5469 | 0.5312 | 0.4844 |
0.5 | 0.4844 | 0.5312 | 0.5312 | 0.5 | 0.5312 | 0.4375 | 0.5469 |
This test [34] is satisfied if the output bits operate independently, i.e., do not depend on each other. More specifically, no statistical dependencies or patterns should be present in the bits of the output vectors. It is intended to boost output bit autonomy for greater security. An S-box is said to be meet the BIC criterion if it satisfies SAC and possess nonlinearity score for all Boolean mappings. The Tables 9 and 10 depict the dependency matrices for BIC-nonlinearity and BIC-SAC respectively. The results show that the proposed S-box conforms to the required BIC standards.
- | 110 | 110 | 112 | 112 | 110 | 112 | 110 |
110 | - | 108 | 110 | 112 | 112 | 108 | 110 |
110 | 108 | - | 110 | 112 | 110 | 110 | 112 |
112 | 110 | 110 | - | 110 | 110 | 110 | 112 |
112 | 112 | 112 | 110 | - | 110 | 110 | 110 |
110 | 112 | 110 | 110 | 110 | - | 112 | 110 |
112 | 108 | 110 | 110 | 110 | 112 | - | 112 |
110 | 110 | 112 | 112 | 110 | 110 | 112 | - |
- | 0.4805 | 0.5098 | 0.4941 | 0.4902 | 0.498 | 0.5215 | 0.4824 |
0.4805 | - | 0.5195 | 0.5176 | 0.4922 | 0.4922 | 0.4766 | 0.4902 |
0.5098 | 0.5195 | - | 0.5 | 0.4902 | 0.5137 | 0.4922 | 0.498 |
0.4941 | 0.5176 | 0.5 | - | 0.4961 | 0.5117 | 0.5273 | 0.4902 |
0.4902 | 0.4922 | 0.4902 | 0.4961 | - | 0.4922 | 0.5293 | 0.5195 |
0.498 | 0.4922 | 0.5137 | 0.5117 | 0.4922 | - | 0.4707 | 0.4863 |
0.5215 | 0.4766 | 0.4922 | 0.5273 | 0.5293 | 0.4707 | - | 0.4883 |
0.4824 | 0.4902 | 0.498 | 0.4902 | 0.5195 | 0.4863 | 0.4883 | - |
Modern block ciphers are designed to create as much complexity among the bits as possible to protect the privacy of the information and to offer protection against various decryption techniques employed by the cryptanalysts. It is accomplished by S-box. The lower the value of LP, the better the capability of S-box to withstand linear attacks. The LP value of an S-box can be calculated by using the following equation [35];
LP=(Γw,Γw'≠0max|#{w∈K:w.Γw=S(w).Γw'}2n−12| | (4.2) |
where K={0,1,...,2n} and Γw and Γw' are the input mask and output mask respectively . The designed S-box has an LP score of 0.0781.
The resistance of S-box to differential cryptanalysis is investigated by DU [35]. To determine DU, a input differential Δσi is uniquely linked to an output differential Δρi, for all i. For a given S-box, its value can be calculated by using the following equation:
DU=(Δσi≠0,Δρmaxi{σi∈Γ:S(σi)⨁S(σi+Δσi)=Δρi} | (4.3) |
It is necessary to develop an S-box with smaller DU values in order to withstand differential cryptanalysis attacks. The maximum DU score of the proposed S-box is 6 (See Table 11), indicating its ability to counter differential attacks.
4 | 4 | 4 | 4 | 4 | 4 | 4 | 4 | 4 | 4 | 4 | 4 | 4 | 4 | 4 | 4 |
4 | 4 | 4 | 2 | 4 | 4 | 4 | 4 | 4 | 4 | 4 | 4 | 4 | 4 | 4 | 4 |
4 | 4 | 4 | 4 | 4 | 4 | 4 | 4 | 4 | 4 | 4 | 4 | 4 | 4 | 4 | 6 |
4 | 4 | 4 | 4 | 4 | 6 | 4 | 4 | 4 | 4 | 4 | 4 | 4 | 4 | 4 | 4 |
4 | 4 | 4 | 4 | 4 | 4 | 4 | 4 | 4 | 4 | 4 | 4 | 4 | 4 | 4 | 4 |
4 | 4 | 4 | 4 | 4 | 4 | 4 | 4 | 4 | 4 | 4 | 4 | 6 | 4 | 4 | 4 |
4 | 4 | 4 | 4 | 4 | 4 | 4 | 4 | 4 | 4 | 4 | 4 | 4 | 4 | 4 | 4 |
4 | 4 | 4 | 4 | 4 | 4 | 4 | 4 | 4 | 4 | 4 | 4 | 4 | 4 | 4 | 4 |
4 | 4 | 4 | 6 | 4 | 4 | 6 | 4 | 4 | 4 | 4 | 4 | 4 | 4 | 4 | 4 |
4 | 4 | 4 | 4 | 4 | 4 | 4 | 4 | 4 | 4 | 4 | 4 | 4 | 6 | 4 | 4 |
4 | 4 | 4 | 4 | 4 | 4 | 4 | 4 | 4 | 4 | 4 | 4 | 4 | 4 | 4 | 4 |
4 | 4 | 4 | 4 | 4 | 4 | 4 | 4 | 4 | 4 | 4 | 4 | 4 | 4 | 4 | 4 |
4 | 4 | 4 | 4 | 4 | 4 | 4 | 4 | 4 | 4 | 4 | 4 | 4 | 4 | 4 | 4 |
4 | 4 | 4 | 4 | 4 | 6 | 4 | 6 | 4 | 4 | 4 | 4 | 4 | 4 | 4 | 4 |
6 | 4 | 4 | 4 | 4 | 4 | 4 | 4 | 6 | 4 | 4 | 4 | 4 | 4 | 4 | 4 |
4 | 4 | 4 | 4 | 4 | 4 | 4 | 4 | 4 | 4 | 4 | 4 | 4 | 4 | 4 | - |
According to the performance study and comparative analysis, our S-box has better cryptographic properties than many recently designed S-boxes based on optimization, chaos and algebraic techniques. The comparison present in Table 12 demonstrates the suggested technique of designing S-boxes outperforms many of the available approaches. Here are our findings:
S-box | Nonlinearity Min Max Average |
SAC | BIC-SAC | BIC-NL | DU | LP | ||
Suggested S-box | 110 | 112 | 110.75 | 0.5051 | 0.4989 | 110.55 | 6 | 0.0781 |
AES [36] | 112 | 112 | 112 | 0.5058 | 0.5046 | 112 | 4 | 0.0625 |
Reference [37] | 106 | 108 | 106.25 | 0.5112 | 0.4975 | 103.93 | 12 | 0.1484 |
Reference [38] | 106 | 110 | 106.5 | 0.5010 | 0.4987 | 103.93 | 10 | 0.125 |
Reference [39] | 106 | 108 | 107 | 0.4949 | 0.5019 | 102.29 | 12 | 0.141 |
Reference [40] | 106 | 110 | 108.5 | 0.4995 | 0.5011 | 103.85 | 10 | 0.109 |
Reference [41] | 108 | 110 | 109.75 | 0.5042 | 0.4987 | 110.6 | 6 | 0.0859 |
Reference [42] | 102 | 110 | 106.5 | 0.4943 | 0.5019 | 103.35 | 12 | 0.1468 |
Reference [43] | 104 | 108 | 105.5 | 0.5065 | 0.5031 | 103.57 | 10 | 0.1328 |
Reference [44] | 104 | 110 | 107 | 0.4993 | 0.5050 | 103.29 | 10 | 0.1328 |
1. The S-box must have a high nonlinear value to resist linear attacks. According to Table 12, the average nonlinearity of our S-box is almost equal to AES, outperforming all other S-boxes show in Table 12. Therefore, there is considerable confusion, which makes the proposed method resistant to all the existing linear cryptanalysis.
2. The prime goal of every S-box designer is to achieve a SAC score close to the optimal value (0.50). From Table 12 demonstrates that the suggested S-box satisfies the requirements of strict avalanche criterion.
3. The reading of BIC-NL and BIC-SAC obtained from the prosed S-box are very encouraging are better than those of most of the S-boxes in Table 12
4. A potent S-box has a smaller DU value. As seen in Table 12, the DU score of the suggested S-box is less than the S-boxes developed in [37,38,39,40,41,42,43,44].
5. A smaller LP score makes an S-box more resistant to linear cryptanalysis. The LP score of our S-box is 0.0781, which is slightly higher than AES but lower than the LP values of all S-boxes in Table 12.
The evaluating the suitability of an S-box to be employed in an encryption process using the majority logic criteria (MLC) is a useful approach [45]. Randomness in the encoded picture is assessed using these five analyses energy, entropy, homogeneity, contrast and correlation.
Homogeneity and energy are utilized to identify the features of the encoded picture. The correlation test assesses the resemblance level between the host and encrypted picture. The lower correlation value implies more distortion caused by encryption. Through contrast, the decrease of brightness of the plaintext image is assessed. The greater the contrast value, the more efficient the encryption procedure. The process of encryption distorts the plaintext, and statistical parameters characterize the resiliency of S-box. The S-box that is formed is utilized to encrypt digital photos. To conduct MLC four 256 × 256 JPEG photos of Cameraman, Pepper, Lena and Baboon are selected. Two steps of substitution using our S-box are performed in the encrypting process. Encryption is accomplished through two steps of S-box substitution. During the 1st phase, the substitution is performed in a forward direction (from the start pixel to the end pixel) and subsequently in an opposite way. All simulations were conducted using the MATLAB programming. The original and encrypted photos are shown in Figure 7. The distorted pictures differ significantly from their corresponding undistorted versions. The level of visual distortion is quite large, since the graphics lack a pattern that promotes security breaches from the host picture. Table 13 shows the findings of all MLC testing performed. Table 14 presents the calculated correlation coefficients for pictures.
Image | Correlation | Entropy | Energy | Homogeneity | Contrast |
Cameraman Host | 0.9227 | 7.0097 | 0.1805 | 0.8952 | 0.5871 |
Cameraman-Enc | 0.0394 | 7.9972 | 0.0149 | 0.3999 | 10.0509 |
Pepper Host | 0.9312 | 7.5326 | 0.1096 | 0.8880 | 0.3849 |
Pepper-Enc | 0.0021 | 7.9972 | 0.0156 | 0.3902 | 10.4802 |
Baboon Host | 0.7983 | 7.2649 | 0.0943 | 0.7820 | 0.6326 |
Baboon-Enc | 0.0071 | 7.9975 | 0.0156 | 0.3945 | 10.3994 |
Lena Host | 0.9024 | 7.4439 | 0.1127 | 0.8622 | 0.4482 |
Lena-Enc | − 0.0379 | 7.9976 | 0.0157 | 0.3822 | 10.8896 |
Image | Cameraman | Pepper | Baboon | Lena | |
Vertical | Plain Image | 0.9745 | 0.9137 | 0.9090 | 0.9321 |
Distorted Image | 0.0310 | − 0.0392 | − 0.0128 | − 0.0117 | |
Horizontal | Plain Image | 0.9610 | 0.9204 | 0.8727 | 0.883 |
Distorted Image | − 0.0026 | − 0.0015 | 0.0039 | − 0.0021 |
The results suggest that the created substitution box is suitable for encryption purposes and are good enough to be used in the systems designed to ensure the reliability and security of sensitive data.
The experimental assessments of the proposed image encryption technique are discussed in this section. The 256×256 pixel grayscale photos of Cameraman, Pepper and Baboon are picked for the experiment. Table 15 contains a variety of image quality measurements that have been suggested for use with two rounds of encryption using S-boxes. These methods have been thoroughly discussed. The findings indicate that the recommended S-box is robust enough to survive various attacks.
