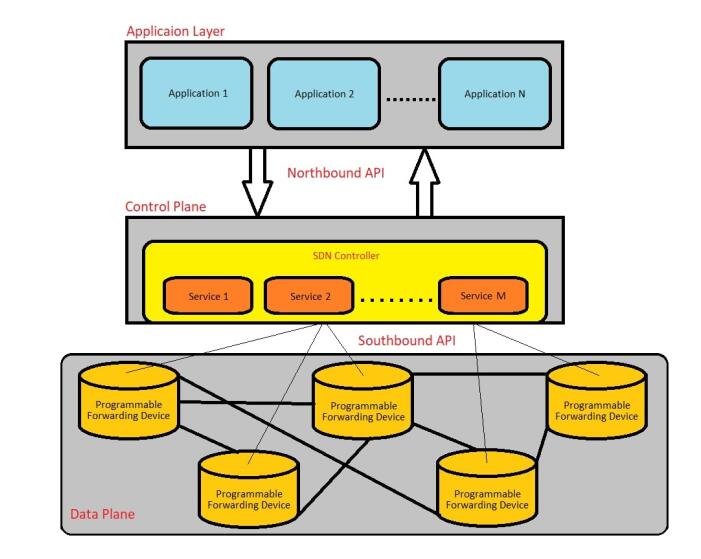
Industrial Cyber-Physical Systems (CPSs) require flexible and tolerant communication networks to overcome commonly occurring security problems and denial-of-service such as links failure and networks congestion that might be due to direct or indirect network attacks. In this work, we take advantage of Software-defined networking (SDN) as an important networking paradigm that provide real-time fault resilience since it is capable of global network visibility and programmability. We consider OpenFlow as an SDN protocol that enables interaction between the SDN controller and forwarding plane of network devices. We employ multiple machine learning algorithms to enhance the decision making in the SDN controller. Integrating machine learning with network resilience solutions can effectively address the challenge of predicting and classifying network traffic and thus, providing real-time network resilience and higher security level. The aim is to address network resilience by proposing an intelligent recommender system that recommends paths in real-time based on predicting link failures and network congestions. We use statistical data of the network such as link propagation delay, the number of packets/bytes received and transmitted by each OpenFlow switch on a specific port. Different state-of-art machine learning models has been implemented such as logistic regression, K-nearest neighbors, support vector machine, and decision tree to train these models in normal state, links failure and congestion conditions. The models are evaluated on the Mininet emulation testbed and provide accuracies ranging from around 91–99% on the test data. The machine learning model with the highest accuracy is utilized in the intelligent recommender system of the SDN controller which helps in selecting resilient paths to achieve a better security and quality-of-service in the network. This real-time recommender system helps the controller to take reactive measures to improve network resilience and security by avoiding faulty paths during path discovery and establishment.
Citation: Abhishek Savaliya, Rutvij H. Jhaveri, Qin Xin, Saad Alqithami, Sagar Ramani, Tariq Ahamed Ahanger. Securing industrial communication with software-defined networking[J]. Mathematical Biosciences and Engineering, 2021, 18(6): 8298-8313. doi: 10.3934/mbe.2021411
[1] | Zhibin Zuo, Rongyu He, Xianwei Zhu, Chaowen Chang . A novel software-defined network packet security tunnel forwarding mechanism. Mathematical Biosciences and Engineering, 2019, 16(5): 4359-4381. doi: 10.3934/mbe.2019217 |
[2] | Muhammad Hassan Jamal, Muazzam A Khan, Safi Ullah, Mohammed S. Alshehri, Sultan Almakdi, Umer Rashid, Abdulwahab Alazeb, Jawad Ahmad . Multi-step attack detection in industrial networks using a hybrid deep learning architecture. Mathematical Biosciences and Engineering, 2023, 20(8): 13824-13848. doi: 10.3934/mbe.2023615 |
[3] | Jiushuang Wang, Ying Liu, Huifen Feng . IFACNN: efficient DDoS attack detection based on improved firefly algorithm to optimize convolutional neural networks. Mathematical Biosciences and Engineering, 2022, 19(2): 1280-1303. doi: 10.3934/mbe.2022059 |
[4] | Shivani Gaba, Ishan Budhiraja, Vimal Kumar, Aaisha Makkar . Advancements in enhancing cyber-physical system security: Practical deep learning solutions for network traffic classification and integration with security technologies. Mathematical Biosciences and Engineering, 2024, 21(1): 1527-1553. doi: 10.3934/mbe.2024066 |
[5] | Aida Ben Chehida Douss, Ryma Abassi, Damien Sauveron . State-of-the-art survey of in-vehicle protocols and automotive Ethernet security and vulnerabilities. Mathematical Biosciences and Engineering, 2023, 20(9): 17057-17095. doi: 10.3934/mbe.2023761 |
[6] | Raghavendra Rao Althar, Abdulrahman Alahmadi, Debabrata Samanta, Mohammad Zubair Khan, Ahmed H. Alahmadi . Mathematical foundations based statistical modeling of software source code for software system evolution. Mathematical Biosciences and Engineering, 2022, 19(4): 3701-3719. doi: 10.3934/mbe.2022170 |
[7] | Jingxu Xiao, Chaowen Chang, Yingying Ma, Chenli Yang, Lu Yuan . Secure multi-path routing for Internet of Things based on trust evaluation. Mathematical Biosciences and Engineering, 2024, 21(2): 3335-3363. doi: 10.3934/mbe.2024148 |
[8] | Yifeng Yin, Zhaobo Wang, Wanyi Zhou, Yong Gan, Yanhua Zhang . Group key agreement protocol for edge computing in industrial internet. Mathematical Biosciences and Engineering, 2022, 19(12): 12730-12743. doi: 10.3934/mbe.2022594 |
[9] | Naila Naz, Muazzam A Khan, Suliman A. Alsuhibany, Muhammad Diyan, Zhiyuan Tan, Muhammad Almas Khan, Jawad Ahmad . Ensemble learning-based IDS for sensors telemetry data in IoT networks. Mathematical Biosciences and Engineering, 2022, 19(10): 10550-10580. doi: 10.3934/mbe.2022493 |
[10] | Long Wen, Yan Dong, Liang Gao . A new ensemble residual convolutional neural network for remaining useful life estimation. Mathematical Biosciences and Engineering, 2019, 16(2): 862-880. doi: 10.3934/mbe.2019040 |
