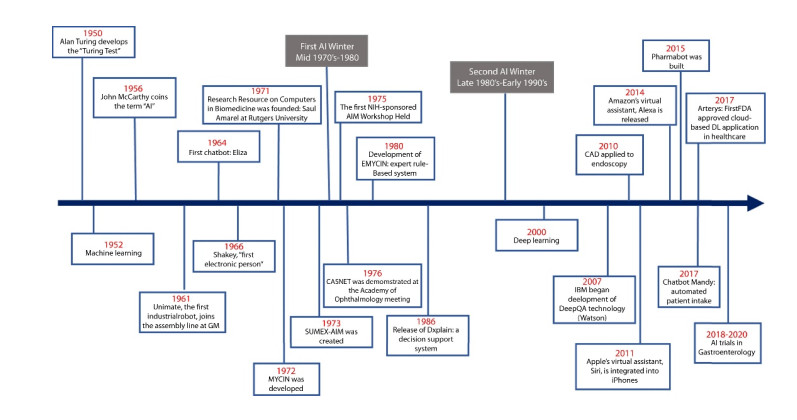
Uveal melanoma (UM) is the most aggressive intraocular tumor worldwide. Accurate prognostic models are urgently needed. The present research aimed to construct and validate a prognostic signature is associated with overall survival (OS) for UM patients based on metabolism-related genes (MRGs).
MRGs were obtained from molecular signature database (MSigDB). The gene expression profiles and patient clinical data were downloaded from The Cancer Genome Atlas (TCGA) database. In the training datasets, MRGs were analyzed through univariate Cox regression analyses and least absolute shrinkage and selection operator (LASSO) Cox analyses to build a prognostic model. The GSE84976 was treated as the validation cohort. In addition, time-dependent receiver operating characteristic (ROC) and Kaplan-Meier survival curve analyses the reliability of the developed model. Then, gene set enrichment analysis (GSEA) was used for gene enrichment analysis. Nomogram that combined the five-gene signature was used to evaluate the predictive OS value of UM patients.
Five MRGs were identified and used to establish the prognostic model for UM patients. The model was successfully validated using the testing cohort. Moreover, ROC analysis demonstrated a strong predictive ability that our prognostic signature had for UM prognosis. Multivariable Cox regression analysis revealed that the risk model was an independent predictor of prognosis. UM patients with a high-risk score showed a higher level of immune checkpoint molecules.
We established a novel metabolism-related signature that could predict survival and might be therapeutic targets for the treatment of UM patients.
Citation: Han Zhao, Yun Chen, Peijun Shen, Lan Gong. Construction and validation of a novel prognostic signature for uveal melanoma based on five metabolism-related genes[J]. Mathematical Biosciences and Engineering, 2021, 18(6): 8045-8063. doi: 10.3934/mbe.2021399
[1] | Isabeau Birindelli, Kevin R. Payne . Principal eigenvalues for k-Hessian operators by maximum principle methods. Mathematics in Engineering, 2021, 3(3): 1-37. doi: 10.3934/mine.2021021 |
[2] | Hitoshi Ishii . The vanishing discount problem for monotone systems of Hamilton-Jacobi equations. Part 1: linear coupling. Mathematics in Engineering, 2021, 3(4): 1-21. doi: 10.3934/mine.2021032 |
[3] | Takeyuki Nagasawa, Kohei Nakamura . Asymptotic analysis for non-local curvature flows for plane curves with a general rotation number. Mathematics in Engineering, 2021, 3(6): 1-26. doi: 10.3934/mine.2021047 |
[4] | L. Dieci, Fabio V. Difonzo, N. Sukumar . Nonnegative moment coordinates on finite element geometries. Mathematics in Engineering, 2024, 6(1): 81-99. doi: 10.3934/mine.2024004 |
[5] | Karl K. Brustad, Erik Lindgren, Peter Lindqvist . The infinity-Laplacian in smooth convex domains and in a square. Mathematics in Engineering, 2023, 5(4): 1-16. doi: 10.3934/mine.2023080 |
[6] | Plamen Stefanov . Conditionally stable unique continuation and applications to thermoacoustic tomography. Mathematics in Engineering, 2019, 1(4): 789-799. doi: 10.3934/mine.2019.4.789 |
[7] | Pengfei Guan . A weighted gradient estimate for solutions of Lp Christoffel-Minkowski problem. Mathematics in Engineering, 2023, 5(3): 1-14. doi: 10.3934/mine.2023067 |
[8] | Antonio Iannizzotto, Giovanni Porru . Optimization problems in rearrangement classes for fractional p-Laplacian equations. Mathematics in Engineering, 2025, 7(1): 13-34. doi: 10.3934/mine.2025002 |
[9] | Neil S. Trudinger . On the local theory of prescribed Jacobian equations revisited. Mathematics in Engineering, 2021, 3(6): 1-17. doi: 10.3934/mine.2021048 |
[10] | Lina Zhao, Eun-Jae Park . A locally conservative staggered least squares method on polygonal meshes. Mathematics in Engineering, 2024, 6(2): 339-362. doi: 10.3934/mine.2024014 |
Uveal melanoma (UM) is the most aggressive intraocular tumor worldwide. Accurate prognostic models are urgently needed. The present research aimed to construct and validate a prognostic signature is associated with overall survival (OS) for UM patients based on metabolism-related genes (MRGs).
MRGs were obtained from molecular signature database (MSigDB). The gene expression profiles and patient clinical data were downloaded from The Cancer Genome Atlas (TCGA) database. In the training datasets, MRGs were analyzed through univariate Cox regression analyses and least absolute shrinkage and selection operator (LASSO) Cox analyses to build a prognostic model. The GSE84976 was treated as the validation cohort. In addition, time-dependent receiver operating characteristic (ROC) and Kaplan-Meier survival curve analyses the reliability of the developed model. Then, gene set enrichment analysis (GSEA) was used for gene enrichment analysis. Nomogram that combined the five-gene signature was used to evaluate the predictive OS value of UM patients.