Test | Cameraman-Enc | Pepper-Enc | Baboon-Enc | Lena-Enc |
MSE | 9212.16 | 8656.41 | 7854.44 | 8414.71 |
MSE [6] | 9079.09 | 8190.01 | 8011.23 | 8239.51 |
MSE [15] | 9189.41 | 8612.09 | 7599.03 | 7930.39 |
MSE [17] | 9187.38 | 8423.61 | 7865.21 | 8274.13 |
PSNR | 8.4723 | 8.8563 | 9.8912 | 8.9912 |
PSNR [6] | 8.1129 | 8.9710 | 8.5539 | 9.1902 |
PSNR [15] | 8.2897 | 8.7091 | 8.1331 | 8.9500 |
PSNR [17] | 8.2891 | 8.3353 | 8.9361 | 8.0032 |
SSIM1 | 0.0009 | 0.0012 | 0.0010 | 0.0011 |
SSIM1 [6] | 0.0013 | 0.0008 | 0.0011 | 0.0012 |
SSIM1 [15] | 0.0010 | 0.0012 | 0.0008 | 0.0014 |
SSIM1 [17] | 0.0009 | 0.0015 | 0.0012 | 0.0013 |
NCC | 0.8633 | 0.8710 | 0.9121 | 0.8803 |
NCC [6] | 0.8537 | 0.8675 | 0.8912 | 0.9016 |
NCC [15] | 0.8733 | 0.8712 | 0.8543 | 0.8461 |
NCC [17] | 0.8640 | 0.87134 | 0.9001 | 0.8692 |
AD | −7.4523 | −4.9812 | −2.3419 | −5.3881 |
AD [6] | −3,4511 | −5.6634 | −1.4529 | −2.3319 |
AD [15] | −6,7819 | −3.8873 | −2.8827 | −4.1198 |
AD [17] | −3.4429 | −4.9821 | −2.3872 | −7.6594 |
SC | 0.8496 | 0.8345 | 0.8456 | 0.8247 |
SC [6] | 0.8455 | 0.8451 | 0.8401 | 0.8342 |
SC [15] | 0.8341 | 0.8489 | 0.8465 | 0.8231 |
SC [17] | 0.8436 | 0.8111 | 0.8265 | 0.8490 |
MD | 240 | 238 | 212 | 234 |
MD [6] | 211 | 231 | 233 | 241 |
MD [15] | 223 | 227 | 227 | 228 |
MD [17] | 234 | 238 | 221 | 219 |
NAE | 0.6358 | 0.6273 | 0.6147 | 0.6384 |
NAE [6] | 0.6455 | 0.5932 | 0.5813 | 0.6459 |
NAE [15] | 0.6040 | 0.6193 | 0.6388 | 0.6026 |
NAE [17] | 0.6219 | 0.6243 | 0.6012 | 0.5856 |
RMSE | 94.6682 | 91.7245 | 84.9561 | 87.9349 |
RMSE [6] | 90.6638 | 92.3402 | 88.3476 | 86.7938 |
RMSE [15] | 93,4428 | 89.7690 | 91.2398 | 87.3947 |
RMSE [17] | 90.4582 | 85.1109 | 84.8934 | 88.1831 |
UQI | 0.0218 | 0.0332 | 0.0314 | 0.0338 |
UQI [6] | 0.0127 | 0.0412 | 0.0279 | 0.0178 |
UQI [15] | 0.0347 | 0.0456 | 0.0127 | 0.0391 |
UQI [17] | 0.0234 | 0.0401 | 0.0298 | 0.0281 |
MI | −1.0292 | −1.0187 | −1.0184 | −1.0281 |
MI [6] | −1.0195 | −1.0490 | −1.0328 | −1.0402 |
MI [15] | −1.0371 | −1.0197 | −1.0294 | −1.0406 |
MI [17] | −1.341 | −1.0198 | −1.0384 | −1.0327 |
During encryption MSE analysis assesses the unpredictability of the encrypted picture [46]. This technique computes the squared difference between the original and distorted picture. It can be computed as follows:
MSE=1U×V∑Uy2=1∑Vy1=1(O(y1,y2)−E(y1,y2))2 | (6.1) |
where U and V represent the dimensions of original O(y1,y2) and distorted E(y1,y2) pictures respectively. For effective encryption methods, the MSE rating must be as high as conceivable [46].
The PSNR test [38] is an ideal criterion for assessing the quality of picture encryption techniques. It estimates how well the original picture matches the ciphertext. PSNR value is calculated using the following formula;
PSNR=10log10V2√MSE | (6.2) |
where V is the amount of variance that was at its highest in the original picture data. It is necessary to have a higher value of PSNR in order to get a superior encoded picture [47].
To determine the average and maximum dissimilarities between the unencrypted O and encrypted E pictures, researchers used the AD and MD test [47]. AD and MD values are determined using the following formulas;
AD=∑Ry2=1∑Sy1=1[O(y1,y2)−E(y1,y2)]R×S | (6.3) |
MD=max|O(y1,y2)−E(y1,y2)| | (6.4) |
MI measures how much information can be retrieved about the original picture from a distorted version of it. Let us denote the joint probability function of O and E by ρ(y1,y2), then the value of MI can be determined by using the formula below;
MI=∑y1∈O∑y2∈Eρ(y1,y2)log2ρ(y1,y2)ρ(y1)ρ(y2) | (6.5) |
The MI value must always be kept to a minimum in a decent encryption system [48].
As stated in reference [49], the UQI method partitions the evaluation of image distortion into three components: luminance, structural comparisons and contrast. The UQI metric for a pair of images O and E can be expressed as follows;
UQ(O,E)=4ρoρEρoE(ρ20−ρ2E)(φ20−φ2E) | (6.6) |
where ρo, ρE represent the mean values of the original and distorted images, respectively, and φo, φE represent the standard deviation of the original and distorted images, respectively.
SSIM is an enhanced version of the UQI designed to assess the similarity between two images. In particular, SSIM evaluates the fidelity of one of the images by assuming that the other image is free from errors. The computation of the SSIM score involves analyzing a pair of windows (R,S) of the image using the following formula:
SSIM(R,S)=(νRνS+a1)(2φRφS+a2)(ν2R+ν2R+a1)(φ2R+φ2R+a2) | (6.7) |
where φR and φS are the variances of R and S and νR and νS are the average scores of R and S respectively. The range of the SSIM score lies between -1 to 1, where a score of 1 indicates that the images are identical. A score close to 0 indicates a strong encryption scheme [48].
As stated in citation [49], the correlation function provides a means of measuring the proximity of two digital images. The NCC method is a well-established technique for assessing the similarity between two images. Its calculation is based on the following formula:
NCC=∑Uy2=1∑Vy1=1(O(y1,y2)×E(y1,y2)∑Uy2=1∑Vy1=1|O(y1,y2)|2) | (6.8) |
NAE [49] can be used to assess the efficiency of an image encryption process by comparing the pixel values of the original image with those of the encrypted (ciphered) image. To calculate the NAE between the plain and ciphered image, the formula is:
NAE=∑Uy2=1∑Vy1=1|O(y1,y2)−E(y1,y2)|∑Uy2=1∑Vy1=1|O(y1,y2)| | (6.9) |
The assessment of an image encryption algorithm's effectiveness can be facilitated by utilizing RMSE as a performance metric. The calculation of RMSE involves determining the square root of the average of all the squared errors [49]. Its frequent use and flexibility make it a versatile and valuable error metric for numerical forecasting. The mathematical expression for RMSE is indicated below;
RMSE=√∑Uy2=1∑Vy1=1|O(y1,y2)−E(y1,y2)|2U×V | (6.10) |
SC is a correlation-based measure that quantifies the similarity between two images. The following mathematical equation is used to compute its score;
SC=∑Uy2=1∑Vy1=1|O(y1,y2)|2∑Uy2=1∑Vy1=1|E(y1,y2)|2 | (6.11) |
A robust cryptosystem is extremely sensitive to modifications in one bit of the plaintext. Through UACI, NPCR and BACI testing, the sensitivity of the system is assessed.
UACI indicates the unified mean intensity change between original and encrypted image while NPCR calculates the number of pixels change rate of the encrypted image if a single pixel is altered in the original image. In BACI analysis, the image difference Δ=abs(E1−E2) is partitioned into blocks of pixels and arranged in a 2 × 2 matrix. This involves dividing the image into smaller, non-overlapping regions, or "blocks", to facilitate the comparison of pixel values before and after the intervention.
The following formulae are used to compute the values of UACI, NPCR and BACI:
UACI=1JK∑j,kE1(j,k)−E2(j,k)255×100% | (7.1) |
NPCR=∑jkD(j,k)JK×100% | (7.2) |
BACI=1(J−1)(K−1)∑(J−1)(K−1)i=1Zi255×100% | (7.3) |
where E1(j,k) and E2(j,k) denote the grayscale values of pixels obtained (j,k) th position and D(j,k)={0 if E1(j,k) and E2(j,k) are equal 1 otherwise and Zi=16(|a1−a2|+|a1−a3|+|a1−a4|+|a2−a3|+|a2−a4|+|a3−a4|) and Δi=[a1a2a3a4].
Table 16 depicts the findings of the differential analysis for NPCR, UACI, and BACI, confirming the excellent performance of encryption effect provided by the designed S-box.
Image | NPCR | UACI | BACI |
Cameraman | 99.63% | 33.12% | 24.60% |
Pepper | 99.81% | 33.21% | 26.38% |
Baboon | 99.76% | 32.86% | 24.25% |
Lena | 99.79% | 33.16% | 23.09% |
Summing up, the present work has discussed and examined the development of modular group coset graphs over a finite field of order 1024 and a matrix transformer for application in S-box construction. An initial S-box is formed through coset graphs and after that the application of a matrix transformer on it enhances its working efficiency significantly, resulting in a robust S-box. Comparison of proposed method with other state-of-the-art S-box construction algorithms shows that the proposed mechanism outperforms other algorithms in terms of mean nonlinear score, LP, SAC, BIC and DU scores. In addition, the performance of the designed S-box when applied to encrypt certain plaintext graphics has been determined to be extraordinary using a variety of assessment tools.
As our experience with applying matrix transformer to coset graph S-box to improve its resilience has been promising, we plan to research novel ways for designing S-boxes combining matrix transformers and chaotic systems. Moreover, we intend to evaluate the application of S-box to cloud encryption.
This work was supported by the Deanship of Scientific Research, Vice Presidency for Graduate Studies and Scientific Research, King Faisal University, Saudi Arabia (Grant No. 3011).
The authors declare there is no conflict of interest.