Industrial Cyber-Physical Systems (CPSs) require flexible and tolerant communication networks to overcome commonly occurring security problems and denial-of-service such as links failure and networks congestion that might be due to direct or indirect network attacks. In this work, we take advantage of Software-defined networking (SDN) as an important networking paradigm that provide real-time fault resilience since it is capable of global network visibility and programmability. We consider OpenFlow as an SDN protocol that enables interaction between the SDN controller and forwarding plane of network devices. We employ multiple machine learning algorithms to enhance the decision making in the SDN controller. Integrating machine learning with network resilience solutions can effectively address the challenge of predicting and classifying network traffic and thus, providing real-time network resilience and higher security level. The aim is to address network resilience by proposing an intelligent recommender system that recommends paths in real-time based on predicting link failures and network congestions. We use statistical data of the network such as link propagation delay, the number of packets/bytes received and transmitted by each OpenFlow switch on a specific port. Different state-of-art machine learning models has been implemented such as logistic regression, K-nearest neighbors, support vector machine, and decision tree to train these models in normal state, links failure and congestion conditions. The models are evaluated on the Mininet emulation testbed and provide accuracies ranging from around 91–99% on the test data. The machine learning model with the highest accuracy is utilized in the intelligent recommender system of the SDN controller which helps in selecting resilient paths to achieve a better security and quality-of-service in the network. This real-time recommender system helps the controller to take reactive measures to improve network resilience and security by avoiding faulty paths during path discovery and establishment.
Cyber-physical system (CPS) is the latest generation of digital systems in which physical processes are networked with computer systems where algorithms monitor and control the physical processes. In general, a full-blown CPS is a nexus network of interacting physical elements. Recently, Academia and industries have shown huge interest in this new research paradigm as it provides diversified advantages such as autonomy, functionality, reliability, and security [1,2]. The combination of CPS and Internet-of-Things (IoT) has an immense capability for supporting complex processes for directing and controlling industrial systems [3]. Thus, large industrial organizations including manufacturing, defense, energy, medical, and many others now adopt CPSs. Such organizations have rigid, overloaded infrastructures that are remaining from many years of preferential repair and poorly connections that introduce the poor Quality of Service (QoS) and reduces the possibility of other service integration leading to a low security level and possible attacks. Therefore, data protection and security as well as lack of prioritization by management among many others are the challenges and risks in the CPS related to industry 4.0. Among those providing network, resilience is a key challenge because better network resilience services also boost security by blocking attacks and guarantee excellent robustness. Improved network resilience solves a wide range of risks such as, poor resilient networks have RTT delay vary from microsecond to second when data size is increased. This is biggest pitfall for time critical application in e-health.
Recently, Software-Defined Networking (SDN) acts as a considerable problem solver. Generally, it is a network architecture approach that separates the control plane from the data plane and adds more programmability at the centralized network controller side. The application layer consists of a network or a business application. The Control layer is considered as the brain of the network which contains a centralized network controller and routing decisions are taken by it. The Data plane contains cyber-physical components. Southbound APIs (usually OpenFlow) is interaction mediums between the network controller and various components of the cyber-physical system and northbound APIs are the link between the applications layer and network controller (refer to Figure 1). Software-defined networking reduces logistics efforts and introduces flexibility and orchestration with its global view of the overall network. It gives consistent network management which can be a part of other complex technology such as the cyber-physical system. Network visibility, optimized network device utilization, and service integration are some advantages of software-defined networking that facilitate the scope of improving network resilience. One possible way for improving network resilience is by implementing smart computer networks with the help of machine learning. As software-defined networking has a centralized network controller, machine learning algorithms can be applied in cyber-physical systems. With this, the network controller can take optimized traffic forwarding decisions and make adaptive routing policies depending upon the stats of the network that improves network resilience.