Five MRGs were identified and used to establish the prognostic model for UM patients. The model was successfully validated using the testing cohort. Moreover, ROC analysis demonstrated a strong predictive ability that our prognostic signature had for UM prognosis. Multivariable Cox regression analysis revealed that the risk model was an independent predictor of prognosis. UM patients with a high-risk score showed a higher level of immune checkpoint molecules.
We established a novel metabolism-related signature that could predict survival and might be therapeutic targets for the treatment of UM patients.
Cerebrovascular disease refers to brain tissue damage caused by obstruction of blood circulation in the brain [1]. Cerebrovascular disease is usually manifested in people who experience acute nonfatal events (i.e., emergency department visit, hospitalization) or fatal events [2], including: stroke [3,4,5], subarachnoid hemorrhage (SAH) [6,7,8], arterial dissection [9,10], cerebral autosomal dominant arteriopathy with subcortical infarcts and leukoencephalopathy (CADASIL) [11,12,13], arteriovenous malformation (AVM) [14,15,16], cerebral venous sinus thrombosis (CVST) [17,18,19], and moyamoya disease [20,21,22]. Stroke is the most common type of cerebrovascular disease [23], which is caused by the blockage or rupture of an artery supplying the brain [1]. Cerebrovascular disease is the leading cause of severe long-term disability and the second leading cause of death worldwide [24]. Cerebrovascular disease has a very high mortality and disability rate. If left untreated, it can lead to lifelong disability or death. Therefore, it is very important to study the diagnosis and treatment of cerebrovascular diseases. Nowadays, the main diagnostic modalities for cerebrovascular disease include electroencephalogram (EEG) [25,26], cerebral angiography (CAG) [27,28,29], MRI [30,31] and transcranial Doppler (TCD) ultrasonography [32,33,34]. TCD ultrasonography can penetrate the skull without trauma. It is easy to perform, reproducible, and allows for continuous, long-term dynamic observation of the patient. More importantly, it can provide important hemodynamic information that cannot be measured by MRI, DSA, single photon emission computed tomography (SPECT), and other imaging techniques. Therefore, it has important significance in the evaluation of cerebrovascular disorders and differential diagnosis.
TCD ultrasonography is a non-invasive examination method to evaluate the hemodynamics of the skull base arteries by using the weak parts of the human skull as the detection acoustic windows (such as the temporal bone, the foramen magnum, and the orbit). [35,36,37]. TCD ultrasonography assesses blood flow status mainly by the level of blood flow velocity [38]. It is the only non-invasive and real-time neuroimaging modality for assessing the blood flow characteristics of the cerebral basal vessels, which can add physiological information to structural imaging [39]. TCD ultrasonography is an inexpensive, rapid, real-time, non-invasive method for measuring cerebrovascular blood flow and is the most convenient method to detect vascular changes in response to intervention during the treatment of clinical acute cerebrovascular events [40]. TCD ultrasonography uses pulsed Doppler technology and a 2 MHz emission frequency to allow the ultrasound beam to penetrate into the thin part of the skull. By directly tracing the Doppler signal of the blood flow in the fundic arteries, the hemodynamic parameters of the fundic arteries are obtained to reflect the functional status of the cerebral vessels and to determine possible intracranial problems. Although TCD ultrasonography can sensitively reflect the functional status of cerebral blood vessels, it cannot guarantee the incidence angle of ultrasound. It requires doctors to clearly understand the path of intracranial blood vessels and be able to perform skilled ultrasound diagnosis. The main drawback of TCD ultrasonography is that the operator cannot see the course of the intracranial vessels and the angle between the vessels and the ultrasound beam, reducing the accuracy of repeated blood flow velocity measurements [41].
Artificial intelligence (AI) is a new technical science that studies theories, methods, technologies and application systems for simulating, extending and expanding human intelligence [42]. AI is a branch of computer science that attempts to understand the essence of intelligence and produce a new intelligent machine that responds in a similar way to human intelligence. Research in this field includes robotics, language recognition, image recognition, natural Language processing (NLP) and expert systems, etc. [43,44]. The rapid development of artificial intelligence has brought many changes to the medical field. AI can detect meaningful relationships in datasets. Artificial Intelligence in Medicine (AIM) mainly uses computer technology to make clinical diagnosis and give treatment recommendations and has been widely used in many clinical situations to diagnose, treat and predict outcomes [45]. In recent years, many studies have shown that AI is increasingly applicable to healthcare, and that algorithms have matched or exceeded the performance of doctors on a growing number of tasks [46]. In addition, research related to AI in emergency medicine is rapidly increasing, especially for acute radiological imaging and prediction-based diagnosis [47,48]. AI has shown great advantages in assisting doctors to predict and diagnose. There have been many studies devoted to the application of AI to TCD ultrasonography, including brain computer interface (BCI) [49,50], TCD signal classification [51,52], noise removal [53,54], and other methods to assist diagnosis and treatment [55,56,57]. AI can greatly improve the accuracy of TCD ultrasonography diagnosis and treatment.
In this paper, w we first review the development, principles, and applications of TCD ultrasonography and other related knowledge, and briefly introduce the development of AI in the field of medicine and emergency medicine. Finally, we summarize in detail the applications and advantages of AI technology in TCD ultrasonography including the establishment of an examination system combining brain computer interface (BCI) and TCD ultrasonography, the classification and noise cancellation of TCD ultrasonography signals using AI algorithms, and the use of intelligent robots to assist physicians in TCD ultrasonography and discuss the prospects for the development of AI in TCD ultrasonography.
