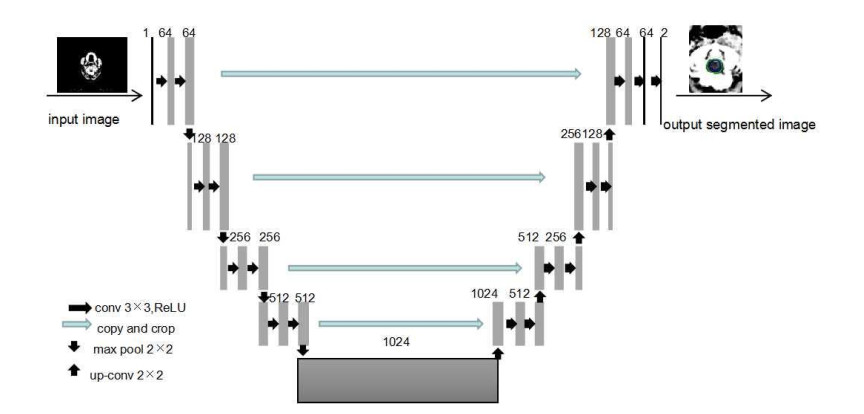
To evaluate the automatic segmentation approach for organ at risk (OARs) and compare the parameters of dose volume histogram (DVH) in radiotherapy. Methodology: Thirty-three patients were selected to contour OARs using automatic segmentation approach which based on U-Net, applying them to a number of the nasopharyngeal carcinoma (NPC), breast, and rectal cancer respectively. The automatic contours were transferred to the Pinnacle System to evaluate contour accuracy and compare the DVH parameters.
The time for manual contour was 56.5 ± 9, 23.12 ± 4.23 and 45.23 ± 2.39min for the OARs of NPC, breast and rectal cancer, and for automatic contour was 1.5 ± 0.23, 1.45 ± 0.78 and 1.8 ± 0.56 min. Automatic contours of Eye with the best Dice-similarity coefficients (DSC) of 0.907 ± 0.02 while with the poorest DSC of 0.459 ± 0.112 of Spinal Cord for NPC; And Lung with the best DSC of 0.944 ± 0.03 while with the poorest DSC of 0.709 ± 0.1 of Spinal Cord for breast; And Bladder with the best DSC of 0.91 ± 0.04 while with the poorest DSC of 0.43 ± 0.1 of Femoral heads for rectal cancer. The contours of Spinal Cord in H & N had poor results due to the division of the medulla oblongata. The contours of Femoral head, which different from what we expect, also due to manual contour result in poor DSC.
The automatic contour approach based deep learning method with sufficient accuracy for research purposes. However, the value of DSC does not fully reflect the accuracy of dose distribution, but can cause dose changes due to the changes in the OARs volume and DSC from the data. Considering the significantly time-saving and good performance in partial OARs, the automatic contouring also plays a supervisory role.
Citation: Han Zhou, Yikun Li, Ying Gu, Zetian Shen, Xixu Zhu, Yun Ge. A deep learning based automatic segmentation approach for anatomical structures in intensity modulation radiotherapy[J]. Mathematical Biosciences and Engineering, 2021, 18(6): 7506-7524. doi: 10.3934/mbe.2021371
[1] | Jinke Wang, Xiangyang Zhang, Liang Guo, Changfa Shi, Shinichi Tamura . Multi-scale attention and deep supervision-based 3D UNet for automatic liver segmentation from CT. Mathematical Biosciences and Engineering, 2023, 20(1): 1297-1316. doi: 10.3934/mbe.2023059 |
[2] | Frédéric Gibou, Doron Levy, Carlos Cárdenas, Pingyu Liu, Arthur Boyer . Partial Differential Equations-Based Segmentation for Radiotherapy Treatment Planning. Mathematical Biosciences and Engineering, 2005, 2(2): 209-226. doi: 10.3934/mbe.2005.2.209 |
[3] | Krzysztof Fujarewicz, Krzysztof Łakomiec . Adjoint sensitivity analysis of a tumor growth model and its application to spatiotemporal radiotherapy optimization. Mathematical Biosciences and Engineering, 2016, 13(6): 1131-1142. doi: 10.3934/mbe.2016034 |
[4] | Xiaoli Zhang, Kunmeng Liu, Kuixing Zhang, Xiang Li, Zhaocai Sun, Benzheng Wei . SAMS-Net: Fusion of attention mechanism and multi-scale features network for tumor infiltrating lymphocytes segmentation. Mathematical Biosciences and Engineering, 2023, 20(2): 2964-2979. doi: 10.3934/mbe.2023140 |
[5] | Yunling Liu, Yaxiong Liu, Jingsong Li, Yaoxing Chen, Fengjuan Xu, Yifa Xu, Jing Cao, Yuntao Ma . ECA-TFUnet: A U-shaped CNN-Transformer network with efficient channel attention for organ segmentation in anatomical sectional images of canines. Mathematical Biosciences and Engineering, 2023, 20(10): 18650-18669. doi: 10.3934/mbe.2023827 |
[6] | Yantao Song, Wenjie Zhang, Yue Zhang . A novel lightweight deep learning approach for simultaneous optic cup and optic disc segmentation in glaucoma detection. Mathematical Biosciences and Engineering, 2024, 21(4): 5092-5117. doi: 10.3934/mbe.2024225 |
[7] | Hong'an Li, Man Liu, Jiangwen Fan, Qingfang Liu . Biomedical image segmentation algorithm based on dense atrous convolution. Mathematical Biosciences and Engineering, 2024, 21(3): 4351-4369. doi: 10.3934/mbe.2024192 |
[8] | M Nisha, T Kannan, K Sivasankari . A semi-supervised deep neuro-fuzzy iterative learning system for automatic segmentation of hippocampus brain MRI. Mathematical Biosciences and Engineering, 2024, 21(12): 7830-7853. doi: 10.3934/mbe.2024344 |
[9] | Yuanhong Jiang, Yiqing Shen, Yuguang Wang, Qiaoqiao Ding . Automatic recognition of white blood cell images with memory efficient superpixel metric GNN: SMGNN. Mathematical Biosciences and Engineering, 2024, 21(2): 2163-2188. doi: 10.3934/mbe.2024095 |
[10] | Yu Li, Meilong Zhu, Guangmin Sun, Jiayang Chen, Xiaorong Zhu, Jinkui Yang . Weakly supervised training for eye fundus lesion segmentation in patients with diabetic retinopathy. Mathematical Biosciences and Engineering, 2022, 19(5): 5293-5311. doi: 10.3934/mbe.2022248 |
To evaluate the automatic segmentation approach for organ at risk (OARs) and compare the parameters of dose volume histogram (DVH) in radiotherapy. Methodology: Thirty-three patients were selected to contour OARs using automatic segmentation approach which based on U-Net, applying them to a number of the nasopharyngeal carcinoma (NPC), breast, and rectal cancer respectively. The automatic contours were transferred to the Pinnacle System to evaluate contour accuracy and compare the DVH parameters.
The time for manual contour was 56.5 ± 9, 23.12 ± 4.23 and 45.23 ± 2.39min for the OARs of NPC, breast and rectal cancer, and for automatic contour was 1.5 ± 0.23, 1.45 ± 0.78 and 1.8 ± 0.56 min. Automatic contours of Eye with the best Dice-similarity coefficients (DSC) of 0.907 ± 0.02 while with the poorest DSC of 0.459 ± 0.112 of Spinal Cord for NPC; And Lung with the best DSC of 0.944 ± 0.03 while with the poorest DSC of 0.709 ± 0.1 of Spinal Cord for breast; And Bladder with the best DSC of 0.91 ± 0.04 while with the poorest DSC of 0.43 ± 0.1 of Femoral heads for rectal cancer. The contours of Spinal Cord in H & N had poor results due to the division of the medulla oblongata. The contours of Femoral head, which different from what we expect, also due to manual contour result in poor DSC.
The automatic contour approach based deep learning method with sufficient accuracy for research purposes. However, the value of DSC does not fully reflect the accuracy of dose distribution, but can cause dose changes due to the changes in the OARs volume and DSC from the data. Considering the significantly time-saving and good performance in partial OARs, the automatic contouring also plays a supervisory role.