Nowadays, smart IoT systems are involving in health sector at a big scale. Such infrastructure has legacy networks which are not resilient. Also in current pandemic, the traffic in e-health networks is increasing as more positive corona cases arrived. Such traffic creates congestion and sometimes fails the links of e-health networks. Thus, it is necessary to make e-health network more resilient towards this problem. In [4] introduces bunch of papers for reliable and secure e-health networks from that Pandey et al. [5] presents a technique for securing the e-health networks from counterfeit medicine using block chain. Khamparia et al. [6] detects and classify cervical cells using transfer learning. For high-end functionality with QoS assurance, Aujla et al. [7] presents integrating Cloud and Edge computing with SDN. This composite framework has three features: a) offloading scheme to support Edge-Cloud interplay, b) an SDN assisted virtualized flow management scheme, and c) a secure Lattice-based cryptosystem. A secured framework for SDN based edge computing in healthcare is introduced in [8]. The proposed framework has better edge collaboration and resource utilization that results low ligancy and higher throughput. Baktir et al. [9] introduces SDN based multi-tier computing and communication architecture. Based on type of health service and demand, the tasks are done externally behalf of end points. Meng et al. [10] presents security enforcement framework for data sharing system of healthcare based on SDN. In that they introduce SRM (service releasing model) that helps service provider for regulating the data services based on authorization of consumer. They also present getaway in the framework that contains information flow model (IFM) and IFM-based virtual machine access control algorithm.
In this paper, we build an intelligent recommender system that recommends routing paths depending on the current state of the network. The advantage is for a secure communication to prevent possible denial-of-service and assure deliverability without any loss of important information allowing for a better task completion. We use OpenFlow as a southbound API to fetch traffic information and network information such as packet size, the number of packets, delays for traveling packets through each link and sends it to the network controller. LLDP (link layer discovery protocol) is commonly used for network discovery and measuring latency for links. LLMP (link-layer measurement protocol) [11] is a prototype proposed for latency estimation which is based on LLDP. The aforementioned traffic information shows non-identical behavior from healthy conditions when different links of the network are congested or failed. These unfortunate conditions compromise the resilience of the network along with QoS. Each attribute of data plays a unique role in the identification of hidden knowledge. We apply different machine learning algorithms that predict failure and congested links and, based on that recommender system of the SDN controller can assist in reactively establishing new paths in real-time by avoiding the faulty links. Thus, the SDN controller can establish a more resilient path. We evaluate the following machine learning algorithms for the proposed intelligent recommender system: (1) decision tree, (2) nearest neighbors, (3) logistic regression, and (4) support vector machine (SVM). Moreover, we compare the accuracy and predicting speed measurements for each of these algorithms. The predicting speed of the algorithms is vital as the intelligent recommender system needs to react in real-time. We extract and visualize performance of machine learning algorithms by different matrices such as macro, micro, and weighted precision-recall-F1 score.
The remaining of the paper is divided into the following parts: Section 2 reviews related work. Section 3 presents the proposed model architecture asa recommender system. Experimental setup and results are discussed in Section 4. Finally, we conclude our research in Section 5 along with the future work.
In this section, we discuss existing work for improving network resilience with different approaches. The various metric of graph robustness for improving network resilience is evaluated and compared in [12]. The proposed work enhances three real-world physical-level networks by introducing a collection of connections to strengthen a given robustness metric. As a result, adding links to balance link betweenness indicates the highest consistency in delivering the best network durability against centrality-based attacks of all studied robustness functions. Improvement of resiliency with the self-healing approach is discussed in [13] where the availability of redundant links allows the network compatibility to be restored. The proposed work model introduces a cavity equation and contrasts an empirical approximation with numerical simulation for the average values of connectivity under random failures. However, these approaches introduce extra links that are not an optimal solution. Mauthe et al. [14] present a range of resilience concepts that will guide the ongoing study in the large community and more importantly, the action's operations. The author discusses studies on structural and operational forms of architectural resilience that should be considered while engineering the networks. But it may need to change those forms in the future for business policies or partial repairs of network and, also gives some rigidity constraints to the networks. For the resilient controller positioning issue, Tanha et al. [15] suggest a new formulation that considers the capability of the controllers as well as the requirement of the switches. This model also minimizes the latency involved in propagation, total incurred cost including the cost of deployment and the number of required controllers achieved while considering different resilience levels to enhance the resilience of the controller plane. An SDN-based microgrid network architecture is proposed in [16] to improve microgrid resilience. In the testbed, three SDN controller functions are proposed which are based on microgrid connectivity specifications, including latency-guaranteed communication, failover recovery and QoS support to achieve the objective. To improve the resilience of networks, Modarresi et al. [17] designed a new architecture that includes fog nodes and integrates with SDN. The fog nodes are connected to OpenFlow switches and can inspect the passing data packets. Maziku et al. [18] developed a quantitatively assess security risks technique in smart grid and suggest a safety score model in the IEC 61850 network. The above works propose a software-defined networking approach for enhancing network resilience. Machine learning technique can effectively improve resilience based on previous network data that implemented by software-defined networking and advances above study objectives solution. In the paper [19], anomaly detection and attack identification are done using different machine learning algorithms to improve resilience.