Under the same conditions, the inner diameter of the cerebral arteries is relatively almost constant. Corresponding changes in regional cerebral blood flow can be inferred from the decrease or increase in cerebral blood flow velocity [58]. The TCD imaging tool assesses flow parameters and cerebrovascular hemodynamics in real time using low-frequency ultrasound (i.e., ≤ 2 MHz) in the basilar cerebral artery. The physiological information provided by these parameters can be used in conjunction with structural information obtained by various existing imaging techniques [59]. TCD is considered to be the most practical technique to observe cerebral blood flow in the diagnosis of cerebrovascular diseases [60], and the four most commonly used acoustic windows for TCD examination are: temporal, suboccipital, transorbital, and submandibular windows [37]. A comprehensive TCD assessment needs to include measurements from each of the four windows and should examine the flow pathways within each major branch of the Willis loop [61]. TCD assesses the physiological health of specific vascular regions by measuring blood flow relative to blood pressure changes (cerebral autoregulation), end-tidal CO2 changes (cerebrovascular reactivity), and response to cognitive and motor activation (neurovascular coupling or functional congestion), which is commonly used in acute ischemic stroke [62,63,64], vasospasm [65,66], SAH [67,68,69], sickle cell disease [70,71,72], and brain death [73,74,75].
In the late 19th century to the early 20th century, after the discovery of the piezoelectric and anti-piezoelectric effects in physics, people found a way to use electronics to generate ultrasound. From then, a chapter in the history of the development and promotion of ultrasound technology was opened. In 1922, the definition of ultrasound was first proposed. Subsequently, ultrasound technology has made great progress in clinical medicine. In the late 1970s, TCD ultrasonography was proposed and used for blood flow detection [76,77,78]. In 1982, Aaslid et al. [79] described a noninvasive method for determining blood flow velocity in the basilar artery of the brain. The middle cerebral artery (MCA) velocity can be determined from the Doppler signal generated by placing the probe of a Doppler ultrasound instrument in the temporal region above the zygomatic arch. The device has been continuously updated and developed in the following decades and has now reached its fourth generation.
TCD ultrasonography is based on the Doppler effect. The Doppler effect refers to the change in the wavelength of an object's radiation due to the relative motion of the source and the observer. The ultrasound beam does not have directional changes when propagating in the same kind of homogeneous medium because of good penetrating ability of ultrasound. When encountering different media surfaces, the ultrasound beam will be partially reflected, and the rest will continue to propagate. When the surface of the media is irregular and the diameter of the obstacle is smaller than the wavelength of the incident wave, scattering of the ultrasound beam occurs. The receiving probe can receive scattered waves at any angle. There are large numbers of red blood cells in the bloodstream, which are seen as scatterers. The scattered wave reflected back is the main component of the Doppler signal. The formula describing the relationship between flow velocity (reflector velocity) and Doppler shift [37] is:
Reflector speed=(Doppler Shift)×Propagation speed2×Incident frequency×cos(θ) | (1) |
where the wave propagation velocity is a constant, and the propagation velocity in various media can be found. θ is the angle of incidence or the angle of the emitted wave relative to the direction of the blood vessel (blood flow). If the angle is zero or if the emitted wave is parallel to the direction of the flow, since the cosine of 0 is 1, we get the most accurate measurement of the flow velocity. The larger the angle and the smaller the cosine value, the larger the error in our velocity measurement. Therefore, it is important to minimize this angle to less than 30 degrees to keep the error below 15% [37].
The detection depth refers to the distance between the examined vessel and the probe. In each group of pulsed ultrasound waves are emitted by the low-frequency pulse probe transmitter, determined by the range gating through the preset interval between transmitting and receiving pulse waves. The detection depth is very important for the identification of intracranial vessels [80].
The blood flow velocity can be calculated from Eq (1) and is an important parameter to determine the pathology of the body. PSV is the initial peak of the TCD waveform for each cardiac cycle. EDV shows the low resistance flow pattern in all major intracranial arteries and is approximately equal to 20-50% of PSV. MFV is equal to EDV plus one third of the difference between PSV and EDV [81,82,83].
PI and RI are descriptive of the spectral pattern. PI equals PSV minus EDV divided by MFV and RI equals PSV minus EDV divided by PSV. PI is one of the most important hemodynamic parameters provided by TCD to assess cerebral hemodynamic changes in patients with acute neurological injury [84]. RI is generally used to measure flow resistance [85].
The spectral feature of blood flow reflects the state of blood flow in blood vessels. The ordinate on the TCD spectrum is the blood flow velocity. The periphery of the spectrum represents the fastest blood flow velocity at a certain point in the cardiac cycle. The baseline represents zero blood flow velocity. The intensity of the spectral signal is represented by color, and the color change of the signal from weak to strong is blue, yellow, and red. That is, where there are more red blood cells, the signal is red, and where there are fewer red blood cells, the signal is blue [86].
Currently, there are two types of transcranial Doppler devices, non-duplex and duplex. Non-duplex devices (non-imaging devices) can "blindly" identify arteries based on audible Doppler shift and spectrum without visual guidance. Specific identification is based on standard criteria, including: cranial window used, probe orientation, sample volume depth, and blood flow direction, etc. [37]. Duplex equipment (imaging equipment) creating images using duplex technology requires ultrasound equipment, phased arrays, 2-3 MHz, sector transducers, and specific post-processing software to display real-time color Doppler images of vessels within the field of view and the spectral waveform of the interrogated vessel [87].