Patients with intensity-modulated radiotherapy need to go through several steps, including positioning, target contour, planning, plan design, plan verification, plan execution, etc. The contour of target volumes is one of the key steps in the preparation of a radiotherapy treatment plan of intensity modulated radiotherapy [1,2]. Nevertheless, manual contour is time taking process and conditional on intra- and inter-observer differences although established on standard guidelines. Multiple studies have shown that the contouring consensus among different oncologists at various hospitals is poor, to solve this radiotherapeutic problem, multiple studies have focused on automatic contouring [3,4].
With the improvement in the speed of computer calculations and the improvement of the images processing makes it possible to develop approach for delineation automatically for radiation therapy. In recent years, automatic segment has become one of the most popular research in the field of radiotherapy, atlas and deep learning based automatic contouring methods are the two mainly technologies at present. As early as 2009, Mahdavi et al. [5] reported and characterized a semi-automatic prostate segmentation method for prostate brachytherapy.
Coupled with the large number of patients in radiotherapy, the research can acquire much more the learning data. In radiation therapy automated methods for sub-volume oriented dose-escalation plans can assist medical examination [6,7], the precise contour can reduce the toxic effect of normal tissues and benefit patients with longer survival periods such as nasopharyngeal carcinoma (NPC), breast and rectal cancer patients.
The objective of this study is to investigate the automatic techniques into NPC, breast and rectal cancer patients radiotherapy planning practice through automatic segmentation approach, which based on the deep learning method. The manual contours were acted as a reference for the study.
Thirteen NPC, ten breast cancer and ten rectal cancer patients, who received IMRT between Oct 1st 2019 to Oct 1st 2020 and underwent contrast-enhanced planning computed tomography (CT), were selected for this study. Written informed consent was obtained from each patient, and all studies involving people, medical records were approved by the Clinical Research Ethics Committee.
The organs at risk (OARs) were contoured by one radiation oncologist who has more than 10 years' experience in the field of contouring (e.g., NPC was contoured by a H & N oncologist, etc.) according to the recommendations of the European Society of Therapeutic Radiation Oncology Guidelines on planning CT. The same radiotherapist contoured OARs for three sequences on Multiplan2.4.1 system (Accuray Inc, USA), the OARs in NPC mainly contain: Lens, Optic-Nerves, Parotids, BrainStem and Spinal Cord; Lung, Heart and Spinal Cord for breast cancer while Bladder and Femoral heads for rectal cancer [8].
The OARs were automatically contoured on the same images using automatic segment approach. The approach based on deep learning and traditional algorithm model, which divided into three parts: data collection, annotation, model training and prediction [9]. The module builds loss function pool, image segmentation model pool and selectable parameter pool after obtaining training set data. The training model is constructed by randomly selecting the corresponding loss function, image segmentation model and training parameter value based on the selectable parameter pool, and the training model is trained based on the training set data to obtain the trained training model [10]. Then, the DSC value of each trained training model is calculated on the corresponding verification set. Finally, the corresponding training model with the highest DSC value is selected as the final OAR automatic.
The default network in deep learning network is an adaptive structure strategy similar to 2D-UNet [11], it contains 5 maximum pooling layers and 5 upper adoption layers, The basic convolutional core channels are 32, size 3 × 3, Behind each convolutional layer are batch normalized (Batch Norm) layers and a linear rectifier function (ReLU), the jump connection is cascaded between the encoder and the decoder, combine high-resolution details and deep image semantic information to improve the effect of sketching the model. The network architecture is shown in Figure 1.
Furthermore, the loss function is set to Dice Loss, learning rate to 3e-4, optimizer set to Adam, Batch Size to 16 and the total number of iterations is 100 epochs.
DiceLoss=1−2|A∩B||A|+|B| |
There are two evaluation methods in approach: cross-validation and training/test separation validation. The first method divides the dataset into the same number of k subsets. Use k-1 subsets for training each time and the other as a test set. Repeat the training for k times and evaluate the results. The second method divides the dataset into two parts for training and testing, which applies to those with a large number of cases. For small dataset, we selected k = 5 for cross-validation while with big dataset we chose 80% for the training and 20% for the test.
For evaluation, we adopted two quantitative evaluation metrics while the manual contouring served as the reference standard for contouring time and DVH parameters comparisons[12].
The quality of the automatic is critical, numerous measures are utilized to compare the volumes between automatic and manual contouring including Time, Dice Similarity Cofficient (DSC), Hausdorff Distances (HD), Jaccard Distance (JD) and DVH parameters [13].
DSC(A,B)=2(A∩B)/(A+B) | (1) |
A and B represented automatically contoured and manually contoured OARs, respectively. As the contours approach agreement, the DSC value approaches 1; as the volumes diverge into two nonoverlapping structures, the DSC value goes to 0. The calculation of DSC in commercially available registration systems is not standard, although its inclusion in commercial software is a recommendation of TG132. However many professional literature reported that the values of DSC > 0.700 shows optimum overlap of contours [14,15].
HD=max(h(A,B),h(B,A)) | (2) |
where h(A, B) is the fixed HD from A to B. where h(A, B) = max(min(||a-b||)),
JD=A∩BA∪B | (3) |
JD is used to describe the dissimilarity between sets. The larger the JD, the higher the sample similarity. Where A and B represented automatically contoured and manually contoured OARs, respectively.
The time needed by the approach to generate contours was measured by when the CT was imported until the end of the entire generation process.
Once the automatic completed, the automatic contours were sent back to the Pinnacle Treatment Planning System (TPS, version 9.8, Philips Radiation Oncology systems, Madison, WI). Then the planning dose based on manual contouring were copied to the automatically contours for planning parameters evaluation and comparison. The prescribe dose are 60Gy/30fx for NPC, 50Gy/25fx for breast cancer and 50Gy/25fx for rectal cancer.
We mainly use the max dose (Dmax), the Dose of the certain percentage of the volume (Dx), and the volume of receiving the certain dose of organs (Vx) for DVH Parameters. Figure 2 shows the whole workflow of the evaluation. Dose assessment of OARs reference RTOG0615 (Radiation Therapy Oncology Group, RTOG0615) [16].
All statistical comparison were performed using Origin2019. A comparison of the time required to contour and DVH parameters were performed using paired t test, with significance set at p < 0.05.
t=X1−X2−d0√S2(1N1+1N2) | (4) |
Here, X1, X2 represented automatically contoured and manually contoured. S2 represented the total variance, d0 represented the average difference between the two samples.
The time required to manually contour the OARs were 56.5 ± 9.0 minutes (mean ± standard deviation) for NPC, 23.12 ± 4.23min for breast cancer and 45.23 ± 2.39 min (mean ± standard deviation) for rectal cancer. And auto contours required 1.5 ± 0.23minutes, 1.45 ± 0.78 and 1.8±0.56 respectively (Table 1). The difference of requiring time savings of 70%, which were all highly significant (p < 0.0001).
Antomical | Manual Contouring Time(SD) | Automatic segment time(SD) | P |
NPC | 56.5± 9 | 1.5 ±0.23 | p < 0.0001 |
breast cancer | 23.12 ± 4.23 | 1.45 ±0.78 | p < 0.0001 |
rectal cancer | 45.23 ± 2.39 | 1.8±0.56 | p < 0.0001 |
Regarding the clinical demand and the clinical use of automatic software, we only selected the partial OARs for comparison. We just selected the Eyes, Lens, Optic path, BrainStem, Spinal Cord, Parotids for NPC, while selected Lung, Heart, Spinal Cord and Bladder, Femoral heads for breast and rectal cancer respectively.
Mean values and standard deviations of parameters that evaluate the contours generated by the automatic software, which use manual contours as a reference, for each organ of the NPC, breast and rectal cancer.