In an SDN-based network, Jhaveri et al. [20] presented a contract-based resilient mechanism, SDN-RM, for time-critical CPS which proposes an end-to-end delay estimation mechanism. Firstly, it presents the experiments to demonstrate the accuracy given by the mechanism in estimating end-to-end delay, and then it demonstrates the resilience of SDN-RM. Experiments depict that SDN-RM performs better than other approaches under different events, detects faults, and recovers quickly from multiple faults by seeking an alternative route. The problem of QoS routing in delay-constrained cyber-physical robotic systems is addressed in [21] where the proposed framework dynamically finds a QoS efficient route with minimum overhead while constantly tracking multi-dimensional cost metric network connections. The machine responds to the irregular network situation to provide resilience in the network by following a plan to move the flows to more reliable alternate routes. The survey of state-of-art methods where ML is more efficiently applicable to satisfy existing security standards is presented in [22]. The authors present the taxonomy of threats that define the overall functionality, composition, forms, and delivery mechanisms of various types of malwares. Past studies introduced the emergence of machine learning within the SDN model to provide resilience [23,24,25,26]. This paper, however, takes previous work to an advanced level where multiple alternate paths are suggested to the network controller but if some alternate paths violate any business policies, then the network controller can avoid suggestions. This introduces some flexibility to the network controller.
After reviewing the past briefly discussed approaches, it becomes clear that recommender system has immense potential in providing real-time resilience in SDN-networks as it is not bound the controller forcefully to follow the suggested route. Thus, this provides flexibility.
We examine security in a communication network of a CPS which is based on the SDN framework. The network uses OpenFlow protocol for programming the OpenFlow switches. The end system elements called hosts generate the traffic flows, which travels through different switches. Switch forwards packets flow according to the forwarding table. The controller can fetch various network statistics by querying OpenFlow enabled switches. Our model aims to make the network more resilient by suggesting routes based on link failure and link congestion. For the prediction of link failure and congestion, the controller fetches several network state data periodically with the help of the LLDP protocol. LLDP is a layer-2 protocol used to advertise device capabilities and identities. In our model, it induces control overhead to measure the network statistics. As presented in [16], estimation of link delays is done using LLDP by calculating the difference between the timestamp of received and transmission of LLDP packets. The SDN controller uses the shortest hop path as the default routing algorithm.
Network resilience that leads to higher security measure is improved through an introduction of an intelligent recommender system based on network link conditions (refer to Figure 2) in the control plane. The architecture of SDN consists of the network controller and OpenFlow enabled forwarding devices in the data plane. The functions of the control plane are to provide signaling, security, load balancing, etc. The components of the data plane follow the instructions transmitted by the SDN controller. In this work, we propose a recommender system for the SDN controller which is divided into two parts: 1) Probabilistic machine learning model and 2) Hypothesis function. The probabilistic machine learning model provides probabilities of getting links congested or failed in the network. This model is a multi-class classifier trained on network statistics provided by the controller. There may exist multiple paths with the same number of hops in the shortest hop routing. Recommender system prioritizes multiple paths with the help of the hypothesis function and suggests to the controller. In the proposed way, the controller gets suggestions of alternative path links that contain a lower probability of getting congested or failed.
The hypothesis function converts link failure or congestion probabilities into a single value that represents path safety. The path is considered safer which has a high hypothesis value. Suppose there are m alternative paths that all have the same number of hops, then the simplest form of hypothesis function designed as follows:
H=1−1n∑ni=0Pi | (1) |
where Pi represents congestion or failure probability of ith link of alternate path among links.
We consider the prediction of congestion and link failure as a multiclass classification problem. Each congestion or a link failure is defined as a separate class and one extra class that represents an ideal condition. Therefore, if there are links then it becomes a + 1 class classification problem. To successfully predict congestion and link failure, we need to create this situation artificially by manually disabling links and sending more traffic than the capacity of any link. Simultaneously, we also generate random traffic flow between any two host systems that represent communication between elements of a CPS. We consider the following measurements for the network (refer to Table 1). A link has two ports and three remaining attributes (LC, PL, LD) and each port has four attributes (Rx-pkts, Rx-bytes, Tx-pkts, Tx-bytes). Thus, the total dimensions of the dataset are *[(2*4) + 3] or *11. We split data into two parts with a ratio of 0.25 for training and testing. We scale the data before applying non-tree-based algorithms. For regularization, we perform appropriate hyperparameter tuning in KNN, LR, SVM algorithms with grid search and post pruning in the decision tree algorithm.
Features | Conventions |
No. of received packets at a particular port of the switch | Rx-pkts |
No. of received bytes at a particular port of the switch | Rx-bytes |
No. of transmitted packets at a particular port of the switch | Tx-pkts |
No. of transmitted bytes at a particular port of the switch | Tx-bytes |
Link capacity | LC |
Packet loss | PL |
Link delay | LD |
The features such as LC, PL, and LD can help to identify abnormal behavior of links in the network. There are two types of packets that travel in the network. (i) LLDP packets for measurements of network states and (ii) data packets forwarded by end systems of the CPS. It is necessary to distinguish these packets. The combination of Rx-pkts, Rx-bytes, Tx-pkts, and Tx-bytes can differentiate these packets. A global view of the network can be obtained by the SDN controller and, it can help to recognize the change in the forwarding flow after congestion or link failure. In normal conditions, switch C forwards the packets flow through the C-A link (refer to Figure 3). There are two possibilities (C-B-A and C-D-A) for routing the packets from C to A after the C-A link gets congested or fails according to the shortest hop path algorithm. Machine learning algorithms can detect these changes in flow by parametric coupling of Rx-pkts, Rx-bytes, Tx-pkts, Tx-bytes of the respected ports of (i) C, B and A or (ii) C, D and A. The recommender system prioritizes both paths based on hypothesis function values and gives recommendations to the controller.