The term "artificial intelligence" (AI) was formally introduced by scholars at a conference at Dartmouth University in 1956 [44]. AI is knowledge engineering that acquires knowledge, analyzes and studies the expressions of knowledge, and uses these expressions to simulate human intellectual activity [88]. AI uses computers to simulate human behavior and train computers to learn human behavior. The birth of AI has greatly promoted the development of society [89]. AI is widely used in medical fields, such as robotics, medical diagnosis, medical statistics, and human biology. There are two main branches of AIM: virtual and physical. The virtual branch includes informatics approaches ranging from deep learning information management to the control of health management systems, including electronic health records, and active guidance of physicians in treatment decisions. The physical branch is represented by robots that help elderly patients or attending surgeons [90]. The development history of AIM is shown in Figure 1 [91]. In the 1970s, scientists began to explore AIM. Stanford University has developed MYCIN system, which can diagnose and prescribe infectious diseases [92], and ONCOCIN system, which can assist diagnosis [93]. Rutgers University has developed the CASNET system for computer-aided medical decision making [94]. Although the early development of AIM was not very successful, scientists have not given up on the search for AIM. So far, the development of AIM has been relatively mature. The best-known is IBM Watson, which can sift through 1.5 million patient records from decades of cancer treatment in seconds and provide doctors with alternative treatments [95]. Emergency medicine is a growing profession that plays a vital role in the event of patients requiring urgent medical care. Emergency medicine is mainly about providing timely medical triage and classifying patients according to urgency and severity [96]. The use of machine learning and deep learning may help the patterns that identify the data collected over the years and provide insights for improving the emergency department admissions process. Machine learning and deep learning can help ongoing medical trials and research and reduce the workload of medical professionals based on their strengths in areas such as data patterns, trend recognition, image analysis and task classification [97,98]. AI in emergency medicine can be used for pre-hospital emergency management, patient triage and disposition, medical disease and condition prediction, emergency department management, etc. [46].
Brain-computer interface (BCI) refers to create a direct connection between the human or animal brain and an external device to realize the function of information exchange between the brain and the device. It can process brain signals related to mental activity to produce thought-controlled reasoning mechanisms that can be used to control external devices [50]. Changes in TCD signals are related to cognitive tasks. Studies have shown that BCI can be developed based on the classification of cognitive tasks performed by users [99]. TCD has some advantages for the development of noninvasive BCI. Like EEG, TCD is portable. However, it is more robust to non-smoothness from external electrical disturbances and internal background brain activity. In addition, it is cheaper compared to functional MRI and magnetoencephalography [100].
In 2011, Andrew et al. investigated the feasibility of TCD ultrasonography based BCI by classifying the changes in cerebral blood flow velocity due to two thought tasks (word generation task and thought rotation task). The researchers simultaneously asked nine healthy adults to alternate between mental activity (such as word creation and brain rotation) and relaxation and measured cerebral blood flow velocity in their left and right cerebral arteries. Using linear discriminant analysis and a set of temporal features, the word generation task and the thought rotation task were classified according to blood flow velocity with mean accuracies of 82.9% + 10.5 and 85.7% + 10.0, respectively, for all participants. However, since there are few studies on TCD combined with BIC, the authors used a rest and activation time of 45 seconds to obtain high classification accuracy, which is relatively long for BCI applications and will limit the data transfer rate in practical use. In addition, the movement of the body or head of the person during the measurement will change the cerebral blood flow velocity, which is also a factor that cannot be ignored in the study of the combination of TCD and BIC [49]. In 2012, Andrew et al. achieve an average classification accuracy of over 70% of the tertiary problems within 20 seconds of the onset of cognitive activity using bilateral TCD measures, time-domain features, and linear classifiers based on previous research, which corresponds to a maximum data transfer rate of 1.2 bits per minute, as shown in Figure 2(a) [101]. In 2015, Goyal et al. demonstrated the feasibility of a three-class online BCI using a task that requires no visual cues at all. The communication system achieves an average information transfer rate of 1.08 ± 0.69 bits/min. The experimental setup is shown in Figure 2(b) [102]. In 2018, Khalaf et al. used data obtained from bilateral functional TCD ultrasound measurements to investigate the possibility of establishing a level 2 and 3 BCI system. Two different methods for building BCI systems are proposed: Incremental Window (IW) and Moving Window (MW). The main difference between the two methods is windowing and the formulation of eigenvectors. Wavelet transform analysis was performed on the raw data for each method. Statistical features are calculated from the wavelet transform coefficients. Wilcoxon test was performed on these features for feature selection. Then use linear kernel support vector machine (SVC) for classification [103]. The algorithmic flow of the data analysis using the moving window (MW) and incremental window (IW) methods is shown in Figure 2(c). All these results suggest that TCD is a promising measurement for BCI studies. BCI can visually express the changes of brain signals, and the establishment of a reasonable BCI system based on the results of TCD ultrasonography has a crucial role in promoting the development of TCD ultrasonography. The proposed BCI can reduce the high dependence of TCD ultrasonography consultation on human.
Although Doppler spectrum images can reflect the physiological and pathological conditions of each blood vessel to a certain extent, errors will occur when diagnosing diseases only by analyzing the images. TCD signals can be viewed as a series of discrete values that vary over time. Using the relevant knowledge of artificial intelligence to extract features that have never been recognized can reduce the error of diagnosis. In 2002, Guler et al. performed spectral analysis of TCD signals using fast Fourier transform (FFT) and adaptive autoregressive moving average (A-Arma) methods. The results show that the spectral density obtained by the A-Arma method is better than that obtained by the FFT method [52], and the filter structure is shown in Figure 3(a). In 2003, Serhatlioğlu et al. extracted features using FFT and classified these features using back propagation (BP) neural network and self-organizing maps (SOM) algorithm to compare the performance of these classifiers. They used a 16-bit sound card to transmit the TCD signals recorded in the temporal regions of the brains of 110 patients to a computer and processed the recorded signals of each patient using the FFT method. To make a correct and rapid diagnosis, the TCD blood flow signals were statistically sorted and classified by artificial neural network (ANN). Training uses BP neural network and SOM algorithm; learning uses momentum algorithm and delta-bar-delta algorithm. The results of these algorithms are compared in the case of classification and learning [104]. In 2008, Ozturk et al. used two different neuro-fuzzy classifiers to classify chaotic invariant features extracted from TCD signals and compared the performance of the classifiers. They performed a chaotic analysis of TCD signals recorded from the middle artery in the temporal region of the brain in 82 patients and 24 healthy individuals. Among the 82 patients, 20 patients had cerebral aneurysm, 10 patients had cerebral hemorrhage, 22 patients had cerebral edema, and 30 patients had brain tumor. It was found that all TCD signals represent nonlinear dynamics and are potentially low-level deterministic. To compare the classifier performance, two different neuro-fuzzy models, ANFIS and NEFCLASS, are constructed on the training set, and the system structure is shown in Figure 3(b). The rule base for the NEFCLASS model is created by applying a sample of 1000 epochs to the training subset. Furthermore, the ANFIS model was trained for 250 epoch samples until the convergence error dropped to 0.42 × 10−5. For samples in the test set, the ANFIS model achieves better classification accuracy than the NEFCLASS model. The classification accuracy after training of ANFIS model and NEFCLASS model are 94.40 and 88.88%, respectively [105].