Tables 5, 8, 10 showed the OAR geometrical quantitative evaluation parameters of 33 patients between automatic contours with manual contours. Automatic contours overlapped with manual contours of Eye with the highest degree DSC of 0.907 ± 0.02 while with the poorest DSC of 0.459 ± 0.112 of Spinal Cord for NPC. Among the HD of OARs, the maximum HD was (23.0 ± 1.5) mm in Spinal Cord, and the minimum was (2.45 ± 0.31) mm in lens. The maximum JD in eye was 0.89 ± 0.03 while the minimum in Spinal Cord was 0.38 ± 0.2; And Lung with the highest degree DSC of 0.944 ± 0.03 while with the poorest DSC of 0.709 ± 0.1 of Spinal Cord for breast. Among the HD of Lung-L, Lung-R, Heart and Spinal Cord, the maximum HD was (20.7 ± 5.3) mm in Lung-L, and the minimum was (3.15 ± 0.8) mm in Spinal Cord. The JD in Lung and Heart were greater than or equal to 0.8 while that in Spinal Cord was 0.7 ± 0.1; And Bladder with the highest degree DSC of 0.91 ± 0.04 while with the poorest DSC of 0.43 ± 0.1 of Femoral heads for rectal cancer. Among the HD of Bladder and femurs, the maximum HD was 35.3 ± 6.9 in Femur, and the minimum was (2.87 ± 1.1) mm in Bladder. JD in Bladder was the greater than 0.9 while the mean value in Femur was 0.4. The contours achieved good contour level, however the Dose parameters were not similarity with the contours. Even if the contours reach the high level, the DVH Parameters of automatic contours had the significantly difference with the manual contours from Tables 2–4, 6, 7 and 9.
Organ at risks | Volume(cc) | D1(cGy) | P (VolumeManually VS VolumeAutomatic) | P (DoseManually VS DoseAutomatic) | |
Eye_R | Manually | 9.93±1.08 | 2551.23±701.2 | 0.02 | 0.002 |
Automatic | 10.45±1.06 | 2911.62±613.1 | |||
Eye_L | Manually | 9.73±0.99 | 2649.62±668.1 | 0.01 | 0.019 |
Automatic | 11.12±1.17 | 2921.08±616.9 | |||
Lens_R | Manually | 0.31±0.08 | 676.92±95.62 | 0.26 | 0.40 |
Automatic | 0.28±0.08 | 663.77±120.8 | |||
Lens_L | Manually | 0.31±0.1 | 674.77±89.56 | 0.81 | 0.66 |
Automatic | 0.31±0.07 | 680.92±118.41 | |||
Spinal Cord | Manually | 25.16±9.17 | 4127.3±330.81 | 0.000 | 0.000 |
Automatic | 10.66±3.46 | 5071.5±394.35 |
Organ at risks | Volume(cc) | Mean Dose | P (VolumeManually VS VolumeAutomatic) | P (DoseManually VS DoseAutomatic) | |
Parotid_L | Manually | 26.9±8.86 | 3426.23±174.19 | 0.017 | 0.001 |
Automatic | 30.2±7.27 | 3646.53±243.32 | |||
Parotid_R | Manually | 26.62±9.86 | 3485.31±280.21 | 0.01 | 0.01 |
Automatic | 29.2±7.85 | 3707.54±478.13 |
Organ at risks | Volume(cc) | D1 | D5 | D50 | P(VolumeManually VS VolumeAutomatic) | P(DoseManually VS DoseAutomatic) | |
OpticNerve_L | Manually | 0.66±0.37 | 5144.08±1186.04 | 4893.54±1132.75 | 3247.54±1150.39 | 0.35 | PD1=0.21; PD5=0.17; PD50=0.97 |
Automatic | 0.53±0.12 | 4943±1052.02 | 4665.08±1018.21 | 3252.46±978.06 | |||
OpticNerve_R | Manually | 0.68±0.4 | 4721.62±1740 | 4540.23±1703.0 | 3196.53±1490.77 | 0.086 | PD1=0.71; PD5=0.64; PD50=0.41 |
Automatic | 0.58±0.16 | 4613.08±1191 | 4405.92±1225.79 | 3099.7±1300.2 | |||
BrainStem | Manually | 29.43±3.32 | 5602.46±180.22 | 5039.31±264.88 | 3645.77±648.88 | 0.000 | PD1=0.13; PD5=0.04; PD50=0.04 |
Automatic | 23.21±2.37 | 5679.61±241.2 | 5105.92±287.77 | 3450.31±693.3 |
Organ at risks | DSC | HD(mm) | JD | |
Eye_R | Manually | 0.904±0.02 | 3.55±0.32 | 0.87±0.05 |
Automatic | ||||
Eye_L | Manually | 0.907±0.02 | 3.6±0.4 | 0.89±0.03 |
Automatic | ||||
Lens_R | Manually | 0.789±0.1 | 2.45±0.31 | 0.72±0.1 |
Automatic | ||||
Lens_L | Manually | 0.789±0.09 | 2.5±0.4 | 0.74±0.07 |
Automatic | ||||
Spinal Cord | Manually | 0.459±0.11 | 23.0±1.5 | 0.38±0.2 |
Automatic | ||||
Parotid_L | Manually | 0.819±0.05 | 17.5±2.1 | 0.75±0.02 |
Automatic | ||||
Parotid_R | Manually | 0.768±0.1 | 17.8±2.3 | 0.75±0.1 |
Automatic | ||||
OpticNerve_L | Manually | 0.652±0.1 | 11.5±1.1 | 0.63±0.2 |
Automatic | ||||
OpticNerve_R | Manually | 0.66±0.12 | 12.3±1.23 | 0.57±0.15 |
Automatic | ||||
BrainStem | Manually | 0.83±0.03 | 21.0±1.5 | 0.77±0.04 |
Automatic |
Organ at risks | Volume(cc) | ipsilateral Lung V5 | ipsilateral Lung V10 | ipsilateral Lung V20 | ipsilateral Lung V30 | ipsilateral Lung V40 | P(VolumeManually VS VolumeAutomatic) | P(VxManually VS VxAutomatic) | |
Lung_L | Manually | 1030.20±238.49 | 68.2±9.87 | 44.8±5.67 | 26.8±4.16 | 18.4±3.97 | 11.7±4 | 0.98 | PV5=0.20; PV10=0.052; PV20=1 PV30=0.59 PV40=0.467 |
Automatic | 1031.3±3625.63 | 66.6±10.7 | 44.1±5.63 | 26.8±3.82 | 18.6±3.56 | 12±3.68 | |||
Lung_R | Manually | 1248.57±273.46 | 0.07 | ||||||
Automatic | 1301±248.54 |
Organ at risks | Volume(cc) | D1 | V30 | V40 | D33 | D67 | P(VolumeManually VS VolumeAutomatic) | P(DoseManually VS DoseAutomatic) | |
Heart | Manually | 677.43±99.59 | 4113.7±1705.38 | 4.5±4.35 | 2.5±2.79 | 897.4±164.9 | 446.8±124.83 | 0.087 | PD1=0.70; PV30=0.16; PV40=0.34; PD33=0.33; PD67=0.33; |
Automatic | 623.24±97.57 | 4183.3±1415.7 | 4.7±4.39 | 2.6±2.91 | 852.1±82.08 | 450.4±128.75 | |||
Spinal Cord | Manually | 48.43±14.85 | 2518.2±1651.02 | 0.15 | PD1=0.21; | ||||
Automatic | 41.37±6 | 2358.5±1629.04 |
Organ at risks | DSC | HD(mm) | JD | |
Lung_L | Manually | 0.944±0.03 | 20.7±5.3 | 0.93±0.02 |
Automatic | ||||
Lung_R | Manually | 0.944±0.03 | 20.31±3.85 | 0.83±0.17 |
Automatic | ||||
Heart | Manually | 0.858±0.15 | 16.6±5.4 | 0.83±0.16 |
Automatic | ||||
Spinal Cord | Manually | 0.709±0.1 | 3.15±0.8 | 0.7±0.1 |
Automatic |
Organ at risks | Volume(cc) | D1 | D5 | D15 | D25 | D35 | D45 | Mean | P(VManually VS VAutomatic) | P(DManually VS DAutomatic) | |
Bladder | Manually | 427.89±131.2 | 5235.8±164.5 | 4908±155.8 | 4760±156.44 | 4619.85±158.8 | 4379.1±222.37 | 4039.9±337.7 | 3766.25±252.9 | 0.06 | PD1=0.16; PD5=0.43; PD15=0.34; PD25=0.059; PD35=0.052; PD45=0.18; PMean=0.74; |
Automatic | 394.32±130.5 | 5026±174.15 | 4902.6±157.04 | 4751.9±158.67 | 4598.1±176.88 | 4357.8±224.14 | 4013.3±331.6 | 3762.6±255.3 | |||
Femur-L | Manually | 65.03±28 | 3833.7±203.4 | 4145.1±282.68 | 3678±271 | 3375.1±238.13 | 3112±253 | 2867.5±279.6 | 2819.1±243.2 | 0.000 | PD1=0.70; PD5=0.000; PD15=0.000; PD25=0.000; PD35=0.000; PD45=0.000; PMean=0.000; |
Automatic | 134.8±36 | 4470.9±257.78 | 3341.7±254.56 | 2761.5±335.85 | 2341±311.4 | 2095.1±271.3 | 1922±267 | 1820.9±268.3 | |||
Femur-R | Manually | 70.8±34.04 | 4479.6±312 | 4159.1±316.5 | 3640.6±258 | 3329.7±217.7 | 3061.1±254.2 | 2772.08±363.1 | 2792.2±260.45 | 0.000 | PD1=0.000; PD5=0.000; PD15=0.000; PD25=0.000; PD35=0.000; PD45=0.000; PMean=0.000; |
Automatic | 132.42±34.3 | 3802.3±300 | 3335.6±284.3 | 2804.2±357.5 | 2429.9±304.85 | 2122±222 | 1910.7±195.76 | 1812.5±18707 |
Organ at risks | DSC | HD(mm) | JD | |
Bladder | Manually | 0.91±0.04 | 2.87±1.1 | 0.9±0.2 |
Automatic | ||||
Femur-L | Manually | 0.43±0.1 | 35.2±7.2 | 0.4±0.08 |
Automatic | ||||
Femur-R | Manually | 0.44±0.11 | 35.3±6.9 | 0.4±0.1 |
Automatic |
We generated dose volume histograms dosimetric parameters for each patient from the automatic contours and the reference contours. Examples of these dosimetric endpoints include the mean dose (Dmean), the minimum dose to the hottest x% of a structure (Dx). The full list of parameters investigated in this study is given in Tables 2 to 9. Studies of Tables gives qualitative representation of the best match contours in our cohort, where the Eyes had best geometric overlap, yet the doses had significantly difference with the manual contours.