1) Decision tree:
A decision tree is a supervised machine learning algorithm that can be used for solving regression and classification problems. The accuracy of the tree is greatly impacted by strategic divisions made. The decision criteria are distinct for classification and regression trees. It uses different algorithms to determine if a node can be divided into two or more sub-nodes. Sub node formation increases the uniformity of the subsequent sub-nodes. The decision tree partitions the nodes into all available variables and then chooses the split that results in most of the sub-nodes being uniform. A complex step in a decision tree algorithm helps to determine which attribute to be put as internal nodes at the root or various tree levels. Researchers have worked and devised several approaches to solving this attribute selection problem such as Entropy, Information gain, Gini-index, etc. Pruning is the method for carrying out regularization tasks in a decision tree algorithm. There are two types of pruning as follows 1) pre-pruning, 2) post-pruning. By restricting growing parameters of the tree, such as max splits and max depth, pre pruning is achieved. In Post pruning, a leaf node takes the place of the subtree, whose label is specified by the most frequent class of the sub-tree.
Entropy(T)=∑ni=1−P⋅log2Pi | (2) |
Entropy(T,X)=∑nc∈XP(c)⋅Entropy(c) | (3) |
InformationGain(T,X)=Entropy(T)−Entropy(T,X) | (4) |
Equation (2) presents Entropy for a single attribute. Where T is the current state, Pi represents the percentage of class i in a node of state T, and n is the total number of samples. Equation (3) presents Entropy for multiple attributes where T is the current state, X is the selected attribute, c is a unique category in X the attribute. P(c) represents the probability of occurring c category in X the attribute.
2) Logistic regression:
Logistic Regression is a supervised algorithm for classification that is omnipresent and commonly used. It is very simple to use and its output in a linearly separable class. This is based on a sample's probability of belonging to one class. A judgment function called Sigmoid or Logistic is used as a threshold function. To reduce the effect of overfitting, ridge, and lasso regularization are used. They shrink the coefficients in the resulting regression by adding some new entity in the cost function. We can categorize logistic regression into three different types based on the behavior of prediction (a) Binomial logistic regression: when there are two classes (b) multinomial logistic regression: when there are more than two classes (c) Ordinal logistic regression: prediction attribute contains order. Depending upon the type of logistic regression cost function and regularization methodology are used.
odds Ratio=P1−P | (5) |
logit(P)=log(P1−P) | (6) |
σ(z)=11+e−z,where z=∑mi=1wi⋅xi+w0 | (7) |
logloss=∑ni=1[y(i)log(σ(z(i)))+(1−y(i))log(1−σ(z(i)))] | (8) |
Equation (5) represents the odds ratio that is the odds in favor of a particular event. It is a measure of association between exposure and outcome. Here, P is the probability of events. Equation (6) represents the logit function as the odd ratio logarithm, which takes input values in the 0 to 1 range and then transforms them to values over the whole range of real numbers. The reverse of the logit function is called the Sigmoid or logistic function represented in Eq (7) where z is dimensional hyperplane, wrepresents weights, andx is the input value. Because of its characteristic form, it is called the sigmoid feature. Sigmoid function mapped real value into the range [0, 1] with intercept 0.5 that considers as a threshold. Equation (8) measures the difference between two probability distributions called cross-entropy that commonly act as cost function where n is total samples and yi is an actual target value of the ith sample.
3) K-Nearest Neighbors (KNN):
KNN is considered as the supervised machine learning algorithm. For a given K algorithm value, the K nearest neighbor of the unseen data point will be identified, and then the class will be allocated to the unseen data point by making the class that has the maximum number of data points out of all K neighbor classes. The algorithm assumes that similar things are near to each other and predict those data as one class. KNN is very sensitive about the distance between data points. Thus, the scaling of input data vectors is necessary before applying the KNN algorithm. The parameter K defines the complexity of the model. As the value of the parameter K is increases, the complexity of the model also increases, and the model introduces more overfitting.
distance(x,x′)=√∑mi=1(xi−x′i)2 | (9) |
P(y=j|X=x)=1K∑i∈AI(y(i)=j) | (10) |
Equation (9) represents the Euclidean distance formula where xandx' are two data points in m dimensional space and xixi' are Euclidean vectors, starting from the origin of the space. The input belongs to the class with a higher probability calculated as in Eq (10) where K is number neighbors.
4) Support vector machine:
There are many hyperplanes possible that can separate two classes. SVM performs classification by locating the hyperplane that separates them with a high margin. To solve the issues of classification and regression, a supervised machine learning algorithm SVM can be used. Support vectors are data points that are closer to the hyperplane and influence the position and orientation of the hyperplane and the margin is the perpendicular distance between two support vectors. Support vectors which are a small subset of training samples fully specify the decision functions. Simple SVM can classify data linearly where kernel SVM can separate data non-linearly. It follows the hypothesis for the classification mentioned in Eq (11). Here, W.X+b represents hyperplane where W is weight vector, b is bias, and X is input data vector. 1 and -1 are the integer values that each represent one class.
h(xi)={−1,W.X+b<01,W.X+b≥0 | (11) |
In the following section, we discuss emulation set up and the outcomes we get.