From 2008 to 2012, Uğuz et al. conducted a series of studies in the field of TCD signal classification. They first implemented a biomedical system to classify TCD signals recorded from the temporal regions of the brains of 82 patients and 24 healthy individuals. Fuzzy discrete hidden Markov model and Rocchio-based hidden Markov model are used, respectively, to improve the accuracy of TCD signal classification. Subsequently, they combined feature ranking and dimensionality reduction methods when classifying TCD signals to improve classification efficiency and accuracy. To evaluate the effectiveness of the proposed method, experiments were conducted using the SVM classifier. When using the proposed method, higher classification accuracy and minimal number of features are obtained. These results show that the proposed new method can improve the classification efficiency and accuracy compared to the use of each method individually. Its feature extraction process and classification architecture are shown in Figure 3(d), (e) [51,106,107]. In 2017, Seera et al. used recurrent neural network (RNN) to classify TCD signals captured from the brain. They collected a total of 35 real anonymous patient records and conducted a series of narrow diagnostic experiments. Features extracted from TCD signals are classified with an RNN model with much loop feedbacks. The model is shown in Figure 3(f). In addition to the individual RNN results, a majority voting method is used to combine individual RNN predictions into an ensemble prediction. The TCD signals recorded from the arterial segment of the Circle of Willis from ischemic stroke were also analyzed. The results show that the ensemble RNN shows good performance with an average accuracy above 70% and a maximum accuracy of 85% [108]. TCD ultrasonography results are very important for the diagnosis and treatment of cerebrovascular diseases, but simple image analysis cannot make a very accurate judgment of the type of disease. As a result, the classification of TCD signals has greatly improved the accuracy of TCD ultrasonography in the management of acute cerebrovascular disease.
Biomedical signals are often contaminated with noise from sources such as power line interference and interference from recording electrode motion. Noise and interference can affect the original information signal. Karaboga et al. conducted a series of studies on noise cancellation of TCD signals. The artificial bee colony (ABC) algorithm based on the swarm intelligence optimization algorithm is used to design the IIR filter to denoise the TCD noise signal. Noise cancels the patient TCD signal. The SSA method was used for data analysis, and finally the SSA method was used to find new TCD signals' parameters for the determination of healthy subjects and patient subjects. Furthermore, the results are compared with those obtained by methods based on popular and recently introduced evolutionary algorithms and conventional methods. On this basis, the performance of the proposed method is tested to remove the noise on the TCD signal with a signal-to-noise ratio value of 2db. The simulation results show that the IIR filter can be well designed for the noise reduction process by using the ABC algorithm. Therefore, this method can improve the TCD interference signal, making it available for further analysis. Spectrogram indicators for clinical application can be accurately obtained from the maximum frequency curve The structure of the adaptive noise cancellation system is shown in Figure 4(a) [53,109,110]. In 2013, Koza et al. introduced a filter design method to eliminate additive white Gaussian noise through an infinite impulse response digital filter using the Harmony Search (HS) algorithm. To optimize the design, additive white Gaussian noise with different signal-to-noise ratios (-4dB, +4dB, -2dB and +2dB) was added to the mitral TCD signal. Four different infinite impulse response digital filters are designed under different noise levels using the HS algorithm, and their performances are compared. On this basis, a fourth degree IIR filter designed by the ABC algorithm is used to filter the noise-added signal. Using the SSA algorithm to analyze the noise of the TCD signals and discover new TCD parameters for diagnosis [54,111]. TCD signals are easily contaminated by noise in the environment, which can lead to some errors in the diagnosis process. Effective noise removal is necessary to ensure the authenticity of the TCD ultrasonography signals and reduce errors in the diagnosis and treatment of acute cerebrovascular diseases.
A significant limitation of using TCD ultrasound is that it is time-consuming and highly operator dependent. Examination of patients using hand-held technology requires the examiner to have a detailed understanding of cerebral vascular anatomy and its variations, as well as ultrasound technology and various TCD indicators of vasospasm [33]. Computer-aided diagnosis systems have become valuable tools for accurate, reliable, and rapid diagnosis of vascular diseases. The application of AIM has been widely reported in previous studies. Esmaeeli et al. propose that a robot assisted TCD system with AI provides an alternative to manual TCD to assess blood flow velocity within the MCA in acute SAH patients, thereby extending the availability of TCD to settings without specialized clinicians. Recently, a robot-assisted ultrasound system integrating ultrasound, robotics, and machine learning has been proposed, which combines TCD with a square earphone containing a robotic rod that uses machine learning to automatically adjust the ultrasound probe to detect intracranial vasculature. The system can also readjust the probe position after the patient moved to continue sonicating previously acquired positions. In addition, the feasibility and accuracy of robotic-assisted TCD for measuring cerebral blood flow velocities in the anterior and MCA in patients with SAH and vasospasm were examined [57]. In 2022, Clare et al. to test the safety and efficacy of the Nova Guide system in patients with acute SAH in the neurological ICU. The Nova Guide system-five degrees of freedom robotic TCD system is shown in Figure 4(b) [112]. Baig et al. report the use of a robotic TCD system with AI as a novel real-time intraoperative neuromonitoring tool in patients undergoing valve replacement. By reporting the quantity and distribution of high-intensity transient signal (HITS) as a marker of micro embolism during surgery, they propose that the use of sentinel brain protection significantly reduces HITS [56]. In the process of TCD ultrasonography, the operator will be affected by the state of his own body, leading to unnecessary errors in the operation. The intelligent robot will not be affected by these factors, and can accurately complete the TCD ultrasonography, greatly improving the reliability of TCD ultrasonography.