Medical image segmentation using deep learning methods has been widely studied at present. All deep learning algorithms are designed to improve the accuracy of automatic segmentation [17,18]. The classical deep learning techniques are mostly based on convolutional neural network (CNN), which can be trained on patched that belong or not the segmentation class [19]. A problem with this approach is that the patches may overlap with the same convolution calculated multiple times. A series of image segmentation models are derived from the CNN, but the improved accuracy depends on an increase in the number of network layers which aggravates the over-fitting problem [20]. In addition, the output image size is far less than the input, and cannot meet the end-to-end medical image segmentation needs. The U-net [21] is based on two-dimensional convolutions on slices of volumetric medical data, it consists of two stages, one contracting (reduction in image size) and one expanding (restoration of the original image size). The approach used in the paper is a flexible deep learning platform and modular back-end architecture which embedded U-net. The approach backend consists of four components, including a data manager, model trainer, model evaluation, and model deployment. The model has been extensively tested previously. Christ et al. [22] segments the liver, U-Net1 segments the liver from the input image and then enters the results into U-Net2 for segmenting the liver tissue, U-Net2 also segments the liver tissue directly from the input image. Two U-Net form complex connections in this way, forming cascading fully convolutional neural networks, achieving results with both liver and lesion segmentation DSC values higher than 94%. By connecting the deep learning network in string and parallel, the network depth and the segmentation specificity are increased.
In our research, we used the clinical automatic segment approach for contouring OARs in NPC, breast and rectal cancer. The automatic system will be widely application in clinical radiotherapy while manual contouring proved to time-consume. From Table 1, the deep-learning automatic contouring approach, allowed significantly time-saving work for the radiation oncologists. And many methods exist to compare the automatic segmentation and manual contour. Ciardo et al. [23] also investigated 47 patients treated with breast cancer with Atlas based segmentation in the supine position during breast conservation surgery or non-conservation surgery, editing atlas-based volumes save time (12%) compared with manual contouring (44%). Henry et al. [24] assessed atlas-based segment (atlas-based segment, ABS) in 9 various OARs, and lymph node volumes in 30 patients with breast cancer through the software result in a 40% decrease in structures contour time. Eldesoky et al. [8] reported efficacy and precision by developing clinically acceptable contours faster compared to manual contours and also declared the average time for manual contour was reduced to 94% for all structure after lumpectomy. Macchia et al. [25] compared 3 commercial software (ABAS, MIM Maestro, VelocityAI) for atlas-based segmentation applying them to a number of the prostate, Head and Neck, and pleura. In this paper, the physician can save one hour for the H & N site, the time saved was about twenty minutes for a mesothelioma patient, and 40 minutes for a prostate patient.
The most distinction advantage of the automatic contour methods compared to the manual contour is saving the time. However, we need to think whether our research can meet the requirement of clinical demand by obtaining faster contouring time. Deep learning framework [26,27] can be utilized for medical image diagnosis, accurate diagnosis is the foundation of accurate contour. Based on prior knowledge, radiotherapy requires accurate radiation delivery and precise contouring of the OARs. CT images typically have high resolution and provide detailed anatomical information. However, limited physiological information can be captured by CT imaging, in order to evaluate target volumes effectively in automatic segment, medical imaging modalities such as the CT and positron emission tomography (PET) are widely used. Song et al. [28] proposed a novel approach for the segmentation of the PET-CT images, which makes use of the strength from both modalities: the functionality information of PET and the anatomical structure information of CT, both Lung and N & head tumor are well validated. Rundo [29] also proposed fully automatic multimodal PET/MRI segmentation method to help the clinicians to define a CTV that includes both metabolic and morphologic information. The precise of the dose delivery with relate to the precise contours of Targets and OARs, and the toxicity correlated with the risk of the OARs dose received. Therefore, we discussed the DVH parameters of each contour which was delineated by automatic segment approach. The Gemetric measures did not predict the accuracy of dosimetric parameters determined by automatic software in our research. The results were similar to those of Robert et al. [30].
This work represents the investigation of the impact of an automated contouring on estimates of radiation dose to the whole body patients. It demonstrates that dosimetric information of automatic contours from CT images is difference with the DSC. We deduced that the result with a perfect Dice-similarity coefficient one would expect to have perfect accuracy with dosimetric endpoints [31]. However, on the contrary we investigated that geometric parameters evaluation such as DSC which was heavily influenced by the volume of a structure, but may not consistently reflect whether an automatic contour accurately represents actual radiation dose.
For the NPC, all the OARs have good DSC (>0.7) besides Lens, Optic Nerve and Spinal Cord which due to the small size. Small size of organs is more likely to contoured error such as Lens. In addition, for small size organs such as OpticNerves with unclear tissue boundaries, there were still a certain deviation between automatic and manual. On the other hand, we can learn from Tables 5 and 8, the volume and the exposed dose of Spinal Cord had big distinction in Head and Neck, we can obtain the differences of Spinal Cord from Figure 3, which because of the precise contour of the boundary between the Spinal Cord and BrainStem. In manual contouring, the Spinal Cord contoured to C2 while contoured to upper C1 by Automatic segment approach. So the dose of Spinal Cord contoured by automatic higher than the manual. In Figure 4, we found that the smaller the size of the Organ the less difference of the dose. But there are great difference of the contouring of small organs, many scholars tried to develop standard model to improve contouring accuracy, Yang et al. [32] proposed an automated structure nomenclature standardization framework simulates clinicians' domain knowledge and recognition mechanisms to identify small-volume organs at risk (OARs) with heavily imbalanced data better than other methods.
For breast cancer, the Lung and the Heart which had big size no matter of the volume (p > 0.05) and DVH distribution (p > 0.05) had no difference between two methods. At the same time, we can see that the Spinal Cord of thorax is more accurate than the Neck. Multiple studies with automatic contouring come from the breast cancer, which mainly because of the boundary of OARs was clear and density contrast was obvious. In the studies of Robert et al. [33], Xu et al. [34] and Henry [24] et al., which are all based on breast cancer patients, their research all have achieved well clinical validation, and the same trend of clinical validation was also found. Therefore good correlation was obtained in terms of DSC, volume or dose parameters.