[1] |
M. C. Chen, Y. H. Hsiao, H. Y. Huang, Semiconductor supply chain planning with decisions of decoupling point and VMI scenario, IEEE Trans. Syst. Man. Cybern. Syst., 47 (2017), 856-868. https://doi.org/10.1109/tsmc.2016.2521740 doi: 10.1109/tsmc.2016.2521740
![]() |
[2] |
K. Govindan, A. Jafarian, R. Khodaverdi, K. Devika, Two-echelon multiple-vehicle location-routing problem with time windows for optimization of sustainable supply chain network of perishable food, Int. J. Prod. Econ., 152 (2014), 9-28. https://doi.org/10.1016/j.ijpe.2013.12.028 doi: 10.1016/j.ijpe.2013.12.028
![]() |
[3] |
T. Trisna, M. Marimin, Y. Arkeman, T. C. Sunarti, Multi-objective optimization for supply chain ma-nagement problem: A literature review, Decis. Sci. Lett., 5 (2016), 283-316. https://doi.org/10.5267/j.dsl.2015.10.003 doi: 10.5267/j.dsl.2015.10.003
![]() |
[4] |
S. K. De, K. Bhattacharya, B. Roy, Solution of a pollution sensitive supply chain model under fuzzy approximate reasoning, Int. J. Intell. Syst., 36 (2021), 5530-5572. https://doi.org/10.1002/int.22522 doi: 10.1002/int.22522
![]() |
[5] |
S. K. Srivastava, Green supply-chain management: A state-of-the-art literature review, Int. J. Manag. Rev., 9 (2007), 53-80. https://doi.org/10.1111/j.1468-2370.2007.00202.x doi: 10.1111/j.1468-2370.2007.00202.x
![]() |
[6] |
S. H. Amin, G. Q. Zhang, M. N. Eldali, A review of closed-loop supply chain models, J. Data Inf. Manage., 2 (2020), 279-307. https://doi.org/10.1007/s42488-020-00034-y doi: 10.1007/s42488-020-00034-y
![]() |
[7] |
B. Mosallanezhad, M. Hajiaghaei-Keshteli, C. Triki, Shrimp closed-loop supply chain network design, Soft Comput., 25 (2021), 7399-7422. https://doi.org/10.1007/s00500-021-05698-1 doi: 10.1007/s00500-021-05698-1
![]() |
[8] | A. Salehi-Amiri, A. Zahedi, N. Akbapour, M, Hajiaghaei-Keshteli, Designing a sustainable closed-loop supply chain network for walnut industry, Renew Sustainable Energy Rev., 141 (2021). https://doi.org/10.1016/j.rser.2021.110821 |
[9] | A. Cheraghalipour, M. M. Paydar, M, Hajiaghaei-Keshteli, A bi-objective optimization for citrus closed-loop supply chain using Pareto-based algorithms, Appl. Soft Comput., 69 (2018), 33-59. https://doi.rog/10.1016/j.asoc.2018.04.022 |
[10] | A. M. Fathollahi-Fard, A. Ahmadi, S. M. J. M. Al-e-Hashem, Sustainable closed-loop supply chain network for an integrated water supply and wastewater collection system under uncertainty, J. Environ. Manag., 275 (2020). https://doi.org/10.1016/j.jenvman.2020.111277 |
[11] |
A. M. Fathollahi-Fard, M, Hajiaghaei-Keshteli, S. Mirjalili, Multi-objective stochastic closed-loop supply chain network design with social considerations, Appl. Soft Comput., 71 (2018), 505-525. https://doi.org/10.1016/j.asoc.2018.07.025 doi: 10.1016/j.asoc.2018.07.025
![]() |
[12] |
V. K. Chouhan, S. H. Khan, M. Hajiaghaei-Keshteli, S. Subramanian, Multi-facility-based improved closed-loop supply chain network for handling uncertain demands, Soft Comput., 24 (2020), 7125-7147, https://doi.org/10.1007/s00500-020-04868-x doi: 10.1007/s00500-020-04868-x
![]() |
[13] | A. M. Fathollahi-Fard, M. A. Dulebenets, M. Hajiaghaei-Keshteli, R. Tavakkoli-Moghaddam, M. Safaeian, H. Mirzahosseinian, Two hybrid meta-heuristic algorithms for a dual-channel closed-loop supply chain network design problem in the tire industry under uncertainty, Adv. Eng. Inf., 50 (2021). https://doi.org/10.1016/j.aei.2021.101418 |
[14] | E. Lesnaia, I. Vasilescu, S. C. Graves, The complexity of safety stock placement in general-network supply chains, in Innovation in Manufacturing Systems and Technology (IMST), 1 (2005). http://hdl.handle.net/1721.1/7537 |
[15] |
A. Niccolai, L. Bettini, R. Zich, Optimization of electric vehicles charging station deployment by means of evolutionary algorithms, Int. J. Intell. Syst., 36 (2021), 5359-5383. https://doi.org/10.1002/int.22515 doi: 10.1002/int.22515
![]() |
[16] |
H. B. Ammar, W. B. Yahia, O, Ayadi, F. Masmoudi, Design of efficient multiobjective binary PSO algorithms for solving multi-item capacitated lot-sizing problem, Int. J. Intell. Syst., 37 (2021), 1-28. https://doi.org/10.1002/int.22693 doi: 10.1002/int.22693
![]() |
[17] | M. Mojtahedi, A. M. Fathollahi-Fard, R. Tavakkoli-Moghaddam, S. Newton, Sustainable vehicle routing problem for coordinated solid waste management, J. Ind. Inf. Integr., 23 (2021). https://doi.org/10.1016/j.jii.2021.100220 |
[18] | A. M. Fathollahi-Fard, A. Ahmadi, B. Karimi, Sustainable and robust home healthcare logistics: A response to the Covid-19 pandemic, Symmetry, 14 (2), 193. https://doi.org/10.3390/sym14020193 |
[19] |
H. J. Ko, G. W. Evans, A genetic algorithm-based heuristic for the dynamic integrated forward/reverse logistics network for 3PLs, Comput. Oper. Res., 34 (2007), 346-366. https://doi.org/10.1016/j.cor.2005.03.004 doi: 10.1016/j.cor.2005.03.004
![]() |
[20] |
S. Hamed, K. Govindan, A hybrid particle swarm optimization and genetic algorithm for closed-loop supply chain network design in large-scale networks, Appl. Math. Model., 39 (2015), 3990-4012. https://doi.org/10.1016/j.apm.2014.12.016 doi: 10.1016/j.apm.2014.12.016
![]() |
[21] | A. S. Abir, I. A. Bhuiyan, M. Arani, M. M. Billal, Multi-objective optimization for sustainable closed-loop supply chain network under demand uncertainty: A genetic algorithm, in 2020 International Conference on Data Analytics for Business and Industry: Way Towards a Sustainable Economy (ICDABI), (2020), 1-5. https://doi.org/10.1109/ICDABI51230.2020.9325648 |
[22] |
X. Zhang, K. J. Du, Z. H. Zhan, S. Kwong, T. L. Gu, J. Zhang, Cooperative coevolutionary bare-bones particle swarm optimization with function independent decomposition for large-scale supply chain network design with uncertainties, IEEE Trans. Cybern., 50 (2020), 4454-4468. https://doi.org/10.1109/TCYB.2019.2937565 doi: 10.1109/TCYB.2019.2937565
![]() |
[23] | W. C. Yeh, T. L. W, C. M. L, Y. C. Lee, Y. Y. Chung, J. S. Lin, Application of simplified swarm optimization algorithm in deteriorate supply chain network problem, in 2016 IEEE Congress on Evolutionary Computation (CEC), (2016), 2695-2700. https://doi.org/10.1109/CEC.2016.7744127 |
[24] |
K. Patne, N. Shukla, S. Kiridena, M. K. Tiwari, Solving closed-loop supply chain problems using game theoretic particle swarm optimisation, Int. J. Prod. Res., 56 (2018), 5836-5853. https://doi.org/10.1080/00207543.2018.1478149 doi: 10.1080/00207543.2018.1478149
![]() |
[25] | V. M. Esteves, M. C. Joao, C. A. Silva, A. P. Póvoa, M. I. Gomes, SCant-design: Closed loop supply chain design using ant colony optimization, in 2012 IEEE Congress on Evolutionary Computation, (2012), 1-8. https://doi.org/10.1109/CEC.2012.6252944 |
[26] | A. Samadi, M, Hajiaghaei-Keshteli, R. Tavakkoli-Moghaddam, Solving a discounted closed-loop supply chain network design problem by recent metaheuristics, in Fuzzy Information and Engineering-2019, Springer Singapore, (2020), 3-24. https://doi.org/10.1007/978-981-15-2419-2_1 |
[27] |
A. M. Fathollahi-Fard, M, Hajiaghaei-Keshteli, S. Mirjalili, Hybrid optimizers to solve a tri-level programming model for a tire closed-loop supply chain network design problem, Appl. Soft Comput., 70 (2018), 701-722. https://doi.org/10.1016/j.asoc.2018.06.021 doi: 10.1016/j.asoc.2018.06.021
![]() |
[28] |
M, Hajiaghaei-Keshteli, A. M. Fathollahi-Fard, Sustainable closed-loop supply chain network design with discount supposition, Neural Comput. Appl., 31 (2019), 5343-5377. https://doi.org/10.1007/s00521-018-3369-5 doi: 10.1007/s00521-018-3369-5
![]() |
[29] |