In 2021, Wang et al. designed an AI neighborhood segmentation algorithm based on the characteristics of TCD ultrasound images of patients with traumatic brain injury to detect neuroendocrine changes in the acute phase of patients with severe traumatic brain injury. Their findings showed that the AI neighborhood segmentation algorithm had significantly lower regional segmentation error and runtime for TCD ultrasonography images, and significantly improved concordance between the Glasgow Coma Scale and pituitary hormone abnormalities. However, there are still some shortcomings. For example, the types of neuroendocrine monitoring hormones included in this study are relatively single, mainly focusing on pituitary hormones, and lack of monitoring indicators for other neuroendocrine hormones. The AI neighborhood segmentation algorithm they researched and designed uses relatively few monitoring indicators in image segmentation quality evaluation. In the future, more algorithm evaluation indicators will be included in the further optimization of the algorithm, and then the value of the algorithm will be comprehensively analyzed from multiple perspectives [113]. Mei et al. explored the diagnostic value of convolutional neural network (CNN) in MAC stenosis by analyzing TCD ultrasonography images. According to the results of cerebral angiography, 278 patients who underwent cerebral vascular TCD and cerebral angiography were divided into stenosis group and non-stenosis group. Manual measurements were made on TCD images. The patients were divided into training and test sets, and the TCD images were classified using a CNN architecture. The diagnostic accuracy of manual measurements of MCA stenosis, CNN and TCD parameters were calculated and compared. The diagnostic value of CNN based on TCD images for MCA stenosis was like manual measurements. The results show that CNN can be used as an auxiliary diagnostic tool to improve the diagnosis of MCA stenosis [55]. The training process is shown in Figure 4(c).
TCD ultrasonography has shown great advantages in the treatment of cerebrovascular diseases, but there are still some key issues that need to be addressed to make it more accurate in determining the type of disease. This will certainly lead to further development of TCD ultrasonography. Scientists have not stopped exploring this field, and the future of TCD ultrasonography has great potential for development.
In clinical, cerebrovascular disease usually manifests itself in people who experience acute nonfatal events (i.e., emergency department visit, hospitalization) or fatal events. It is one of the causes of severe human disability and is also the second leading cause of death worldwide. Therefore, timely prediction and diagnosis of cerebrovascular disease can enable timely early treatment [2,114,115]. TCD is a relatively inexpensive, rapid, real-time, noninvasive measure of cerebrovascular blood flow and is the most convenient method for detecting vascular changes during the treatment of acute clinical cerebrovascular events in response to interventions. It has shown great potential in the diagnosis and treatment of cerebrovascular diseases [39,40].AI has demonstrated great advantages in several fields of society since its introduction. It has also brought various important technological tools to the medical field [45,46,47,48]. Meanwhile, in the field of emergency medicine, AI has shown great advantages in pre-hospital emergency management, patient triage and disposal, medical disease and condition prediction, and emergency department management [46,48,97,98]. Until now, there have been many studies on the use of AI for TCD ultrasonography. These include the combination of TCD ultrasonography and BCI [49,50,99,100,101,102], the classification and noise cancellation of TCD signals using AI [51,52,53,54,104,105,106,107,108,109,110,111], and the use of intelligent robots and computerized diagnostic systems to assist physicians in disease diagnosis and treatment [33,55,56,57,112,113]. The development of AI has improved the accuracy of TCD ultrasonography in several ways and greatly promoted the diagnosis of TCD in acute cerebrovascular disease development. The birth of AI has given new impetus to the development of medicine. TCD ultrasonography has more advantages than other diagnostic and treatment methods. Therefore, the combination of AI with it will further promote the application and development of TCD ultrasonography.
TCD ultrasonography has made rapid development after it was proposed, and it has shown great advantages in the diagnosis of acute cerebrovascular diseases. However, the detection process requires the operator to have extremely high proficiency, and the influence of some external factors will reduce the accuracy of the detection results. With the development of AI, people are committed to using AI methods to improve the accuracy of TCD ultrasonography, including AI based TCD signals classification, TCD signals noise removal, and intelligent robots to assist doctors in diagnosis and treatment. With the further development of AI, TCD ultrasonography also has more potential for development.
The authors declare there is no conflict of interest.