For rectal cancer, it can meet the clinical requirement and also have the similar dose of the Bladder because of the obvious boundary. Other studies have investigated the automatic contouring of rectal cancer using different methods, Song et al. [3] using deep learning for rectal cancer postoperative radiotherapy, it concluded that convolutional neural networks at various feature resolution levels well delineated rectal cancer CTVs and OARs, displaying high quality and requiring shorter computation and manual correction time. Because the femoral head has obvious bone markers, it should be well delineated automatically. However in Table 10, we found the DSC and JD was lower than the clinical predict, the contour and the dose all had the differences. From Figure 3, we can clearly see that manual contour the partial Femoral heads. After communicating with radiation oncologist, the contouring method of different department present small difference according to the guideline.
In conclusion, we investigated the contour of NPC, breast and rectal cancer by automatic segment approach. The value of DSC does not fully reflect the accuracy of dose distribution. For the obvious boundary of the Heart, Lung, Bladder, they can be completely dependent on automatic contouring. However, the strict validation is also required for small size OARs. In addition, for the Femoral heads, Spinal Cord and BrainStem contour guideline can be obtained using the semi-automatic method and validation when required. In the future, we will take more effort at improving the contouring accuracy of small volume organs and establishing the standardization of contouring. Furthermore, we also will extend our work to the segmentation of the tumor from multi-modalities.
The authors would like to express their sincere gratitude to the technicians from MANTEIA Inc. and radiotherapists at the Radiotherapy Department of Jinling Hospital for their assistance in preparing the image scans.
The authors declare no conflicts of interest.
[1] | S. Li, J. Xiao, L. He, X. Yuan, The Tumor Target Segmentation of Nasopharyngeal Cancer in CT Images Based on Deep Learning Methods, Technol. Cancer Res. Treat., 18 (2019), 1533033819884561. |
[2] | S. Gresswell, P. Renz, D. Werts, Y. Arshoun, Impact of Increasing Atlas Size on Accuracy of an Atlas-Based Auto-Segmentation Program (ABAS) for Organs-at-Risk (OARS) in Head and Neck (H & N) Cancer Patients, Int. J. Radiat. Oncol. Biol. Phys., 98 (2017), E31. |
[3] |
Y. Song, J. Hu, Q. Wu, F. Xu, S. Nie, Y. Zhao, et al., Automatic delineation of the clinical target volume and organs at risk by deep learning for rectal cancer postoperative radiotherapy, Radiother. Oncol., 145 (2020), 186-192. doi: 10.1016/j.radonc.2020.01.020
![]() |
[4] |
S. H. Ahn, A. U. Yeo, K. H. Kim, C. Kim, Y. Goh, S. Cho, et al., Comparative clinical evaluation of atlas and deep-learning-based auto-segmentation of organ structures in liver cancer, Radiat. Oncol., 14 (2019), 1-13. doi: 10.1186/s13014-018-1191-y
![]() |
[5] | S. S. Mahdavi, S. E. Salcudean, W. J. Morris, I. Spandinger, A semi-automatic segmentation method for prostate boundary delineation, Brachytherapy, 8 (2009), P175. |
[6] |
L. Rundo, C. Militello, A. Tangherloni, G. Russo, S. Vitabile, M. C. Gilardi, et al., NeXt for neuro-radiosurgery: A fully automatic approach for necrosis extraction in brain tumor MRI using an unsupervised machine learning technique, Int. J. Imaging Syst. Technol., 28 (2018), 21-37. doi: 10.1002/ima.22253
![]() |
[7] |
X. Wang, H. Cui, G. Gong, Z. Fu, J. Zhou, J. Gu, et al., Computational delineation and quantitative heterogeneity analysis of lung tumor on 18F-FDG PET for radiation dose-escalation, Sci. Rep., 8 (2018), 10649. doi: 10.1038/s41598-018-28818-8
![]() |
[8] |
A. R. Eldesoky, E. S. Yates, T. B. Nyeng, M. S. Thomsen, H. M. Nielsen, et al., Internal and external validation of an ESTRO delineation guideline - dependent automated segmentation tool for loco-regional radiation therapy of early breast cancer, Radiother. Oncol., 121 (2016), 424-430. doi: 10.1016/j.radonc.2016.09.005
![]() |
[9] |
Z. Liu, X. Liu, H. Guan, H. Zhen, Y. Sun, Q. Chen, et al., Development and Validation of A Deep Learning Algorithm for Auto-Delineation of Clinical Target Volume and Organs at Risk in Cervical Cancer Radiotherapy, Radiother. Oncol., 153 (2020), 172-179. doi: 10.1016/j.radonc.2020.09.060
![]() |
[10] |
L. Li, D. Qi, Y. M. Jin, G. Q. Zhou, Y. Q. Tang, W. L. Chen, et al., Deep Learning for Automated Contouring of Primary Tumor Volumes by MRI for Nasopharyngeal Carcinoma, Radiol., 291 (2019), 677-686. doi: 10.1148/radiol.2019182012
![]() |
[11] | O. Ronneberger, P. Fischer, T. Brox, U-Net: Convolutional Networks for Biomedical Image Segmentation, Int. Conf. Med. Image Comput. Comput.-Assist. Interv., (2015), 234-241. |
[12] |
A. V. Young, A. Wortham, I. Wernick, A. Evans, R. D. Ennis, Atlas-Based Segmentation Improves Consistency and Decreases Time Required for Contouring Postoperative Endometrial Cancer Nodal Volumes, Int. J. Radiat. Oncol. Biol. Phys., 79 (2011), 943-947. doi: 10.1016/j.ijrobp.2010.04.063
![]() |
[13] | K. Brock, S. Mutic, T. Mcnutt, H. Li, M. L. Kessler, Use of image registration and fusion algorithms and techniques in radiotherapy: Report of the AAPM Radiation Therapy Committee Task Group No. 132, Med. Phys., 44 (2017), e43-e76. |
[14] |
S. H. Ahn, A. U. Yeo, K. H. Kim, C. Kim, Y. Goh, S. Cho, et al., Comparative clinical evaluation of atlas anddeep-learning-based auto-segmentation oforgan structures in liver cancer, Radiat. Oncol., 14 (2019), 1-13. doi: 10.1186/s13014-018-1191-y
![]() |
[15] | N. T. C. Fung, W. M. Hung, C. K. Sze, M. C. H. Lee, W. T. Ng, Automatic segmentation for adaptive planning in nasopharyngeal carcinoma IMRT: Time, geometrical, and dosimetric analysis, Med. Dosim., 45 (2020), 60-65. |