M. Gen, F. Altiparmak, L. Lin, A genetic algorithm for two-stage transportation problem using priority-based encoding, OR Spectrum, 28 (2006), 337-354. https://doi.org/10.1007/s00291-005-0029-9 doi: 10.1007/s00291-005-0029-9
![]() |
[30] |
B. Fahimnia, H. Davarzani, A. Eshragh, Planning of complex supply chains: A performance comp-arison of three meta-heuristic algorithms, Comput. Oper. Res., 89 (2018), 241-252. https://doi.org/10.1016/j.cor.2015.10.008 doi: 10.1016/j.cor.2015.10.008
![]() |
[31] |
N. Sahebjamnia, A. M. Fathollahi-Fard, M. Hajiaghaei-Keshteli, Sustainable tire closed-loop supply chain network design: Hybrid metaheuristic algorithms for large-scale networks, J. Clean Prod., 196 (2018), 273-296. https://doi.org/10.1016/j.jclepro.2018.05.245 doi: 10.1016/j.jclepro.2018.05.245
![]() |
[32] | S. S. Theagarajan, H. L. Manohar, Lean management practices to improve supply chain p-erformance of leather footwear industry, in 2015 International Conference on IndustrialEngineering and Operations Management (IEOM), (2015), 1-5. https://doi.org/10.1109/IEOM.2015.7093717 |
[33] |
M. A. Dulebenets, An adaptive polyploid memetic algorithm for scheduling trucks at a cross-docking terminal, Inf. Sci., 565 (2021), 390-421. https://doi.org/10.1016/j.ins.2021.02.039 doi: 10.1016/j.ins.2021.02.039
![]() |
[34] |
M. A. Dulebenets, A comprehensive multi-objective optimization model for the vessel sc-heduling problem in liner shipping, Int. J. Prod. Econ., 196 (2018), 293-318. https://doi.org/10.1016/j.ijpe.2017.10.027 doi: 10.1016/j.ijpe.2017.10.027
![]() |
[35] |
J. Pasha, M. A. Dulebenets, M. Kavoosi, O. F. Abloye, H. Wang, W. Guo, An optimiz-ation model and solution algorithms for the vehicle routing problem with a ''factory-in-a-box'', IEEE Access, 8 (2020), 134743-134763. https://doi.org/10.1109/ACCESS.2020.3010176 doi: 10.1109/ACCESS.2020.3010176
![]() |
[36] |
H. Zhao, C. Zhang, An online-learning-based evolutionary many-objective algorithm, Inf. Sci., 509 (2020), 1-21. https://doi.org/10.1016/j.ins.2019.08.069 doi: 10.1016/j.ins.2019.08.069
![]() |
[37] |
Y. Tian, T. Zhang, J. Xiao, X. Zhang, Y. Jin, A coevolutionary framework for constrained multi-objective optimization problems, IEEE Trans. Evol. Comput., 25 (2021), 102-116. https://doi.org/10.1109/TEVC.2020.3004012 doi: 10.1109/TEVC.2020.3004012
![]() |
[38] |
K. Li, R. Chen, G. Fu, X. Yao, Two-archive evolutionary algorithm for constrained multiobjective optimization, IEEE Trans. Evol. Comput., 23 (2019), 303-315. https://doi.org/10.1109/TEVC.2018.2855411 doi: 10.1109/TEVC.2018.2855411
![]() |
[39] | P. Wang, B. Xue, M. Zhang, J. Liang, A grid-dominance based multi-objective algorithm for feature selection in classification, in 2021 IEEE Congress on Evolutionary Computation (CEC), (2021), 2053-2060. https://doi.org/10.1109/CEC45853.2021.9504832 |
[40] | A. Li, B. Xue, M. Zhang, A forward search inspired particle swarm optimization algorithm for feature selection in classification, in 2021 IEEE Congress on Evolutionary Computation (CEC), (2021), 786-793. https://doi.org/10.1109/CEC45853.2021.9504949 |
[41] | A. Lipowski, D. Lipowska, Roulette-wheel selection via stochastic acceptance, Phys. A, 391 (2012), 2193-2196. https://doi.org/doi.org/10.1016/j.physa.2011.12.004 |
[42] |
S. Das, P. N. Suganthan, Differential evolution: A survey of the state-of-the-art, IEEE Trans. Evol. Comput., 15 (2011), 4-31. https://doi.org/10.1109/tevc.2010.2059031 doi: 10.1109/tevc.2010.2059031
![]() |
[43] |
R. Cheng, Y. C. Jin, A competitive swarm optimizer for large scale optimization, IEEE Trans. Cybern., 45 (2015), 191-204. https://doi.org/10.1109/TCYB.2014.2322602 doi: 10.1109/TCYB.2014.2322602
![]() |
[44] |
A. Faramarzi, M. Heidarinejad, S. Mirjalili, A. H. Gandomi, Marine Predators Algorithm: A nature-inspired metaheuristic, Expert Syst. Appl., 152 (2020), 1-28. https://doi.org/10.1016/j.eswa.2020.113377 doi: 10.1016/j.eswa.2020.113377
![]() |
[45] |
A. M. Fathollahi-Fard, M. Hajiaghaei-Keshteli, R. Tavakkoli-Moghaddam, Red deer algorithm (RDA):a new nature-inspired meta-heuristic, Soft Comput., 24 (2020), 14637-14665. https://doi.org/10.1007/s00500-020-04812-z doi: 10.1007/s00500-020-04812-z
![]() |
[46] |
R. Cheng, Y. C. Jin, A social learning particle swarm optimization algorithm for scalable optimization, Inf. Sci., 291 (2015), 43-60. https://doi.org/10.1016/j.ins.2014.08.039 doi: 10.1016/j.ins.2014.08.039
![]() |
[47] | L. Feng, Y. Huang, L. Zhou, J. Zhong, A. Gupta, K. Tang, et al., Explicit evolutionary multitasking for combinatorial optimization: A case study on capacitated vehicle routing problem, IEEE Trans. Evol. Comput., 51 (2021), 3143-3156. https://doi.org/10.1109/TCYB.2019.2962865 |
[48] | L. Feng, L. Zhou, A. Gupta, J. Zhong, Z. Zhu, K. C. Tan, et al., Solving generalized vehicle routing problem with occasional drivers via evolutionary multitasking, IEEE Trans. Cybern., 51 (2021), 3171-3184. https://doi.org/10.1109/TCYB.2019.2955599 |
1. | Abdul Razaq, Louai A. Maghrabi, Musheer Ahmad, Farrah Aslam, Wei Feng, Fuzzy Logic-Based Substitution-Box for Robust Medical Image Encryption in Telemedicine, 2024, 12, 2169-3536, 7584, 10.1109/ACCESS.2024.3351794 | |
2. | Abdul Razaq, Louai A. Maghrabi, Musheer Ahmad, Qamar H. Naith, Novel substitution-box generation using group theory for secure medical image encryption in E-healthcare, 2024, 9, 2473-6988, 6207, 10.3934/math.2024303 | |
3. | Mohammad Mazyad Hazzazi, Souad Ahmad Baowidan, Awais Yousaf, Muhammad Adeel, An Innovative Algorithm Based on Chaotic Maps Amalgamated with Bit-Level Permutations for Robust S-Box Construction and Its Application in Medical Image Privacy, 2024, 16, 2073-8994, 1070, 10.3390/sym16081070 | |
4. | Dhakshinamoorthy Vignesh, Nur Aisyah Abdul Fataf, Santo Banerjee, A Novel Fractional Sine Chaotic Map and Its Application to Image Encryption and Watermarking, 2023, 13, 2076-3417, 6556, 10.3390/app13116556 | |
5. | Ahmet Malal, Cihangir Tezcan, FPGA-friendly compact and efficient AES-like 8 × 8 S-box, 2024, 105, 01419331, 105007, 10.1016/j.micpro.2024.105007 | |
6. | Yilmaz Aydin, Ali Murat Garipcan, Fatih Özkaynak, A Novel Secure S-box Design Methodology Based on FPGA and SHA-256 Hash Algorithm for Block Cipher Algorithms, 2024, 2193-567X, 10.1007/s13369-024-09251-8 | |
7. | Jieun Ryu, Yongbhin Kim, Seungtai Yoon, Ju-Sung Kang, Yongjin Yeom, IPCC7: Post-Quantum Encryption Scheme Based on a Perfect Dominating Set in 3-Regular Graph, 2024, 12, 2169-3536, 4575, 10.1109/ACCESS.2024.3349704 | |
8. | Anand Prakash Dube, Raghav Yadav, 2024, Enhanced Dynamic S-Box Design Based on Adaptive Heuristic Evolution and Multi-Stage Nonlinearity for Securing IoT-Based Remote Health Monitoring Systems, 979-8-3315-0843-2, 1, 10.1109/ICEC59683.2024.10837025 | |
9. | Yong Zhang, Image encryption algorithm based on butterfly module and chaos, 2025, 03784754, 10.1016/j.matcom.2025.01.011 |
Binary form | Decimal | 𝐺𝐹 (210) | Binary form | Decimal | 𝐺𝐹(210) | Binary form | Decimal | 𝐺𝐹(210) | Binary form | Decimal | 𝐺𝐹(210) |
0000000000 | 0 | 0 | 0000000001 | 1 | 1 | 0000000010 | 2 | κ1 | 0000000100 | 4 | κ2 |
0000001000 | 8 | κ3 | 0000010000 | 16 | κ4 | 0000100000 | 32 | κ5 | 0001000000 | 64 | κ6 |
0010000000 | 128 | κ7 | 0100000000 | 256 | κ8 | 1000000000 | 512 | κ9 | 0010000001 | 129 | κ10 |
0100000010 | 258 | κ11 | 1000000100 | 516 | κ12 | 0010001001 | 137 | κ13 | 0100010010 | 274 | κ14 |
1000100100 | 548 | κ15 | 0011001001 | 201 | κ16 | 0110010010 | 402 | κ17 | 1100100100 | 804 | κ18 |
1011001001 | 713 | κ19 | 0100010011 | 275 | κ20 | 1000100110 | 550 | κ21 | 0011001101 | 205 | κ22 |
… | … | … | … | … | … | … | … | … | … | … | … |
… | … | … | … | … | … | … | … | … | … | … | … |
… | … | … | … | … | … | … | … | … | … | … | … |