[1] | A. D. Singh, M. E. Turell, A. K. Topham, Uveal melanoma: trends in incidence, treatment, and survival, Ophthalmology, 118 (2011), 1881-1885. |
[2] |
M. H. Abdel-Rahman, K. M. Sample, R. Pilarski, T. Qalsh, T. Grosel, D. Kinnamon, et al., Whole exome sequencing identifies candidate genes associated with hereditary predisposition to uveal melanoma, Ophthalmology, 127 (2020), 668-678. doi: 10.1016/j.ophtha.2019.11.009
![]() |
[3] |
M. W. Wilson, J. L. Hungerford, Comparison of episcleral plaque and proton beam radiation therapy for the treatment of choroidal melanoma, Ophthalmology, 106 (1999), 1579-1587. doi: 10.1016/S0161-6420(99)90456-6
![]() |
[4] |
B. Damato, S. E. Coupland, An audit of conjunctival melanoma treatment in liverpool, Eye, 23 (2009), 801-809. doi: 10.1038/eye.2008.154
![]() |
[5] | S. Tuomaala, S. Eskelin, A. Tarkkanen, T. Kivela, Population-based assessment of clinical characteristics predicting outcome of conjunctival melanoma in whites, Invest. Ophth. Vis. Sci., 43 (2002), 3399-3408. |
[6] |
P. Rietschel, K. S. Panageas, C. Hanlon, A. Patel, D. H. Abramson, P. B. Chapman, Variates of survival in metastatic uveal melanoma, J. Clin. Oncol., 23 (2005), 8076-8080. doi: 10.1200/JCO.2005.02.6534
![]() |
[7] |
M. V. Heppt, T. Steeb, J. G. Schlager, S. Rosumeck, C. Dressler, T. Ruzicka, et al., Immune checkpoint blockade for unresectable or metastatic uveal melanoma: A systematic review, Cancer Treat. Rev., 60 (2017), 44-52. doi: 10.1016/j.ctrv.2017.08.009
![]() |
[8] |
M. G. Vander Heiden, R. J. DeBerardinis, Understanding the intersections between metabolism and cancer biology, Cell, 168 (2017), 657-669. doi: 10.1016/j.cell.2016.12.039
![]() |
[9] | S. Katada, A. Imhof, P. Sassone-Corsi, Connecting threads: epigenetics and metabolism, Cell, 148 (2012), 24-28. |
[10] |
O. Warburg, On the origin of cancer cells, Sci., 123 (1956), 309-314. doi: 10.1126/science.123.3191.309
![]() |
[11] |
C. Chattopadhyay, J. Oba, J. Roszik, J. R. Marszalek, K. Chen, Y. Qi, et al., Elevated endogenous SDHA drives pathological metabolism in highly metastatic uveal melanoma, Invest. Ophth. Vis. Sci., 60 (2019), 4187-4195. doi: 10.1167/iovs.19-28082
![]() |
[12] | S. Vardanyan, A. Brosig, H. Merz, M. Ranjbar, V. Kakkassery, S. Grisanti, et al., Metastasis of uveal melanoma with monosomy-3 is associated with a less glycogenetic gene expression profile and the dysregulation of glycogen storage, Cancers, 12 (2020), 2101. |
[13] | K. Slater, A. B. Heeran, S. Garcia-Mulero, H. Kalirai, R. Sanz-Pamplona, A. Rahman, et al., High cysteinyl leukotriene receptor 1 expression correlates with poor survival of uveal melanoma patients and cognate antagonist drugs modulate the growth, cancer secretome, and metabolism of uveal melanoma cells, Cancers, 12 (2020), 2950. |
[14] | L. B. Yan, K. Shi, Z. T. Bing, Y. L. Sun, Y. Shen, Proteomic analysis of energy metabolism and signal transduction in irradiated melanoma cells, Int. J. Ophthalmol., 6 (2013), 286-294. |
[15] | D. Szklarczyk, A. L. Gable, D. Lyon, A. Junge, S. Wyder, J. Huerta-Cepas, et al., String v11: protein-protein association networks with increased coverage, supporting functional discovery in genome-wide experimental datasets, Nucleic Acids Res., 47 (2019), D607-d613. |
[16] |
R. Tibshirani, The lasso method for variable selection in the Cox model, Stat. Med., 16 (1997), 385-395. doi: 10.1002/(SICI)1097-0258(19970228)16:4<385::AID-SIM380>3.0.CO;2-3
![]() |
[17] | J. Friedman, T. Hastie, R. Tibshirani, Regularization paths for generalized linear models via coordinate descent, J. Stat. Softw., 33 (2010), 1-22. |
[18] |
C. L. Dieck, A. Ferrando, Genetics and mechanisms of NT5C2-driven chemotherapy resistance in relapsed ALL, Blood, 133 (2019), 2263-2268. doi: 10.1182/blood-2019-01-852392
![]() |
[19] |
F. Cividini, E. Cros-Perrial, R. Pesi, C. Machon, S. Allegrini, M. Camici, et al., Cell proliferation and drug sensitivity of human glioblastoma cells are altered by the stable modulation of cytosolic 5'-nucleotidase Ⅱ, Int. J. Biochem. Cell Biol., 65 (2015), 222-229. doi: 10.1016/j.biocel.2015.06.011
![]() |
[20] |
A. D. Lietzan, M. Maurice, Functionally diverse biotin-dependent enzymes with oxaloacetate decarboxylase activity, Arch. Biochem. Biophys., 544 (2014), 75-86. doi: 10.1016/j.abb.2013.10.014
![]() |
[21] |
K. Sellers, M. P. Fox, M. Bousamra, S. P. Slone, R. M. Higashi, D. M. Miller, et al., Pyruvate carboxylase is critical for non-small-cell lung cancer proliferation, J. Clin. Invest., 125 (2015), 687-698. doi: 10.1172/JCI72873
![]() |
[22] | T. Delgado-Goñ i, T. C. Galobart, S. Wantuch, D. Normantaite, M. O. Leach, S. R. Whittaker, et al, Increased inflammatory lipid metabolism and anaplerotic mitochondrial activation follow acquired resistance to vemurafenib in BRAF-mutant melanoma cells, Brit. J. Cancer, 122 (2020), 72-81. |
[23] |