Table 2 summarizes the tools and techniques used in our experimental elevation.
Ubuntu (20.0.4) | Operating system |
Mininet (2.2.2) | Network emulator |
Ryu (4.34) | Network controller |
OpenFlow (1.3) | Southbound API |
Python (2.7) | Programming language |
Iperf (3.1.3) | Traffic generator |
Scikit-learn (0.23.2) | Machine learning tool |
Pandas (1.1.4) | Data manipulation tool |
Numpy (1.19) | Tool for Multidimensional Array operation |
Matplotlib (3.3.3) | Visualization module |
We follow the topology of the OpenFlow switch present in Figure 4. The generation of more traffic than a bandwidth stimulates congestion while link failures are made manually.
In Figure 4, the "s" represents OpenFlow switches and "h" represents end systems of network. The "c" represents controller of the network.
We visualize the accuracy and performance of the machine learning algorithm in this part. Also, we campier the predicting speed of each algorithm and discuss feasibility.
The performance of the decision tree is higher than all algorithms as it separates the classes nonlinearly. KNNs have the low test and train accuracy and support vector machine and logistic regression has almost same and moderate accuracy (refer to Figure 5). The above situation is maintained in every link capacity.
As Figure 6 shows, KNNs have a higher time required for prediction than all algorithms for each link capacity as it considers all positions of data points while predicting. Decision tree and support vector machine has low prediction time that shows the best performance in real-time path prediction. Logistic regression has moderate time prediction.
The behavior of F1 score, precision, and recall is the same as test accuracy (refer to Figures 7–10). Here precision quantifies number of predicted fault links that are fault links, recall quantifies how many links fault predicted among total fault links and F-score is metric that balances both precision-recall. Macro represents matric by considering total true positives, false negatives, and false positives. For each label, and to find the unrated mean micro is used as an evaluation matric. However, it does not consider the label imbalance. To find the average weight by support (the number of true instances for each label) for each label weighted is used as an evaluating metric. This alters 'macro' to account for label imbalance. KNN has lower accuracies and PRF at all link capacities as it only considers the positions of data points and classifies based on majority vote system that may avoid similarities among data points. On other hand decision tree can separates data non-linearly that may cause high accuracies and PRF. From the results of simulation, decision tree is the best fit for the recommender system as it has lowest time prediction and highest accuracy, and it also lies in the category of white box algorithms (the algorithms that justifies why it takes decision). These properties are very crucial in the application of healthcare [27,28].
The article presents a security architecture that predict path quality based on link failure and congestion to overcome possible denial-of-service and assure deliverability of information within the network. The proposed intelligent system along with SDN architecture recommends the path for packet routing to the network controller. Link failure and congestion are considered as two important security factors for best path prioritization. As a result, network controller only takes those routes which has lower probability of getting links failed or congested, i.e., communication avoids faulty paths during path discovery. Thus, network architecture becomes more resilient towards congestion and link failure and leads to higher security level. The proposed architecture introduces low time delay and better Quality of Service which is very crucial in e-health and current pandemic where network needs to face more traffic and scalability in a secure environment. To predict the probability of quality path, we applied four machine learning algorithms namely, 1) Decision tree 2) K-nearest neighbors 3) Logistic regression 4) Support vector machine. We discuss the feature's importance for the prediction of link failure and congestion. We mathematically elaborate all these machine learning algorithms. We also measure the test accuracy for applied algorithms and F1 score, precision, recall for classification. Then we visualize the prediction speed of each algorithm. The future work should consider exploiting different complexity levels in the business policy and examine more security issues affecting path routing and communication. More complex machine learning algorithms can also be exemplified for comparisons such as artificial neural networks, gradient boosting, and assemble models which are unforeseen at the moment.
The authors declare that they have no conflicts of interest to report regarding the present study.