1010111011 | 699 | κ311 | 0111110111 | 503 | κ312 | 1111101110 | 1006 | κ313 | 1101011101 | 861 | κ314 |
1000111011 | 571 | κ315 | 0011110111 | 247 | κ316 | 0111101110 | 494 | κ317 | 1111011100 | 988 | κ318 |
1100111001 | 825 | κ319 | 1011110011 | 755 | κ320 | 0101100111 | 359 | κ321 | 1011001110 | 718 | κ322 |
0100011101 | 285 | κ323 | 1000111010 | 570 | κ324 | 0011110101 | 245 | κ325 | 0111101010 | 490 | κ326 |
… | … | … | … | … | … | … | … | … | … | … | … |
… | … | … | … | … | … | … | … | … | … | … | … |
… | … | … | … | … | … | … | … | … | … | … | … |
0100110000 | 304 | κ1007 | 1001100000 | 608 | κ1008 | 0001000001 | 65 | κ1009 | 0010000010 | 130 | κ1010 |
0100000100 | 260 | κ1011 | 1000001000 | 520 | κ1012 | 0010010001 | 145 | κ1013 | 0100100010 | 290 | κ1014 |
1001000100 | 580 | κ1015 | 0000001001 | 9 | κ1016 | 0000010010 | 18 | κ1017 | 0000100100 | 36 | κ1018 |
0001001000 | 72 | κ1019 | 0010010000 | 144 | κ1020 | 0100100000 | 288 | κ1021 | 1001000000 | 576 | κ1022 |
κ1 | κ77 | κ1021 | κ869 | κ1013 | κ5 | κ513 | κ1017 | κ1009 | κ9 | κ189 | κ13 | κ301 | κ709 | κ353 | κ193 |
κ209 | κ17 | κ729 | κ1005 | κ393 | κ21 | κ305 | κ1001 | κ645 | κ937 | κ25 | κ385 | κ997 | κ421 | κ817 | κ29 |
κ537 | κ457 | κ993 | κ317 | κ265 | κ637 | κ605 | κ33 | κ989 | κ401 | κ909 | κ149 | κ237 | κ273 | κ37 | κ161 |
κ985 | κ473 | κ545 | κ41 | κ849 | κ981 | κ413 | κ45 | κ977 | κ897 | κ673 | κ397 | κ49 | κ225 | κ749 | κ973 |
κ253 | κ109 | κ965 | κ181 | κ233 | κ53 | κ481 | κ969 | κ665 | κ213 | κ865 | κ57 | κ437 | κ529 | κ345 | κ737 |
κ449 | κ389 | κ61 | κ917 | κ961 | κ493 | κ65 | κ621 | κ957 | κ297 | κ945 | κ145 | κ153 | κ221 | κ69 | κ953 |
κ725 | κ261 | κ549 | κ477 | κ73 | κ949 | κ701 | κ405 | κ81 | κ177 | κ765 | κ941 | κ593 | κ785 | κ321 | κ113 |
κ197 | κ85 | κ349 | κ589 | κ489 | κ577 | κ89 | κ893 | κ933 | κ761 | κ93 | κ657 | κ929 | κ229 | κ721 | κ97 |
κ925 | κ573 | κ165 | κ417 | κ517 | κ101 | κ789 | κ133 | κ921 | κ805 | κ601 | κ525 | κ661 | κ557 | κ105 | κ837 |
κ269 | κ913 | κ597 | κ169 | κ705 | κ117 | κ461 | κ445 | κ905 | κ333 | κ553 | κ125 | κ245 | κ121 | κ357 | κ901 |
κ689 | κ257 | κ521 | κ649 | κ129 | κ561 | κ429 | κ889 | κ733 | κ329 | κ829 | κ717 | κ581 | κ137 | κ885 | κ873 |
κ141 | κ881 | κ501 | κ541 | κ877 | κ777 | κ853 | κ325 | κ157 | κ361 | κ505 | κ569 | κ613 | κ861 | κ669 | κ857 |
κ381 | κ797 | κ629 | κ173 | κ313 | κ845 | κ585 | κ841 | κ425 | κ465 | κ741 | κ185 | κ377 | κ565 | κ833 | κ609 |
κ825 | κ693 | κ201 | κ745 | κ821 | κ757 | κ205 | κ813 | κ441 | κ249 | κ809 | κ485 | κ409 | κ625 | κ217 | κ337 |
κ469 | κ801 | κ685 | κ793 | κ617 | κ773 | κ533 | κ241 | κ681 | κ781 | κ309 | κ497 | κ293 | κ769 | κ509 | κ433 |
κ633 | κ653 | κ753 | κ365 | κ277 | κ281 | κ453 | κ289 | κ285 | κ677 | κ641 | κ713 | κ373 | κ697 | κ369 | κ381 |
Binary form | Decimal | 𝐺𝐹 (28) | Binary form | Decimal | 𝐺𝐹 (28) | Binary form | Decimal | 𝐺𝐹 (28) | Binary form | Decimal | 𝐺𝐹 (28) |
00000000 | 0 | 0 | 00000001 | 1 | 1 | 00000010 | 2 | β1 | 00000100 | 4 | β2 |
00001000 | 8 | β3 | 00010000 | 16 | β4 | 00100000 | 32 | β5 | 01000000 | 64 | β6 |
10000000 | 128 | β7 | 01101001 | 105 | β8 | 11010010 | 210 | β9 | 11001101 | 205 | β10 |
11110011 | 243 | β11 | 10001111 | 143 | β12 | 01110111 | 119 | β13 | 11101110 | 238 | β14 |
… | … | … | … | … | … | … | … | … | … | … | … |
… | … | … | … | … | … | … | … | … | … | … | … |
… | … | … | … | … | … | … | … | … | … | … | … |
10101100 | 172 | β163 | 00110001 | 49 | β164 | 01100010 | 98 | β165 | 11000100 | 196 | β166 |
11100001 | 225 | β167 | 10101011 | 171 | β168 | 00111111 | 63 | β169 | 01111110 | 126 | β170 |
11111100 | 252 | β171 | 10010001 | 145 | β172 | 01001011 | 75 | β173 | 10010110 | 150 | β174 |
… | … | … | … | … | … | … | … | … | … | … | … |
… | … | … | … | … | … | … | … | … | … | … | … |
… | … | … | … | … | … | … | … | … | … | … | … |
11010111 | 215 | β243 | 11000111 | 199 | β244 | 11100111 | 231 | β245 | 10100111 | 167 | β246 |
00100111 | 39 | β247 | 01001110 | 78 | β248 | 10011100 | 156 | β249 | 01010001 | 81 | β250 |
10100010 | 162 | β251 | 00101101 | 45 | β252 | 01011010 | 90 | β253 | 10110100 | 180 | β254 |
24 | 41 | 1 | 180 | 2 | 140 | 113 | 52 | 32 | 4 | 190 | 42 | 125 | 102 | 90 | 60 |
8 | 128 | 205 | 45 | 253 | 16 | 250 | 86 | 162 | 109 | 17 | 115 | 244 | 81 | 217 | 64 |
238 | 48 | 101 | 57 | 156 | 59 | 148 | 78 | 35 | 105 | 122 | 98 | 100 | 39 | 210 | 182 |
104 | 167 | 50 | 239 | 46 | 231 | 38 | 13 | 243 | 221 | 213 | 97 | 72 | 132 | 199 | 143 |
248 | 164 | 83 | 215 | 119 | 55 | 214 | 223 | 219 | 181 | 177 | 200 | 3 | 135 | 224 | 235 |
111 | 216 | 6 | 54 | 80 | 99 | 108 | 241 | 12 | 73 | 30 | 94 | 27 | 76 | 149 | 185 |
96 | 232 | 87 | 129 | 192 | 195 | 67 | 116 | 240 | 166 | 233 | 188 | 254 | 23 | 69 | 58 |
7 | 187 | 133 | 51 | 203 | 63 | 212 | 29 | 68 | 31 | 153 | 186 | 62 | 74 | 93 | 79 |
124 | 157 | 28 | 173 | 154 | 141 | 77 | 14 | 92 | 146 | 171 | 91 | 229 | 137 | 144 | 85 |
5 | 155 | 193 | 10 | 82 | 36 | 20 | 168 | 174 | 230 | 18 | 121 | 21 | 40 | 71 | 249 |
227 | 130 | 196 | 9 | 252 | 61 | 176 | 134 | 201 | 160 | 178 | 43 | 88 | 117 | 107 | 44 |
22 | 208 | 11 | 150 | 33 | 19 | 66 | 236 | 114 | 246 | 112 | 202 | 118 | 152 | 34 | 194 |
138 | 251 | 197 | 169 | 237 | 26 | 145 | 158 | 56 | 179 | 95 | 15 | 255 | 161 | 159 | 106 |
234 | 120 | 220 | 198 | 110 | 222 | 147 | 183 | 142 | 218 | 123 | 191 | 228 | 131 | 139 | 151 |
245 | 165 | 89 | 163 | 209 | 189 | 206 | 65 | 47 | 225 | 175 | 103 | 53 | 247 | 207 | 75 |
211 | 136 | 226 | 25 | 170 | 242 | 70 | 184 | 126 | 84 | 172 | 49 | 37 | 127 | 204 | 0 |
102 | 62 | 108 | 235 | 184 | 163 | 44 | 240 | 53 | 89 | 70 | 150 | 160 | 155 | 220 | 164 |
191 | 172 | 135 | 79 | 174 | 109 | 12 | 201 | 144 | 251 | 133 | 186 | 134 | 71 | 228 | 147 |
96 | 14 | 50 | 114 | 65 | 32 | 106 | 120 | 255 | 218 | 94 | 177 | 136 | 233 | 115 | 219 |
226 | 250 | 211 | 176 | 68 | 230 | 6 | 199 | 156 | 61 | 9 | 165 | 26 | 196 | 139 | 41 |
1 | 22 | 209 | 125 | 215 | 180 | 63 | 113 | 193 | 192 | 241 | 43 | 17 | 127 | 20 | 67 |
169 | 208 | 256 | 198 | 33 | 28 | 243 | 54 | 234 | 45 | 247 | 101 | 73 | 202 | 252 | 248 |
246 | 154 | 207 | 78 | 19 | 3 | 232 | 236 | 224 | 131 | 59 | 31 | 171 | 39 | 238 | 34 |
40 | 24 | 142 | 72 | 83 | 217 | 103 | 82 | 187 | 52 | 210 | 23 | 7 | 205 | 124 | 123 |
64 | 110 | 170 | 153 | 57 | 112 | 253 | 189 | 56 | 229 | 188 | 60 | 86 | 42 | 36 | 121 |
30 | 140 | 76 | 168 | 122 | 141 | 97 | 152 | 146 | 137 | 27 | 16 | 162 | 195 | 145 | 25 |
221 | 105 | 111 | 69 | 81 | 13 | 194 | 15 | 107 | 48 | 249 | 119 | 8 | 74 | 254 | 35 |
117 | 128 | 173 | 2 | 18 | 242 | 90 | 84 | 167 | 116 | 143 | 132 | 11 | 26 | 99 | 46 |
138 | 88 | 190 | 77 | 104 | 231 | 200 | 204 | 151 | 197 | 178 | 158 | 183 | 213 | 87 | 222 |
55 | 58 | 92 | 161 | 37 | 95 | 100 | 166 | 157 | 85 | 148 | 245 | 91 | 179 | 181 | 4 |
75 | 214 | 38 | 98 | 212 | 149 | 5 | 130 | 244 | 175 | 21 | 203 | 51 | 227 | 182 | 66 |
185 | 225 | 47 | 10 | 129 | 206 | 118 | 49 | 80 | 223 | 216 | 237 | 239 | 126 | 93 | 159 |
248 | 150 | 195 | 151 | 206 | 38 | 62 | 88 | 213 | 25 | 118 | 250 | 61 | 48 | 134 | 121 |
3 | 33 | 192 | 224 | 175 | 164 | 186 | 187 | 249 | 152 | 18 | 99 | 72 | 5 | 188 | 59 |
80 | 58 | 44 | 144 | 110 | 89 | 23 | 7 | 143 | 137 | 200 | 113 | 73 | 194 | 226 | 160 |
184 | 101 | 53 | 31 | 32 | 241 | 234 | 92 | 154 | 120 | 233 | 91 | 221 | 41 | 214 | 124 |
156 | 75 | 235 | 46 | 9 | 190 | 81 | 51 | 27 | 117 | 245 | 193 | 169 | 129 | 45 | 126 |
252 | 29 | 203 | 236 | 218 | 229 | 159 | 251 | 4 | 66 | 228 | 220 | 204 | 122 | 222 | 238 |
20 | 163 | 34 | 147 | 94 | 24 | 212 | 237 | 130 | 148 | 201 | 1 | 16 | 157 | 196 | 141 |
223 | 57 | 210 | 246 | 22 | 70 | 43 | 78 | 85 | 82 | 79 | 93 | 215 | 127 | 135 | 208 |