[16] | N. Lee, Q. Zhang, J. Kim, A. S. Garden, J. Mechalakos, K. Hu, et al., Phase II Study of Concurrent and Adjuvant Chemotherapy with Intensity Modulated Radiation Therapy (IMRT) or Three-dimensional Conformal Radiotherapy (3D-CRT) + Bevacizumab (BV) for Locally or Regionally Advanced Nasopharyngeal Cancer (NPC)[RTOG 0615]: Preliminary tocicity report, Int. J. Radiat. Oncol., Biol., Phys., 78 (2010), S103-S104. |
[17] |
J. Shi, Y. Ye, D. Zhu, L. Su, Y. Huang, J. Huang, Automatic Segmentation of Cardiac Magnetic Resonance Images based on Multi-input Fusion Network, Comput. Methods Programs Biomed., 209 (2021), 106323. doi: 10.1016/j.cmpb.2021.106323
![]() |
[18] |
Y. Ye, J. Shi, D. Zhu, L. Su, Y. Huang, J. Huang, Comparative analysis of pulmonary nodules segmentation using multiscale residual U-Net and fuzzy C-means clustering, Comput. Methods Programs Biomed., 209 (2021), 106332. doi: 10.1016/j.cmpb.2021.106332
![]() |
[19] | G. E. Hinton, S. Osindero, Y. W. Teh, A Fast Learning Algorithm for Deep Belief Nets, Neural Comput., 18 (2014), 1527-1554. |
[20] |
S. Liang, F. Tang, X. Huang, K. Yang, T. Zhong, R. Hu, et al., Deep-learning-based detection and segmentation of organs at risk in nasopharyngeal carcinoma computed tomographic images for radiotherapy planning, Eur. Radiol., 29 (2019), 1961-1967. doi: 10.1007/s00330-018-5748-9
![]() |
[21] |
D. Shen, G. Wu, H. I. Suk, Deep Learning in Medical Image Analysis, Annu. Rev. Biomed. Eng., 19 (2017), 221-248. doi: 10.1146/annurev-bioeng-071516-044442
![]() |
[22] | P. F. Christ, F. Ettlinger, F. Grun, M. E. A. Elshaera, J. Lipkova, S. Schlecht, et al., Automatic Liver and Lesion Segmentation in CT Using Cascaded Fully Convolutional Neural Networks and 3D Conditional Random Fields, Int. Confer. Med. Image Comput. Comput. Assist. Interv., (2016), 415-423. |
[23] |
D. Ciardo, M. A. Gerardi, S. Vigorito, A. Morra, V. Dell'Acqua, F. J. Diaz, et al., Atlas-based segmentation in breast cancer radiotherapy: Evaluation of specific and generic-purpose atlases, Breast, 32 (2017), 44-52. doi: 10.1016/j.breast.2016.12.010
![]() |
[24] | A. Arsène-Henry, H. P. Xu, M. Robilliard, W. E. Amine, E. Costa, Y. M. Kirova, Evaluation of an automatic delineation software for organs at risk and lymph nodes in breast cancer, Radiother. Oncol., 22 (2018), 241-247. |
[25] |
M. La Macchia, F. Fellin, M. Amichetti, M. Cianchetti, S. Gianolini, V. Paola, et al., Systematic evaluation of three different commercial software solutions for automatic segmentation for adaptive therapy in head-and-neck, prostate and pleural cancer, Radiat. Oncol., 7 (2012), 160. doi: 10.1186/1748-717X-7-160
![]() |
[26] |
Z. Tang, G. Zhao, T. Ouyang, Two-phase deep learning model for short-term wind direction forecasting, Renew. Energ., 173 (2021), 1005-1016. doi: 10.1016/j.renene.2021.04.041
![]() |
[27] |
K. Wong, G. Fortino, D. Abbott, Deep learning-based cardiovascular image diagnosis: A promising challenge, Future Gener. Comput. Syst., 110 (2020), 802-811. doi: 10.1016/j.future.2019.09.047
![]() |
[28] |
L. Rundo, A. Stefano, C. Militello, G. Russo, M. G. Sabini, C. D''Arrigo, et al., A fully automatic approach for multimodal PET and MR image segmentation in Gamma Knife treatment planning, Comput. Methods Programs Biomed., 144 (2017), 77-96. doi: 10.1016/j.cmpb.2017.03.011
![]() |
[29] |
Q. Song, J. Bai, D. Han, S. Bhatia, W. Sun, W. Rockey, et al., Optimal co-segmentation of tumor in PET-CT images with context information, IEEE Trans. Med. Imaging, 32 (2013), 1685-1697. doi: 10.1109/TMI.2013.2263388
![]() |
[30] |
R. Kaderka, E. F. Gillespie, R. C. Mundt, A. K. Bryant, C. B. Sanudo, A. L. Harrison, et al., Geometric and dosimetric evaluation of atlas based auto-segmentation of cardiac structures in breast cancer patients, Radiother. Oncol., 131 (2019), 215-220. doi: 10.1016/j.radonc.2018.07.013
![]() |
[31] |
Y. Tong, Y. Yin, P. Cheng, G. Gong, Impact of deformable image registration on dose accumulation applied electrocardiograph-gated 4DCT in the heart and left ventricular myocardium during esophageal cancer radiotherapy, Radiat. Oncol., 13 (2018), 145. doi: 10.1186/s13014-018-1093-z
![]() |
[32] |
Q. Yang, H. Chao, D. Nguyen, S. Jiang, Mining Domain Knowledge: Improved Framework Towards Automatically Standardizing Anatomical Structure Nomenclature in Radiotherapy, IEEE Access, 8 (2020), 105286-105300. doi: 10.1109/ACCESS.2020.2999079
![]() |
[33] | R. A. Mitchell, P. Wai, R. Colgan, A. M. Kirby, E. M. Donovan, Improving the efficiency of breast radiotherapy treatment planning using a semi-automated approach, J. Appl. Clin. Med. Phys., 18 (2017), 18-24. |
[34] |
H. P. Xu, A. Arsène-Henry, M. Robillard, M. Amessis, Y. Kirova, The use of new delineation tool "MIRADA" at the level of regional lymph nodes, step-by-step development and first results for early-stage breast cancer patients, Br. J. Radiol., 91 (2018), 20180095. doi: 10.1259/bjr.20180095
![]() |
1. | Curtise K. C. Ng, Vincent W. S. Leung, Rico H. M. Hung, Clinical Evaluation of Deep Learning and Atlas-Based Auto-Contouring for Head and Neck Radiation Therapy, 2022, 12, 2076-3417, 11681, 10.3390/app122211681 | |
2. | K. Mackay, D. Bernstein, B. Glocker, K. Kamnitsas, A. Taylor, A Review of the Metrics Used to Assess Auto-Contouring Systems in Radiotherapy, 2023, 09366555, 10.1016/j.clon.2023.01.016 | |
3. | Seo Hee Choi, Jong Won Park, Yeona Cho, Gowoon Yang, Hong In Yoon, Automated Organ Segmentation for Radiation Therapy: A Comparative Analysis of AI-Based Tools Versus Manual Contouring in Korean Cancer Patients, 2024, 16, 2072-6694, 3670, 10.3390/cancers16213670 | |