[1] | K. D. Kim, P. R. Kumar, An overview and some challenges in cyber-physical systems, J. Indian Inst. Sci., 93 (2013), 341-352. |
[2] | G. Greenwood, J. Gallagher, E. Matson, Cyber-physical systems: the next generation of evolvable hardware research and applications, in 18th Asia Pacific Symposium on Intelligent and Evolutionary Systems, (2015), 285-296. |
[3] | H. He, C. Maple, T. Watson, A. Tiwari, J. Mehnen, Y. Jin, et al., The security challenges in the IoT enabled cyber-physical systems and opportunities for evolutionary computing other computational intelligence, in 2016 IEEE Congress on Evolutionary Computation (CEC), (2015), 1015-1021. |
[4] |
H. Toral-Cruz, D. He, A. D. Mihovska, K. K. R. Choo, M. K. Khan, Reliable and Secure e-Health Networks, Wireless Pers. Commun., 117 (2021), 1-6. doi: 10.1007/s11277-021-08104-z
![]() |
[5] |
P. Pandey, R. Litoriya, Securing e-health networks from counterfeit medicine penetration using blockchain, Wireless Pers. Commun., 117 (2021), 7-25. doi: 10.1007/s11277-020-07041-7
![]() |
[6] | A. Khamparia, D. Gupta, V. H. C. De Albuquerque, Internet of health things-driven deep learning system for detection and classification of cervical cells using transfer learning, J. Supercomput, 76 (2020), 8590-8608. |
[7] |
G. S. Aujla, R. Chaudhary, K. Kaur, S. Garg, N. Kumar, R. Ranjan, SAFE: SDN-assisted framework for edge-cloud interplay in secure healthcare ecosystem, IEEE Trans. Ind. Inf., 15 (2019), 469-480. doi: 10.1109/TII.2018.2866917
![]() |
[8] |
J. Li, J. Cai, F. Khan, A. U. Rehman, V. Balasubramaniam, J. Sun, et al., A secured framework for sdn-based edge computing in IOT-enabled healthcare system, IEEE Access, 8 (2020), 135479-135490. doi: 10.1109/ACCESS.2020.3011503
![]() |
[9] |
A. C. Baktir, C. Tunca, A. Ozgovde, G. Salur, C. Ersoy, SDN-based multi-tier computing and communication architecture for pervasive healthcare, IEEE Access, 6 (2018), 56765-56781. doi: 10.1109/ACCESS.2018.2873907
![]() |
[10] | Y. Meng, Z. Huang, G. Shen, C. Ke, SDN-based security enforcement framework for data sharing systems of smart healthcare, IEEE Trans. Network Serv. Manage., 17 (2019), 308-318. |
[11] |
Y. Li, Z. P. Cai, H. Xu, LLMP: exploiting LLDP for latency measurement in software-defined data center networks, J. Comput. Sci. Technol., 33 (2018), 277-285. doi: 10.1007/s11390-018-1819-2
![]() |
[12] | M. J. F. Alenazi, J. P. G. Sterbenz, Evaluation an comparison of several graph robustness metrics to improve network resilience, 2015 7th International Workshop on Reliable Networks Design and Modeling (RNDM), (2015), 7-13. |
[13] | F. Morone, L. Ma, H. Makse, A. Scala, Enhancing network resilience via self-healing, in 2016 IEEE Workshop on Environmental, Energy, and Structural Monitoring Systems (EESMS), (2016), 1-5. |
[14] | A. Mauthe, D. Hutchison, E. K. Cetinkaya, I. Ganchev, J. Rak, James P. G. Sterbenz, et al., Disaster-resilient communication networks: Principles and best practices, in 2016 8th International Workshop on Resilient Networks Design and Modeling (RNDM), (2016), 1-10. |
[15] | M. Tanha, D. Sajjadi, J. Pan, Enduring node failures through resilient controller placement for software defined networks, in 2016 IEEE Global Communications Conference (GLOBECOM), (2016), 1-7. |
[16] |
L. Ren, Y. Qin, B. Wang, P. Zhang, P. B. Luh, R. Jin, Enabling resilient microgrid through programmable network, IEEE Trans. Smart Grid, 8 (2017), 2826-2836. doi: 10.1109/TSG.2016.2589903
![]() |
[17] | A. Modarresi, S. Gangadhar, J. P. G. Sterbenz, A framework for improving network resilience using SDN and fog nodes, in 2017 9th International Workshop on Resilient Networks Design and Modeling (RNDM), (2017), 1-7. |
[18] | H. Maziku, S. Shetty, Software defined networking enabled resilience for IEC 61850-based substation communication systems, in 2017 International Conference on Computing, Networking and Communications (ICNC), (2017), 690-694. |
[19] | A. Hussein, A. Chehab, A. Kayssi, I. H. Elhajj, Machine learning for network resilience: The start of a journey, in 2018 Fifth International Conference on Software Defined Systems (SDS), (2018), 59-66. |
[20] | R. H. Jhaveri, R. Tan, A. Easwaran, S. V. Ramani, Managing industrial communication delays with software-defined networking, in 2019 IEEE 25th International Conference on Embedded and Real-Time Computing Systems and Applications (RTCSA), (2019), 1-11. |
[21] | R. H. Jhaveri, R. Tan, S. V. Ramani, Real-time QoS routing scheme in SDN-based robotic cyber-physical systems QoS routing with SDN for manufacturing robotics, in 2019 IEEE 5th International Conference on Mechatronics System and Robots (ICMSR), (2019), 18-23. |
[22] |
R. Sagar, R. Jhaveri, C. Borrego, Applications in security and evasions in machine learning: A survey, Electronics, 9 (2020), 97. doi: 10.3390/electronics9010097