170 | 65 | 166 | 202 | 17 | 244 | 205 | 100 | 230 | 138 | 199 | 155 | 36 | 133 | 112 | 162 |
71 | 174 | 64 | 123 | 189 | 42 | 56 | 168 | 173 | 232 | 102 | 243 | 142 | 15 | 0 | 125 |
198 | 21 | 140 | 60 | 97 | 183 | 114 | 10 | 111 | 28 | 254 | 128 | 11 | 253 | 225 | 239 |
98 | 95 | 131 | 55 | 139 | 207 | 255 | 2 | 211 | 115 | 68 | 171 | 14 | 197 | 8 | 181 |
219 | 176 | 40 | 132 | 179 | 54 | 13 | 145 | 86 | 76 | 103 | 158 | 74 | 242 | 87 | 216 |
83 | 153 | 50 | 209 | 161 | 165 | 119 | 172 | 63 | 105 | 182 | 90 | 227 | 106 | 84 | 240 |
136 | 104 | 247 | 217 | 146 | 231 | 26 | 67 | 39 | 12 | 180 | 116 | 49 | 69 | 37 | 52 |
177 | 19 | 108 | 47 | 191 | 96 | 30 | 185 | 178 | 167 | 109 | 149 | 77 | 107 | 35 | 6 |
Boolean mapping | θ0 | θ1 | θ2 | θ3 | θ4 | θ5 | θ6 | θ7 | Mean |
NL score | 110 | 112 | 110 | 112 | 110 | 110 | 110 | 112 | 110 |
0.4844 | 0.5469 | 0.4688 | 0.5625 | 0.5312 | 0.5312 | 0.5156 | 0.4844 |
0.4531 | 0.4844 | 0.5469 | 0.5 | 0.5 | 0.4844 | 0.4844 | 0.5625 |
0.5312 | 0.4688 | 0.5312 | 0.5312 | 0.4375 | 0.4688 | 0.5156 | 0.5 |
0.4375 | 0.5 | 0.5469 | 0.5 | 0.5469 | 0.5312 | 0.4844 | 0.5156 |
0.4531 | 0.5625 | 0.5625 | 0.4688 | 0.4688 | 0.5156 | 0.4375 | 0.5312 |
0.5781 | 0.4844 | 0.5312 | 0.5469 | 0.5156 | 0.5 | 0.5156 | 0.5 |
0.5 | 0.4531 | 0.4531 | 0.4219 | 0.5156 | 0.5469 | 0.5312 | 0.4844 |
0.5 | 0.4844 | 0.5312 | 0.5312 | 0.5 | 0.5312 | 0.4375 | 0.5469 |
- | 110 | 110 | 112 | 112 | 110 | 112 | 110 |
110 | - | 108 | 110 | 112 | 112 | 108 | 110 |
110 | 108 | - | 110 | 112 | 110 | 110 | 112 |
112 | 110 | 110 | - | 110 | 110 | 110 | 112 |
112 | 112 | 112 | 110 | - | 110 | 110 | 110 |
110 | 112 | 110 | 110 | 110 | - | 112 | 110 |
112 | 108 | 110 | 110 | 110 | 112 | - | 112 |
110 | 110 | 112 | 112 | 110 | 110 | 112 | - |
- | 0.4805 | 0.5098 | 0.4941 | 0.4902 | 0.498 | 0.5215 | 0.4824 |
0.4805 | - | 0.5195 | 0.5176 | 0.4922 | 0.4922 | 0.4766 | 0.4902 |
0.5098 | 0.5195 | - | 0.5 | 0.4902 | 0.5137 | 0.4922 | 0.498 |
0.4941 | 0.5176 | 0.5 | - | 0.4961 | 0.5117 | 0.5273 | 0.4902 |
0.4902 | 0.4922 | 0.4902 | 0.4961 | - | 0.4922 | 0.5293 | 0.5195 |
0.498 | 0.4922 | 0.5137 | 0.5117 | 0.4922 | - | 0.4707 | 0.4863 |
0.5215 | 0.4766 | 0.4922 | 0.5273 | 0.5293 | 0.4707 | - | 0.4883 |
0.4824 | 0.4902 | 0.498 | 0.4902 | 0.5195 | 0.4863 | 0.4883 | - |
4 | 4 | 4 | 4 | 4 | 4 | 4 | 4 | 4 | 4 | 4 | 4 | 4 | 4 | 4 | 4 |
4 | 4 | 4 | 2 | 4 | 4 | 4 | 4 | 4 | 4 | 4 | 4 | 4 | 4 | 4 | 4 |
4 | 4 | 4 | 4 | 4 | 4 | 4 | 4 | 4 | 4 | 4 | 4 | 4 | 4 | 4 | 6 |
4 | 4 | 4 | 4 | 4 | 6 | 4 | 4 | 4 | 4 | 4 | 4 | 4 | 4 | 4 | 4 |
4 | 4 | 4 | 4 | 4 | 4 | 4 | 4 | 4 | 4 | 4 | 4 | 4 | 4 | 4 | 4 |
4 | 4 | 4 | 4 | 4 | 4 | 4 | 4 | 4 | 4 | 4 | 4 | 6 | 4 | 4 | 4 |
4 | 4 | 4 | 4 | 4 | 4 | 4 | 4 | 4 | 4 | 4 | 4 | 4 | 4 | 4 | 4 |
4 | 4 | 4 | 4 | 4 | 4 | 4 | 4 | 4 | 4 | 4 | 4 | 4 | 4 | 4 | 4 |
4 | 4 | 4 | 6 | 4 | 4 | 6 | 4 | 4 | 4 | 4 | 4 | 4 | 4 | 4 | 4 |
4 | 4 | 4 | 4 | 4 | 4 | 4 | 4 | 4 | 4 | 4 | 4 | 4 | 6 | 4 | 4 |
4 | 4 | 4 | 4 | 4 | 4 | 4 | 4 | 4 | 4 | 4 | 4 | 4 | 4 | 4 | 4 |
4 | 4 | 4 | 4 | 4 | 4 | 4 | 4 | 4 | 4 | 4 | 4 | 4 | 4 | 4 | 4 |
4 | 4 | 4 | 4 | 4 | 4 | 4 | 4 | 4 | 4 | 4 | 4 | 4 | 4 | 4 | 4 |
4 | 4 | 4 | 4 | 4 | 6 | 4 | 6 | 4 | 4 | 4 | 4 | 4 | 4 | 4 | 4 |
6 | 4 | 4 | 4 | 4 | 4 | 4 | 4 | 6 | 4 | 4 | 4 | 4 | 4 | 4 | 4 |
4 | 4 | 4 | 4 | 4 | 4 | 4 | 4 | 4 | 4 | 4 | 4 | 4 | 4 | 4 | - |
S-box | Nonlinearity Min Max Average |
SAC | BIC-SAC | BIC-NL | DU | LP | ||
Suggested S-box | 110 | 112 | 110.75 | 0.5051 | 0.4989 | 110.55 | 6 | 0.0781 |
AES [36] | 112 | 112 | 112 | 0.5058 | 0.5046 | 112 | 4 | 0.0625 |
Reference [37] | 106 | 108 | 106.25 | 0.5112 | 0.4975 | 103.93 | 12 | 0.1484 |
Reference [38] | 106 | 110 | 106.5 | 0.5010 | 0.4987 | 103.93 | 10 | 0.125 |
Reference [39] | 106 | 108 | 107 | 0.4949 | 0.5019 | 102.29 | 12 | 0.141 |
Reference [40] | 106 | 110 | 108.5 | 0.4995 | 0.5011 | 103.85 | 10 | 0.109 |
Reference [41] | 108 | 110 | 109.75 | 0.5042 | 0.4987 | 110.6 | 6 | 0.0859 |
Reference [42] | 102 | 110 | 106.5 | 0.4943 | 0.5019 | 103.35 | 12 | 0.1468 |
Reference [43] | 104 | 108 | 105.5 | 0.5065 | 0.5031 | 103.57 | 10 | 0.1328 |
Reference [44] | 104 | 110 | 107 | 0.4993 | 0.5050 | 103.29 | 10 | 0.1328 |
Image | Correlation | Entropy | Energy | Homogeneity | Contrast |
Cameraman Host | 0.9227 | 7.0097 | 0.1805 | 0.8952 | 0.5871 |
Cameraman-Enc | 0.0394 | 7.9972 | 0.0149 | 0.3999 | 10.0509 |
Pepper Host | 0.9312 | 7.5326 | 0.1096 | 0.8880 | 0.3849 |
Pepper-Enc | 0.0021 | 7.9972 | 0.0156 | 0.3902 | 10.4802 |
Baboon Host | 0.7983 | 7.2649 | 0.0943 | 0.7820 | 0.6326 |
Baboon-Enc | 0.0071 | 7.9975 | 0.0156 | 0.3945 | 10.3994 |
Lena Host | 0.9024 | 7.4439 | 0.1127 | 0.8622 | 0.4482 |
Lena-Enc | − 0.0379 | 7.9976 | 0.0157 | 0.3822 | 10.8896 |
Image | Cameraman | Pepper | Baboon | Lena | |
Vertical | Plain Image | 0.9745 | 0.9137 | 0.9090 | 0.9321 |
Distorted Image | 0.0310 | − 0.0392 | − 0.0128 | − 0.0117 | |
Horizontal | Plain Image | 0.9610 | 0.9204 | 0.8727 | 0.883 |
Distorted Image | − 0.0026 | − 0.0015 | 0.0039 | − 0.0021 |
Test | Cameraman-Enc | Pepper-Enc | Baboon-Enc | Lena-Enc |
MSE | 9212.16 | 8656.41 | 7854.44 | 8414.71 |
MSE [6] | 9079.09 | 8190.01 | 8011.23 | 8239.51 |
MSE [15] | 9189.41 | 8612.09 | 7599.03 | 7930.39 |
MSE [17] | 9187.38 | 8423.61 | 7865.21 | 8274.13 |
PSNR | 8.4723 | 8.8563 | 9.8912 | 8.9912 |
PSNR [6] | 8.1129 | 8.9710 | 8.5539 | 9.1902 |
PSNR [15] | 8.2897 | 8.7091 | 8.1331 | 8.9500 |
PSNR [17] | 8.2891 | 8.3353 | 8.9361 | 8.0032 |
SSIM1 | 0.0009 | 0.0012 | 0.0010 | 0.0011 |
SSIM1 [6] | 0.0013 | 0.0008 | 0.0011 | 0.0012 |
SSIM1 [15] | 0.0010 | 0.0012 | 0.0008 | 0.0014 |
SSIM1 [17] | 0.0009 | 0.0015 | 0.0012 | 0.0013 |
NCC | 0.8633 | 0.8710 | 0.9121 | 0.8803 |
NCC [6] | 0.8537 | 0.8675 | 0.8912 | 0.9016 |
NCC [15] | 0.8733 | 0.8712 | 0.8543 | 0.8461 |
NCC [17] | 0.8640 | 0.87134 | 0.9001 | 0.8692 |
AD | −7.4523 | −4.9812 | −2.3419 | −5.3881 |
AD [6] | −3,4511 | −5.6634 | −1.4529 | −2.3319 |
AD [15] | −6,7819 | −3.8873 | −2.8827 | −4.1198 |
AD [17] | −3.4429 | −4.9821 | −2.3872 | −7.6594 |
SC | 0.8496 | 0.8345 | 0.8456 | 0.8247 |
SC [6] | 0.8455 | 0.8451 | 0.8401 | 0.8342 |
SC [15] | 0.8341 | 0.8489 | 0.8465 | 0.8231 |
SC [17] | 0.8436 | 0.8111 | 0.8265 | 0.8490 |
MD | 240 | 238 | 212 | 234 |
MD [6] | 211 | 231 | 233 | 241 |
MD [15] | 223 | 227 | 227 | 228 |
MD [17] | 234 | 238 | 221 | 219 |
NAE | 0.6358 | 0.6273 | 0.6147 | 0.6384 |
NAE [6] | 0.6455 | 0.5932 | 0.5813 | 0.6459 |
NAE [15] | 0.6040 | 0.6193 | 0.6388 | 0.6026 |
NAE [17] | 0.6219 | 0.6243 | 0.6012 | 0.5856 |
RMSE | 94.6682 | 91.7245 | 84.9561 | 87.9349 |
RMSE [6] | 90.6638 | 92.3402 | 88.3476 | 86.7938 |
RMSE [15] | 93,4428 | 89.7690 | 91.2398 | 87.3947 |
RMSE [17] | 90.4582 | 85.1109 | 84.8934 | 88.1831 |
UQI | 0.0218 | 0.0332 | 0.0314 | 0.0338 |
UQI [6] | 0.0127 | 0.0412 | 0.0279 | 0.0178 |
UQI [15] | 0.0347 | 0.0456 | 0.0127 | 0.0391 |
UQI [17] | 0.0234 | 0.0401 | 0.0298 | 0.0281 |
MI | −1.0292 | −1.0187 | −1.0184 | −1.0281 |
MI [6] | −1.0195 | −1.0490 | −1.0328 | −1.0402 |
MI [15] | −1.0371 | −1.0197 | −1.0294 | −1.0406 |
MI [17] | −1.341 | −1.0198 | −1.0384 | −1.0327 |
Image | NPCR | UACI | BACI |
Cameraman | 99.63% | 33.12% | 24.60% |
Pepper | 99.81% | 33.21% | 26.38% |
Baboon | 99.76% | 32.86% | 24.25% |
Lena | 99.79% | 33.16% | 23.09% |
Binary form | Decimal | 𝐺𝐹 (210) | Binary form | Decimal | 𝐺𝐹(210) | Binary form | Decimal | 𝐺𝐹(210) | Binary form | Decimal | 𝐺𝐹(210) |
0000000000 | 0 | 0 | 0000000001 | 1 | 1 | 0000000010 | 2 | κ1 | 0000000100 | 4 | κ2 |
0000001000 | 8 | κ3 | 0000010000 | 16 | κ4 | 0000100000 | 32 | κ5 | 0001000000 | 64 | κ6 |
0010000000 | 128 | κ7 | 0100000000 | 256 | κ8 | 1000000000 | 512 | κ9 | 0010000001 | 129 | κ10 |