4. | Guoping Shan, Shunfei Yu, Zhongjun Lai, Zhiqiang Xuan, Jie Zhang, Binbing Wang, Yun Ge, A Review of Artificial Intelligence Application for Radiotherapy, 2024, 22, 1559-3258, 10.1177/15593258241263687 | |
5. | Curtise K. C. Ng, Performance of Commercial Deep Learning-Based Auto-Segmentation Software for Breast Cancer Radiation Therapy Planning: A Systematic Review, 2024, 8, 2414-4088, 114, 10.3390/mti8120114 |
Antomical | Manual Contouring Time(SD) | Automatic segment time(SD) | P |
NPC | 56.5± 9 | 1.5 ±0.23 | p < 0.0001 |
breast cancer | 23.12 ± 4.23 | 1.45 ±0.78 | p < 0.0001 |
rectal cancer | 45.23 ± 2.39 | 1.8±0.56 | p < 0.0001 |
Organ at risks | Volume(cc) | D1(cGy) | P (VolumeManually VS VolumeAutomatic) | P (DoseManually VS DoseAutomatic) | |
Eye_R | Manually | 9.93±1.08 | 2551.23±701.2 | 0.02 | 0.002 |
Automatic | 10.45±1.06 | 2911.62±613.1 | |||
Eye_L | Manually | 9.73±0.99 | 2649.62±668.1 | 0.01 | 0.019 |
Automatic | 11.12±1.17 | 2921.08±616.9 | |||
Lens_R | Manually | 0.31±0.08 | 676.92±95.62 | 0.26 | 0.40 |
Automatic | 0.28±0.08 | 663.77±120.8 | |||
Lens_L | Manually | 0.31±0.1 | 674.77±89.56 | 0.81 | 0.66 |
Automatic | 0.31±0.07 | 680.92±118.41 | |||
Spinal Cord | Manually | 25.16±9.17 | 4127.3±330.81 | 0.000 | 0.000 |
Automatic | 10.66±3.46 | 5071.5±394.35 |
Organ at risks | Volume(cc) | Mean Dose | P (VolumeManually VS VolumeAutomatic) | P (DoseManually VS DoseAutomatic) | |
Parotid_L | Manually | 26.9±8.86 | 3426.23±174.19 | 0.017 | 0.001 |
Automatic | 30.2±7.27 | 3646.53±243.32 | |||
Parotid_R | Manually | 26.62±9.86 | 3485.31±280.21 | 0.01 | 0.01 |
Automatic | 29.2±7.85 | 3707.54±478.13 |
Organ at risks | Volume(cc) | D1 | D5 | D50 | P(VolumeManually VS VolumeAutomatic) | P(DoseManually VS DoseAutomatic) | |
OpticNerve_L | Manually | 0.66±0.37 | 5144.08±1186.04 | 4893.54±1132.75 | 3247.54±1150.39 | 0.35 | PD1=0.21; PD5=0.17; PD50=0.97 |
Automatic | 0.53±0.12 | 4943±1052.02 | 4665.08±1018.21 | 3252.46±978.06 | |||
OpticNerve_R | Manually | 0.68±0.4 | 4721.62±1740 | 4540.23±1703.0 | 3196.53±1490.77 | 0.086 | PD1=0.71; PD5=0.64; PD50=0.41 |
Automatic | 0.58±0.16 | 4613.08±1191 | 4405.92±1225.79 | 3099.7±1300.2 | |||
BrainStem | Manually | 29.43±3.32 | 5602.46±180.22 | 5039.31±264.88 | 3645.77±648.88 | 0.000 | PD1=0.13; PD5=0.04; PD50=0.04 |
Automatic | 23.21±2.37 | 5679.61±241.2 | 5105.92±287.77 | 3450.31±693.3 |
Organ at risks | DSC | HD(mm) | JD | |
Eye_R | Manually | 0.904±0.02 | 3.55±0.32 | 0.87±0.05 |
Automatic | ||||
Eye_L | Manually | 0.907±0.02 | 3.6±0.4 | 0.89±0.03 |
Automatic | ||||
Lens_R | Manually | 0.789±0.1 | 2.45±0.31 | 0.72±0.1 |
Automatic | ||||
Lens_L | Manually | 0.789±0.09 | 2.5±0.4 | 0.74±0.07 |
Automatic | ||||
Spinal Cord | Manually | 0.459±0.11 | 23.0±1.5 | 0.38±0.2 |
Automatic | ||||
Parotid_L | Manually | 0.819±0.05 | 17.5±2.1 | 0.75±0.02 |
Automatic | ||||
Parotid_R | Manually | 0.768±0.1 | 17.8±2.3 | 0.75±0.1 |
Automatic | ||||
OpticNerve_L | Manually | 0.652±0.1 | 11.5±1.1 | 0.63±0.2 |
Automatic | ||||
OpticNerve_R | Manually | 0.66±0.12 | 12.3±1.23 | 0.57±0.15 |
Automatic | ||||
BrainStem | Manually | 0.83±0.03 | 21.0±1.5 | 0.77±0.04 |
Automatic |
Organ at risks | Volume(cc) | ipsilateral Lung V5 | ipsilateral Lung V10 | ipsilateral Lung V20 | ipsilateral Lung V30 | ipsilateral Lung V40 | P(VolumeManually VS VolumeAutomatic) | P(VxManually VS VxAutomatic) | |
Lung_L | Manually | 1030.20±238.49 | 68.2±9.87 | 44.8±5.67 | 26.8±4.16 | 18.4±3.97 | 11.7±4 | 0.98 | PV5=0.20; PV10=0.052; PV20=1 PV30=0.59 PV40=0.467 |
Automatic | 1031.3±3625.63 | 66.6±10.7 | 44.1±5.63 | 26.8±3.82 | 18.6±3.56 | 12±3.68 | |||
Lung_R | Manually | 1248.57±273.46 | 0.07 | ||||||
Automatic | 1301±248.54 |
Organ at risks | Volume(cc) | D1 | V30 | V40 | D33 | D67 | P(VolumeManually VS VolumeAutomatic) | P(DoseManually VS DoseAutomatic) | |
Heart | Manually | 677.43±99.59 | 4113.7±1705.38 | 4.5±4.35 | 2.5±2.79 | 897.4±164.9 | 446.8±124.83 | 0.087 | PD1=0.70; PV30=0.16; PV40=0.34; PD33=0.33; PD67=0.33; |
Automatic | 623.24±97.57 | 4183.3±1415.7 | 4.7±4.39 | 2.6±2.91 | 852.1±82.08 | 450.4±128.75 | |||
Spinal Cord | Manually | 48.43±14.85 | 2518.2±1651.02 | 0.15 | PD1=0.21; | ||||
Automatic | 41.37±6 | 2358.5±1629.04 |
Organ at risks | DSC | HD(mm) | JD | |
Lung_L | Manually | 0.944±0.03 | 20.7±5.3 | 0.93±0.02 |
Automatic | ||||
Lung_R | Manually | 0.944±0.03 | 20.31±3.85 | 0.83±0.17 |
Automatic | ||||
Heart | Manually | 0.858±0.15 | 16.6±5.4 | 0.83±0.16 |
Automatic | ||||
Spinal Cord | Manually | 0.709±0.1 | 3.15±0.8 | 0.7±0.1 |
Automatic |
Organ at risks | Volume(cc) | D1 | D5 | D15 | D25 | D35 | D45 | Mean | P(VManually VS VAutomatic) | P(DManually VS DAutomatic) | |
Bladder | Manually | 427.89±131.2 | 5235.8±164.5 | 4908±155.8 | 4760±156.44 | 4619.85±158.8 | 4379.1±222.37 | 4039.9±337.7 | 3766.25±252.9 | 0.06 | PD1=0.16; PD5=0.43; PD15=0.34; PD25=0.059; PD35=0.052; PD45=0.18; PMean=0.74; |
Automatic | 394.32±130.5 | 5026±174.15 | 4902.6±157.04 | 4751.9±158.67 | 4598.1±176.88 | 4357.8±224.14 | 4013.3±331.6 | 3762.6±255.3 | |||
Femur-L | Manually | 65.03±28 | 3833.7±203.4 | 4145.1±282.68 | 3678±271 | 3375.1±238.13 | 3112±253 | 2867.5±279.6 | 2819.1±243.2 | 0.000 | PD1=0.70; PD5=0.000; PD15=0.000; PD25=0.000; PD35=0.000; PD45=0.000; PMean=0.000; |
Automatic | 134.8±36 | 4470.9±257.78 | 3341.7±254.56 | 2761.5±335.85 | 2341±311.4 | 2095.1±271.3 | 1922±267 | 1820.9±268.3 | |||
Femur-R | Manually | 70.8±34.04 | 4479.6±312 | 4159.1±316.5 | 3640.6±258 | 3329.7±217.7 | 3061.1±254.2 | 2772.08±363.1 | 2792.2±260.45 | 0.000 | PD1=0.000; PD5=0.000; PD15=0.000; PD25=0.000; PD35=0.000; PD45=0.000; PMean=0.000; |
Automatic | 132.42±34.3 | 3802.3±300 | 3335.6±284.3 | 2804.2±357.5 | 2429.9±304.85 | 2122±222 | 1910.7±195.76 | 1812.5±18707 |
Organ at risks | DSC | HD(mm) | JD | |