![]() |
[23] |
S. P. R-M, P. K. R. Maddikunta, M. Parimala, S. Koppu, T. R. Gadekallua, C. L. Chowdhary, et al., An effective feature engineering for DNN using hybrid PCA-GWO for intrusion detection in IoMT architecture, Comput. Commun., 160 (2020), 139-149. doi: 10.1016/j.comcom.2020.05.048
![]() |
[24] |
C. Iwendi, M. A. Alqarni, J. H. Anajemba, A. S. Alfakeeh, Z. Zhang, A. K. Bashir, Robust navigational control of a two-wheeled self-balancing robot in a sensed environment, IEEE Access, 7 (2019), 82337-82348. doi: 10.1109/ACCESS.2019.2923916
![]() |
[25] |
J. H. Anajemba, T. Yue, C. Iwendi, M. Alenezi, M. Mittal, Optimal cooperative offloading scheme for energy efficient multi-access edge computation, IEEE Access, 8 (2020), 53931-53941. doi: 10.1109/ACCESS.2020.2980196
![]() |
[26] |
C. Iwendi, S. Khan, J. H. Anajemba, M. Mittal, M. Alenezi, M. Alazab, The use of ensemble models for multiple class and binary class classification for improving intrusion detection systems, Sensors, 20 (2020), 2559. doi: 10.3390/s20092559
![]() |
[27] |
T. R. Gadekallu, N. Khare, S. Bhattacharya, S. Singh, P. K. Maddikunta, I. Ra, et al., Early detection of diabetic retinopathy using PCA-firefly based deep learning model, Electronics, 9 (2020), 274. doi: 10.3390/electronics9020274
![]() |
[28] |
S. Bhattacharya, P. K. R. Maddikunta, Q. V. Pham, T. R. Gadekallu, C. L. Chowdhary, M. Alazab, et al., Deep learning and medical image processing for coronavirus (COVID-19) pandemic: A survey, Sustainable Cities Soc., 65 (2021), 102589. doi: 10.1016/j.scs.2020.102589
![]() |
1. | Sagar Ramani, Rutvij H. Jhaveri, ML-Based Delay Attack Detection and Isolation for Fault-Tolerant Software-Defined Industrial Networks, 2022, 22, 1424-8220, 6958, 10.3390/s22186958 | |
2. | T. Bala Murali Krishna, S. Phani Praveen, Shakeel Ahmed, Parvathaneni Naga Srinivasu, Software-driven secure framework for mobile healthcare applications in IoMT, 2022, 18724981, 1, 10.3233/IDT-220132 | |
3. | P. Naga Srinivasu, G. JayaLakshmi, Rutvij H. Jhaveri, S. Phani Praveen, Juraj Machaj, Ambient Assistive Living for Monitoring the Physical Activity of Diabetic Adults through Body Area Networks, 2022, 2022, 1875-905X, 1, 10.1155/2022/3169927 | |
4. | Lei Wang, SiJiang Xie, Can Cao, Chen Li, 2022, Research on Security Service Model of Software Defined Network, 978-1-6654-5488-9, 347, 10.1109/ISCSIC57216.2022.00078 | |
5. | Nteziriza Nkerabahizi Josbert, Min Wei, Ping Wang, Ahsan Rafiq, A look into smart factory for Industrial IoT driven by SDN technology: A comprehensive survey of taxonomy, architectures, issues and future research orientations, 2024, 36, 13191578, 102069, 10.1016/j.jksuci.2024.102069 | |
6. | Nteziriza Nkerabahizi Josbert, Min Wei, Ping Wang, Industrial IoT regulated by Software-Defined Networking platform for fast and dynamic fault tolerance application, 2024, 135, 1569190X, 102963, 10.1016/j.simpat.2024.102963 | |
7. | Feng Qiu, Hui Xu, Fukui Li, Applying modified golden jackal optimization to intrusion detection for Software-Defined Networking, 2023, 32, 2688-1594, 418, 10.3934/era.2024021 | |
8. | João Pavão, Rute Bastardo, Nelson Pacheco Rocha, 2024, Chapter 42, 978-981-99-8323-0, 501, 10.1007/978-981-99-8324-7_42 |
Features | Conventions |
No. of received packets at a particular port of the switch | Rx-pkts |
No. of received bytes at a particular port of the switch | Rx-bytes |
No. of transmitted packets at a particular port of the switch | Tx-pkts |
No. of transmitted bytes at a particular port of the switch | Tx-bytes |
Link capacity | LC |
Packet loss | PL |
Link delay | LD |
Ubuntu (20.0.4) | Operating system |
Mininet (2.2.2) | Network emulator |
Ryu (4.34) | Network controller |
OpenFlow (1.3) | Southbound API |
Python (2.7) | Programming language |
Iperf (3.1.3) | Traffic generator |
Scikit-learn (0.23.2) | Machine learning tool |
Pandas (1.1.4) | Data manipulation tool |
Numpy (1.19) | Tool for Multidimensional Array operation |
Matplotlib (3.3.3) | Visualization module |
Features | Conventions |
No. of received packets at a particular port of the switch | Rx-pkts |
No. of received bytes at a particular port of the switch | Rx-bytes |
No. of transmitted packets at a particular port of the switch | Tx-pkts |
No. of transmitted bytes at a particular port of the switch | Tx-bytes |
Link capacity | LC |
Packet loss | PL |
Link delay | LD |
Ubuntu (20.0.4) | Operating system |
Mininet (2.2.2) | Network emulator |
Ryu (4.34) | Network controller |
OpenFlow (1.3) | Southbound API |
Python (2.7) | Programming language |
Iperf (3.1.3) | Traffic generator |
Scikit-learn (0.23.2) | Machine learning tool |
Pandas (1.1.4) | Data manipulation tool |
Numpy (1.19) | Tool for Multidimensional Array operation |
Matplotlib (3.3.3) | Visualization module |