0100000010 | 258 | κ11 | 1000000100 | 516 | κ12 | 0010001001 | 137 | κ13 | 0100010010 | 274 | κ14 |
1000100100 | 548 | κ15 | 0011001001 | 201 | κ16 | 0110010010 | 402 | κ17 | 1100100100 | 804 | κ18 |
1011001001 | 713 | κ19 | 0100010011 | 275 | κ20 | 1000100110 | 550 | κ21 | 0011001101 | 205 | κ22 |
… | … | … | … | … | … | … | … | … | … | … | … |
… | … | … | … | … | … | … | … | … | … | … | … |
… | … | … | … | … | … | … | … | … | … | … | … |
1010111011 | 699 | κ311 | 0111110111 | 503 | κ312 | 1111101110 | 1006 | κ313 | 1101011101 | 861 | κ314 |
1000111011 | 571 | κ315 | 0011110111 | 247 | κ316 | 0111101110 | 494 | κ317 | 1111011100 | 988 | κ318 |
1100111001 | 825 | κ319 | 1011110011 | 755 | κ320 | 0101100111 | 359 | κ321 | 1011001110 | 718 | κ322 |
0100011101 | 285 | κ323 | 1000111010 | 570 | κ324 | 0011110101 | 245 | κ325 | 0111101010 | 490 | κ326 |
… | … | … | … | … | … | … | … | … | … | … | … |
… | … | … | … | … | … | … | … | … | … | … | … |
… | … | … | … | … | … | … | … | … | … | … | … |
0100110000 | 304 | κ1007 | 1001100000 | 608 | κ1008 | 0001000001 | 65 | κ1009 | 0010000010 | 130 | κ1010 |
0100000100 | 260 | κ1011 | 1000001000 | 520 | κ1012 | 0010010001 | 145 | κ1013 | 0100100010 | 290 | κ1014 |
1001000100 | 580 | κ1015 | 0000001001 | 9 | κ1016 | 0000010010 | 18 | κ1017 | 0000100100 | 36 | κ1018 |
0001001000 | 72 | κ1019 | 0010010000 | 144 | κ1020 | 0100100000 | 288 | κ1021 | 1001000000 | 576 | κ1022 |
κ1 | κ77 | κ1021 | κ869 | κ1013 | κ5 | κ513 | κ1017 | κ1009 | κ9 | κ189 | κ13 | κ301 | κ709 | κ353 | κ193 |
κ209 | κ17 | κ729 | κ1005 | κ393 | κ21 | κ305 | κ1001 | κ645 | κ937 | κ25 | κ385 | κ997 | κ421 | κ817 | κ29 |
κ537 | κ457 | κ993 | κ317 | κ265 | κ637 | κ605 | κ33 | κ989 | κ401 | κ909 | κ149 | κ237 | κ273 | κ37 | κ161 |
κ985 | κ473 | κ545 | κ41 | κ849 | κ981 | κ413 | κ45 | κ977 | κ897 | κ673 | κ397 | κ49 | κ225 | κ749 | κ973 |
κ253 | κ109 | κ965 | κ181 | κ233 | κ53 | κ481 | κ969 | κ665 | κ213 | κ865 | κ57 | κ437 | κ529 | κ345 | κ737 |
κ449 | κ389 | κ61 | κ917 | κ961 | κ493 | κ65 | κ621 | κ957 | κ297 | κ945 | κ145 | κ153 | κ221 | κ69 | κ953 |
κ725 | κ261 | κ549 | κ477 | κ73 | κ949 | κ701 | κ405 | κ81 | κ177 | κ765 | κ941 | κ593 | κ785 | κ321 | κ113 |
κ197 | κ85 | κ349 | κ589 | κ489 | κ577 | κ89 | κ893 | κ933 | κ761 | κ93 | κ657 | κ929 | κ229 | κ721 | κ97 |
κ925 | κ573 | κ165 | κ417 | κ517 | κ101 | κ789 | κ133 | κ921 | κ805 | κ601 | κ525 | κ661 | κ557 | κ105 | κ837 |
κ269 | κ913 | κ597 | κ169 | κ705 | κ117 | κ461 | κ445 | κ905 | κ333 | κ553 | κ125 | κ245 | κ121 | κ357 | κ901 |
κ689 | κ257 | κ521 | κ649 | κ129 | κ561 | κ429 | κ889 | κ733 | κ329 | κ829 | κ717 | κ581 | κ137 | κ885 | κ873 |
κ141 | κ881 | κ501 | κ541 | κ877 | κ777 | κ853 | κ325 | κ157 | κ361 | κ505 | κ569 | κ613 | κ861 | κ669 | κ857 |
κ381 | κ797 | κ629 | κ173 | κ313 | κ845 | κ585 | κ841 | κ425 | κ465 | κ741 | κ185 | κ377 | κ565 | κ833 | κ609 |
κ825 | κ693 | κ201 | κ745 | κ821 | κ757 | κ205 | κ813 | κ441 | κ249 | κ809 | κ485 | κ409 | κ625 | κ217 | κ337 |
κ469 | κ801 | κ685 | κ793 | κ617 | κ773 | κ533 | κ241 | κ681 | κ781 | κ309 | κ497 | κ293 | κ769 | κ509 | κ433 |
κ633 | κ653 | κ753 | κ365 | κ277 | κ281 | κ453 | κ289 | κ285 | κ677 | κ641 | κ713 | κ373 | κ697 | κ369 | κ381 |
Binary form | Decimal | 𝐺𝐹 (28) | Binary form | Decimal | 𝐺𝐹 (28) | Binary form | Decimal | 𝐺𝐹 (28) | Binary form | Decimal | 𝐺𝐹 (28) |
00000000 | 0 | 0 | 00000001 | 1 | 1 | 00000010 | 2 | β1 | 00000100 | 4 | β2 |
00001000 | 8 | β3 | 00010000 | 16 | β4 | 00100000 | 32 | β5 | 01000000 | 64 | β6 |
10000000 | 128 | β7 | 01101001 | 105 | β8 | 11010010 | 210 | β9 | 11001101 | 205 | β10 |
11110011 | 243 | β11 | 10001111 | 143 | β12 | 01110111 | 119 | β13 | 11101110 | 238 | β14 |
… | … | … | … | … | … | … | … | … | … | … | … |
… | … | … | … | … | … | … | … | … | … | … | … |
… | … | … | … | … | … | … | … | … | … | … | … |
10101100 | 172 | β163 | 00110001 | 49 | β164 | 01100010 | 98 | β165 | 11000100 | 196 | β166 |
11100001 | 225 | β167 | 10101011 | 171 | β168 | 00111111 | 63 | β169 | 01111110 | 126 | β170 |
11111100 | 252 | β171 | 10010001 | 145 | β172 | 01001011 | 75 | β173 | 10010110 | 150 | β174 |
… | … | … | … | … | … | … | … | … | … | … | … |
… | … | … | … | … | … | … | … | … | … | … | … |
… | … | … | … | … | … | … | … | … | … | … | … |
11010111 | 215 | β243 | 11000111 | 199 | β244 | 11100111 | 231 | β245 | 10100111 | 167 | β246 |
00100111 | 39 | β247 | 01001110 | 78 | β248 | 10011100 | 156 | β249 | 01010001 | 81 | β250 |
10100010 | 162 | β251 | 00101101 | 45 | β252 | 01011010 | 90 | β253 | 10110100 | 180 | β254 |
24 | 41 | 1 | 180 | 2 | 140 | 113 | 52 | 32 | 4 | 190 | 42 | 125 | 102 | 90 | 60 |
8 | 128 | 205 | 45 | 253 | 16 | 250 | 86 | 162 | 109 | 17 | 115 | 244 | 81 | 217 | 64 |
238 | 48 | 101 | 57 | 156 | 59 | 148 | 78 | 35 | 105 | 122 | 98 | 100 | 39 | 210 | 182 |
104 | 167 | 50 | 239 | 46 | 231 | 38 | 13 | 243 | 221 | 213 | 97 | 72 | 132 | 199 | 143 |
248 | 164 | 83 | 215 | 119 | 55 | 214 | 223 | 219 | 181 | 177 | 200 | 3 | 135 | 224 | 235 |
111 | 216 | 6 | 54 | 80 | 99 | 108 | 241 | 12 | 73 | 30 | 94 | 27 | 76 | 149 | 185 |
96 | 232 | 87 | 129 | 192 | 195 | 67 | 116 | 240 | 166 | 233 | 188 | 254 | 23 | 69 | 58 |
7 | 187 | 133 | 51 | 203 | 63 | 212 | 29 | 68 | 31 | 153 | 186 | 62 | 74 | 93 | 79 |
124 | 157 | 28 | 173 | 154 | 141 | 77 | 14 | 92 | 146 | 171 | 91 | 229 | 137 | 144 | 85 |
5 | 155 | 193 | 10 | 82 | 36 | 20 | 168 | 174 | 230 | 18 | 121 | 21 | 40 | 71 | 249 |
227 | 130 | 196 | 9 | 252 | 61 | 176 | 134 | 201 | 160 | 178 | 43 | 88 | 117 | 107 | 44 |
22 | 208 | 11 | 150 | 33 | 19 | 66 | 236 | 114 | 246 | 112 | 202 | 118 | 152 | 34 | 194 |
138 | 251 | 197 | 169 | 237 | 26 | 145 | 158 | 56 | 179 | 95 | 15 | 255 | 161 | 159 | 106 |
234 | 120 | 220 | 198 | 110 | 222 | 147 | 183 | 142 | 218 | 123 | 191 | 228 | 131 | 139 | 151 |
245 | 165 | 89 | 163 | 209 | 189 | 206 | 65 | 47 | 225 | 175 | 103 | 53 | 247 | 207 | 75 |
211 | 136 | 226 | 25 | 170 | 242 | 70 | 184 | 126 | 84 | 172 | 49 | 37 | 127 | 204 | 0 |
102 | 62 | 108 | 235 | 184 | 163 | 44 | 240 | 53 | 89 | 70 | 150 | 160 | 155 | 220 | 164 |
191 | 172 | 135 | 79 | 174 | 109 | 12 | 201 | 144 | 251 | 133 | 186 | 134 | 71 | 228 | 147 |
96 | 14 | 50 | 114 | 65 | 32 | 106 | 120 | 255 | 218 | 94 | 177 | 136 | 233 | 115 | 219 |
226 | 250 | 211 | 176 | 68 | 230 | 6 | 199 | 156 | 61 | 9 | 165 | 26 | 196 | 139 | 41 |
1 | 22 | 209 | 125 | 215 | 180 | 63 | 113 | 193 | 192 | 241 | 43 | 17 | 127 | 20 | 67 |
169 | 208 | 256 | 198 | 33 | 28 | 243 | 54 | 234 | 45 | 247 | 101 | 73 | 202 | 252 | 248 |
246 | 154 | 207 | 78 | 19 | 3 | 232 | 236 | 224 | 131 | 59 | 31 | 171 | 39 | 238 | 34 |
40 | 24 | 142 | 72 | 83 | 217 | 103 | 82 | 187 | 52 | 210 | 23 | 7 | 205 | 124 | 123 |
64 | 110 | 170 | 153 | 57 | 112 | 253 | 189 | 56 | 229 | 188 | 60 | 86 | 42 | 36 | 121 |
30 | 140 | 76 | 168 | 122 | 141 | 97 | 152 | 146 | 137 | 27 | 16 | 162 | 195 | 145 | 25 |
221 | 105 | 111 | 69 | 81 | 13 | 194 | 15 | 107 | 48 | 249 | 119 | 8 | 74 | 254 | 35 |
117 | 128 | 173 | 2 | 18 | 242 | 90 | 84 | 167 | 116 | 143 | 132 | 11 | 26 | 99 | 46 |
138 | 88 | 190 | 77 | 104 | 231 | 200 | 204 | 151 | 197 | 178 | 158 | 183 | 213 | 87 | 222 |
55 | 58 | 92 | 161 | 37 | 95 | 100 | 166 | 157 | 85 | 148 | 245 | 91 | 179 | 181 | 4 |
75 | 214 | 38 | 98 | 212 | 149 | 5 | 130 | 244 | 175 | 21 | 203 | 51 | 227 | 182 | 66 |
185 | 225 | 47 | 10 | 129 | 206 | 118 | 49 | 80 | 223 | 216 | 237 | 239 | 126 | 93 | 159 |
248 | 150 | 195 | 151 | 206 | 38 | 62 | 88 | 213 | 25 | 118 | 250 | 61 | 48 | 134 | 121 |
3 | 33 | 192 | 224 | 175 | 164 | 186 | 187 | 249 | 152 | 18 | 99 | 72 | 5 | 188 | 59 |
80 | 58 | 44 | 144 | 110 | 89 | 23 | 7 | 143 | 137 | 200 | 113 | 73 | 194 | 226 | 160 |