T. Delgado-Goni, M. F. Miniotis, S. Wantuch, H. G. Parkes, R. Marais, P. Workman, et al. The BRAF inhibitor vemurafenib activates mitochondrial metabolism and inhibits hyperpolarized pyruvate-lactate exchange in BRAF-mutant human melanoma cells, Mol. Cancer Ther., 15 (2016), 2987-2999. doi: 10.1158/1535-7163.MCT-16-0068
![]() |
[24] | P. Minárik, N. Tomásková, M. Kollárová, M. Antalik, Malate dehydrogenases-structure and function, Gen. Physiol. Biophys., 21 (2002), 257-265. |
[25] |
Y. Zhuang, J. Xiang, W. Bao, Y. Sun, L. Wang, M. Tan, et al., MDH2 stimulated by estrogen-GPR30 pathway down-regulated pten expression promoting the proliferation and invasion of cells in endometrial cancer, Transl. Oncol., 10 (2017), 203-210. doi: 10.1016/j.tranon.2017.01.009
![]() |
[26] |
R. Naik, H. S. Ban, K. Jang, I. Kim, X. Xu, D. Harmalkar, et al., Methyl 3-(3-(4-(2, 4, 4-Trimethylpentan-2-yl)phenoxy)-propanamido)benzoate as a novel and dual malate dehydrogenase (MDH) 1/2 inhibitor targeting cancer metabolism, J Med. Chem., 60 (2017), 8631-8646. doi: 10.1021/acs.jmedchem.7b01231
![]() |
[27] |
K. Kato, H. Nishimasu, S. Okudaira, E. Mihara, R. Ishitani, J. Takagi, et al., Crystal structure of Enpp1, an extracellular glycoprotein involved in bone mineralization and insulin signaling, P. Natl. Acad. Sci. USA, 109 (2012), 16876-16881. doi: 10.1073/pnas.1208017109
![]() |
[28] | M. Hu, W. Guo, Y. Liao, D. Xu, B. Sun, H. Song, et al., Dysregulated ENPP1 increases the malignancy of human lung cancer by inducing epithelial-mesenchymal transition phenotypes and stem cell features, Am. J. Cancer Res., 9 (2019), 134-144. |
[29] |
M. Kawaguchi, X. Han, T. Hisada, S. Nishikawa, K. Kano, N. Ieda, et al., Development of an ENPP1 fluorescence probe for inhibitor screening, cellular imaging, and prognostic assessment of malignant breast cancer, J. Med. Chem., 62 (2019), 9254-9269. doi: 10.1021/acs.jmedchem.9b01213
![]() |
[30] |
S. Qu, J. Long, Q. Cai, X. O. Shu, H. Cai, Y. T. Gao, et al., Genetic polymorphisms of metastasis suppressor gene NME1 and breast cancer survival, Clin. Cancer Res., 14 (2008), 4787-4793. doi: 10.1158/1078-0432.CCR-08-0083
![]() |
[31] |
N. Pamidimukkala, G. S. Puts, M. K. Leonard, D. Snyder, S. Dabernat, E. C. De Fabo, et al., Nme1 and Nme2 genes exert metastasis-suppressor activities in a genetically engineered mouse model of UV-induced melanoma, Brit. J. Cancer, 124 (2021), 161-165. doi: 10.1038/s41416-020-01096-w
![]() |
[32] |
Y. Wang, M. K. Leonard, D. E. Snyder, M. L. Fisher, R. L. Eckert, D. M. Kaetzel, NME1 Drives Expansion of Melanoma Cells with Enhanced Tumor Growth and Metastatic Properties, Mol. Cancer Res., 17 (2019), 1665-1674. doi: 10.1158/1541-7786.MCR-18-0019
![]() |
[33] |
A. J. Freemerman, A. R. Johnson, G. N. Sacks, J. J. Milner, E. L. Kirk, M. A. Troester, et al., Metabolic reprogramming of macrophages: glucose transporter 1 (GLUT1)-mediated glucose metabolism drives a proinflammatory phenotype, J. Biol. Chem., 289 (2014), 7884-7896. doi: 10.1074/jbc.M113.522037
![]() |
[34] |
T. Cascone, J. A. McKenzie, R. M. Mbofung, S. Punt, Z. Wang, C. Xu, et al., Increased tumor glycolysis characterizes immune resistance to adoptive T cell therapy, Cell Metab., 27 (2018), 977-987. doi: 10.1016/j.cmet.2018.02.024
![]() |
[35] |
S. R. Jacobs, C. E. Herman, N. J. MacIver, J. A. Wofford, H. L. Wieman, J. J. Hammen, et al., Glucose uptake is limiting in T cell activation and requires CD28-mediated Akt-dependent and independent pathways, J. Immunol., 180 (2008), 4476-4486. doi: 10.4049/jimmunol.180.7.4476
![]() |
[36] | R. Samaniego, A. Gutiérrez-González, A. Gutiérrez-Seijo, S. Sánchez-Gregorio, J. García-Giménez, E. Mercader, et al., CCL20 expression by tumor-associated macrophages predicts progression of human primary cutaneous melanoma, Cancer Immunol. Res., 6 (2018), 267-275. |
[37] | H. Bagheri, M. H. Pourhanifeh, M. Derakhshan, M. Mahjoubin-Tehran, F. Ghasemi, S. Mousavi, et al, CXCL-10: a new candidate for melanoma therapy?, Cell. Oncol., 43 (2020), 353-365. |
[38] |
Z. S. Chheda, R. K. Sharma, V. R. Jala, A. D. Luster, B. Haribabu, Chemoattractant receptors BLT1 and CXCR3 regulate antitumor immunity by facilitating CD8+ t cell migration into tumors, J. Immunol., 197 (2016), 2016-2026. doi: 10.4049/jimmunol.1502376
![]() |
[39] |
R. R. Ji, S. D. Chasalow, L. Wang, O. Hamid, H. Schmidt, J. Cogswell, et al., An immune-active tumor microenvironment favors clinical response to ipilimumab, Cancer Immunol. Immun., 61 (2012), 1019-1031. doi: 10.1007/s00262-011-1172-6
![]() |
[40] |
D. Bedognetti, T. L. Spivey, Y. Zhao, L. Uccellini, S. Tomei, M. E. Dudley, et al., CXCR3/CCR5 pathways in metastatic melanoma patients treated with adoptive therapy and interleukin-2, Brit. J. Cancer, 109 (2013), 2412-2423. doi: 10.1038/bjc.2013.557
![]() |
![]() |
![]() |
1. | Dario Mazzoleni, Benedetta Pellacci, Calculus of variations and nonlinear analysis: advances and applications, 2023, 5, 2640-3501, 1, 10.3934/mine.2023059 | |
2. | Delio Mugnolo, Marvin Plümer, On torsional rigidity and ground-state energy of compact quantum graphs, 2023, 62, 0944-2669, 10.1007/s00526-022-02363-9 |