Bladder | Manually | 0.91±0.04 | 2.87±1.1 | 0.9±0.2 |
Automatic | ||||
Femur-L | Manually | 0.43±0.1 | 35.2±7.2 | 0.4±0.08 |
Automatic | ||||
Femur-R | Manually | 0.44±0.11 | 35.3±6.9 | 0.4±0.1 |
Automatic |
Antomical | Manual Contouring Time(SD) | Automatic segment time(SD) | P |
NPC | 56.5± 9 | 1.5 ±0.23 | p < 0.0001 |
breast cancer | 23.12 ± 4.23 | 1.45 ±0.78 | p < 0.0001 |
rectal cancer | 45.23 ± 2.39 | 1.8±0.56 | p < 0.0001 |
Organ at risks | Volume(cc) | D1(cGy) | P (VolumeManually VS VolumeAutomatic) | P (DoseManually VS DoseAutomatic) | |
Eye_R | Manually | 9.93±1.08 | 2551.23±701.2 | 0.02 | 0.002 |
Automatic | 10.45±1.06 | 2911.62±613.1 | |||
Eye_L | Manually | 9.73±0.99 | 2649.62±668.1 | 0.01 | 0.019 |
Automatic | 11.12±1.17 | 2921.08±616.9 | |||
Lens_R | Manually | 0.31±0.08 | 676.92±95.62 | 0.26 | 0.40 |
Automatic | 0.28±0.08 | 663.77±120.8 | |||
Lens_L | Manually | 0.31±0.1 | 674.77±89.56 | 0.81 | 0.66 |
Automatic | 0.31±0.07 | 680.92±118.41 | |||
Spinal Cord | Manually | 25.16±9.17 | 4127.3±330.81 | 0.000 | 0.000 |
Automatic | 10.66±3.46 | 5071.5±394.35 |
Organ at risks | Volume(cc) | Mean Dose | P (VolumeManually VS VolumeAutomatic) | P (DoseManually VS DoseAutomatic) | |
Parotid_L | Manually | 26.9±8.86 | 3426.23±174.19 | 0.017 | 0.001 |
Automatic | 30.2±7.27 | 3646.53±243.32 | |||
Parotid_R | Manually | 26.62±9.86 | 3485.31±280.21 | 0.01 | 0.01 |
Automatic | 29.2±7.85 | 3707.54±478.13 |
Organ at risks | Volume(cc) | D1 | D5 | D50 | P(VolumeManually VS VolumeAutomatic) | P(DoseManually VS DoseAutomatic) | |
OpticNerve_L | Manually | 0.66±0.37 | 5144.08±1186.04 | 4893.54±1132.75 | 3247.54±1150.39 | 0.35 | PD1=0.21; PD5=0.17; PD50=0.97 |
Automatic | 0.53±0.12 | 4943±1052.02 | 4665.08±1018.21 | 3252.46±978.06 | |||
OpticNerve_R | Manually | 0.68±0.4 | 4721.62±1740 | 4540.23±1703.0 | 3196.53±1490.77 | 0.086 | PD1=0.71; PD5=0.64; PD50=0.41 |
Automatic | 0.58±0.16 | 4613.08±1191 | 4405.92±1225.79 | 3099.7±1300.2 | |||
BrainStem | Manually | 29.43±3.32 | 5602.46±180.22 | 5039.31±264.88 | 3645.77±648.88 | 0.000 | PD1=0.13; PD5=0.04; PD50=0.04 |
Automatic | 23.21±2.37 | 5679.61±241.2 | 5105.92±287.77 | 3450.31±693.3 |
Organ at risks | DSC | HD(mm) | JD | |
Eye_R | Manually | 0.904±0.02 | 3.55±0.32 | 0.87±0.05 |
Automatic | ||||
Eye_L | Manually | 0.907±0.02 | 3.6±0.4 | 0.89±0.03 |
Automatic | ||||
Lens_R | Manually | 0.789±0.1 | 2.45±0.31 | 0.72±0.1 |
Automatic | ||||
Lens_L | Manually | 0.789±0.09 | 2.5±0.4 | 0.74±0.07 |
Automatic | ||||
Spinal Cord | Manually | 0.459±0.11 | 23.0±1.5 | 0.38±0.2 |
Automatic | ||||
Parotid_L | Manually | 0.819±0.05 | 17.5±2.1 | 0.75±0.02 |
Automatic | ||||
Parotid_R | Manually | 0.768±0.1 | 17.8±2.3 | 0.75±0.1 |
Automatic | ||||
OpticNerve_L | Manually | 0.652±0.1 | 11.5±1.1 | 0.63±0.2 |
Automatic | ||||
OpticNerve_R | Manually | 0.66±0.12 | 12.3±1.23 | 0.57±0.15 |
Automatic | ||||
BrainStem | Manually | 0.83±0.03 | 21.0±1.5 | 0.77±0.04 |
Automatic |
Organ at risks | Volume(cc) | ipsilateral Lung V5 | ipsilateral Lung V10 | ipsilateral Lung V20 | ipsilateral Lung V30 | ipsilateral Lung V40 | P(VolumeManually VS VolumeAutomatic) | P(VxManually VS VxAutomatic) | |
Lung_L | Manually | 1030.20±238.49 | 68.2±9.87 | 44.8±5.67 | 26.8±4.16 | 18.4±3.97 | 11.7±4 | 0.98 | PV5=0.20; PV10=0.052; PV20=1 PV30=0.59 PV40=0.467 |
Automatic | 1031.3±3625.63 | 66.6±10.7 | 44.1±5.63 | 26.8±3.82 | 18.6±3.56 | 12±3.68 | |||
Lung_R | Manually | 1248.57±273.46 | 0.07 | ||||||
Automatic | 1301±248.54 |
Organ at risks | Volume(cc) | D1 | V30 | V40 | D33 | D67 | P(VolumeManually VS VolumeAutomatic) | P(DoseManually VS DoseAutomatic) | |
Heart | Manually | 677.43±99.59 | 4113.7±1705.38 | 4.5±4.35 | 2.5±2.79 | 897.4±164.9 | 446.8±124.83 | 0.087 | PD1=0.70; PV30=0.16; PV40=0.34; PD33=0.33; PD67=0.33; |
Automatic | 623.24±97.57 | 4183.3±1415.7 | 4.7±4.39 | 2.6±2.91 | 852.1±82.08 | 450.4±128.75 | |||
Spinal Cord | Manually | 48.43±14.85 | 2518.2±1651.02 | 0.15 | PD1=0.21; | ||||
Automatic | 41.37±6 | 2358.5±1629.04 |
Organ at risks | DSC | HD(mm) | JD | |
Lung_L | Manually | 0.944±0.03 | 20.7±5.3 | 0.93±0.02 |
Automatic | ||||
Lung_R | Manually | 0.944±0.03 | 20.31±3.85 | 0.83±0.17 |
Automatic | ||||
Heart | Manually | 0.858±0.15 | 16.6±5.4 | 0.83±0.16 |
Automatic | ||||
Spinal Cord | Manually | 0.709±0.1 | 3.15±0.8 | 0.7±0.1 |
Automatic |
Organ at risks | Volume(cc) | D1 | D5 | D15 | D25 | D35 | D45 | Mean | P(VManually VS VAutomatic) | P(DManually VS DAutomatic) | |
Bladder | Manually | 427.89±131.2 | 5235.8±164.5 | 4908±155.8 | 4760±156.44 | 4619.85±158.8 | 4379.1±222.37 | 4039.9±337.7 | 3766.25±252.9 | 0.06 | PD1=0.16; PD5=0.43; PD15=0.34; PD25=0.059; PD35=0.052; PD45=0.18; PMean=0.74; |
Automatic | 394.32±130.5 | 5026±174.15 | 4902.6±157.04 | 4751.9±158.67 | 4598.1±176.88 | 4357.8±224.14 | 4013.3±331.6 | 3762.6±255.3 | |||
Femur-L | Manually | 65.03±28 | 3833.7±203.4 | 4145.1±282.68 | 3678±271 | 3375.1±238.13 | 3112±253 | 2867.5±279.6 | 2819.1±243.2 | 0.000 | PD1=0.70; PD5=0.000; PD15=0.000; PD25=0.000; PD35=0.000; PD45=0.000; PMean=0.000; |
Automatic | 134.8±36 | 4470.9±257.78 | 3341.7±254.56 | 2761.5±335.85 | 2341±311.4 | 2095.1±271.3 | 1922±267 | 1820.9±268.3 | |||
Femur-R | Manually | 70.8±34.04 | 4479.6±312 | 4159.1±316.5 | 3640.6±258 | 3329.7±217.7 | 3061.1±254.2 | 2772.08±363.1 | 2792.2±260.45 | 0.000 | PD1=0.000; PD5=0.000; PD15=0.000; PD25=0.000; PD35=0.000; PD45=0.000; PMean=0.000; |
Automatic | 132.42±34.3 | 3802.3±300 | 3335.6±284.3 | 2804.2±357.5 | 2429.9±304.85 | 2122±222 | 1910.7±195.76 | 1812.5±18707 |
Organ at risks | DSC | HD(mm) | JD | |
Bladder | Manually | 0.91±0.04 | 2.87±1.1 | 0.9±0.2 |
Automatic | ||||
Femur-L | Manually | 0.43±0.1 | 35.2±7.2 | 0.4±0.08 |
Automatic | ||||
Femur-R | Manually | 0.44±0.11 | 35.3±6.9 | 0.4±0.1 |
Automatic |