184 | 101 | 53 | 31 | 32 | 241 | 234 | 92 | 154 | 120 | 233 | 91 | 221 | 41 | 214 | 124 |
156 | 75 | 235 | 46 | 9 | 190 | 81 | 51 | 27 | 117 | 245 | 193 | 169 | 129 | 45 | 126 |
252 | 29 | 203 | 236 | 218 | 229 | 159 | 251 | 4 | 66 | 228 | 220 | 204 | 122 | 222 | 238 |
20 | 163 | 34 | 147 | 94 | 24 | 212 | 237 | 130 | 148 | 201 | 1 | 16 | 157 | 196 | 141 |
223 | 57 | 210 | 246 | 22 | 70 | 43 | 78 | 85 | 82 | 79 | 93 | 215 | 127 | 135 | 208 |
170 | 65 | 166 | 202 | 17 | 244 | 205 | 100 | 230 | 138 | 199 | 155 | 36 | 133 | 112 | 162 |
71 | 174 | 64 | 123 | 189 | 42 | 56 | 168 | 173 | 232 | 102 | 243 | 142 | 15 | 0 | 125 |
198 | 21 | 140 | 60 | 97 | 183 | 114 | 10 | 111 | 28 | 254 | 128 | 11 | 253 | 225 | 239 |
98 | 95 | 131 | 55 | 139 | 207 | 255 | 2 | 211 | 115 | 68 | 171 | 14 | 197 | 8 | 181 |
219 | 176 | 40 | 132 | 179 | 54 | 13 | 145 | 86 | 76 | 103 | 158 | 74 | 242 | 87 | 216 |
83 | 153 | 50 | 209 | 161 | 165 | 119 | 172 | 63 | 105 | 182 | 90 | 227 | 106 | 84 | 240 |
136 | 104 | 247 | 217 | 146 | 231 | 26 | 67 | 39 | 12 | 180 | 116 | 49 | 69 | 37 | 52 |
177 | 19 | 108 | 47 | 191 | 96 | 30 | 185 | 178 | 167 | 109 | 149 | 77 | 107 | 35 | 6 |
Boolean mapping | θ0 | θ1 | θ2 | θ3 | θ4 | θ5 | θ6 | θ7 | Mean |
NL score | 110 | 112 | 110 | 112 | 110 | 110 | 110 | 112 | 110 |
0.4844 | 0.5469 | 0.4688 | 0.5625 | 0.5312 | 0.5312 | 0.5156 | 0.4844 |
0.4531 | 0.4844 | 0.5469 | 0.5 | 0.5 | 0.4844 | 0.4844 | 0.5625 |
0.5312 | 0.4688 | 0.5312 | 0.5312 | 0.4375 | 0.4688 | 0.5156 | 0.5 |
0.4375 | 0.5 | 0.5469 | 0.5 | 0.5469 | 0.5312 | 0.4844 | 0.5156 |
0.4531 | 0.5625 | 0.5625 | 0.4688 | 0.4688 | 0.5156 | 0.4375 | 0.5312 |
0.5781 | 0.4844 | 0.5312 | 0.5469 | 0.5156 | 0.5 | 0.5156 | 0.5 |
0.5 | 0.4531 | 0.4531 | 0.4219 | 0.5156 | 0.5469 | 0.5312 | 0.4844 |
0.5 | 0.4844 | 0.5312 | 0.5312 | 0.5 | 0.5312 | 0.4375 | 0.5469 |
- | 110 | 110 | 112 | 112 | 110 | 112 | 110 |
110 | - | 108 | 110 | 112 | 112 | 108 | 110 |
110 | 108 | - | 110 | 112 | 110 | 110 | 112 |
112 | 110 | 110 | - | 110 | 110 | 110 | 112 |
112 | 112 | 112 | 110 | - | 110 | 110 | 110 |
110 | 112 | 110 | 110 | 110 | - | 112 | 110 |
112 | 108 | 110 | 110 | 110 | 112 | - | 112 |
110 | 110 | 112 | 112 | 110 | 110 | 112 | - |
- | 0.4805 | 0.5098 | 0.4941 | 0.4902 | 0.498 | 0.5215 | 0.4824 |
0.4805 | - | 0.5195 | 0.5176 | 0.4922 | 0.4922 | 0.4766 | 0.4902 |
0.5098 | 0.5195 | - | 0.5 | 0.4902 | 0.5137 | 0.4922 | 0.498 |
0.4941 | 0.5176 | 0.5 | - | 0.4961 | 0.5117 | 0.5273 | 0.4902 |
0.4902 | 0.4922 | 0.4902 | 0.4961 | - | 0.4922 | 0.5293 | 0.5195 |
0.498 | 0.4922 | 0.5137 | 0.5117 | 0.4922 | - | 0.4707 | 0.4863 |
0.5215 | 0.4766 | 0.4922 | 0.5273 | 0.5293 | 0.4707 | - | 0.4883 |
0.4824 | 0.4902 | 0.498 | 0.4902 | 0.5195 | 0.4863 | 0.4883 | - |
4 | 4 | 4 | 4 | 4 | 4 | 4 | 4 | 4 | 4 | 4 | 4 | 4 | 4 | 4 | 4 |
4 | 4 | 4 | 2 | 4 | 4 | 4 | 4 | 4 | 4 | 4 | 4 | 4 | 4 | 4 | 4 |
4 | 4 | 4 | 4 | 4 | 4 | 4 | 4 | 4 | 4 | 4 | 4 | 4 | 4 | 4 | 6 |
4 | 4 | 4 | 4 | 4 | 6 | 4 | 4 | 4 | 4 | 4 | 4 | 4 | 4 | 4 | 4 |
4 | 4 | 4 | 4 | 4 | 4 | 4 | 4 | 4 | 4 | 4 | 4 | 4 | 4 | 4 | 4 |
4 | 4 | 4 | 4 | 4 | 4 | 4 | 4 | 4 | 4 | 4 | 4 | 6 | 4 | 4 | 4 |
4 | 4 | 4 | 4 | 4 | 4 | 4 | 4 | 4 | 4 | 4 | 4 | 4 | 4 | 4 | 4 |
4 | 4 | 4 | 4 | 4 | 4 | 4 | 4 | 4 | 4 | 4 | 4 | 4 | 4 | 4 | 4 |
4 | 4 | 4 | 6 | 4 | 4 | 6 | 4 | 4 | 4 | 4 | 4 | 4 | 4 | 4 | 4 |
4 | 4 | 4 | 4 | 4 | 4 | 4 | 4 | 4 | 4 | 4 | 4 | 4 | 6 | 4 | 4 |
4 | 4 | 4 | 4 | 4 | 4 | 4 | 4 | 4 | 4 | 4 | 4 | 4 | 4 | 4 | 4 |
4 | 4 | 4 | 4 | 4 | 4 | 4 | 4 | 4 | 4 | 4 | 4 | 4 | 4 | 4 | 4 |
4 | 4 | 4 | 4 | 4 | 4 | 4 | 4 | 4 | 4 | 4 | 4 | 4 | 4 | 4 | 4 |
4 | 4 | 4 | 4 | 4 | 6 | 4 | 6 | 4 | 4 | 4 | 4 | 4 | 4 | 4 | 4 |
6 | 4 | 4 | 4 | 4 | 4 | 4 | 4 | 6 | 4 | 4 | 4 | 4 | 4 | 4 | 4 |
4 | 4 | 4 | 4 | 4 | 4 | 4 | 4 | 4 | 4 | 4 | 4 | 4 | 4 | 4 | - |
S-box | Nonlinearity Min Max Average |
SAC | BIC-SAC | BIC-NL | DU | LP | ||
Suggested S-box | 110 | 112 | 110.75 | 0.5051 | 0.4989 | 110.55 | 6 | 0.0781 |
AES [36] | 112 | 112 | 112 | 0.5058 | 0.5046 | 112 | 4 | 0.0625 |
Reference [37] | 106 | 108 | 106.25 | 0.5112 | 0.4975 | 103.93 | 12 | 0.1484 |
Reference [38] | 106 | 110 | 106.5 | 0.5010 | 0.4987 | 103.93 | 10 | 0.125 |
Reference [39] | 106 | 108 | 107 | 0.4949 | 0.5019 | 102.29 | 12 | 0.141 |
Reference [40] | 106 | 110 | 108.5 | 0.4995 | 0.5011 | 103.85 | 10 | 0.109 |
Reference [41] | 108 | 110 | 109.75 | 0.5042 | 0.4987 | 110.6 | 6 | 0.0859 |
Reference [42] | 102 | 110 | 106.5 | 0.4943 | 0.5019 | 103.35 | 12 | 0.1468 |
Reference [43] | 104 | 108 | 105.5 | 0.5065 | 0.5031 | 103.57 | 10 | 0.1328 |
Reference [44] | 104 | 110 | 107 | 0.4993 | 0.5050 | 103.29 | 10 | 0.1328 |
Image | Correlation | Entropy | Energy | Homogeneity | Contrast |
Cameraman Host | 0.9227 | 7.0097 | 0.1805 | 0.8952 | 0.5871 |
Cameraman-Enc | 0.0394 | 7.9972 | 0.0149 | 0.3999 | 10.0509 |
Pepper Host | 0.9312 | 7.5326 | 0.1096 | 0.8880 | 0.3849 |
Pepper-Enc | 0.0021 | 7.9972 | 0.0156 | 0.3902 | 10.4802 |
Baboon Host | 0.7983 | 7.2649 | 0.0943 | 0.7820 | 0.6326 |
Baboon-Enc | 0.0071 | 7.9975 | 0.0156 | 0.3945 | 10.3994 |
Lena Host | 0.9024 | 7.4439 | 0.1127 | 0.8622 | 0.4482 |
Lena-Enc | − 0.0379 | 7.9976 | 0.0157 | 0.3822 | 10.8896 |
Image | Cameraman | Pepper | Baboon | Lena | |
Vertical | Plain Image | 0.9745 | 0.9137 | 0.9090 | 0.9321 |
Distorted Image | 0.0310 | − 0.0392 | − 0.0128 | − 0.0117 | |
Horizontal | Plain Image | 0.9610 | 0.9204 | 0.8727 | 0.883 |
Distorted Image | − 0.0026 | − 0.0015 | 0.0039 | − 0.0021 |
Test | Cameraman-Enc | Pepper-Enc | Baboon-Enc | Lena-Enc |
MSE | 9212.16 | 8656.41 | 7854.44 | 8414.71 |
MSE [6] | 9079.09 | 8190.01 | 8011.23 | 8239.51 |
MSE [15] | 9189.41 | 8612.09 | 7599.03 | 7930.39 |
MSE [17] | 9187.38 | 8423.61 | 7865.21 | 8274.13 |
PSNR | 8.4723 | 8.8563 | 9.8912 | 8.9912 |
PSNR [6] | 8.1129 | 8.9710 | 8.5539 | 9.1902 |
PSNR [15] | 8.2897 | 8.7091 | 8.1331 | 8.9500 |
PSNR [17] | 8.2891 | 8.3353 | 8.9361 | 8.0032 |
SSIM1 | 0.0009 | 0.0012 | 0.0010 | 0.0011 |
SSIM1 [6] | 0.0013 | 0.0008 | 0.0011 | 0.0012 |
SSIM1 [15] | 0.0010 | 0.0012 | 0.0008 | 0.0014 |
SSIM1 [17] | 0.0009 | 0.0015 | 0.0012 | 0.0013 |
NCC | 0.8633 | 0.8710 | 0.9121 | 0.8803 |
NCC [6] | 0.8537 | 0.8675 | 0.8912 | 0.9016 |
NCC [15] | 0.8733 | 0.8712 | 0.8543 | 0.8461 |
NCC [17] | 0.8640 | 0.87134 | 0.9001 | 0.8692 |
AD | −7.4523 | −4.9812 | −2.3419 | −5.3881 |
AD [6] | −3,4511 | −5.6634 | −1.4529 | −2.3319 |
AD [15] | −6,7819 | −3.8873 | −2.8827 | −4.1198 |
AD [17] | −3.4429 | −4.9821 | −2.3872 | −7.6594 |
SC | 0.8496 | 0.8345 | 0.8456 | 0.8247 |
SC [6] | 0.8455 | 0.8451 | 0.8401 | 0.8342 |
SC [15] | 0.8341 | 0.8489 | 0.8465 | 0.8231 |
SC [17] | 0.8436 | 0.8111 | 0.8265 | 0.8490 |
MD | 240 | 238 | 212 | 234 |
MD [6] | 211 | 231 | 233 | 241 |
MD [15] | 223 | 227 | 227 | 228 |
MD [17] | 234 | 238 | 221 | 219 |
NAE | 0.6358 | 0.6273 | 0.6147 | 0.6384 |
NAE [6] | 0.6455 | 0.5932 | 0.5813 | 0.6459 |
NAE [15] | 0.6040 | 0.6193 | 0.6388 | 0.6026 |
NAE [17] | 0.6219 | 0.6243 | 0.6012 | 0.5856 |
RMSE | 94.6682 | 91.7245 | 84.9561 | 87.9349 |
RMSE [6] | 90.6638 | 92.3402 | 88.3476 | 86.7938 |
RMSE [15] | 93,4428 | 89.7690 | 91.2398 | 87.3947 |
RMSE [17] | 90.4582 | 85.1109 | 84.8934 | 88.1831 |
UQI | 0.0218 | 0.0332 | 0.0314 | 0.0338 |
UQI [6] | 0.0127 | 0.0412 | 0.0279 | 0.0178 |
UQI [15] | 0.0347 | 0.0456 | 0.0127 | 0.0391 |
UQI [17] | 0.0234 | 0.0401 | 0.0298 | 0.0281 |
MI | −1.0292 | −1.0187 | −1.0184 | −1.0281 |
MI [6] | −1.0195 | −1.0490 | −1.0328 | −1.0402 |
MI [15] | −1.0371 | −1.0197 | −1.0294 | −1.0406 |
MI [17] | −1.341 | −1.0198 | −1.0384 | −1.0327 |
Image | NPCR | UACI | BACI |
Cameraman | 99.63% | 33.12% | 24.60% |
Pepper | 99.81% | 33.21% | 26.38% |
Baboon | 99.76% | 32.86% | 24.25% |
Lena | 99.79% | 33.16% | 23.09% |